- 1Radiotherapy Center, Affiliated Cancer Hospital & Institute of Guangzhou Medical University, Guangzhou, China
- 2Department of Oncology, The First People's Hospital of Changde City, Changde, China
Radiotherapy plays an irreplaceable and unique role in treating thoracic tumors, but the occurrence of radiation-induced lung injury has limited the increase in tumor target doses and has influenced patients’ quality of life. However, the introduction of functional lung imaging has been incorporating functional lungs into radiotherapy planning. The design of the functional lung protection plan, while meeting the target dose requirements and dose limitations of the organs at risk (OARs), minimizes the radiation dose to the functional lung, thus reducing the occurrence of radiation-induced lung injury. In this manuscript, we mainly reviewed the lung ventilation or/and perfusion functional imaging modalities, application, and progress, as well as the results based on the functional lung protection planning in thoracic tumors. In addition, we also discussed the problems that should be explored and further studied in the practical application based on functional lung radiotherapy planning.
Introduction
Radiotherapy occupies an essential role in tumor treatment. Approximately 50% of cancer patients require radiotherapy at a certain stage of the entire disease course, and it accounts for 40% of the tumor cure rate (1). In the past, radiotherapy was based on the assumption of the consistency of spatial tissue function without considering the heterogeneity of tissue function (2). However, due to the organ’s structure or the disease, functional heterogeneity is likely to exist (such as in the lung, liver, and parotid gland) (3, 4). Previous studies have demonstrated that lung function distribution in diverse lung tissue regions of patients with lung cancer is uneven (3, 5). Different locations and functional states respond differently to radiation (i.e., the better the functional state, the more sensitive) (3, 6). The degree of regional lung function decline after radiotherapy has a positive linear relationship with the radiation dose in this area (7). In the radiotherapy of thoracic tumors (such as lung cancer, and esophageal cancer), the existence of radiation-induced lung injury (RILI) not only limits the increase in tumor target dose but also seriously affects patients’ quality of life after radiotherapy (8). Furthermore, the risk of RILI is further increased in patients with older age, smoking, chronic obstructive pulmonary disease, interstitial lung disease, and concurrent chemotherapy (9).
In recent decades, more clinical evidence has shown the crucial role of functional imaging in tumor target delineation (10). Furthermore, the introduction of multi-detector computed tomography (MDCT), four‐dimensional CT (4DCT), dual-energy CT (DECT), magnetic resonance imaging (MRI), single-photon emission CT (SPECT), and positron emission tomography (PET) functional imaging modalities is of significant benefit in the design and evaluation of radiotherapy planning. Studies have shown that integrating functional imaging into radiation treatment planning can reduce the incidence of RILI by limiting the dose of functional lung irradiation (3, 11). This manuscript reviewed the principle, progress, and clinical application of multiple functional imaging modalities used to detect lung ventilation or perfusion function. It also discusses the problems that should be considered in practical applications and should be further explored and resolved.
Lung ventilation and perfusion
Air and blood are the fundamental participants in the gas exchange process, and the two must be maintained in an appropriate ratio to ensure sufficient and adequate progress (2). Pulmonary ventilation function measurement is the amount of air inhaled or exhaled by the lungs per time. In clinical, pulmonary function tests (PFT) are commonly used to evaluate patients’ ventilatory function, which usually reflects that the overall function of the lung cannot distinguish the differences between local lung functions and the sensitivity to early functional changes of the disease is limited (12). Numerous invasive techniques or radioisotope imaging have been tried to directly or indirectly quantify regional ventilation. However, these methods are restricted in clinical use by invasiveness and poor temporal and spatial resolution (13). Lung ventilation functional imaging was primarily used to detect pulmonary embolism, asthma, and chronic obstructive pulmonary disease (14, 15). It was relatively late when combined with radiotherapy to protect the lung function.
Pulmonary perfusion function measurement refers to the blood flow of lung tissue per time. Ventilation and perfusion can influence each other through a series of mechanisms, such as lack of adequate ventilation to cause hypoxia contraction of blood vessels, which in turn causes a decrease in matched perfusion (2, 4). Other diseases (pulmonary embolism, tumor-causing bronchial or aortic obstruction) can cause mismatch defects (2, 16). Lung ventilation/perfusion functional imaging is a two- or three-dimensional map showing the changes in lung volume during the entire respiratory cycle/blood flow distribution (2). The corresponding color-coded maps can be generated to observe the differences in overall lung function distribution more intuitively. The excessive radiation exposure of lung tissue leads to RILI, which is physiologically characterized by a reduction of airflow (ventilation) and blood flow (perfusion) (17).
Functional lung imaging modalities
Computed tomography
Multidetector CT
The previous CT imaging speed was slow, and it was difficult to use in clinical practice. However, electron beam CT (EBCT) uses parallel X-ray targets to obtain higher scanning speeds, a valuable tool for ventilation imaging and perfusion imaging, but it is expensive (18, 19). Until the advent of MDCT, scanning speed and imaging resolution have been greatly improved, so a noninvasive lung ventilation function method that is determined based on the wash-in and/or wash-out rate of the nonradioactive gas xenon (Xe) and forms a color-coded image of regional ventilation has been developed (13, 19–21). It means that the CT intensity of the lung image can be used to calculate the local air volume distribution at the two lung volumes, and through image registration, the mapping between the two images is obtained to get the regional ventilation change of the object (22). There are some problems in practical applications: one is radiation dose; another is the need to track the gas washing in and/or washing out at a fixed chest level, which limits the coverage of the lungs on the z-axis; the third is the image registration error; and the fourth is the accuracy of ventilation measurement in restricted areas where Xe will dissolve in the blood (15, 19, 23, 24). Moreover, Mahnken et al. (24) showed in a rabbit study that although krypton has a worse enhancement effect than Xe ventilation and requires a higher concentration, it is feasible to evaluate lung ventilation in MDCT.
In addition, Eslick et al. (25) directly performed an expiratory/inspiratory breath-hold CT scan on the patient. They proved that the lung ventilation function image obtained based on the change of Hounsfield unit (HU) has good consistency with the ventilation image obtained by PET. Moreover, MDCT and iodine-containing contrast agents can be used to assess regional lung perfusion dynamically, but the limited scanning range is its main drawback (13, 26).
Dual-energy CT
According to the imaging principle of DECT, it can be divided into two categories: one is through an X-ray tube to achieve energy separation (rapid KV switch CT, dual-source CT, twin-beam CT), and the other is achieved by the detector (dual-layer detector CT) (26–29). DECT can obtain two datasets of different energies at the same time through one acquisition, and a series of postprocessing techniques reconstruct them into virtual monoenergetic imaging (removing metal artifacts and improving image quality) and basic material imaging (substance separation and quantification) (30–32). In the past, DECT was mainly used for diagnosis and differential diagnosis, characterized tumor differentiation and gene expression, staging, and evaluating prognosis (33).
Through the introduction of DECT, it is possible to inhale a mixture of Xe and oxygen in one breath and hold the breath for imaging, and then use the basic material decomposition algorithm to separate the Xe signal from other signals for lung ventilation imaging reconstruction (15, 34). Fuld et al. (23) confirmed that proper calibration and the use of 40%Xe/40%He/20%O2 mixed gas could reduce the influence of gravity on the distribution of Xe and help with accurate quantitative measurement. However, Xe ventilation imaging was limited to high radiation doses and adverse effects of Xe, and it requires special equipment to transfer (14, 35). Chung et al. (36) then studied the effects of a series of krypton concentrations to enhance ventilation and confirmed that it is feasible to replace Xe to perform DECT lung ventilation imaging in rabbits when the krypton concentration is greater than 70%, but there are also some drawbacks. Furthermore, Zhang et al. (35) evaluated the feasibility of using DECT to evaluate lung ventilation after aerosol inhalation of iodine-containing contrast agents in rabbits. The results showed that the findings on the DECT ventilation image were highly consistent with the changes in lung parenchyma on the thin-section CT image and histopathological findings. Iodine-enhanced DECT ventilation imaging has the advantages of high spatial resolution and easier implementation, but the safety of inhalation of iodinated contrast agents in humans has not been verified.
The DECT lung perfusion image can be performed after injecting an iodine-containing contrast agent. The iodine map can be formed according to the basic material decomposition algorithm principle to evaluate the blood perfusion (35). Studies have proved that the pulmonary perfusion blood volume assessed by DECT is reliable and can replace the actual regional lung parenchymal perfusion measurement (15, 37). Through the optimization of the contrast media injection parameters (including the use of saline tracker bolus), high concentration (>300 mg I/ml), holding on breath, and performing DECT scans in the direction of the caudal cranium, the lung perfusion image quality can be improved (26, 38). Si-Mohamed et al. (39) quantitatively evaluated the relative lobe perfusion correlation between the DECT iodine map and SPECT/CT perfusion image. The Pearson correlation coefficient was 0.93 (r = 0.93), which indicated a high similarity in the shape and severity of perfusion defects. In another study, Bahig et al. (40) also confirmed that the Pearson correlation coefficient is 0.89 (r = 0.89), and the average of fV5 (volume of functional lung receiving ≥5 Gy) and mean functional lung dose (f-MLD) between the anatomical and functional lung volumes difference is statistically significant, which shows that it is necessary to optimize the radiotherapy planning to protect the functional lung. Although a clear iodine threshold has not been established to delimit functional and nonfunctional lungs, this paves the way for the next step in designing DECT-based functional radiotherapy planning.
As mentioned above, the actual physiology of lung function is gas exchange (involving proper ventilation and perfusion ratio). However, in our opinion, there are probably no functional imaging methods that can be used to detect ventilation and perfusion functions simultaneously because they require inhalation of a gas tracer or intravenous contrast agent, and it will increase the radiation dose exposure and scanning time. However, the emergence of DECT realized this process, Hong et al. (41) proved that, based on the DECT basic material decomposition algorithm, the krypton and iodine concentrations can be obtained at the same time through one scan to achieve the assessment of lung ventilation and perfusion function.
Four‐dimensional CT
The 4DCT scan consists of a series of CT image sets taken at particular points throughout the entire respiratory cycle (usually 10 respiratory phases), and the data used for lung ventilation function imaging reconstruction are usually obtained through the end-inspiratory and end-expiratory phases (2, 42). The real-time position management system (RPM) is commonly used to monitor the patient’s respiratory movements (based on an infrared camera). 4DCT lung function ventilation images are obtained by CT images of different respiratory stages for deformation image registration (DIR), and then the ventilation imaging algorithm (VIA) is used to quantitatively calculate the changes (3, 43, 44). In order to guarantee the accuracy of 4DCT lung ventilation measurement, studies have compared with clinical PFT, MRI, SPECT, and PET lung ventilation imaging, all suggesting that 4DCT ventilation can provide reliable lung function assessment (45–49). In addition, 4DCT can also remove motion artifacts and reflect the tumor’s internal target volume (ITV) for individualized radiotherapy planning (50).
4DCT lung ventilation imaging involves two main steps, one of which is the quantitative calculation of CT value (HU), Jacobian, or volume of the voxel (ΔV) changes in different respiratory phases (3, 43, 51). Latifi et al. (43) compared the above three VIAs, and the results showed that the similarity between ΔV and Jacobian is higher than that between HU and Jacobian, and ΔV and HU (Figure 1). Kida et al. (52) compared the calculation of lung ventilation based on HU and Jacobian with SPECT ventilation, respectively, and confirmed that there are smaller differences and a stronger correlation between HU and SPECT lung ventilation. Recently, Tian et al. (53) proposed a simplified algorithm for calculating lung ventilation (VIA AVG), with higher accuracy, efficiency, and fewer input requirements. Moreover, Liu et al. (54) developed deep learning (DL) method to calculate 4DCT lung ventilation. The results confirmed that compared with the method based on HU and Jacobian, the DL method has the highest similarity with SPECT lung ventilation.
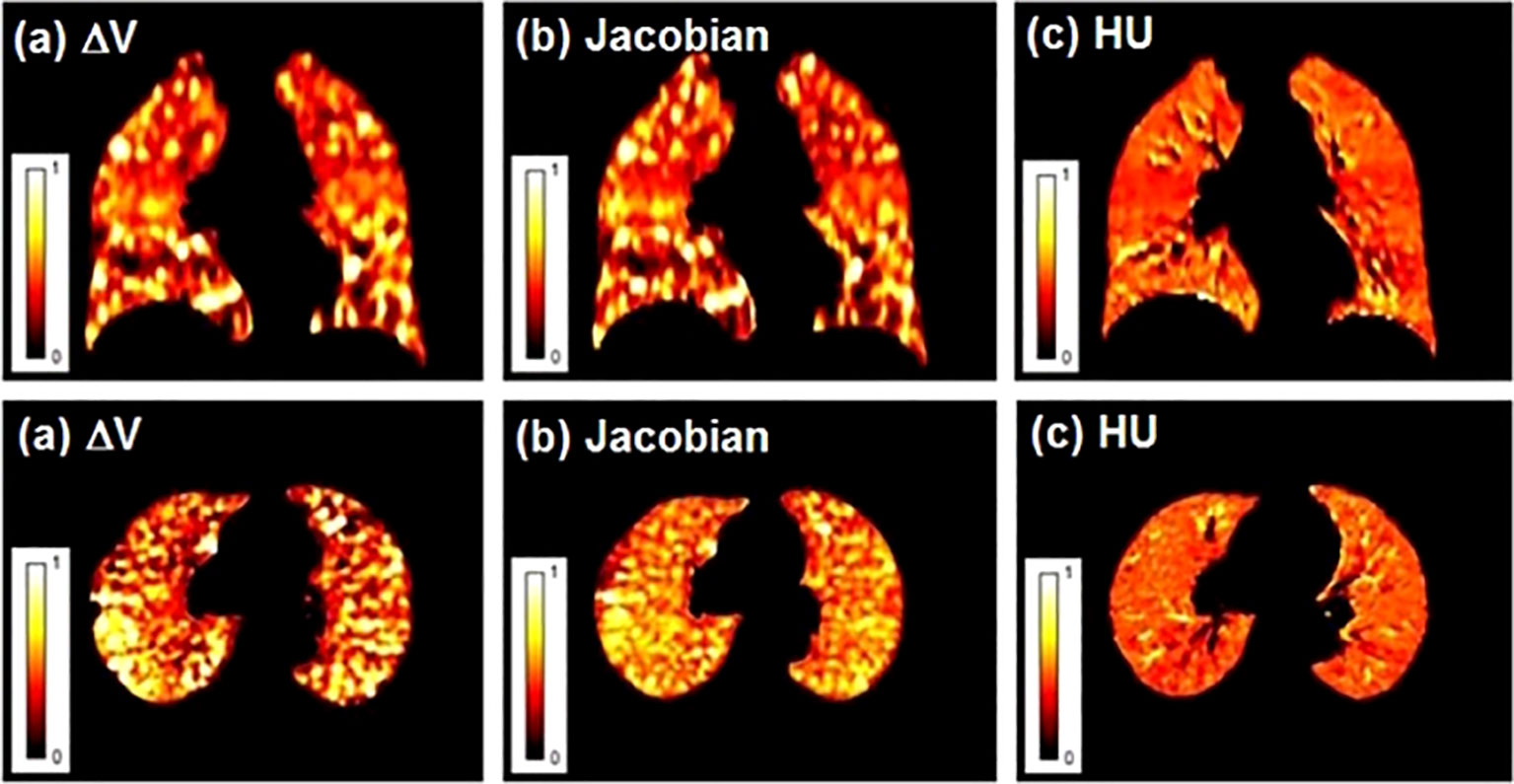
Figure 1 Lung ventilation function images obtained by different ventilation imaging algorithms (VIAs). (A) ΔV; (B) Jacobian; (C) HU. Reproduced from reference (43) with permission from Wiley, copyright 2013.
The second is the DIR algorithm, which mainly has two methods: surface-based registration and nonparametric registration based on volume (3). DIR algorithm is one of the cornerstones of 4DCT ventilation, and its accuracy will affect the final ventilation image. Latifi et al. (43) found that HU-based ventilation imaging is less dependent on different DIR algorithms than ΔV-based and Jacobian-based. Kipritidis et al. (55) proposed a simplified HU-based VIA that uses the 4D time-averaged product of regional air and tissue density for each voxel without relying on DIR. Results show that the new HU-based method is more related to the voxel of PET ventilation imaging than the HU-based DIR algorithm. Recently, Zhong et al. (56) developed a method based on deep convolutional neural networks, using inhalation peak and expiration peak phases as input data and HU-based DIR ventilation images as label data for training. The results demonstrate that the predicted ventilation images can be directly derived from 4DCT without explicit image registration and show a high degree of similarity with label data.
Magnetic resonance imaging
MRI has more advantages than CT, including excellent soft-tissue resolution, no ionizing radiation, the potential to obtain different nuclear information, and provides higher spatial resolution than nuclear medicine imaging (2, 13). Wielputz et al. (57) stated that the performance of MRI to evaluate perfusion, ventilation, and respiratory mechanics is better than MDCT. The main disadvantages of MRI are organ movement, lack of protons in the lung parenchyma, and field inhomogeneities, which significantly reduce the signal-to-noise ratio (11, 58). Several methods have been adopted to overcome these drawbacks in the past few years. A study has shown that Fourier decomposition/matrix pencil MRI (FD/MP-MRI) or hyperpolarized gas can be used to assess lung ventilation, and dynamic contrast-enhanced MRI (DCE-MRI) or no-contrast method (FD/MP-MRI) can be used to assess lung perfusion (59).
FD-MRI uses the short-echo method available on the scanner and the weak contrast generated when air enters and leaves the lungs during free breathing to generate lung ventilation maps. Acquire time-resolved two-dimensional datasets to generate perfusion maps without inhalation or injecting the tracer (12, 21). Kaireit et al. (60) confirmed that FD-MRI ventilation imaging and PFT have a good correlation and short-term repeatability (61). Furthermore, Bauman et al. (62) proved the qualitative consistency of FD-MR imaging and SPECT/CT in animal experiments assessing regional lung ventilation and blood perfusion. Other studies have shown that image quality can be improved by using nonuniform FD to replace standard fast FD (63) or ultra-fast steady-state free precession (64). MP-MRI is a derivative of FD-MRI that does not require tracers and respiratory restriction (59, 65). Compared with FD technology, MP decomposition can automatically, reliably, and accurately estimate the modulation amplitude of respiratory and cardiac signals (Figure 2) (66). From here, we can know that through FD-MRI or MP-MRI, ventilation and perfusion images can be obtained from one scan without a tracer.
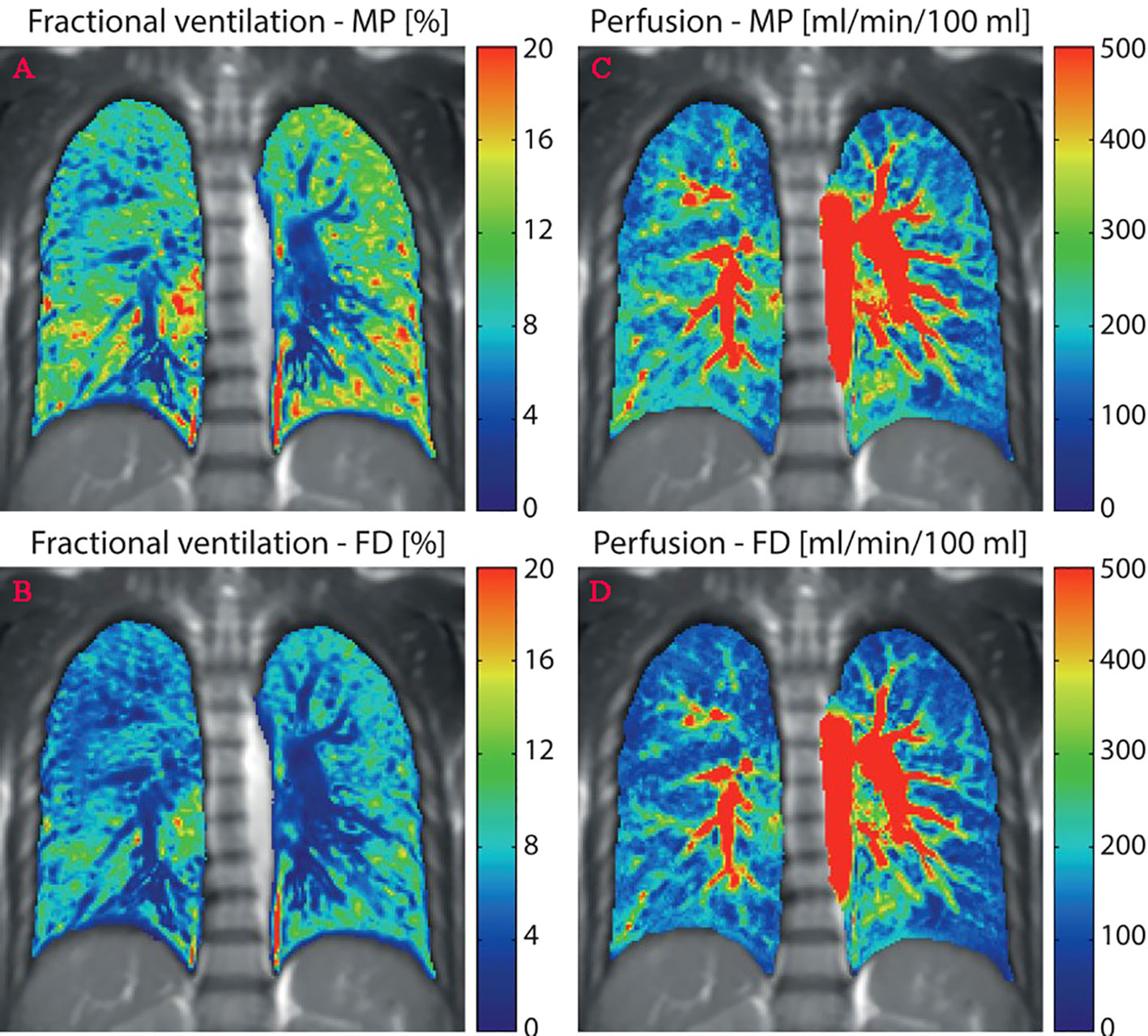
Figure 2 Quantitative maps of ventilation and perfusion calculated using the matrix pencil (MP) and Fourier decomposition (FD) methods. (A, C), MP-MRI ventilation/perfusion; (B, D), FD-MRI ventilation/perfusion. Reproduced from reference (64) with permission from Wiley, copyright 2016.
Some inert gases can be artificially hyperpolarized, and a magnetic resonance phenomenon occurs, so it can be used as an inhalable tracer to show lung ventilation (e.g., 3He, 129Xe, 19F) (12, 13, 21). The disadvantage of 3He and 129Xe is that the magnetization decays quickly and the storage time is short, while 19F has sufficient polarization ability for imaging at room temperature, but the image quality is obviously poor, and the inspection time is long at the same resolution (12, 21). Moreover, oxygen itself has paramagnetic properties and can also be directly used for lung ventilation imaging by inhaling 100% oxygen to obtain an image enhancement signal and subtracting the image signal of inhaling room air (20% oxygen) to detect ventilation (21, 58). Doganay et al. (67) stated that hyperpolarized 129Xe MRI ventilation is closely related to SPECT/CT ventilation imaging. However, Rankine et al. (68) stated that there are differences in f-MLD and volume of functional lung receiving ≥20 Gy (fV20) between the ventilation function represented by 129Xe MRI and the functional planning representing the actual regional lung function (gas exchange imaging), and 129Xe-MRI-based ventilation function is not an effective substitute for true regional lung function in all patients. All gas-based MRI ventilation imaging has its inherent shortcomings, and it is relatively rare to detect ventilation clinically.
DCE-MRI is an intravenous injection of a paramagnetic contrast agent (e.g., gadolinium-diethylene triamine penta-acetic acid (Ga-DTPA)), resulting in an increase in the signal in the T1-weighted image, which allows the visualization of local perfusion (12, 13). Johns et al. (69) showed that DCE-MRI perfusion has higher sensitivity in detecting chronic thromboembolism pulmonary hypertension compared with SPECT perfusion imaging. In fact, DCE-MRI and FD-MRI have higher consistency in the semiquantitative assessment of lung perfusion, but DCE-MRI has better image quality (70). However, Maxien et al. (71) developed a new DCE-MRI acquisition technology that shows that the free-breathing state does not increase the movement compared with the breath-hold state, and it is more suitable for quantitative assessment of lung perfusion.
Nuclear medicine imaging
Single-photon emission CT
In SPECT, one or more cameras rotate around the patient to generate planar images from multiple angles, which are then used to generate a set of tomography images of the distribution of radiotracers in the lungs (72). In the past 10 years, the emergence of SPECT/CT scanners has not only obtained SPECT ventilation and perfusion imaging data but also obtained CT imaging data of low-dose radiation dose levels for attenuation correction, and it has higher image quality and diagnostic accuracy compared with planar scintigraphy (72, 73). Since radionuclide imaging has been widely used to assess lung function for a long time and maintains relative evaluation standards, SPECT/CT can provide better spatial resolution and 3D anatomical information. So, it has been selected as a reference for assessing lung ventilation and perfusion standards (40).
The radioactive tracers used in SPECT/CT ventilation imaging include gas type (133Xe,81mKr), aerosol type (99mTc-DTPA), and solid type (99mTc-Technegas), and the radioactive perfusion tracer uses technetium-99m-labelled macroaggregated albumin (99mTc-MAA) (2, 72–74). However, due to the high cost of radioactive tracer gas, short half-life, and difficulty in storage and collection, the 99mTc-Technegas and 99mTc-MAA are now the most commonly used radiotracers for ventilation and perfusion imaging, respectively (73).
Positron emission tomography
Compared with SPECT, both are advanced technologies for image acquisition, but PET has higher sensitivity for radioactive decay detection, higher temporal and spatial resolution, excellent quantification capabilities, and shorter acquisition time (73, 75, 76). It can also be combined with CT scans to obtain higher image resolution and is currently commonly used in lung cancer radiation therapy planning (77). Even respiration gating can be used to reduce respiratory motion artifacts, and 4D ventilation and 4D perfusion of PET/CT can even be obtained by combining 4DCT (11, 73, 75). In the past, most PET radiotracers used to study lung physiology only had a short lifetime (such as 15O, 13N, and 11C), but now they are replaced by Galligas and Gallium-68 macroaggregated albumin (68G-MAA) for lung ventilation and perfusion, respectively (2, 75, 76, 78). The study by Le Roux et al. (79) found a strong correlation between PET/CT pulmonary function and PFT parameters, and they then proposed an automatic segmentation method that can be used for semiautomatic delineation (80, 81).
Clinical practice
Reducing functional lung radiation dose
Multiple functional lung imaging modalities have been applied to the clinic to optimize radiotherapy planning and reduce the radiation dose of functional lungs. Yamamoto et al. (82) compared the difference between the 4DCT ventilation function image-guided IMRT planning (f-IMRT) and the anatomical planning for patients with stage IIIB nonsmall cell lung cancer (NSCLC). The results showed that lung fV20 decreased by 5% while maintaining the target dose and meeting the dose limits of other critical organs. Wang et al. (83) showed that the five manually optimized beams for functional lung protection IMRT plans were more effective in reducing the dose to functional lungs compared to the five equally spaced beams for functional lung protection IMRT plans. As for integrating MRI-based functional lungs into clinical radiotherapy planning, a randomized, double-blind trial of functional lung avoidance based on 3He ventilation to evaluate pulmonary toxicity was prepared to achieve a reduction of 1.5 Gy and 3% in f-MLD and fV20, respectively (84). A meta-analysis based on utilized functional lung imaging modalities (including CT, 4DCT, SPECT, and PET) showed that f-MLD and fV20 decreased by 2.2 Gy and 4.2% when optimizing functional lung protection planning compared with conventional anatomical CT planning (more details in reference 17; Table 3) (17).
Bates et al. (85) stated that compared with the anatomical IMRT planning, the fV20 and f-MLD of the f-IMRT planning (based on SPECT/CT) were reduced by 2.5% and 0.4 Gy, respectively. Combining PET/CT ventilation/perfusion into radiotherapy planning, Siva et al. (86) studied the 30% maximum standard uptake value (SUV max) of 4D-PET/CT perfusion to determine the well-perfused lung volume. They optimized the 3D-CRT to protect the well-perfused lung volume. As a result, the functional V30, V40, V50, and V60 were improved, and the f-MLD of the lung improved by 0.86 Gy. In another study, the author found that the 70% SUV max threshold of the 4D-PET/CT scan was used to describe the “highly perfused” (HP-Lung) and “highly ventilated” (HV-Lung) lung volumes and used this to optimize IMRT planning. The results showed that HP-Lung-based f-MLD was significantly reduced by 13.0% (1.7 Gy), and fV5, fV10, and fV20 were improved by 13.2%, 7.3%, and 3.8%, respectively. At the same time, the LDVP in HV-Lung was not significantly different from the anatomical planning (87). A meta-analysis based only on PET/CT and SPECT/CT indicated that the integration of perfusion images into the radiotherapy planning of lung cancer patients could improve functional LDVP compared to conventional anatomical CT planning (f-MLD and fV20 decreased by 0.24 Gy and 0.41%, respectively), but ventilation also did not show significant improvement (88).
Not only that, but functional lung imaging has also been extended to proton therapy (PT). Leko et al. (89) compared the dosimetric differences between three-dimensional conformal radiotherapy (3D-CRT), VMAT, and PT functional lung protection plans guided by 4DCT ventilation images. The results showed that PT compared with VMAT and 3D-CRT, the fV5 was 10.7%, 21.9%, and 26.0%, and the f-MLD was 3.8, 5.2, and 5.6 Gy, respectively, which indicate that the PT-based functional lung sparing planning can further reduce the functional lung dose (example shown in Figure 3). The functional IMPT (intensity-modulated proton therapy) plans show significant dose reduction when compared to the functional VMAT plans for the f-MLD at 14.49 Gy vs. 7.31 Gy, fV20 at 22.66% vs. 13.48% (90) Huang et al. (44) further compared two functional proton plans based on 4DCT ventilation images (double scattering proton therapy (DSPT) and intensity-modulated proton therapy (IMPT)) with IMRT in preserving high-functioning lungs. The results show that DSPT and IMPT plans are better than IMRT in protecting the low-dose areas of the total lung (V5), and functional DSPT and functional IMPT have benefits in protecting high-functioning lungs, respectively, compared with their anatomical planning.
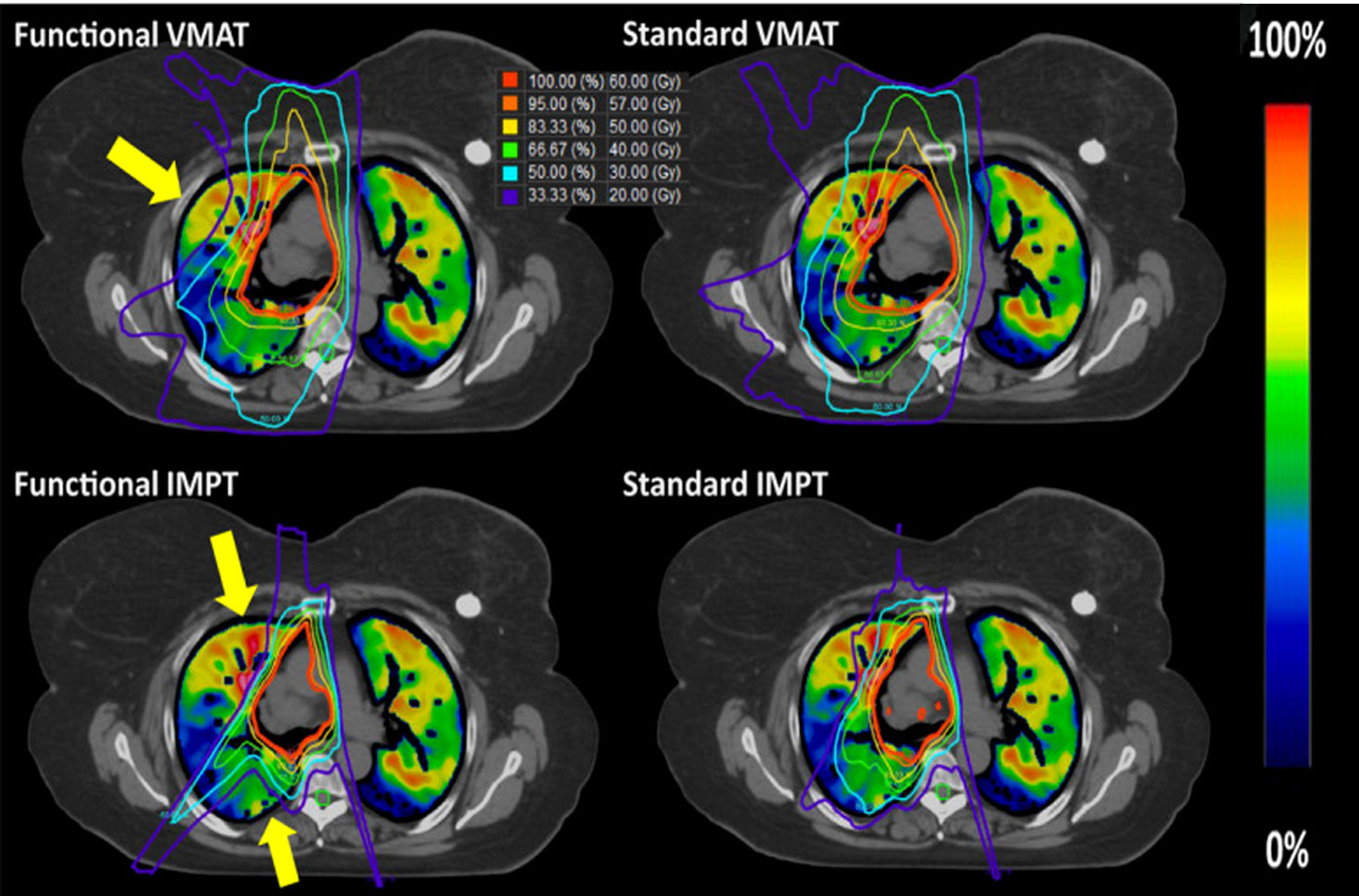
Figure 3 Examples of the standard and functional plans with VMAT (top) and three-field IMPT (bottom) techniques. The yellow arrow indicates where the most functional sparing occurs for this patient. Reproduced from reference (90) with permission from Wiley, copyright 2021.
Predicting radiation pneumonitis
In the past, lung dose-volume parameters (LDVP) such as MLD, V5, and V20 were used to predict the occurrence of radiation pneumonitis, but since the introduction of functional planning, the occurrence of radiation pneumonitis can be predicted more accurately through functional LDVP (f-MLD, fV20, etc.). Farr et al. (91) study showed that the area under the curve (AUC) for f-MLD and MLD in predicting G2+ radiation pneumonitis was 0.812 and 0.716, respectively (the same for fV20 and V20 was 0.792 and 0.716, respectively). Similar results were obtained in another study (92). Recent studies have shown that SPECT/CT-based ventilation and perfusion functional LDVP are used to predict RILI, indicating that if the patient has central-type NSCLC or chronic obstructive pulmonary disease, ventilation-based (V-fV20, V-f-MLD) is better than perfusion-based (Q-fV20, Q-f-MLD) to predict RILI (93).
Although it is generally said that functional LDVP can more accurately predict RILI, it is not clear what the threshold for defining functional lung can be a more accurate prediction. Ding et al. (94) seem to answer this question; that is, when 20% of the maximum value of SPECT/CT lung perfusion image count was used as the threshold, the highest predictive ability was obtained with fV20 of 0.928. However, it is true that there is no consensus on the optimal threshold for other functional imaging modalities.
Reducing radiation pneumonitis incidence
Regarding the risk of pulmonary radiation toxicity, Faught et al. (95) found that using 4DCT lung ventilation function imaging-guided IMRT planning can decrease 2+ and 3+ grade radiation pneumonitis by 7.1% and 4.7% (based on Normal tissue complication probability models), respectively.
According to the recent phase II clinical trial, Vinogradskiy et al. (96) showed that the 4DCT ventilation function image-based functional lung protection planning reduced the incidence of G2+ radiation pneumonitis to 14.9% in lung cancer patients receiving curative chemoradiotherapy (lower than the historically reported 25%), which did meet the expected phase II criteria (NCT02528942). Currently, clinical trials that have been registered at ClinicalTrials.gov for functional lung protection planning-guided radiotherapy are summarized in Table 1.
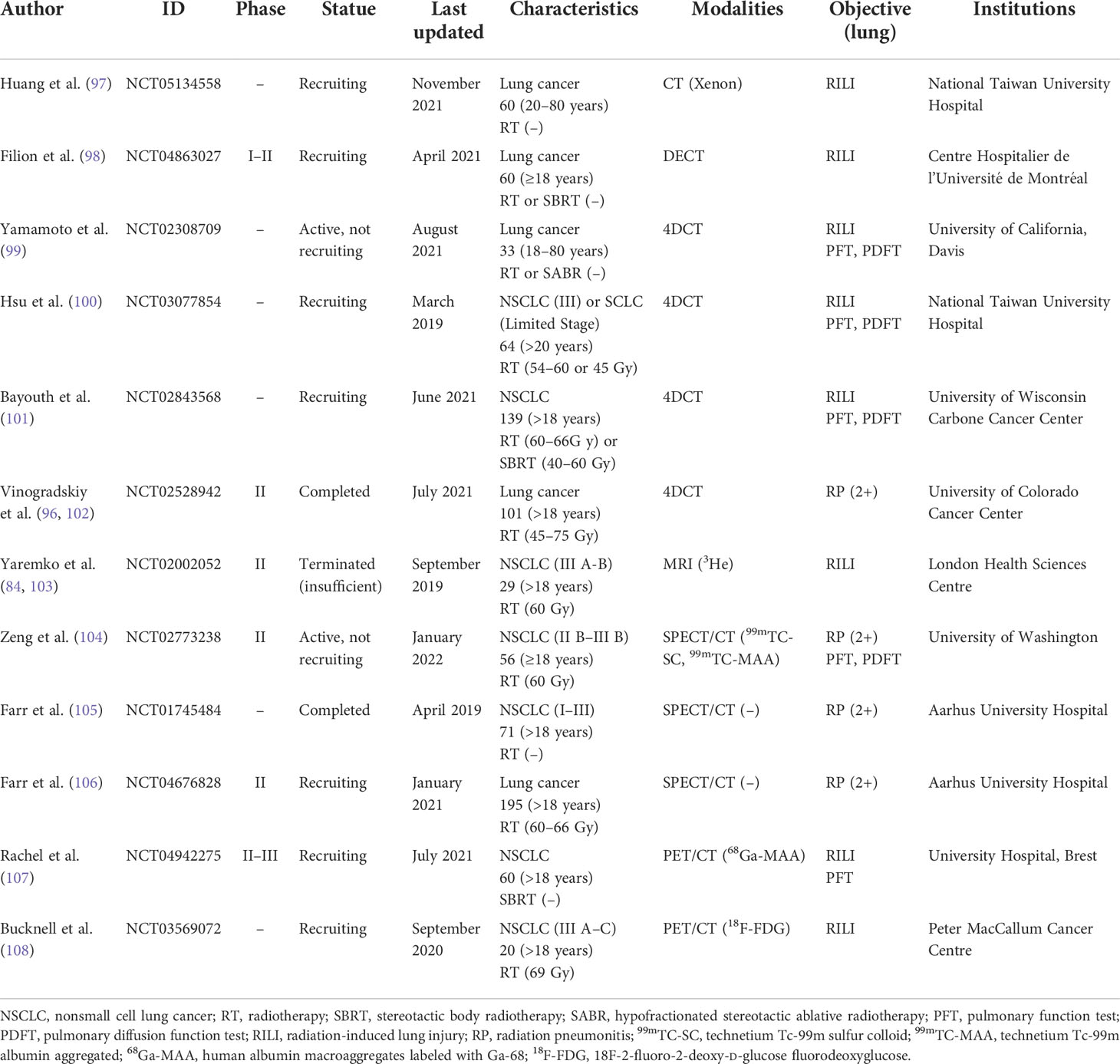
Table 1 Registered clinical trials of functional lung sparing planning-guided radiotherapy (from ClinicalTrials.gov).
Current problems and future research directions
1. Which functional lung imaging modalities and parameters should be selected? Although CT imaging is the gold standard for radiotherapy plan design and can save time and cost, MRI does not involve ionizing radiation and improves soft tissue contrast (109), and the reference standard for functional lung imaging is SPECT/CT and PET/CT (40).
2. Choose functional lung imaging-guided radiotherapy plan optimization based on ventilation or perfusion or ventilation-perfusion ratio. There have been many studies showing that no matter whether ventilation-based or perfusion-based imaging is included in the functional lung protection planning-guided radiotherapy, it is beneficial to reduce the functional lung dose, while Yuan et al. (16) study showed that only 61% of patients included in the study have ventilation and perfusion defects matching, the application of SPECT/CT ventilation-perfusion ratio to protect the functional lung will change the functional lung protection plan based on perfusion alone in 39% of patients. Forghani et al. (110) also reported that approximately 25% of patients with stage III lung cancer showed lower agreement in SPECT/CT ventilation and perfusion (example shown in Figure 4).
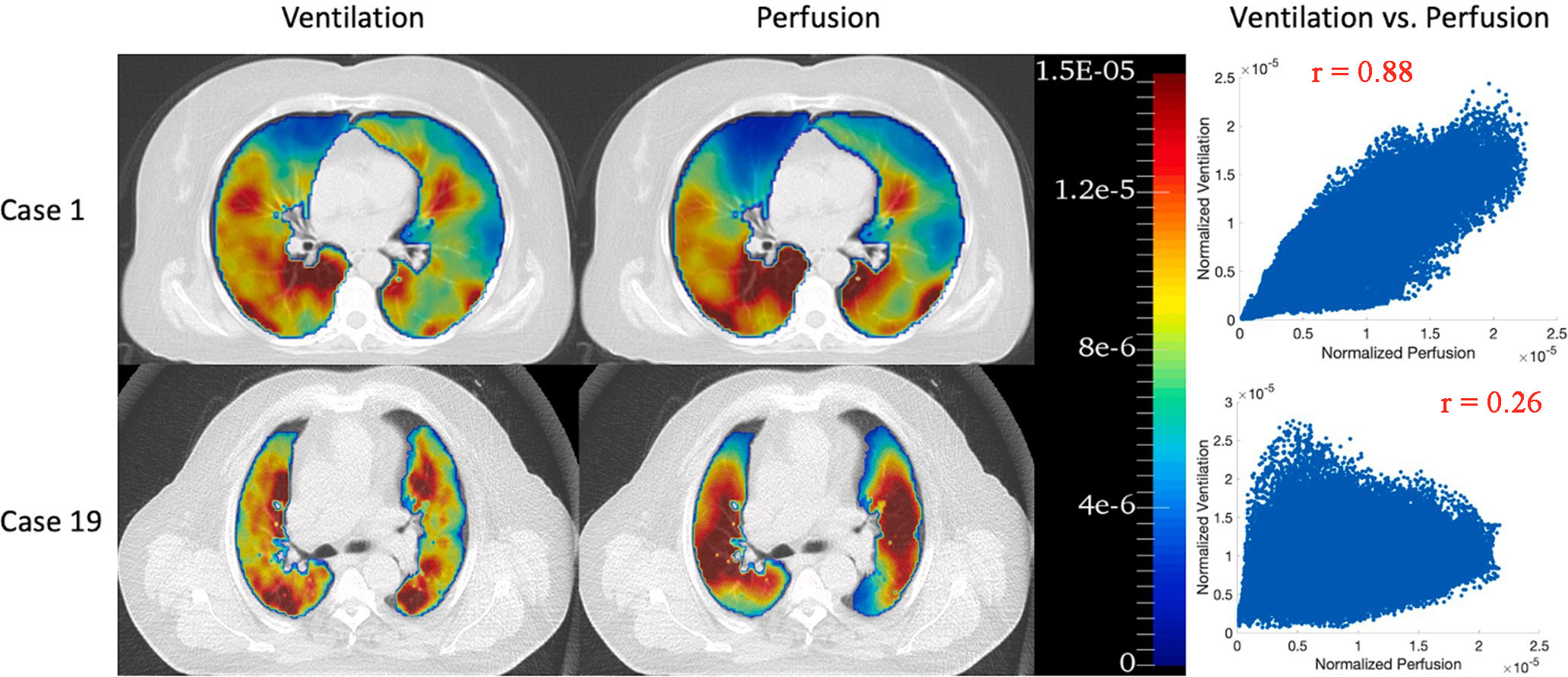
Figure 4 68Ga PET/CT ventilation and perfusion images and voxel-wise correlations between ventilation and perfusion for two representative patients: case 1 with the highest correlation (r = 0.88) and case 19 with the lowest correlation (r = 0.26). Reproduced from reference (111) with permission from Elsevier, copyright 2022.
3. Is it necessary to divide the functional lung into different areas according to different thresholds to optimize radiotherapy planning? Yuan et al. (16) and Meng et al. (112) divided the total lung function into five areas according to different underlying causes and thresholds (type A: tumor occupied lung regions; type B1: complete function defect areas induced by unrecoverable diseases; type B2: reduced lung function areas induced by unrecoverable diseases; type B3: temporarily dysfunctional lung due to tumor or other potentially reversible conditions; type C: normal functional lung). However, previous studies usually directly divide the whole lung into two areas: functional lung and non-functional lung (113). Moreover, Shioyama et al. (114) used 50% and 90% of the maximum count of SPECT/CT perfusion images as thresholds for f-IMRT planning (f-IMRT50%, f-IMRT90%), and compared their differences with anatomical planning. The results showed that f-MLD and fV20 were reduced by 2.2 and 4.2 Gy and 5.1% and 6.8%, respectively (f-IMRT50% vs. f-IMRT90%). So, whether the planning is designed to protect one threshold-defined functional lung or to divide the whole lung into multiple regions with different functional states for separate protection (multiple threshold-defined), the planning must be carefully considered.
4. When radiotherapy restores temporary lung function defects caused by tumors or other potentially reversible diseases, is it necessary to redesign the functional lung protection radiotherapy planning? What is then the best time to re-planning? Yuan et al. (115) observed that lung cancer patients’ local ventilation and perfusion were significantly improved when the radiotherapy reached 45 Gy compared to before the radiotherapy. In addition, Meng et al. (112) stated that potential changes in these functional lung regions could affect lung dose. Moreover, Yamamoto et al. (116) used two re-planning to protect the functional lung (two-time points are 16–20 Gy and 30–34 Gy), and the cumulative dose of the functional lung (f-MLD) was further reduced than that without re-planning (reduced by 5.0% and 3.6%, respectively). This shows that re-planning is necessary, but there is no uniform standard for the timing and frequency of re-planning.
Conclusion
The research of lung ventilation or perfusion imaging has been applied to clinical diagnosis for a long time. The combination of lung function imaging into radiotherapy planning has also attracted widespread attention, and studies continue to report new modalities and clinical application values of functional lung imaging (e.g., predict RILI, decrease the incidence of RILI). For DECT and 4DCT, it may be a better conventional method for pulmonary function imaging because the radiotherapy planning is based on CT as the gold standard. Imaging can be performed during disease diagnosis or simulated positioning without too much time and cost. DECT can obtain ventilation and perfusion images simultaneously by inhaling krypton and an intravenous injection of an iodine-containing contrast agent. However, the functional lung obtained by the above imaging modalities has not been physiologically verified, and the optimal imaging modalities and functional lung definition thresholds have not been clearly determined. We need to perform further verification tests and clinical trials (lack of phase III clinical trial results), but it is undeniable that the existing research evidence shows that the planning of integrated functional lung imaging to protect the functional lung is beneficial to reduce the occurrence of radiation-induced lung injury.
Author contributions
All authors listed have made a substantial, direct, and intellectual contribution to the work, and approved it for publication.
Conflict of interest
The authors declare that the research was conducted in the absence of any commercial or financial relationships that could be construed as a potential conflict of interest.
Publisher’s note
All claims expressed in this article are solely those of the authors and do not necessarily represent those of their affiliated organizations, or those of the publisher, the editors and the reviewers. Any product that may be evaluated in this article, or claim that may be made by its manufacturer, is not guaranteed or endorsed by the publisher.
References
1. Baskar R, Lee KA, Yeo R, Yeoh K. Cancer and radiation therapy: Current advances and future directions. Int J Med Sci (2012) 9:193–9. doi: 10.7150/ijms.3635
2. Zhang G, Dilling TJ, Stevens CW, Forster KM. Functional lung imaging in thoracic cancer radiotherapy. Cancer Control (2008) 15:112–9. doi: 10.1177/107327480801500203
3. Partridge M, Yamamoto T, Grau C, Høyer M, Muren LP. Imaging of normal lung, liver and parotid gland function for radiotherapy. Acta Oncol (2010) 49:997–1011. doi: 10.3109/0284186X.2010.504735
4. Petersson J, Glenny RW. Gas exchange and ventilation–perfusion relationships in the lung. Eur Respir J (2014) 44:1023–41. doi: 10.1183/09031936.00037014
5. Roach PJ, Gradinscak DJ, Schembri GP, Bailey EA, Willowson KP, Bailey DL. SPECT/CT in V/Q scanning. Semin Nucl Med (2010) 40:455–66. doi: 10.1053/j.semnuclmed.2010.07.005
6. De Ruysscher D, Niedermann G, Burnet NG, Siva S, Lee AWM, Hegi-Johnson F. Radiotherapy toxicity. Nat Rev Dis Primers. (2019) 5:13. doi: 10.1038/s41572-019-0064-5
7. Siva S, Hardcastle N, Kron T, Bressel M, Callahan J, MacManus MP, et al. Ventilation/Perfusion positron emission tomography–based assessment of radiation injury to lung. Int J Radiat Oncol Biol Phys (2015) 93:408–17. doi: 10.1016/j.ijrobp.2015.06.005
8. Giuranno L, Ient J, De Ruysscher D, Vooijs MA. Radiation-induced lung injury (RILI). Front Oncol (2019) 9:877. doi: 10.3389/fonc.2019.00877
9. Hanania AN, Mainwaring W, Ghebre YT, Hanania NA, Ludwig M. Radiation-induced lung injury: Assessment and management. Chest (2019) 156:150–62. doi: 10.1016/j.chest.2019.03.033
10. Verma V, Choi JI, Sawant A, Gullapalli RP, Chen W, Alavi A, et al. Use of PET and other functional imaging to guide target delineation in radiation oncology. Semin Radiat Oncol (2018) 28:171–7. doi: 10.1016/j.semradonc.2018.02.001
11. Ireland RH, Tahir BA, Wild JM, Lee CE, Hatton MQ. Functional image-guided radiotherapy planning for normal lung avoidance. Clin Oncol (R Coll Radiol). (2016) 28:695–707. doi: 10.1016/j.clon.2016.08.005
12. Sommer G, Bauman G. [MRI methods for pulmonary ventilation and perfusion imaging]. Radiologe (2016) 56:106–12. doi: 10.1007/s00117-015-0074-6
13. van Beek EJR, Hoffman EA. Functional imaging: CT and MRI. Clin Chest Med (2008) 29:195–216. doi: 10.1016/j.ccm.2007.12.003
14. Zhang LJ, Zhou CS, Schoepf UJ, Sheng HX, Wu SY, Krazinski AW, et al. Dual-energy CT lung ventilation/perfusion imaging for diagnosing pulmonary embolism. Eur Radiol (2013) 23:2666–75. doi: 10.1007/s00330-013-2907-x
15. Hwang HJ, Hoffman EA, Lee CH, Goo JM, Levin DL, Kauczor H, et al. The role of dual-energy computed tomography in the assessment of pulmonary function. Eur J Radiol (2017) 86:320–34. doi: 10.1016/j.ejrad.2016.11.010
16. Yuan ST, Frey KA, Gross MD, Hayman JA, Arenberg D, Curtis JL, et al. Semiquantification and classification of local pulmonary function by V/Q single photon emission computed tomography in patients with non-small cell lung cancer: Potential indication for radiotherapy planning. J Thorac Oncol (2011) 6:71–8. doi: 10.1097/JTO.0b013e3181f77b40
17. Bucknell NW, Hardcastle N, Bressel M, Hofman MS, Kron T, Ball D, et al. Functional lung imaging in radiation therapy for lung cancer: A systematic review and meta-analysis. Radiother Oncol (2018) 129:196–208. doi: 10.1016/j.radonc.2018.07.014
18. Jones AT, Hansell DM, Evans TW. Pulmonary perfusion quantified by electron-beam computed tomography: Effects of hypoxia and inhaled NO. Eur Respir J (2003) 21:855–61. doi: 10.1183/09031936.03.00085002
19. Hoffman EA, Chon D. Computed tomography studies of lung ventilation and perfusion. Proc Am Thorac Soc (2005) 2:492–8. doi: 10.1513/pats.200509-099DS
20. Chon D, Simon BA, Beck KC, Shikata H, Saba OI, Won C, et al. Differences in regional wash-in and wash-out time constants for xenon-CT ventilation studies. Respir Physiol Neurobiol (2005) 148:65–83. doi: 10.1016/j.resp.2005.06.003
21. Sheikh K, Coxson HO, Parraga G. This is what COPD looks like. Respirology (2016) 21:224–36. doi: 10.1111/resp.12611
22. Miyawaki S, Tawhai MH, Hoffman EA, Lin C. Effect of carrier gas properties on aerosol distribution in a CT-based human airway numerical model. Ann BioMed Eng. (2012) 40:1495–507. doi: 10.1007/s10439-011-0503-2
23. Fuld MK, Halaweish AF, Newell JDJ, Krauss B, Hoffman EA. Optimization of dual-energy xenon-computed tomography for quantitative assessment of regional pulmonary ventilation. Invest Radiol (2013) 48:629–37. doi: 10.1097/RLI.0b013e31828ad647
24. Mahnken AH, Jost G, Pietsch H. Krypton for computed tomography lung ventilation imaging: Preliminary animal data. Invest Radiol (2015) 50:305–8. doi: 10.1097/RLI.0000000000000130
25. Eslick EM, Kipritidis J, Gradinscak D, Stevens MJ, Bailey DL, Harris B, et al. CT ventilation imaging derived from breath hold CT exhibits good regional accuracy with galligas PET. Radiother Oncol (2018) 127:267–73. doi: 10.1016/j.radonc.2017.12.010
26. Ohno Y, Koyama H, Lee HY, Miura S, Yoshikawa T, Sugimura K. Contrast-enhanced CT- and MRI-based perfusion assessment for pulmonary diseases: Basics and clinical applications. Diagn Interv Radiol (2016) 22:407–21. doi: 10.5152/dir.2016.16123
27. Petersilka M, Bruder H, Krauss B, Stierstorfer K, Flohr TG. Technical principles of dual source CT. Eur J Radiol (2008) 68:362–8. doi: 10.1016/j.ejrad.2008.08.013
28. Johnson TRC. Dual-energy CT: General principles. AJR Am J Roentgenol. (2012) 199:S3–8. doi: 10.2214/AJR.12.9116
29. Forghani R, De Man B, Gupta R. Dual-energy computed tomography: Physical principles, approaches to scanning, usage, and implementation: Part 1. Neuroimaging Clin N Am (2017) 27:371–84. doi: 10.1016/j.nic.2017.03.002
30. Forghani R, De Man B, Gupta R. Dual-energy computed tomography: Physical principles, approaches to scanning, usage, and implementation: Part 2. Neuroimaging Clin N Am (2017) 27:385–400. doi: 10.1016/j.nic.2017.03.003
31. D'Angelo T, Cicero G, Mazziotti S, Ascenti G, Albrecht MH, Martin SS, et al. Dual energy computed tomography virtual monoenergetic imaging: Technique and clinical applications. Br J Radiol (2019) 92:20180546. doi: 10.1259/bjr.20180546
32. McCollough CH, Leng S, Yu L, Fletcher JG. Dual- and multi-energy CT: Principles, technical approaches, and clinical. Radiology (2015) 276:637–53. doi: 10.1148/radiol.2015142631
33. Odisio EG, Truong MT, Duran C, de Groot PM, Godoy MC. Role of dual-energy computed tomography in thoracic oncology. Radiol Clin N Am (2018) 56:535–48. doi: 10.1016/j.rcl.2018.03.011
34. Thieme SF, Hoegl S, Nikolaou K, Fisahn J, Irlbeck M, Maxien D, et al. Pulmonary ventilation and perfusion imaging with dual-energy CT. Eur Radiol (2010) 20:2882–9. doi: 10.1007/s00330-010-1866-8
35. Zhang L, Wang ZJ, Lu L, Zhu K, Chai X, Zhao YE, et al. Dual energy CT ventilation imaging after aerosol inhalation of iodinated contrast medium in rabbits. Eur J Radiol (2011) 78:266–71. doi: 10.1016/j.ejrad.2010.10.029
36. Chung YE, Hong SR, Lee M, Lee M, Lee H. Krypton-enhanced ventilation CT with dual energy technique: Experimental study for optimal krypton concentration. Exp Lung Res (2014) 40:439–46. doi: 10.3109/01902148.2014.946630
37. Fuld MK, Halaweish AF, Haynes SE, Divekar AA, Guo J, Hoffman EA. Pulmonary perfused blood volume with dual-energy CT as surrogate for pulmonary perfusion assessed with dynamic multidetector CT. Radiology (2013) 267:747–56. doi: 10.1148/radiol.12112789
38. Lu GM, Zhao Y, Zhang LJ, Schoepf UJ. Dual-energy CT of the lung. AJR Am J Roentgenol. (2012) 199:S40–53. doi: 10.2214/AJR.12.9112
39. Si-Mohamed S, Moreau-Triby C, Tylski P, Tatard-Leitman V, Wdowik Q, Boccalini S, et al. Head-to-head comparison of lung perfusion with dual-energy CT and SPECT-CT. Diagn Interv Imaging. (2020) 101:299–310. doi: 10.1016/j.diii.2020.02.006
40. Bahig H, Campeau M, Lapointe A, Bedwani S, Roberge D, de Guise J, et al. Phase 1-2 study of dual-energy computed tomography for assessment of pulmonary function in radiation therapy planning. Int J Radiat Oncol Biol Phys (2017) 99:334–43. doi: 10.1016/j.ijrobp.2017.05.051
41. Hong SR, Chang S, Im DJ, Suh YJ, Hong YJ, Hur J, et al. Feasibility of single scan for simultaneous evaluation of regional krypton and iodine concentrations with dual-energy CT: An experimental study. Radiology (2016) 281:597–605. doi: 10.1148/radiol.16152429
42. Waxweiler T, Schubert L, Diot Q, Faught A, Stuhr K, Castillo R, et al. A complete 4DCT-ventilation functional avoidance virtual trial: Developing strategies for prospective clinical trials. J Appl Clin Med Phys (2017) 18:144–52. doi: 10.1002/acm2.12086
43. Latifi K, Forster KM, Hoffe SE, Dilling TJ, van Elmpt W, Dekker A, et al. Dependence of ventilation image derived from 4D CT on deformable image registration and ventilation algorithms. J Appl Clin Med Phys (2013) 14:150–62. doi: 10.1120/jacmp.v14i4.4247
44. Huang Q, Jabbour SK, Xiao Z, Yue N, Wang X, Cao H, et al. Dosimetric feasibility of 4DCT-ventilation imaging guided proton therapy for locally advanced non-small-cell lung cancer. Radiat Oncol (2018) 13:78. doi: 10.1186/s13014-018-1018-x
45. Brennan D, Schubert L, Diot Q, Castillo R, Castillo E, Guerrero T, et al. Clinical validation of 4-dimensional computed tomography ventilation with pulmonary function test data. Int J Radiat Oncol Biol Phys (2015) 92:423–9. doi: 10.1016/j.ijrobp.2015.01.019
46. Mathew L, Wheatley A, Castillo R, Castillo E, Rodrigues G, Guerrero T, et al. Hyperpolarized (3)He magnetic resonance imaging: Comparison with four-dimensional x-ray computed tomography imaging in lung cancer. Acad Radiol (2012) 19:1546–53. doi: 10.1016/j.acra.2012.08.007
47. Vinogradskiy Y, Koo PJ, Castillo R, Castillo E, Guerrero T, Gaspar LE, et al. Comparison of 4-dimensional computed tomography ventilation with nuclear medicine ventilation-perfusion imaging: A clinical validation study. Int J Radiat Oncol Biol Phys (2014) 89:199–205. doi: 10.1016/j.ijrobp.2014.01.009
48. Yamamoto T, Kabus S, Lorenz C, Mittra E, Hong JC, Chung M, et al. Pulmonary ventilation imaging based on 4-dimensional computed tomography: Comparison with pulmonary function tests and SPECT ventilation images. Int J Radiat Oncol Biol Phys (2014) 90:414–22. doi: 10.1016/j.ijrobp.2014.06.006
49. Kipritidis J, Siva S, Hofman MS, Callahan J, Hicks RJ, Keall PJ. Validating and improving CT ventilation imaging by correlating with ventilation 4D-PET/CT using 68Ga-labeled nanoparticles. Med Phys (2014) 41:11910. doi: 10.1118/1.4856055
50. Borm KJ, Oechsner M, Wiegandt M, Hofmeister A, Combs SE, Duma MN. Moving targets in 4D-CTs versus MIP and AIP: Comparison of patients data to phantom data. BMC Cancer. (2018) 18:760. doi: 10.1186/s12885-018-4647-4
51. Castillo R, Castillo E, Martinez J, Guerrero T. Ventilation from four-dimensional computed tomography: Density versus Jacobian methods. Phys Med Biol (2010) 55:4661–85. doi: 10.1088/0031-9155/55/16/004
52. Kida S, Bal M, Kabus S, Negahdar M, Shan X, Loo BW, et al. CT ventilation functional image-based IMRT treatment plans are comparable to SPECT ventilation functional image-based plans. Radiother Oncol (2016) 118:521–7. doi: 10.1016/j.radonc.2016.02.019
53. Tian Y, Miao J, Liu Z, Huang P, Wang W, Wang X, et al. Availability of a simplified lung ventilation imaging algorithm based on four-dimensional computed tomography. Phys Med (2019) 65:53–8. doi: 10.1016/j.ejmp.2019.08.006
54. Liu Z, Miao J, Huang P, Wang W, Wang X, Zhai Y, et al. A deep learning method for producing ventilation images from 4DCT: First comparison with technegas SPECT ventilation. Med Phys (2020) 47:1249–57. doi: 10.1002/mp.14004
55. Kipritidis J, Hofman MS, Siva S, Callahan J, Le Roux P, Woodruff HC, et al. Estimating lung ventilation directly from 4D CT hounsfield unit values. Med Phys (2016) 43:33. doi: 10.1118/1.4937599
56. Zhong Y, Vinogradskiy Y, Chen L, Myziuk N, Castillo R, Castillo E, et al. Technical note: Deriving ventilation imaging from 4DCT by deep convolutional neural network. Med Phys (2019) 46:2323–9. doi: 10.1002/mp.13421
57. Wielputz M, Kauczor H. MRI Of the lung–state of the art. Diagn Interv Radiol (2011) 18:344–53. doi: 10.4261/1305-3825.DIR.5365-11.0
58. Mirsadraee S, van Beek EJR. Functional imaging: Computed tomography and MRI. Clin Chest Med (2015) 36:349–63. doi: 10.1016/j.ccm.2015.02.014
59. Nyilas S, Bauman G, Sommer G. [Assessment of lung impairment in patients with cystic fibrosis: Novel magnetic resonance imaging methods]. Radiologe (2020) 60:823–30. doi: 10.1007/s00117-020-00730-1
60. Kaireit TF, Gutberlet M, Voskrebenzev A, Freise J, Welte T, Hohlfeld JM, et al. Comparison of quantitative regional ventilation-weighted fourier decomposition MRI with dynamic fluorinated gas washout MRI and lung function testing in COPD patients. J Magn Reson Imaging. (2018) 47:1534–41. doi: 10.1002/jmri.25902
61. Nyilas S, Bauman G, Pusterla O, Ramsey K, Singer F, Stranzinger E, et al. Ventilation and perfusion assessed by functional MRI in children with CF: Reproducibility in comparison to lung function. J Cyst Fibros. (2019) 18:543–50. doi: 10.1016/j.jcf.2018.10.003
62. Bauman G, Lützen U, Ullrich M, Gaass T, Dinkel J, Elke G, et al. Pulmonary functional imaging: Qualitative comparison of fourier decomposition MR imaging with SPECT/CT in porcine lung. Radiology (2011) 260:551–9. doi: 10.1148/radiol.11102313
63. Bondesson D, Schneider MJ, Gaass T, Kühn B, Bauman G, Dietrich O, et al. Nonuniform Fourier-decomposition MRI for ventilation- and perfusion-weighted imaging of the lung. Magn Reson Med (2019) 82:1312–21. doi: 10.1002/mrm.27803
64. Bauman G, Pusterla O, Bieri O. Ultra-fast steady-state free precession pulse sequence for fourier decomposition pulmonary MRI. Magn Reson Med (2016) 75:1647–53. doi: 10.1002/mrm.25697
65. Bauman G, Pusterla O, Bieri O. Functional lung imaging with transient spoiled gradient echo. Magn Reson Med (2019) 81:1915–23. doi: 10.1002/mrm.27535
66. Bauman G, Bieri O. Matrix pencil decomposition of time-resolved proton MRI for robust and improved assessment of pulmonary ventilation and perfusion. Magn Reson Med (2017) 77:336–42. doi: 10.1002/mrm.26096
67. Doganay O, Matin T, Chen M, Kim M, McIntyre A, McGowan DR, et al. Time-series hyperpolarized xenon-129 MRI of lobar lung ventilation of COPD in comparison to V/Q-SPECT/CT and CT. Eur Radiol (2019) 29:4058–67. doi: 10.1007/s00330-018-5888-y
68. Rankine LJ, Wang Z, Driehuys B, Marks LB, Kelsey CR, Das SK. Correlation of regional lung ventilation and gas transfer to red blood cells: Implications for functional-avoidance radiation therapy planning. Int J Radiat Oncol Biol Phys (2018) 101:1113–22. doi: 10.1016/j.ijrobp.2018.04.017
69. Johns CS, Swift AJ, Rajaram S, Hughes PJC, Capener DJ, Kiely DG, et al. Lung perfusion: MRI vs. SPECT for screening in suspected chronic thromboembolic pulmonary hypertension. J Magn Reson Imaging (2017) 46:1693–7. doi: 10.1002/jmri.25714
70. Sommer G, Bauman G, Koenigkam-Santos M, Draenkow C, Heussel CP, Kauczor H, et al. Non-contrast-enhanced preoperative assessment of lung perfusion in patients with non-small-cell lung cancer using Fourier decomposition magnetic resonance imaging. Eur J Radiol (2013) 82:e879–87. doi: 10.1016/j.ejrad.2013.06.030
71. Maxien D, Ingrisch M, Meinel F, Reiser M, Dietrich O, Nikolaou K. Quantification of pulmonary perfusion with free-breathing dynamic contrast-enhanced MRI–a pilot study in healthy volunteers. Rofo (2013) 185:1175–81. doi: 10.1055/s-0033-1350128
72. Elojeimy S, Cruite I, Bowen S, Zeng J, Vesselle H. Overview of the novel and improved pulmonary ventilation-perfusion imaging applications in the era of SPECT/CT. AJR Am J Roentgenol. (2016) 207:1307–15. doi: 10.2214/AJR.15.15071
73. Bailey DL, Roach PJ. A brief history of lung ventilation and perfusion imaging over the 50-year tenure of the editors of seminars in nuclear medicine. Semin Nucl Med (2020) 50:75–86. doi: 10.1053/j.semnuclmed.2019.07.004
74. Hess S, Madsen PH. Radionuclide diagnosis of pulmonary embolism. Adv Exp Med Biol (2017) 906:49–65. doi: 10.1007/5584_2016_105
75. Le Roux P, Hicks RJ, Siva S, Hofman MS. PET/CT lung ventilation and perfusion scanning using galligas and gallium-68-MAA. Semin Nucl Med (2019) 49:71–81. doi: 10.1053/j.semnuclmed.2018.10.013
76. Bailey DL, Eslick EM, Schembri GP, Roach PJ. (68)Ga PET ventilation and perfusion lung imaging-current status and future challenges. Semin Nucl Med (2016) 46:428–35. doi: 10.1053/j.semnuclmed.2016.04.007
77. Das SK, McGurk R, Miften M, Mutic S, Bowsher J, Bayouth J, et al. Task group 174 report: Utilization of [(18) F]Fluorodeoxyglucose positron emission tomography ([(18) F]FDG-PET) in radiation therapy. Med Phys (2019) 46:e706–25. doi: 10.1002/mp.13676
78. Oehme L, Zöphel K, Golgor E, Andreeff M, Wunderlich G, Brogsitter C, et al. Quantitative analysis of regional lung ventilation and perfusion PET with (68)Ga-labelled tracers. Nucl Med Commun (2014) 35:501–10. doi: 10.1097/MNM.0000000000000084
79. Le Roux P, Siva S, Steinfort DP, Callahan J, Eu P, Irving LB, et al. Correlation of 68Ga ventilation-perfusion PET/CT with pulmonary function test indices for assessing lung function. J Nucl Med (2015) 56:1718–23. doi: 10.2967/jnumed.115.162586
80. Le Roux P, Siva S, Callahan J, Claudic Y, Bourhis D, Steinfort DP, et al. Automatic delineation of functional lung volumes with (68)Ga-ventilation/perfusion PET/CT. EJNMMI Res (2017) 7:82. doi: 10.1186/s13550-017-0332-x
81. Hatt M, Lee JA, Schmidtlein CR, Naqa IE, Caldwell C, De Bernardi E, et al. Classification and evaluation strategies of auto-segmentation approaches for PET: Report of AAPM task group no. 211. Med Phys (2017) 44:e1–42. doi: 10.1002/mp.12124
82. Yamamoto T, Kabus S, Bal M, Keall P, Benedict S, Daly M. The first patient treatment of computed tomography ventilation functional image-guided radiotherapy for lung cancer. Radiother Oncol (2016) 118:227–31. doi: 10.1016/j.radonc.2015.11.006
83. Wang R, Zhang S, Yu H, Lin S, Zhang G, Tang R, et al. Optimal beam arrangement for pulmonary ventilation image-guided intensity-modulated radiotherapy for lung cancer. Radiat Oncol (2014) 9:184. doi: 10.1186/1748-717X-9-184
84. Hoover DA, Capaldi DP, Sheikh K, Palma DA, Rodrigues GB, Dar AR, et al. Functional lung avoidance for individualized radiotherapy (FLAIR): Study protocol for a randomized, double-blind clinical trial. BMC Cancer (2014) 14:934. doi: 10.1186/1471-2407-14-934
85. Bates EL, Bragg CM, Wild JM, Hatton MQF, Ireland RH. Functional image-based radiotherapy planning for non-small cell lung cancer: A simulation study. Radiother Oncol (2009) 93:32–6. doi: 10.1016/j.radonc.2009.05.018
86. Siva S, Devereux T, Ball DL, MacManus MP, Hardcastle N, Kron T, et al. Ga-68 MAA perfusion 4D-PET/CT scanning allows for functional lung avoidance using conformal radiation therapy planning. Technol Cancer Res Treat (2016) 15:114–21. doi: 10.1177/1533034614565534
87. Siva S, Thomas R, Callahan J, Hardcastle N, Pham D, Kron T, et al. High-resolution pulmonary ventilation and perfusion PET/CT allows for functionally adapted intensity modulated radiotherapy in lung cancer. Radiother Oncol (2015) 115:157–62. doi: 10.1016/j.radonc.2015.04.013
88. Lee SJ, Park HJ. Single photon emission computed tomography (SPECT) or positron emission tomography (PET) imaging for radiotherapy planning in patients with lung cancer: A meta-analysis. Sci Rep (2020) 10:14864. doi: 10.1038/s41598-020-71445-5
89. Ieko Y, Kadoya N, Kanai T, Nakajima Y, Arai K, Kato T, et al. The impact of 4DCT-ventilation imaging-guided proton therapy on stereotactic body radiotherapy for lung cancer. Radiol Phys Technol (2020) 13:230–7. doi: 10.1007/s12194-020-00572-5
90. Dougherty JM, Castillo E, Castillo R, Faught AM, Pepin M, Park SS, et al. Functional avoidance-based intensity modulated proton therapy with 4DCT derived ventilation imaging for lung cancer. J Appl Clin Med Phys (2021) 22:276–85. doi: 10.1002/acm2.13323
91. Farr KP, Kallehauge JF, Møller DS, Khalil AA, Kramer S, Bluhme H, et al. Inclusion of functional information from perfusion SPECT improves predictive value of dose-volume parameters in lung toxicity outcome after radiotherapy for non-small cell lung cancer: A prospective study. Radiother Oncol (2015) 117:9–16. doi: 10.1016/j.radonc.2015.08.005
92. Hoover DA, Reid RH, Wong E, Stitt L, Sabondjian E, Rodrigues GB, et al. SPECT-based functional lung imaging for the prediction of radiation pneumonitis: A clinical and dosimetric correlation. J Med Imaging Radiat Oncol (2014) 58:214–22. doi: 10.1111/1754-9485.12145
93. Xiao L, Yang G, Chen J, Wang X, Wu Q, Huo Z, et al. To find a better dosimetric parameter in the predicting of radiation-induced lung toxicity individually: Ventilation, perfusion or CT based. Sci Rep (2017) 7:44646. doi: 10.1038/srep44646
94. Ding X, Man X, Sun M, Xing J, Fan T, Gao M, et al. Which is the optimal threshold for defining functional lung in single-photon emission computed tomography lung perfusion imaging of lung cancer patients? Nucl Med Commun (2018) 39:103–9. doi: 10.1097/MNM.0000000000000774
95. Faught AM, Miyasaka Y, Kadoya N, Castillo R, Castillo E, Vinogradskiy Y, et al. Evaluating the toxicity reduction with computed tomographic ventilation functional avoidance radiation therapy. Int J Radiat Oncol Biol Phys (2017) 99:325–33. doi: 10.1016/j.ijrobp.2017.04.024
96. Vinogradskiy Y, Castillo R, Castillo E, Schubert L, Jones BL, Faught A, et al. Results of a multi-institutional phase 2 clinical trial for 4DCT-ventilation functional avoidance thoracic radiation therapy. Int J Radiat Oncol Biol Phys (2022) 112:986–95. doi: 10.1016/j.ijrobp.2021.10.147
97. Huang Y. Xenon-enhanced ventilation CT-guided radiotherapy for lung cancer treatment. ClinicalTrials.gov.
98. Filion E. Use of dual energy computed tomography in thoracic radiotherapy planning. ClinicalTrials.gov.
100. Hsu F. Image-guided functional lung avoidance thoracic radiotherapy for lung cancer: A single-blind randomized trial. ClinicalTrials.gov. Available at: https://clinicaltrials.gov/ct2/show/NCT03077854.
102. Vinogradskiy Y. Feasibility study incorporating lung function imaging into radiation therapy for lung cancer patients. ClinicalTrials.gov. Available at: https://clinicaltrials.gov/ct2/show/NCT02528942.
103. Hoover DA, Capaldi DP, Sheikh K, Palma DA, Rodrigues GB, Dar AR, et al. Functional lung avoidance for individualized radiotherapy (FLAIR): Study protocol for a randomized, double-blind clinical trial. BMC Cancer. (2014) 14:934. doi: 10.1186/1471-2407-14-934
104. Zeng J. FLARE RT for patients with stage IIB-IIIB non-small cell lung cancer: Personalizing radiation therapy using PET/CT and SPECT/CT imaging. ClinicalTrials.gov.
105. Farr K. Role of SPECT in radiotherapy of lung cancer and toxicity evaluation. ClinicalTrials.gov. Available at: https://clinicaltrials.gov/ct2/show/NCT01745484.
106. Khalil AA, Hau E, Gebski V, Grau C, Gee H, Nyeng TB, et al. Personal innovative approach in radiation therapy of lung cancer- functional lung avoidance SPECT-guided (ASPECT) radiation therapy: A study protocol for phase II randomised double-blind clinical trial. BMC Cancer (2021) 21:940. doi: 10.1186/s12885-021-08663-1
107. Rachel V. Lung perfusion PET/CT using Ga68-MAA for preservation of lung function during stereotactic pulmonary radiation therapy. ClinicalTrials.gov. Available at: https://clinicaltrials.gov/ct2/show/NCT04942275.
108. Bucknell N, Hardcastle N, Jackson P, Hofman M, Callahan J, Eu P, et al. Single-arm prospective interventional study assessing feasibility of using gallium-68 ventilation and perfusion PET/CT to avoid functional lung in patients with stage III non-small cell lung cancer. BMJ Open (2020) 10:e42465. doi: 10.1136/bmjopen-2020-042465
109. Sim AJ, Kaza E, Singer L, Rosenberg SA. A review of the role of MRI in diagnosis and treatment of early stage lung cancer. Clin Transl Radiat Oncol (2020) 24:16–22. doi: 10.1016/j.ctro.2020.06.002
110. Forghani F, Patton T, Kwak J, Thomas D, Diot Q, Rusthoven C, et al. Characterizing spatial differences between SPECT-ventilation and SPECT-perfusion in patients with lung cancer undergoing radiotherapy. Radiother Oncol (2021) 160:120–4. doi: 10.1016/j.radonc.2021.04.022
111. Li Z, Le Roux P, Callahan J, Hardcastle N, Hofman MS, Siva S, et al. Quantitative assessment of ventilationperfusion relationships with gallium-68 positron emission tomography/computed tomography imaging in lung cancer patients. Phys Imaging Radiat Oncol (2022) 22:8–12. doi: 10.1016/j.phro.2022.03.005
112. Meng X, Frey K, Matuszak M, Paul S, Ten Haken R, Yu J, et al. Changes in functional lung regions during the course of radiation therapy and their potential impact on lung dosimetry for non-small cell lung cancer. Int J Radiat Oncol Biol Phys (2014) 89:145–51. doi: 10.1016/j.ijrobp.2014.01.044
113. Yin Y, Chen J, Li B, Liu T, Lu J, Bai T, et al. Protection of lung function by introducing single photon emission computed tomography lung perfusion image into radiotherapy plan of lung cancer. Chin Med J (Engl). (2009) 122:509–13. doi: 10.3760/cma.j.issn.0366-6999.2009.05.005
114. Shioyama YMDP, Jang SYPD, Liu HHPD, Guerrero TMDP, Wang XPD, Gayed IWMD, et al. Preserving functional lung using perfusion imaging and intensity-modulated radiation therapy for advanced-stage non–small cell lung cancer. Int J Radiat Oncol Biol Phys (2007) 68:1349–58. doi: 10.1016/j.ijrobp.2007.02.015
115. Yuan ST, Frey KA, Gross MD, Hayman JA, Arenberg D, Cai X, et al. Changes in global function and regional ventilation and perfusion on SPECT during the course of radiotherapy in patients with non-Small-Cell lung cancer. Int J Radiat Oncol Biol Phys (2012) 82:e631–8. doi: 10.1016/j.ijrobp.2011.07.044
116. Yamamoto T, Kabus S, Bal M, Bzdusek K, Keall PJ, Wright C, et al. Changes in regional ventilation during treatment and dosimetric advantages of CT ventilation image guided radiation therapy for locally advanced lung cancer. Int J Radiat Oncol Biol Phys (2018) 102:1366–73. doi: 10.1016/j.ijrobp.2018.04.063
Keywords: functional lung, ventilation (V), perfusion (Q), radiotherapy, four-dimensional CT, magnetic resonance imaging (MRI), single-photon emission computed tomography (SPECT), positron emission tomography (PET)
Citation: Zhou P-X and Zhang S-X (2022) Functional lung imaging in thoracic tumor radiotherapy: Application and progress. Front. Oncol. 12:908345. doi: 10.3389/fonc.2022.908345
Received: 30 March 2022; Accepted: 17 August 2022;
Published: 23 September 2022.
Edited by:
Emanuele Neri, University of Pisa, ItalyReviewed by:
Sunyoung Jang, Princeton Radiation Oncology Center, United StatesYilin Liu, Memorial Sloan Kettering Cancer Center, United States
Copyright © 2022 Zhou and Zhang. This is an open-access article distributed under the terms of the Creative Commons Attribution License (CC BY). The use, distribution or reproduction in other forums is permitted, provided the original author(s) and the copyright owner(s) are credited and that the original publication in this journal is cited, in accordance with accepted academic practice. No use, distribution or reproduction is permitted which does not comply with these terms.
*Correspondence: Shu-Xu Zhang, gthzsx@gzhmu.edu.cn