- 1Department of Respiratory Medicine, Gansu Provincial Hospital, Lanzhou, China
- 2The First School of Clinical Medicine, Lanzhou University, Lanzhou, China
- 3Respiratory Medicine Unit, Department of Medicine, Karolinska Institute, Stockholm, Sweden
- 4Department of Pulmonary Medicine, University of Helsinki and Helsinki University Hospital, Helsinki, Finland
- 5Department of Pathology, Gansu Provincial Hospital, Lanzhou, China
Background: The competing endogenous RNA (ceRNA) network-mediated regulatory mechanisms in small cell lung cancer (SCLC) remain largely unknown. This study aimed to integrate multi-omics profiles, including the transcriptome, regulome, genome and pharmacogenome profiles, to elucidate prioritised ceRNA characteristics, pathways and drug candidates in SCLC.
Method: We determined the plasma messenger RNA (mRNA), microRNA (miRNA), long noncoding RNA (lncRNA) and circular RNA (circRNA) expression levels using whole-transcriptome sequencing technology in our SCLC plasma cohort. Significantly expressed plasma mRNAs were then overlapped with the Gene Expression Omnibus (GEO) tissue mRNA data (GSE 40275, SCLC tissue cohort). Next, we applied a multistep multi-omics (transcriptome, regulome, genome and pharmacogenome) integration analysis to first construct the network and then to identify the lncRNA/circRNA-miRNA-mRNA ceRNA characteristics, genomic alterations, pathways and drug candidates in SCLC.
Results: The multi-omics integration-based prioritisation of SCLC ceRNA regulatory networks consisted of downregulated mRNAs (CSF3R/GAA), lncRNAs (AC005005.4-201/DLX6-AS1-201/NEAT1-203) and circRNAs (hsa_HLA-B_1/hsa_VEGFC_8) as well as upregulated miRNAs (hsa-miR-4525/hsa-miR-6747-3p). lncRNAs (lncRNA-AC005005.4-201 and NEAT1-203) and circRNAs (circRNA-hsa_HLA-B_1 and hsa_VEGFC_8) may regulate the inhibited effects of hsa-miR-6747-3p for CSF3R expression in SCLC, while lncRNA-DLX6-AS1-201 or circRNA-hsa_HLA-B_1 may neutralise the negative regulation of hsa-miR-4525 for GAA in SCLC. CSF3R and GAA were present in the genomic alteration, and further identified as targets of FavId and Trastuzumab deruxtecan, respectively. In the SCLC-associated pathway analysis, CSF3R was involved in the autophagy pathways, while GAA was involved in the glucose metabolism pathways.
Conclusions: We identified potential lncRNA/cirRNA-miRNA-mRNA ceRNA regulatory mechanisms, pathways and promising drug candidates in SCLC, providing novel potential diagnostics and therapeutic targets in SCLC.
Introduction
Small cell lung cancer (SCLC) is a highly heterogeneous malignancy of neuroendocrine origin accounting for approximately 15% of all cases of lung cancer. SCLC is characterised by the early development of metastases, rapid recurrence and a low survival rate (1–4). The 5-year overall survival rate in SCLC barely reaches 5%, while average overall survival reaches only 2 to 4 months in untreated patients (1, 5, 6). Early diagnosis of SCLC remains quite challenging given its nonspecific symptoms and fast-growing tumours (7). Currently, chemotherapy and immunotherapy represent the most common treatment for SCLC, whereby chemotherapy alone remains the basis of standard treatment for the management of SCLC (7). While the initial response rate for first-line chemotherapy reaches approximately 60% in SCLC, patients may still quickly succumb given rapid recurrence following chemotherapy, primary or secondary drug resistance and ineffective second-line treatment options (8–10). Thus, limited effective therapies remain the primary reason for poor outcomes in SCLC (7, 8). The mechanisms behind the pathogenesis of SCLC are complex, and as yet unexplained by a single biomarker or specific mechanism (11). As such, an increased and comprehensive understanding of SCLC characteristics is crucial to guiding both diagnosis and treatment. Omics studies are emerging rapidly and offer tremendous potential to better understand the underlying disease mechanisms, as well as advancing early diagnostics and identifying potential drug targets.
Competitive endogenous RNA (ceRNA) is a novel layer of gene regulation in diseases, regulating each other at the post-transcription level by competing for shared microRNAs (miRNAs) (12). ceRNA networks link the function of protein-coding messenger RNA (mRNA) with noncoding RNAs (ncRNAs), which primarily include long noncoding RNAs (lncRNAs), circular RNAs (circRNAs) and miRNAs (12–15). The integrative assessment of the expressions of lncRNAs, circRNAs, miRNAs and mRNAs construct ceRNA networks (14–18). Several studies demonstrated that lung cancer associates with the dysregulation of the expression of ncRNAs including both lncRNAs and miRNAs, and the expression of several signalling pathways and oncogenes, while circRNAs may play a key role in lung cancer tumorigenesis, progression, invasion and metastasis (14, 18). miRNAs could control the target genes involved in cellular processes by downregulating gene expression through repressing or degrading mRNA targets (19–21). In addition, the majority of lncRNAs compete with miRNAs to prevent miRNA binding to their target mRNA, leading to the transcriptional activation of target genes (22, 23). Furthermore, after binding to several sites for a particular miRNA or RNA-binding proteins (RBPs), cirRNAs regulate alternative splicing and gene transcription through interaction (15, 23, 24). Consequently, these aberrantly expressed transcripts in the ceRNA network may represent potential therapeutic targets, diagnostic markers and prognostic markers in SCLC. In addition to transcriptomics, gene mutations play significant roles in new drug development in cancer. For instance, gene mutation profiles have facilitated the development of targeted agents in therapeutics for adenocarcinomas of the lung (25). Drug databases are developing rapidly, and the integrative analysis of omics data and drug databases provide us with excellent opportunities for drug development such as through pharmacogenomics (26). The rapidly expanding field of systems biology has proven reasonably effective at summarising knowledge related to cancer pathways, perhaps most importantly using the cancer literature to elucidate the molecular networks via which cancer develops. Thus, methodology which employs an integrative analysis of the literature could contribute to understanding the SCLC pathways (27).
In an attempt to understand the complexity and heterogeneity of SCLC, our study aimed to identify plasma mRNAs and compare them with the expression levels found in tissue to identify SCLC-specific mRNAs (28, 29) and, further, to evaluate the lncRNA/circRNA-miRNA-mRNA ceRNA regulatory network. Next, we applied a multi-omics integration analysis (transcriptome, regulome, genome and pharmacogenome) to discuss ceRNA regulation, genomic alterations, pathways and drug candidates in SCLC (see Figure 1) (30–32). Understanding the characteristics of the ceRNA regulatory network can potentially shed light on the screening of SCLC biomarkers, particularly those related to genomic alterations and novel therapeutic targets.
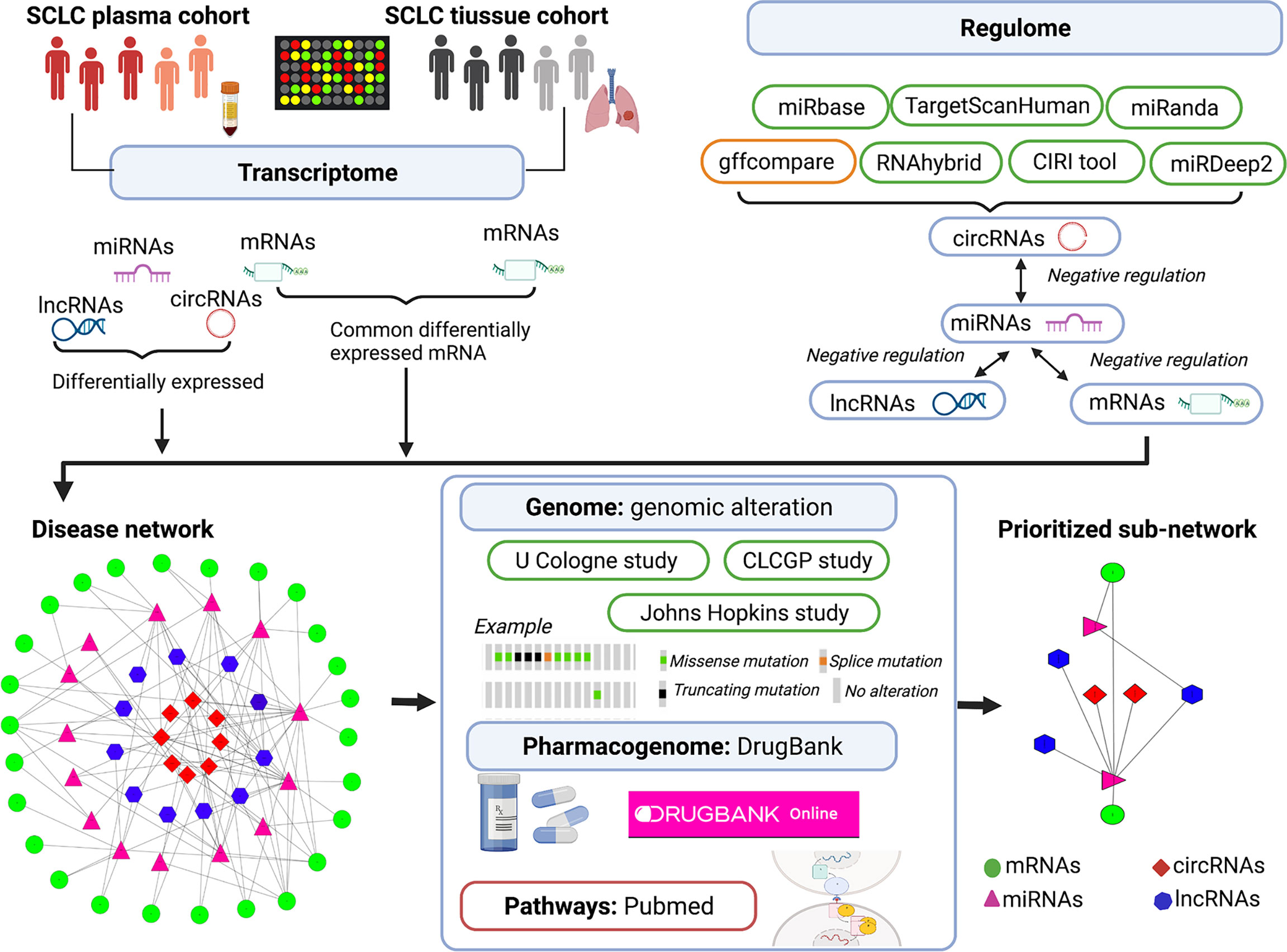
Figure 1 Illustration of multi-omics–based prioritisation of ceRNAs and pathways. CLCGP, Clinical Lung Cancer Genome Project; CIRI, circRNA identifier; ceRNA, competitive endogenous RNA; circRNA, circular RNAs; DE, differentially expressed; SCLC, small cell lung cancer; lncRNA, long noncoding RNA; miRNA, microRNA; mRNA, messenger RNA; cBioPortal database (https://www.cbioportal.org/datasets); DrugBank database (https://go.drugbank.com/); Genecards database (https://www.genecards.org/); PubMed (https://pubmed.ncbi.nlm.nih.gov/).
Materials and Methods
In-House SCLC Plasma Cohort and SCLC Lung Tissue Cohort
In this study, we analysed two SCLC cohorts: an in-house SCLC plasma cohort (n = 12) and an SCLC lung tissue cohort (from GSE40275, n = 62) (33). The mRNA data in the SCLC tissue cohort were obtained from the lung tissue samples of SCLCs and adjacent nontumour regions. Our in-house SCLC plasma cohort includes eight SCLC patients and four healthy controls, collected between August and November 2020 at Gansu Provincial Hospital, China. The inclusion criteria of patients in our SCLC plasma cohort consisted of a histologically or cytologically confirmed initial SCLC without previous chemotherapy, radiotherapy, molecular-targeted therapy, immunotherapy or surgery. We excluded patients from our SCLC plasma cohort based on the following: (1) presence of other combined cancers; (2) pregnant or lactating patient; and (3) presentation with cardiopulmonary insufficiency, serious cardiovascular disease, a serious infection or severe malnutrition (34, 35). The mRNA data in the SCLC tissue cohort were obtained from the lung tissue samples of SCLCs and adjacent nontumour regions. In addition, the tissue mRNA expression levels were evaluated in the Gene Expression Omnibus database (GEO, https://www.ncbi.nlm.nih.gov/gds/) using the term “small cell lung cancer” with “homo sapiens”, “series” and “expression profiling by array”. The 19 SCLC lung tissue datasets were obtained, and no suitable plasma SCLC dataset could be extracted. Finally, we selected the GSE40275 tissue dataset of SCLC for further analysis, since this dataset was obtained from a single-sequencing platform, thereby avoiding a potential bias from inconsistencies in probes stemming from different sequencing platforms. This cohort study received ethical approval from the Ethics Committee of the Gansu Provincial Hospital, China (27 July 2020, No. 2020-183). Informed consent was obtained from all participants in the whole-transcriptome sequencing experiment, and the research adhered to the principles of the Declaration of Helsinki.
Whole-Transcriptome Sequencing Analysis in the Plasma SCLC Cohort
We determined the plasma messenger RNA (mRNA), microRNA (miRNA), long noncoding RNA (lncRNA) and circular RNA (circRNA) expression levels using the whole-transcriptome sequencing technology in our SCLC plasma cohort. The extraction of total RNA from the plasma samples relied on the miRNeasy Mini Kit (Qiagen, Hilden, Germany) following the manufacturer’s protocol. The details appear in Supplemental File 1. A total of 1.5-μg RNA per sample was used as the input material for the lncRNA sequencing analysis, and a total of 2.5-ng RNA was used as the input material for the miRNA sequencing analysis. The details of the lncRNA and miRNA sequencing appear in Supplemental File 1. The steps to generating the mRNA, lncRNA, circRNA and miRNA profiles appear in Supplemental File 2. In addition, our SCLC plasma data were uploaded to a public platform [uploaded to the Sequence Read Archive (SRA) database (BioProject PRJNA 759049 (miRNA data) and BioProject PRJNA 762578 (mRNA, lncRNA and circRNA data)].
Identification of Differentially Expressed mRNA, miRNA, circRNA and lncRNA in SCLC
The significant differentially expressed mRNAs (DEmRNAs) in the SCLC tissue cohort were identified by comparing SCLC lung tissue and adjacent nontumour tissue from SCLC using the GEO2R tools from the R package “limma” in GSE40275 [|fold change (FC)| > 1.5, p < 0.05, and false discovery rate (FDR) < 0.2)]. DEmRNAs in the SCLC plasma cohort were identified by comparing SCLC and healthy samples using the likelihood ratio test (LRT) in the R package “DESeq” (|FC| > 1.5, p < 0.05). Then, the commonly expressed DEmRNAs (Co-DEmRNAs, SCLC-specific mRNAs) were defined as the overlapping DEmRNAs between the SCLC plasma cohort and the SCLC lung tissue cohort (|FC| > 1.5, p < 0.05). The significant DEmiRNAs, DEcircRNAs and DElncRNAs in the SCLC plasma cohort were identified by comparing SCLC and healthy plasma samples using LRT in the R package “DESeq” (|FC| > 1.5, p < 0.05, and FDR < 0.2). FDR was computed using the methodology described by Benjamini and Hochberg (36). The volcano plots were created using the R package “ggplot2”. Finally, the Co-DEmRNAs, DEmiRNAs, DEcircRNAs and DElncRNAs were subsequently used in the ceRNA network construction.
Construction of the lncRNA/cirRNA-miRNA-mRNA ceRNA-Mediated Regulatory Network
The previous step identifying the DEmiRNAs, DElncRNAs, DEcircRNAs and Co-DEmRNAs in SCLC was used to construct the lncRNA/circRNA-miRNA-mRNA ceRNA regulatory network. The regulome analysis was based on the targeted mRNA–miRNA, lncRNA–miRNA and circRNA–miRNA prediction using online analytical software tools. The targeted mRNAs of the miRNAs were predicted using two online analytical software tools: miRanda (version 3.3.a) (37) and TargetScanHuman database (version 5.0) (38). The targeted lncRNAs of the miRNAs were predicted using the online analytical software tools from the miRbase database (version 22.0) (37). The targeted circRNAs of the miRNAs were predicted using three online analytical software tools: RNAhybrid database (version 2.1.1) (39), miRanda (version 3.3.a) (40) and TargetScanHuman database (version 5.0) (38). The negative regulation of mRNA–miRNA, lncRNA–miRNA and circRNA–miRNA was selected in the further ceRNA network construction. Next, the lncRNAs, circRNAs and miRNAs were identified as known or novel using several analytical software tools: the gffcompare program (41), the circRNA identifier (CIRI) tool (42), the miRbase database (version 22.0) (37) and the miRDeep2 tools (43). Based on these results, we constructed the lncRNA/circRNA-miRNA-mRNA ceRNA regulatory network using the Cytoscape software (version 3.7.0) (44). Next, the differentially expressed lncRNA, circRNAs, miRNAs and mRNAs in the SCLC ceRNA network were analysed using the gene ontology (GO) analysis and the Kyoto Encyclopaedia of Genes and Genomes (KEGG) pathway analysis. For the GO analysis, the differentially expressed lncRNA, circRNAs, miRNAs and mRNAs were classified into three categories: biological process (BP), cellular component (CC) and molecular function (MF). The KEGG pathway analysis was performed to analyse the potential pathways enriched by the differentially expressed lncRNA, circRNAs, miRNAs and mRNAs. The enrichment analysis was evaluated using the R package ClusterProfiler (45), for which we considered an adjusted p < 0.05 as statistically significant (46).
Evaluation of Genomic Alterations, Drug Candidates/Repurposing and Pathway Analysis in SCLC ceRNA Networks
The genomic alterations of mRNAs in the SCLC ceRNA network were determined through three datasets (47–49) from the cBioPortal database (https://www.cbioportal.org/datasets), including the Clinical Lung Cancer Genome Project (CLCGP) study (47), the Johns Hopkins study (48) and the University of Cologne study (U Cologne study) (49). The pharmacogenomics data were downloaded from the DrugBank database (release 5.0) (https://go.drugbank.com/), including the rich drugs data and the drug–target genes data (50). The results obtained from the pharmacogenomics DrugBank database were further mined through the “Targets” tool using manual searches. The pathways of the mRNAs were first evaluated and annotated using the Genecards database (https://www.genecards.org/) (51), then the SCLC-associated pathways were further filtered through a literature search from PubMed (https://pubmed.ncbi.nlm.nih.gov/) using the terms “small cell lung cancer [Title/Abstract] OR SCLC [Title/Abstract] OR small cell lung cancer [MeSH Terms]” and “pathways [Title/Abstract]”.
Results
Identification of Differentially Expressed mRNA, miRNA, circRNA and lncRNA in SCLC
We identified eight SCLC patients (62.5% male, median age of 62 years, 100% Asian and 50.0% advanced stage) and four healthy controls (75.0% male, median age of 66 years) in our SCLC plasma cohort, and 19 SCLC patients (84.2% male, median age of 66 years and 100% European) in the SCLC tissue cohort (GSE40275) (Table 1). Through our in-house whole-transcriptome sequencing data comparing SCLC plasma samples and healthy plasma samples, we harvested a total of 652 DEmRNAs (326 upregulated and 326 downregulated), 281 DEmiRNAs (178 upregulated and 103 downregulated), 286 DEcircRNAs (166 upregulated and 120 downregulated) and 1753 DElncRNAs (1036 upregulated and 717 downregulated) for subsequent analysis. Overall, 8429 DEmRNAs (4808 upregulated and 3621 downregulated) were identified in the SCLC tissue cohort, ultimately resulting in 135 DEmRNAs (32 upregulated and 103 downregulated) expressed in two cohorts as common DEmRNAs (Co-DEmRNAs), and also identified as SCLC-specific mRNAs (Figure 2).
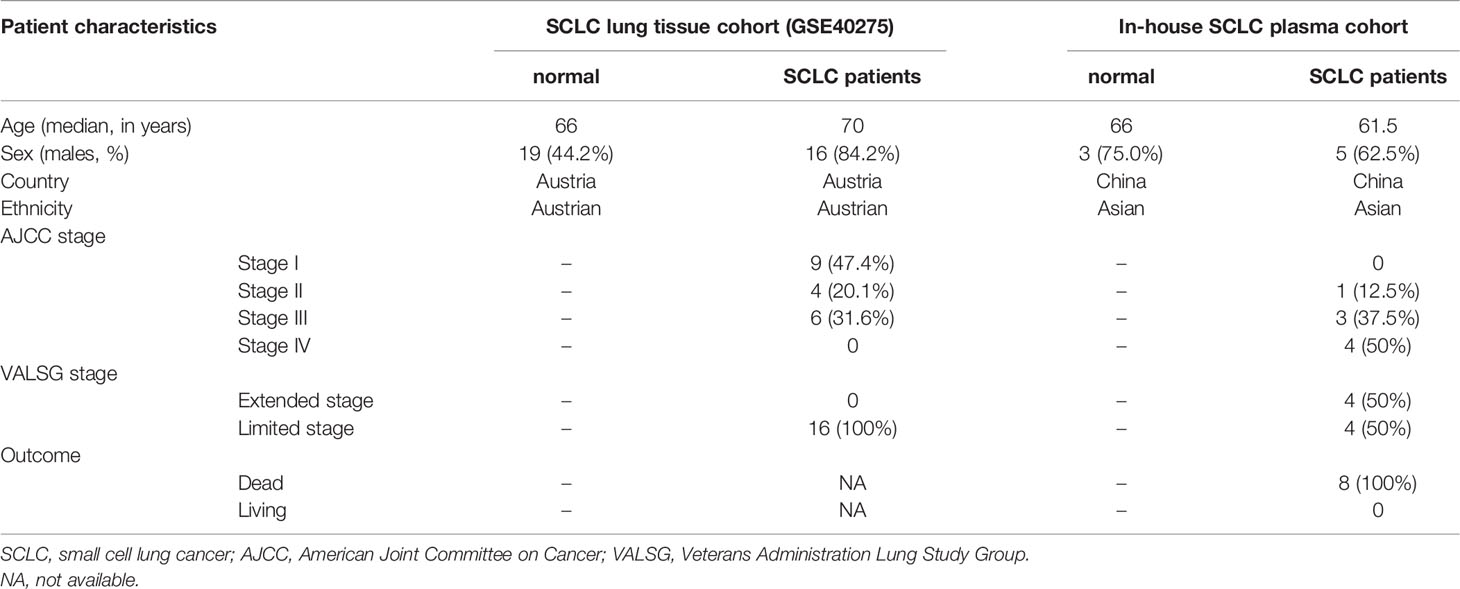
Table 1 Patient characteristics for the in-house SCLC plasma cohort (n = 12) and SCLC lung tissue cohort (from GSE40275, n = 62).
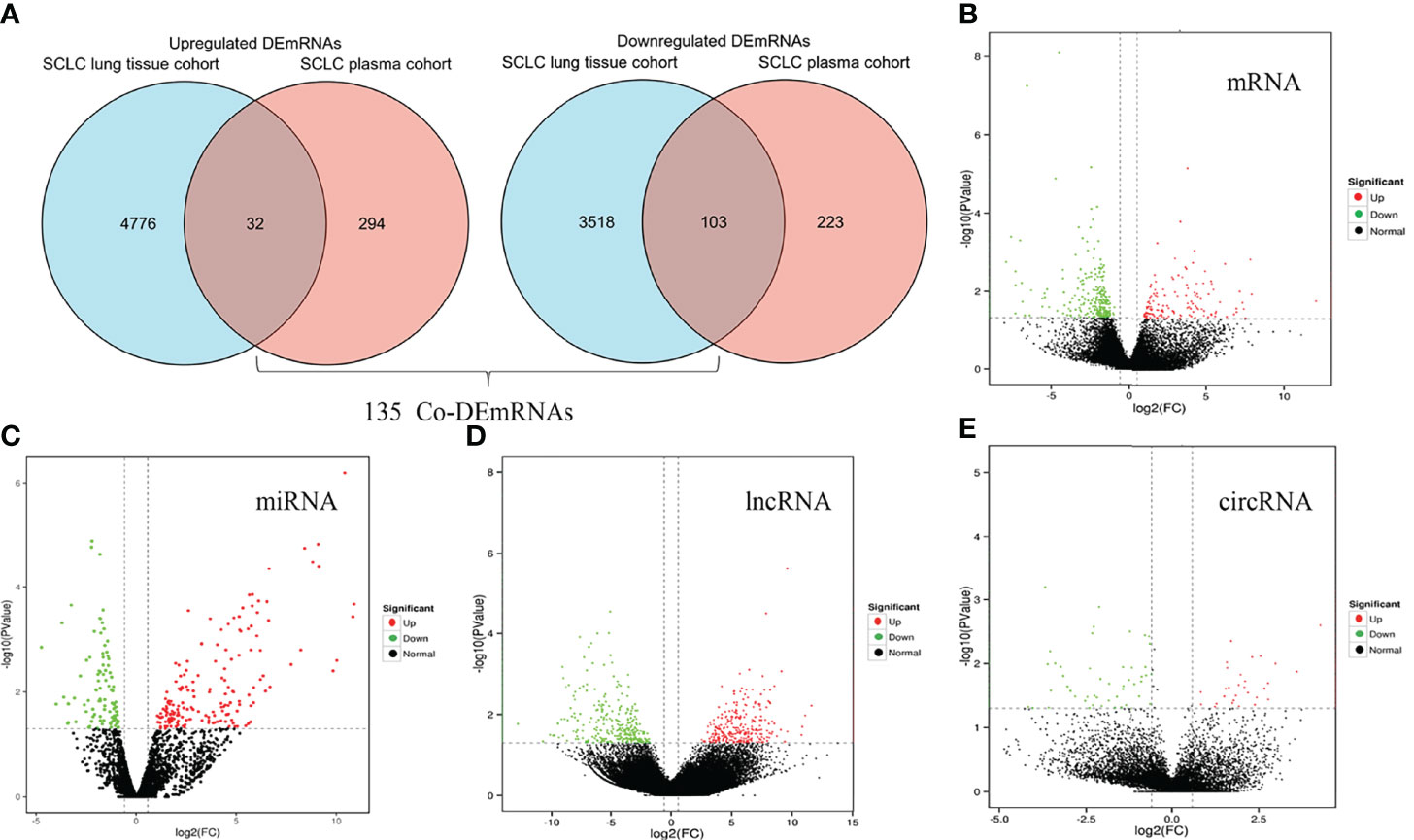
Figure 2 Identification of differentially expressed mRNAs, miRNAs, lncRNAs and circRNAs in SCLC. (A) Common differentially expressed mRNAs (Co-DEmRNAs) in the in-house SCLC plasma cohort and the SCLC lung tissue cohort (GSE40275). (B) Up- and downregulated mRNAs in our cohort. (C) Up- and downregulated miRNAs in our cohort. (D) Up- and downregulated lncRNAs in our cohort. (E) Up- and downregulated circRNAs in our cohort. Red indicates upregulated and green indicates downregulated; circRNA, circular RNAs; lncRNA, long noncoding RNA; miRNA, microRNA; mRNA, messenger RNA; SCLC, small cell lung cancer.
Construction of the lncRNA/circRNA-miRNA-mRNA ceRNA Network
The obtained 281 DEmiRNAs, 1753 DElncRNAs, 286 DEcircRNAs and 135 Co-DEmRNAs in SCLC were initially involved in the ceRNA regulatory network construction. Integrating the selection rules described in the methods section, the SCLC lncRNA/circRNA-miRNA-mRNA ceRNA regulatory network was constructed, which included 58 mRNAs (4 upregulated and 54 downregulated), 301 lncRNAs (40 upregulated and 261 downregulated), 16 circRNAs (5 upregulated and 11 downregulated) and 24 miRNAs (20 upregulated and 4 downregulated) (Figures 3 and 4; Supplemental Tables 1 and 2). The lncRNA-miRNA-mRNA ceRNA regulatory network consisted of 381 nodes (301 lncRNAs, 23 miRNAs and 57 mRNAs) with 707 edges (Figure 3). In the lncRNA-miRNA-mRNA ceRNA network, the expression levels of 53 mRNAs and 261 lncRNAs decreased in SCLC and the expression levels of 19 miRNAs increased in SCLC, while the expression levels of 4 mRNAs and 40 lncRNAs increased in SCLC and the expression levels of 4 miRNAs decreased in SCLC (Supplemental Table 1). The circRNA-miRNA-mRNA ceRNA network consisted of 82 nodes (16 cirRNAs, 19 miRNAs and 47 mRNAs) with 165 edges (Figure 4). In the circRNA-miRNA-mRNA ceRNA network, the expression levels of 43 mRNAs and 11 circRNAs decreased in SCLC and the expression levels of 16 miRNAs increased in SCLC, while the expression levels of four mRNAs and five cirRNAs increased in SCLC and the expression levels of three miRNAs decreased in SCLC (Supplemental Table 2).
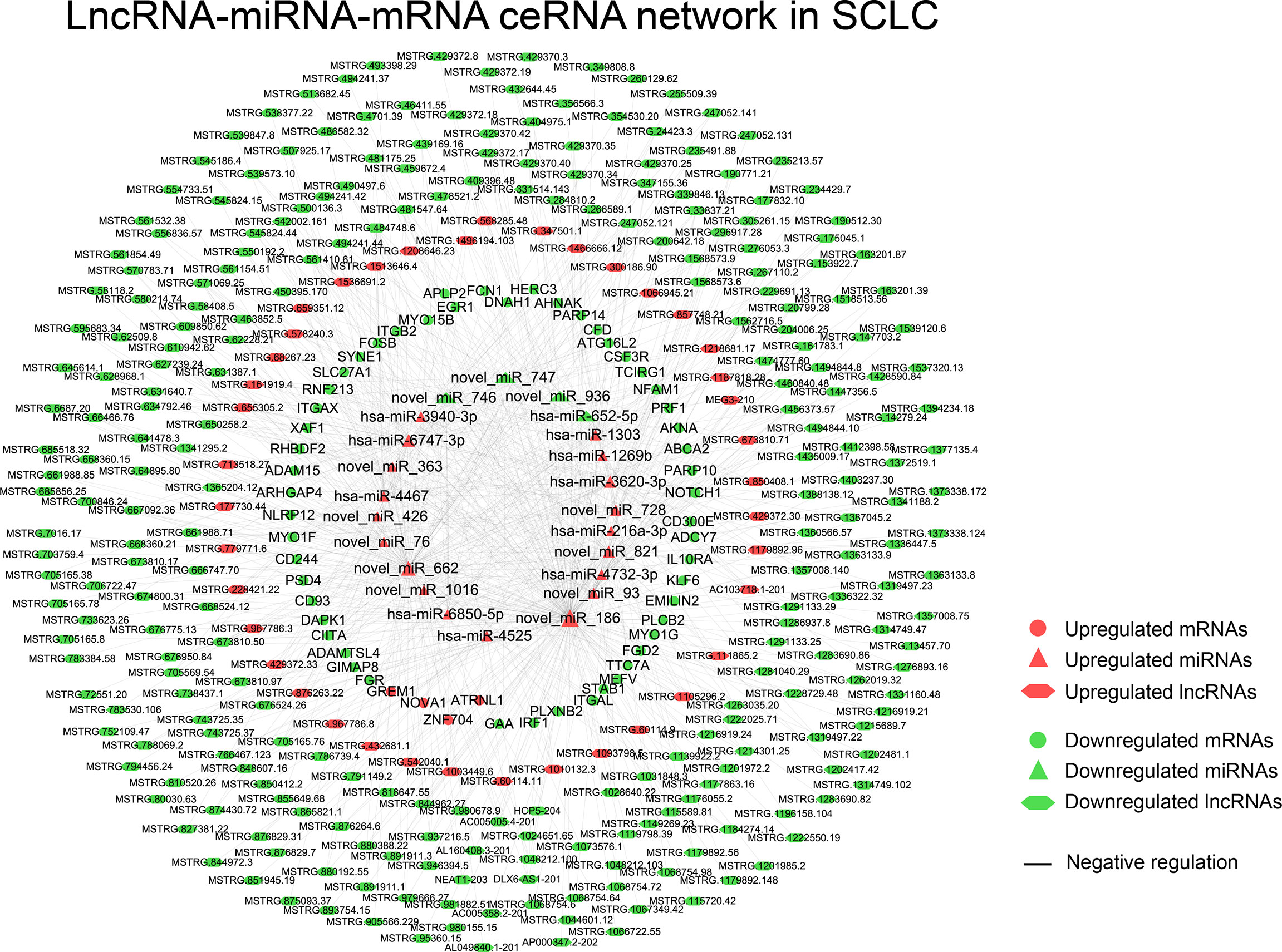
Figure 3 The lncRNA-miRNA-mRNA ceRNAs network in SCLC. lncRNA, long noncoding RNA; miRNA, microRNA; mRNA, messenger RNA; SCLC, small cell lung cancer.
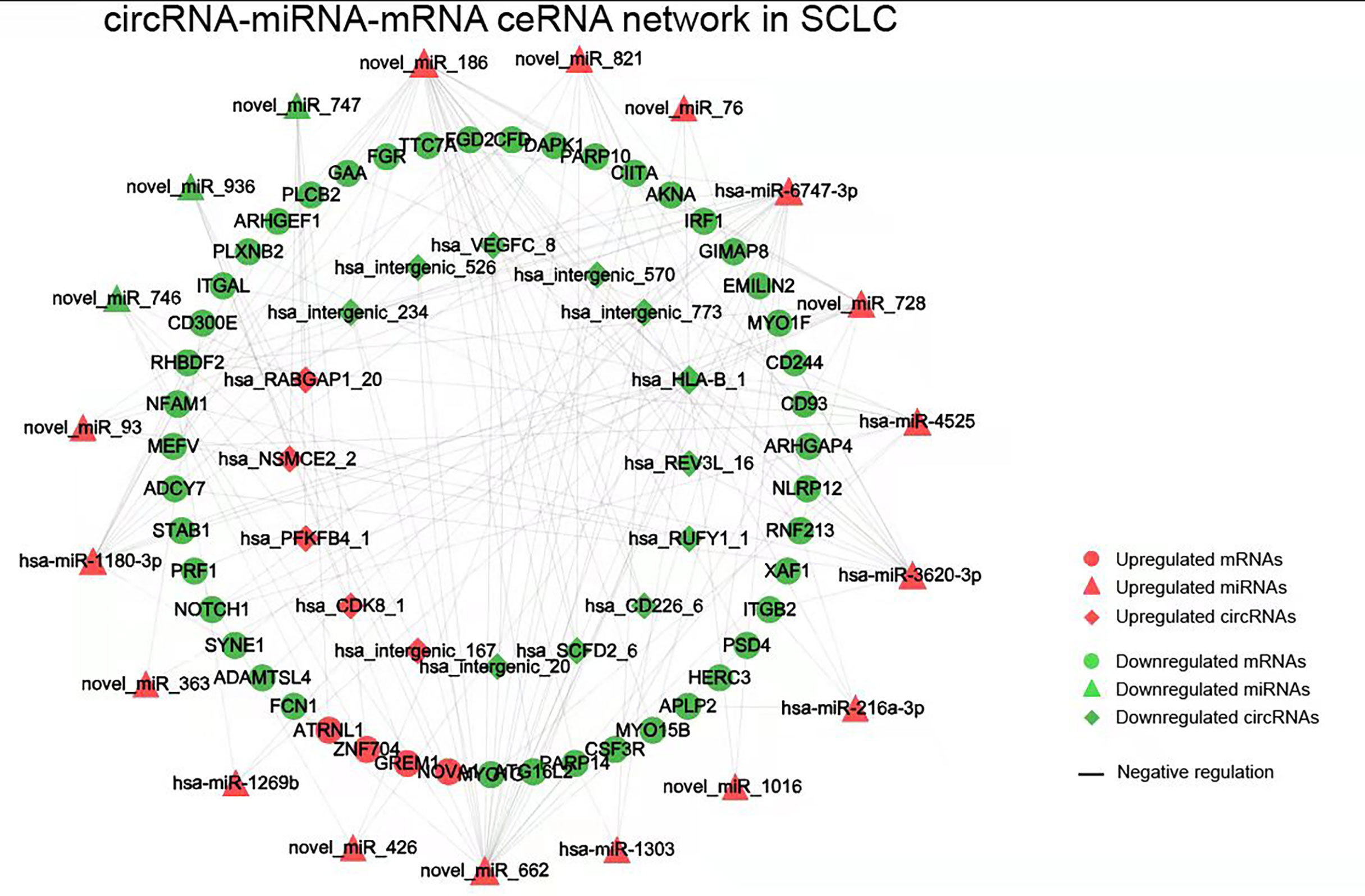
Figure 4 The circRNA-miRNA-mRNA ceRNAs network in SCLC. circRNA, circular RNA; miRNA, microRNA; mRNA, messenger RNA; SCLC, small cell lung cancer.
Functional Enrichment Analysis of mRNA, miRNA, circRNA and lncRNA in the ceRNA Network in SCLC
The differentially expressed levels of 58 mRNAs in the ceRNA network appear in Table 2. In the SCLC plasma cohort, the top three downregulated genes in the fold change (FC) were early growth response 1 (EGR1), complement factor D (CFD) and FosB proto-oncogene AP-1 transcription factor subunit (FOSB), while the top three upregulated genes in FC were zinc finger protein 704 (ZNF704), NOVA alternative splicing regulator 1 (NOVA1) and attractin like 1 (ATRNL1) (Table 2). Table 3 summarises 23 results from 58 mRNAs in the ceRNA network included in the GO analysis. This GO analysis indicated that the DEmRNAs were associated with numerous important biological processes and cellular components. The present study indicated that the biological processes of DEmRNAs primarily included processes such as neutrophil degranulation, neutrophil activation involved in the immune response, neutrophil activation, neutrophil-mediated immunity and an integrin-mediated signalling pathway among others. These biological functions associate with the protumour/prometastatic roles of inflammatory cells in cancer development and metastasis (Table 3) (52, 53). In terms of the cellular components, they mainly included the protein complex involved in cell adhesion and the integrin complex (Table 3), functions associated with tumorigenesis (54, 55). In addition, no results were obtained from the molecular function of the GO analysis and the KEGG pathways analysis, given that adjusted p > 0.05 in these functional analyses. In addition, we also reported the differentially expressed levels of lncRNAs, circRNAs and miRNAs in the ceRNA network (Supplemental Tables 3-5). The functional GO analyses primarily revealed cell survival and proliferation in 42 functional results from 301 lncRNAs, the inflammatory and immune response function in 32 functional results from 32 circRNAs and inflammatory and immune response and cell proliferation in 66 functional results from 24 miRNAs, respectively (Tables 4–6). Among these functions, many tumour-related terms were significantly enriched, such as regulating the cell cycle, the negative regulation of cell growth, DNA recombination and the MyD88-independent toll-like receptor signalling pathway, as well as the regulation of dendritic cell differentiation. In the KEGG pathways analyses, five pathways were identified in the lncRNAs, consisting of olfactory transduction, the neuroactive ligand–receptor interaction, nicotine addiction, carbohydrate digestion and absorption, and the protein digestion and absorption pathway (Table 4). The 60 pathways found in the miRNAs and mainly tumour-related pathways were significantly enriched, including the cAMP signalling pathway, focal adhesion, the MAPK signalling pathway, the Hippo signalling pathway and the ECM–receptor interaction (Table 7).
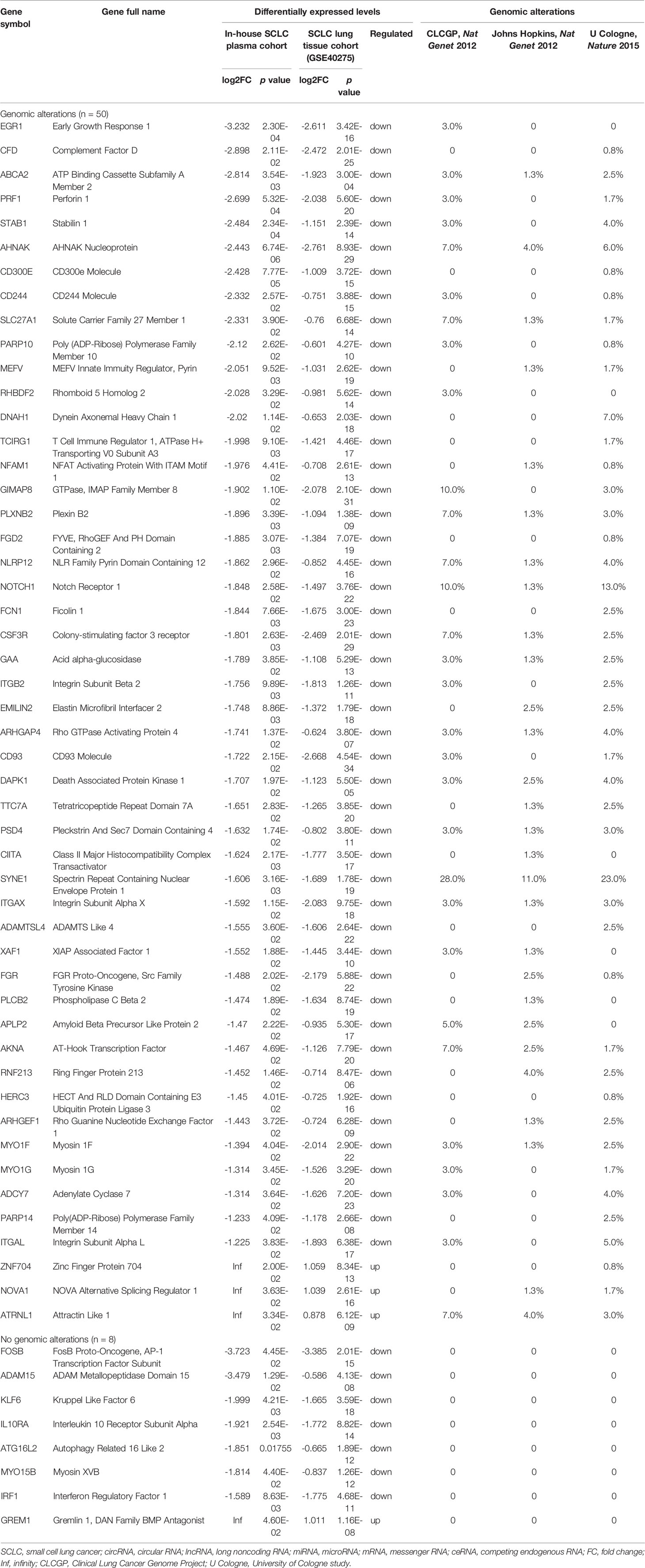
Table 2 Differentially expressed levels and genomic alterations of mRNAs in the ceRNA regulatory network in SCLC.
Evaluation of Genomic Alterations, Drug Candidates/Repurposing and Pathways in SCLC ceRNA Network
In total, 50 of 58 mRNAs in the ceRNA network presented genomic alterations, with the percentage of genomic alterations ranging from 0.8% to 28% (Table 2). The drug–target gene pharmacogenomics analysis showed that three [colony-stimulating factor 3 receptor (CSF3R) (alterations range 1.3–7.0%, FC (in plasma cohort): -1.801, p = 2.63 x 10-3), acid alpha-glucosidase (GAA) (alterations range 1.3–3.0%, FC: -1.789 and p = 3.85 x 10-2), FGR proto-oncogene Src family tyrosine kinase (FGR) (alterations range 0–2.5%, FC: -1.488, p = 2.02 x 10-2)] of 50 mRNAs in the ceRNA network were identified as potential drug targets (Tables 2 and 8). CSF3R and GAA were identified as targets of FavId and Trastuzumab deruxtecan, respectively, while FGR was confirmed as a target of Dasatinib and Zanubrutinib (Table 8). Next, the pathway analysis found that CSF3R, GAA and FGR were annotated in the 13 pathways in the Genecards database (Table 9). The SCLC-associated pathways were further identified through a literature review (56–58). We concluded that CSF3R was involved in the autophagy pathway and GAA was involved in the glucose metabolism pathway, while these two pathways were involved in SCLC occurrence and progression from the literature (Table 9) (56–58).
Identification of Multi-Omics Integration-Based Prioritisation of the ceRNA SCLC Network
The multi-omics integration-based prioritisation of the ceRNA regulatory network in SCLC consisted of two mRNAs, two miRNAs, three lncRNAs and two circRNAs (Figure 5). In this ceRNA network, the expression levels of mRNAs (CSF3R/GAA), lncRNAs (AC005005.4-201/DLX6-AS1-201/NEAT1-203) and circRNAs (hsa_HLA-B_1/hsa_VEGFC_8) decreased in SCLC, while the expression levels of miRNAs (hsa-miR-4525/hsa-miR-6747-3p) increased in SCLC. The primary regulatory axes in the ceRNA network were identified as follows: 1) lncRNA-miRNA-mRNA: AC005005.4-201/NEAT1-203-hsa-miR-6747-3p-CSF3R and DLX6-AS1-201-hsa-miR-4525-GAA; and 2) circRNA-miRNA-mRNA: hsa_HLA-B_1/hsa_VEGFC_8-hsa-miR-6747-3p-CSF3R and hsa_HLA-B_1-hsa-miR-4525-GAA (Figure 5). Thus, lncRNAs (lncRNA-AC005005.4-201 and NEAT1-203) and circRNAs (circRNA-hsa_HLA-B_1 and hsa_VEGFC_8) may regulate the inhibited effects of hsa-miR-6747-3p for CSF3R expression in SCLC, and lncRNA-DLX6-AS1-201 or circRNA-hsa_HLA-B_1 may neutralise the negative regulation of hsa-miR-4525 for GAA in SCLC.
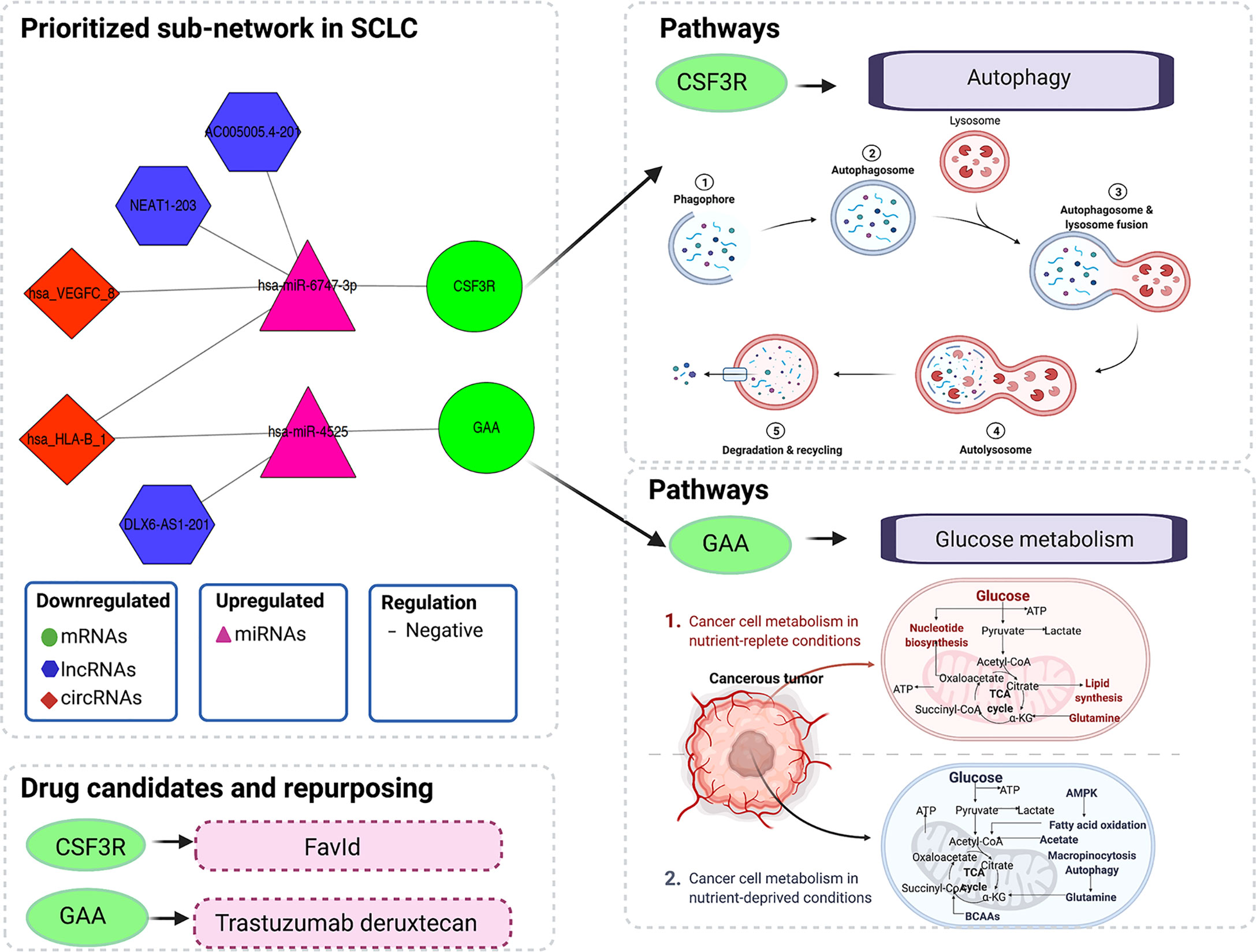
Figure 5 Illustration of multi-omics–based prioritisation of the ceRNA subnetwork, drug candidates and pathways. ATP, adenosine triphosphatase; AMPK, AMP-activated protein kinase; BCAAs, branched-chain amino acids; CoA, coenzyme A; ceRNA, competitive endogenous; RNA; circRNA, circular RNA; CSF3R, colony-stimulating factor 3 receptor; GAA, acid alpha-glucosidase; lncRNA, long noncoding RNA; miRNA, microRNA; mRNA, messenger RNA; SCLC, small cell lung cancer; TCA, tricarboxylic acid.
Discussion
Here, we integrated our own omics data (transcriptome and regulome) and public omics data (genome and pharmacogenome) to elucidate the multi-omics integration-based prioritisation of ceRNA-mediated network characteristics, pathways and drug candidates in SCLC. The prioritisation of the SCLC ceRNA regulatory network consisted of two mRNAs (CSF3R/GAA), two miRNAs (hsa-miR-4525/hsa-miR-6747-3p), three lncRNAs (AC005005.4-201/DLX6-AS1-201/NEAT1-203) and two circRNAs (hsa_HLA-B_1/hsa_VEGFC_8). The expression levels of mRNAs, lncRNAs and circRNAs decreased in SCLC, while the expression levels of miRNAs increased in SCLC. In addition, lncRNAs (lncRNA-AC005005.4-201 and NEAT1-203) and circRNAs (circRNA-hsa_HLA-B_1 and hsa_VEGFC_8) may regulate the inhibited effects of hsa-miR-6747-3p for CSF3R expression in SCLC, and lncRNA-DLX6-AS1-201 or circRNA-hsa_HLA-B_1 may neutralise the negative regulation of hsa-miR-4525 related to GAA in SCLC. The pharmacogenomics analysis identified CSF3R and GAA as targets of FavId and Trastuzumab deruxtecan, respectively. The SCLC-associated pathway analysis revealed that CSF3R was involved in the autophagy pathway, while GAA was involved in the glucose metabolism pathway. These findings may contribute to understanding the molecular pathogenesis of SCLC, supporting the development of novel diagnostics and therapeutic compounds for SCLC patients in clinical settings.
In this study, we first reported the multi-omics integration-based prioritisation of the lncRNA/circRNA-miRNA-mRNA ceRNA disease network, as well as the molecular characteristics and drug candidates or repurposed drugs in SCLC. The ceRNA is a layer of gene regulation in diseases, and the transcripts can regulate each other at the post-transcription level by competing for shared miRNAs (12, 16, 17). Here, we found that two lncRNAs (lncRNA-AC005005.4-201 and NEAT1-203) and two circRNAs (circRNA-hsa_HLA-B_1 and hsa_VEGFC_8) may regulate the inhibiting effects of hsa-miR-6747-3p for CSF3R expression, while lncRNA-DLX6-AS1-201 or circRNA-hsa_HLA-B_1 may neutralise the negative regulation of hsa-miR-4525 for GAA. Consistent with our findings for dysregulated lncRNAs in SCLC, previous studies found that lncRNAs DLX6-AS1 and NEAT1 were significantly dysregulated in non-SCLC, gastric cancer and pancreatic cancer (59–62). Specifically, upregulated DLX6-AS1 in gastric cancer tissue associated with distant metastasis and a poor clinical prognosis, while siRNA-DLX6-AS1 may inhibit gastric cancer cell proliferation, migration, invasion and the epithelial–mesenchymal transition in vitro (18). In addition, our study identified the regulatory axis in lncRNA-DLX6-AS1-201/hsa-miR-4525/GAA, which associated with the glucose metabolism pathway in SCLC. Interestingly, Qian et al. reported that sh-DLX6-AS1 may modulate glucose metabolism and cell growth via miR-4290/3-phosphoinositide-dependent protein kinase 1 in gastric cancer cells (63). Considering the role of DLX6-AS1 in glucose metabolism, we inferred that DLX6-AS1 could affect the occurrence and progression of SCLC via glucose metabolism through modulating hsa-miR-4525/GAA in SCLC. Similar to the other dysregulated lncRNA reports (59–62), Xu et al. found that lncRNA-NEAT1 may promote gastric cancer angiogenesis by enhancing the proliferation, migration and tube formation ability of endothelial cells through the miR-17-5p/transforming growth factor-β receptor 2 (TGFβR2) pathway (61), while lncRNA-NEAT1 may play a vital role in tumorigenesis and the development of SCLC through the hsa-miR-6747-3p/CSF3R axis. Importantly, in addition to lncRNA-DLX6-AS1 and NEAT1, we are the first to report another potential regulatory axis of ceRNA, while the regulatory mechanisms require further exploration through in vivo and in vitro studies. Our findings, however, suggest that the promising lncRNA/circRNA-miRNA-mRNA ceRNA regulatory characteristics in SCLC may provide new potential mechanisms and therapeutic targets.
To the best of our knowledge, this is also the first study to investigate the roles of CSF3R and GAA in the SCLC ceRNA regulation networks, pathways and drug candidates. CSF3R is a type 1 cytokine receptor, encoding the receptor for granulocyte colony-stimulating factor (G-CSF) and playing a crucial role in granulocyte proliferation and differentiation (64, 65). The altered CSF3R expression or activating heterozygous variants in CSF3R have been identified as risk factors in the development of multiple malignancies, such as colorectal cancer, myeloid malignancies and lymphoid malignancies (65–67). This is particularly the case for mutations in CSF3R commonly present in chronic neutrophilic leukaemia or atypical chronic myeloid leukaemia (68). Given the roles of CSF3R reported in chronic neutrophilic leukaemia or atypical chronic myeloid leukaemia (66, 68), our findings suggest that CSF3R might play a pivotal role in the occurrence and development of SCLC. Furthermore, our results suggest that CSF3R might modulate the autophagy pathway, which associated with SCLC (57, 58). The functions of autophagy in cancer may involve an anticancer or a cancer effect (69). Previous studies suggested that a hypoxia-HIF1A-AS2-autophagy interaction may play a role in drug sensitivity in SCLC, while a high expression of secreted phosphoprotein 1 (SPP1) inhibited autophagy and apoptosis, promoting the development of SCLC (57, 58). In addition, Rupniewska et al. found that SCLC cells may be more sensitive to autophagy inhibitors (70). In our study, CSF3R was identified as the potential drug target of FavId. FavId is an active immunotherapy with stimulating tumour-specific T cells and humoural immunity (71, 72). Alissafi et al. reported that autophagy-deficient therapy exhibited a mediated suppression of antitumour immunity via the efficient activation of tumour-specific CD4+ T cells (73), which was consistent with the mechanism of FavId in a tumour. Thus, our results suggest that genetic alterations or an altered expression of CSF3R may serve as a risk factor in SCLC development and associate with the autophagy pathway, while FavId could serve as a potential drug therapy through the CSF3R target to treat SCLC, even though additional in vivo or in vitro studies are needed to clarify these associations in SCLC. GAA, as one of the lysosomal enzymes, was the other key gene in our study. This is the first study to find that GAA might participant in the occurrence and development of SCLC via glucose metabolism. Similarly, Hamura et al. reported that the modulation of GAA could affect cell proliferation and apoptosis and manipulate chemoresistance in pancreatic cancer cells via malfunctional mitochondria (74). The dysregulated metabolism of glucose in mitochondria is known as an adverse microenvironment in solid tumours, referred to as the Warburg effect, including glucose deprivation and lactic acidosis, potentially resulting in an elevated glycolytic activity in tumour cells (75–78). Yan et al. showed that glucose metabolic reprogramming improves SCLC cell proliferation and metastasis, suggesting it could be a potential regulatory strategy interfering with glucose metabolism in SCLC (56). Considering the function of GAA, which catalyses the production of glucose from glycogen in lysosomes, altering the GAA expression or genetic status could inhibit tumorigenesis in SCLC through the lysosome pathway (56, 74–78). Interestingly, the DrugBank analysis showed that the drug targeting GAA was Trastuzumab-deruxtecan. Trastuzumab-deruxtecan is primarily used for patients with human epidermal growth factor receptor 2 (HER2)–mutant tumours including non-SCLC and in the absence of SCLC (79–81). Upon binding to HER2, Trastuzumab-deruxtecan disrupts the HER2 signalling, undergoes internalisation and intracellular linker cleavage by lysosomal enzymes and ultimately causes DNA damage and apoptotic cell death (80). In addition, Martinho et al. found that the inhibitors of the HER family (mainly HER2) reduced cervical cancer aggressiveness by blocking glucose metabolism (82). Combined with the roles of the glucose metabolism pathway in SCLC and the antitumour roles of Trastuzumab-deruxtecan via the glucose metabolism pathway, our findings suggest that Trastuzumab-deruxtecan may be a promising drug candidate via GAA in SCLC through the glucose metabolism pathway. However, further in vivo or in vitro studies are needed to clarify these promising drug candidates’ ability to treat SCLC.
The strength of this study is our use of network-based multi-omics integration to prioritise ceRNA characteristics and drug candidates in SCLC from two well-characterised study cohorts, including newly tested whole-transcriptome sequencing data in the SCLC study, and the data were uploaded to a public platform [the Sequence Read Archive (SRA) database]. In addition to these strengths, we also note several limitations. First, our study included our own omics data and public data. In addition, the relatively small size of our cohort represents a limitation to our findings, although the results of the mRNA study were validated in a relatively large cohort. Second, the ceRNA characteristics and drug candidates and repurposing are quite promising, although further mechanistic studies from cells and animal models, as well as clinical validation studies, are needed. In addition, we performed no survival analysis in this study, since no available and suitable survival data were obtained from public databases, including the Cancer Genome Atlas (TCGA) and Kaplan–Meier plotter databases. Finally, the survival data in our SCLC plasma cohort were incapable of producing useful results for the prognostic analysis given the relatively small sample sizes and quite limited follow-up time.
In conclusion, we report primary findings related to a multi-omics integration-based prioritisation of the lncRNA/circRNA-miRNA-mRNA ceRNA regulatory network, pathways and promising drug candidates in SCLC. These findings indicate novel, potential diagnostic and therapeutic targets in SCLC.
Data Availability Statement
The datasets presented in this study can be found in online repositories. The names of the repository/repositories and accession number(s) can be found in the article/Supplementary Material.
Ethics Statement
This study received ethical approval from the Ethics Committee of the Gansu Provincial Hospital, China (27 July 2020, No. 2020-183). The patients/participants provided their written informed consent to participate in this study.
Author Contributions
W-DH, MZ, X-JW and JG contributed to the design of the study. X-JW and W-DH performed the sample collection, analysis and downloaded the data. X-JW and JG contributed to the data analysis and to writing the manuscript. W-DH, MZ, QY, X-JW and JG revised the manuscript. All authors approved the final version of the manuscript.
Funding
This study was supported by the Science–Technology Foundation for Young Scientist of the Gansu Province of China (Grant no.18JR3RA059), the Science–Technology Foundation for Scientists of the Gansu Province of China (Grant no.21JR7RA595), the Science–Technology Foundation for Lanzhou City of China (Grant no.2018-4-65) and the Scientists Fund of the Gansu Provincial Hospital of China (Grant no.18GSS4-25). Jing Gao was also supported by the Swedish Heart–Lung Foundation, the Swedish Asthma and Allergy Foundation, the Sigrid Jusélius Foundation and the Väinö and Laina Kivi Foundation.
Conflict of Interest
The authors declare that the research was conducted in the absence of any commercial or financial relationships that could be construed as a potential conflict of interest.
Publisher’s Note
All claims expressed in this article are solely those of the authors and do not necessarily represent those of their affiliated organizations, or those of the publisher, the editors and the reviewers. Any product that may be evaluated in this article, or claim that may be made by its manufacturer, is not guaranteed or endorsed by the publisher.
Acknowledgments
We extend our deepest gratitude to all of the patients who volunteered to participate in our study. We thank Jin Li, from the Faculty of Information Technology and Communication Sciences, Tampere University (Finland), for assistance with the tables and figures. We also extend our gratitude to Vanessa L Fuller, from Language Services at the University of Helsinki (Finland), for assistance with the initial English-language revision of this manuscript. In addition, we thank the Biomarker Technologies Corporation (Beijing, China) for sequencing technology and support. Figures were created using the BioRender software (©biorender.com).
Supplementary Material
The Supplementary Material for this article can be found online at: https://www.frontiersin.org/articles/10.3389/fonc.2022.904865/full#supplementary-material.
Supplementary Table 1 | The lncRNAs, miRNAs and mRNAs in the lncRNA-miRNA-mRNA ceRNA network.
Supplementary Table 2 | The circRNAs, miRNAs and mRNAs in the circRNA-miRNA-mRNA ceRNA network.
Supplementary Table 3 | Differentially expressed levels of 301 lncRNAs in the ceRNA network.
Supplementary Table 4 | Differentially expressed levels of 16 circRNAs in the ceRNA network.
Supplementary Table 5 | Differentially expressed levels of 24 miRNAs in the ceRNA network.
Supplemental File 1 | The whole-transcriptome sequencing process.
Supplemental File 2 | Data cleaning process.
References
1. Byers LA, Rudin CM. Small Cell Lung Cancer: Where do We Go From Here? Cancer Am Cancer Soc (2015) 121(5):664–72. doi: 10.1002/cncr.29098
2. Bernhardt EB, Jalal SI. Small Cell Lung Cancer. Cancer Treat Res (2016) 170:301–22. doi: 10.1007/978-3-319-40389-2_14
3. Wang Y, Zou S, Zhao Z, Liu P, Ke C, Xu S. New Insights Into Small-Cell Lung Cancer Development and Therapy. Cell Biol Int (2020) 44(8):1564–76. doi: 10.1002/cbin.11359
4. Johal S, Hettle R, Carroll J, Maguire P, Wynne T. Real-World Treatment Patterns and Outcomes in Small-Cell Lung Cancer: A Systematic Literature Review. J Thorac Dis (2021) 13(6):3692–707. doi: 10.21037/jtd-20-3034
5. Demedts IK, Vermaelen KY, van Meerbeeck JP. Treatment of Extensive-Stage Small Cell Lung Carcinoma: Current Status and Future Prospects. Eur Respir J (2010) 35(1):202–15. doi: 10.1183/09031936.00105009
6. Stinchcombe TE, Gore EM. Limited-Stage Small Cell Lung Cancer: Current Chemoradiotherapy Treatment Paradigms. Oncologist (2010) 15(2):187–95. doi: 10.1634/theoncologist.2009-0298
7. Yang S, Zhang Z, Wang Q. Emerging Therapies for Small Cell Lung Cancer. J Hematol Oncol (2019) 12(1):47. doi: 10.1186/s13045-019-0736-3
8. Koinis F, Kotsakis A, Georgoulias V. Small Cell Lung Cancer (SCLC): No Treatment Advances in Recent Years. Transl Lung Cancer Res (2016) 5(1):39–50. doi: 10.3978/j.issn.2218-6751.2016.01.03
9. Rudin CM, Awad MM, Navarro A, Gottfried M, Peters S, Csoszi T, et al. Pembrolizumab or Placebo Plus Etoposide and Platinum as First-Line Therapy for Extensive-Stage Small-Cell Lung Cancer: Randomized, Double-Blind, Phase III KEYNOTE-604 Study. J Clin Oncol (2020) 38(21):2369–79. doi: 10.1200/JCO.20.00793
10. Hiddinga BI, Raskin J, Janssens A, Pauwels P, Van Meerbeeck JP. Recent Developments in the Treatment of Small Cell Lung Cancer. Eur Respir Rev (2021) 30(161):210079. doi: 10.1183/16000617.0079-2021
11. Amini A, Byers LA, Welsh JW, Komaki RU. Progress in the Management of Limited-Stage Small Cell Lung Cancer. Cancer Am Cancer Soc (2014) 120(6):790–8. doi: 10.1002/cncr.28505
12. Qi X, Zhang DH, Wu N, Xiao JH, Wang X, Ma W. ceRNA in Cancer: Possible Functions and Clinical Implications. J Med Genet (2015) 52(10):710–8. doi: 10.1136/jmedgenet-2015-103334
13. Matsui M, Corey DR. Non-Coding RNAs as Drug Targets. Nat Rev Drug Discov (2017) 16(3):167–79. doi: 10.1038/nrd.2016.117
14. Slack FJ, Chinnaiyan AM. The Role of Non-Coding RNAs in Oncology. Cell (2019) 179(5):1033–55. doi: 10.1016/j.cell.2019.10.017
15. Ghafouri-Fard S, Shoorei H, Branicki W, Taheri M. Non-Coding RNA Profile in Lung Cancer. Exp Mol Pathol (2020) 114:104411. doi: 10.1016/j.yexmp.2020.104411
16. Salmena L, Poliseno L, Tay Y, Kats L, Pandolfi PP. A ceRNA Hypothesis: The Rosetta Stone of a Hidden RNA Language? Cell (2011) 146(3):353–8. doi: 10.1016/j.cell.2011.07.014
17. Zhou RS, Zhang EX, Sun QF, Ye ZJ, Liu JW, Zhou DH, et al. Integrated Analysis of lncRNA-miRNA-mRNA ceRNA Network in Squamous Cell Carcinoma of Tongue. BMC Cancer (2019) 19(1):779. doi: 10.1186/s12885-019-5983-8
18. Liang Y, Zhang CD, Zhang C, Dai DQ. DLX6-AS1/miR-204-5p/OCT1 Positive Feedback Loop Promotes Tumor Progression and Epithelial-Mesenchymal Transition in Gastric Cancer. Gastric Cancer (2020) 23(2):212–27. doi: 10.1007/s10120-019-01002-1
19. Di Leva G, Garofalo M, Croce CM. MicroRNAs in Cancer. Annu Rev Pathol (2014) 9:287–314. doi: 10.1146/annurev-pathol-012513-104715
20. Azizi M, Othman I, Naidu R. The Role of MicroRNAs in Lung Cancer Metabolism. Cancers (Basel) (2021) 13(7):1716. doi: 10.3390/cancers13071716
21. Wang XJ, Gao J, Wang Z, Yu Q. Identification of a Potentially Functional microRNA-mRNA Regulatory Network in Lung Adenocarcinoma Using a Bioinformatics Analysis. Front Cell Dev Biol (2021) 9:641840. doi: 10.3389/fcell.2021.641840
22. Wu T, Du Y. LncRNAs: From Basic Research to Medical Application. Int J Biol Sci (2017) 13(3):295–307. doi: 10.7150/ijbs.16968
23. Kumar S, Pandey M, Sharawat SK. Biological Functions of Long Noncoding RNAs and Circular RNAs in Small-Cell Lung Cancer. Epigenomics-Uk (2020) 12(19):1751–63. doi: 10.2217/epi-2020-0214
24. Barrett SP, Wang PL, Salzman J. Circular RNA Biogenesis can Proceed Through an Exon-Containing Lariat Precursor. Elife (2015) 4:e7540. doi: 10.7554/eLife.07540
25. Stewart PA, Welsh EA, Slebos R, Fang B, Izumi V, Chambers M, et al. Proteogenomic Landscape of Squamous Cell Lung Cancer. Nat Commun (2019) 10(1):3578. doi: 10.1038/s41467-019-11452-x
26. Gong L, Zhang D, Dong Y, Lei Y, Qian Y, Tan X, et al. Integrated Bioinformatics Analysis for Identificating the Therapeutic Targets of Aspirin in Small Cell Lung Cancer. J BioMed Inform (2018) 88:20–8. doi: 10.1016/j.jbi.2018.11.001
27. Kuenzi BM, Ideker T. A Census of Pathway Maps in Cancer Systems Biology. Nat Rev Cancer (2020) 20(4):233–46. doi: 10.1038/s41568-020-0240-7
28. Chakraborty S, Andrieux G, Hasan A, Ahmed M, Hosen MI, Rahman T, et al. Harnessing the Tissue and Plasma lncRNA-Peptidome to Discover Peptide-Based Cancer Biomarkers. Sci Rep (2019) 9(1):12322. doi: 10.1038/s41598-019-48774-1
29. Cojocneanu R, Braicu C, Raduly L, Jurj A, Zanoaga O, Magdo L, et al. Plasma and Tissue Specific miRNA Expression Pattern and Functional Analysis Associated to Colorectal Cancer Patients. Cancers (Basel) (2020) 12(4):843. doi: 10.3390/cancers12040843
30. Chen X, Li C, Li Y, Wu S, Liu W, Lin T, et al. Characterization of METTL7B to Evaluate TME and Predict Prognosis by Integrative Analysis of Multi-Omics Data in Glioma. Front Mol Biosci (2021) 8:727481. doi: 10.3389/fmolb.2021.727481
31. Lu Y, Jin J, Du Q, Hu M, Wei Y, Wang M, et al. Multi-Omics Analysis of the Anti-Tumor Synergistic Mechanism and Potential Application of Immune Checkpoint Blockade Combined With Lenvatinib. Front Cell Dev Biol (2021) 9:730240. doi: 10.3389/fcell.2021.730240
32. Ponzi E, Thoresen M, Haugdahl NT, Mollersen K. Integrative, Multi-Omics, Analysis of Blood Samples Improves Model Predictions: Applications to Cancer. BMC Bioinf (2021) 22(1):395. doi: 10.1186/s12859-021-04296-0
33. Kastner S, Voss T, Keuerleber S, Glockel C, Freissmuth M, Sommergruber W. Expression of G Protein-Coupled Receptor 19 in Human Lung Cancer Cells is Triggered by Entry Into S-Phase and Supports G(2)-M Cell-Cycle Progression. Mol Cancer Res (2012) 10(10):1343–58. doi: 10.1158/1541-7786.MCR-12-0139
34. Wang L, Meng L, Wang XW, Ma GY, Chen JH. Expression of RRM1 and RRM2 as a Novel Prognostic Marker in Advanced non-Small Cell Lung Cancer Receiving Chemotherapy. Tumour Biol (2014) 35(3):1899–906. doi: 10.1007/s13277-013-1255-4
35. Li L, Zhang L, Tian Y, Zhang T, Duan G, Liu Y, et al. Serum Chemokine CXCL7 as a Diagnostic Biomarker for Colorectal Cancer. Front Oncol (2019) 9:921. doi: 10.3389/fonc.2019.00921
36. Feser WJ, Fingerlin TE, Strand MJ, Glueck DH. Calculating Average Power for the Benjamini-Hochberg Procedure. J Stat Theory Appl (2009) 8(3):325–52.
37. Kozomara A, Birgaoanu M, Griffiths-Jones S. Mirbase: From microRNA Sequences to Function. Nucleic Acids Res (2019) 47(D1):D155–62. doi: 10.1093/nar/gky1141
38. Lewis BP, Shih IH, Jones-Rhoades MW, Bartel DP, Burge CB. Prediction of Mammalian microRNA Targets. Cell (2003) 115(7):787–98. doi: 10.1016/s0092-8674(03)01018-3
39. Rehmsmeier M, Steffen P, Hochsmann M, Giegerich R. Fast and Effective Prediction of microRNA/Target Duplexes. Rna (2004) 10(10):1507–17. doi: 10.1261/rna.5248604
40. Betel D, Wilson M, Gabow A, Marks DS, Sander C. The microRNA.Org Resource: Targets and Expression. Nucleic Acids Res (2008) 36(Database issue):D149–53. doi: 10.1093/nar/gkm995
41. Pertea G, Pertea M. GFF Utilities: GffRead and GffCompare. F1000Res (2020) 9:ISCB Comm J–304. doi: 10.12688/f1000research.23297.2
42. Gao Y, Wang J, Zhao F. CIRI: An Efficient and Unbiased Algorithm for De Novo Circular RNA Identification. Genome Biol (2015) 16:4. doi: 10.1186/s13059-014-0571-3
43. Friedlander MR, Mackowiak SD, Li N, Chen W, Rajewsky N. Mirdeep2 Accurately Identifies Known and Hundreds of Novel microRNA Genes in Seven Animal Clades. Nucleic Acids Res (2012) 40(1):37–52. doi: 10.1093/nar/gkr688
44. Shannon P, Markiel A, Ozier O, Baliga NS, Wang JT, Ramage D, et al. Cytoscape: A Software Environment for Integrated Models of Biomolecular Interaction Networks. Genome Res (2003) 13(11):2498–504. doi: 10.1101/gr.1239303
45. Yu G, Wang LG, Han Y, He QY. Clusterprofiler: An R Package for Comparing Biological Themes Among Gene Clusters. Omics (2012) 16(5):284–7. doi: 10.1089/omi.2011.0118
46. Yu K, Kuang L, Fu T, Zhang C, Zhou Y, Zhu C, et al. CREM Is Correlated With Immune-Suppressive Microenvironment and Predicts Poor Prognosis in Gastric Adenocarcinoma. Front Cell Dev Biol (2021) 9:697748. doi: 10.3389/fcell.2021.697748
47. Peifer M, Fernandez-Cuesta L, Sos ML, George J, Seidel D, Kasper LH, et al. Integrative Genome Analyses Identify Key Somatic Driver Mutations of Small-Cell Lung Cancer. Nat Genet (2012) 44(10):1104–10. doi: 10.1038/ng.2396
48. Rudin CM, Durinck S, Stawiski EW, Poirier JT, Modrusan Z, Shames DS, et al. Comprehensive Genomic Analysis Identifies SOX2 as a Frequently Amplified Gene in Small-Cell Lung Cancer. Nat Genet (2012) 44(10):1111–6. doi: 10.1038/ng.2405
49. George J, Lim JS, Jang SJ, Cun Y, Ozretic L, Kong G, et al. Comprehensive Genomic Profiles of Small Cell Lung Cancer. Nature (2015) 524(7563):47–53. doi: 10.1038/nature14664
50. Wishart DS, Feunang YD, Guo AC, Lo EJ, Marcu A, Grant JR, et al. DrugBank 5.0: A Major Update to the DrugBank Database for 2018. Nucleic Acids Res (2018) 46(D1):D1074–82. doi: 10.1093/nar/gkx1037
51. Wang Y, Yang R, Yan F, Jin Y, Liu X, Wang T. Medicarpin Protects Cerebral Microvascular Endothelial Cells Against Oxygen-Glucose Deprivation/Reoxygenation-Induced Injury via the PI3K/Akt/FoxO Pathway: A Study of Network Pharmacology Analysis and Experimental Validation. Neurochem Res (2022) 47(2):347–57. doi: 10.1007/s11064-021-03449-0
52. Wu M, Ma M, Tan Z, Zheng H, Liu X. Neutrophil: A New Player in Metastatic Cancers. Front Immunol (2020) 11:565165. doi: 10.3389/fimmu.2020.565165
53. Rawat K, Syeda S, Shrivastava A. Neutrophil-Derived Granule Cargoes: Paving the Way for Tumor Growth and Progression. Cancer Metastasis Rev (2021) 40(1):221–44. doi: 10.1007/s10555-020-09951-1
54. Hou S, Wang J, Li W, Hao X, Hang Q. Roles of Integrins in Gastrointestinal Cancer Metastasis. Front Mol Biosci (2021) 8:708779. doi: 10.3389/fmolb.2021.708779
55. Roehrig AE, Klupsch K, Oses-Prieto JA, Chaib S, Henderson S, Emmett W, et al. Cell-Cell Adhesion Regulates Merlin/NF2 Interaction With the PAF Complex. PLoS One (2021) 16(8):e254697. doi: 10.1371/journal.pone.0254697
56. Yan X, Li F, Dozmorov I, Frank MB, Dao M, Centola M, et al. External Qi of Yan Xin Qigong Induces Cell Death and Gene Expression Alterations Promoting Apoptosis and Inhibiting Proliferation, Migration and Glucose Metabolism in Small-Cell Lung Cancer Cells. Mol Cell Biochem (2012) 363(1-2):245–55. doi: 10.1007/s11010-011-1176-8
57. Liu H, Wei S, Zhang L, Yuan C, Duan Y, Wang Q. Secreted Phosphoprotein 1 Promotes the Development of Small Cell Lung Cancer Cells by Inhibiting Autophagy and Apoptosis. Pathol Oncol Res (2019) 25(4):1487–95. doi: 10.1007/s12253-018-0504-7
58. Guclu E, Eroglu GC, Kurar E, Vural H. Knockdown of lncRNA HIF1A-AS2 Increases Drug Sensitivity of SCLC Cells in Association With Autophagy. Med Oncol (2021) 38(9):113. doi: 10.1007/s12032-021-01562-2
59. Fu X, Deng X, Xiao W, Huang B, Yi X, Zou Y. Downregulation of NEAT1 Sensitizes Gemcitabine-Resistant Pancreatic Cancer Cells to Gemcitabine Through Modulation of the miR-506-3p/ZEB2/EMT Axis. Am J Cancer Res (2021) 11(8):3841–56. doi: 10.1186/s13059-014-0571-3
60. Li K, Yao T, Zhang Y, Li W, Wang Z. NEAT1 as a Competing Endogenous RNA in Tumorigenesis of Various Cancers: Role, Mechanism and Therapeutic Potential. Int J Biol Sci (2021) 17(13):3428–40. doi: 10.7150/ijbs.62728
61. Xu Y, Li Y, Qiu Y, Sun F, Zhu G, Sun J, et al. LncRNA NEAT1 Promotes Gastric Cancer Progression Through miR-17-5p/TGFbetaR2 Axis Up-Regulated Angiogenesis. Front Cell Dev Biol (2021) 9:705697. doi: 10.3389/fcell.2021.705697
62. Zheng Q, Gu X, Yang Q, Chu Q, Dai Y, Chen Z. DLX6-AS1 is a Potential Biomarker and Therapeutic Target in Cancer Initiation and Progression. Clin Chim Acta (2021) 517:1–8. doi: 10.1016/j.cca.2021.02.006
63. Qian Y, Song W, Wu X, Hou G, Wang H, Hang X, et al. DLX6 Antisense RNA 1 Modulates Glucose Metabolism and Cell Growth in Gastric Cancer by Targeting microRNA-4290. Dig Dis Sci (2021) 66(2):460–73. doi: 10.1007/s10620-020-06223-4
64. Touw IP, van de Geijn GJ. Granulocyte Colony-Stimulating Factor and its Receptor in Normal Myeloid Cell Development, Leukemia and Related Blood Cell Disorders. Front Biosci (2007) 12:800–15. doi: 10.2741/2103
65. Trottier AM, Druhan LJ, Kraft IL, Lance A, Feurstein S, Helgeson M, et al. Heterozygous Germ Line CSF3R Variants as Risk Alleles for Development of Hematologic Malignancies. Blood Adv (2020) 4(20):5269–84. doi: 10.1182/bloodadvances.2020002013
66. Rashid M, Alasiri A, Al BM, Alkhaldi A, Alsuhaibani A, Alsultan A, et al. Identification of CSF3R Mutations in B-Lineage Acute Lymphoblastic Leukemia Using Comprehensive Cancer Panel and Next-Generation Sequencing. Genes (Basel) (2021) 12(9):1326. doi: 10.3390/genes12091326
67. Saunders AS, Bender DE, Ray AL, Wu X, Morris KT. Colony-Stimulating Factor 3 Signaling in Colon and Rectal Cancers: Immune Response and CMS Classification in TCGA Data. PLoS One (2021) 16(2):e247233. doi: 10.1371/journal.pone.0247233
68. Maxson JE, Gotlib J, Pollyea DA, Fleischman AG, Agarwal A, Eide CA, et al. Oncogenic CSF3R Mutations in Chronic Neutrophilic Leukemia and Atypical CML. N Engl J Med (2013) 368(19):1781–90. doi: 10.1056/NEJMoa1214514
69. Wang Z, Zhou C, Yang S. The Roles, Controversies, and Combination Therapies of Autophagy in Lung Cancer. Cell Biol Int (2022) 46(1):3–11. doi: 10.1002/cbin.11704
70. Rupniewska E, Roy R, Mauri FA, Liu X, Kaliszczak M, Bellezza G, et al. Targeting Autophagy Sensitises Lung Cancer Cells to Src Family Kinase Inhibitors. Oncotarget (2018) 9(44):27346–62. doi: 10.18632/oncotarget.25213
71. Hurvitz SA, Timmerman JM. Recombinant, Tumour-Derived Idiotype Vaccination for Indolent B Cell non-Hodgkin's Lymphomas: A Focus on FavId. Expert Opin Biol Ther (2005) 5(6):841–52. doi: 10.1517/14712598.5.6.841
72. Reinis M. Drug Evaluation: FavId, a Patient-Specific Idiotypic Vaccine for non-Hodgkin's Lymphoma. Curr Opin Mol Ther (2007) 9(3):291–8.
73. Alissafi T, Hatzioannou A, Mintzas K, Barouni RM, Banos A, Sormendi S, et al. Autophagy Orchestrates the Regulatory Program of Tumor-Associated Myeloid-Derived Suppressor Cells. J Clin Invest (2018) 128(9):3840–52. doi: 10.1172/JCI120888
74. Hamura R, Shirai Y, Shimada Y, Saito N, Taniai T, Horiuchi T, et al. Suppression of Lysosomal Acid Alpha-Glucosidase Impacts the Modulation of Transcription Factor EB Translocation in Pancreatic Cancer. Cancer Sci (2021) 112(6):2335–48. doi: 10.1111/cas.14921
75. Vanhove K, Graulus GJ, Mesotten L, Thomeer M, Derveaux E, Noben JP, et al. The Metabolic Landscape of Lung Cancer: New Insights in a Disturbed Glucose Metabolism. Front Oncol (2019) 9:1215. doi: 10.3389/fonc.2019.01215
76. Chisari A, Golan I, Campisano S, Gelabert C, Moustakas A, Sancho P, et al. Glucose and Amino Acid Metabolic Dependencies Linked to Stemness and Metastasis in Different Aggressive Cancer Types. Front Pharmacol (2021) 12:723798. doi: 10.3389/fphar.2021.723798
77. Qi X, Li Q, Che X, Wang Q, Wu G. The Uniqueness of Clear Cell Renal Cell Carcinoma: Summary of the Process and Abnormality of Glucose Metabolism and Lipid Metabolism in ccRCC. Front Oncol (2021) 11:727778. doi: 10.3389/fonc.2021.727778
78. Shin E, Koo JS. Glucose Metabolism and Glucose Transporters in Breast Cancer. Front Cell Dev Biol (2021) 9:728759. doi: 10.3389/fcell.2021.728759
79. Grieb BC, Agarwal R. HER2-Directed Therapy in Advanced Gastric and Gastroesophageal Adenocarcinoma: Triumphs and Troubles. Curr Treat Options Oncol (2021) 22(10):88. doi: 10.1007/s11864-021-00884-7
80. Indini A, Rijavec E, Grossi F. Trastuzumab Deruxtecan: Changing the Destiny of HER2 Expressing Solid Tumors. Int J Mol Sci (2021) 22(9):4774. doi: 10.3390/ijms22094774
81. Riudavets M, Sullivan I, Abdayem P, Planchard D. Targeting HER2 in non-Small-Cell Lung Cancer (NSCLC): A Glimpse of Hope? An Updated Review on Therapeutic Strategies in NSCLC Harbouring HER2 Alterations. ESMO Open (2021) 6(5):100260. doi: 10.1016/j.esmoop.2021.100260
82. Martinho O, Silva-Oliveira R, Cury FP, Barbosa AM, Granja S, Evangelista AF, et al. HER Family Receptors are Important Theranostic Biomarkers for Cervical Cancer: Blocking Glucose Metabolism Enhances the Therapeutic Effect of HER Inhibitors. Theranostics (2017) 7(3):717–32. doi: 10.7150/thno.17154
Keywords: small cell lung cancer (SCLC), multi-omics integration, competing endogenous RNA (ceRNA), long noncoding RNA (lncRNA), circular RNA (circRNA), microRNA (miRNA)
Citation: Wang X-J, Gao J, Yu Q, Zhang M and Hu W-D (2022) Multi-Omics Integration-Based Prioritisation of Competing Endogenous RNA Regulation Networks in Small Cell Lung Cancer: Molecular Characteristics and Drug Candidates. Front. Oncol. 12:904865. doi: 10.3389/fonc.2022.904865
Received: 26 March 2022; Accepted: 02 June 2022;
Published: 04 July 2022.
Edited by:
Tianyi Zhao, Harbin Institute of Technology, ChinaCopyright © 2022 Wang, Gao, Yu, Zhang and Hu. This is an open-access article distributed under the terms of the Creative Commons Attribution License (CC BY). The use, distribution or reproduction in other forums is permitted, provided the original author(s) and the copyright owner(s) are credited and that the original publication in this journal is cited, in accordance with accepted academic practice. No use, distribution or reproduction is permitted which does not comply with these terms.
*Correspondence: Wei-Dong Hu, weidong_618@163.com; Min Zhang, sallyzhangmin@126.com; Jing Gao, jing.gao@helsinki.fi
†These authors have contributed equally to this work