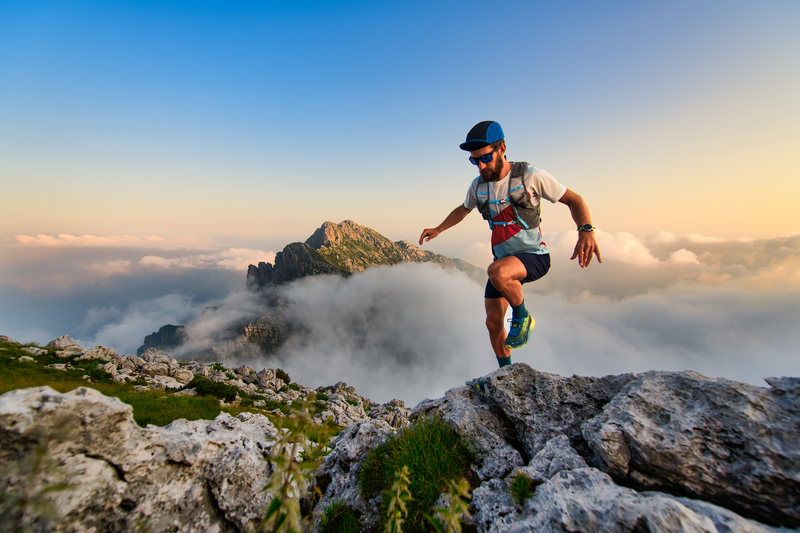
94% of researchers rate our articles as excellent or good
Learn more about the work of our research integrity team to safeguard the quality of each article we publish.
Find out more
MINI REVIEW article
Front. Oncol. , 10 May 2022
Sec. Genitourinary Oncology
Volume 12 - 2022 | https://doi.org/10.3389/fonc.2022.889686
This article is part of the Research Topic In Vitro and In Vivo Non-Clinical Models of Kidney Cancers View all 8 articles
Renal cell carcinoma (RCC) is among the most lethal urological malignancies once metastatic. The introduction of immune checkpoint inhibitors has revolutionized the therapeutic landscape of metastatic RCC, nevertheless, a significant proportion of patients will experience disease progression. Novel treatment options are therefore still needed and in vitro and in vivo model systems are crucial to ultimately improve disease control. At the same time, RCC is characterized by a number of molecular and functional peculiarities that have the potential to limit the utility of pre-clinical model systems. This includes not only the well-known genomic intratumoral heterogeneity (ITH) of RCC but also a remarkable functional ITH that can be shaped by influences of the tumor microenvironment. Importantly, RCC is among the tumor entities, in which a high number of intratumoral cytotoxic T cells is associated with a poor prognosis. In fact, many of these T cells are exhausted, which represents a major challenge for modeling tumor-immune cell interactions. Lastly, pre-clinical drug development commonly relies on using phenotypic screening of 2D or 3D RCC cell culture models, however, the problem of “reverse engineering” can prevent the identification of the precise mode of action of drug candidates thus impeding their translation to the clinic. In conclusion, a holistic approach to model the complex “ecosystem RCC” will likely require not only a combination of model systems but also an integration of concepts and methods using artificial intelligence to further improve pre-clinical drug discovery.
Metastatic renal cell carcinoma (RCC) is among the most lethal urological malignancies (1, 2). These tumors are largely resistant to conventional chemo- or radiotherapy but vulnerable to immune modulatory treatment and agents that target the VEGF/VEGFR axis (3). The notion that spontaneous remissions can occur in RCC has spurred efforts to harness the patient’s antitumoral immune response (4, 5). Initially, this was attempted by using immune stimulatory cytokines such as interleukin-2 alone or in combination with interferon-alpha (6). This early form of immunotherapy was found to lead to remarkably durable responses, however, only in a relatively small subgroup of patients (7). Nevertheless, this observation has stimulated more in-depth research into the mechanisms of immune evasion that culminated in the development of immune checkpoint inhibitors (ICIs) (8). The period between the cytokine and the ICI eras was dominated by VEGF/VEGFR targeting agents, most prominently tyrosine kinase inhibitors (TKIs) such as sunitinib (9). This treatment approach was based on a better understanding of the basic biology in particular of clear cell RCC (ccRCC), which commonly harbors an inactivation of the VHL gene and deregulation of the HIF/VEGF transcriptional network (3). Currently, combination therapies of ICIs and TKIs such as pembrolizumab and axitinib are the standard of care for patients with metastatic ccRCC (10). Despite the recent innovations in the therapeutic landscape, a significant proportion of patients will experience disease progression within a year or two after the initiation of systemic treatment (11). Hence, there is still an urgent need for new approaches to treat patients with advanced RCC. As highlighted in this Special Issue, suitable model systems are pivotal for successful pre-clinical drug development. At the same time, RCC is characterized by several peculiarities that need to be taken into consideration when using and developing RCC model systems.
While ccRCC represents the most common form of kidney cancer (approximately 75%), several other subtypes with non-clear cell RCC (nccRCC) histology such as papillary (approximately 10-20%) or chromophobe (approximately 5-7%) RCC exist. In fact, the 2016 WHO classification of tumors of the kidney lists more than 50 entities including 15 entities of renal cell tumors besides ccRCC (12). Among the newly included renal cell tumors in the 2016 WHO classification were some entities with specific molecular alterations such as fumarate hydratase (FH)-deficient RCC, succinate dehydrogenase (SDH)-deficient renal carcinoma and others such as tubulocystic RCC, clear cell papillary RCC and acquired cyctic disease-associated RCC (12, 13). There is a remarkable and still emerging diversification in the classification of papillary RCC that goes beyond the “classical” type 1 and type 2 subtypes (13). Emerging variants of papillary renal tumors are for example, papillary renal neoplasm with reversed polarity, biphasic squamoid/alveolar RCC, biphasic hyalinizing psammomatous RCC or thyroid-like follicular RCC (14). There are also RCCs that are defined by gene translocations such as the MiT family translocation RCCs (harboring TFE3 or TFEB translocations) or ALK-translocated RCCs, which frequently show aggressive growth characteristics (14).
Since nccRCCs are relatively rare, only a limited number of randomized, prospective clinical trials exist (15). Treatment modalities that have been extrapolated from ccRCC such as VEGF- or mTOR-targeting agents have been explored in metastatic nccRCC, however, with suboptimal results (16, 17). A randomized phase II trial has shown a progression-free survival benefit with the MET inhibitor cabozantinib over sunitinib in patients with metastatic papillary RCC, in which MET alterations are enriched (18). In line with this finding, the MET inhibitor savolitinib was found to show efficacy in MET-driven papillary RCC (19). Recent phase II trials that explore immune checkpoint inhibitors alone or in combination with cabozantinib in nccRCC show promising activity in patients with papillary RCC but at the same time highlight the therapeutic problem of chromophobe RCC (20, 21). Most nccRCC cell line and patient-derived xenograft (PDX) models have been established for the “classical” papillary RCC (22) and there is an urgent need to further expand model systems to other, less common nccRCC entities, including chromophobe RCC, for a further optimization and individualization of pre-clinical drug discovery.
In a seminal study published in 2012, metastatic ccRCC was shown to be characterized by a high degree of genomic ITH with indications for evolutionary processes with clonal (“truncal”) and subclonal mutations (23). These findings were later conceptualized in a new classification of ccRCC based on evolutionary subtypes (24). The proposed seven subtypes of ccRCC show distinct combinations of clonal and subclonal alterations including a sequence of mutational events (24). PDX models of RCC have been suggested to reflect, at least to a certain degree, the ITH found in the tumor of origin (25, 26). While it is conceivable that truncal driver aberrations in genes such as VHL or PBRM1 are preserved, it remains unclear whether PDX models can reflect the entire spectrum of subclonal driver mutations due to an inevitable sampling bias. Subclonal drivers can not only promote tumor expansion but have also been implicated as a therapeutic target or in the development of drug resistance (27). Moreover, subclonal as well as certain clonal drivers have been reported to show a regional variability (28). Hence, models of RCC that rely on regional sampling of the patient tumor are likely to underestimate the number and spectrum of driver events. This shortcoming may lead to an overestimation of drug efficacy and an underestimation of drug resistance. In this context, it is noteworthy that some widely used ccRCC cell line models do not harbor inactivated VHL (29) and may hence rather rely on subclonal driver events.
One idea to overcome, at least in part, the problem of ITH is the use of liquid biopsies (30). However, it is currently not entirely clear whether and to what extent circulating tumor cells or tumor-derived circulating DNA represent the whole subclonal architecture of RCC. Despite some promising results, principal issues such as low levels of circulating tumor DNA may preclude a wider use of liquid biopsies to characterize ITH in RCC (31).
A number of studies have analyzed the genomic composition or matched primary and metastatic RCC samples (23, 32, 33). There are very few, if any, patient-derived models of matched primary and metastatic sites. However, given the observed genetic discordance between primary tumor and metastases (33), such models would be highly desirable, in particular in patients with synchronous metastatic dissemination.
In addition to genomic ITH, functional ITH also needs to be taken into consideration when modeling RCC (34). One manifestation of functional ITH in ccRCC is the enhanced proliferation and intracellular signaling activity at the tumor invasion front (35). While no specific mutations could be pinpointed to explain this finding, some observations suggest that factors from the tumor microenvironment may be involved (36). The tumor periphery may hence represent a spatial niche that harbors tumor cells with an increased fitness or potentially higher aggressiveness. A recent study used computational modeling to suggest that such a “surface growth” pattern could be associated with an enhanced subclonal diversification (37). While this computational study did not include the microenvironment as a factor that may influence tumor growth, it nevertheless underscores that tumor evolution and growth patterns are very likely connected. It will be important to show whether and to what extent pre-clinical RCC models can reflect this interrelation.
Recently, synthetic DNA barcode tracking and single cell transcriptomics have revealed the existence of spatially confined clonal expansion in PDX models (38). Clonal dominance was driven by differential gene expression, which may not only influence the adaptation to the host microenvironment but also PDX engraftment efficiency in general. Consequently, tumor cell engraftment is subject to selection processes that can ultimately affect the cellular composition of PDXs and therefore drug vulnerabilities.
Besides a remarkable inter- and intratumoral genomic and functional heterogeneity (23, 35), ccRCC shows a number of immunological features that distinguish it from other tumor entities and that may impose challenges for modeling RCC in vitro and in vivo. First, a high number of tumor-infiltrating lymphocytes, in particular T cells, is associated with a poorer prognosis in RCC and not with a more favorable outcome as in most other malignancies (39–42). Conversely, immune excluded tumors were generally found to be associated with a better patient prognosis (43). Tumor-infiltrating T cells have been found to be frequently exhausted and to express high levels of inhibitory checkpoint proteins (44). The exhaustion of cytotoxic T cells was found to increase with disease progression together with a reduced clonotypic diversity indicating antigen exposure (45). T cell exhaustion in more advanced ccRCCs appears to involve interactions with immunosuppressive M2 macrophages and not, like in other cancers, regulatory T cells (45). In fact, a vicious cycle has been proposed in which terminally exhausted CD8-positive T cells drive M2 polarization, which, in turn, further promotes T cell exhaustion (45). These findings underscore that malignant tumors including ccRCCs initially represent complex and dynamic ecosystems, in which certain cellular interactions are fostered that later lead to an “ecological collapse” with a breakdown of immune cell diversity and function thus promoting tumor progression.
Besides T cells, recent findings highlight the important role of B cells in antitumor immunity (46). B cells produce antibodies and present antigens to T cells, which involves the formation of tertiary lymphoid structures (TLSs). Tumors with enhanced TLSs have in general a more favorable prognosis (47), which may also be the case in RCC (48). The development of non-clinical RCC models that reflect immune cell exhaustion, T cell-macrophage interactions and TLS formation will remain a daunting task.
While the tumor immune microenvironment conceivably plays an important role in shaping tumor progression, non-immune cells may likewise support clonal and subclonal expansion. Growth factors and cytokines secreted by non-immune cells of the tumor microenvironment with stimulatory effects on tumor growth include FGF-2, among others (36, 49, 50). Efforts to co-culture RCC cells with non-malignant cells of the microenvironment are therefore laudable, however, the functional state of such cells may not adequately reflect the situation in vivo, where cells of the tumor microenvironment may become re-programmed by numerous cellular interactions as well as extrinsic insults such as inflammation and necrosis (51). Together, these findings highlight the complexity of microenvironmental cell-cell interactions in RCC that remain a challenge to RCC model development.
In vitro drug screening has long been an important approach to characterize therapeutic vulnerabilities of cancer cells. Conceivably, the success of a drug screen depends on numerous factors including the source of tumor cells, the presence or absence of cells representing the tumor (immune) microenvironment, culture and growth conditions, drug availability, and read-out techniques (52). The high drop-out rate associated with cell-based pre-clinical drug discovery has been attributed to confounding factors of the experimental set-up that leads to an unsatisfying replicability and reproducibility (53). Organoids have been established for a number of human malignancies, however, reports on using organoids for drug screening purposes in RCC are relatively scarce (54–56). In particular patient-derived air-liquid interface organoid models appear to be promising for pre-clinical drug discovery since they maintain features of the original tumor including components of the microenvironment (57, 58). Ultimately, a systemic collection of model systems may help to overcome some problems in pre-clinical drug discovery (58, 59).
Nevertheless, one issue that appears to be quite common is the problem of “reverse engineering” i.e., the inability to characterize the mode of action of a drug that was identified as a hit in a screen. This could be related to unknown drug activities or reflect complex tumor cell-intrinsic molecular interactions and adaptation processes. For example, our group identified the MET inhibitor crizotinib in a compound screen using a primary patient-derived ccRCC cell line with a MET missense mutation (R988C) (60). This mutation has been suggested to function as a driver mutation in lung cancer (61, 62). Perplexingly, further experiments showed that MET was not a driver in the patient-derived cell line and the mode of action of crizotinib very likely involved its function to block the transmembrane transporter P-glycoprotein (60). A broader implication of this finding is that candidate driver events may not necessarily represent the prime target for tumor cell eradication and that there could be a disconnection between a genotype and the observed phenotype. It is furthermore possible that driver mutations vary with respect to their potency i.e., there could be strong drivers and weak drivers depending on the cellular context and positive or negative driver cooperativity.
The high degree of inter- and intratumoral heterogeneity represents a major obstacle for predicting therapeutic responses in patients with advanced RCC (63, 64). This problem also applies to multimodal treatment strategies e.g., the inclusion of surgical interventions during a patient’s path through the disease. Given the intrinsic difficulty to faithfully mirror a tumor ecosystem in its entirety, AI may help to connect pre-clinical RCC models with clinical data and vice versa as envisioned in Figure 1. Tools to estimate genetic ITH have been developed (65) and may be integrated into such AI algorithms.
Figure 1 AI holds the promise to link data from RCC model systems to complex and comprehensive clinical data to improve pre-clinical drug discovery.
There are currently two major AI concepts, one that has a comprehensive view on patient data, e.g. the “digital twin” and a second consisting of highly focused decision-support tasks that frequently use deep learning applications and pattern recognition algorithms (66). AI has the potential to support virtually all aspects of pre-clinical drug discovery (52, 67). For example, machine learning approaches have been developed to aid target/driver gene identification (68). Structure- or ligand-based virtual screening can significantly improve speed and efficacy of lead compound development. Lastly, AI tools can also help to predict pharmacokinetic properties of a new agent (67). It is important to mention that drug-related adverse events in a mostly palliative situation need special consideration. AI and pre-clinical models, for example for cardiovascular toxicity, may help not only to predict adverse events but also to develop protective strategies (67, 69).
These findings underscore the role of AI in the pre-clinical drug development process. Nevertheless, in precision oncology, the global question of “drug sensitivity” of a patient with advanced RCC may be too broad and may need to be subdivided into “narrow-task” questions (66) such as response to a given experimental drug, immune oncological agents, TKIs or others. Ultimately, prospective clinical trials will be needed to determine the impact of AI on patient outcome.
Pre-clinical models are vital to further develop the therapeutic landscape for metastatic ccRCC, yet there are a number of biological peculiarities that may represent serious obstacles for the identification of novel immunological and non-immunological therapies. One approach to overcome these hurdles could be to combine different modeling approaches such as 2D and 3D cell culture models and PDXs (70) despite the difficulty to adequately incorporate immune responses. Moreover, AI has the potential to transform pre-clinical drug development by combining data from non-clinical models with comprehensive patient data including multi-omics analyses. Still, the magnitude and complexity of cellular and molecular interactions that characterizes the “ecosystem RCC” will continue to challenge future generations of tumor biologists.
Conceptualization of the manuscript, contributing ideas and information: all authors. Writing the first draft of the manuscript: SD. Critical reading of the manuscript: all authors. All authors contributed to the article and approved the submitted version.
Bundesministerium für Wirtschaft und Klimaschutz.
The authors declare that the research was conducted in the absence of any commercial or financial relationships that could be construed as a potential conflict of interest.
All claims expressed in this article are solely those of the authors and do not necessarily represent those of their affiliated organizations, or those of the publisher, the editors and the reviewers. Any product that may be evaluated in this article, or claim that may be made by its manufacturer, is not guaranteed or endorsed by the publisher.
The authors’ laboratory is supported by the German Federal Ministry for Economic Affairs and Climate Action.
1. Siegel RL, Miller KD, Jemal A. Cancer Statistics, 2020. CA Cancer J Clin (2020) 70:7–30. doi: 10.3322/caac.21590
2. Choueiri TK, Motzer RJ. Systemic Therapy for Metastatic Renal-Cell Carcinoma. N Engl J Med (2017) 376:354–66. doi: 10.1056/NEJMra1601333
3. Choueiri TK, Kaelin WG. Targeting the HIF2-VEGF Axis in Renal Cell Carcinoma. Nat Med (2020) 26:1519–30. doi: 10.1038/s41591-020-1093-z
4. Janiszewska AD, Poletajew S, Wasiutyński A. Spontaneous Regression of Renal Cell Carcinoma. Contemp Oncol (Pozn) (2013) 17:123–7. doi: 10.5114/wo.2013.34613
5. Huang JJ, Hsieh JJ. The Therapeutic Landscape of Renal Cell Carcinoma: From the Dark Age to the Golden Age. Semin Nephrol (2020) 40:28–41. doi: 10.1016/j.semnephrol.2019.12.004
6. Rosenberg SA. IL-2: The First Effective Immunotherapy for Human Cancer. J Immunol (2014) 192:5451–8. doi: 10.4049/jimmunol.1490019
7. Fishman M, Dutcher JP, Clark JI, Alva A, Miletello GP, Curti B, et al. Overall Survival by Clinical Risk Category for High Dose Interleukin-2 (HD IL-2) Treated Patients With Metastatic Renal Cell Cancer (mRCC): Data From the PROCLAIMSM Registry. J Immunother Cancer (2019) 7:84. doi: 10.1186/s40425-019-0567-3
8. Sharma P, Allison JP. Dissecting the Mechanisms of Immune Checkpoint Therapy. Nat Rev Immunol (2020) 20:75–6. doi: 10.1038/s41577-020-0275-8
9. Motzer RJ, Hutson TE, Tomczak P, Michaelson MD, Bukowski RM, Rixe O, et al. Sunitinib Versus Interferon Alfa in Metastatic Renal-Cell Carcinoma. N Engl J Med (2007) 356:115–24. doi: 10.1056/NEJMoa065044
10. Rini BI, Plimack ER, Stus V, Gafanov R, Hawkins R, Nosov D, et al. Pembrolizumab Plus Axitinib Versus Sunitinib for Advanced Renal-Cell Carcinoma. N Engl J Med (2019) 380:1116–27. doi: 10.1056/NEJMoa1816714
11. Hemminki O, Perlis N, Bjorklund J, Finelli A, Zlotta AR, Hemminki A. Treatment of Advanced Renal Cell Carcinoma: Immunotherapies Have Demonstrated Overall Survival Benefits While Targeted Therapies Have Not. Eur Urol Open Sci (2020) 22:61–73. doi: 10.1016/j.euros.2020.11.003
12. Moch H, Cubilla AL, Humphrey PA, Reuter VE, Ulbright TM. The 2016 WHO Classification of Tumours of the Urinary System and Male Genital Organs-Part A: Renal, Penile, and Testicular Tumours. Eur Urol (2016) 70:93–105. doi: 10.1016/j.eururo.2016.02.029
13. Cimadamore A, Cheng L, Scarpelli M, Massari F, Mollica V, Santoni M, et al. Towards A New WHO Classification of Renal Cell Tumor: What the Clinician Needs to Know-a Narrative Review. Transl Androl Urol (2021) 10:1506–20. doi: 10.21037/tau-20-1150
14. Lobo J, Ohashi R, Helmchen BM, Rupp NJ, Rüschoff JH, Moch H. The Morphological Spectrum of Papillary Renal Cell Carcinoma and Prevalence of Provisional/Emerging Renal Tumor Entities With Papillary Growth. Biomedicines (2021) 9:1418. doi: 10.3390/biomedicines9101418
15. Sepe P, Ottini A, Pircher CC, Franza A, Claps M, Guadalupi V, et al. Characteristics and Treatment Challenges of Non-Clear Cell Renal Cell Carcinoma. Cancers (Basel) (2021) 13:3807. doi: 10.3390/cancers13153807
16. Tannir NM, Jonasch E, Albiges L, Altinmakas E, Ng CS, Matin SF, et al. Everolimus Versus Sunitinib Prospective Evaluation in Metastatic Non-Clear Cell Renal Cell Carcinoma (ESPN): A Randomized Multicenter Phase 2 Trial. Eur Urol (2016) 69:866–74. doi: 10.1016/j.eururo.2015.10.049
17. Armstrong AJ, Halabi S, Eisen T, Broderick S, Stadler WM, Jones RJ, et al. Everolimus Versus Sunitinib for Patients With Metastatic Non-Clear Cell Renal Cell Carcinoma (ASPEN): A Multicentre, Open-Label, Randomised Phase 2 Trial. Lancet Oncol (2016) 17:378–88. doi: 10.1016/S1470-2045(15)00515-X
18. Pal SK, Tangen C, Thompson IM, Balzer-Haas N, George DJ, Heng DYC, et al. A Comparison of Sunitinib With Cabozantinib, Crizotinib, and Savolitinib for Treatment of Advanced Papillary Renal Cell Carcinoma: A Randomised, Open-Label, Phase 2 Trial. Lancet (2021) 397:695–703. doi: 10.1016/S0140-6736(21)00152-5
19. Choueiri TK, Heng DYC, Lee J-L, Cancel M, Verheijen RB, Mellemgaard A, et al. Efficacy of Savolitinib vs Sunitinib in Patients With MET-Driven Papillary Renal Cell Carcinoma: The SAVOIR Phase 3 Randomized Clinical Trial. JAMA Oncol (2020) 6:1247–55. doi: 10.1001/jamaoncol.2020.2218
20. McDermott DF, Lee J-L, Ziobro M, Suarez C, Langiewicz P, Matveev VB, et al. Open-Label, Single-Arm, Phase II Study of Pembrolizumab Monotherapy as First-Line Therapy in Patients With Advanced Non-Clear Cell Renal Cell Carcinoma. J Clin Oncol (2021) 39:1029–39. doi: 10.1200/JCO.20.02365
21. Lee C-H, Voss MH, Carlo MI, Chen Y-B, Zucker M, Knezevic A, et al. Phase II Trial of Cabozantinib Plus Nivolumab in Patients With Non-Clear-Cell Renal Cell Carcinoma and Genomic Correlates. J Clin Oncol (2022) JCO2101944. doi: 10.1200/JCO.21.01944
22. Yang Y, Ricketts CJ, Vocke CD, Killian JK, Padilla-Nash HM, Lang M, et al. Characterization of Genetically Defined Sporadic and Hereditary Type 1 Papillary Renal Cell Carcinoma Cell Lines. Genes Chromosomes Cancer (2021) 60:434–46. doi: 10.1002/gcc.22940
23. Gerlinger M, Rowan AJ, Horswell S, Larkin J, Endesfelder D, Gronroos E, et al. Intratumor Heterogeneity and Branched Evolution Revealed by Multiregion Sequencing. N Engl J Med (2012) 366:883–92. doi: 10.1056/NEJMoa1113205
24. Turajlic S, Xu H, Litchfield K, Rowan A, Horswell S, Chambers T, et al. Deterministic Evolutionary Trajectories Influence Primary Tumor Growth: TRACERx Renal. Cell (2018) 173:595–610.e11. doi: 10.1016/j.cell.2018.03.043
25. Ding L, Ellis MJ, Li S, Larson DE, Chen K, Wallis JW, et al. Genome Remodelling in a Basal-Like Breast Cancer Metastasis and Xenograft. Nature (2010) 464:999–1005. doi: 10.1038/nature08989
26. Lin D, Wyatt AW, Xue H, Wang Y, Dong X, Haegert A, et al. High Fidelity Patient-Derived Xenografts for Accelerating Prostate Cancer Discovery and Drug Development. Cancer Res (2014) 74:1272–83. doi: 10.1158/0008-5472.CAN-13-2921-T
27. McGranahan N, Favero F, de Bruin EC, Birkbak NJ, Szallasi Z, Swanton C. Clonal Status of Actionable Driver Events and the Timing of Mutational Processes in Cancer Evolution. Sci Transl Med (2015) 7:283ra54. doi: 10.1126/scitranslmed.aaa1408
28. Gerlinger M, Horswell S, Larkin J, Rowan AJ, Salm MP, Varela I, et al. Genomic Architecture and Evolution of Clear Cell Renal Cell Carcinomas Defined by Multiregion Sequencing. Nat Genet (2014) 46:225–33. doi: 10.1038/ng.2891
29. Brodaczewska KK, Szczylik C, Fiedorowicz M, Porta C, Czarnecka AM. Choosing the Right Cell Line for Renal Cell Cancer Research. Mol Cancer (2016) 15:83–15. doi: 10.1186/s12943-016-0565-8
30. Keller L, Pantel K. Unravelling Tumour Heterogeneity by Single-Cell Profiling of Circulating Tumour Cells. Nat Rev Cancer (2019) 19:553–67. doi: 10.1038/s41568-019-0180-2
31. Smith CG, Moser T, Mouliere F, Field-Rayner J, Eldridge M, Riediger AL, et al. Comprehensive Characterization of Cell-Free Tumor DNA in Plasma and Urine of Patients With Renal Tumors. Genome Med (2020) 12:23–17. doi: 10.1186/s13073-020-00723-8
32. Dietz S, Sültmann H, Du Y, Reisinger E, Riediger AL, Volckmar A-L, et al. Patient-Specific Molecular Alterations Are Associated With Metastatic Clear Cell Renal Cell Cancer Progressing Under Tyrosine Kinase Inhibitor Therapy. Oncotarget (2017) 8:74049–57. doi: 10.18632/oncotarget.18200
33. Becerra MF, Reznik E, Redzematovic A, Tennenbaum DM, Kashan M, Ghanaat M, et al. Comparative Genomic Profiling of Matched Primary and Metastatic Tumors in Renal Cell Carcinoma. Eur Urol Focus (2018) 4:986–94. doi: 10.1016/j.euf.2017.09.016
34. Almendro V, Kim HJ, Cheng Y-K, Gönen M, Itzkovitz S, Argani P, et al. Genetic and Phenotypic Diversity in Breast Tumor Metastases. Cancer Res (2014) 74:1338–48. doi: 10.1158/0008-5472.CAN-13-2357-T
35. Hoefflin R, Lahrmann B, Warsow G, Hübschmann D, Spath C, Walter B, et al. Spatial Niche Formation But Not Malignant Progression Is a Driving Force for Intratumoural Heterogeneity. Nat Commun (2016) 7:ncomms11845. doi: 10.1038/ncomms11845
36. Hou W, Kaczorowski A, Lantwin P, Kippenberger M, Schütz V, Franke D, et al. Microenvironment-Derived FGF-2 Stimulates Renal Cell Carcinoma Cell Proliferation Through Modulation of p27Kip1: Implications for Spatial Niche Formation and Functional Intratumoral Heterogeneity. Pathobiology (2020) 87:114–24. doi: 10.1159/000506709
37. Fu X, Zhao Y, Lopez JI, Rowan A, Au L, Fendler A, et al. Spatial Patterns of Tumour Growth Impact Clonal Diversification in a Computational Model and the TRACERx Renal Study. Nat Ecol Evol (2022) 6:88–102. doi: 10.1038/s41559-021-01586-x
38. Contreras-Trujillo H, Eerdeng J, Akre S, Jiang D, Contreras J, Gala B, et al. Deciphering Intratumoral Heterogeneity Using Integrated Clonal Tracking and Single-Cell Transcriptome Analyses. Nat Commun (2021) 12:6522–14. doi: 10.1038/s41467-021-26771-1
39. Webster WS, Lohse CM, Thompson RH, Dong H, Frigola X, Dicks DL, et al. Mononuclear Cell Infiltration in Clear-Cell Renal Cell Carcinoma Independently Predicts Patient Survival. Cancer (2006) 107:46–53. doi: 10.1002/cncr.21951
40. Siddiqui SA, Frigola X, Bonne-Annee S, Mercader M, Kuntz SM, Krambeck AE, et al. Tumor-Infiltrating Foxp3-CD4+CD25+ T Cells Predict Poor Survival in Renal Cell Carcinoma. Clin Cancer Res (2007) 13:2075–81. doi: 10.1158/1078-0432.CCR-06-2139
41. Giraldo NA, Becht E, Vano Y, Petitprez F, Lacroix L, Validire P, et al. Tumor-Infiltrating and Peripheral Blood T-Cell Immunophenotypes Predict Early Relapse in Localized Clear Cell Renal Cell Carcinoma. Clin Cancer Res (2017) 23:4416–28. doi: 10.1158/1078-0432.CCR-16-2848
42. Fridman WH, Zitvogel L, Sautès-Fridman C, Kroemer G. The Immune Contexture in Cancer Prognosis and Treatment. Nat Rev Clin Oncol (2017) 14:717–34. doi: 10.1038/nrclinonc.2017.101
43. Brück O, Lee MH, Turkki R, Uski I, Penttilä P, Paavolainen L, et al. Spatial Immunoprofiling of the Intratumoral and Peritumoral Tissue of Renal Cell Carcinoma Patients. Mod Pathol (2021) 34:2229–41. doi: 10.1038/s41379-021-00864-0
44. Chevrier S, Levine JH, Zanotelli VRT, Silina K, Schulz D, Bacac M, et al. An Immune Atlas of Clear Cell Renal Cell Carcinoma. Cell (2017) 169:736–749.e18. doi: 10.1016/j.cell.2017.04.016
45. Braun DA, Street K, Burke KP, Cookmeyer DL, Denize T, Pedersen CB, et al. Progressive Immune Dysfunction With Advancing Disease Stage in Renal Cell Carcinoma. Cancer Cell (2021) 39:632–648.e8. doi: 10.1016/j.ccell.2021.02.013
46. Fridman WH, Meylan M, Petitprez F, Sun C-M, Italiano A, Sautès-Fridman C. B Cells and Tertiary Lymphoid Structures as Determinants of Tumour Immune Contexture and Clinical Outcome. Nat Rev Clin Oncol (2022) 1–17. doi: 10.1038/s41571-022-00619-z
47. Ruffin AT, Cillo AR, Tabib T, Liu A, Onkar S, Kunning SR, et al. B Cell Signatures and Tertiary Lymphoid Structures Contribute to Outcome in Head and Neck Squamous Cell Carcinoma. Nat Commun (2021) 12:3349–16. doi: 10.1038/s41467-021-23355-x
48. Sautès-Fridman C, Lawand M, Giraldo NA, Kaplon H, Germain C, Fridman WH, et al. Tertiary Lymphoid Structures in Cancers: Prognostic Value, Regulation, and Manipulation for Therapeutic Intervention. Front Immunol (2016) 7:407. doi: 10.3389/fimmu.2016.00407
49. Kominsky SL, Doucet M, Brady K, Weber KL. TGF-Beta Promotes the Establishment of Renal Cell Carcinoma Bone Metastasis. J Bone Miner Res (2007) 22:37–44. doi: 10.1359/jbmr.061005
50. Heidegger I, Pircher A, Pichler R. Targeting the Tumor Microenvironment in Renal Cell Cancer Biology and Therapy. Front Oncol (2019) 9:490. doi: 10.3389/fonc.2019.00490
51. Sahai E, Astsaturov I, Cukierman E, DeNardo DG, Egeblad M, Evans RM, et al. A Framework for Advancing Our Understanding of Cancer-Associated Fibroblasts. Nat Rev Cancer (2020) 20:174–86. doi: 10.1038/s41568-019-0238-1
52. Hughes JP, Rees S, Kalindjian SB, Philpott KL. Principles of Early Drug Discovery. Br J Pharmacol (2011) 162:1239–49. doi: 10.1111/j.1476-5381.2010.01127.x
53. Larsson P, Engqvist H, Biermann J, Werner Rönnerman E, Forssell-Aronsson E, Kovács A, et al. Optimization of Cell Viability Assays to Improve Replicability and Reproducibility of Cancer Drug Sensitivity Screens. Sci Rep (2020) 10:5798–12. doi: 10.1038/s41598-020-62848-5
54. Lobo NC, Gedye C, Apostoli AJ, Brown KR, Paterson J, Stickle N,R, et al. Efficient Generation of Patient-matched Malignant and Normal Primary Cell Cultures from Clear Cell Renal Cell Carcinoma Patients: Clinically Relevant Models for Research and Personalized Medicine. BMC Cancer (2016) 16:485–15. doi: 10.1186/s12885-016-2539-z
55. Grassi L, Alfonsi R, Francescangeli F, Signore M, De Angelis ML, Addario A, et al. Organoids as a New Model for Improving Regenerative Medicine and Cancer Personalized Therapy in Renal Diseases. Cell Death Dis (2019) 10:201–15. doi: 10.1038/s41419-019-1453-0
56. Fendler A, Bauer D, Busch J, Jung K, Wulf-Goldenberg A, Kunz S, et al. Inhibiting WNT and NOTCH in Renal Cancer Stem Cells and the Implications for Human Patients. Nat Commun (2020) 11:929–16. doi: 10.1038/s41467-020-14700-7
57. Neal JT, Li X, Zhu J, Giangarra V, Grzeskowiak CL, Ju J, et al. Organoid Modeling of the Tumor Immune Microenvironment. Cell (2018) 175:1972–1988.e16. doi: 10.1016/j.cell.2018.11.021
58. Esser LK, Branchi V, Leonardelli S, Pelusi N, Simon AG, Klümper N, et al. Cultivation of Clear Cell Renal Cell Carcinoma Patient-Derived Organoids in an Air-Liquid Interface System as a Tool for Studying Individualized Therapy. Front Oncol (2020) 10:1775. doi: 10.3389/fonc.2020.01775
59. Lang H, Béraud C, Bethry A, Danilin S, Lindner V, Coquard C, et al. Establishment of a Large Panel of Patient-Derived Preclinical Models of Human Renal Cell Carcinoma. Oncotarget (2016) 7:59336–59. doi: 10.18632/oncotarget.10659
60. Schneider M, Schüler J, Höfflin R, Korzeniewski N, Grüllich C, Roth W, et al. Phenotypic Drug Screening and Target Validation for Improved Personalized Therapy Reveal the Complexity of Phenotype-Genotype Correlations in Clear Cell Renal Cell Carcinoma. Urol Oncol (2014) 32:877–84. doi: 10.1016/j.urolonc.2014.03.011
61. Ma PC, Jagadeeswaran R, Jagadeesh S, Tretiakova MS, Nallasura V, Fox EA, et al. Functional Expression and Mutations of C-Met and Its Therapeutic Inhibition With SU11274 and Small Interfering RNA in Non-Small Cell Lung Cancer. Cancer Res (2005) 65:1479–88. doi: 10.1158/0008-5472.CAN-04-2650
62. Ricciuti B, Marcomigni L, Metro G, Bellezza G, Caselli E, Baglivo S, et al. Identification of EML4-ALK Rearrangement and MET Exon 14 R988C Mutation in a Patient With High-Grade Neuroendocrine Lung Carcinoma Who Experienced a Lazarus Response to Crizotinib. J Thorac Oncol (2018) 13:e220–2. doi: 10.1016/j.jtho.2018.05.035
63. Marusyk A, Janiszewska M, Polyak K. Intratumor Heterogeneity: The Rosetta Stone of Therapy Resistance. Cancer Cell (2020) 37:471–84. doi: 10.1016/j.ccell.2020.03.007
64. Fisher R, Pusztai L, Swanton C. Cancer Heterogeneity: Implications for Targeted Therapeutics. Br J Cancer (2013) 108:479–85. doi: 10.1038/bjc.2012.581
65. Mroz EA, Rocco JW. MATH, A Novel Measure of Intratumor Genetic Heterogeneity, Is High in Poor-Outcome Classes of Head and Neck Squamous Cell Carcinoma. Oral Oncol (2013) 49:211–5. doi: 10.1016/j.oraloncology.2012.09.007
66. Kann BH, Hosny A, Aerts HJWL. Artificial Intelligence for Clinical Oncology. Cancer Cell (2021) 39:916–27. doi: 10.1016/j.ccell.2021.04.002
67. Gupta R, Srivastava D, Sahu M, Tiwari S, Ambasta RK, Kumar P. Artificial Intelligence to Deep Learning: Machine Intelligence Approach for Drug Discovery. Mol Divers (2021) 25:1315–60. doi: 10.1007/s11030-021-10217-3
68. Han Y, Yang J, Qian X, Cheng W-C, Liu S-H, Hua X, et al. DriverML: A Machine Learning Algorithm for Identifying Driver Genes in Cancer Sequencing Studies. Nucleic Acids Res (2019) 47:e45. doi: 10.1093/nar/gkz096
69. L'Abbate S, Chianca M, Fabiani I, Del Franco A, Giannoni A, Vergaro G, et al. In Vivo Murine Models of Cardiotoxicity Due to Anticancer Drugs: Challenges and Opportunities for Clinical Translation. J Cardiovasc Transl Res (2022) 1–20. doi: 10.1007/s12265-022-10231-2
Keywords: renal cell carcinoma, intratumoral heterogeneity (ITH), drug development, patient-derived xenografts (PDX), preclinical studies
Citation: Pohl L, Friedhoff J, Jurcic C, Teroerde M, Schindler I, Strepi K, Schneider F, Kaczorowski A, Hohenfellner M, Duensing A and Duensing S (2022) Kidney Cancer Models for Pre-Clinical Drug Discovery: Challenges and Opportunities. Front. Oncol. 12:889686. doi: 10.3389/fonc.2022.889686
Received: 04 March 2022; Accepted: 13 April 2022;
Published: 10 May 2022.
Edited by:
Thierry Massfelder, Institut National de la Santé et de la Recherche Médicale (INSERM), FranceReviewed by:
João Lobo, Portuguese Oncology Institute, PortugalCopyright © 2022 Pohl, Friedhoff, Jurcic, Teroerde, Schindler, Strepi, Schneider, Kaczorowski, Hohenfellner, Duensing and Duensing. This is an open-access article distributed under the terms of the Creative Commons Attribution License (CC BY). The use, distribution or reproduction in other forums is permitted, provided the original author(s) and the copyright owner(s) are credited and that the original publication in this journal is cited, in accordance with accepted academic practice. No use, distribution or reproduction is permitted which does not comply with these terms.
*Correspondence: Stefan Duensing, c3RlZmFuLmR1ZW5zaW5nQG1lZC51bmktaGVpZGVsYmVyZy5kZQ==
Disclaimer: All claims expressed in this article are solely those of the authors and do not necessarily represent those of their affiliated organizations, or those of the publisher, the editors and the reviewers. Any product that may be evaluated in this article or claim that may be made by its manufacturer is not guaranteed or endorsed by the publisher.
Research integrity at Frontiers
Learn more about the work of our research integrity team to safeguard the quality of each article we publish.