- 1Department of Urology, People’s Hospital of Putuo District, Shanghai, China
- 2Department of Urology, Affiliated Zhongda Hospital of Southeast University, Nanjing, China
- 3Department of Urology, Wuxi No.2 Hospital, Nanjing Medical University, Wuxi, China
- 4Nanjing Lishui District People’s Hospital, Zhongda Hospital Lishui Branch, Southeast University, Nanjing, China
Purpose: Clear cell renal cell carcinoma (ccRCC) is one of the most common malignancies of the urinary system. This study was conducted to discover a new target that can predict the prognosis and promote the treatment of ccRCC.
Methods: The raw data were downloaded from the TCGA database, and the predictive value of ASNS for various clinicopathological features was verified in the following analysis. Then, we analyzed the potential involvement of ASNS in tumor immunity and obtained the possible pathways involving ASNS through GO/KEGG enrichment analysis and GSEA. We also further verified our findings in pathological specimens of ccRCC patients.
Results: ASNS expression was significantly increased in ccRCC, which was associated with advanced clinicopathological characteristics. It was an independent prognostic factor for overall survival in 535 patients with ccRCC. Immune cell infiltration analysis revealed that ASNS expression was related to T lymphocyte infiltration of tumors and poor prognosis. Moreover, we performed relevant functional enrichment analyses of ASNS.
Conclusions: ASNS might play a significant role in the development and immune cell infiltration of ccRCC and serve as a valuable clinical prognostic biomarker.
Introduction
Renal cell carcinoma (RCC) is a common cancer type, and approximately 430,000 new global cases and 170,000 RCC-related deaths occurred in 2020 (1). RCC accounts for approximately 3% of all cancers, with the highest incidence in Western countries, and 80%–90% of RCC are clear cell renal cell carcinoma (ccRCC) (2). None of the treatments were effective in patients with renal tumors subjected to the same surgical procedures and patients with advanced disease treated with similar drugs (3). Although an occasional response was reported, the available systemic therapies did not increase the survival of patients with advanced disease (2). Early detection and screening are priorities for RCC research (4). Therefore, discovering a new target of ccRCC, especially for those with advanced and metastatic diseases, is crucial.
Asparagine synthase (ASNS) catalyzes the synthesis of asparagine and glutamate from aspartic acid and glutamine in an ATP-dependent amidotransferase reaction, accompanied by glutamine deamidation (5). Large-scale loss-of-function analysis in vitro identified ASNS as cancer dependent in several solid malignancies; however, the specific mechanism has not been discovered (6). Knott et al. highlighted the role of ASNS in tumor growth and metastatic dissemination in a breast cancer model (7), prompting the necessity to evaluate the expression of ASNS and facilitating the prognosis of patients with ccRCC.
We downloaded raw data from The Cancer Genome Atlas (TCGA) database and verified the predictive value of ASNS for various clinicopathological features in the following analyses. We analyzed the potential involvement of ASNS in tumor immunity and identified the possible pathways associated with ASNS through Gene Ontology (GO)/Kyoto Encyclopedia of Genes and Genomes (KEGG) enrichment analyses and gene set enrichment analysis (GSEA). We further verified our findings in the pathological specimens of patients with ccRCC. To conclude, we found that ASNS was highly expressed in ccRCC patients, and its high expression could lead to a worse prognosis. Thus, our findings revealed that ASNS might play a significant role in the development and immune cell infiltration of ccRCC and serve as a valuable clinical prognostic biomarker.
Materials and methods
Public database collection
Gene expression (535 tumor and 72 normal samples), DNA methylation, phenotype, and survival data were downloaded from http://xena.ucsc.edu/. |logFC| > 1.5 and p < 0.01 were defined as the criteria for differentially expressed genes (DEGs), and DEGs were identified using the limma R package.
COX univariate and multivariate analysis
Cox univariate and multivariate analyses were performed to determine the risk factors for ccRCC prognosis using R software.
Survival analysis
Overall survival (OS) and progression-free survival rates of patients with ccRCC were investigated by Kaplan–Meier analysis.
Correlation between immune infiltration and expression
TIMER2.0 (http://timer.comp-genomics.org/) was used for the comprehensive analysis of the relationship between ASNS expression and tumor-infiltrating immune cell levels, namely, CD4+ T cells, Tregs, CD8+ T cells, CTLA4, and PD-L1 (CD274) (8).
Expression of hub genes and survival analysis
The STRING (https://string-db.org/) website analyzes protein–protein interactions using a unique set of computer prediction models (9). The expression levels of hub genes in tumor and normal tissues based on the ccRCC dataset were compared using the Wilcoxon rank-sum test (p < 0.05). OS analysis for the expression of hub genes between the high- and low-expression groups was performed, with a p-value of <0.05 indicating statistical significance.
GEPIA2
GEPIA2 (http://gepia2.cancer-pku.cn/#index) uses standard processing procedures to analyze the data using many tumor samples and normal tissue samples (10). GEPIA2 analyzes the OS or disease-free survival of cancer patients based on gene expression. GEPIA2 uses the Log-rank test for hypothesis testing. The hazard ratio and 95% confidence interval are also included in survival plots.
GO and KEGG enrichment analyses
According to ASNS expression level, ccRCC samples were sorted and divided into two groups of high and low expression, and the genes with differential expression were screened separately (|logFC| > 0.5, p-value < 0.05). The Clusterprofiler R package was used for GO enrichment analysis, which included molecular function, biological processes, and cellular components of DEGs, and KEGG enrichment analysis.
Gene set enrichment analysis
The molecular mechanisms involved in ccRCC with different levels of ASNS expression were examined by the GSEA approach (11). The reference gene set used for GSEA was obtained from c7.immunesigdb.v7.5.1.symbols.gmt. Gene sets with a nominal p-value less than 0.05, |Normalized Enrichment Score| greater than 1, and false discovery rate less than 0.25 in the GSEA report were considered statistically significant.
Western blotting
Western blotting was performed as described in our previous study (12).
Immunohistochemistry
The expression and distribution of ASNS protein were detected by immunohistochemistry in paraffin-embedded tissue sections of each group. After routine paraffin dewaxing to water, antigen repair was performed. Hydrogen peroxide solution (3%) was added to remove endogenous catalase. Bovine serum albumin sealing solution (1%) was added, and the slides were incubated for 15 min. After the blocking solution was added, the primary antibody against ASNS (1:100 dilution) was added, and the slides were incubated overnight at 4°C. Thereafter, they were washed thrice with phosphate-buffered saline with Tween 20. The secondary antibody (1:100 dilution) was added, and the slides were incubated for 1 h at room temperature. DAB was added for color development, after which the slides were re-dyed with hematoxylin for 30 s. After washing with running water for 1 min, the slides were treated with 0.1% sodium bicarbonate to develop blue color. Then, the slides were subjected to dehydration and xylene treatment until the sections became transparent, mounted with neutral gum, observed under a microscope, and photographed.
The immunohistochemical staining intensity of ASNS was assessed using ImageJ software with the assistance of experienced pathologists in Affiliated Zhongda Hospital of Southeast University (13, 14).
Sample information
Clinical data of 81 patients were collected at the Affiliated Zhongda Hospital of Southeast University from March 2019 to May 2022. All patients were diagnosed with ccRCC (unilateral) and had no other carcinomas. All patients did not undergo any preoperative therapy for the carcinoma (chemotherapy, targeted therapy, immunotherapy, etc.). Age distribution: 19–85 years old. Surgical modalities: Partial nephrectomy or radical nephrectomy. Neoplasm histological grade criteria: Fuhrman nuclear grading system. Pathological stage criteria: American Joint Committee on Cancer (AJCC).
Statistical analysis
Analyses were performed using IBM SPSS for MAC version 26.0. The Mann–Whitney test was used to compare continuous variables between the two groups. The immunohistochemical staining intensity and clinical characteristics, such as gender, age, location, tumor size, TNM stage, AJCC stage, and grade were subjected to Spearman rank correlation analysis. p-values less than 0.05 were considered statistically significant.
Results
Overexpression of ASNS mRNA in ccRCC is related to poor prognosis
The expression pattern of ASNS in multiple pan-cancers was evaluated by using data from TIMER2.0, and the prognostic values of ASNS in multiple cancers (invasive breast carcinoma, cervical squamous cell carcinoma, endocervical adenocarcinoma, cholangiocarcinoma, esophageal carcinoma, kidney renal clear cell carcinoma, hepatocellular carcinoma, lung adenocarcinoma, lung squamous cell carcinoma, pancreatic adenocarcinoma, and stomach adenocarcinoma) are shown in the survival map prepared using GEPIA2 (Figures 1A, B). The pan-cancer overexpression of ASNS was linked to poor prognosis. The mRNA data of ccRCC were downloaded from TCGA (https://xenabrowser.net/datapages/) and included 535 tumor tissues and 72 normal tissues. ASNS was highly expressed in ccRCC compared with normal renal tissues (p < 0.001; Figure 1C) and was correlated with poor prognosis, i.e., OS (p < 0.001; Figure 1D). Furthermore, the high protein expression level of ASNS in ccRCC was verified by data from the Human Protein Atlas (HPA) databases (https://www.proteinatlas.org/) (Figure 1E).
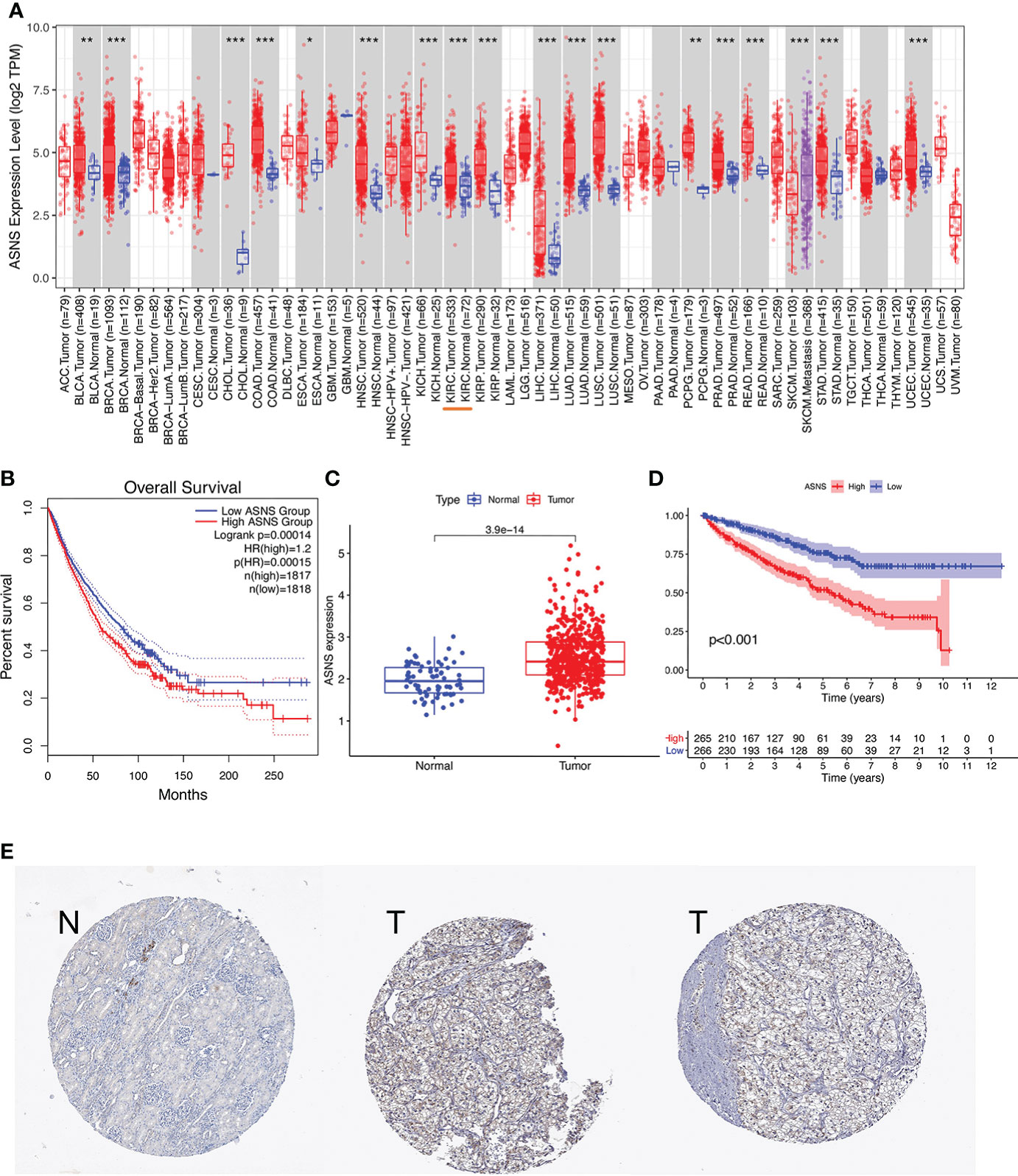
Figure 1 Expression of ASNS. (A) Pan-cancer expression of ASNS. (B) Prognostic values of ASNS gene in multiple cancers (BRCA, CESC, CHOL, ESCA, KIRC, LIHC, LUAD, LUSC, PAAD, and STAD). (C) ASNS expression in ccRCC and normal tissues. (D) Relationship between ASNS expression levels and prognosis, i.e., overall survival (OS). (E) ASNS protein level in ccRCC from the HPA database. The statistical significance computed by the Wilcoxon test is annotated by the number of stars (*: p-value <0.05; **: p-value <0.01; ***: p-value <0.001).
For further clinical research, the expression pattern of ASNS was studied in relation to several clinicopathological features, and the data demonstrated that ASNS expression gradually increased with tumor progression (Figure 2). Therefore, ASNS could be an independent factor predicting ccRCC prognosis.
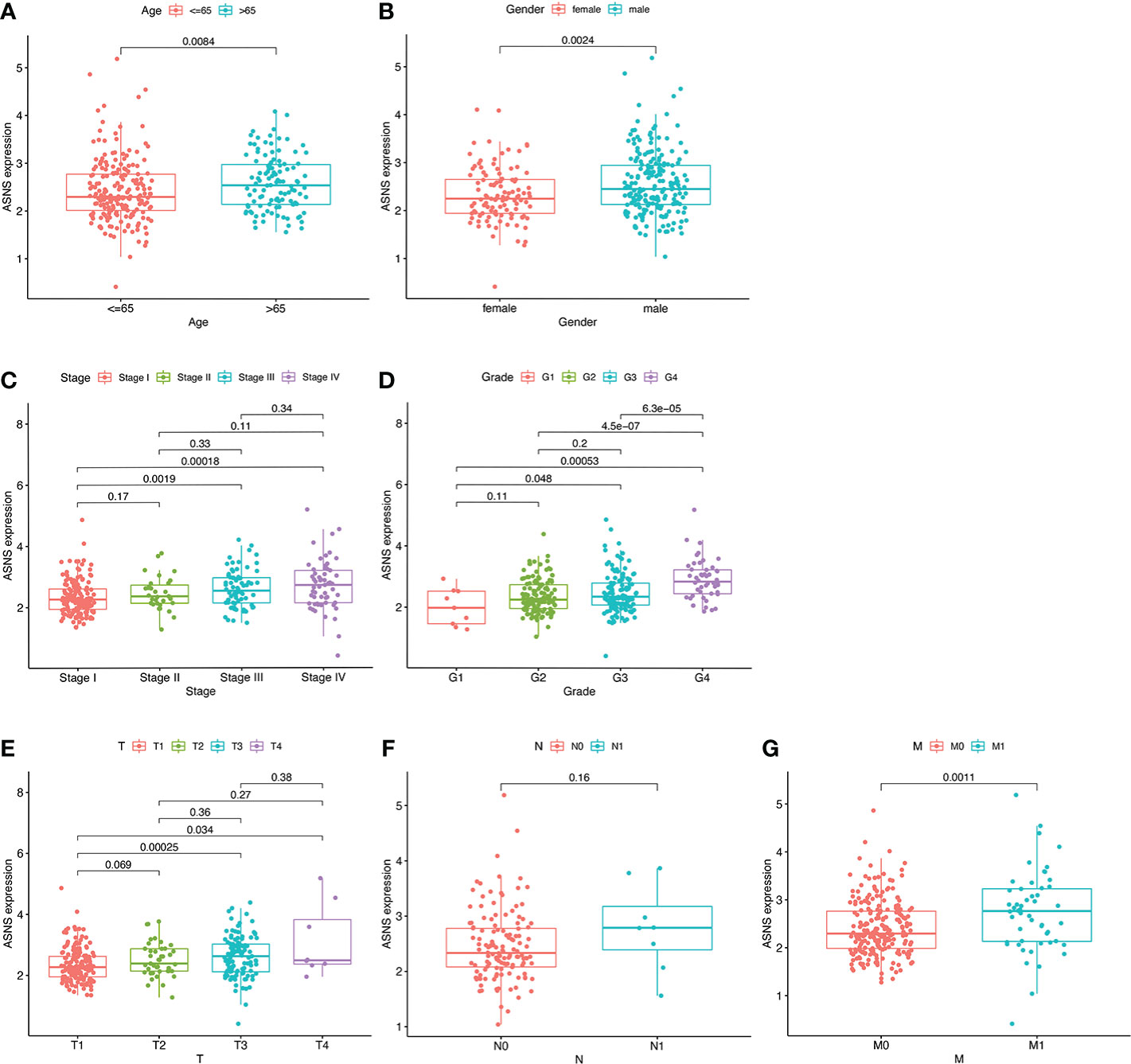
Figure 2 Expression pattern of ASNS in relation to several clinicopathological features. (A, B) ASNS expression in samples from patients stratified by age and gender. (C–G) ASNS expression in samples stratified by pathological stage, neoplasm histological grade, T stage, N stage, and M stage, respectively.
ASNS is an independent prognostic factor for ccRCC
Univariate independent prognostic analysis demonstrated that neoplasm histologic grades, pathological T&M stages, tumor stages, and the expression of ASNS were significant factors that could predict ccRCC prognosis (p < 0.001; hazard ratio [HR] > 1; Figure 3A). Meanwhile, multivariate prognostic analysis showed that ASNS could be an independent prognostic factor for ccRCC (p < 0.001; HR > 1; Figure 3B). Moreover, the receiver operating characteristic (ROC) curve was used to analyze the accuracy of ASNS to predict the survival of ccRCC. ASNS predicted the survival period of ccRCC patients at 1, 3, and 5 years, which was significant (area under the curve [AUC] > 0.6; Figure 3C). Subsequently, serial ROC analysis showed favorable diagnostic values for ASNS to predict various clinicopathological features (Figures 3D–K). Ultimately, the above results were validated using nomogram and calibration plots, which showed that ASNS had excellent potential for predicting clinicopathological features in ccRCC (Figures 3L, M).
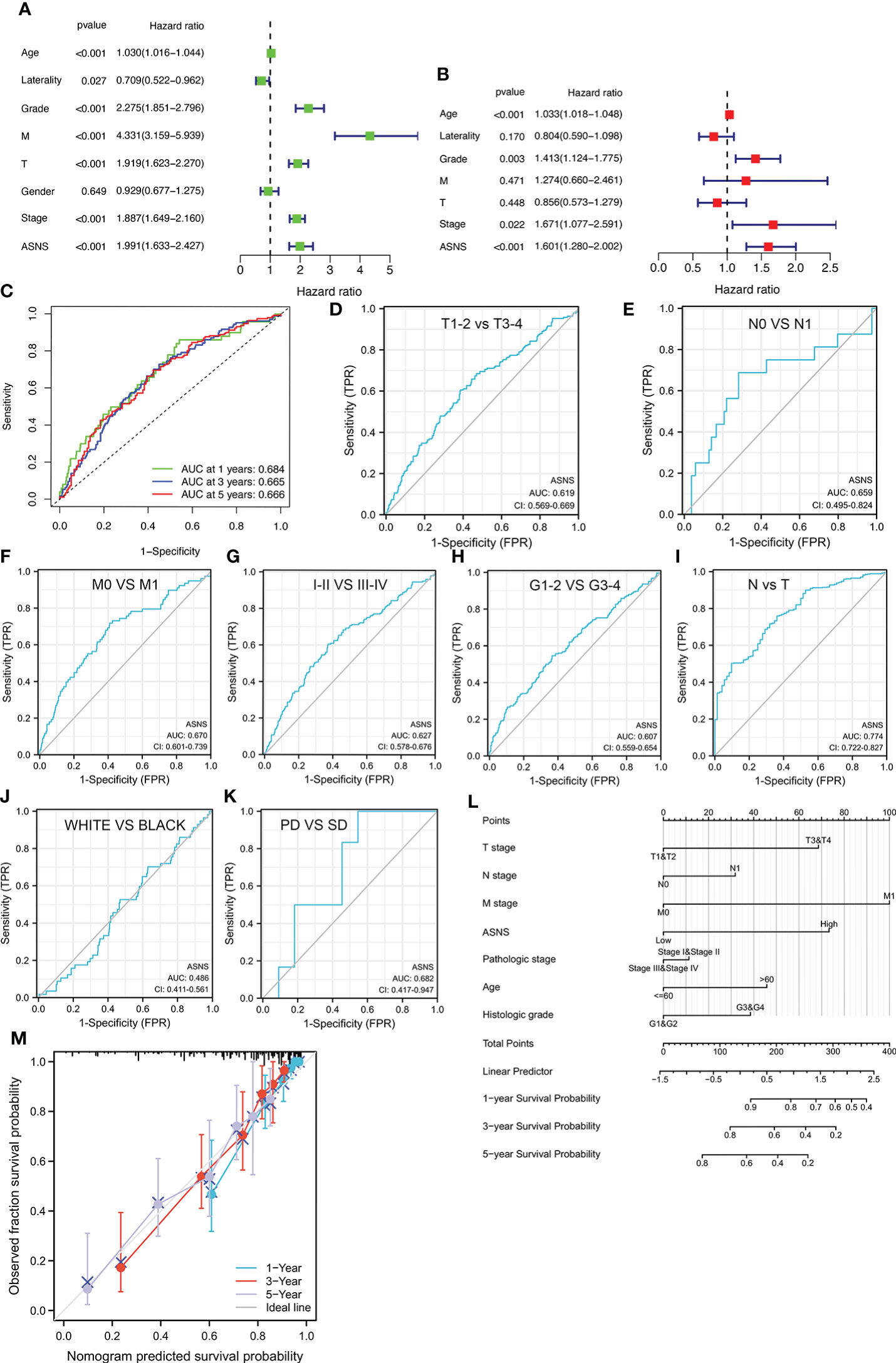
Figure 3 Identification of ASNS as an independent prognostic factor for ccRCC. (A, B) Univariate and multivariate independent prognostic analysis of ASNS. (C) Receiver operating characteristic (ROC) curve predicting the correlation between ASNS expression and OS. (D–K) ROC curve predicting the correlation between ASNS expression and clinicopathological features [T1–2 vs. T3–4, N0 vs. N1, M0 vs. M1, pathological stage I–II vs. III–IV, neoplasm histological grade 1–2 vs. 3–4, normal vs. tumor, race (white vs. black), and primary therapeutic outcome, i.e., partial disease vs. stable disease]. (L, M) Nomogram and calibration plots, respectively.
DNA methylation analysis of ASNS gene
To further explore the regulation of ASNS in ccRCC, we performed a series of methylation analyses. Methylation levels were detected at the promoter region sites of the ASNS gene (Figure 4A), and ASNS expression was inversely proportional to the methylation level (Figures 4B, C). We further determined the relationship between ASNS gene methylation level and various clinicopathological features such as pathologic TNM stages (Figures 4D–F), tumor stages (Figure 4G), and neoplasm histologic grades (Figure 4H) of ccRCC. The results demonstrated that the methylation level of ASNS gradually decreased with tumor progression. Furthermore, the lower methylation level of ASNS led to a worse prognosis, such as poorer OS rates (p < 0.001; Figures 4I–K) and poorer progression-free survival (p < 0.001; Figure 4L). The decrease in the methylation level of ASNS with tumor progression indicates that the methylation modification of ASNS plays a key regulatory role in the expression of ASNS.
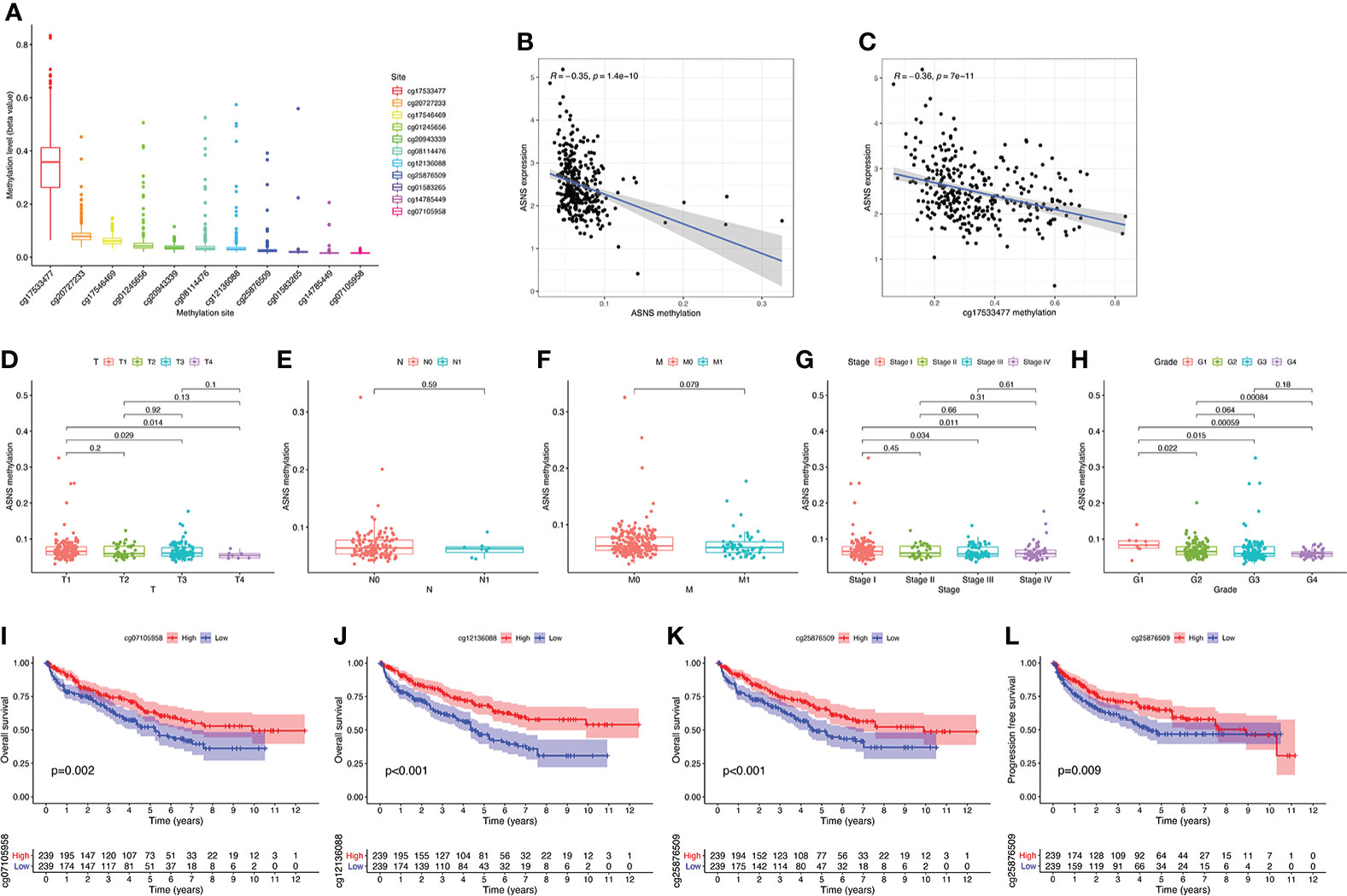
Figure 4 DNA methylation analysis of ASNS. (A) Methylation levels at different sites of ASNS. (B, C) The relationship between ASNS expression level and methylation level. (D–H) ASNS methylation in samples stratified by T stage, N stage, M stage, neoplasm histological grade, and pathological stage. (I–L) Methylation levels of ASNS on the prognosis of ccRCC (OS and progression-free survival rates).
Immune infiltration analysis of ASNS in ccRCC
ccRCC has a relatively high stromal score and immune score among common cancers (15). High immune scores and infiltration of Tregs are significantly associated with poor OS, high tumor stage, and more chances of metastases in ccRCC (16). To further investigate the relationship between ASNS expression and the immune microenvironment in ccRCC, we performed immune infiltration analysis using TIMER2.0. The immune infiltration level of non-regulatory CD4+ T cells was negatively correlated with ASNS expression (Figure 5B), whereas Tregs were positively correlated (Figure 5C). Subsequent analyses showed that higher CD4+ T-cell infiltration was associated with better prognosis (Figure 5E), and higher Tregs infiltration was associated with poorer prognosis (Figure 5F). The correlation between CD8+ T-cell infiltration and ASNS was also analyzed; however, the results were insignificant (Figures 5A, D). Finally, ASNS expression correlated positively with the expression of immune checkpoints such as CTLA4, and PD-L1 (CD274) was positive (Figures 5G, H).
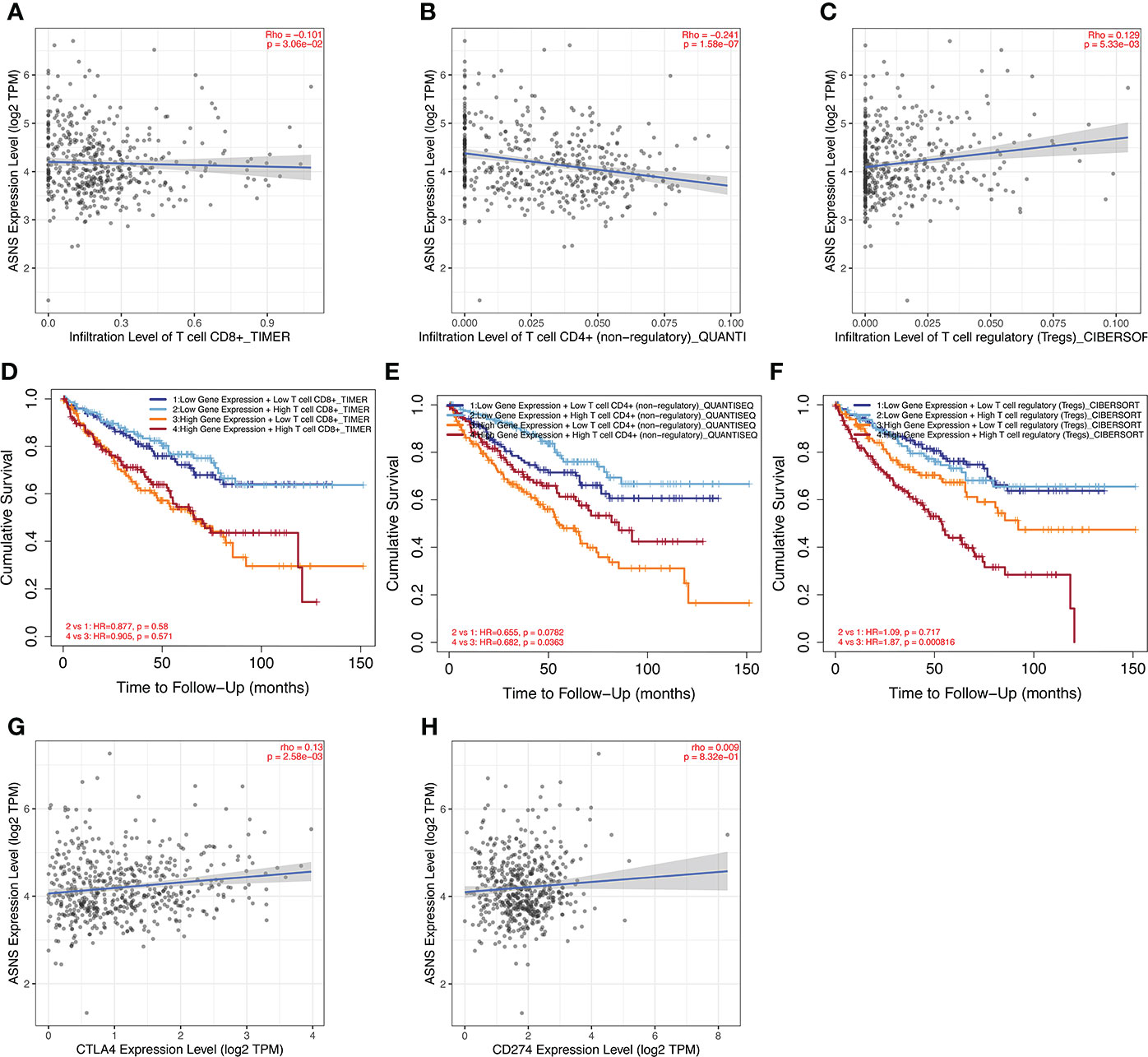
Figure 5 Immune infiltration analysis of ASNS in ccRCC. (A–C) Correlation between ASNS expression and immune cell infiltration in ccRCC. (D–F) Correlation between immune cell infiltration and prognosis. (G, H) Correlation between ASNS and expression level of immune checkpoint molecules.
Cell function and pathway analysis in ASNS
We analyzed the positively and negatively correlated genes of ASNS in ccRCC and plotted heatmaps to show the expression patterns of the top 20 ASNS-associated genes (Figure 6A). To further determine the functions of ASNS in tumor progression and prognosis, GO and KEGG enrichment analyses were performed. GO enrichment analysis found that ASNS protein may be located on the cell membrane and regulate the molecular transport in cells (q-value <0.01; Figure 6B). KEGG enrichment analysis indicated that ASNS might participate in the regulation of insulin resistance, PPAR signaling pathway, and amino acid metabolism (q-value <0.05; Figure 6C). Subsequent GSEA suggested that ASNS might be involved in CD 4+ T-cell infiltration-related signaling in ccRCC (Figures 6D, E), which is also consistent with the previous analysis results.
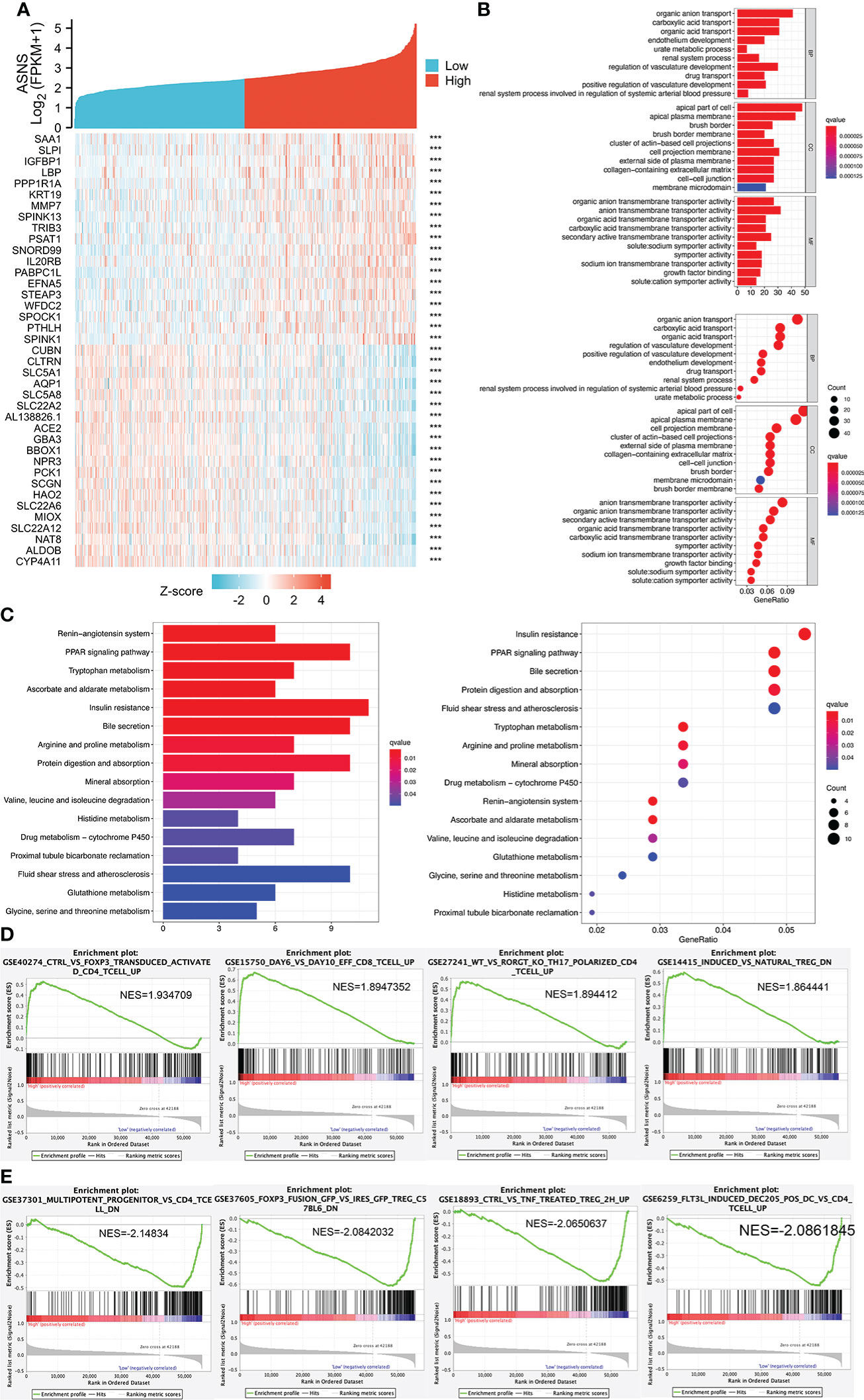
Figure 6 Cell function and pathway analysis involved in ASNS. (A) Heatmap of the expression patterns of the top 20 positively and negatively correlated genes of ASNS in ccRCC. (B) GO enrichment analysis. (C) KEGG enrichment analysis. (D, E) Gene set enrichment analysis (GSEA).
Protein–protein interaction network of ASNS
We performed a PPI network analysis of ASNS using the STRING website to explore potential interactions between ASNS and other proteins. The top 10 hub genes were selected from the PPI network, and the expression patterns and prognostic values in ccRCC were studied (Figure 7).
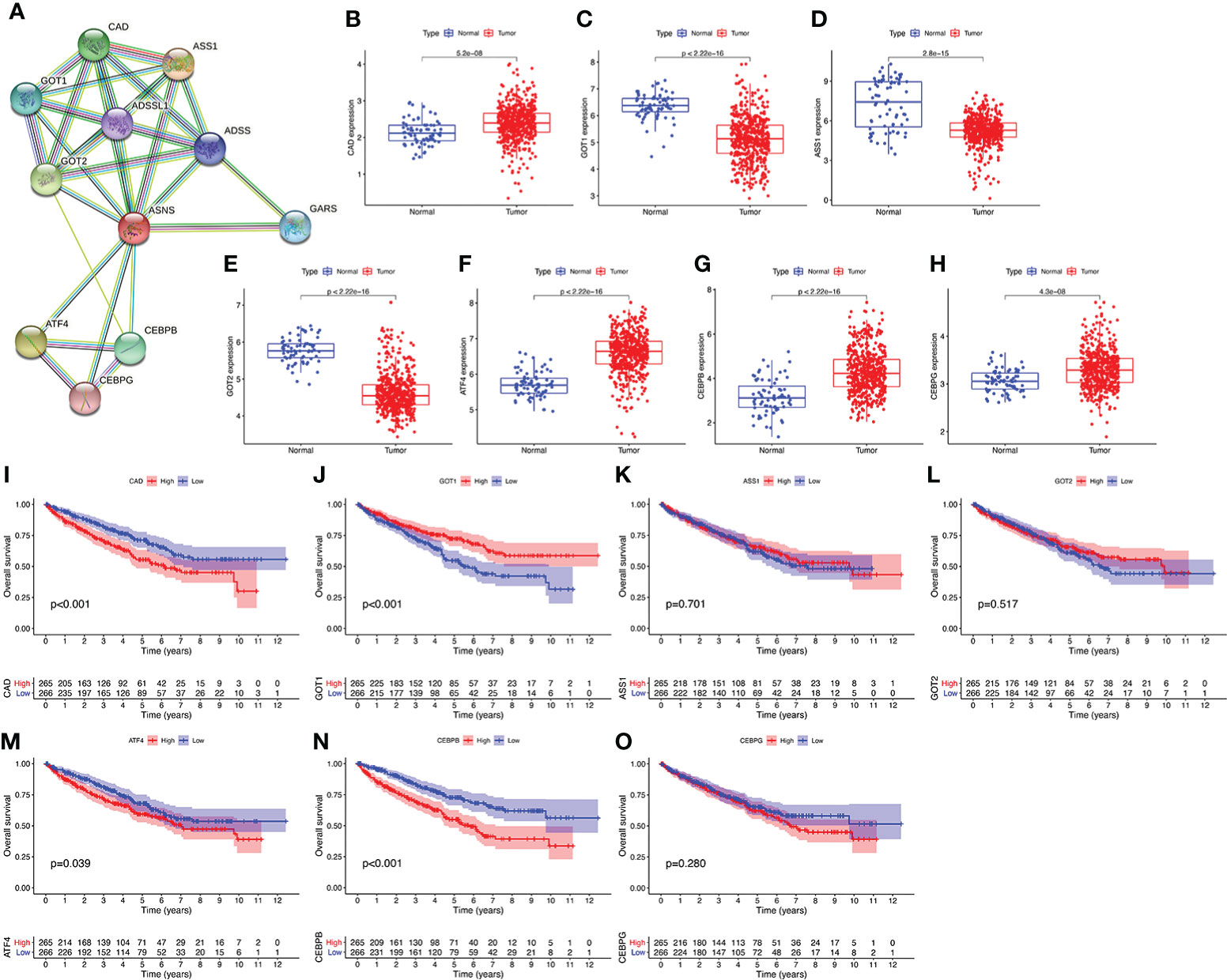
Figure 7 Protein–protein interaction (PPI) network analysis of ASNS. (A) The top 10 hub genes of the PPI network. (B–O) The expression pattern and prognostic values of these hub genes in ccRCC (the three missing genes were not found in the TCGA-KIRC database).
Validation of ASNS in cells and pathological tissues of ccRCC
To further verify the effect of ASNS on ccRCC, we evaluated the expression of ASNS in ccRCC tissues and human ccRCC cell lines. Compared with adjacent normal renal tissues, ASNS was highly expressed in ccRCC tissues (Figure 8A). ImageJ software was used to quantitatively analyze the expression of ASNS (Figures 8B–E). Finally, we evaluated the expression of ASNS protein in 786-O cells (human ccRCC) and HK-2 cells (Human Kidney-2 cells), and the results were significant (Figure 8F).
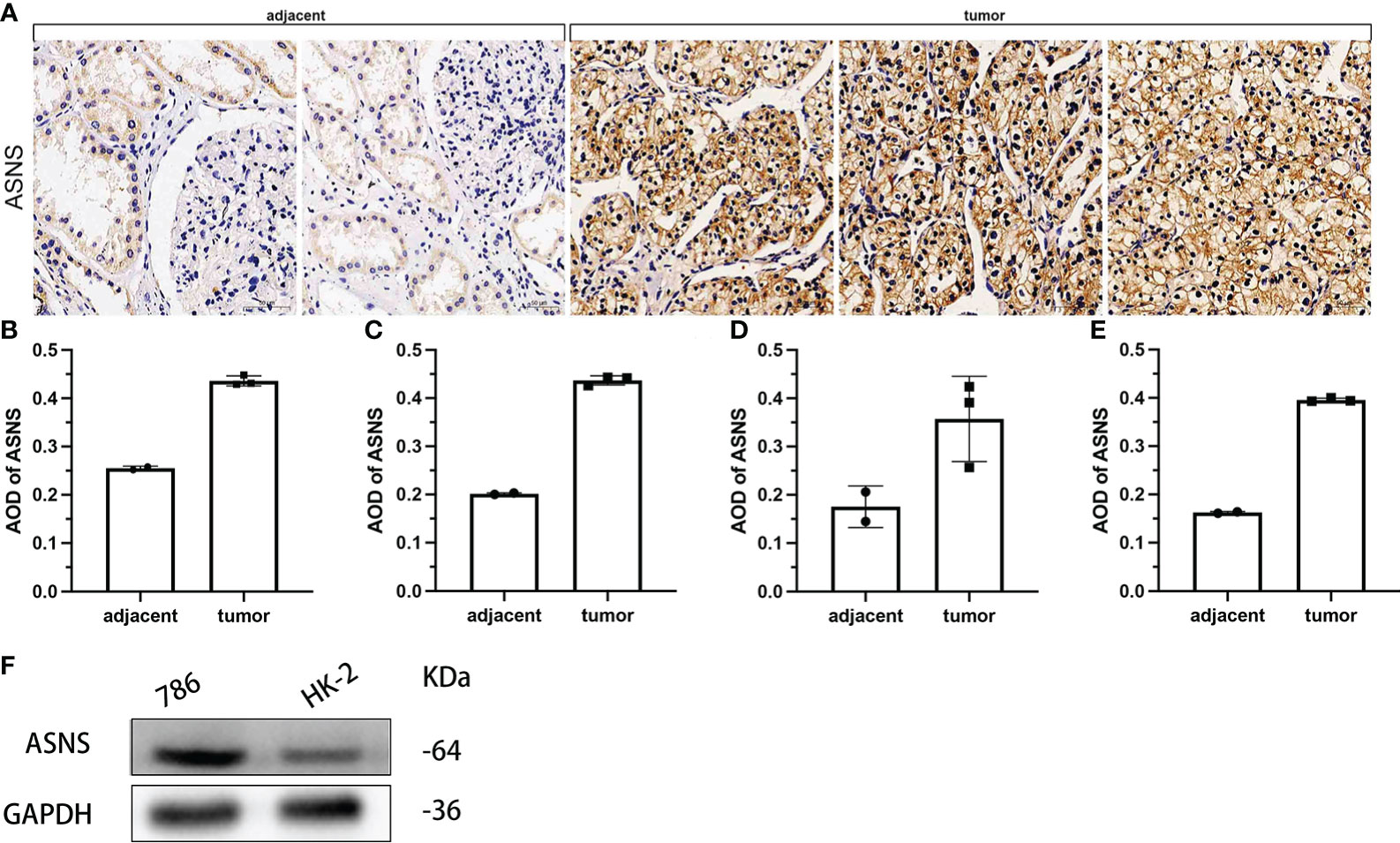
Figure 8 Validation of ASNS in cells and pathological tissues of ccRCC. (A) Results of immunohistochemical analysis of ASNS in ccRCC tissues and adjacent normal tissues. (B–E) Quantitative immunohistochemical analysis results of ASNS in four samples. (F) Western blot result of ASNS in 786-O and HK-2 cells.
In addition, the above results were further validated using the data from our cohort. It was further verified that ASNS was highly expressed in ccRCC tissues compared with adjacent tissues (p < 0.01, Table 1). Moreover, high ASNS expression was not correlated with age, gender, and tumor location (p > 0.05, Table 2), but was significantly positively associated with tumor size, T stage, the American Joint Committee on Cancer (AJCC) status, and neoplasm histological grade (p < 0.001, Table 2). These were also consistent with our previous results.
Discussion
ccRCC is one of the most common malignancies of the urinary system. Several biomarkers such as ciRS-7 (17), CA9 (18), Ki-67 (19), Bcl-2 (20), and PTEN (21) can predict ccRCC prognosis. We aimed to identify a novel biomarker to predict the prognosis of ccRCC. We found that ASNS might play a significant role in the development of ccRCC and serve as a valuable clinical prognostic biomarker of ccRCC.
The upregulation of ASNS expression responds to single or combined restrictions on many amino acids, including the most essential amino acids (22). Amino acid starvation-induced upregulation of ASNS is mediated by activating transcription factor 4 (ATF4). ASNS is the transcriptional target of ATF4, responding to amino acid starvation via the GCN2/eIF2α axis. The GCN2/eIF2α/ATF4 pathway is activated in primary solid tumors, indicating that the regulation of asparagine production in a nutrient-limited environment is essential for the progression of solid tumors (23). Maintaining intracellular asparagine levels is necessary for cancer cell growth (24). Asparagine is an important regulator of cancer cell amino acid homeostasis, anabolic metabolism, and proliferation (24). Therefore, we suspected that ASNS might play an important role in regulating the intracellular and extracellular metabolism of amino acids, thereby promoting the development of ccRCC, consistent with our results (Figure 6).
ASNS knockdown leads to cell death even in the presence of glutamine, which can be reversed by adding exogenous asparagine (25). The standard treatment for childhood acute lymphoblastic leukemia includes the infusion of bacterial ASNase as a principal component of combination chemotherapy (26). Circulating ASNase causes the rapid consumption of plasma asparagine and depletion of intracellular asparagine, starving leukemia cells and preventing their further growth (27). Hence, the growth of solid tumors might be regulated by ASNS protein levels. A study showed that in about 70% of human pancreatic ductal cancer samples, the level of ASNS protein was below the detection level, which indicates that some pancreatic tumors may be sensitive to ASNase treatment (28). In another study using ovarian cell lines, a negative correlation was observed between ASNase treatment efficacy and ASNS protein levels rather than ASNS mRNA levels (29). Pancreatic cancer cells overexpressing ASNS exhibit increased resistance to apoptosis induced by cis-diamine-dichloro platinum, which is related to the inhibition of JUN NH2-terminal kinase activation by ASNS (30). Therefore, whether ASNase treatment can inhibit tumor cells in ccRCC needs to be researched.
As shown by immunohistochemistry of human pancreatic tissues, pancreatic ASNS protein expression was largely correlated with exocrine cells (28). The ASNS protein is released from primary mouse tumors into the serum at a rate proportional to tumor growth; therefore, serum ASNS activity may be a valuable marker for the lysis of pancreatic exocrine cells (31). The secretion pattern of ASNS protein needs to be investigated in ccRCC.
Several studies on asparagine-dependent and asparagine-independent cell lines revealed the correlation between DNA methylation in the ASNS locus and ASNS expression (32, 33). High ASNS promoter methylation is associated with low ASNS expression, and 5-Aza-dC treatment enhances ASNS expression (34). In acute lymphoblastic leukemia bone marrow samples, most B cells and T cells showed methylation of the ASNS promoter, in contrast to the lack of methylation observed in brain and breast tumors (35). Akagi et al. hypothesized that ASNS methylation might be the basis for the susceptibility of acute lymphoblastic leukemia cells to ASNase chemotherapy (35). We found that the cg17533477 site of ASNS had high-level methylation in ccRCC (Figure 4A), indicating that ccRCC is more sensitive to ASNase chemotherapy. Moreover, the higher methylation level of the ASNS gene leads to a better prognosis (Figures 4I–L).
Multiple studies have shown that tumor-infiltrating immune cells regulate cancer progression and promote tumor development (36, 37). CD4+ helper T cells and cytotoxic CD8+ T cells play an important role in tumor prevention by targeting antigenic tumor cells, and CD8+ T cells are associated with better clinical outcomes and response to immunotherapy in many cancers (38–40). T cells (CD4+ T cells and CD8+ T cells) are the primary type of immune cells in ccRCC tumors (41). Effector T cells and mature dendritic cells may contribute to antitumor immune responses, whereas Tregs have the opposite effect (42), consistent with our findings (Figures 5B, C, E, F).
In our study, we analyzed the significant role of ASNS in the development of ccRCC and verified the possibility and feasibility of using it as an independent prognostic factor for ccRCC prognosis (Figure 9). Taken together, ASNS could act as an independent prognostic factor for ccRCC and might play a crucial role in tumor progression and immune cell infiltration. However, robust experimental data are not available to confirm our findings. Only preliminary analyses showed the function of ASNS in ccRCC, but certain signaling pathways in which it plays an important role remain to be studied. Due to the small number of data included in this center (only 81 cases), our validation results did not show that ASNS expression levels were associated with lymph node metastases and distant metastases. To further explore the vital role of ASNS in ccRCC, more detailed basic experimental research and clinical studies are required.
Data availability statement
The datasets presented in this study can be found in online repositories. The names of the repository/repositories and accession number(s) can be found below: https://xenabrowser.net/datapages/.
Ethics statement
The studies involving human participants were reviewed and approved by Ethics Committee and Institutional Review Board for Clinical Research of Zhongda Hospital (ZDKYSB077) (43, 44, 45, 46). This study is a retrospective study that only collected clinical data from patients, and the ethical committee approved the exemption of informed consent.
Author contributions
XG, RL, MC, WM, NF, and HC designed the study. XG and RL conducted the study, maintained the data, analyzed the data, and prepared the figures. XG, RL, WM, and NF reviewed and revised the manuscript. All authors contributed to the article and approved the submitted version.
Funding
This study was funded by The National Natural Science Foundation of China (No. 81370849, 81300472, 81070592, 81202268, 81202034); Natural Science Foundation of Jiangsu Province (BK20161434, BL2013032, BK20150642 and BK2012336). Major Project of Jiangsu Commission of Health: (No: ZD2021002); Wuxi “Taihu Talents Program” Medical Expert Team Project (No: THRCJH20200901, THRCJH20200902).
Acknowledgments
We thank Bullet Edits Limited for the linguistic editing and proofreading of the manuscript.
Conflict of interest
The authors declare that the research was conducted in the absence of any commercial or financial relationships that could be construed as a potential conflict of interest.
Publisher’s note
All claims expressed in this article are solely those of the authors and do not necessarily represent those of their affiliated organizations, or those of the publisher, the editors and the reviewers. Any product that may be evaluated in this article, or claim that may be made by its manufacturer, is not guaranteed or endorsed by the publisher.
References
1. Sung HA-O, Ferlay J, Siegel RL, Laversanne M, Soerjomataram I, Jemal A, et al. Global cancer statistics 2020: GLOBOCAN estimates of incidence and mortality worldwide for 36 cancers in 185 countries. CA Cancer J Clin (2021) 71(3):209–49. doi: 10.3322/caac.21660
2. Ljungberg B, Albiges L, Abu-Ghanem Y, Bensalah K, Dabestani S, Fernández-Pello S, et al. European Association of urology guidelines on renal cell carcinoma: The 2019 update. Eur Urol (2019) 75:799–810. doi: 10.1016/j.eururo.2019.02.011
3. Linehan WM, Schmidt LS, Crooks DR, Wei D, Srinivasan R, Lang M, et al. The metabolic basis of kidney cancer. Cancer Discov (2019) 9:1006–21. doi: 10.1158/2159-8290.CD-18-1354
4. Rossi SH, Fielding A, Blick C, Handforth C, Brown JE, Stewart GD. Setting research priorities in partnership with patients to provide patient-centred urological cancer care. Eur Urol (2019) 75:891–3. doi: 10.1016/j.eururo.2019.03.008
5. Richards NG, Kilberg MS. Asparagine synthetase chemotherapy. Annu Rev Biochem (2006) 75:629–54. doi: 10.1146/annurev.biochem.75.103004.142520
6. McDonald ER 3rd, de Weck A, Schlabach MR, Billy E, Mavrakis KJ, Hoffman GR, et al. Project DRIVE: A compendium of cancer dependencies and synthetic lethal relationships uncovered by Large-scale, deep RNAi screening. Cell (2017) 170(3):577–92.e10. doi: 10.1016/j.cell.2017.07.005
7. Knott S, Wagenblast E, Khan S, Kim SY, Soto M, Wagner M, et al. Asparagine bioavailability governs metastasis in a model of breast cancer. Nature (2018) 554(7692):378–81. doi: 10.1038/nature25465
8. Li T, Fu J, Zeng Z, Cohen D, Li J, Chen Q, et al. TIMER2.0 for analysis of tumor-infiltrating immune cells. Nucleic Acids Res (2020) 48(W1):W509–14. doi: 10.1093/nar/gkaa407
9. Szklarczyk D, Gable AL, Lyon D, Junge A, Wyder S, Huerta-Cepas J, et al. STRING v11: protein-protein association networks with increased coverage, supporting functional discovery in genome-wide experimental datasets. Nucleic Acids Res (2019) 47:D607–13. doi: 10.1093/nar/gky1131
10. Li C, Tang Z, Zhang W, Ye Z, Liu F. GEPIA2021: Integrating multiple deconvolution-based analysis into GEPIA. Nucleic Acids Res (2021) 49:W242–6. doi: 10.1093/nar/gkab418
11. Subramanian A, Tamayo P, Mootha VK, Mukherjee S, Ebert BL, Gillette MA, et al. Gene set enrichment analysis: A knowledge-based approach for interpreting genome-wide expression profiles. Proc Natl Acad Sci USA (2005) 102:15545–50. doi: 10.1073/pnas.0506580102
12. Liu R-J, Xu Z-P, Li S-Y, Yu J-J, Feng N-H, Xu B, et al. BAP1-related ceRNA (NEAT1/miR-10a-5p/SERPINE1) promotes proliferation and migration of kidney cancer cells. Front Oncol (2022) 12:852515. doi: 10.3389/fonc.2022.852515
13. Schneider CA, Rasband WS, Eliceiri KW. NIH Image to ImageJ: 25 years of image analysis. Nat Methods (2012) 9(7):671–5. doi: 10.1038/nmeth.2089
14. Rangan GK, Tesch GH. Quantification of renal pathology by image analysis (Methods in renal research). Nephrology (2007) 12(6):553–8. doi: 10.1111/j.1440-1797.2007.00855.x
15. Yoshihara K, Shahmoradgoli M, Martínez E, Vegesna R, Kim H, Torres-Garcia W, et al. Inferring tumour purity and stromal and immune cell admixture from expression data. Nat Commun (2013) 4:2612. doi: 10.1038/ncomms3612
16. Wang Y, Yang J, Zhang Q, Xia J, Wang Z. Extent and characteristics of immune infiltration in clear cell renal cell carcinoma and the prognostic value. Transl Androl Urol (2019) 8(6):609–18. doi: 10.21037/tau.2019.10.19
17. Mao W, Wang K, Xu B, Zhang H, Sun S, Hu Q, et al. ciRS-7 is a prognostic biomarker and potential gene therapy target for renal cell carcinoma. Mol Cancer (2021) 20(1):142. doi: 10.1186/s12943-021-01443-2
18. Bui MH, Visapaa H, Seligson D, Kim H, Han KR, Huang Y, et al. Prognostic value of carbonic anhydrase IX and KI67 as predictors of survival for renal clear cell carcinoma. J Urol (2004) 171:2461–6. doi: 10.1097/01.ju.0000116444.08690.e2
19. Gayed BA, Youssef RF, Bagrodia A, Darwish OM, Kapur P, Sagalowsky A, et al. Ki67 is an independent predictor of oncological outcomes in patients with localized clear-cell renal cell carcinoma. BJU Int (2014) 113(4):668–73. doi: 10.1111/bju.12263
20. Itoi T, Yamana K, Bilim V, Takahashi K, Tomita F. Impact of frequent bcl-2 expression on better prognosis in renal cell carcinoma patients. Br J Cancer (2004) 90(1):200–5. doi: 10.1038/sj.bjc.6601454
21. Shin Lee J, Seok Kim H, Bok Kim Y, Cheol Lee M, Soo Park C. Expression of PTEN in renal cell carcinoma and its relation to tumor behavior and growth. J Surg Oncol (2003) 84(3):166–72. doi: 10.1002/jso.10302
22. Gong SS, Guerrini L, Basilico C. Regulation of asparagine synthetase geneexpression by amino acid starvation. Mol Cell Biol (1991) 11(12):6059–66. doi: 10.1128/mcb.11.12.6059-6066.1991
23. Horiguchi M, Koyanagi S, Okamoto A, Suzuki SO, Matsunaga N, Ohdo S. Stress-regulated transcription factor ATF4 promotes neoplastic transformation by suppressing expression of the INK4a/ARF cell senescence factors. Cancer Res (2012) 72(2):395–401. doi: 10.1158/0008-5472.CAN-11-1891
24. Krall AS, Xu S, Graeber TG, Braas D, Christofk HR. Asparagine promotes cancer cell proliferation through use as an amino acid exchange factor. Nat Commun (2016) 7:11457. doi: 10.1038/ncomms11457
25. Zhang J, Fan J, Venneti S, Cross JR, Takagi T, Bhinder B, et al. Asparagine plays a critical role in regulating cellular adaptation to glutamine depletion. Mol Cell (2014) 56(2):205–18. doi: 10.1016/j.molcel.2014.08.018
26. Pieters R, Hunger SP, Boos J, Rizzari C, Silverman L, Baruchel A, et al. L-asparaginase treatment in acute lymphoblastic leukemia: A focus on erwinia asparaginase. Cancer (2011) 117(2):238–49. doi: 10.1002/cncr.25489
27. Avramis VI. Asparaginases: biochemical pharmacology and modes of drug resistance. Anticancer Res (2012) 32(7):2423–37.
28. Dufour E, Gay F, Aguera K, Scoazec JY, Horand F, Lorenzi PL, et al. Pancreatic tumor sensitivity to plasma l-asparagine starvation. Pancreas (2012) 41(6):940–8. doi: 10.1097/MPA.0b013e318247d903
29. Lorenzi PL, Llamas J, Gunsior M, Ozbun L, Reinhold WC, Varma S, et al. Asparagine synthetase is a predictive biomarker of l-asparaginase activity in ovarian cancer cell lines. Mol Cancer Ther (2008) 7(10):3123–8. doi: 10.1158/1535-7163.MCT-08-0589
30. Cui H, Darmanin S, Natsuisaka M, Kondo T, Asaka M, Shindoh M, et al. Enhanced expression of asparagine synthetase under glucose-deprived conditions protects pancreatic cancer cells from apoptosis induced by glucose deprivation and cisplatin. Cancer Res (2007) 67(7):3345–55. doi: 10.1158/0008-5472.CAN-06-2519
31. Cooney DA, King VD, Cable RG, Taylor JB, Wodinsky I. L-asparagine synthetase in serum as a marker for neoplasia. Cancer Res (1976) 36(9 pt.1):3238–45. doi:
32. Peng H, Shen N, Qian L, Sun XL, Koduru P, Goodwin LO, et al. Hypermethylation of CpG islands in the mouse asparagine synthetase gene: relationship to asparaginase sensitivity in lymphoma cells. partial methylation in normal cells. Br J Cancer (2001) 85(6):930–5. doi: 10.1054/bjoc.2001.2000
33. Worton KS, Kerbel RS, Andrulis IL. Hypomethylation and reactivation of the asparagine synthetase gene induced by l-asparaginase and ethyl methanesulfonate. Cancer Res (1991) 51(3):985–9
34. Ren Y, Roy S, Ding Y, Iqbal J, Broome JD. Methylation of the asparagine synthetase promoter in human leukemic cell lines is associated with a specific methyl binding protein. Oncogene (2004) 23(22):3953–61. doi: 10.1038/sj.onc.1207498
35. Akagi T, Yin D, Kawamata N, Bartram CR, Hofmann WK, Wolf I, et al. Methylation analysis of asparagine synthetase gene in acute lymphoblastic leukemia cells. Leukemia (2006) 20(7):1303–6. doi: 10.1038/sj.leu.2404216
36. Grivennikov SI, Greten FR, Karin M. Immunity, inflammation, and cancer. Cell (2010) 140(6):883–99. doi: 10.1016/j.cell.2010.01.025
37. Kitamura T, Qian BZ, Pollard JW. Immune cell promotion of metastasis. Nat Rev Immunol (2015) 15(2):73–86. doi: 10.1038/nri3789
38. Vesely MD, Kershaw MH, Schreiber RD, Smyth MJ. Natural innate and adaptive immunity to cancer. Annu Rev Immunol (2011) 29:235–71. doi: 10.1146/annurev-immunol-031210-101324
39. Yao J, Xi W, Zhu Y, Wang H, Hu X, Guo J, et al. Checkpoint molecule PD-1-assisted CD8+ T lymphocyte count in tumor microenvironment predicts overall survival of patients with metastatic renal cell carcinoma treated with tyrosine kinase inhibitors. Cancer Manag Res (2018) 10:3419–31. doi: 10.2147/CMAR.S172039
40. Pagès F, Kirilovsky A, Mlecnik B, Asslaber M, Tosolini M, Bindea G, et al. In situ cytotoxic and memory T cells predict outcome in patients with early-stage colorectal cancer. J Clin Oncol (2009) 27(35):5944–51. doi: 10.1200/JCO.2008.19.6147
41. Chevrier S, Levine JH, Zanotelli VRT, Silina K, Schulz D, Bacac M, et al. An immune atlas of clear cell renal cell carcinoma. Cell (2017) 169:736–49.e18. doi: 10.1016/j.cell.2017.04.016
42. Seledtsov VI, Goncharov AG, Seledtsova GV. Clinically feasible approaches to potentiating cancer cell-based immunotherapies. Hum Vaccines Immunotherapeut (2015) 11(4):851–69. doi: 10.1080/21645515.2015.1009814
43. Mao W, Wang K, Wu Y, Ni J, Zhang H, Wang Y, et al. Prognostic significance of modified advanced lung cancer inflammation index in patients with renal cell carcinoma undergoing laparoscopic nephrectomy: A multi-institutional, propensity score matching cohort study. Front Nutr (2022) 8:781647. doi: 10.3389/fnut.2021.781647
44. Zhang G, Zhang L, Sun S, Chen M. Identification of a novel defined immune-Autophagy-Related gene signature associated with clinical and prognostic features of kidney renal clear cell carcinoma. Front Mol Biosci (2021) 8:790804. doi: 10.3389/fmolb.2021.790804
45. He T, Zhu W, Wang C, Lu H, Wu T, Pan K, et al. Relationship between pre-operative blood glucose level and length of hospital stay in patients with renal cell carcinoma undergoing laparoscopic nephrectomy. Front Surg (2021) 8:659365. doi: 10.3389/fsurg.2021.659365
Keywords: clear cell renal cell carcinoma, asparagine synthase, tumor microenvironment, immune infiltration, prognosis
Citation: Gan X, Liu R, Cheng H, Mao W, Feng N and Chen M (2022) ASNS can predict the poor prognosis of clear cell renal cell carcinoma. Front. Oncol. 12:882888. doi: 10.3389/fonc.2022.882888
Received: 24 February 2022; Accepted: 19 July 2022;
Published: 16 August 2022.
Edited by:
Takeshi Yuasa, Japanese Foundation for Cancer Research, JapanReviewed by:
Dongjun Lee, Pusan National University, South KoreaSimin Li, Southern Medical University, China
Copyright © 2022 Gan, Liu, Cheng, Mao, Feng and Chen. This is an open-access article distributed under the terms of the Creative Commons Attribution License (CC BY). The use, distribution or reproduction in other forums is permitted, provided the original author(s) and the copyright owner(s) are credited and that the original publication in this journal is cited, in accordance with accepted academic practice. No use, distribution or reproduction is permitted which does not comply with these terms.
*Correspondence: Hong Cheng, TmptdWNoc3NAMTYzLmNvbQ==; Ninghan Feng, bi5mZW5nQG5qbXUuZWR1LmNu; Weipu Mao, bWFvd2VpcHVAdG9uZ2ppLmVkdS5jbg==; Ming Chen, bWluZ2NoZW5zZXVAMTI2LmNvbQ==
†These authors have contributed equally to this work and share first authorship