- 1Beijing Key Laboratory of Molecular Targeted Diagnosis and Therapy in Nuclear Medicine, Department of Nuclear Medicine, Peking Union Medical College Peking Union Medical College (PUMC) Hospital, Chinese Academy of Medical Science and Peking Union Medical College (PUMC), Beijing, China
- 2Nanfang PET Center, Nanfang Hospital, Southern Medical University, Guangzhou, China
Background: This is a pilot study of radiomics based on 68Ga-NOTA-PRGD2 [NOTA-PEG4-E[c(RGDfK)]2)] and 18F-FDG PET/CT to (i) evaluate the diagnostic efficacy of radiomics features of 68Ga-NOTA-PRGD2 PET in the differential diagnosis of benign and malignant pulmonary space-occupying lesions and (ii) compare the diagnostic efficacy of multi-modality and multi-probe images.
Methods: We utilized a dataset of 48 patients who participated in 68Ga-NOTA-PRGD2 PET/CT and 18F-FDG PET/CT clinical trials to extract image features and evaluate their diagnostic efficacy in the differentiation of benign and malignant lesions by the Mann-Whitney U test. After feature selection with sequential forward selection, random forest models were developed with tenfold cross-validation. The diagnostic performance of models based on different image features was visualized by receiver operating characteristic (ROC) curves and compared by permutation tests.
Results: Fourteen of the 68Ga-NOTA-PRGD2 PET features between benign and malignant pulmonary space-occupying lesions had significant differences (P<0.05, Mann-Whitney U test). Eighteen of the 68Ga-NOTA-PRGD2 PET features demonstrated higher AUC values than all CT features in the differential diagnosis of pulmonary lesions. The AUC value (0.908) of the three-modal feature model was significantly higher (P<0.05, permutation test) than those of the single- and dual-modal models.
Conclusion: 68Ga-NOTA-PRGD2 PET features have better diagnostic capacity than CT features for pulmonary space-occupying lesions. The combination of multi-modality and multi-probe images can improve the diagnostic efficiency of models. Our preliminary clinical hypothesis of using radiomics based on 68Ga-NOTA-PRGD2 PET images and multimodal images as a diagnostic tool warrants further validation in a larger multicenter sample size.
Introduction
18F-fluorodeoxyglucose (18F-FDG) positron emission tomography/computed tomography (PET/CT), a technology that integrates functional and anatomical imaging, has been applied widely in the discovery, identification and prognostic evaluation of pulmonary space-occupying lesions (1). Cells in abnormal proliferation, infection and inflammation can accumulate the glucose analogue 18F-FDG, and malignant lesions usually showed higher 18F-FDG uptake than benign lesions. Rise of glucose metabolism can be captured by 18F-FDG PET and 18F-FDG PET/CT has been proved to be valuable in the diagnosis and differentiation of pulmonary space-occupying lesions (2).
However, 18F-FDG is not specific enough as an imaging agent. For one thing, inflammatory cells can show 18F-FDG uptake (3, 4), resulting in a high standardized uptake value (SUV) and false positives (5, 6). For another, some malignant tumors with a high degree of differentiation and low metabolism can show low 18F-FDG uptake, resulting in false negatives (5, 7).
Integrin receptor imaging has been a promising nuclear medicine imaging method for the diagnosis of lung cancer from pulmonary space-occupying lesions (8). The integrin family plays an important role in a variety of physiological and pathological processes (9). Among them, the integrin receptor αvβ3 is a key molecule involved in the process of tumor angiogenesis, invasion and metastasis (10). Arginine-glycine-aspartic acid (RGD) tripeptide sequences, as one kind of αvβ3 ligand, could reveal the presence of increased angiogenesis in the tumor microenvironment of NSCLC in PET/CT imaging (11). Zheng et al. found that SUVs of 68Ga-labeled RGD dimer 68Ga-NOTA-PRGD2 PET/CT had better specificity but lower sensitivity than 18F-FDG PET/CT in the differential diagnosis of benign and malignant lesions (12).
Since the concept of ‘radiomics’ was proposed in 2012 (13), radiomics analysis based on PET images has been applied to identify lung-occupying lesions before treatment (14).Previous studies have proved the value of radiomics methods based on CT or 18F-FDG PET/CT images (15). However, the diagnostic value of radiomics features of 68Ga-NOTA-PRGD2 PET images has not been evaluated, and we are curious about how it compare to radiomics methods based on CT or 18F-FDG PET/CT. Furthermore, it would be rather valuable to investigate whether multi-modality and multi-probe radiomics method can make a difference in discriminating malignant from benign lesions.
This was the first study performed to extract and evaluate the diagnostic efficacy of radiomics features of 68Ga-NOTA-PRGD2 comparing with that of CT or 18F-FDG PET/CT in discriminating benign and malignant pulmonary space-occupying lesions. And this is also pilot in establishing and comparing the diagnostic efficacy of radiomics models based on multi-modality and multi-probe images.
Materials and Methods
Patients
The study was approved by the Peking Union Medical College Hospital Medical Ethics Committee, and the requirement for informed consent was waived. Forty-eight patients with pulmonary space-occupying lesions in Peking Union Medical College Hospital from 2011 to 2013 were enrolled, and all participated in 68Ga-NOTA-PRGD2 PET/CT and 18F-FDG PET/CT clinical trials. The pulmonary space-occupying lesions of all included patients were confirmed by pathological diagnosis.
The inclusion criteria were as follows: (a) a single clearly identifiable lung lesion with a volume > 3 cm3 on CT and PET images; (b) good image quality; (c) confirmed by a clear pathological diagnosis; and (d) did not receive radiation therapy or chemotherapy prior to PET/CT scanning. The exclusion criteria were as follows: image distortion, motion artifacts, or metal artifacts.
Image Acquisition and Reconstruction
PET/CT scanning was performed with a Siemens Biograph 128 mCT X PET/CT. All patients were intravenously injected with 68Ga-NOTA-PRGD2 of approximately 111 MBq and then underwent CT scanning (120 kV, 50 mAs, pitch 1:1, layer thickness 3 mm, interval 3 mm, matrix 512×512). PET scanning was performed 30 ± 10 minutes after injection (reconstruction method using TrueX, the layer thickness was 3 mm, and the matrix was 168×168). Standard routine 18F-FDG PET/CT examinations were performed within 1 week. After fasting for at least 6 hours, all patients received low-dose CT and PET scanning 1 hour after an injection of 7.4 MBq/kg 18F-FDG.
Image Segmentation and Feature Extraction
Imaging data were imported into the LIFEx v5.10 (LIFExsoft) platform (16), and an experienced physician manually drew the volume of interest (VOI) layer by layer along the contour of lesions on the PET and CT images. The VOIs (see Figure 1) were checked and confirmed by another experienced physician. Basic features, shape features (roundness, density, volume, etc.), and texture features (first-order texture features, gray-level co-occurrence matrix (GLCM) features, gray-level run-length matrix (GLRLM) features, neighborhood gray-level different matrix (NGLMD) features, and gray-level zone length matrix (GLZLM) features) of the VOIs of PET and CT images were extracted (see Table 1). Among them, PET features were extracted in the range of SUV values 0-20, and the interval was 64. CT features were extracted in the range of -1500~1000 HU (Hounsfield unit), and the interval was 400. Filtering and smoothing were not used in image processing.
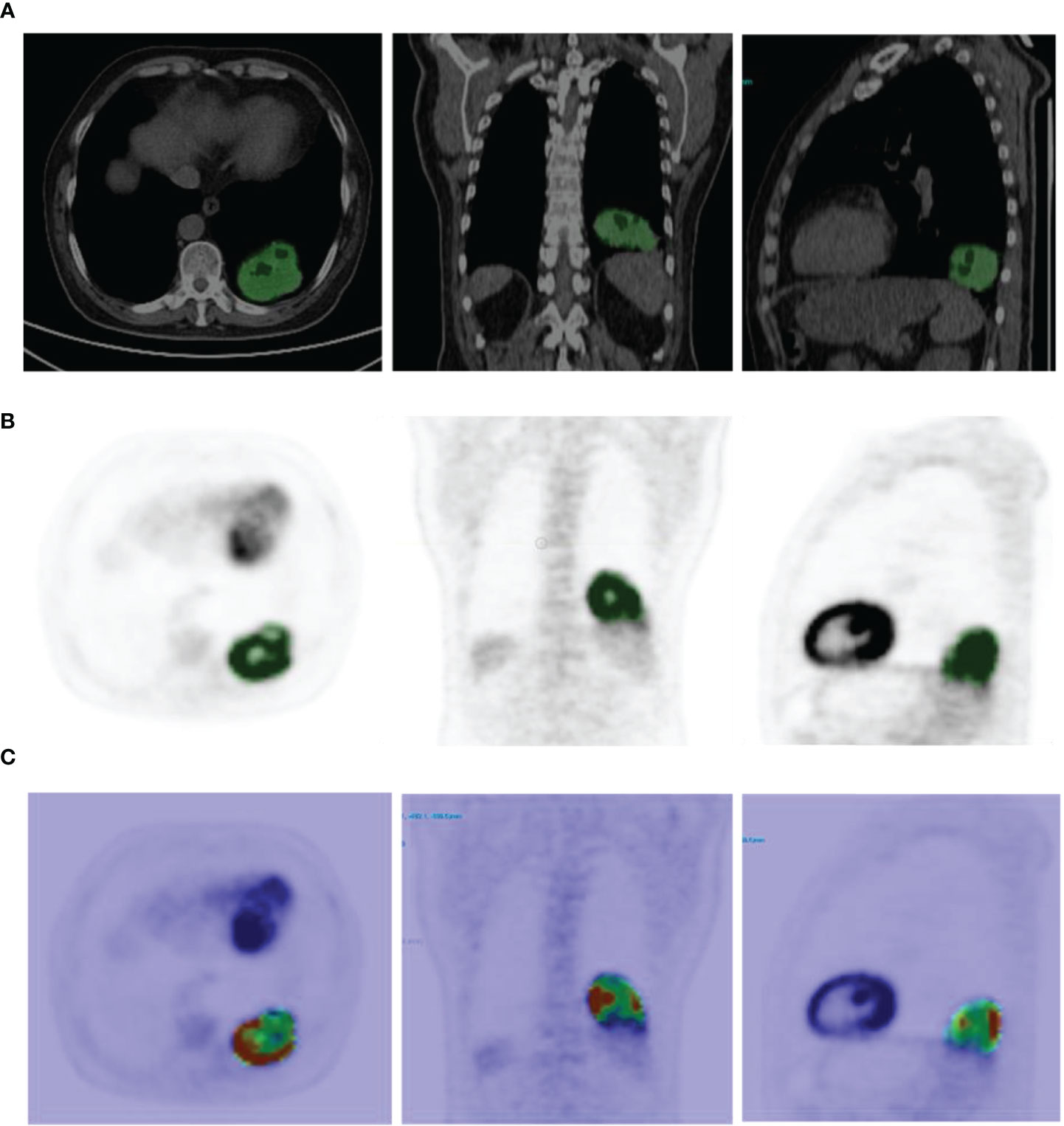
Figure 1 (A) Example of CT image segmentation (the green area is the VOI drawn, the same as below). (B) Example of 68Ga-NOTA-PRGD2 PET image segmentation. (C) Example of feature map extracted (GLCM Dissimilarity).
Data Analysis and Model Establishment
R 3.5.1 was used to analyze all data. The analysis process was as follows (see Figure 2) (1). Feature evaluation: The Mann-Whitney U test was used to compare differences in features between the benign and malignant groups. Correlations were assessed between 18F-FDG PET features and 68Ga-NOTA-PRGD2 PET features. A single-factor logistic model was established for all radiomics features, and the AUC value of each model was calculated to evaluate the diagnostic efficacy of each feature. (2) Data preprocessing: To avoid some features overpowering others, each feature was standardized (Z-score normalization). (3) Model establishment: This study used the random forest algorithm for modeling. CT features, 18F-FDG PET features, 68Ga-NOTA-PRGD2 PET features, CT & 18F-FDG PET features, CT & 68Ga-NOTA-PRGD2 PET features, 18F-FDG PET & 68Ga-NOTA-PRGD2 PET features, and a combination of all features were used to establish random forest models to predict benign and malignant lesions. The sequence forward selection algorithm was used in the feature selection process. The average AUC value, accuracy, sensitivity, and specificity of the models were evaluated by 1000 times 10-fold cross-validation. The permutation test was used to evaluate the significance of the difference in the mean AUCs of different models.
Results
Patient Characteristics
A total of 48 patients were included in this study, of which 23 (47.9%) were men and 25 (52.1%) were women, with an average age of 55.0 years (24~78 years). Among them, 36 cases (75%) were malignant, and 12 cases (25%) were benign. The pathological types were 22 cases of adenocarcinoma, 8 cases of squamous cell carcinoma, 2 cases of malignant mesothelioma, 2 cases of lymphoma, 2 cases of metastasis, 9 cases of chronic inflammation, 1 case of thymoma (AB type), 1 case of hemangioma, and 1 case of epithelioid hemangioendothelioma (see Table 2).
Feature Evaluation
The predictive value (AUC values) of features for benign and malignant lung lesions and the comparison of features between the two groups are shown in Supplementary Table 1. Fourteen of the 68Ga-NOTA-PRGD2 PET features between benign and malignant pulmonary space-occupying lesions had significant differences (P<0.05, Mann-Whitney U test). The 18F-FDG PET feature with the best predictive effect was GLZLM SZLGE with an AUC value of 0.794, the 68Ga-NOTA-PRGD2 PET feature with the best predictive effect was GLCM homogeneity with an AUC value of 0.788, and the CT feature with the best predictive effect was HUmax with an AUC value of 0.660. Eighteen of the 68Ga-NOTA-PRGD2 PET features demonstrated higher AUC values than all CT features in the differential diagnosis of pulmonary lesions. The image feature correlation coefficient map (Figure 3) showed that CT, 18F-FDG PET and 68Ga-NOTA-PRGD2 PET images had certain correlations, but these correlations were lower than the correlations of features within certain modalities.
Comparison of the Models for Differentiating Benign and Malignant Lesions
The features included in each model and the AUC, accuracy, sensitivity, specificity and significance test results of these models are shown in Tables 3, 4. The AUC values of the benign and malignant identification models constructed based on CT features, 18F-FDG PET features, 68Ga-NOTA-PRGD2 PET features, CT & 18F-FDG PET features, CT & 68Ga-NOTA-PRGD2 PET features, 18F-FDG PET & 68Ga-NOTA-PRGD2 PET features, and the combination of all were 0.870, 0.895, 0.853, 0.903, 0.876, 0.892 and 0.908; the accuracy rates were 0.770, 0.787, 0.758, 0.805, 0.802, 0.811 and 0.828; the sensitivities were 0.937, 0.932, 0.915, 0.902, 0.943, 0.924 and 0.948; and the specificities were 0.267, 0.351, 0.285, 0.519, 0.378, 0.473, 0.467, respectively. The three-modal image model had a higher AUC than the dual-modal image models in all cases (P<0.05), and the dual-modal image models had a higher AUC than the single-modal image models as its component in most cases (P<0.05). The 18F-FDG PET model had a higher AUC than the 68Ga-NOTA-PRGD2 PET feature model (P<0.05). The smoothed receiver operating characteristic (ROC) curves of all models are shown in Figure 4.
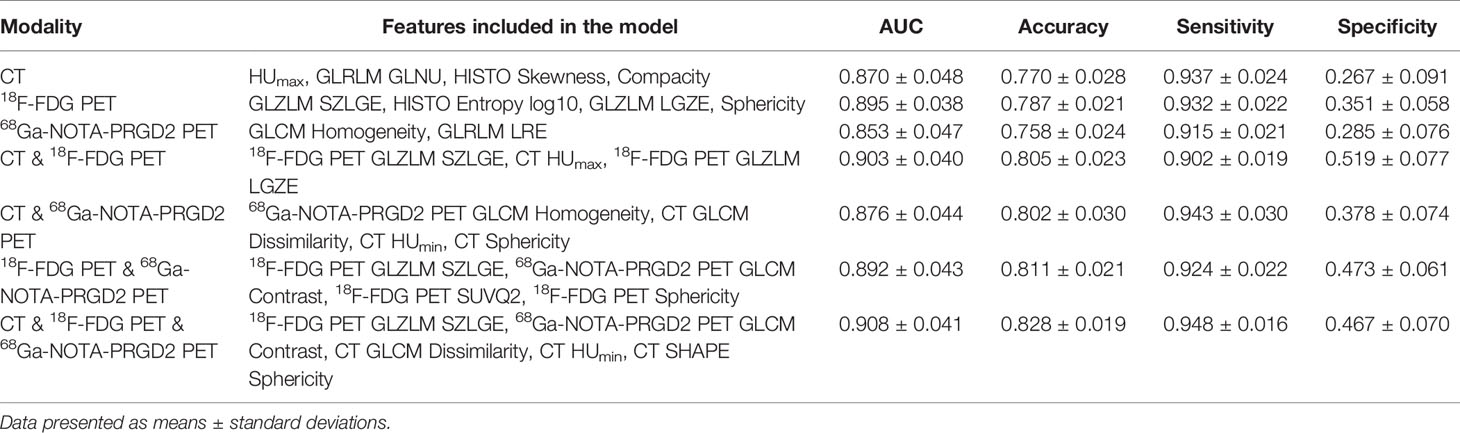
Table 3 Feature composition and evaluation results of models for the differentiation of benign and malignant lesions.
Discussion
Lung cancer is the most common type of malignant lung space-occupying lesion and the main cause of cancer mortality (17). The early diagnosis and treatment of lung cancer from all forms of lung space-occupying lesions have been crucial for the success of surgical resection and extension of survival time (18, 19).
At present, there have been a number of studies on 18F-FDG PET/CT radiomics to assist in the differentiation of pulmonary space-occupying lesions (20–25), indicating the excellent value of radiomics in nuclear medicine imaging. It was generally considered that the 18F-FDG PET & CT combined model performed better than the PET feature model or CT feature model, and the PET feature model was better than the CT feature model (26). Zhang et al. (27) showed that the AUC of the 18F-FDG PET & CT model was higher than that of the CT model (P = 0.018), and the AUC of the 18F-FDG PET model was higher than that of the CT model (0.874 vs. 0.820) but without a statistically significant difierence. Kang et al. reported that the AUC of the 18F-FDG PET model was higher than that of the CT model (0.88 vs. 0.74) (22). 68Ga-NOTA-PRGD2 PET/CT, an imaging method with dimeric RGD peptide-binding integrin receptors, showed a similar sensitivity and higher specificity than 18F-FDG PET/CT in the detection and differentiation of lung lesions (12). However, to the best of our knowledge, there have been no radiomics studies on integrin receptor imaging in lung lesions.
In our study, a variety of intensity, shape and texture features were extracted from the images of 48 patients who underwent 68Ga-NOTA-PRGD2 PET/CT and 18F-FDG PET/CT scans, and these features were analyzed separately to predict benign and malignant pulmonary lesions. The results showed that 68Ga-NOTA-PRGD2 PET and 18F-FDG PET features have similar diagnostic capacity for pulmonary space-occupying lesions. 68Ga-NOTA-PRGD2 PET image features have better diagnostic capacity than CT, and 18 of the 68Ga-NOTA-PRGD2 PET features demonstrated higher AUC values and better diagnostic capacity than all CT features in the differential diagnosis of pulmonary lesions. The radiomics features of the three imaging methods had certain potential predictive value for benign and malignant lung lesions, but the AUC values did not exceed 0.80, which indicated that these features had certain limitations as predictors of benign and malignant lesions alone.
Among the 18F-FDG PET features, a total of 11 features had significant differences (P<0.05) between the benign and malignant groups. Among the 68Ga-NOTA-PRGD2 PET features, a total of 14 features were significantly different (P<0.05) between the two groups. There were no significant differences (P<0.05) in CT features between the two groups. This finding shows that the features of benign and malignant pulmonary space-occupying lesions on 18F-FDG PET or 68Ga-NOTA-PRGD2 PET images had certain differences. Since image features are affected by multiple factors (28, 29), such as scanning parameters, reconstruction methods and quantification methods, this difference needs to be further verified. The image feature correlation coefficient map showed that CT, 18F-FDG PET and 68Ga-NOTA-PRGD2 PET images had certain correlations, but these correlations were lower than the correlations of features within certain imaging methods, suggesting that the information extracted from different sequences could be complementary.
This study further used the sequence forward selection algorithm to select features, applied the random forest algorithm to establish 7 classification models to distinguish between benign and malignant lung lesions, and used 1000 times 10-fold cross-validation to evaluate the diagnostic efficacy of the models. The established models were the CT feature model, 18F-FDG PET feature model, 68Ga-NOTA-PRGD2 PET feature model, CT & 18F-FDG PET feature model, CT & 68Ga-NOTA-PRGD2 PET feature model, 18F-FDG PET & 68Ga -NOTA-PRGD2 PET feature model, and the model of combination of all features. The results showed that the diagnostic efficacy of these seven models was good, and the corresponding average AUC values were 0.870, 0.895, 0.853, 0.903, 0.876, 0.892, and 0.908. Considering the intuitive difference in AUC values, if these models must be sorted, we believe that the performance from high to low is as follows: the model of combination of all features, CT & 18F-FDG PET feature model, 18F-FDG PET feature model, 18F-FDG PET & 68Ga-NOTA-PRGD2 PET feature model, CT & 68Ga-NOTA-PRGD2 PET feature model, CT feature model, and 68Ga-NOTA-PRGD2 PET feature model. Previous studies (27) generally believed that CT & 18F-FDG PET feature models were better than 18F-FDG PET feature models, and the 18F-FDG PET feature model was better than the CT feature model. Our study also supported this result. In the future, different modeling methods could be used to try to develop a model with optimal prediction performance.
We also found that the AUC value of the three-modal image feature model was better than those of the dual-modal image feature models, and the AUC values of the dual-modal image feature models were usually better than those of the single-modal image feature models, which further extended the results of Zhang (27), Teramoto (30), and Blemker (31). This may result from the combination of multi-modality and multi-probe images extracting more lesion information than single-modality or single-probe image features and improving the diagnostic efficiency of the models. The multi-modality and multi-probe imaging method was certainly better than the single-modality or single-probe imaging method in the diagnosis of benign and malignant lung lesions, but in clinical practice, considering the high penetration rate, low cost and high sensitivity of CT, screening malignant lung lesions based on CT is still a good choice under limited conditions.
We discussed the radiomics model based on a new imaging method, 68Ga-NOTA-PRGD2 PET. The established model had an AUC value of 0.853, which was significantly lower than that of the 18F-FDG PET feature model (AUC of 0.895, P<0.001), and its sensitivity and specificity were both lower than the latter, which may indicate that the 68Ga-NOTA-PRGD2 PET feature model performed worse than the 18F-FDG PET feature model. The possible reason, on the one hand, was related to the modeling methods and deviation of VOI. On the other hand, the radiation dose of 68Ga-NOTA-PRGD2 PET was lower than that of 18F-FDG PET, which resulted in relatively poor imaging quality and less information. After the addition of CT, the diagnostic efficiency of both methods increased. The sensitivity of the CT & 68Ga-NOTA-PRGD2 PET feature model was even higher than that of the CT & 18F-FDG PET feature model, though with a lower AUC value (P<0.001). Besides, the addition of 68Ga-NOTA-PRGD2 PET features to the CT & 18F-FDG PET feature model can significantly improve the diagnostic efficiency of the model (AUC: 0.908 vs. 0.903, P = 0.003 < 0.05), which indicated the potential value of the multi-modality and multi-probe models and warranted further validation in the future. Our study did not report radiomics analysis on metastases detection of lung cancer based on 68Ga-NOTA-PRGD2 PET and it was our future direction.
Our study has some limitations as follows. (1) Our study was a retrospective analysis that enrolled 48 patients with difierent benign or malignant pulmonary space-occupying lesions. The amount of data was small, and all came from a single center, so we made efforts to eliminate the uncertainty and instability of the features as much as possible, such as using cross-validation instead of separating another validation set for the limited data and setting segmentation standards for extracting features from images. (2) In the differentiation of benign and malignant lesions, the number of benign and malignant cases was slightly unbalanced, which needs to be further considered in future studies.
In conclusion, this is a rather novel study to suggest that some 68Ga-NOTA-PRGD2 PET image features solely could have even better diagnostic capacity than all CT features for pulmonary space-occupying lesions. Apart from that, this is also a pilot study to show that classification models developed based on multi-modality and multi-probe images can extract more information about lesions and improve the diagnostic efficiency of radiomics models than single-modality and single-probe image models. For future study, our preliminary clinical hypothesis of using radiomics based on 68Ga-NOTA-PRGD2 PET images and multi-modality and multi-probe images as a diagnostic tool warrants further validation in a larger multicenter sample size.
Data Availability Statement
The raw data supporting the conclusions of this article will be made available by the authors, without undue reservation.
Ethics Statement
The studies involving human participants were reviewed and approved by Institute Review Board of Peking Union Medical College Hospital, Chinese Academy of Medical Sciences and Peking Union Medical College. Written informed consent for participation was not required for this study in accordance with the national legislation and the institutional requirements.
Author Contributions
ZZ, FX, and KZ conceived of the project, analyzed the data, and wrote the paper. LL, LF, and XJ provided expert guidance, data, or analysis tools, and reviewed the manuscript. All authors contributed to the article and approved the submitted version.
Funding
This study has received funding by the Capital Health Development Scientific Research Project (2018-1-4011), the Chinese Academy of Medical Science Clinical and Translational Medicine Research Foundation (2019XK320032), and the National Natural Science Foundation of China (81871392).
Conflict of Interest
The authors declare that the research was conducted in the absence of any commercial or financial relationships that could be construed as a potential conflict of interest.
Publisher’s Note
All claims expressed in this article are solely those of the authors and do not necessarily represent those of their affiliated organizations, or those of the publisher, the editors and the reviewers. Any product that may be evaluated in this article, or claim that may be made by its manufacturer, is not guaranteed or endorsed by the publisher.
Supplementary Material
The Supplementary Material for this article can be found online at: https://www.frontiersin.org/articles/10.3389/fonc.2022.877501/full#supplementary-material
References
1. Gould MK, Maclean CC, Kuschner WG, Rydzak CE, Owens DK. Accuracy of Positron Emission Tomography for Diagnosis of Pulmonary Nodules and Mass Lesions: A Meta-Analysis. JAMA (2001) 285(7):914–24. doi: 10.1001/jama.285.7.914
2. Sim YT, Goh YG, Dempsey MF, Han S, Poon FW. PET-CT Evaluation of Solitary Pulmonary Nodules: Correlation With Maximum Standardized Uptake Value and Pathology. Lung (2013) 191(6):625–32. doi: 10.1007/s00408-013-9500-6
3. Palestro CJ. Molecular Imaging of Infection: The First 50 Years. Semin Nucl Med (2020) 50(1):23–34. doi: 10.1053/j.semnuclmed.2019.10.002
4. Jones MA, MacCuaig WM, Frickenstein AN, Camalan S, Gurcan MN, Holter-Chakrabarty J, et al. Molecular Imaging of Inflammatory Disease. Biomedicines (2021) 9(2):152. doi: 10.3390/biomedicines9020152
5. Groheux D, Quere G, Blanc E, Lemarignier C, Vercellino L, de Margerie-Mellon C, et al. FDG PET-CT for Solitary Pulmonary Nodule and Lung Cancer: Literature Review. Diagn Interv Imaging (2016) 97(10):1003–17. doi: 10.1016/j.diii.2016.06.020
6. Rahman WT, Wale DJ, Viglianti BL, Townsend DM, Manganaro MS, Gross MD, et al. The Impact of Infection and Inflammation in Oncologic (18)F-FDG PET/CT Imaging. BioMed Pharmacother (2019) 117:109168. doi: 10.1016/j.biopha.2019.109168
7. Liu Y. Lung Neoplasms With Low F18-Fluorodeoxyglucose Avidity. PET Clin (2018) 13(1):11–8. doi: 10.1016/j.cpet.2017.08.002
8. Debordeaux F, Chansel-Debordeaux L, Pinaquy JB, Fernandez P, Schulz J. What About Alphavbeta3 Integrins in Molecular Imaging in Oncology? Nucl Med Biol (2018) 62-63:31–46. doi: 10.1016/j.nucmedbio.2018.04.006
9. Hynes RO. Integrins: A Family of Cell Surface Receptors. Cell (1987) 48(4):549–54. doi: 10.1016/0092-8674(87)90233-9
10. Kwakwa KA, Sterling JA. Integrin Alphavbeta3 Signaling in Tumor-Induced Bone Disease. Cancers (Basel) (2017) 9(7):84. doi: 10.3390/cancers9070084
11. Li L, Zhao W, Sun X, Liu N, Zhou Y, Luan X, et al. (18)F-RGD PET/CT Imaging Reveals Characteristics of Angiogenesis in non-Small Cell Lung Cancer. Transl Lung Cancer Res (2020) 9(4):1324–32. doi: 10.21037/tlcr-20-187
12. Zheng K, Liang N, Zhang J, Lang L, Zhang W, Li S, et al. 68Ga-NOTA-PRGD2 PET/CT for Integrin Imaging in Patients With Lung Cancer. J Nucl Med (2015) 56(12):1823–7. doi: 10.2967/jnumed.115.160648
13. Lambin P, Rios-Velazquez E, Leijenaar R, Carvalho S, van Stiphout RG, Granton P, et al. Radiomics: Extracting More Information From Medical Images Using Advanced Feature Analysis. Eur J Cancer (2012) 48(4):441–6. doi: 10.1016/j.ejca.2011.11.036
14. Thawani R, McLane M, Beig N, Ghose S, Prasanna P, Velcheti V, et al. Radiomics and Radiogenomics in Lung Cancer: A Review for the Clinician. Lung Cancer (2018) 115:34–41. doi: 10.1016/j.lungcan.2017.10.015
15. Avanzo M, Stancanello J, Pirrone G, Sartor G. Radiomics and Deep Learning in Lung Cancer. Strahlenther Onkol (2020) 196(10):879–87. doi: 10.1007/s00066-020-01625-9
16. Nioche C, Orlhac F, Boughdad S, Reuze S, Goya-Outi J, Robert C, et al. LIFEx: A Freeware for Radiomic Feature Calculation in Multimodality Imaging to Accelerate Advances in the Characterization of Tumor Heterogeneity. Cancer Res (2018) 78(16):4786–9. doi: 10.1158/0008-5472.CAN-18-0125
17. Siegel RL, Miller KD, Jemal A. Cancer Statistics, 2020. CA Cancer J Clin (2020) 70(1):7–30. doi: 10.3322/caac.21590
18. Ezer N, Navasakulpong A, Schwartzman K, Ofiara L, Gonzalez AV. Impact of Rapid Investigation Clinic on Timeliness of Lung Cancer Diagnosis and Treatment. BMC Pulm Med (2017) 17(1):178. doi: 10.1186/s12890-017-0504-5
19. Duma N, Santana-Davila R, Molina JR. Non-Small Cell Lung Cancer: Epidemiology, Screening, Diagnosis, and Treatment. Mayo Clin Proc (2019) 94(8):1623–40. doi: 10.1016/j.mayocp.2019.01.013
20. Manafi-Farid R, Karamzade-Ziarati N, Vali R, Mottaghy FM, Beheshti M. 2-[(18)F]FDG PET/CT Radiomics in Lung Cancer: An Overview of the Technical Aspect and its Emerging Role in Management of the Disease. Methods (2021) 188:84–97. doi: 10.1016/j.ymeth.2020.05.023
21. Zhou Y, Ma XL, Zhang T, Wang J, Zhang T, Tian R. Use of Radiomics Based on (18)F-FDG PET/CT and Machine Learning Methods to Aid Clinical Decision-Making in the Classification of Solitary Pulmonary Lesions: An Innovative Approach. Eur J Nucl Med Mol Imaging (2021) 48(9):2904-13. doi: 10.1007/s00259-021-05220-7
22. Kang F, Mu W, Gong J, Wang S, Li G, Li G, et al. Integrating Manual Diagnosis Into Radiomics for Reducing the False Positive Rate of (18)F-FDG PET/CT Diagnosis in Patients With Suspected Lung Cancer. Eur J Nucl Med Mol Imaging (2019) 46(13):2770–9. doi: 10.1007/s00259-019-04418-0
23. Shao X, Niu R, Shao X, Jiang Z, Wang Y. Value of (18)F-FDG PET/CT-Based Radiomics Model to Distinguish the Growth Patterns of Early Invasive Lung Adenocarcinoma Manifesting as Ground-Glass Opacity Nodules. EJNMMI Res (2020) 10(1):80. doi: 10.1186/s13550-020-00668-4
24. Kirienko M, Cozzi L, Rossi A, Voulaz E, Antunovic L, Fogliata A, et al. Ability of FDG PET and CT Radiomics Features to Differentiate Between Primary and Metastatic Lung Lesions. Eur J Nucl Med Mol Imaging (2018) 45(10):1649–60. doi: 10.1007/s00259-018-3987-2
25. Yan M, Wang W. Development of a Radiomics Prediction Model for Histological Type Diagnosis in Solitary Pulmonary Nodules: The Combination of CT and FDG PET. Front Oncol (2020) 10:555514. doi: 10.3389/fonc.2020.555514
26. Kubota K, Murakami K, Inoue T, Saga T, Shiomi S. Additional Effects of FDG-PET to Thin-Section CT for the Differential Diagnosis of Lung Nodules: A Japanese Multicenter Clinical Study. Ann Nucl Med (2011) 25(10):787–95. doi: 10.1007/s12149-011-0528-5
27. Zhang R, Zhu L, Cai Z, Jiang W, Li J, Yang C, et al. Potential Feature Exploration and Model Development Based on 18F-FDG PET/CT Images for Differentiating Benign and Malignant Lung Lesions. Eur J Radiol (2019) 121:108735. doi: 10.1016/j.ejrad.2019.108735
28. Galavis PE, Hollensen C, Jallow N, Paliwal B, Jeraj R. Variability of Textural Features in FDG PET Images Due to Different Acquisition Modes and Reconstruction Parameters. Acta Oncol (2010) 49(7):1012–6. doi: 10.3109/0284186X.2010.498437
29. Leijenaar RT, Nalbantov G, Carvalho S, van Elmpt WJ, Troost EG, Boellaard R, et al. The Effect of SUV Discretization in Quantitative FDG-PET Radiomics: The Need for Standardized Methodology in Tumor Texture Analysis. Sci Rep (2015) 5:11075. doi: 10.1038/srep11075
30. Teramoto A, Tsujimoto M, Inoue T, Tsukamoto T, Imaizumi K, Toyama H, et al. Automated Classification of Pulmonary Nodules Through a Retrospective Analysis of Conventional CT and Two-Phase PET Images in Patients Undergoing Biopsy. Asia Ocean J Nucl Med Biol (2019) 7(1):29–37. doi: 10.22038/AOJNMB.2018.12014
Keywords: 18F-FDG, 68Ga-NOTA-PRGD2, positron emission tomography computed tomography, pulmonary space-occupying lesions, radiomics
Citation: Xie F, Zheng K, Liu L, Jin X, Fu L and Zhu Z (2022) A Pilot Study of Radiomics Models Combining Multi-Probe and Multi-Modality Images of 68Ga-NOTA-PRGD2 and 18F-FDG PET/CT for Differentiating Benign and Malignant Pulmonary Space-Occupying Lesions. Front. Oncol. 12:877501. doi: 10.3389/fonc.2022.877501
Received: 16 February 2022; Accepted: 04 May 2022;
Published: 02 June 2022.
Edited by:
Ryogo Minamimoto, National Center For Global Health and Medicine, JapanReviewed by:
Lian-Ming Wu, Shanghai Jiao Tong University, ChinaFang Xie, Fudan University, China
Shuanghu Yuan, Shandong First Medical University and Shandong Academy of Medical Sciences, China
Copyright © 2022 Xie, Zheng, Liu, Jin, Fu and Zhu. This is an open-access article distributed under the terms of the Creative Commons Attribution License (CC BY). The use, distribution or reproduction in other forums is permitted, provided the original author(s) and the copyright owner(s) are credited and that the original publication in this journal is cited, in accordance with accepted academic practice. No use, distribution or reproduction is permitted which does not comply with these terms.
*Correspondence: Zhaohui Zhu, 13611093752@163.com
†These authors have contributed equally to this work and share first authorship