- Department of Medical Oncology, Qilu Hospital, Cheeloo College of Medicine, Shandong University, Jinan, China
The application of immune checkpoint inhibitors (ICIs) in non-small cell lung cancer has significantly improved patient survival. However, most patients fail to respond to ICIs or develop drug resistance during treatment. Therefore, novel biomarkers are needed to predict the efficacy of ICIs or provide clues on how to overcome drug resistance. Here, it was revealed that cell division cycle 25C (CDC25C) expression was upregulated in lung adenocarcinoma (LUAD) compared to that of normal lung tissue in multiple databases. This was further verified by q-PCR. Furthermore, higher CDC25C expression was associated with shorter overall survival and worse pathological stage. Most importantly, a higher CDC25C expression was associated with shorter progression-free survival in LUAD patients treated with nivolumab, suggesting the role of the cell cycle in immunotherapy. In addition, CDC25C expression was significantly associated with immune cell infiltration and immune-related signatures in the LUAD tumor microenvironment. Moreover, CDC25C was differentially expressed and correlated with overall survival in multiple tumors, indicating that CDC25C is a broad-spectrum biomarker. Taken together, our study demonstrates that CDC25C is a prognostic biomarker for LUAD patients, especially for patients treated with ICIs. Our study also provides strong evidence for the role of the cell cycle in ICIs therapy and tumor microenvironment.
Introduction
Lung cancer is one of the most common cancers in the world. According to the GLOBOCAN 2020 estimation, there were approximately 19.3 million newly diagnosed cancer patients in 2020, of which lung cancer accounted for 11.4%. Notably, the latest data show that lung cancer ranks first in cancer-related deaths (1, 2). Based on the pathological classification, lung cancer can be divided into small cell lung cancer and non-small cell lung cancer (NSCLC), in which lung adenocarcinoma (LUAD) is the most common subtype (3). Over the past few decades, advances have been made in the treatment of NSCLC, including targeted therapy and immune checkpoint inhibitors (ICIs) therapy, especially for patients with LUAD (4). The introduction of ICIs, including antibodies targeting programmed cell death protein 1 (PD-1), programmed death-ligand 1 (PD-L1), and cytotoxic T lymphocyte antigen 4 (CTLA-4), have significantly prolonged survival of patients with advanced NSCLC, with 5-year survival rates from < 5% to 23.2%, reaching 31.9% in patients with PD-L1 >50% (5–7). However, only a small portion of patients (approximately 14% - 40%) with NSCLC responded to ICIs, even in patients with high PD-L1 expression (8–11). Therefore, developing novel biomarkers that predict the response to ICIs and screen patients who benefit from ICIs-based therapies is a crucial mandate. Currently, it has been demonstrated that patients with high PD-L1 expression and high tumor mutation burden (TMB) are more likely to benefit from ICIs (12). Nevertheless, clinicians cannot accurately identify patients that would benefit from ICIs treatment since some patients with low PD-L1 or TMB were reported to respond to ICIs (13, 14). Indeed, various factors involved in the tumor microenvironment (TME) or cancer-immunity cycle participate in the regulation of ICIs therapy. Notably, recent studies have found that many genes play critical roles in the transformation of TME types. Guo et al. revealed that Zeb1 induced an immunosuppressive TME (15), which mediated tumor cell escaping from immune surveillance. Therefore, it is necessary to explore more biomarkers that influence or predict the efficacy of ICIs from this perspective.
Aberrant cell cycle is one of the crucial mechanisms leading to uncontrolled proliferation of cancer cells, which is an important hallmark of cancer (16). Currently, strong evidence indicates cell cycle progression is closely related to cancer invasion, metastasis, anti-apoptosis, and chemotherapy-resistance (17). Therefore, targeting cell-cycle proteins is a promising anti-tumor modality and has been extensively studied (16). Notably, cyclin-dependent kinase 4/6 (CDK4/6, cell cycle-related genes) inhibitors have shown significant activity against several cancers and some (namely palbociclib, ribociclib and abemaciclib) are approved for treatment of patients with advanced breast cancer (18). Actually, the regulation of cell cycle involves numerous genes. Besides CDK4/6, the cell division cyclin 25 (CDC25) family, consisting of CDC25A, CDC25B, and CDC25C, are also involved cell-cycle progression (19). Among them, CDC25C, a specific cyclin and dual-specificity phosphatase, plays a predominant role in regulating the initiation of cell division and controlling the cell cycle (19). Currently, CDC25C has been found to play a pro-tumor role in various tumors. Higher expression of CDC25C has been observed in multiple cancers, including bladder (20), gastric (21) and colorectal cancers (22), associated with poor prognosis. Intriguingly, reciprocal activation between CDC25C and cyclin B1/CDK1 is observed. In other words, CDC25C activates cyclin B1/CDK1 complex, which in turn phosphorylates CDC25C, thereby accelerating cell division by positive feedback (19). Notably, accumulating evidence suggested that inhibition of CDKs not only contributes to cell cycle arrest but also triggers anti-tumor immunity (23–26) and enhances the efficiency of anti-PD-1 therapy (27), thus CDC25C might also have potential immunoregulatory effect via interaction with CDKs. However, the role of CDC25C in lung cancer especially its influence on the tumor immune microenvironment and ICIs efficacies has not been clarified.
In the study, the prognostic role of CDC25C in patients with LUAD was investigated, especially in patients treated with ICIs, using the TCGA and GEO databases. In addition, the infiltration levels of various immune cells or immune-related signatures in TME and gene enrichment analysis were evaluated to explore the potential mechanism of CDC25C in ICIs treatment. Finally, the role of CDC25C in pan-cancer was analyzed using TCGA database.
Materials and Methods
RNA Seq and Clinical Data From TCGA and GEO
RNA-seq and clinical data were downloaded from The Cancer Genome Atlas (TCGA) for 33 cancers, including LUAD and lung squamous carcinoma (LUSC). LUAD data consisted of 59 adjacent nontumor tissues and 535 LUAD tissues. LUSC data consisted of 49 adjacent nontumor tissues and 502 LUSC tissues. In addition, GSE13213, GSE157001, GSE157009, and GSE126044 datasets were downloaded from the GEO database. Patients were classified as responders when the response (PR) or stable disease (SD) is > 6 months according to Response Evaluation Criteria in Solid Tumors (RECIST) ver. 1.1. When progressive disease (PD) or SD is ≤ 6 months, patients were classified as non-responders by RECIST ver. 1.1.
CDC25C Expression Analysis in LUAD and LUSC
The CDC25C expression in LUAD and LUSC was analyzed by the R software ggplot2 package using the downloaded TCGA data. The Oncomine database (28) was then used to validate the expression of CDC25C in LUAD. Next, the Human Protein Atlas (HPA) database was used to explore the expression of CDC25C in LUAD versus normal lung tissue at the protein level. In addition, q-PCR was performed using lung normal epithelial cell line (BEAS-2B) and LUAD cell lines (PC-9 and H1299) for further validation at the mRNA level. Based on clinical data from TCGA LUAD, CDC25C expression was analyzed in patients stratified by age, gender, smoking history, T-stage, N-stage, M-stage, and pathological stage. Among the pathological stage analysis, we defined stage I and II as local, stage III as locally advanced, and stage IV as metastatic. Furthermore, through the UALCAN database (29), the relationship between CDC25C expression and TP53 mutation status was explored in LUAD patients.
mRNA-Based Survival Analysis of LUAD and LUSC Patients
Survival analysis was performed using LUAD and LUSC data from TCGA, including RNA-seq and survival data. The cut-off value for high and low CDC25C in this study was determined by the median CDC25C within each cancer type. According to the median risk score, the patients were divided into low- and high-risk groups. The formula is as follows: risk score = Exp × Coef (Exp = gene expression value, Coef = coefficient). The “survminer” and “survivor” packages were used for the analyses. The ROC curves were constructed in LUAD using “pROC” and “tmieROC” packages. Univariate and multivariate Cox regression analyses were performed to further investigate the correlation between multivariable characteristics and overall survival (OS) using LUAD data (30). The GEO datasets were used to further validate the relationship between CDC25C expression and lung cancer prognosis.
Pathway Enrichment Analysis
The gene expression profiles of LUAD from TCGA were downloaded and partitioned into two groups according to the median value of CDC25C expression. The R package “edgeR” was used to obtain the differentially expressed genes between the two groups (31). In addition, Gene Set Enrichment Analysis (GSEA) was performed using the “ClusterProfiler” package (32). A gene set with a p-value lower than 0.05 was considered significantly enriched in Kyoto Encyclopedia of Genes and Genomes (KEGG) and Gene Ontology (GO) terms.
Immune Cell Infiltration Analysis in LUAD
As described in previous literature, the single-sample gene set enrichment analysis (ssGSEA) was performed to quantify the relative abundance of tumor-infiltrating immune cells in LUAD patients with a high or low CDC25C expression using the “GSVA” R package (33, 34). Then, Spearman was used to analyze the correlation between immune cell infiltration and CDC25C expression. Next, the relationship between CDC25C and multiple types of immune cell infiltration was analyzed in LUAD tissues using TISIDB database (35). In addition, the TIMER database was used to explore the relationship between the copy number of CDC25C and immune cell infiltration. Moreover, four immune-related signatures (immuno-stimulators, immuno-inhibitors, MHC molecules, and chemokines) were compared between high CDC25C and low CDC25C expression LUAD using data from the TCGA database.
Identification of 10 Hub Genes Co-Expressed With CDC25C
Through the STRING database (36), we found 10 hub genes that were co-expressed with CDC25C in LUAD patients. Then, GEPIA (http://gepia.cancer-pku.cn/index.html) was used to explore the correlation between CDC25C expression and these hub genes. In addition, the expression of these hub genes in normal and LUAD tissues was explored using TCGA database. Furthermore, the prognostic values of the 10 hub genes were explored using TCGA LUAD data.
Analysis of CDC25C in Pan-Cancer
The expression of CDC25C in pan-cancer was analyzed using the UALCAN database (29) and the Oncomine database (28). Then, through the TCGA database, the relationship between CDC25C expression and OS was analyzed in pan-cancer.
RNA Extraction and q-PCR
Total RNA of BEAS-2B, PC9, and H1299 cells was isolated using the TRIzol reagent (Thermo Fisher Scientific). Then, cDNA was synthesized from 1 ug of total RNA using reverse transcription reagents (Accurate Biology, China) according to the manufacturer’s protocol. Next, LightCycler 480 device (Roche Diagnostics) and SYBR® Green Premix Pro Taq HS qPCR kit (Accurate Biology, China) were used to perform PCR. β-actin was used to normalize gene expression. Primer sequences were as follows:
CDC25C forward primer: GCAGAAGTGGCCTATATCGCT
CDC25C reverse primer: TTCCACCTGCTTCAGTCTTGG
β-actin forward primer: GAAGAGCTACGAGCTGCCTGA
β-actin reverse primer: CAGACAGCACTGTGTTGGCG
Statistical Analysis
The CDC25C expression between tumor and normal tissues was analyzed by Wilcoxon rank-sum test, and the q-PCR results were analyzed by t-test. In addition, the correlation between CDC25C and clinical characteristic variables was analyzed by the Pearson chi-square test. The Kaplan-Meier method and log-rank tests were used to perform the survival analysis. Moreover, univariate and multivariate analyses were conducted using the Cox proportional hazards regression model. In this study, the R software (version 4.1.1) and the GraphPad Prism (version 9.0) were used for data analysis. Also, multiple online databases, including TIMER, UALCAN, HPA, GEPIA, and STRING, were used for analysis. A two-tailed P-value < 0.05 was considered statistically significant.
Results
The Expression Level of CDC25C in Lung Cancer
First, to investigate whether CDC25C was differentially expressed between lung cancer and normal tissue, LUAD and lung squamous carcinoma (LUSC) RNA-seq data were downloaded from TCGA. It was observed that CDC25C expression was significantly upregulated in both LUAD and LUSC compared with normal tissues (all P-values < 0.001) (Figures 1A, B and Figures S1A, B). Although CDC25C expression was significantly upregulated in LUSC, subsequent survival analysis revealed no significant correlation between CDC25C expression and patient survival. Therefore, this study mainly focused on the relationship between CDC25C and LUAD. Next, the Oncomine database was used for further validation. By the t-test, box plot and peak plot of CDC25C in LUAD of Selamat Lung were shown in Figure 1C (t-test = 11.062, Fold Change = 1.45, P = 1.97E-16). A meta-analysis using data from 11 published studies showed that CDC25C was increased in LUAD compared to normal lung tissues (Median Rank = 873.0, P = 2.90E-10) (Figure 1D). Moreover, the HPA database was used to further validate this result at the protein level. As illustrated in Figure 1E, the expression of CDC25C was higher in LUAD tissues than in normal lung tissues. We further explored the differential expression of CDC25C by q-PCR using a normal lung epithelial cell line (BEAS-2B) and LUAD cell lines (PC9 and H1299). As shown in Figure 1F, the CDC25C expression was higher in PC9 (P < 0.001) and H1299 (P < 0.001) when compared to that of BEAS-2B.
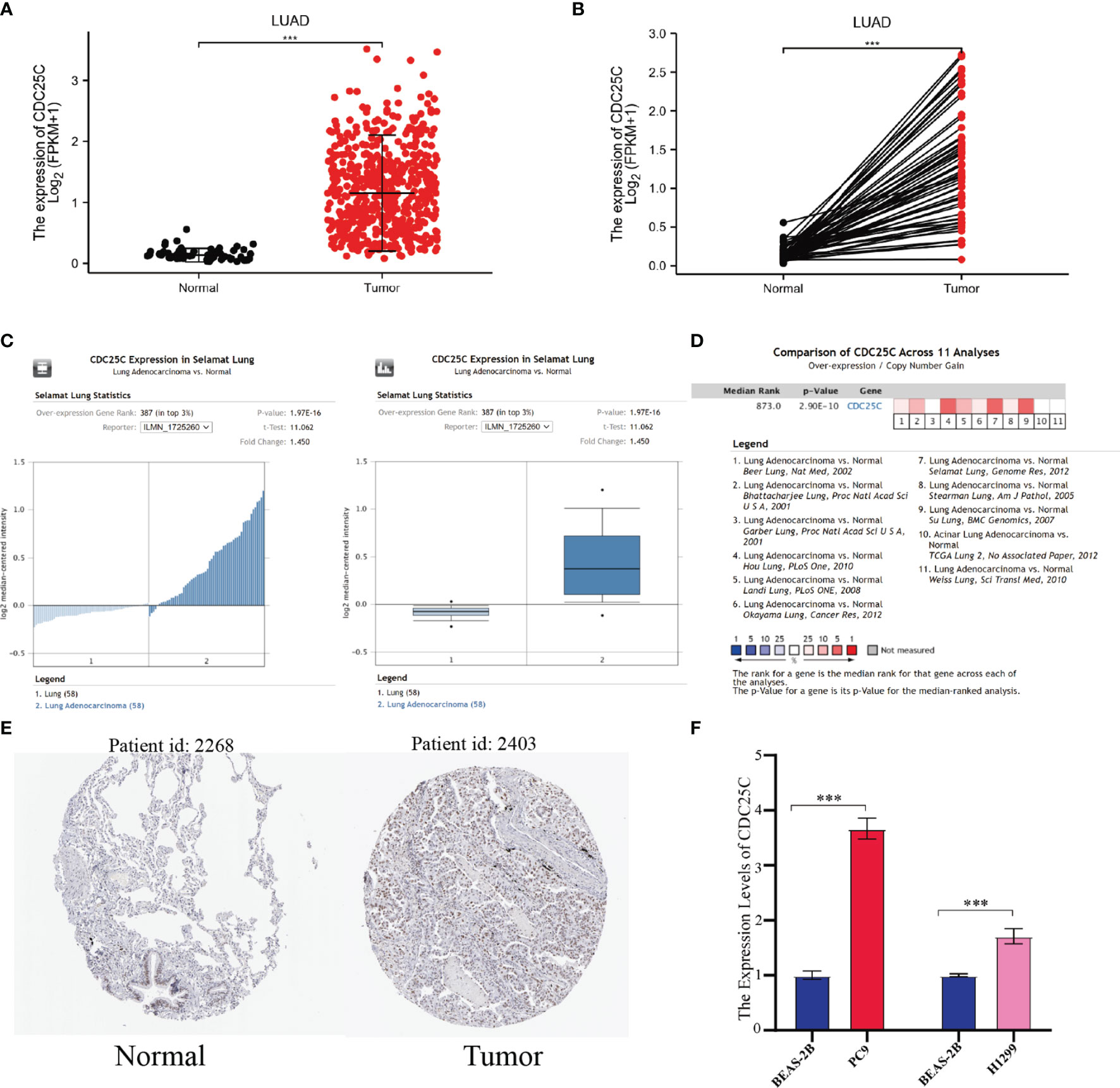
Figure 1 The expression of CDC25C in lung cancer. (A, B) CDC25C expression between tumors and normal tissues of non-paired (A) and paired (B) LUAD patients from the TCGA database. (C) By t-test, box plot and peak plot of CDC25C in LUAD. (D) A meta-analysis using data from 11 published studies showed that CDC25C was upregulated in LUAD. (E) Immunohistochemical results of CDC25C in normal lung tissues and LUAD tissues from the human protein atlas database. (F) q-PCR analysis for CDC25C in normal lung epithelial cell line (BEAS-2B) and lung adenocarcinoma cell lines (PC9, H1299) (***P < 0.001).
Prognostic Significance of CDC25C Expression in LUAD
Survival analysis was conducted using the TCGA database to determine the correlation between CDC25C expression and lung cancer prognosis. Results showed that higher CDC25C expression was statistically associated with a shorter overall survival (OS) (P < 0.001), shorter progress free interval (PFI) (P = 0.005) and shorter disease-specific survival (DSS) (P = 0.001) in LUAD (Figures 2A–C), but not in LUSC (all P values > 0.05) (Figures S1C–E). For further validation, survival analysis was performed using LUAD and LUSC data from the GEO database and results were strikingly similar (Figures S1F–H). In addition, an univariate analysis demonstrated that T-stage (HR: 2.317, 95% CI: 1.591-3.375, p < 0.001), N-stage (HR: 2.601, 95% CI: 1.944-3.480, p < 0.001), M-stage (HR: 2.136, 95%CI: 1.248-3.653, p = 0.006), pathological stage (HR: 2.664, 95%CI: 1.960-3.621, p < 0.001), and CDC25C expression (HR: 1.509, 95%CI: 1.235-1.844, p < 0.001) were independent prognostic biomarkers for LUAD patients (Figure 2D). Meanwhile, high CDC25C expression was found to be an independent prognosis biomarker for poor OS for LUAD patients in multivariate analysis (HR: 1.637, 95%CI: 1.267-2.116, p < 0.001) (Table 1). Based on the risk score, we divided the population into high and low-risk groups and found that the high-risk group had a worse prognosis and higher CDC25C expression, when compared to the low-risk group (Figure 2E). Of note, CDC25C could better predict the 1-year (AUC 0.631), 3-year (AUC 0.612) and 5-year (AUC 0.602) survival rate of LUAD patients (Figure 2F). The ROC curve presented that CDC25C had a very low false-positive rate and a very high true-positive rate (AUC:0.984, CI:0.974-0.993) (Figure 2G), which indicated that CDC25C possessed an incredible diagnostic power for LUAD.
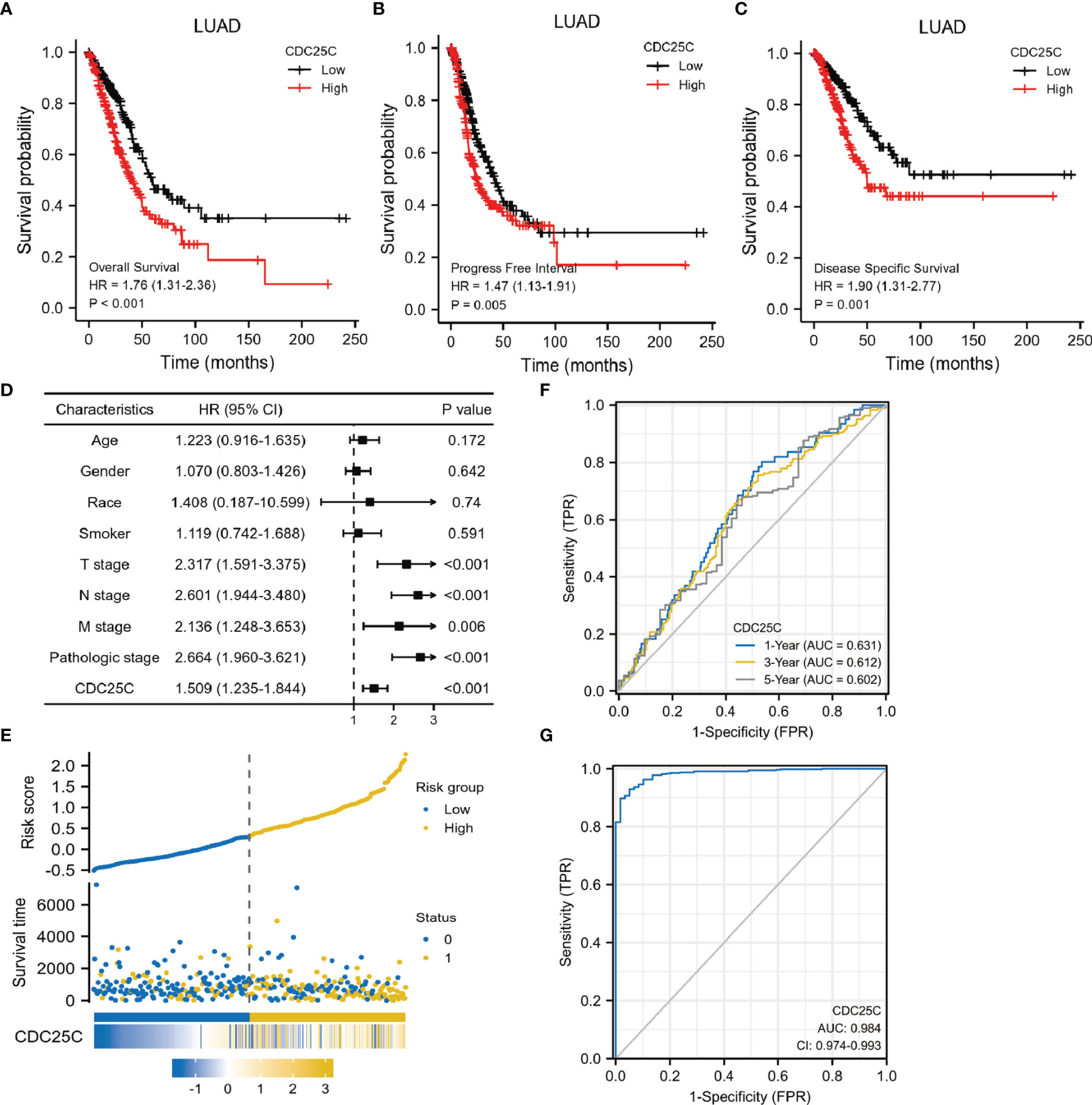
Figure 2 Prognostic role of CDC25C in lung cancer. (A–C) Correlation among OS, PFI, DSS and CDC25C expression of LUAD in TCGA database. (D) Forest plot of the univariate analysis results assessing independent prognostic factors for OS of LUAD. (E) The high and low risk maps. (F) The AUC curve of 1, 3 and 5 years to forecast the survival of LUAD patients. (G) The diagnostic value of CDC25C in LUAD patients.
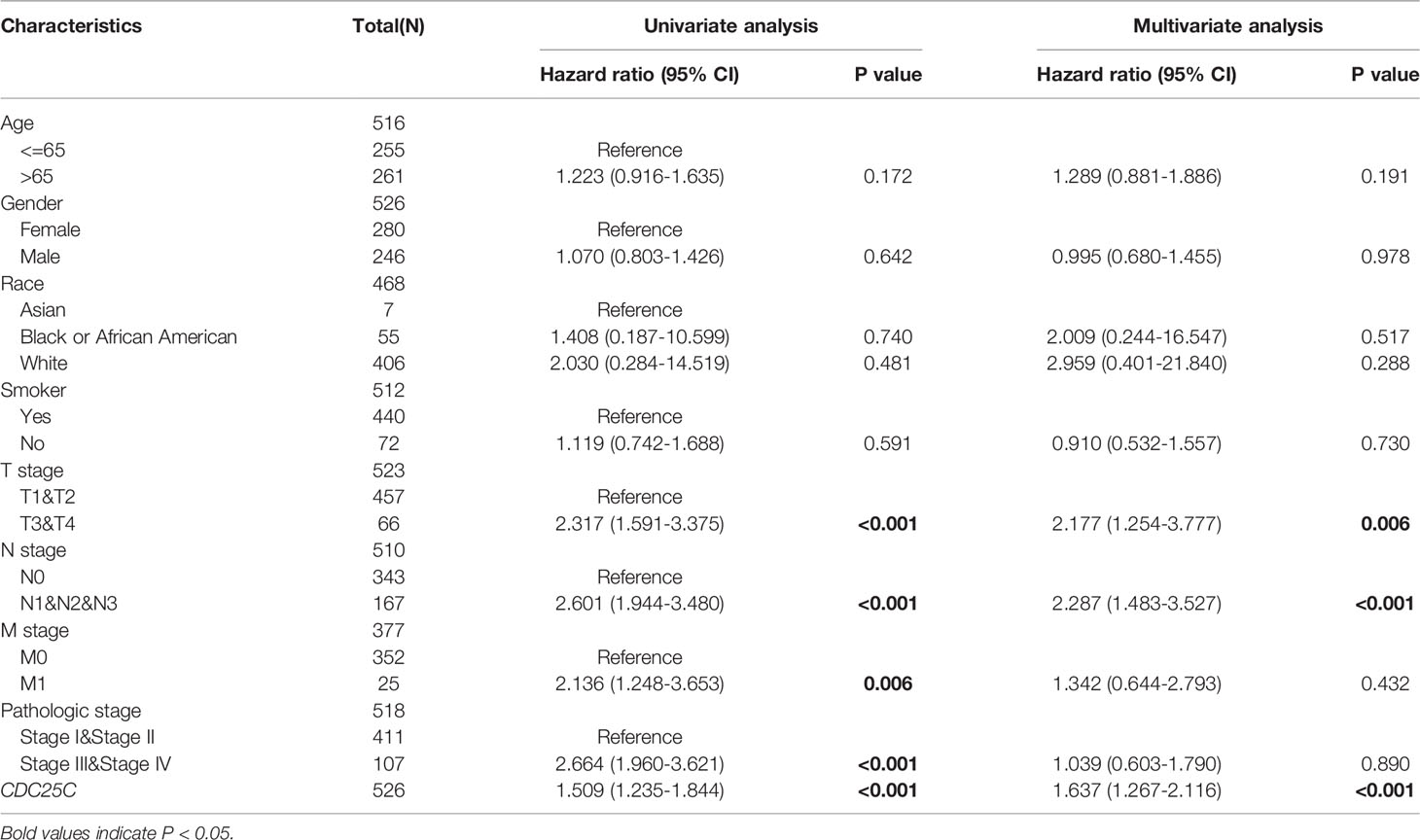
Table 1 Correlation between overall survival and multivariable characteristics in TCGA patients via Cox regression multivariate survival model.
Relationship Between CDC25C Expression and Clinical Features in LUAD Patients
By grouping TCGA data according to different clinical characteristics, we observed that CDC25C expression was closely associated with several clinical characteristics of LUAD patients. LUAD patients of low age (P < 0.01), male (P < 0.01), and with a history of smoking (P < 0.05), expressed higher CDC25C (Figures 3A–C). Besides, CDC25C was more highly expressed in T2-stage tumors than in T1-stage ones (P < 0.001) (Figure 3D). Regarding N-stage, higher CDC25C expression was observed in N1 and N2 stage tumors than in N0 stage tumors (all P values < 0.05) (Figure 3E). Meanwhile, M1-stage tumors expressed higher CDC25C than the M0-stage (P < 0.05) (Figure 3F). In addition, higher CDC25C expression significantly correlated with later pathological stages (local vs locally advanced: P < 0.05; local vs metastatic: P < 0.05) (Figure 3G). Moreover, LUAD bearing TP53 mutation had a higher CDC25C expression (P < 0.001) (Figure 3H). Thus, CDC25C expression was associated with the malignant progression of LUAD.
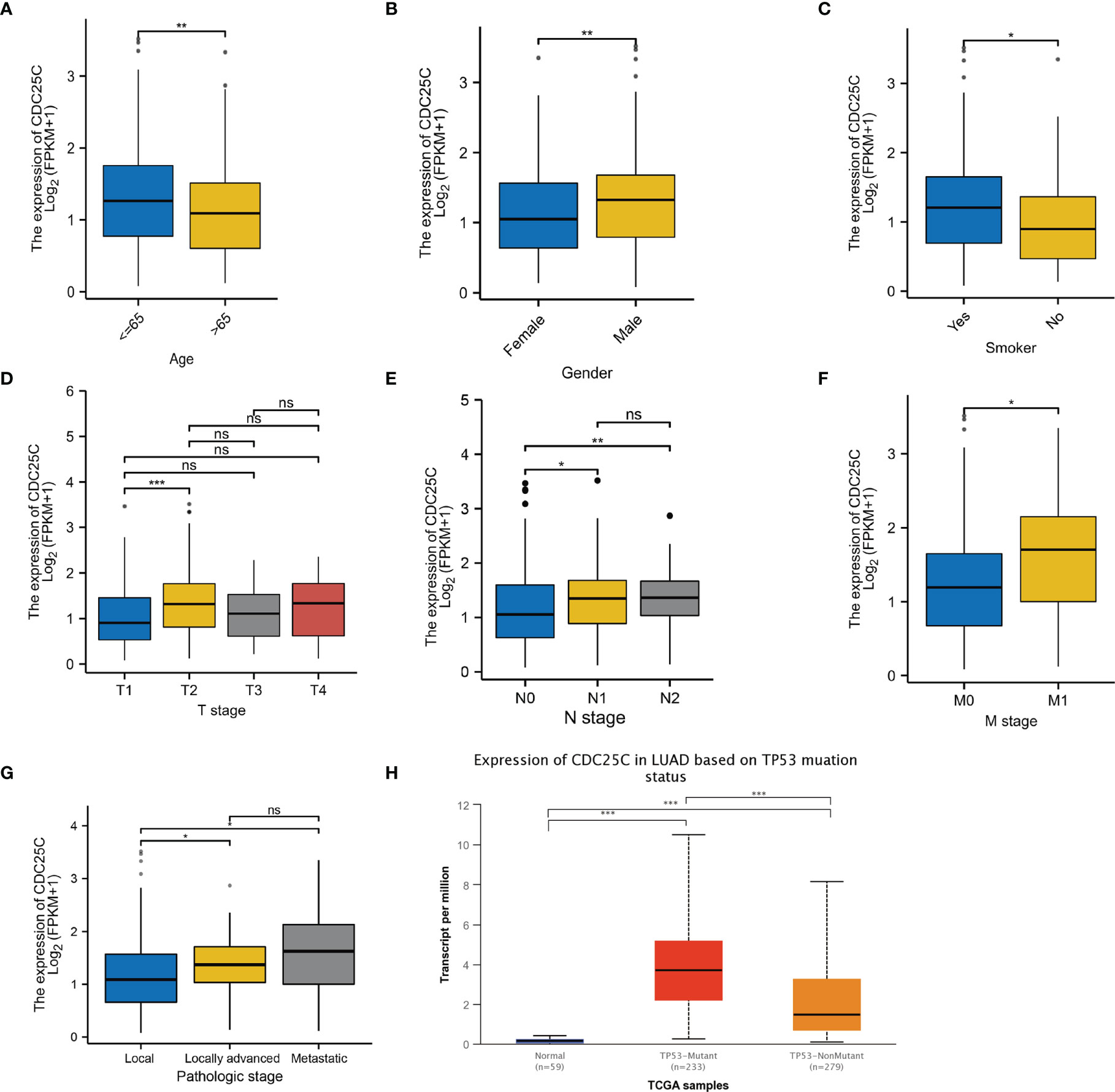
Figure 3 The relationship between CDC25C expression and clinical features of LUAD. (A–G) Analysis of LUAD data from the TCGA database showed that CDC25C expression correlated with age, gender, smoking history, T-stage, N-stage, M-stage, and pathological stage. (H) Through the UALCAN database, we found that CDC25C expression correlated with TP53 mutation status in LUAD (ns, not significant, *P < 0.05, **P < 0.01, ***P < 0.001).
Pathway Enrichment Analysis of CDC25C in LUAD
In order to elucidate the underlying mechanism of CDC25C action in LUAD, functional enrichment analysis was performed. First, we identified ten enriched signaling pathways positively associated with CDC25C expression (Figures 4A, B and Table 2), including: condensed chromosome, chromosome segregation, mitotic sister chromatid segregation, nuclear chromosome segregation, sister chromatid segregation, cell cycle checkpoints, M phase, DNA replication, mitotic metaphase, and anaphase. Then, ten enriched signaling pathways negatively associated with CDC25C expression were also identified (Figures 4C, D, and Table 2), including: axonemal dynein complex assembly, surfactant metabolism, diseases associated with surfactant metabolism and linoleic acid metabolism. From the enrichment analysis results, it was evident that CDC25C was associated with cell mitosis and metabolism.
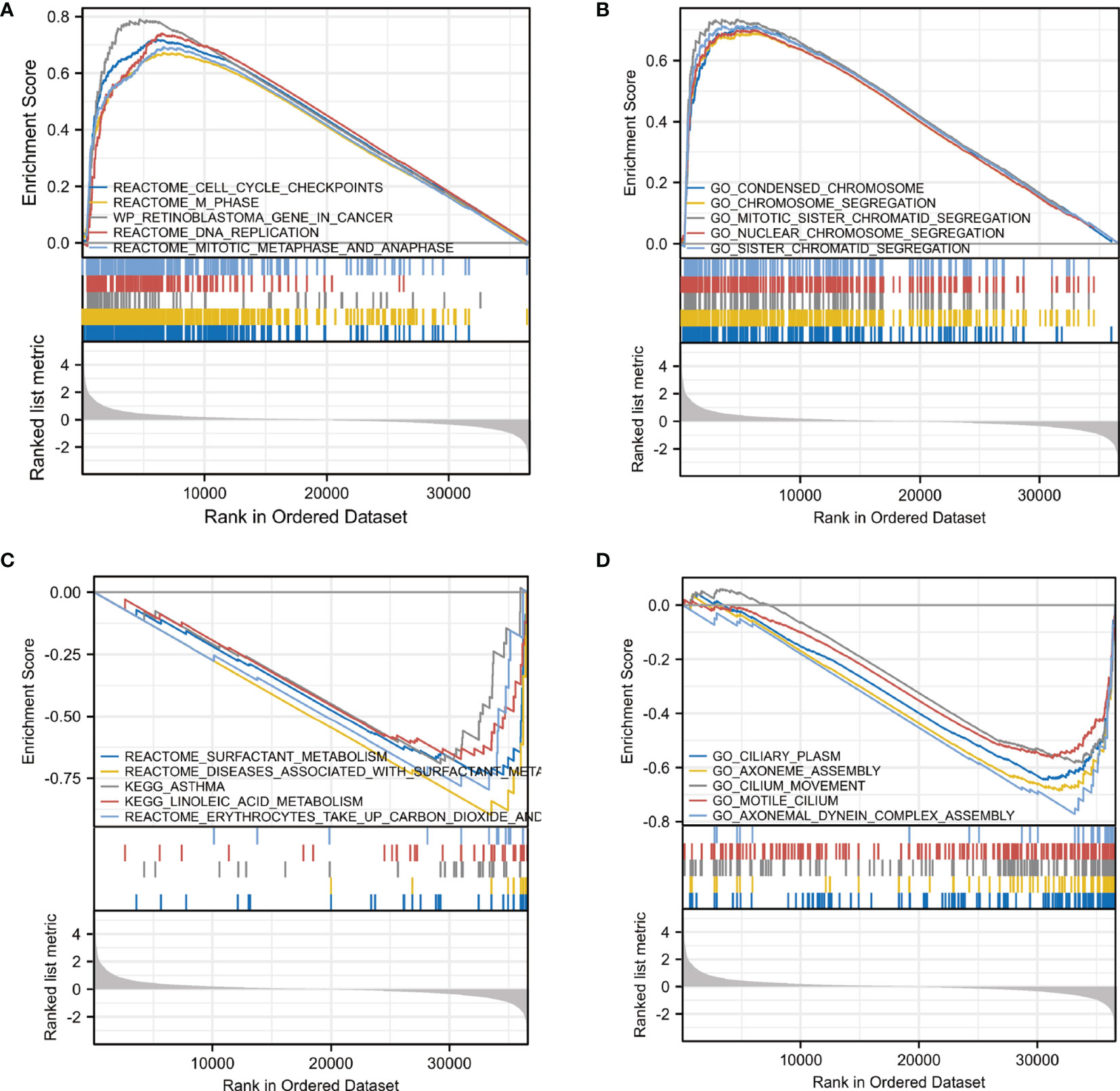
Figure 4 Pathway enrichment analysis of CDC25C in LUAD. (A, B) Gene enrichment plots displayed enrichment pathways in the high CDC25C expression LUAD. (C, D) Gene enrichment plots displayed enrichment pathways in the low CDC25C expression LUAD.
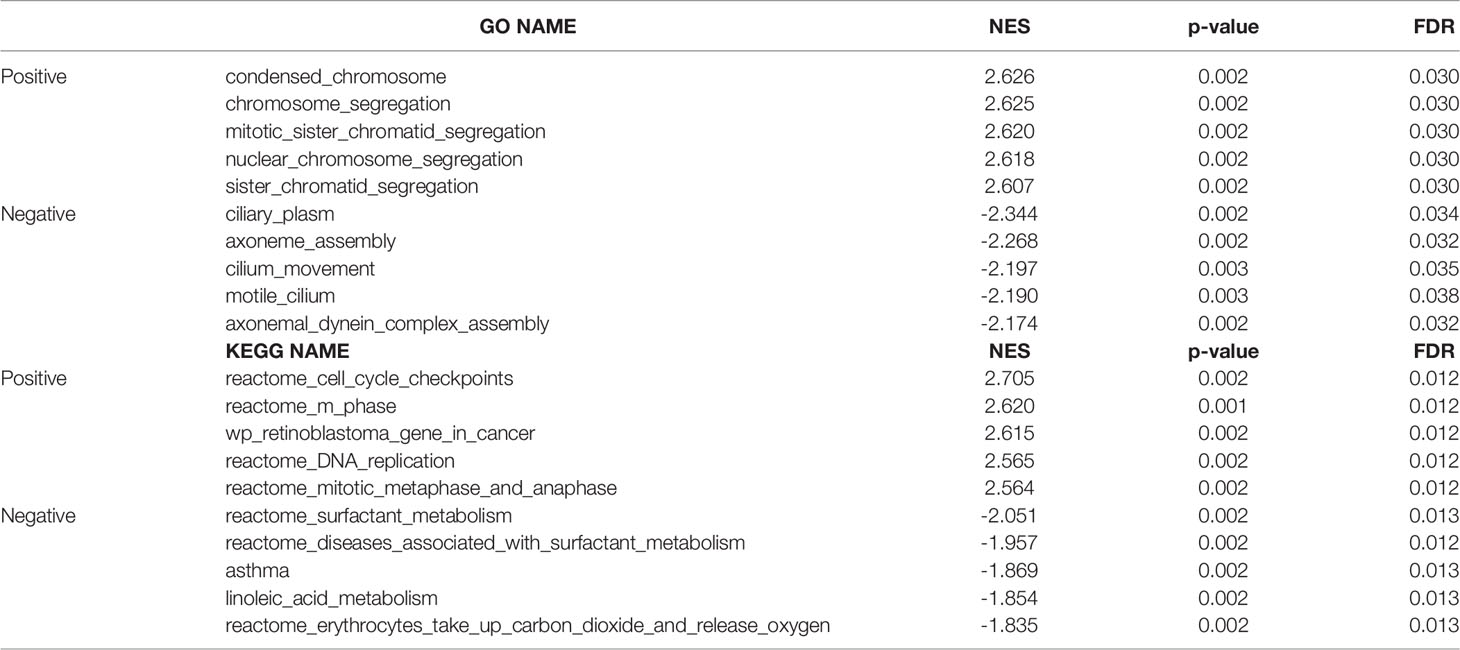
Table 2 Signaling pathways most significantly correlated with CDC25C expression based on their normalized enrichment score (NES) and p-value.
Correlation Between CDC25C Expression and Immune Cell Infiltration
The relationship between CDC25C expression and immune cells infiltration in the TME of LUAD tissues was further investigated. Figure 5A showed the infiltration of 15 immune cells in high- and low-CDC25C expression groups. Among these, the infiltration levels of 8 types of immune cells were lower in the high CDC25C expression group than in the low expression group, including CD8+T cells, pDC, iDC, DC, NK cells, mast cells, macrophages, and eosinophils (all P values < 0.05). In contrast, NK CD56dim cells and aDC had higher infiltration levels in the high CDC25C expression group (all P values < 0.05). Moreover, the correlation between CDC25C expression and infiltration of 24 immune cells was analyzed. Most immune cell infiltration negatively correlated with CDC25C expression, including CD8+ T cells, B cells, NK cells, and DC (all P values < 0.05) (Figure 5B). In contrast, CDC25C positively correlated with Th2 cells and Treg (all P values < 0.05) (Figure 5B). The TISIDB database was further explored, and similar results were obtained (Figure S2). Moreover, it was observed that CDC25C copy number was related to immune cell infiltration in LUAD tissues. Deep deletion of CDC25C correlated with higher immune cell infiltration (Figure 5C).
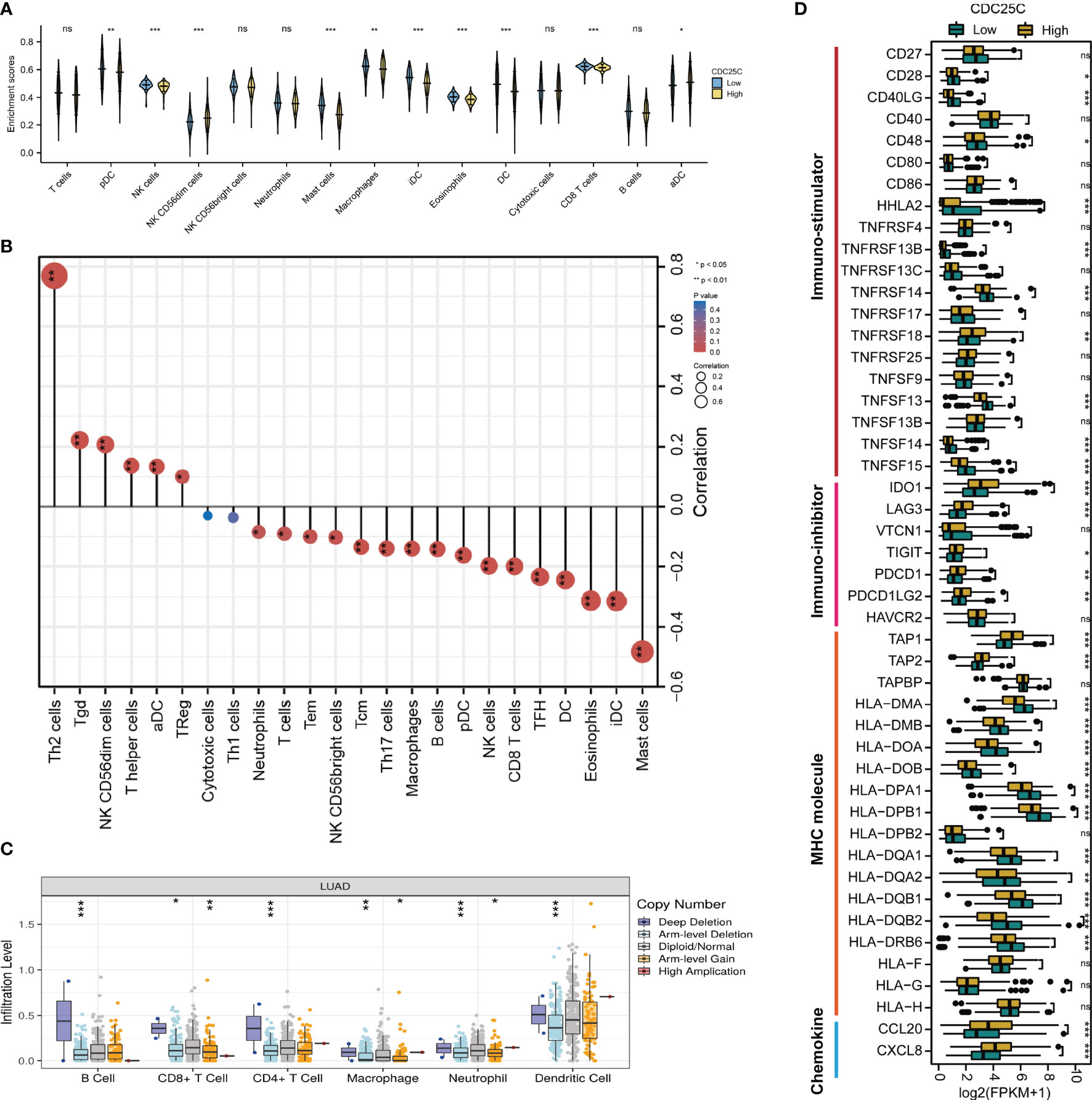
Figure 5 Characteristics of CDC25C immune cell infiltration. (A) Infiltration levels of various types of immune cells in high CDC25C and low CDC25C expression groups. (B) The correlation between CDC25C expression and immune cell infiltration. (C) Relationship between CDC25C copy number and immune cell infiltration in LUAD. (D) Comparison of the expression of immune-related signatures, including immuno-stimulators, immuno-inhibitors, MHC molecules, and chemokines, between high CDC25C and low CDC25C expression LUAD (not, not significant, *P < 0.05, **P < 0.01, ***P < 0.001).
Multiple immune-related signatures, including immuno-stimulators, immuno-inhibitors, MHC molecules, and chemokines were compared between high- and low- CDC25C expression LUAD. Regarding immuno-stimulators, it was demonstrated that CD28, CD40LG, CD48, HHLA2, TNFRSF13B, TNFRSF14, TNFSF13, TNFSF14, and TNFSF15 were dramatically abundant in low-CDC25C expression LUAD (all P values < 0.05) (Figure 5D). Conversely, various immuno-inhibitors, including IDO1, LAG3, TIGIT, PDCD1, and PDCD1LG2, were more abundant in high-CDC25C expression LUAD (all P values < 0.05) (Figure 5D). In addition, we found that most MHC molecules were more highly expressed in low-CDC25C LUAD (most P values < 0.05) (Figure 5D). Besides, compared to that in low-CDC25C expression LUAD, chemokines, CCL20 and CXCL8, were significantly higher in high-CDC25C expression LUAD (all P values < 0.05) (Figure 5D).
Correlation Between CDC25C Expression and the Efficacy of Nivolumab in Patients With LUAD
To further verify the relationship between CDC25C expression and the efficacy of ICIs therapy, the GSE126044 dataset was downloaded, involving 16 patients receiving nivolumab, of which 7 patients with LUAD and 9 patients with LUSC. Firstly, the association of CDC25C expression with survival and response was analyzed using data from these 16 patients. There was a trend towards shorter PFS for non-small cell lung cancer patients with higher CDC25C expression (high vs. low: 1.55 months vs. 5.45 months, P = 0.0693) (Figure S3A). Besides, the non-response rate of 87.5% for patients with high CDC25C expression was higher than 50% for patients with low CDC25C expression (Figure S3B). Next, data regarding 7 LUAD samples were collected to further analyze the effect of CDC25C on the survival of LUAD patients treated with nivolumab. Notably, the analysis demonstrated that higher CDC25C expression was associated with shorter PFS (high vs. low: 1.0 month vs. 8.55 months, P = 0.0143) (Figure 6A). In addition, higher CDC25C expression correlated with lower response to nivolumab, with a response rate of 0% in the high CDC25C expression group and 50% in the low CDC25C expression group (Figure 6B).
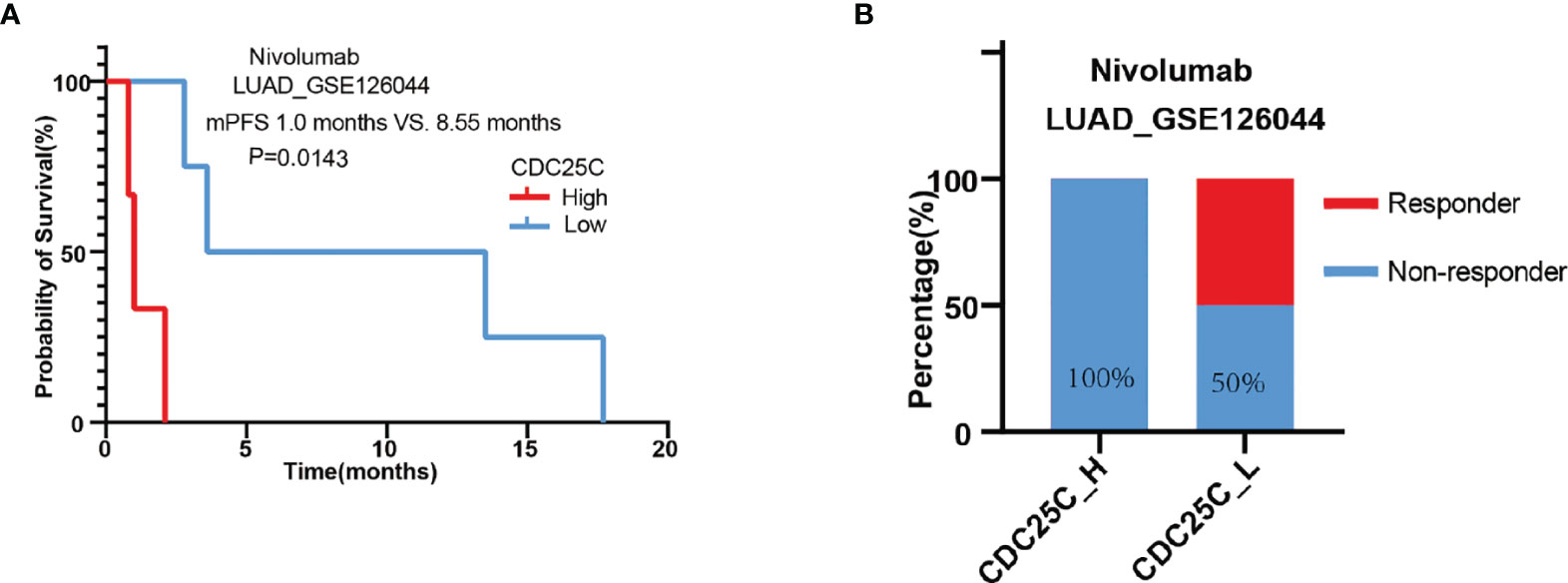
Figure 6 Correlation between CDC25C expression and outcomes of LUAD patients treated with nivolumab. (A) The correlation between CDC25C expression and PFS in LUAD patients treated with nivolumab. (B) The response rate between high and low CDC25C expression in LUAD patients treated with nivolumab.
Identification of 10 Hub Genes Co-Expressed With CDC25C Using the STRING Database
Through the STRING database, we found 10 hub genes that were co-expressed with CDC25C, namely CCNB1, CCNB2, CHEK1, CHEK2, PLK1, PLK3, YWHAB, YWHAE, YWHAZ and CDK1 (Figure 7A). By reviewing the literature, the main functions of these hub genes were summarized in Table S1. As shown in Figures 7B–K, these hub genes were closely related to CDC25C. Next, the expression of the 10 hub genes was explored using LUAD data from the TCGA database. The results showed that nine genes were differentially expressed in LUAD versus normal tissues (all P values < 0.01), in which seven genes were upregulated (CCNB1, CCNB2, CHEK1, CHEK2, PLK1, YWHAZ and CDK1) and two genes were downregulated (PLK3 and YWHAB) (Figure 8A). Moreover, the same data was used to explore the prognostic value of these hub genes in LUAD, of which higher expression of six genes (CCNB1, CCNB2, CHEK1, PLK1, YWHAZ and CDK1) was associated with a shorter OS, while the other four genes (CHEK2, PLK3, YWHAB and YWHAE) were not significantly associated with OS (Figures 8B–K). Notably, the six co-expressed genes (CCNB1, CCNB2, CHEK1, PLK1, YWHAZ and CDK1) could compose a better predictive model for the survival of LUAD patients (Figure S4).
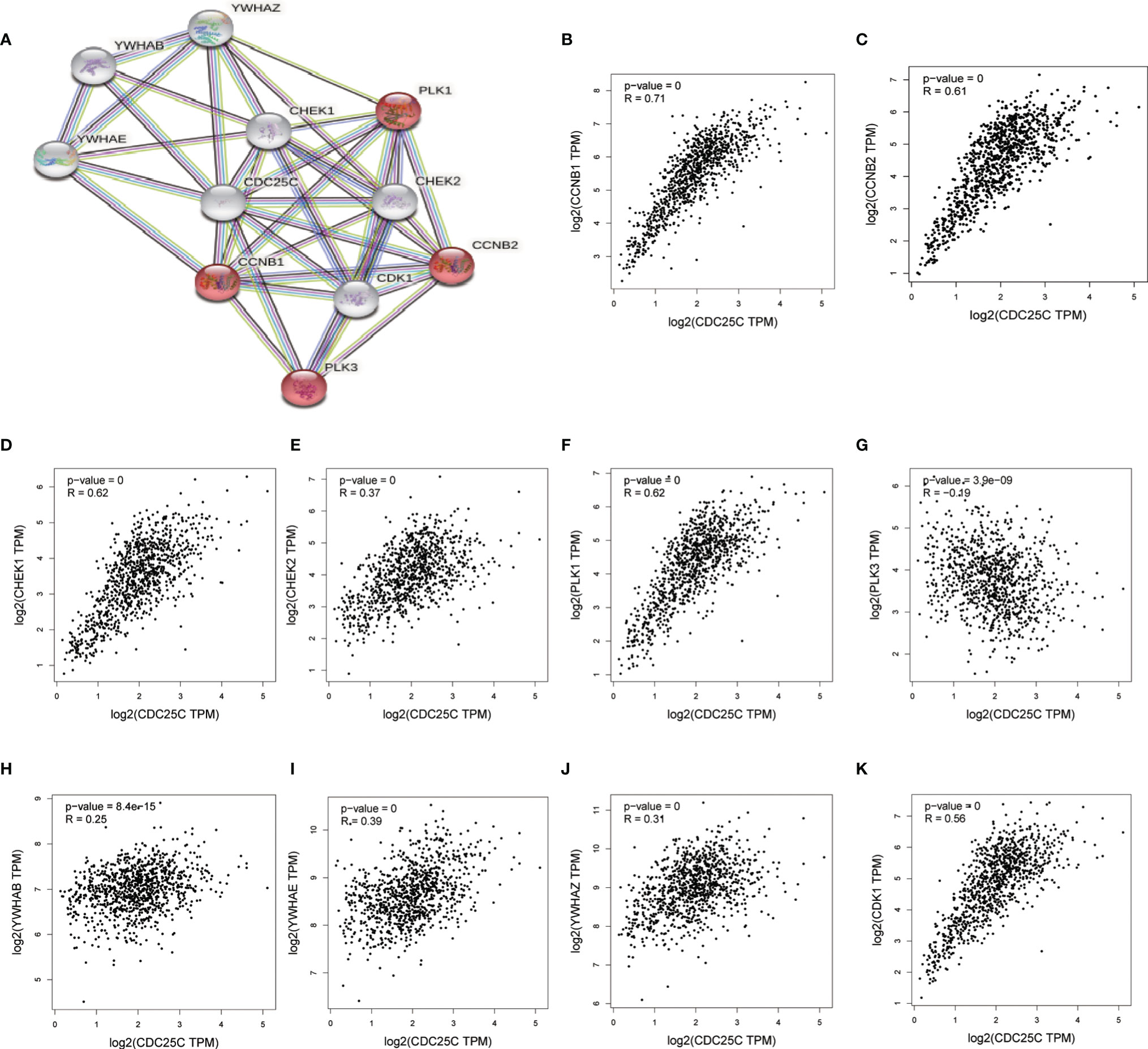
Figure 7 Identification of 10 hub genes closely related to CDC25C using STRING. (A) The PPI network plot for CDC25C including 10 hub genes co-expressed with CDC25C (B–K) Correlation analysis between CDC25C and 10 genes co-expressed with CDC25C using GEPIA (CCNB1, CCNB2, CHEK1, CHEK2, PLK1, PLK3, YWHAB, YWHAE, YWHAZ, CDK1).
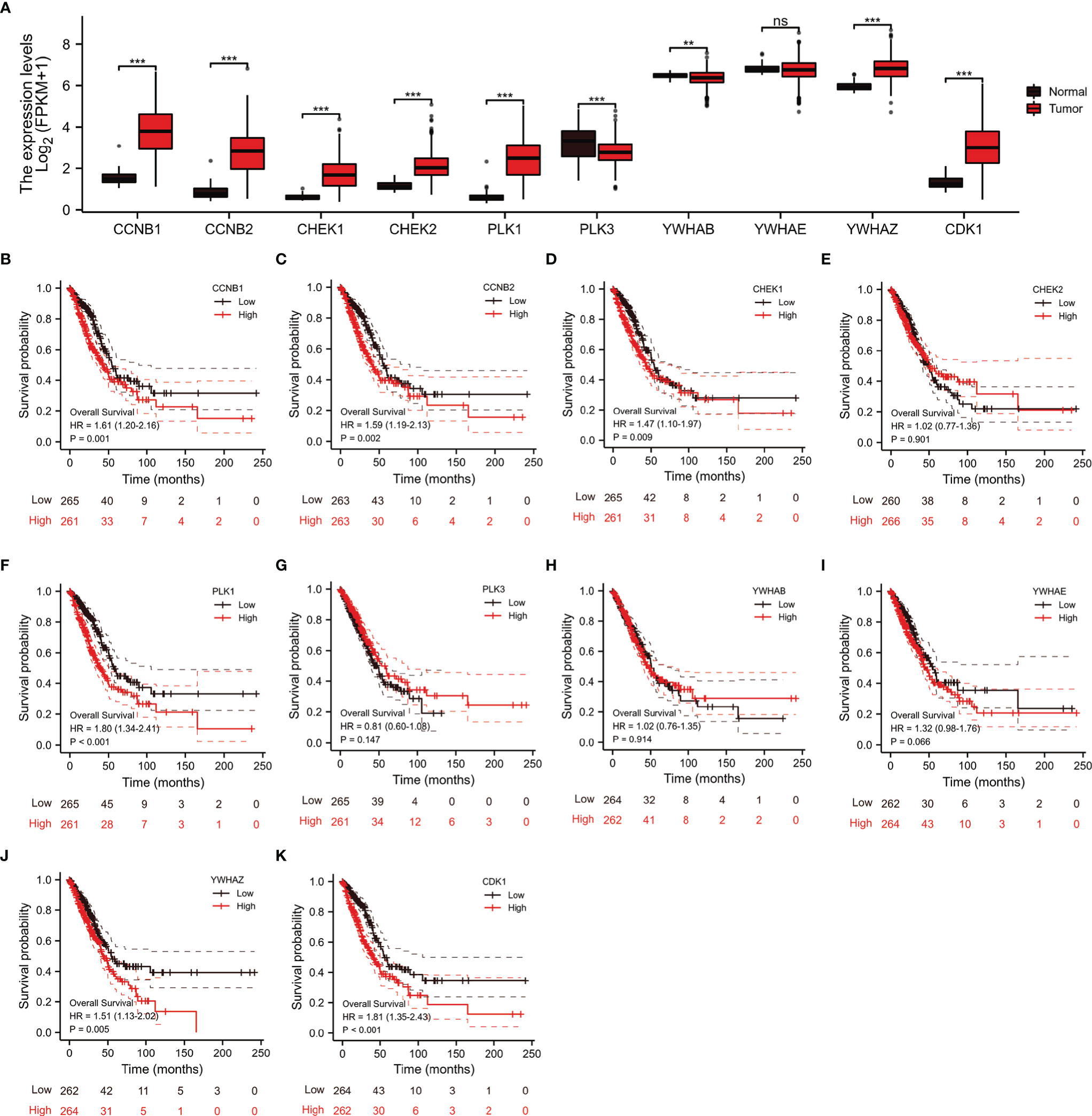
Figure 8 Analysis of the expression and prognostic significance of 10 genes in LUAD patients from the TCGA database. (A) Expression of 10 genes in LUAD versus normal tissue. (B–K) Association between 10 genes expression and OS in patients with LUAD (ns, not significant, **P < 0.01, ***P < 0.001).
The Expression and Prognostic Value of CDC25C in Pan-Cancer
The pan-cancer analysis was performed to explore the role of CDC25C in other cancers. Through UALCAN database, the CDC25C expression was found significantly higher in 22 cancers when compared to that of normal tissues (Figure 9A). Similar results were obtained in the Oncomine database (Figure 9C). Figure 9B showed the relative amount of CDC25C expression in 33 cancers, in which CDC25C expression in LUAD was at an intermediate level. Strikingly, CDC25C expression was significantly correlated with the OS of 13 cancers, among which negative correlations were observed in 9 cancers (all P values < 0.05) (Figures 9D–L), and positive correlations were observed in 4 cancers (all P values < 0.05) (Figures 9M–P). Survival analysis in the other 18 cancers showed no evident correlation between CDC25C expression and OS (Figure S5). Based on the above analyses, it was revealed that the effect of CDC25C varied among different cancers.
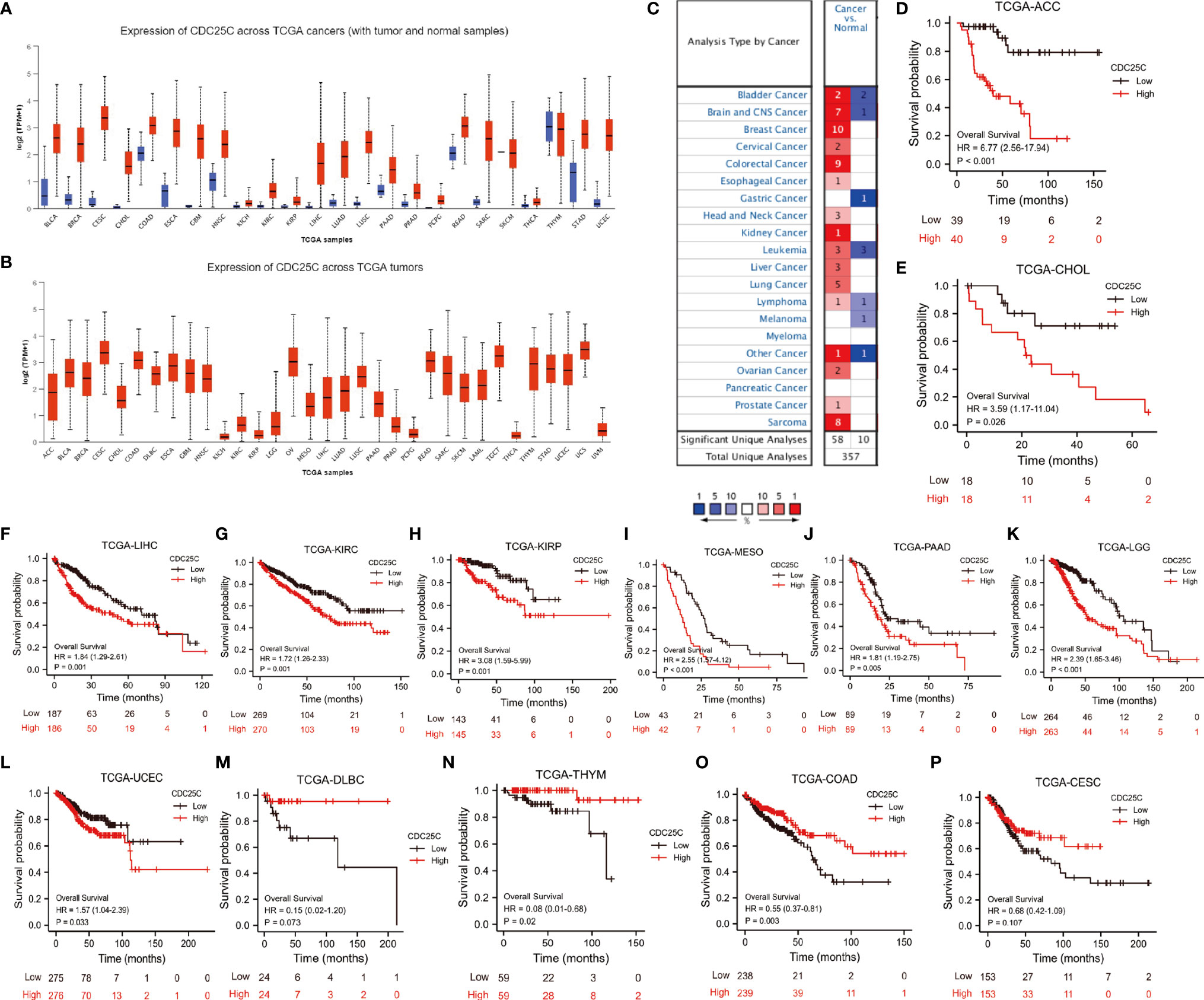
Figure 9 The Expression and prognostic value of CDC25C in Pan-Cancer. (A) Expression of CDC25C among multiple human tumors and corresponding normal tissues in the UALCAN database. ◼ is tumor, ◼ is normal tissue. (B) Expression of CDC25C in 33 tumors based on UALCAN database. (C) Expression of CDC25C among multiple human tumors and corresponding normal tissues in the Oncomine database. (D–L) In 9 kinds of tumors from the TCGA database, high CDC25C expression correlated with poor prognosis. (M–P) In 4 kinds of tumors from the TCGA database, higher CDC25C expression correlated with better prognosis. (ACC, adrenocortical carcinoma; CHOL, cholangiocarcinoma; LIHC, liver hepatocellular carcinoma; KIRC, kidney renal clear cell carcinoma; KIRP, kidney renal papillary cell carcinoma; MESO, kesothelioma; PAAD, pancreatic adenocarcinoma; LGG,brain lower grade glioma; UCEC, uterine corpus endometrial carcinoma; DLBC, lymphoid neoplasm diffuse large B-cell lymphoma; THYM, thymoma; COAD, colon adenocarcinoma; CESC, cervical squamous cell carcinoma and endocervical adenocarcinoma).
Discussion
The approval of ICIs in lung cancer is a significant milestone in the history of lung cancer treatment. However, only limited patients respond to immunotherapy. Even though predictive markers such as PD-L1 and TMB have been identified, clinicians failed to precisely and sensitively screen the population that would benefit from ICIs therapy (12). Therefore, more biomarkers are needed to accurately predict the efficacy of ICIs and provide clues to overcome drug resistance.
Previous studies have found that an abnormal cell cycle is closely related to uncontrollable tumor cell proliferation (37). CDC25C, a cell cycle regulatory protein, has been confirmed to be associated with tumorigenesis and tumor progression (38, 39). Consistently, LUAD of high CDC25C expression was mainly enriched in mitosis-related phases, indicating the critical role of CDC25C in LUAD proliferation. In the study, the expression of CDC25C and its relationship with the prognosis of LUAD patients were evaluated. CDC25C expression was upregulated in LUAD compared to normal tissue in multiple databases, including TCGA, Oncomine, and HPA. Furthermore, q-PCR showed that CDC25C expression was higher in LUAD cell lines (PC9 and H1299) than in the normal lung epithelial tissue cell line (BEAS-2B), which was consistent with the previously published report (40). In vulvar squamous cell carcinomas, overexpression of CDC25C was associated with a later FIGO stage (41). However, there are few studies on the association of CDC25C with LUAD. Our study revealed that higher CDC25C expression was associated with a shorter OS using multiple databases, including the TCGA and GEO databases. Moreover, multivariate and univariate Cox regression analyses confirmed that CDC25C was an independent prognostic factor in LUAD. In addition, higher CDC25C expression correlated with a later T-stage, N-stage, M-stage, and pathological stage, suggesting that CDC25C could be a potential biomarker for tumor staging. TP53, a tumor suppressor gene regulating cell cycle transcription (42), whose mutation is associated with an unrestricted cell cycle (43). In line with this, higher CDC25C expression was observed in TP53 mutant tumors, suggesting that CDC25C may be an essential mediator of TP53-mediated tumor progression.
Multiple studies have proven that abnormal cell cycle activity is strongly associated with chemotherapy resistance (17). Notably, recent studies have revealed that cell cycle-related genes could modulate the TME influencing the efficacy of immunotherapy (44). CDK4/6 inhibitors have been proved to promote CD8+ T memory cell formation, thereby enhancing anti-tumor immunity (26). Besides, CDK7 inhibitors could enhance the efficacy of anti-PD-1 therapy in NSCLC (27, 45). Additionally, Petroni etc. have revealed that the amplification of cyclin D1 and CDK4, two cell cycle-related genes, was associated with a low response to ICIs treatment in solid tumors (46). Strikingly, our study first demonstrated that higher CDC25C expression was associated with shorter PFS and lower response rate in LUAD patients treated with nivolumab, suggesting that CDC25C is likely to be associated with immunotherapy efficacy. Given that the analysis was based on 7 LUAD patients, its reliability extremely requires further validation with larger samples. It is acknowledged that TME is a critical factor in modulating the efficacy of immunotherapy (47). In our study, CDC25C was significantly associated with the infiltration levels of multiple immune cells, with negative correlations with anti-tumor immune cells (CD8+ T cells, B cells, NK cells, and DC) (48), but positive correlations with immunosuppressive immune cells (Th2 and Treg cells) (48). In other words, these findings indicated that CDC25C promotes an immunosuppressive TME, which could impair the efficacy of immunotherapy. Additionally, immuno-stimulators and immuno-inhibitors are crucial in modulating cancer-immunity cycle (49). Higher levels of immuno-stimulators and lower levels of immuno-inhibitors in low CDC25C LUAD could promote enhanced anti-tumor immunity, supporting prolonged survival of patients with low CDC25C. MHC molecules, playing an essential role in antigen presentation, are indispensable in the recognizing and killing of tumor cells by immune cells (50). Lower MHC molecules in high CDC25C LUAD could mediate tumor immune escape, weakening the efficacy of immunotherapy. Higher CCL20 and CXCL8 in high CDC25C LUAD, which could attract Treg cells into tumors to form an immunosuppressive TME (51), providing stronger evidence for poor prognosis in nivolumab-treated patients with high CDC25C. Taken together, these findings suggested that high CDC25C facilitates an immunosuppressive TME, providing potential mechanisms for shortened survival and low response in nivolumab-treated patients with high CDC25C and potentially paving the way for the combination of CDC25C inhibitors with immunotherapy. Growing evidence suggests the critical role of metabolism in immunotherapy (52). Strong correlation of CDC25C with metabolism-related processes suggested that metabolism may be another vital mechanism underlying the impact of CDC25C on immunotherapy.
The expression and prognostic value of CDC25C in 31 tumors were further explored. Of note, prolonged survival with high CDC25C expression was observed in several cancers. Indeed, the effects of genes on cancer progression or prognosis are not only associated with intrinsic properties of genes themselves, but also subject to a variety of factors, such as treatment regimens and tumor types (53). Although there is sufficient evidence that CDC25C promotes tumor progression, high CDC25C expression enhances the sensitivity of esophageal cancer to radiotherapy (54). KRAS, the oncogene mutated highly in cancers, is associated with poor prognosis of LUAD (55). However, patients harboring KRAS mutation are more vulnerable to benefit from ICIs (56). In addition, ID4 acts as a tumor suppressor in prostate cancer, while serves as a proto-oncogene in bladder cancer (57). Therefore, we hypothesize that the disparity of prognosis for CDC25C in diverse tumors may also be related to these factors.
Here we demonstrated that a higher CDC25C expression was associated with shorter survival and lower response to ICIs, as well as an immunosuppressive tumor microenvironment, in patients with LUAD. These finding suggest that targeting inhibition of key regulators of cell cycle in cancers might augment anti-tumor immunity and increase the response to ICIs treatment, which provide a rational for further combination therapies. Even though a comprehensive and systematic analysis was performed, there are still some limitations. The data in this study were extracted mainly from public and different databases, and some specific information is not available, so our results are prone to errors. Although we first confirmed the role of CDC25C in ICIs treatment, the conclusion is likely to be biased due to the small sample size, thus validation in a larger sample is extremely warranted. Besides, the potential mechanism of how CDC25C modulates the efficacy of immunotherapy requires more investigation using in vitro experiments and in vivo animal models. Nevertheless, multiple methods were used to validate and similar results were obtained in our analyses, giving a more reliable relationship that high CDC25C expression correlated with an immunosuppressive TME of LUAD. Undeniably, since the study focused on the relationship between CDC25C and LUAD, only survival and expression analyses were performed in the pan-cancer. More in-depth studies of each type of cancer are warranted.
Conclusion
In conclusion, it was demonstrated that CDC25C expression was upregulated in LUAD compared to normal lung tissue at the mRNA and protein levels. Based on survival analysis, CDC25C was confirmed to be an independent risk factor for LUAD. Most importantly, higher CDC25C expression was associated with a shorter PFS and lower response rate for LUAD patients treated with nivolumab, which provided more robust evidence for the role of the cell cycle in immunotherapy. In addition, higher CDC25C expression was associated with an immunosuppressive TME, suggesting the role of cell cycle in the TME modulation.
Data Availability Statement
The datasets presented in this study can be found in online repositories. The names of the repository/repositories and accession number(s) can be found in the article/Supplementary Material.
Author Contributions
XW and YL conceived and guide the study. WZ and XS collected the data, performed analysis, and drafted the manuscript. FY, NL, WH, and HX collected the literature, edited figures and revised the manuscript. All authors contributed to the article and approved the final version.
Funding
This work was supported by grants from the National Natural Science Foundation of China (No. 81874044) and the Shandong Provincial Natural Science Foundation (No.ZR2020MH236 and No. ZR2019MH050).
Conflict of Interest
The authors declare that the research was conducted in the absence of any commercial or financial relationships that could be construed as a potential conflict of interest.
Publisher’s Note
All claims expressed in this article are solely those of the authors and do not necessarily represent those of their affiliated organizations, or those of the publisher, the editors and the reviewers. Any product that may be evaluated in this article, or claim that may be made by its manufacturer, is not guaranteed or endorsed by the publisher.
Supplementary Material
The Supplementary Material for this article can be found online at: https://www.frontiersin.org/articles/10.3389/fonc.2022.867788/full#supplementary-material
Abbreviations
CDC25C, cell division cycle 25c; DSS, disease specific survival; GEO, Gene Expression Omnibus; GO, Gene Ontology; GSEA, Gene Set Enrichment Analysis; HPA, The Human Protein Atlas; ICIs, immune checkpoint inhibitors; KEGG, Kyoto Encyclopedia of Genes and Genomes; LUAD, lung adenocarcinoma; LUSC, lung squamous carcinoma; NSCLC, non-small cell lung cancer; OS, overall survival; q-PCR, real-time quantitative polymerase chain reaction; PFS, progression free survival; PFI, progress free interval; PPI, protein-protein interaction; TCGA, The Cancer Genome Atlas; TMB, tumor mutational burden; TME, tumor microenvironment.
References
1. Sung H, Ferlay J, Siegel RL, Laversanne M, Soerjomataram I, Jemal A, et al. Global Cancer Statistics 2020: GLOBOCAN Estimates of Incidence and Mortality Worldwide for 36 Cancers in 185 Countries. CA Cancer J Clin (2021) 3:209–49. doi: 10.3322/caac.21660
2. Zhang Y, Luo G, Etxeberria J, Hao Y. Global Patterns and Trends in Lung Cancer Incidence: A Population-Based Study. J Thorac Oncol (2021) 6:933–44. doi: 10.1016/j.jtho.2021.01.1626
3. Thai AA, Solomon BJ, Sequist LV, Gainor JF, Heist RS. Lung Cancer. Lancet (2021) 10299:535–54. doi: 10.1016/s0140-6736(21)00312-3
4. Herbst RS, Morgensztern D, Boshoff C. The Biology and Management of non-Small Cell Lung Cancer. Nature (2018) 7689:446–54. doi: 10.1038/nature25183
5. Noone A, Howlader N, Krapcho M, Miller D, Brest A, Yu M, et al. SEER Cancer Statistics Review, 1975-2015 (2018). Available at: https://seer.cancer.gov/csr/1975_2015/.
6. Rangachari D, Costa DB. Five-Year Overall Survival for Patients With Advanced Non‒Small-Cell Lung Cancer Treated With Pembrolizumab: Results From the Phase I KEYNOTE-001 Study. J Clin Oncol (2019) 28:2511–3. doi: 10.1200/JCO.19.01207
7. Reck MA-O, Rodríguez-Abreu DA-O, Robinson AA-O, Hui R, Csőszi T, Fülöp A, et al. Five-Year Outcomes With Pembrolizumab Versus Chemotherapy for Metastatic Non-Small-Cell Lung Cancer With PD-L1 Tumor Proportion Score ≥ 50. J Clin Oncol (2021) 39:2339–49. doi: 10.1200/JCO.21.00174
8. Brahmer J, Reckamp KL, Baas P, Crino L, Eberhardt WE, Poddubskaya E, et al. Nivolumab Versus Docetaxel in Advanced Squamous-Cell Non-Small-Cell Lung Cancer. N Engl J Med (2015) 2:123–35. doi: 10.1056/NEJMoa1504627
9. Rittmeyer A, Barlesi F, Waterkamp D, Park K, Ciardiello F, von Pawel J, et al. Atezolizumab Versus Docetaxel in Patients With Previously Treated non-Small-Cell Lung Cancer (OAK): A Phase 3, Open-Label, Multicentre Randomised Controlled Trial. Lancet (2017) 10066:255–65. doi: 10.1016/s0140-6736(16)32517-x
10. Reck M, Rodríguez-Abreu D, Robinson AG, Hui R, Csőszi T, Fülöp A, et al. Pembrolizumab Versus Chemotherapy for PD-L1-Positive Non-Small-Cell Lung Cancer. N Engl J Med (2016) 375: 1533–4406. doi: 10.1056/NEJMoa1606774
11. Herbst RS, Giaccone G, de Marinis F, Reinmuth N, Vergnenegre A, Barrios CH, et al. Atezolizumab for First-Line Treatment of PD-L1-Selected Patients With NSCLC. N Engl J Med (2020) doi: 10.1056/NEJMoa1917346
12. Morad G, Helmink BA, Sharma P, Wargo JA. Hallmarks of Response, Resistance, and Toxicity to Immune Checkpoint Blockade. Cell (2021) 21:5309–37. doi: 10.1016/j.cell.2021.09.020
13. Gandhi L, Rodriguez-Abreu D, Gadgeel S, Esteban E, Felip E, De Angelis F, et al. Pembrolizumab Plus Chemotherapy in Metastatic Non-Small-Cell Lung Cancer. N Engl J Med (2018) 22:2078–92. doi: 10.1056/NEJMoa1801005
14. Socinski MA, Jotte RM, Cappuzzo F, Orlandi F, Stroyakovskiy D, Nogami N, et al. Atezolizumab for First-Line Treatment of Metastatic Nonsquamous NSCLC. N Engl J Med (2018) 24:2288–301. doi: 10.1056/NEJMoa1716948
15. Guo Y, Lu X, Chen Y, Rendon B, Mitchell RA, Cuatrecasas M, et al. Zeb1 Induces Immune Checkpoints to Form an Immunosuppressive Envelope Around Invading Cancer Cells. Sci Adv (2021) 21:eabd7455. doi: 10.1126/sciadv.abd7455
16. Suski JM, Braun M, Strmiska V, Sicinski P. Targeting Cell-Cycle Machinery in Cancer. Cancer Cell (2021) 6:759–78. doi: 10.1016/j.ccell.2021.03.010
17. Liu J, Peng Y, Wei W. Cell Cycle on the Crossroad of Tumorigenesis and Cancer Therapy. Trends Cell Biol (2022) 1:30–44. doi: 10.1016/j.tcb.2021.07.001
18. Piezzo M, Cocco S, Caputo R, Cianniello D, Gioia GD, Lauro VD, et al. Targeting Cell Cycle in Breast Cancer: CDK4/6 Inhibitors. Int J Mol Sci (2020) 21:6479. doi: 10.3390/ijms21186479
19. Liu K, Zheng M, Lu R, Du J, Zhao Q, Li Z, et al. The Role of CDC25C in Cell Cycle Regulation and Clinical Cancer Therapy: A Systematic Review. Cancer Cell Int (2020) 20:213. doi: 10.1186/s12935-020-01304-w
20. Skowron KB, Pitroda SP, Namm JP, Balogun O, Beckett MA, Zenner ML, et al. Basal Tumor Cell Isolation and Patient-Derived Xenograft Engraftment Identify High-Risk Clinical Bladder Cancers. Sci Rep (2016) 6:35854. doi: 10.1038/srep35854
21. Li FN, Zhang QY, Li O, Liu SL, Yang ZY, Pan LJ, et al. ESRRA Promotes Gastric Cancer Development by Regulating the CDC25C/CDK1/CyclinB1 Pathway via DSN1. Int J Biol Sci (2021) 8:1909–24. doi: 10.7150/ijbs.57623
22. Maimaiti A, Aizezi A, Anniwaer J, Ayitula, Ali B, Dilixiati M. Zinc Finger of the Cerebellum 5 Promotes Colorectal Cancer Cell Proliferation and Cell Cycle Progression Through Enhanced CDK1/CDC25c Signaling. Arch Med Sci (2021) 2:449–61. doi: 10.5114/aoms.2019.89677
23. Goel S, DeCristo MJ, Watt AC, BrinJones H, Sceneay J, Li BB, et al. CDK4/6 Inhibition Triggers Anti-Tumour Immunity. Nature (2017) 7668:471–5. doi: 10.1038/nature23465
24. Deng J, Wang ES, Jenkins RW, Li S, Dries R, Yates K, et al. CDK4/6 Inhibition Augments Antitumor Immunity by Enhancing T-Cell Activation. Cancer Discov (2018) 2:216–33. doi: 10.1158/2159-8290.CD-17-0915
25. Lai AY, Sorrentino JA, Dragnev KH, Weiss JM, Owonikoko TK, Rytlewski JA, et al. CDK4/6 Inhibition Enhances Antitumor Efficacy of Chemotherapy and Immune Checkpoint Inhibitor Combinations in Preclinical Models and Enhances T-Cell Activation in Patients With SCLC Receiving Chemotherapy. J Immunother Cancer (2020) 8:e000847. doi: 10.1136/jitc-2020-000847
26. Heckler M, Ali LR, Clancy-Thompson E, Qiang L, Ventre KS, Lenehan P, et al. Inhibition of CDK4/6 Promotes CD8 T-Cell Memory Formation. Cancer Discov (2021) 10:2564–81. doi: 10.1158/2159-8290.CD-20-1540
27. Wang J, Zhang R, Lin Z, Zhang S, Chen Y, Tang J, et al. CDK7 Inhibitor THZ1 Enhances antiPD-1 Therapy Efficacy via the P38alpha/MYC/PD-L1 Signaling in Non-Small Cell Lung Cancer. J Hematol Oncol (2020) 1:99. doi: 10.1186/s13045-020-00926-x
28. Rhodes DR, Kalyana-Sundaram S, Mahavisno V, Varambally R, Yu J, Briggs BB, et al. Oncomine 3.0: Genes, Pathways, and Networks in a Collection of 18,000 Cancer Gene Expression Profiles. Neoplasia (2007) 2:166–80. doi: 10.1593/neo.07112
29. Chandrashekar DS, Bashel B, Balasubramanya SAH, Creighton CJ, Ponce-Rodriguez I, Chakravarthi B, et al. UALCAN: A Portal for Facilitating Tumor Subgroup Gene Expression and Survival Analyses. Neoplasia (2017) 8:649–58. doi: 10.1016/j.neo.2017.05.002
30. Liu J, Lichtenberg T, Hoadley KA, Poisson LM, Lazar AJ, Cherniack AD, et al. An Integrated TCGA Pan-Cancer Clinical Data Resource to Drive High-Quality Survival Outcome Analytics. Cell (2018) 2:400–16e411. doi: 10.1016/j.cell.2018.02.052
31. Robinson MD, McCarthy DJ, Smyth GK. Edger: A Bioconductor Package for Differential Expression Analysis of Digital Gene Expression Data. Bioinformatics (2010) 1:139–40. doi: 10.1093/bioinformatics/btp616
32. Yu G, Wang LG, Han Y, He QY. Clusterprofiler: An R Package for Comparing Biological Themes Among Gene Clusters. OMICS (2012) 5:284–7. doi: 10.1089/omi.2011.0118
33. Hanzelmann S, Castelo R, Guinney J. GSVA: Gene Set Variation Analysis for Microarray and RNA-Seq Data. BMC Bioinf (2013) 14:7. doi: 10.1186/1471-2105-14-7
34. Li T, Fan J, Wang B, Traugh N, Chen Q, Liu JS, et al. TIMER: A Web Server for Comprehensive Analysis of Tumor-Infiltrating Immune Cells. Cancer Res (2017) 21:e108–10. doi: 10.1158/0008-5472.CAN-17-0307
35. Ru B, Wong CN, Tong Y, Zhong JY, Zhong SSW, Wu WC, et al. TISIDB: An Integrated Repository Portal for Tumor-Immune System Interactions. Bioinformatics (2019) 20:4200–2. doi: 10.1093/bioinformatics/btz210
36. Szklarczyk D, Gable AL, Nastou KC, Lyon D, Kirsch R, Pyysalo S, et al. The STRING Database in 2021: Customizable Protein-Protein Networks, and Functional Characterization of User-Uploaded Gene/Measurement Sets. Nucleic Acids Res (2021) D1:D605–12. doi: 10.1093/nar/gkaa1074
37. Matthews HK, Bertoli C, de Bruin RAM. Cell Cycle Control in Cancer. Nat Rev Mol Cell Biol (2021) 23:74–88. doi: 10.1038/s41580-021-00404-3
38. Abraham RT. Cell Cycle Checkpoint Signaling Through the ATM and ATR Kinases. Genes Dev (2001) 17:2177–96. doi: 10.1101/gad.914401
39. Wang JN, Zhang ZR, Che Y, Yuan ZY, Lu ZL, Li Y, et al. Acetyl-Macrocalin B, an Ent-Kaurane Diterpenoid, Initiates Apoptosis Through the ROS-P38-Caspase 9-Dependent Pathway and Induces G2/M Phase Arrest via the Chk1/2-Cdc25C-Cdc2/cyclin B Axis in Non-Small Cell Lung Cancer. Cancer Biol Ther (2018) 7:609–21. doi: 10.1080/15384047.2018.1449613
40. Chen CY, Hsu YL, Tsai YC, Kuo PL. Kotomolide A Arrests Cell Cycle Progression and Induces Apoptosis Through the Induction of ATM/p53 and the Initiation of Mitochondrial System in Human Non-Small Cell Lung Cancer A549 Cells. Food Chem Toxicol (2008) 7:2476–84. doi: 10.1016/j.fct.2008.04.016
41. Wang Z, Trope CG, Florenes VA, Suo Z, Nesland JM, Holm R. Overexpression of CDC25B, CDC25C and Phospho-CDC25C (Ser216) in Vulvar Squamous Cell Carcinomas Are Associated With Malignant Features and Aggressive Cancer Phenotypes. BMC Cancer (2010) 10:233. doi: 10.1186/1471-2407-10-233
42. Hainaut P, Pfeifer GP. Somatic TP53 Mutations in the Era of Genome Sequencing. Cold Spring Harb Perspect Med (2016) 6:a026179. doi: 10.1101/cshperspect.a026179
43. Marei HE, Althani A, Afifi N, Hasan A, Caceci T, Pozzoli G, et al. P53 Signaling in Cancer Progression and Therapy. Cancer Cell Int (2021) 1:703. doi: 10.1186/s12935-021-02396-8
44. Li J, Stanger BZ. Cell Cycle Regulation Meets Tumor Immunosuppression. Trends Immunol (2020) 10:859–63. doi: 10.1016/j.it.2020.07.010
45. Zhang H, Christensen CL, Dries R, Oser MG, Deng J, Diskin B, et al. CDK7 Inhibition Potentiates Genome Instability Triggering Anti-Tumor Immunity in Small Cell Lung Cancer. Cancer Cell (2020) 1:37–54.e39. doi: 10.1016/j.ccell.2019.11.003
46. Petroni G, Formenti SC, Chen-Kiang S, Galluzzi L. Immunomodulation by Anticancer Cell Cycle Inhibitors. Nat Rev Immunol (2020) 11:669–79. doi: 10.1038/s41577-020-0300-y
47. Chen DS, Mellman I. Elements of Cancer Immunity and the Cancer-Immune Set Point. Nature (2017) 7637:321–30. doi: 10.1038/nature21349
48. Palucka AK, Coussens LM. The Basis of Oncoimmunology. Cell (2016) 6:1233–47. doi: 10.1016/j.cell.2016.01.049
49. Chen DS, Mellman I. Oncology Meets Immunology: The Cancer-Immunity Cycle. Immunity (2013) 1:1–10. doi: 10.1016/j.immuni.2013.07.012
50. Abualrous ET, Sticht J, Freund C. Major Histocompatibility Complex (MHC) Class I and Class II Proteins: Impact of Polymorphism on Antigen Presentation. Curr Opin Immunol (2021) 70:95–104. doi: 10.1016/j.coi.2021.04.009
51. Matsuo K, Yoshie O, Nakayama T. Multifaceted Roles of Chemokines and Chemokine Receptors in Tumor Immunity. Cancers (Basel) (2021) 13:6132. doi: 10.3390/cancers13236132
52. Elia I, Haigis MC. Metabolites and the Tumour Microenvironment: From Cellular Mechanisms to Systemic Metabolism. Nat Metab (2021) 1:21–32. doi: 10.1038/s42255-020-00317-z
53. Sack LM, Davoli T, Li MZ, Li Y, Xu Q, Naxerova K, et al. Profound Tissue Specificity in Proliferation Control Underlies Cancer Drivers and Aneuploidy Patterns. Cell (2018) 2:499–514.e423. doi: 10.1016/j.cell.2018.02.037
54. Li BZ, Chen ZL, Shi SS, Feng XL, Tan XG, Zhou F, et al. Overexpression of Cdc25C Predicts Response to Radiotherapy and Survival in Esophageal Squamous Cell Carcinoma Patients Treated With Radiotherapy Followed by Surgery. Chin J Cancer (2013) 7:403–9. doi: 10.5732/cjc.012.10233
55. Wood K, Hensing T, Malik R, Salgia R. Prognostic and Predictive Value in KRAS in Non-Small-Cell Lung Cancer: A Review. JAMA Oncol (2016) 6:805–12. doi: 10.1001/jamaoncol.2016.0405
56. Liu C, Zheng S, Jin R, Wang X, Wang F, Zang R, et al. The Superior Efficacy of Anti-PD-1/PD-L1 Immunotherapy in KRAS-Mutant Non-Small Cell Lung Cancer That Correlates With an Inflammatory Phenotype and Increased Immunogenicity. Cancer Lett (2020) 470:95–105. doi: 10.1016/j.canlet.2019.10.027
Keywords: CDC25C, immune checkpoint inhibitors, biomarker, lung adenocarcinoma, tumor microenvironment
Citation: Zhang W, Shang X, Yang F, Han W, Xia H, Liu N, Liu Y and Wang X (2022) CDC25C as a Predictive Biomarker for Immune Checkpoint Inhibitors in Patients With Lung Adenocarcinoma. Front. Oncol. 12:867788. doi: 10.3389/fonc.2022.867788
Received: 01 February 2022; Accepted: 04 April 2022;
Published: 29 April 2022.
Edited by:
Luciano Mutti, Temple University, United StatesReviewed by:
Rajat Thawani, Oregon Health & Science University, United StatesFabiana Napolitano, University of Naples Federico II, Italy
Copyright © 2022 Zhang, Shang, Yang, Han, Xia, Liu, Liu and Wang. This is an open-access article distributed under the terms of the Creative Commons Attribution License (CC BY). The use, distribution or reproduction in other forums is permitted, provided the original author(s) and the copyright owner(s) are credited and that the original publication in this journal is cited, in accordance with accepted academic practice. No use, distribution or reproduction is permitted which does not comply with these terms.
*Correspondence: Yanguo Liu, bGl1eWFuZ3VvQHNkdS5lZHUuY24=; Xiuwen Wang, eGl1d2Vud2FuZzEyQHNkdS5lZHUuY24=