- 1Department of Obstetrics and Gynecology, Tongji Medical College, Tongji Hospital, Huazhong University of Science and Technology, Wuhan, China
- 2Cancer Centre and Center of Reproduction, Development and Aging, Faculty of Health Sciences, University of Macau, Macau, Macau SAR, China
Cervical cancer remains a leading cause of cancer death in women, seriously threatening their physical and mental health. It is an easily preventable cancer with early screening and diagnosis. Although technical advancements have significantly improved the early diagnosis of cervical cancer, accurate diagnosis remains difficult owing to various factors. In recent years, artificial intelligence (AI)-based medical diagnostic applications have been on the rise and have excellent applicability in the screening and diagnosis of cervical cancer. Their benefits include reduced time consumption, reduced need for professional and technical personnel, and no bias owing to subjective factors. We, thus, aimed to discuss how AI can be used in cervical cancer screening and diagnosis, particularly to improve the accuracy of early diagnosis. The application and challenges of using AI in the diagnosis and treatment of cervical cancer are also discussed.
1 Introduction
Cervical cancer is one of the most common malignancies in women, with 604,000 new cases and 342,000 deaths in 2020 (1). It is the only cancer that can be eliminated via primary prevention strategies comprising a fully effective 9-valent human papillomavirus (HPV) vaccine, early detection, and timely treatment (2).
Almost all cases of cervical cancer are caused by persistent infection of the cervical epithelium with one of the 15 genotypes of the carcinogenic HPV. The four major steps in the development of cervical cancer are as follows: infection of the metaplastic epithelium at the cervical transformation zone, persistent HPV infection, progression of persistently infected epithelium to cervical precancer, and invasion through the basement membrane of the epithelium (3). The HPV vaccines can protect girls and young woman against infection with the HPV virus. But HPV vaccine coverage rate is very low at present (even in some developed countries) (2, 4) and the beneficiaries are limited to young women aged <26 in terms of 9-valent HPV vaccines. According to American Cancer Society, vaccinated women are also recommended that be screened the same as unvaccinated women because it is impossible to avoid risk completely (5). Hence, routine screening for cervical cancer is still important to women. Approximately 30% of cervical intraepithelial neoplasia (CIN) grade 3 lesions develop into invasive cancers within 30 years. Slow progression offers many opportunities for the detection and treatment of these lesions (6). Screening and treatment of precancerous lesions in women is a cost-effective way to prevent cervical cancer (7). Ideally, screening strategies should be able to detect early lesions that may develop into cervical cancer, while avoiding the detection of transient HPV infections and benign abnormalities that can lead to overtreatment and other hazards associated with screening (5). With the continuous improvement of screening techniques, there has been an increase in the detection rate of cervical cancer and a decrease in the mortality rate; however, most deaths occur in low- and middle-income countries (8). Despite new developments in effective screening programs, many of these cannot be implemented or maintained because of weak health infrastructure (9, 10). Moreover, the accuracy of manual screening is not always 100% (11), resulting in some related lesions that cannot be timely diagnosed. Thus, developing a more accurate and economical cervical cancer screening method is the main challenge for the early diagnosis of cervical cancer.
In recent years, AI has been increasingly applied in the diagnosis of various diseases, such as the classification of skin tumors (12, 13), diagnosis and classification of retinal diseases (14), and imaging diagnosis of tumors (15), and has shown promising application value. AI can automatically recognize images, extract features, learn classification, and process data using complex algorithms. The application of AI in the early screening and diagnosis of cervical cancer is conducive to addressing limited human resources and improving diagnostic accuracy.
This article aimed to introduce recent AI technologies and demonstrate their utility and potential for the screening and early diagnosis of cervical cancer. This review also discusses the current challenges and proposes future research directions.
2 Methods for Cervical Cancer Screening and Diagnosis
The latest World Health Organization guidelines recommend the following three screening methods for the early detection of cervical cancer: HPV testing, cytology (including traditional pap smear and liquid-based cytology smear), and visual inspection with acetic acid (VIA) (16). We focused on the first two methods because VIA is only used when the first two are not available. HPV testing and cytology uses brushed exfoliated cells from the cervix as test samples. HPV testing detects high-risk types of HPV infection in the cervix, whereas cytological examination uses a microscope to identify cells taken from the cervix for possible cervical cancer or precancerous lesions (17). Figure 1 shows the evolution of cervical cancer screening methods. However, colposcopy-guided biopsy remains as the gold standard for cervical cancer diagnosis, followed by staging according to the clinical examination and imaging results.
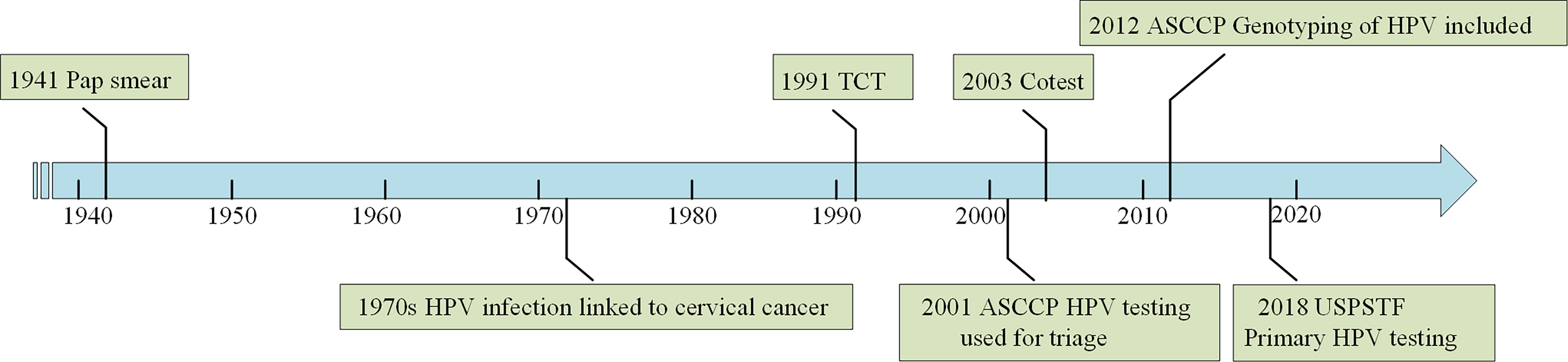
Figure 1 Evolution of cervical cancer screening methods. The figure shows major milestones in the evolution of cervical cancer screening. The main screening methods for cervical cancer are HPV testing and TCT (cytology) nowadays.
2.1 Introduction to Cytology
A conventional Pap smear (CPS) is a manual screening procedure used to identify and classify exfoliated cervical cells under a microscope according to the color and characteristics of the nucleus and cytoplasm (18). Liquid-based cytology (LBC) can improve preparation techniques (19). The LBC specimen is better fixed in glass slides, easier to preserve and perform artificial removal, and has a more uniform sample distribution than CPS (20).
Cytology results are described according to the Bethesda system (TBS) (21). Cervical cells are grouped into specific categories according to their abnormal changes about nuclear size, degree of dyeing etc (22). Abnormal epithelial cells include atypical squamous cells and atypical glandular cells. The TBS nomenclature details are listed in Table 1.
2.2 Introduction to Colposcopy
Colposcopy is defined as the use of a specific instrument to magnify the fully exposed cervix by 5 to 40 times for a real-time visual assessment of the cervix, especially the transformation area, for the detection of CIN or squamous intraepithelial lesion (SIL) and invasive cancer (23). A colposcopy-guided biopsy of the suspected site is performed to determine whether further treatment, such as conization or cryotherapy, is needed, which is important in patients with high-grade CIN or more severe disease (24).
2.3 Procedures for Early Screening and Diagnosis of Cervical Cancer
According to the latest recommendations of the American Cancer Society on cervical cancer screening, women with a cervix aged ≥25 years are recommended to undergo cervical cancer screening. For women between the ages of 25 and 65, primary HPV test should be performed every five years (5). If a primary HPV test is not available, co-testing (HPV testing in combination with cytology) or cytology evaluation can be performed every three years. Colposcopy or recommended screening methods can be performed based on the results (25), which are shown in the Figure 2.
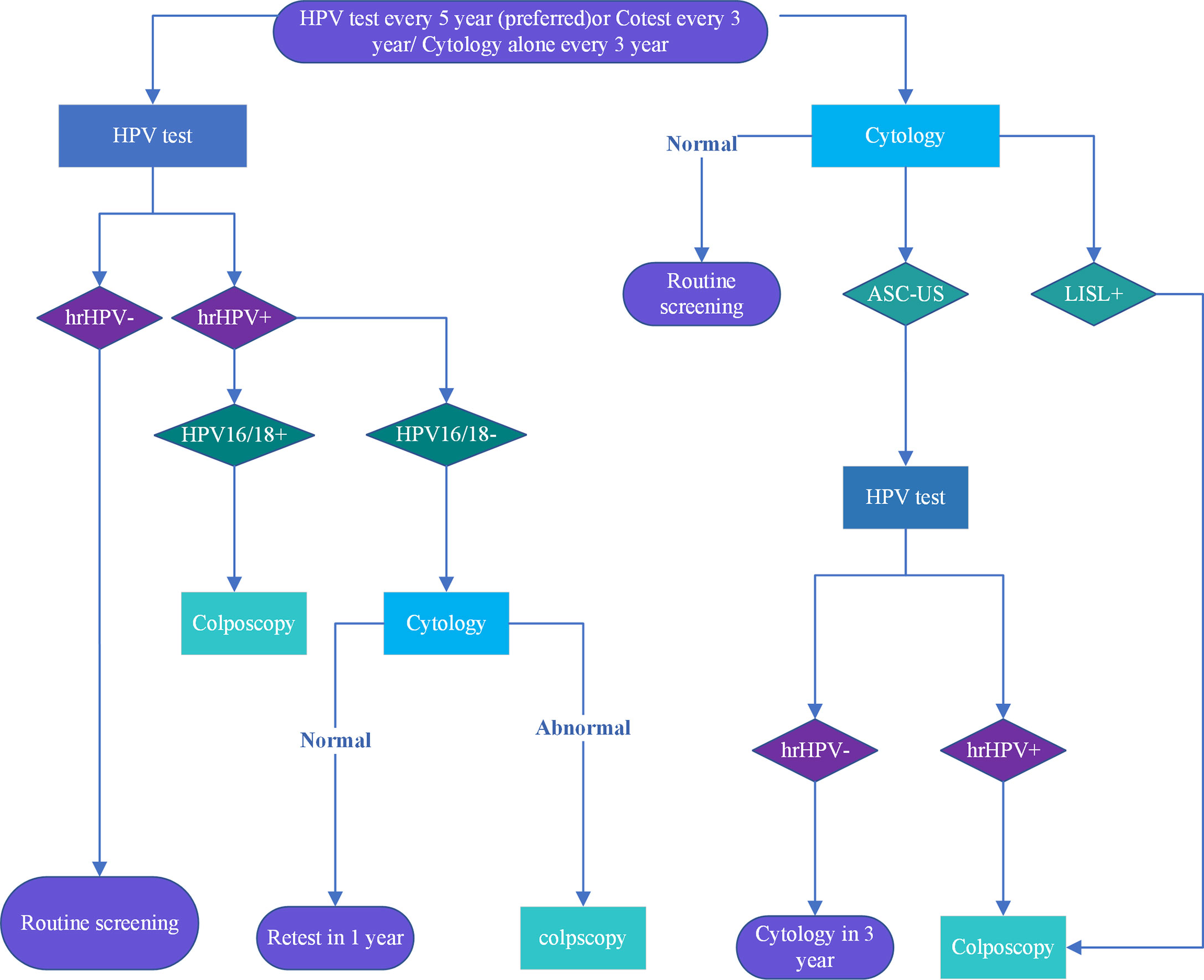
Figure 2 Cervical cancer screening procedures are recommended for women aged 25 to 65. The American Cancer Society recommends screening starting at age 25 Colposcopy is recommended for HPV16/18 +, ASC-US and high risk HPV+ cytology with cytological results above ASC-H. Re-screening is recommended after 1 year for other abnormalities, and after 3 years for normal ones.
Referral colposcopy further determines the presence of CIN and identifies or excludes invasive carcinoma (4). Patients with a high suspicion of invasive cancer should undergo colposcopy-guided biopsy, the gold standard for the diagnosis of cervical cancer and plays a key role in the early detection of cervical cancer (26).
3 Applications of AI in Early Screening of Cervical Cancer
3.1 HPV Typing and Detection
Continuous high-risk HPV infection can lead to cervical cancer (27). HPV testing can detect HPV infection and help screen high-risk populations. Genotyping of HPV will make it easier to assess the risk of women with positive cervical smear results and HPV DNA-positive results (28), thus making it more conducive for cervical cancer screening and management. AI learning technology uses research related to HPV testing to improve accuracy and diversify the use of HPV testing in cervical cancer screening. These studies are summarized in Table 2.
Different types of HPV are associated with different types of lesions. For example, cervical adenocarcinomas are usually associated with HPV 18 type and tend to shed fewer cells; thus, they are difficult to detect by cytology (32). Having high-risk HPV types (e.g., 16, 18, and 31) contributes to a greater risk of developing cervical malignancies (11). Therefore, distinguishing among the specific types of HPV will make it easier to classify and manage HPV-infected women. Based on the additional genotyping information provided by Onclarity, Wong et al. derived a decision system with 94.32% specificity of the best classifier. The system highlighted the patients who are at high risk of developing CIN2/3+, demonstrated that some infections involving multiple HPV types carry additional risks, and identified the most important gene combinations (29). Pathania proposed the HPV AI surveillance, which uses a deep learning (DL) algorithm combined with digital micro-holography, and reported excellent sensitivity and specificity (100% coincidence) in detecting HPV 16 DNA and HPV 18 DNA in cell lines (30).
However, more studies are underway. The high sensitivity of HPV testing results in an increased rate of colposcopy referrals, which may lead to more potentially harmful treatments (33). Tian et al. analyzed HPV integration status, somatic mutation, and copy number variation through capture-based next-generation sequencing and obtained enriched biomarkers of CIN 2. They then used a machine learning algorithm (random forest) to build a risk stratification model for cervical precursor lesions, which successfully predicted CIN2+ with an average accuracy probability score of 0.814 (31). This method effectively stratified the risk of cervical lesions and provided valuable integrated triage strategies.
At present, HPV typing mostly depends on test kits; however, these have some disadvantages, such as false-negative rate and high cost. AI has shown great potential and application in the detection of HPV types and associated molecular markers that can aid in the diagnosis of cervical lesions.
3.2 Screening of Cervical Cytology
Cytology-based cervical cancer prevention programs have reduced the incidence of cervical cancer in many Western countries (34). Cytology screening for high-grade cervical precancerous lesions is highly specific but less sensitive (50–70%) (11) and requires careful microscopy observation by well-trained cytologists. Each process is cumbersome, labor-intensive, and error-prone (35). In addition, cytological reproducibility is low, resulting in low accuracy (36). Furthermore, changing the observer yields inconsistent and subjective results (37). So the researchers hope to develop automatic image analysis methods to relieve these pressures.
The first commercial automatic screening system was PAPNET (38) in 1992. The system was approved as a method of re-screening for slides that were judged negative by cytologists. In 2004, FAD approved the Thinprep imaging system as a commercial screening product. The system can select the 22 most concerned fields of view (FOV) according to the proprietary algorithm, and if abnormal cells are found, cytotechnologists need to manually screen the entire slide (39). The system improves the sensitivity and efficiency of screening. Later, the Focal point GS imaging system emerged in 2008. It identified 10 FOV of cervical cells most likely to be abnormal and stratified the risk to improve the efficiency (40). However, some reviews indicate that the cost-effectiveness of these automation systems is limited and is not suitable for use in low-and medium-developing countries (41). And its research technology still has weaknesses (42) and still depends on the final manual screening process. Therefore, some researchers continue to optimize the application of artificial intelligence technology in cervical cytology.
3.2.1 Segmentation of Cervical Cells
A typical automatic smear analysis system comprises the following five stages: image acquisition, preprocessing, segmentation, feature extraction, and classification (43). AI technology is applied in the segmentation and classification stages for the automatic analysis of a smear, which is helpful to improve screening efficiency.
The first step in cytological diagnosis is the accurate identification of cells and their respective structural components. As the diagnostic criteria for cervical cytology are mainly based on nuclear and cytoplasm abnormalities, accurate segmentation is a prerequisite for screening solutions (44–46). Studies on the application of AI in cell segmentation have been carried out continuously and have shown good results in the segmentation of hepatoma cells (47), human metaphase II oocytes (43), and pluripotent stem cells (48). It has also been introduced for the automatic segmentation of cervical cells, and good results have been reported (49). For example, Chankong et al. used fuzzy c-means clustering technology to segment single-cell images into the nucleus, cytoplasm, and background to realize whole-cell segmentation (41). Some researchers extracted adaptive shape from cytoplasmic contour fragments and shape statistics to segment the overlapped cytoplasm of cells in cervical smear images using supervised learning. Experimental results show that this method is efficient and always superior to the most advanced methods (50). Segmentation model on images from pap smear slide also was explored which has been achieved through using nucleus localization to classify normal and abnormal cells, combined with single cell classification algorithm. The accuracy and sensitivity are 91.7% respectively and the model consists of two stages as shown in Mask-RCNN architecture part in Figure 3 (51). Review of relevant literature results are provided in Table 3.
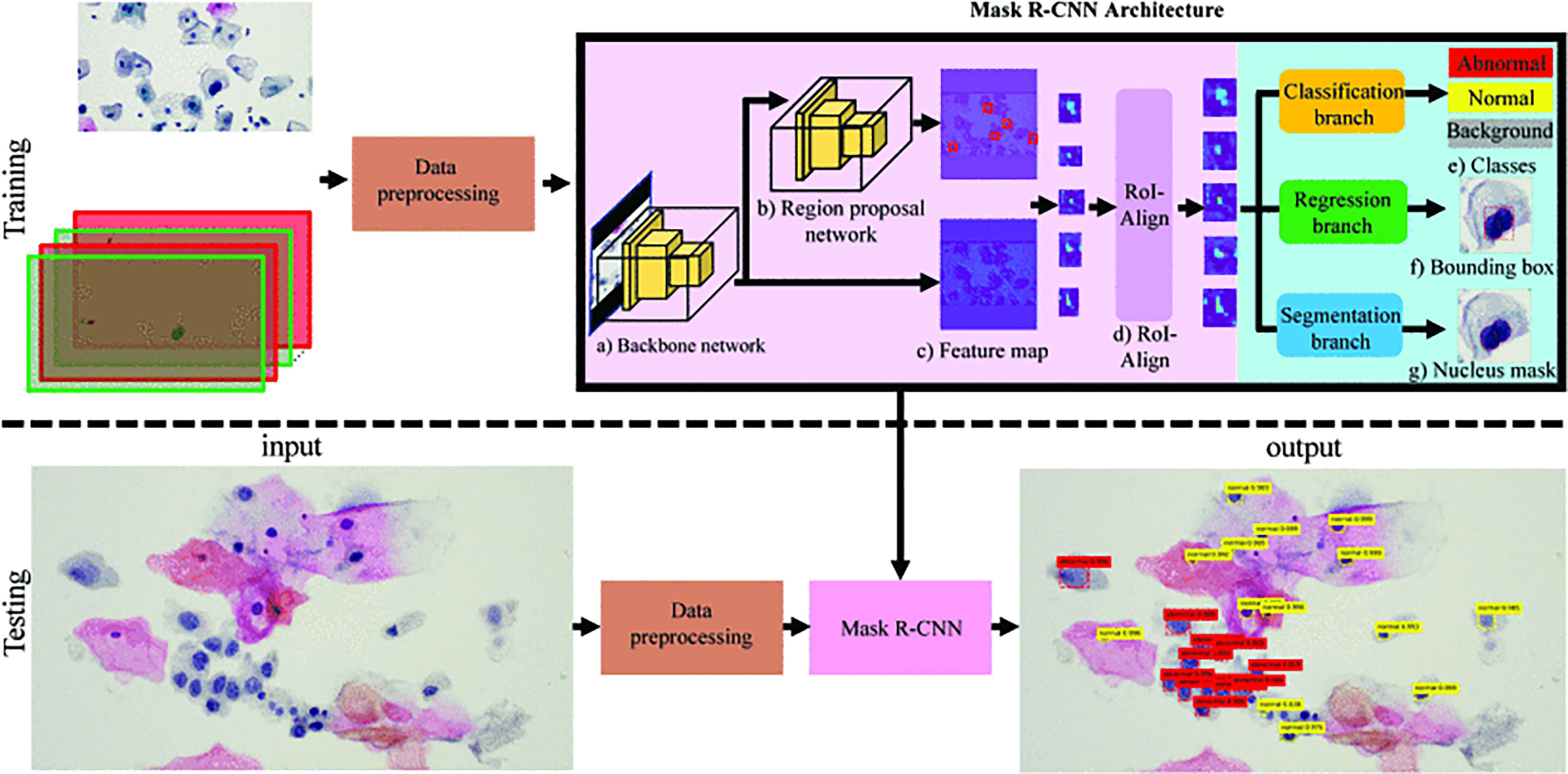
Figure 3 A example segmentation model based on Mask-RCNN architecture. Reproduced with the permission of ref. (51), copyright@IEEE, 2019. In training phase, input was pap smear slide image and nucleus ground truth mask with class label was preprocessed and then trained in Mask R-CNN. In testing phase, pap smear slide image was preprocessed. Mask RCNN was used to specify bounding box, nucleus mask, and class of each cell.
AI makes cervical cell automatic segmentation true, accurate, and unified. Thus, time-intensive manual segmentation process and subjective shortcomings can be overcome to realize accurate abnormal cell classification.
3.2.2 Classification of Cervical Cells
Accurate classification of cervical cells in smears is a crucial step in cervical cancer screening. The low accuracy of manual classification and the high requirement for professional degree of the observer limit the application of cytology (52), particularly in areas where trained cytopathologists are scarce. The use of AI has addressed these limitations (Table 4).
In the past few decades, many classification methods have been proposed, most of which are based on segmentation or texture feature extraction. Chankong et al. segmented the cervical single-cell image into the nucleus, cytoplasm, and background, and obtained the morphological features to realize automatic multi-label classification. The results showed an accuracy rate of more than 93% (44). Mariarputham et al. extracted seven groups of texture features of cervical cells for classification. The support vector machine (SVM) classifier had the highest accuracy and the best performance (57). Three classifiers, i.e., least square support vector machine (LSSVM), multilayer perceptron (MLP) and random forest (RF), were used in the integrated classifier designed by Kden et al. The accuracy of these classifiers was 98.11% at the smear level and 99.01% at the cell level (53). Reducing the time of manual observation eliminates observer bias and improves efficiency.
Classification that does not rely on an accurate segmentation algorithm has also been proposed and accepted by an increasing number of scholars. Zhang et al. applied DL and transfer learning (58) to cervical cell classification for the first time. Automatic extraction of embedded deep-level features in cell images for classification is a superior method compared with previous algorithms in terms of classification accuracy (98.3%), AUC (0.99), and specificity (98.3%) (54). Six different convolutional neural networks (CNNs) were used for the first time for the diagnosis of cervical precancerous lesions. The accuracy, sensitivity, and specificity of the integrated classifier were 0.989, 0.978, and 0.979, respectively (43). Shi et al. proposed a method of cervical cell classification based on a graph convolution network to explore the potential relationship between cervical cell images and improvement of classification performance. Its accuracy (98.37%), sensitivity (99.80%), specificity (99.60%), and F-measure (99.80%) were all better than those of the existing method (55). In addition, hybrid deep feature fusion techniques were proposed, with high accuracy in the SIPAKMeD dataset (56).
3.2.3 AI Improves the Screening accuracy of Cervical Intraepithelial Lesions
After the establishment of a good automated cytological detection model based on the AI method, some studies have found that cytological examination assisted by AI can classify cervical cells to guide triage and improved the detection rate of CIN compared with that of standard pathological biopsy results (32, 59) (Table 5).
A prospective cohort study of 700,000 women in a population-based cervical cancer screening program using a validated AI-assisted cytological diagnostic system was conducted by Bao et al. They reported a total coincidence rate of 94.7% and a 5.8% increase in sensitivity (3.0–8.6%) compared to manual reading (60). Another observational study was conducted to evaluate the ability of AI-assisted cytology to histologically detect CIN or cancer. Detection rates for CIN 2 and CIN 3+ of 92.6% and 96.1%, respectively, were obtained. These were significantly higher than those of manual reading (61). Furthermore, Wang et al. established a DL-based cervical disease diagnosis system that detects high squamous intraepithelial lesions (HSILs) or higher, and an accuracy of 0.93 was achieved (62). Zhu et al. developed an AI-assisted TBS (AIATBS) (21) diagnostic system, which showed higher sensitivity than the diagnosis by senior cytologists. The sensitivity of the AIATBS in detecting CIN was 94.74% in a clinical prospective validation (63).
The evidence above overall indicates that AI has been widely used for HPV testing and cytology and has achieved a good detection rate and accuracy. More studies and applications are underway in this regard. For example, Tang et al. developed an AI microscope with an augmented reality (AR) display for cervical cytology screening. They reported that it significantly improved detection sensitivity for low squamous intraepithelial lesion (LSIL) and HSIL and consistency in multiple classifications and atypical squamous cells of uncertain significance recognition. In addition to diagnostic applications, training novice cytopathologists could be another potential application for AI microscopes (64).
4 Applications of AI in Cervical Cancer Diagnosis
Cervical cancer and precancerous lesions are diagnosed by colposcopy-guided biopsy and staged according to the Federation International of Gynecology and Obstetrics (FIGO) staging standard (65). In 2018, the FIGO allowed the use of imaging and pathologic findings (if any) in staging (66). AI technology has been used in colposcopy and magnetic resonance imaging (MRI) to assist in the diagnosis and staging of cervical cancer and has shown satisfactory results. Figure 4 illustrates the workflow of the CNN model and transfer learning used in colposcopy image classification.
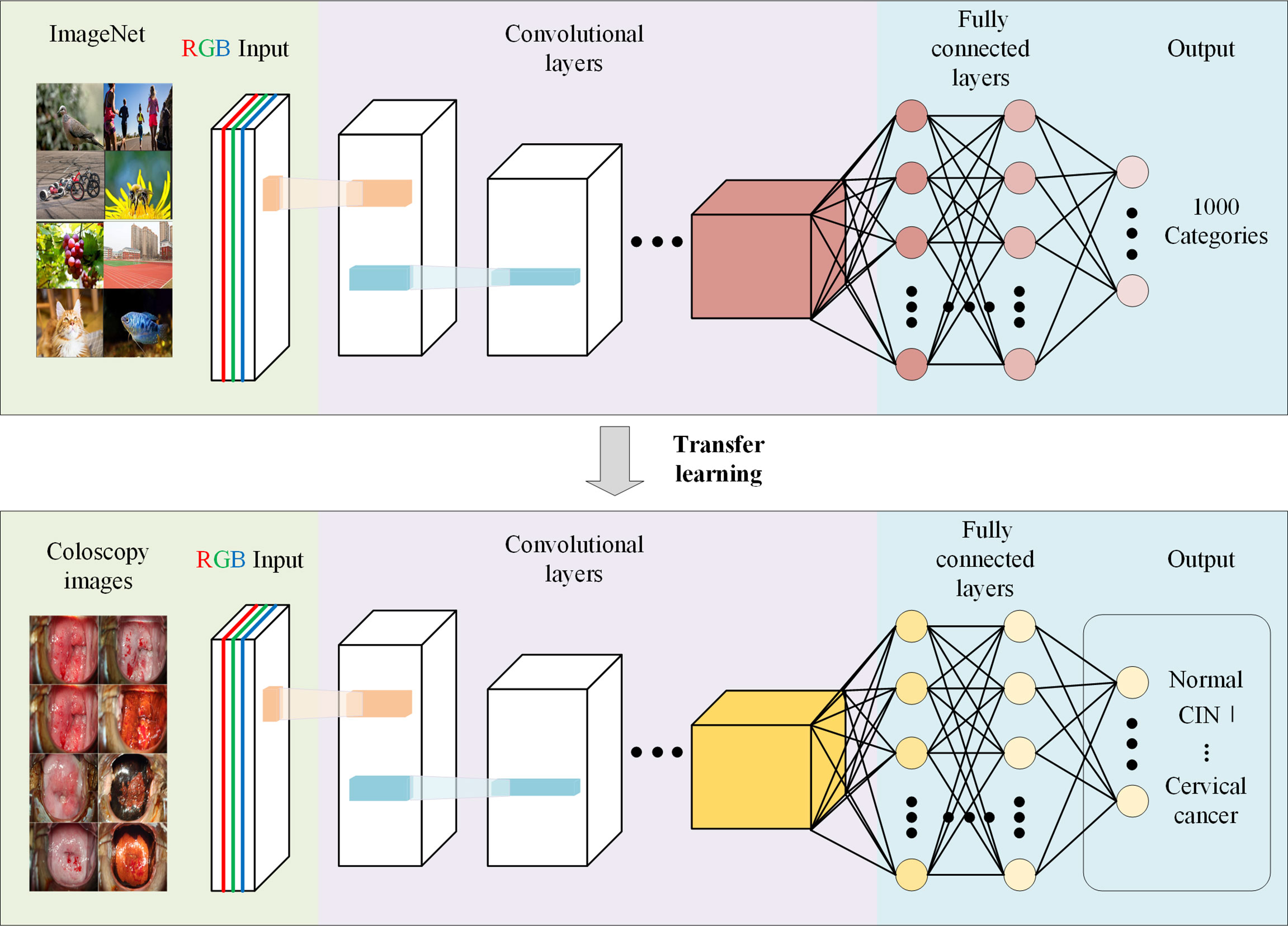
Figure 4 Schematic representation of application of Convolutional Neural Network in colposcopy images. Schematic depicting that a CNN pre-trained on other large-scale image datasets can be adapted to significantly increase the accuracy and shorten the training duration of a network trained on a novel dataset of colposcopy images.
4.1 Coloscopy
At present, the consistency between colposcopy and pathology is poor, which may lead to misdiagnosis and missed diagnosis. Colposcopy performed by an unskilled clinician could result in potential harm (including bleeding, infection, vaginal discharge, pain, or discomfort) and therefore, requires adequate training and experience to achieve proficiency and ensure maintenance of operating procedures. However, the long training period of professional colposcopy doctors and the lack of qualified personnel create challenges for the use of colposcopy in the diagnosis of cervical cancer (67).
4.1.1 AI Boosts Image Classification Performance
Recently, DL has been widely used in medical imaging (15). The application of DL technology in the classification of colposcopy is helpful in solving the bottleneck of traditional colposcopy and improving its diagnostic performance (Table 6).
Miyagi et al. developed and trained a CNN AI classifier for the LISIL/HSIL classification of colposcopy images. The accuracy, sensitivity, and specificity of the AI classifier and oncologist in diagnosing HSIL were 0.823 and 0.797, 0.800 and 0.831, and 0.882 and 0.773, respectively (24). Later, a classifier based on DL was developed, which uses HPV types and cervical SIL images to classify HSIL/LSIL. The accuracy of the classifier was 0.941 (73). Xue et al. developed an AI method (CAIADS) to grade the colposcopy impression and guide the biopsy. The consistency of CAIADS-graded colposcopy impression and pathological results (82.2%) was higher than that traditional colposcopy (65.9%) (74). The ResNet model based on DL was established by Yuan et al. Its sensitivity and specificity based on DL were 85.38% and 82.62%, respectively. The model helps in colposcopy diagnosis and guides biopsy (26). The C-RCNN algorithm was proposed by Yue et al. to classify cervical lesions, and the time and spatial features were extracted using long-term and short-term memory network. Models with better specificity (98.22%), sensitivity (95.09%), accuracy (96.13%), and area under the curve (0.94%) were obtained (75).
4.1.2 AI Helps Detect High-Grade Cervical Lesions and Guides Biopsy
Clinically, one of the most important goals of cervical cancer screening is to distinguish between normal/CIN 1 and CIN 2/3+. If the lesion is classified as CIN 2/3+, treatment is required. In contrast, mild dysplasia in CIN 1 is usually cleared following approximately a year of immune response and can therefore be observed or treated more conservatively. Kim et al. developed a data-driven computer interpretation algorithm for cervical images based on color and texture. They obtained a sensitivity of 74% and a specificity of 90% in differentiating high-grade cervical lesions (CIN 3+) from low-grade lesions and normal tissues (68). Hu et al. conducted a longitudinal cohort study on 9,406 women for 7 years. The cervical images obtained were used to validate the model based on the fast R-CNN method. The AUC of the model for diagnosing CIN 2+ was 0.91, which exceeded the interpretation of the same image by the colposcope evaluator and was superior to those of traditional Pap smears and alternative types of cytology (70). Cho et al. developed a binary decision model to determine the need to biopsy for a cervical lesion. The Need-To-Biopsy was defined as ‘not being normal’, referring to CIN+ and LSIL+. The performance of the best RESNET-152 model showed an average AUC of 0.947, a sensitivity of 85.2%, and a specificity of 88.2% (71); thus, the model helps an inexperienced clinician judge whether to perform a cervical biopsy or refer the patient to a specialist.
The powerful image analysis ability of AI has solved the problem of diagnosing cervical cancer using a large number of colposcopy images. With the assistance of AI technology, the accuracy of detecting lesions and performing biopsy under colposcopy becomes relatively high, thus reducing the misdiagnosis rate of colposcopy (69, 72, 76, 77).
4.2 Pelvic MRI
MRI has proven to be highly accurate in the preoperative staging of cervical cancer (78, 79). Therefore, MRI is the first choice for local staging, evaluation of treatment response, detection of tumor recurrence, and follow-up of patients with cervical cancer (80). The primary objective of MRI is to determine the presence of peritumoral infiltration and lymph node metastasis (LNM) (81) (Table 7).
4.2.1 Segmentation of Cervical Cancer Lesions
MRI has a higher soft tissue resolution than CT. It can determine tumor size and adjacent pelvic structures and assess periuterine invasion and uterine and vaginal involvement (68). Lin et al. developed a U-Net CNN to accurately locate and segment cervical carcinoma in diffuse-weighted imaging (DWI). They reported the highest learning efficiency during image training, with a dice coefficient of 0.8, sensitivity of 0.89, and a positive predictive value of 0.92 (82). Liang et al. established a computational model of a DL algorithm based on wireless network, which can be used to segment cervical cancer MRI images with a 98% accuracy, which is evidently better than that of traditional depth-learning algorithms (<90%) (84). AI is more accurate, objective, and faster than manual segmentation. Wang et al. established a non-invasive radiologic model based on T2-weighted imaging (T2WI) and DWI, divided MRI images, and extracted features to predict periuterine invasion. The AUCs of T2WI and T2WI combined with DWI in the validation cohort were 0.780 and 0.921, respectively (83).
4.2.2 Diagnosis of Cervical Cancer LNM
AI also contributes to the early diagnosis of cervical cancer LNM. Although the accuracy of computed tomography and MRI in assessing lymph node involvement only ranged from 83% to 85%, their specificity was very high, ranging from 66% to 93% (74). In 2018, the cervical cancer staging system was revised to include lymph node status as a staging criterion for the first time. Cervical cancer with lymph node involvement on imaging or pathology was classified as stage IIIC (66). Wu et al. developed a DL model using preoperative MRI to predict LNM in patients with cervical cancer. The AUC using both intratumoral and peritumoral DL models in T1WI was 0.844, whereas that of the hybrid model, which combines the tumor image information from DL mining with the lymph node status reported by MRI, was 0.933 (89), thus improving the detection rate of LNM.
Over the past decade, radiology has evolved to bridge the gap between imaging and precision medicine. Radiology uses complex image analysis tools combined with statistical analysis to extract rich information hidden in medical images (90). Wu et al. used MRI radiomic analysis to improve the diagnostic level of LNM in patients with cervical cancer. The combination of T2WI and decision tree of lymph node status had the best diagnostic effect; in the training and validation cohorts, the AUC and sensitivity were 0.895% and 94.3% and 0.847% and 100%, respectively (89). Wang et al. showed that radiographic images based on T2WI and DWI showed a good predictive power for pelvic LNM in early cervical cancer (83). More values are listed in Table 7 (85–89).
5 Limitations and Future Directions
AI performs well in both computing and image analyses. These characteristics make it a rising star in the field of medical research, helping clinicians in decision-making, reducing the workload of doctors, and reducing the rate of misdiagnosis. Overall, AI can improve the specificity and accuracy of screening and diagnostic programs, overcome time constraints and limited professional and technical personnel, and avoid bias caused by subjective factors, which will enable cervical cancer screening to be implemented in resource-poor areas, thus markedly reducing the incidence of cervical cancer.
However, the application of AI involves some challenges. First is data; machine learning (ML) algorithms sometimes lack data in that they typically require millions of observations to achieve acceptable performance levels (86). However, current clinical data may be scarce, lack markers, and have uncertain quality. The management of medical data is another major obstacle for the development of automated clinical solutions (15). The establishment of not only multiple but also standardized and large databases is a future concern. Data security issues and overfitting should also be considered as they can give rise to exaggerated results that can lead to overdiagnosis (90). Second, models established using AI have not been applied and popularized in clinical practice; therefore, a series of prospective clinical studies are urgently needed to verify these results. Third, AI cannot replace clinicians as it is only an auxiliary diagnostic approach. AI may also cause system paralysis, requiring technical maintenance skills. Furthermore, maintenance systems need to be trained and established.
AI is promising in cervical cancer screening, especially its application in cervical cytology is relatively mature. But the segmentation technology is still faced with many challenges which is important for automatic classification. Such as the segmentation of overlapping nuclei, the processing of non-target cells, and fragments and the quality control of slide dyeing differences are still problem that needs to be optimized. We also mentioned that some classification methods do not rely on segmentation techniques in the previous. It will avoid many pre-processing steps and may be a direction for future development. In addition to the application of early screening and diagnosis mentioned in this paper, AI can be applied to the treatment, prognosis prediction, and prevention of cervical cancer. In the future, more research on treatment and prediction is needed for better treatment decision-making. This will facilitate cervical cancer eradication programs worldwide. Furthermore, as the incidence of cervical adenocarcinoma and other rare pathological types increases, AI should be applied for the early diagnosis of such diseases in the future. AI can also be used for noninvasive differentiation of cervical cancer from other diseases. Further development of AI technologies will greatly enhance the prediction of cervical cancer, maximize the improvements in cervical cancer screening and diagnosis, optimize staging systems, and improve patient prognosis.
Author Contributions
XH and YL contributed to the collection of relevant literature. GS and YL contributed to literature analysis. XH reorganized the literature and wrote the original draft of the manuscript. LZ and TW provided considerable help in the revision of the manuscript. XM was responsible for the design of the review and revision of the manuscript. All authors contributed to the article and approved the submitted version.
Funding
This work was supported by the National Key Research & Development Program of China (grant number 2021YFC2701402).
Conflict of Interest
The authors declare that the research was conducted in the absence of any commercial or financial relationships that could be construed as a potential conflict of interest.
Publisher’s Note
All claims expressed in this article are solely those of the authors and do not necessarily represent those of their affiliated organizations, or those of the publisher, the editors and the reviewers. Any product that may be evaluated in this article, or claim that may be made by its manufacturer, is not guaranteed or endorsed by the publisher.
Acknowledgments
We would like to thank our colleagues for their help in the completion of this manuscript.
References
1. Sung H, Ferlay J, Siegel RL, Laversanne M, Soerjomataram I, Jemal A, et al. Global Cancer Statistics 2020: GLOBOCAN Estimates of Incidence and Mortality Worldwide for 36 Cancers in 185 Countries. CA: A Cancer J Clin (2021) 71:209–49. doi: 10.3322/caac.21660
2. Brisson M, Kim JJ, Canfell K, Drolet M, Gingras G, Burger EA, et al. Impact of HPV Vaccination and Cervical Screening on Cervical Cancer Elimination: A Comparative Modelling Analysis in 78 Low-Income and Lower-Middle-Income Countries. Lancet (2020) 395(10224):575–90. doi: 10.1016/S0140-6736(20)30068-4
3. Schiffman M, Castle PE, Jeronimo J, Rodriguez AC, Wacholder S. Human Papillomavirus and Cervical Cancer. Lancet (2007) 370(9590):890–907. doi: 10.1016/S0140-6736(07)61416-0
4. Simms KT, Steinberg J, Caruana M, Smith MA, Lew JB, Soerjomataram I, et al. Impact of Scaled Up Human Papillomavirus Vaccination and Cervical Screening and the Potential for Global Elimination of Cervical Cancer in 181 Countries, 2020-99: A Modelling Study. Lancet Oncol (2019) 20(3):394–407. doi: 10.1016/S1470-2045(18)30836-2
5. Fontham ETH, Wolf AMD, Church TR, Etzioni R, Flowers CR, Herzig A, et al. Cervical Cancer Screening for Individuals at Average Risk: 2020 Guideline Update From the American Cancer Society. CA Cancer J Clin (2020) 70(5):321–46. doi: 10.3322/caac.21628
6. Redman CWE, Kesic V, Cruickshank ME, Gultekin M, Carcopino X, Castro Sanchez M, et al. European Federation for Colposcopy and Pathology of the Lower Genital Tract (EFC) and the European Society of Gynecologic Oncology (ESGO). Eur Consensus Statement Essential Colposcopy Eur J Obstet Gynecol Reprod Biol (2021) 256:57–62. doi: 10.1016/jejogrb.2020.06.029
7. WHO. World Health Organization Human Papillomavirus (HPV) and Cervical Cancer, Fact Sheet. Available at: https://www.who.int/news-room/fact-sheets/detail/human-papillomavirus-(hpv)-and-cervical-cancer (Accessed 17 September 2019).
8. Bray F, Ferlay J, Soerjomataram I, Siegel RL, Torre LA, Jemal A. Global Cancer Statistics 2018: GLOBOCAN Estimates of Incidence and Mortality Worldwide for 36 Cancers in 185 Countries. CA Cancer J Clin (2018) 68(6):394–424. doi: 10.3322/caac.21492
9. Pollack AE, Tsu VD. Preventing Cervical Cancer in Low-Resource Settings: Building a Case for the Possible. Int J Gynaecol Obstet (2005) 89 Suppl 2:S1–3. doi: 10.1016/j.ijgo.2005.01.014
10. World Health Organization. Cervical Cancer Screening in Developing Countries: Report of a WHO Consultation. Geneva: World Health Organization (2002).
11. Nanda K, McCrory DC, Myers ER, Bastian LA, Hasselblad V, Matchar DB, et al. Accuracy of the Papanicolaou Test in Screening for and Follow-Up of Cervical Cytologic Abnormalities: A Systematic Review. Ann Intern Med (2000) 132(10):810–9. doi: 10.7326/0003-4819-132-10-200005160-00009
12. Esteva A, Kuprel B, Novoa RA, Ko J, Swetter SM, Blau HM, et al. Dermatologist-Level Classification of Skin Cancer With Deep Neural Networks. Nature (2017) 542(7639):115–8. doi: 10.1038/nature21056
13. Maron RC, Weichenthal M, Utikal JS, Hekler A, Berking C, Hauschild A, et al. Systematic Outperformance of 112 Dermatologists in Multiclass Skin Cancer Image Classification by Convolutional Neural Networks. Eur J Cancer (2019) 119:57–65. doi: 10.1016/j.ejca.2019.06.013
14. Schmidt-Erfurth U, Sadeghipour A, Gerendas BS, Waldstein SM, Bogunović H. Artificial Intelligence in Retina. Prog Retin Eye Res (2018) 67:1–29. doi: 10.1016/j.preteyeres.2018.07.004
15. Bi WL, Hosny A, Schabath MB, Giger ML, Birkbak NJ, Mehrtash A, et al. Artificial Intelligence in Cancer Imaging: Clinical Challenges and Applications. CA Cancer J Clin (2019) 69(2):127–57. doi: 10.3322/caac.21552
16. Marth C, Landoni F, Mahner S, McCormack M, Gonzalez-Martin A, Colombo N. Cervical Cancer: ESMO Clinical Practice Guidelines for Diagnosis, Treatment and Follow-Up. Ann Oncol (2017) 28(suppl_4):iv72–83. doi: 10.1093/annonc/mdx220
18. Cox S. Guidelines for Papanicolaou Test Screening and Follow-Up. J Midwifery Wom Heal (2012) 57:86–9. doi: 10.1111/j.1542-2011.2011.00116.x
19. Phaliwong P, Pariyawateekul P, Khuakoonratt N, Sirichai W, Bhamarapravatana K, Suwannarurk K. Cervical Cancer Detection Between Conventional and Liquid Based Cervical Cytology: A 6-Year Experience in Northern Bangkok Thailand. Asian Pac J Cancer Prev (2018) 19(5):1331–6. doi: 10.22034/APJCP.2018.19.5.1331
20. Hoda RS, Loukeris K, Abdul-Karim FW. Gynecologic Cytology on Conventional and Liquid-Based Preparations: A Comprehensive Review of Similarities and Differences. Diagn Cytopathol (2013) 41(3):257–78. doi: 10.1002/dc.22842
21. Nayar R, Wilbur DC. The Pap Test and Bethesda 2014. Cancer Cytopathol (2015) 123(5):271–81. doi: 10.1002/cncy.21521
22. Hussain E, Mahanta LB, Das CR, Choudhury M, Chowdhury M. A Shape Context Fully Convolutional Neural Network for Segmentation and Classification of Cervical Nuclei in Pap Smear Images. Artif Intell Med (2020) 107:101897. doi: 10.1016/j.artmed.2020.101897
23. Khan MJ, Werner CL, Darragh TM, Guido RS, Mathews C, Moscicki AB, et al. ASCCP Colposcopy Standards: Role of Colposcopy, Benefits, Potential Harms, and Terminology for Colposcopic Practice. J Low Genit Tract Dis (2017) 21(4):223–9. doi: 10.1097/LGT.0000000000000338
24. Miyagi Y, Takehara K, Miyake T. Application of Deep Learning to the Classification of Uterine Cervical Squamous Epithelial Lesion From Colposcopy Images. Mol Clin Oncol (2019) 11(6):583–9. doi: 10.3892/mco.2019.1932
25. Sawaya GF, Smith-McCune K, Kuppermann M. Cervical Cancer Screening: More Choices in 2019. JAMA (2019) 321(20):2018–9. doi: 10.1001/jama.2019.4595
26. Yuan C, Yao Y, Cheng B, Cheng Y, Li Y, Li Y, et al. The Application of Deep Learning Based Diagnostic System to Cervical Squamous Intraepithelial Lesions Recognition in Colposcopy Images. Sci Rep (2020) 10(1):11639. doi: 10.1038/s41598-020-68252-3
27. Walboomers JM, Jacobs MV, Manos MM, Bosch FX, Kummer JA, Shah KV, et al. Human Papillomavirus Is a Necessary Cause of Invasive Cervical Cancer Worldwide. J Pathol (1999) 189(1):12–9. doi: 10.1002/(SICI)1096-9896(199909)189:1<12:AID-PATH431>3.0.CO;2-F
28. Guo M, Gong Y, Wang J, Dawlett M, Patel S, Liu P, et al. The Role of Human Papillomavirus Type 16/18 Genotyping in Predicting High-Grade Cervical/Vaginal Intraepithelial Neoplasm in Women With Mildly Abnormal Papanicolaou Results. Cancer Cytopathol (2013) 121(2):79–85. doi: 10.1002/cncy.21240
29. Wong OGW, Ng IFY, Tsun OKL, Pang HH, Ip PPC, Cheung ANY. Machine Learning Interpretation of Extended Human Papillomavirus Genotyping by Onclarity in an Asian Cervical Cancer Screening Population. J Clin Microbiol (2019) 57(12):e00997–19. doi: 10.1128/JCM.00997-19
30. Pathania D, Landeros C, Rohrer L, D'Agostino V, Hong S, Degani I, et al. Point-of-Care Cervical Cancer Screening Using Deep Learning-Based Microholography. Theranostics (2019) 9(26):8438–47. doi: 10.7150/thno.37187
31. Tian R, Cui Z, He D, Tian X, Gao Q, Ma X, et al. Risk Stratification of Cervical Lesions Using Capture Sequencing and Machine Learning Method Based on HPV and Human Integrated Genomic Profiles. Carcinogenesis (2019) 40(10):1220–8. doi: 10.1093/carcin/bgz094
32. Yu K, Hyun N, Fetterman B, Lorey T, Raine-Bennett TR, Zhang H, et al. Automated Cervical Screening and Triage, Based on HPV Testing and Computer-Interpreted Cytology. J Natl Cancer Inst (2018) 110(11):1222–8. doi: 10.1093/jnci/djy044
33. Melnikow J, Henderson JT, Burda BU, Senger CA, Durbin S, Weyrich MS. Screening for Cervical Cancer With High-Risk Human Papillomavirus Testing: Updated Evidence Report and Systematic Review for the US Preventive Services Task Force. JAMA (2018) 320(7):687–705. doi: 10.1001/jama.2018.10400
34. Dijkstra MG, Snijders PJ, Arbyn M, Rijkaart DC, Berkhof J, Meijer CJ. Cervical Cancer Screening: On the Way to a Shift From Cytology to Full Molecular Screening. Ann Oncol (2014) 25(5):927–35. doi: 10.1093/annonc/mdt538
35. Perkins RB, Langrish SM, Stern LJ, Figueroa J, Simon CJ. Comparison of Visual Inspection and Papanicolau (PAP) Smears for Cervical Cancer Screening in Honduras: Should PAP Smears be Abandoned? Trop Med Int Heal (2007) 12(9):1018–25. doi: 10.1111/j.1365-3156.2007.01888.x
36. Stoler MH, Schiffman M. Interobserver Reproducibility of Cervical Cytologic and Histologic Interpretations: Realistic Estimates From the ASCUS-LSIL Triage Study. JAMA (2001) 285(11):1500–5. doi: 10.1001/jama.285.11.1500
37. William W, Ware A, Basaza-Ejiri AH, Obungoloch J. A Review of Image Analysis and Machine Learning Techniques for Automated Cervical Cancer Screening From Pap-Smear Images. Comput Methods Programs Biomed (2018) 164:15–22. doi: 10.1016/j.cmpb.2018.05.034
38. Bengtsson E, Malm P. Screening for Cervical Cancer Using Automated Analysis of PAP-Smears. Comput Math Methods Med (2014) 2014:842037. doi: 10.1155/2014/842037
39. Chivukula M, Saad RS, Elishaev E, White S, Mauser N, Dabbs DJ. Introduction of the Thin Prep Imaging System (TIS): Experience in a High Volume Academic Practice. Cytojournal (2007) 4:6. doi: 10.1186/1742-6413-4-6
40. Thrall MJ. Automated Screening of Papanicolaou Tests: A Review of the Literature. Diagn Cytopathol (2019) 47(1):20–7. doi: 10.1002/dc.23931
41. Chankong T, Theera-Umpon N, Auephanwiriyakul S. Automatic Cervical Cell Segmentation and Classification in Pap Smears. Comput Methods Programs Biomed (2014) 113(2):539–56. doi: 10.1016/j.cmpb.2013.12.012
42. Landau MS, Pantanowitz L. Artificial Intelligence in Cytopathology: A Review of the Literature and Overview of Commercial Landscape. J Am Soc Cytopathol (2019) 8(4):230–41. doi: 10.1016/j.jasc.2019.03.003
43. Firuzinia S, Afzali SM, Ghasemian F, Mirroshandel SA. A Robust Deep Learning-Based Multiclass Segmentation Method for Analyzing Human Metaphase II Oocyte Images. Comput Methods Programs Biomed (2021) 201:105946. doi: 10.1016/j.cmpb.2021.105946
44. Wang P, Wang L, Li Y, Song Q, Lv S, Hu X. Automatic Cell Nuclei Segmentation and Classification of Cervical Pap Smear Images. Biomed Signal Process Control (2019) 48:93–103. doi: 10.1016/j.bspc.2018.09.008
45. Zhao L, Li K, Wang M, Yin J, Zhu En, Wu C, et al. Automatic Cytoplasm and Nuclei Segmentation for Color Cervical Smear Image Using an Efficient Gap-Search MRF. Comput Biol Med (2016) 71:46–56. doi: 10.1016/j.compbiomed.2016.01.025. ISSN 0010-4825.
46. Gautam S, Bhavsar A, Sao AK, Harinarayan KK. CNN Based Segmentation of Nuclei in PAP-Smear Images With Selective Pre-Processing. Digital Pathol (2018) 10581:105810X. doi: 10.1117/12.2293526
47. Lal S, Das D, Alabhya K, Kanfade A, Kumar A, Kini J. NucleiSegNet: Robust Deep Learning Architecture for the Nuclei Segmentation of Liver Cancer Histopathology Images. Comput Biol Med (2021) 128:104075. doi: 10.1016/j.compbiomed.2020.104075
48. Piotrowski T, Rippel O, Elanzew A, Nießing B, Stucken S, Jung S, et al. Deep-Learning-Based Multi-Class Segmentation for Automated, Non-Invasive Routine Assessment of Human Pluripotent Stem Cell Culture Status. Comput Biol Med (2021) 129:104172. doi: 10.1016/j.compbiomed.2020.104172
49. Conceição T, Braga C, Rosado L, Vasconcelos MJM. A Review of Computational Methods for Cervical Cells Segmentation and Abnormality Classification. Int J Mol Sci (2019) 20(20):5114. doi: 10.3390/ijms20205114
50. Song Y, Zhu L, Qin J, Lei B, Sheng B, Choi KS. Segmentation of Overlapping Cytoplasm in Cervical Smear Images via Adaptive Shape Priors Extracted From Contour Fragments. IEEE Trans Med Imaging (2019) 38(12):2849–62. doi: 10.1109/TMI.2019.2915633
51. Sompawong N, Mopan J, Pooprasert P, Himakhun W, Suwannarurk K, Ngamvirojcharoen J, et al. Automated Pap Smear Cervical Cancer Screening Using Deep Learning. Annu Int Conf IEEE Eng Med Biol Soc (2019) 2019:7044–8. doi: 10.1109/EMBC.2019.8856369
52. Hussain E, Mahanta LB, Das CR, Talukdar RK. A Comprehensive Study on the Multi-Class Cervical Cancer Diagnostic Prediction on Pap Smear Images Using a Fusion-Based Decision From Ensemble Deep Convolutional Neural Network. Tissue Cell (2020) 65:101347. doi: 10.1016/j.tice.2020.101347
53. Bora K, Chowdhury M, Mahanta LB, Kundu MK, Das AK. Automated Classification of Pap Smear Images to Detect Cervical Dysplasia. Comput Methods Programs Biomed (2017) 138:31–47. doi: 10.1016/j.cmpb.2016.10.001
54. Zhang L, Lu Le, Nogues I, Summers RM, Liu S, Yao J. DeepPap: Deep Convolutional Networks for Cervical Cell Classification. IEEE J BioMed Health Inform (2017) 21(6):1633–43. doi: 10.1109/JBHI.2017.2705583
55. Shi J, Wang R, Zheng Y, Jiang Z, Zhang H, Yu L. Cervical Cell Classification With Graph Convolutional Network. Comput Methods Programs Biomed (2021) 198:105807. doi: 10.1016/j.cmpb.2020.105807
56. Rahaman MM, Li C, Yao Y, Kulwa F, Wu X, Li X, et al. DeepCervix: A Deep Learning-Based Framework for the Classification of Cervical Cells Using Hybrid Deep Feature Fusion Techniques. Comput Biol Med (2021) 136:104649. doi: 10.1016/j.compbiomed.2021.104649
57. Mariarputham EJ, Stephen A. Nominated Texture Based Cervical Cancer Classification. Comput Math Methods Med (2015) 2015:1–10. doi: 10.1155/2015/586928
58. Yosinski J, Clune J, Bengio Y, Lipson H. How Transferable Are Features in Deep Neural Networks? Adv Neural Inf Process Syst (2014) 27:3320–8.
59. Holmström O, Linder N, Kaingu H, Mbuuko N, Mbete J, Kinyua F, et al. Point-Of-Care Digital Cytology With Artificial Intelligence for Cervical Cancer Screening in a Resource-Limited Setting. JAMA Netw Open (2021) 4(3):e211740. doi: 10.1001/jamanetworkopen.2021.1740
60. Bao H, Sun X, Zhang Y, Pang B, Li H, Zhou L, et al. The Artificial Intelligence-Assisted Cytology Diagnostic System in Large-Scale Cervical Cancer Screening: A Population-Based Cohort Study of 0.7 Million Women. Cancer Med (2020) 9(18):6896–906. doi: 10.1002/cam4.3296
61. Bao H, Bi H, Zhang X, Zhao Y, Dong Y, Luo X, et al. Artificial Intelligence-Assisted Cytology for Detection of Cervical Intraepithelial Neoplasia or Invasive Cancer: A Multicenter, Clinical-Based, Observational Study. Gynecol Oncol (2020) 159(1):171–8. doi: 10.1016/j.ygyno.2020.07.099
62. Wang CW, Liou YA, Lin YJ, Chang CC, Chu PH, Lee YC, et al. Artificial Intelligence-Assisted Fast Screening Cervical High Grade Squamous Intraepithelial Lesion and Squamous Cell Carcinoma Diagnosis and Treatment Planning. Sci Rep (2021) 11(1):16244. doi: 10.1038/s41598-021-95545-y
63. Zhu X, Li X, Ong K, Zhang W, Li W, Li L, et al. Hybrid AI-Assistive Diagnostic Model Permits Rapid TBS Classification of Cervical Liquid-Based Thin-Layer Cell Smears. Nat Commun (2021) 12(1):3541. doi: 10.1038/s41467-021-23913-3
64. Tang HP, Cai D, Kong YQ, Ye H, Ma ZX, Lv HS, et al. Cervical Cytology Screening Facilitated by an Artificial Intelligence Microscope: A Preliminary Study. Cancer Cytopathol (2021). doi: 10.1002/cncy.22425
65. Janicek MF, Averette HE. Cervical Cancer: Prevention, Diagnosis, and Therapeutics. CA Cancer J Clin (2001) 51(2):92–114; quiz 115-8. doi: 10.3322/canjclin.51.2.92
66. Bhatla N, Berek JS, Cuello Fredes M, Denny LA, Grenman S, Karunaratne K, et al. Revised FIGO Staging For Carcinoma of The Cervix Uteri. Int J Gynaecol Obstet. (2019) 145(1):129-35. doi: 10.1002/ijgo.12749
67. Xue P, Ng MTA, Qiao Y. The Challenges of Colposcopy for Cervical Cancer Screening in LMICs and Solutions by Artificial Intelligence. BMC Med (2020) 18(1):169. doi: 10.1186/s12916-020-01613-x
68. Kim E, Huang X. A Data Driven Approach to Cervigram Image Analysis and Classification. In: Celebi ME, Schaefer G, editors. Color Medical Image Analysis. Lecture Notes in Computational Vision and Biomechanics 6. Dordrecht: Springer Netherlands (2013) 1-13. doi: 10.1007/978-94-007-5389-1_1
69. Song D, Kim E, Huang X, Patruno J, Munoz-Avila H, Heflin J, et al. Multimodal Entity Coreference for Cervical Dysplasia Diagnosis. IEEE Trans Med Imaging (2015) 34(1):229–45. doi: 10.1109/TMI.2014.2352311
70. Hu L, Bell D, Antani S, Xue Z, Yu K, Horning MP, et al. An Observational Study of Deep Learning and Automated Evaluation of Cervical Images for Cancer Screening. J Natl Cancer Inst (2019) 111(9):923–32. doi: 10.1093/jnci/djy225
71. Cho BJ, Choi YJ, Lee MJ, Kim JH, Son GH, Park SH, et al. Classification of Cervical Neoplasms on Colposcopic Photography Using Deep Learning. Sci Rep (2020) 10(1):13652. doi: 10.1038/s41598-020-70490-4
72. Asiedu MN, Simhal A, Chaudhary U, Mueller JL, Lam CT, Schmitt JW, et al. Development of Algorithms for Automated Detection of Cervical Pre-Cancers With a Low-Cost, Point-of-Care, Pocket Colposcope. IEEE Trans BioMed Eng (2019) 66(8):2306–18. doi: 10.1109/TBME.2018.2887208
73. Miyagi Y, Takehara K, Nagayasu Y, Miyake T. Application of Deep Learning to the Classification of Uterine Cervical Squamous Epithelial Lesion From Colposcopy Images Combined With HPV Types. Oncol Lett (2020) 19(2):1602–10. doi: 10.3892/ol.2019.11214
74. Xue P, Tang C, Li Q, Li Y, Shen Y, Zhao Y, et al. Development and Validation of an Artificial Intelligence System for Grading Colposcopic Impressions and Guiding Biopsies. BMC Med (2020) 18(1):406. doi: 10.1186/s12916-020-01860-y
75. Yue Z, Ding S, Zhao W, Wang H, Ma J, Zhang Y, et al. Automatic CIN Grades Prediction of Sequential Cervigram Image Using LSTM With Multistate CNN Features. IEEE J BioMed Health Inform (2020) 24(3):844–54. doi: 10.1109/JBHI.2019.2922682
76. Chandran V, Sumithra MG, Karthick A, George T, Deivakani M, Elakkiya B, et al. Diagnosis of Cervical Cancer Based on Ensemble Deep Learning Network Using Colposcopy Images. BioMed Res Int (2021) 2021:5584004. doi: 10.1155/2021/5584004
77. Peng G, Dong H, Liang T, Li L, Liu J. Diagnosis of Cervical Precancerous Lesions Based on Multimodal Feature Changes. Comput Biol Med (2021) 130:104209. doi: 10.1016/j.compbiomed.2021.104209
78. Choi SH, Kim SH, Choi HJ, Park BK, Lee HJ. Preoperative Magnetic Resonance Imaging Staging of Uterine Cervical Carcinoma: Results of Prospective Study. J Comput Assist Tomogr (2004) 28(5):620–7. doi: 10.1097/01.rct.0000138007.77725.0a
79. Hricak H, Gatsonis C, Chi DS, Amendola MA, Brandt K, Schwartz LH, et al. American College of Radiology Imaging Network 6651; Gynecologic Oncology Group 183. Role of Imaging in Pretreatment Evaluation of Early Invasive Cervical Cancer: Results of the Intergroup Study American College of Radiology Imaging Network 6651-Gynecologic Oncology Group 183. J Clin Oncol (2005) 23(36):9329–37. doi: 10.1200/JCO.2005.02.0354
80. Merz J, Bossart M, Bamberg F, Eisenblaetter M. Revised FIGO Staging for Cervical Cancer - A New Role for MRI. Rofo (2020) 192(10):937–44. doi: 10.1055/a-1198-5729
81. Cohen PA, Jhingran A, Oaknin A, Denny L. Cervical Cancer. Lancet (2019) 393(10167):169–82. doi: 10.1016/S0140-6736(18)32470-X
82. Lin YC, Lin CH, Lu HY, Chiang HJ, Wang HK, Huang YT, et al. Deep Learning for Fully Automated Tumor Segmentation and Extraction of Magnetic Resonance Radiomics Features in Cervical Cancer. Eur Radiol (2020) 30(3):1297–305. doi: 10.1007/s00330-019-06467-3
83. Wang T, Gao T, Guo H, Wang Y, Zhou X, Tian J, et al. Preoperative Prediction of Parametrial Invasion in Early-Stage Cervical Cancer With MRI-Based Radiomics Nomogram. Eur Radiol (2020) 30(6):3585–93. doi: 10.1007/s00330-019-06655-1
84. Liang P, Sun G, Wei S. Application of Deep Learning Algorithm in Cervical Cancer MRI Image Segmentation Based on Wireless Sensor. J Med Syst (2019) 43(6):156. doi: 10.1007/s10916-019-1284-7
85. Yu YY, Zhang R, Dong RT, Hu QY, Yu T, Liu F, et al. Feasibility of an ADC-Based Radiomics Model for Predicting Pelvic Lymph Node Metastases in Patients With Stage IB-IIA Cervical Squamous Cell Carcinoma. Br J Radiol (2019) 92(1097):20180986. doi: 10.1259/bjr.20180986
86. Obermeyer Z, Emanuel EJ. Predicting the Future - Big Data, Machine Learning, and Clinical Medicine. N Engl J Med (2016) 375(13):1216–9. doi: 10.1056/NEJMp1606181
87. Wang T, Gao T, Yang J, Yan X, Wang Y, Zhou X, et al. Preoperative Prediction of Pelvic Lymph Nodes Metastasis in Early-Stage Cervical Cancer Using Radiomics Nomogram Developed Based on T2-Weighted MRI and Diffusion-Weighted Imaging. Eur J Radiol (2019) 114:128–35. doi: 10.1016/j.ejrad.2019.01.003
88. Xiao M, Ma F, Li Y, Li Y, Li M, Zhang G, et al. Multiparametric MRI-Based Radiomics Nomogram for Predicting Lymph Node Metastasis in Early-Stage Cervical Cancer. J Magn Reson Imaging (2020) 52(3):885–96. doi: 10.1002/jmri.27101
89. Wu Q, Wang S, Zhang S, Wang M, Ding Y, Fang J, et al. Development of a Deep Learning Model to Identify Lymph Node Metastasis on Magnetic Resonance Imaging in Patients With Cervical Cancer. JAMA Netw Open (2020) 3(7):e2011625. doi: 10.1001/jamanetworkopen.2020.11625
Keywords: cervical cancer, cervical intraepithelial neoplasia (CIN), artificial intelligence, deep learning, cytology, colposcopy, early screening and diagnosis
Citation: Hou X, Shen G, Zhou L, Li Y, Wang T and Ma X (2022) Artificial Intelligence in Cervical Cancer Screening and Diagnosis. Front. Oncol. 12:851367. doi: 10.3389/fonc.2022.851367
Received: 09 January 2022; Accepted: 10 February 2022;
Published: 11 March 2022.
Edited by:
Jian-Jun Wei, Northwestern University, United StatesReviewed by:
Lee Cooper, Northwestern University, United StatesJorge E. Novo, Northwestern Medicine, United States
Copyright © 2022 Hou, Shen, Zhou, Li, Wang and Ma. This is an open-access article distributed under the terms of the Creative Commons Attribution License (CC BY). The use, distribution or reproduction in other forums is permitted, provided the original author(s) and the copyright owner(s) are credited and that the original publication in this journal is cited, in accordance with accepted academic practice. No use, distribution or reproduction is permitted which does not comply with these terms.
*Correspondence: Xiangyi Ma, eHltYUB0amgudGptdS5lZHUuY24=