- 1Henan Provincial People’s Hospital, Institute of Hematology of Henan Provincial People’s Hospital, Zhengzhou, China
- 2Henan Provincial People’s Hospital, Henan Key laboratory of Stem Cell Differentiation and Modification, Zhengzhou, China
- 3Department of Pediatrics, The First Affiliated Hospital of Zhengzhou University, Zhengzhou, China
- 4Department of Hematology, People’s Hospital of Zhengzhou University, Zhengzhou, China
Non-Hodgkin’s lymphoma (NHL) is the third most common malignant tumor among children. However, at initial NHL diagnosis, most cases are at an advanced stage because of nonspecific clinical manifestations and currently limited diagnostic methods. This study aimed to screen and verify potential serum biomarkers of pediatric NHL using isobaric tags for relative and absolute quantification (iTRAQ)-based proteomic analysis. Serum protein expression profiles from children with B-NHL (n=20) and T-NHL (n=20) and healthy controls (n=20) were detected by utilizing iTRAQ in combination with two-dimensional liquid chromatography-tandem mass spectrometry (2D LC–MS/MS) and analyzed by applying Ingenuity Pathway Analysis (IPA). The candidate biomarkers S100A8 and LRG1 were further validated by using enzyme-linked immunosorbent assays (ELISAs). Receiver operating characteristic (ROC) analysis based on ELISA data was used to evaluate diagnostic efficacy. In total, 534 proteins were identified twice using iTRAQ combined with 2D LC–MS/MS. Further analysis identified 79 and 73 differentially expressed proteins in B-NHL and T-NHL serum, respectively, compared with control serum according to our defined criteria; 34 proteins were overexpressed and 45 proteins underexpressed in B-NHL, whereas 45 proteins were overexpressed and 28 proteins underexpressed in T-NHL (p < 0.05). IPA demonstrated a variety of signaling pathways, including acute phase response signaling and liver X receptor/retinoid X receptor (LXR/RXR) activation, to be strongly associated with pediatric NHL. S100A8 and LRG1 were elevated in NHL patients compared to normal controls according to ELISA (p < 0.05), which was consistent with iTRAQ results. The areas under the ROC curves of S100A8, LRG1, and the combination of S100A8 and LRG1 were 0.873, 0.898 and 0.970, respectively. Our findings indicate that analysis of the serum proteome using iTRAQ combined with 2D LC–MS/MS is a feasible approach for biomarker discovery. Serum S100A8 and LRG1 are promising candidate biomarkers for pediatric NHL, and these differential proteins illustrate a novel pathogenesis and may be clinically helpful for NHL diagnosis in the future.
Introduction
Non-Hodgkin’s lymphoma (NHL) is the most common hematologic malignancy in the world, with numerous biologically and clinically heterogeneous subtypes (1). NHL is characterized by great differences in clinical manifestations, biological characteristics and survival outcomes among different ages groups (2). For example, pediatric NHL exhibits significant differences in the distribution of histologic subtypes compared to NHL in adults. In the pediatric age range, NHL is the third most common malignancy, accounting for approximately 6% of new cancers, and is primarily represented by only a few histologic subtypes (1, 2). Children often develop highly aggressive lymphoma. The principal subtypes by incidence in children are the mature B-cell lymphomas Burkitt lymphoma/leukemia (BL), lymphoblastic lymphomas (LBL) of the precursor B- or T-cell type and diffuse large B-cell lymphomas (3). Compared to Hodgkin’s lymphomas (HLs), NHLs are more common in childhood and have considerable phenotypic and biological heterogeneity. Pediatric NHL usually presents as aggressive disseminated disease or as a rapidly growing mass with multicenter origin, long-distance transmission, and extranodal infiltration, as well as great differences in clinical manifestations. Therefore, most NHLs are diagnosed as stage III or IV (4). At present, there is no specific early diagnostic method or standard treatment for NHL in children. Without timely diagnosis and reasonable treatment, children with NHL may die in a short time and have an overall poor prognosis (4). The initial manifestations of some pediatric NHLs are complex, diverse and lack specificity. In addition, the poor expression ability of children and the concealment of some disease sites often lead to missed diagnosis or misdiagnosis (5).
The incidence of NHL is increasing yearly, and the clinical prognosis of early and late cases is very different. Consequently, it is of great significance to explore early diagnosis indicators for NHL prognosis. Serum tumor markers are commonly used as clinical reference indicators for tumor diagnosis, with an important role in the diagnosis of various malignant tumors. Thus, the screening of new biomarkers for the early diagnosis of pediatric NHL, as well as potential drug targets, is urgently needed. Furthermore, it has important clinical value for the in-depth study of the pathogenesis of NHL and for the identification of new therapeutic targets.
Proteomic-based approaches have become a very powerful technique for biomarker screening (6, 7). Among them, isobaric tags for relative and absolute quantification (iTRAQ) is extensively utilized as a high-content screening assay for identifying cancer protein biomarkers and is able to analyze eight kinds of different samples at the same time (8–10). In our research, we applied an iTRAQ-based approach to detect the differences in serum proteomes from NHL patients and controls (B-NHL vs. control and T-NHL vs. control), which was followed by bioinformatic analysis utilizing Ingenuity Pathway Analysis (IPA) to evaluate the changed proteome associated with NHL in children. Our findings will help identify potential serum biomarkers of pediatric NHL and explore their clinical value.
Materials and Methods
Clinical Serum Sample Collection
All serum specimens of children with B-NHL and T-NHL and healthy controls were collected from the First Affiliated Hospital of Zhengzhou University. This study followed the Declaration of Helsinki and was approved by the Institutional Ethics Committee of the Department of Medicine of the First Affiliated Hospital of Zhengzhou University. Informed consent was obtained from the parents or guardians prior to study initiation. All serum samples were discarded after clinical use. Newly diagnosed patients with NHL without radiotherapy or chemotherapy between January 2013 and December 2014 were included in this analysis. The diagnosis of primary NHL was based on a histological and immunohistochemical examination of tumor tissue and was classified according to the 2016 World Health Organization criteria (3). The staging of NHL was based on the St. Jude classification by Murphy, which has been used since 1980 (11). The essential features of the research subjects are shown in Table 1. Serum samples were stored at −80°C until analysis.
Sample Preparation and iTRAQ Labeling
The serum in the same volume from each of the 20 subjects in each group (B-NHL, T-NHL and healthy controls) was mixed. Then, the high-abundance proteins of each group were removed by applying the Human 14 Multiple Affinity Removal System (Agilent, Santa Clara, CA, USA). Proteins were extracted, and the concentration was measured using a Bradford Protein Assay Kit (Bio-Rad, Richmond, CA). For each sample, 100 μg protein (Control, B-NHL and T-NHL) was reductively alkylated, and trypsin was used for enzymatic hydrolysis. The resulting peptides were labeled with the 8-plex iTRAQ reagent kit according to the manufacturer’s protocol (Applied ABI, USA), and the conditions for each labeled reagent was performed as follows: Control group, iTRAQ reagent 113; B-NHL group, iTRAQ reagent 119 and T-NHL, iTRAQ reagent 121. The detailed procedures of iTRAQ have been described previously (8). After labeling, the three samples were mixed and desalted using a Sep-Pak Vac C18 column (Waters, USA) and vacuum-dried.
2D LC-MS/MS Analysis and Data Analysis
Peptides were fractioned, identified and quantified as previously described (8). In brief, labeled peptides were pre-separated using an ultraperformance liquid chromatography (UPLC) system with a C18 reverse-phase BEH column (50×2.1 mm, 1.7 µm, 130 Å, Waters, USA). The fractions were separated using a nano-HPLC system with a secondary reversed-phase analytical column (C18 column, 150 mm ×75 µm, 3 µm, 300 Å, Eksigent, USA) system and then subjected to ESI-Q-TOF mass spectrometry (Triple TOF4600, AB SCIEX, USA) for protein identification. For MS scans, the m/z scan range was 350–1250 Da. For MS/MS scans, the m/z scan range was 100–1250. Mascot 2.3.02 (Matrix Science, London, UK) and Scaffold (Proteome Software, Portland, OR, USA) software were used for the identification and quantification of proteins based on data from the international Swiss-Prot human database (20111015, human). Only proteins identified with at least 95% confidence, a fold change>1.2 (ratio > 1.2 or < 0.8) and a p value<0.05 were considered to be differentially expressed proteins (DEPs).
Bioinformatic Analyses
Ingenuity Pathway Analysis software (IPA) (version 7.1, Ingenuity System Inc., Redwood City, CA, USA; www.ingenuity.com) was used to analyze the biological functions, predominant canonical pathways, and protein-interaction networks associated with the DEPs. Significance levels were assessed by Fisher’s exact tests (p<0.05).
Enzyme-Linked Immunosorbent Assay-Based Validation
Serum concentrations of S100A8 and LRG1 were measured using human S100A8 and LRG1 ELISA assay kits (Uscn, China), respectively. ELISAs were performed as previously described (12) according to manufacturer’s instructions. The absorbance values of the standards and samples were determined by spectrophotometry at 450 nm using a microplate reader. Each sample was analyzed in duplicate.
Statistical Analysis
All data are presented as the mean ± standard deviation, with one-way analysis of variance (ANOVA) and unpaired Student’s t-tests employed for comparisons among the three experimental groups. The statistical analyses were performed using IBM SPSS Statistics for Windows (version 20.0, Armonk, NY, USA). Receiver operating characteristic (ROC) curves and the area under the ROC curve (AUC) were used to access the sensitivity and specificity, with p <0.05 considered statistically significant.
Results
In our research, we utilized iTRAQ combined with two-dimensional liquid chromatography-tandem mass spectrometry (2D LC–MS/MS) to screen serum DEPs, followed by enrichment analysis using IPA software and clinical validation by ELISA. The whole workflow of this study is shown in Figure 1.
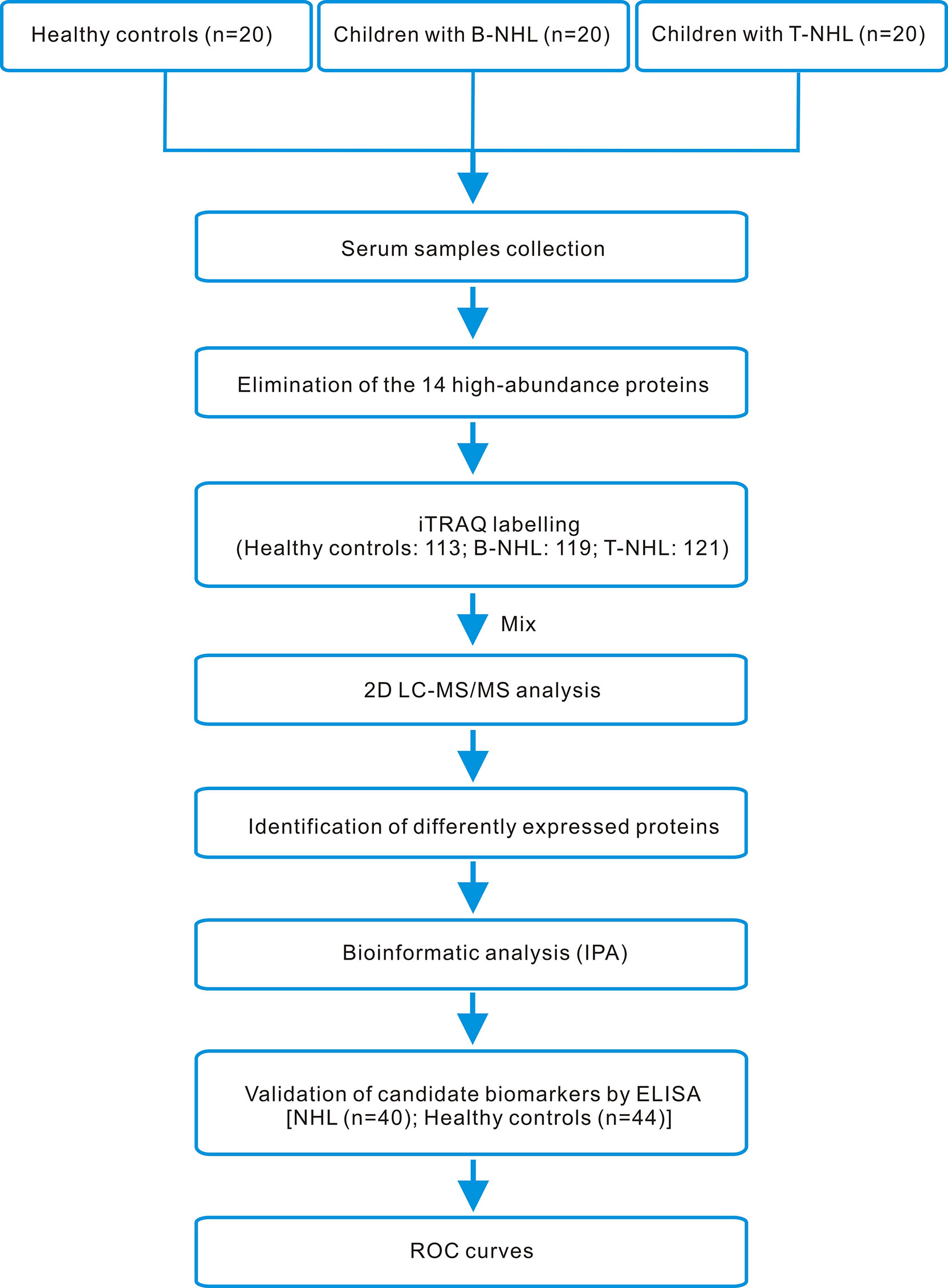
Figure 1 iTRAQ-based proteomics workflow of this study. Serum samples from healthy controls and children with B-NHL and T-NHL were pooled. After elimination of the 14 high-abundance proteins by applying the Human 14 Multiple Affinity Removal System (MARS), tryptic digestion and iTRAQ labeling, the peptide mixture was analyzed by 2D LC–MS/MS. The differentially expressed proteins were analyzed by the bioinformatics software IPA. The candidate biomarkers S100A8 and LRG1 were selected for further validation using ELISA. The efficacy of the candidate biomarkers was then evaluated using ROC curves.
Profiling of Serum Proteomes With iTRAQ-Based Proteomic Analysis
To identify novel serum biomarkers of pediatric B-NHL and T-NHL, the proteomes of serum samples from children with B-NHL (n=20) and T-NHL (n=20) and healthy controls (n=20) were quantitatively profiled using iTRAQ combined with 2D LC–MS/MS. As a result, we identified 534 nonredundant proteins containing at least one peptide. Detailed information on the identified proteins and the raw MS data are provided in Additional File 1. We defined proteins with a change in relative abundance greater than 1.2 times and p <0.05 as significant. Based on the cutoff value, the iTRAQ-based analyses revealed that the levels of 34 and 45 proteins were elevated and decreased, respectively, in B-NHL compared with healthy controls. Moreover, 45 and 28 proteins were overexpressed and underexpressed, respectively, in T-NHL compared with healthy controls. The results suggested that these DEPs had the potential to be relevant markers for pediatric NHL screening. The details of all DEPs are shown in Table 2 and Figure 2. Further analysis showed that the quantity of overexpressed proteins was greater than that of underexpressed proteins in the T-NHL group compared with the control group. In comparing the B-NHL group with the control group, the numbers of upregulated and downregulated proteins were similar. Moreover, among the DEPs, 19 overexpressed and 17 underexpressed proteins were shared by both B-NHL and T-NHL. 42 and 36 DEPs were specifically implicated in B-NHL and T-NHL, respectively. In addition, thymosin beta-4 was overexpressed in T-NHL but was underexpressed in B-NHL.
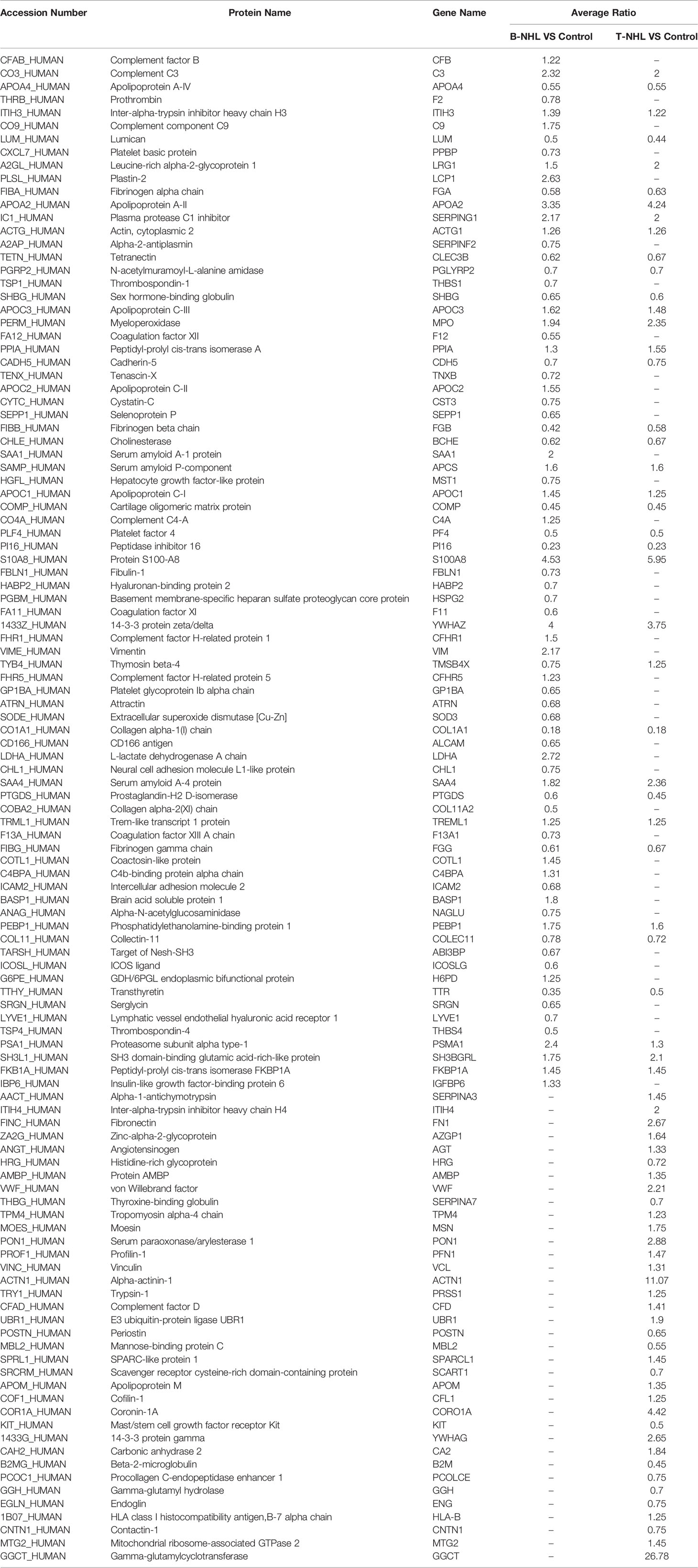
Table 2 Differentially expressed proteins identified in B-NHL and T-NHL compared with the control by iTRAQ-based proteomic analysis.
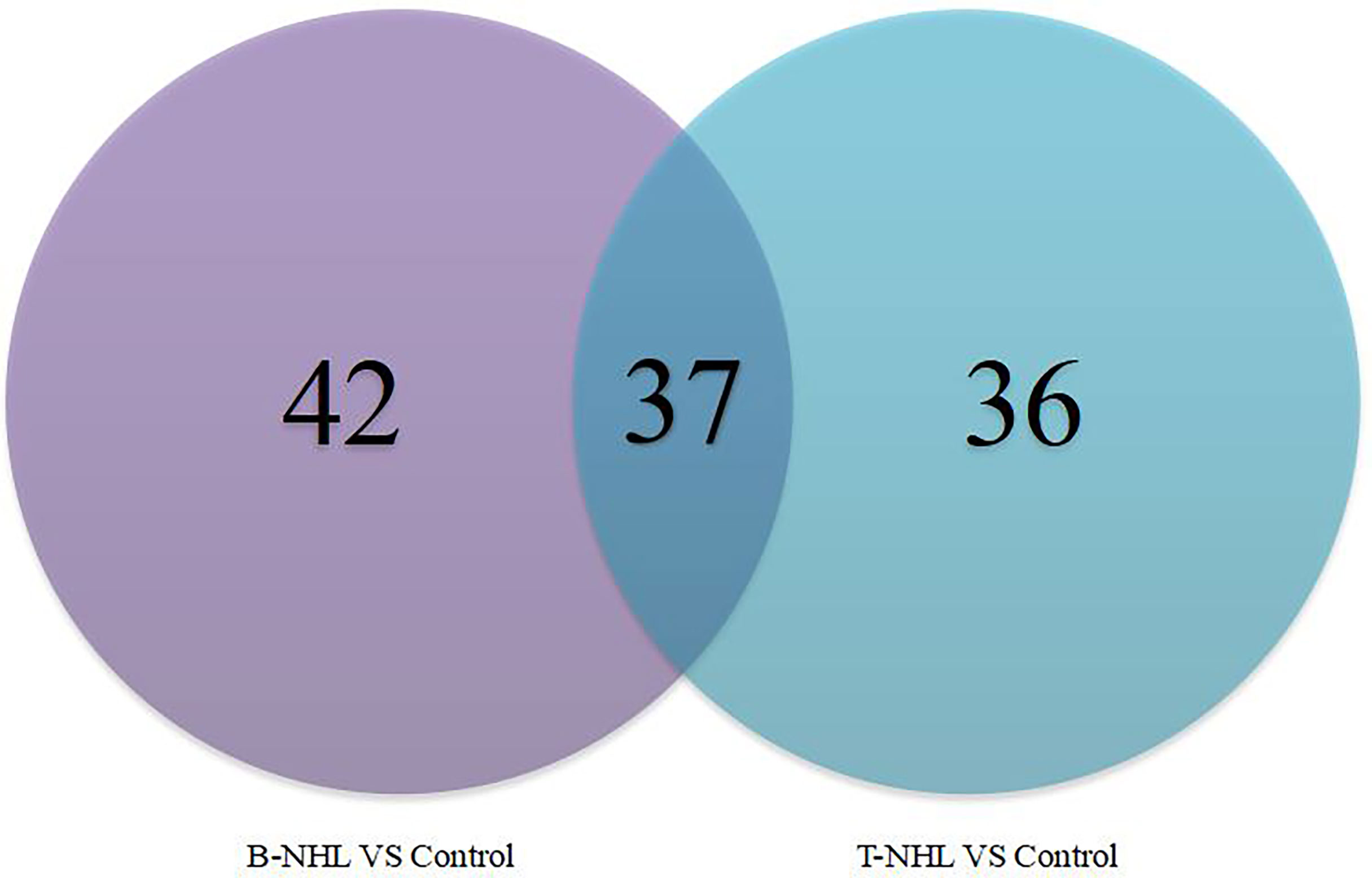
Figure 2 Venn diagram indicating the serum differentially expressed proteins between the pediatric B-NHL and T-NHL groups and the control group.
Bioinformatics Analysis of DEPs
To further explore the biological functions, pathways, and networks likely involved in pediatric NHL, we subjected the DEPs to IPA. The top 10 significant biological functions and canonical pathways are listed in Figures 3, 4, respectively. Among them, the functional analysis suggested that similar results were shared by the B-NHL and T-NHL groups. In IPA canonical pathway analysis, acute phase response signaling and liver X receptor/retinoid X receptor (LXR/RXR) activation were the 2 most prominent enriched pathways. The enriched pathways of DEPs in the B-NHL group mostly overlapped with those in the T-NHL group in comparison with the control group.
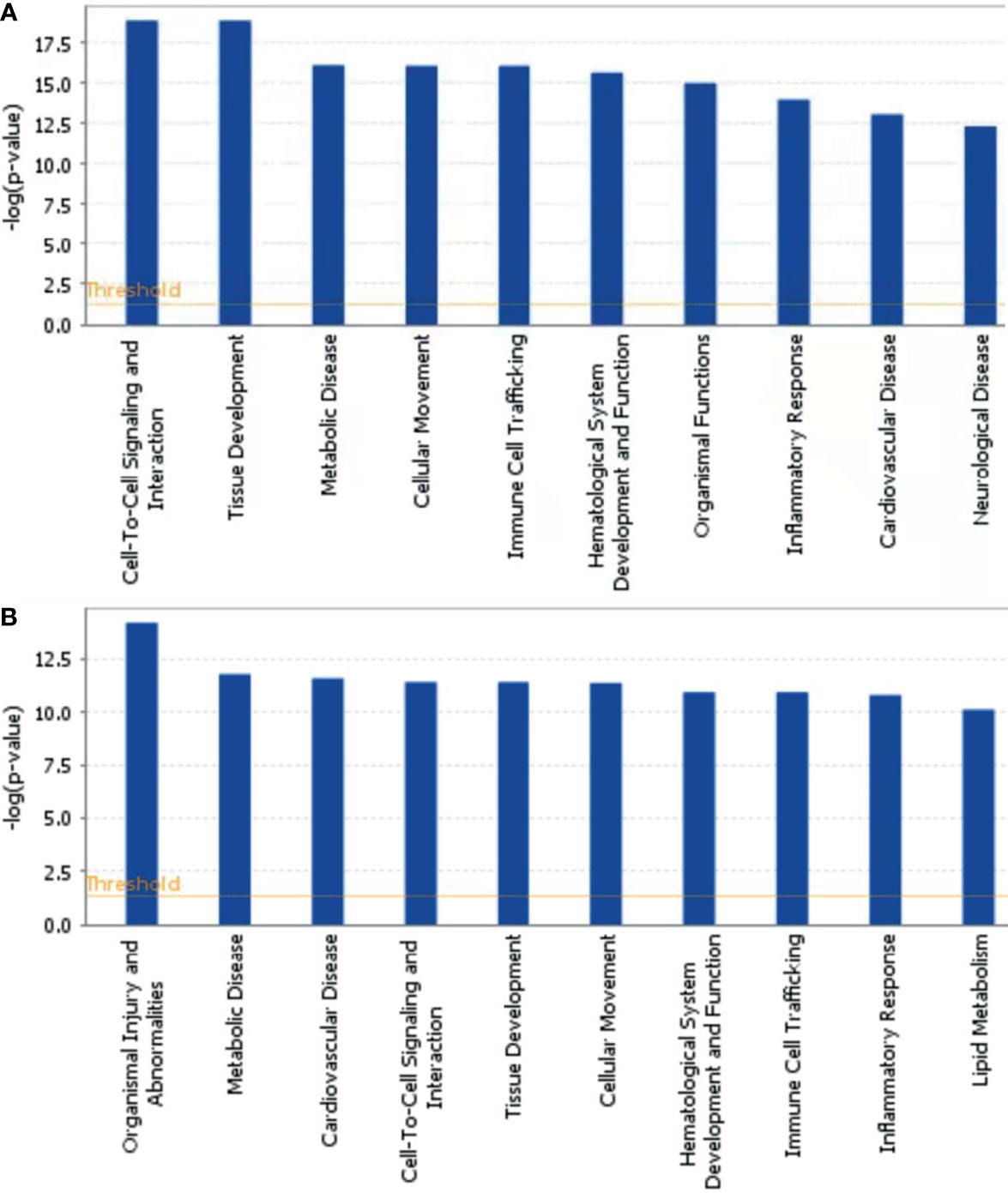
Figure 3 Predominant molecular functions based on differentially expressed proteins in B-NHL (A) and T-NHL (B) compared with the control. The cutoff of p value was set to 0.05.
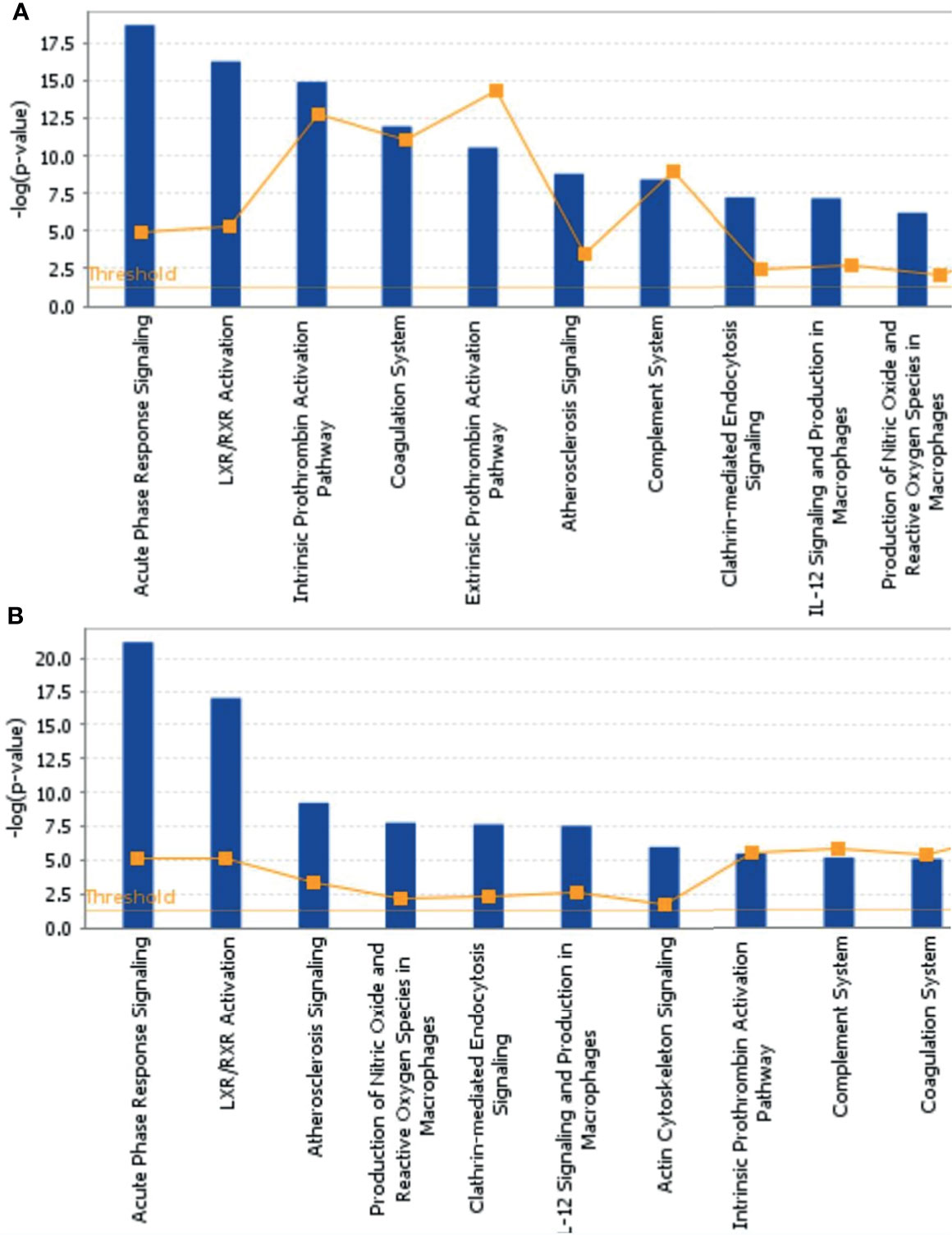
Figure 4 Predominant canonical pathways based on differentially expressed proteins in B-NHL (A) and T-NHL (B) compared with the control. The cutoff of p value was set to 0.05.
To further understand the DEPs in the B-NHL and T-NHL groups, protein–protein interaction network analyses were carried out. The results were shown in Additional Files 2, 3. In B-NHL, the 2 most significant networks were involved in hematological system development and function/organismal functions/humoral immune response, including 12 overexpressed and 12 underexpressed target molecules (score = 47), and cancer/gastrointestinal disease/cellular assembly and organization, with a score of 36 and containing 10 overexpressed and 8 underexpressed proteins (Figures 5A, B). The top 2 prominent networks in T-NHL were closely related to lipid metabolism/molecular transport/small molecule biochemistry, containing 13 upregulated and 8 downregulated proteins (score = 43), and dermatological diseases and conditions/immunological disease/inflammatory disease, with a score of 39 and containing 15 overexpressed and 3 underexpressed proteins (Figures 5C, D).
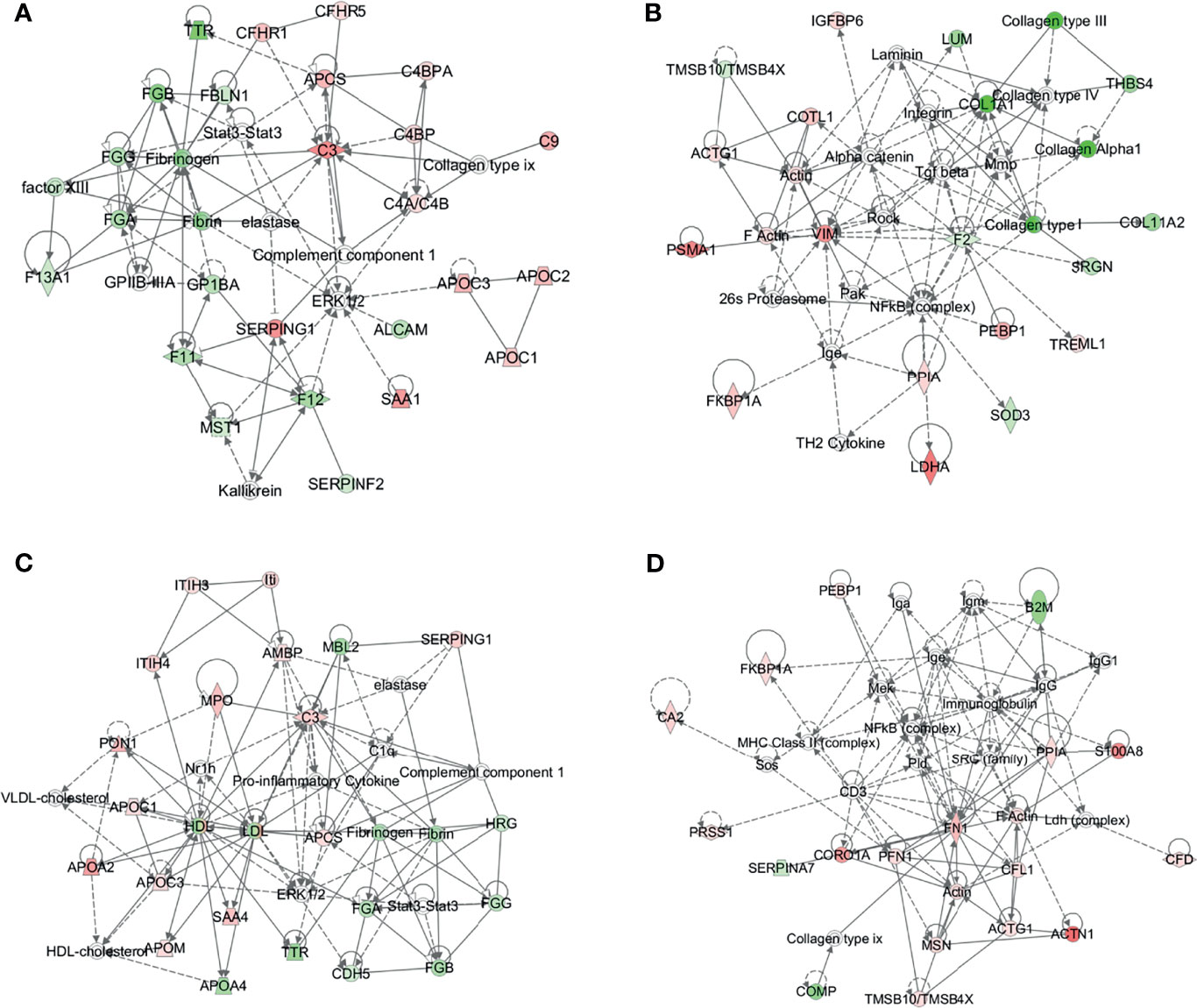
Figure 5 Protein–protein interaction (PPI) networks based on the differentially expressed proteins by IPA. The 2 representative PPI networks based on the differentially expressed proteins in B-NHL compared with the control (A, B). The 2 representative PPI networks based on the differentially expressed proteins in T-NHL compared with the control (C, D). Red denotes upregulated proteins, while green denotes downregulated proteins. Solid and dashed lines represent direct and indirect interactions, respectively.
Verification of DEPs by ELISA
To ascertain the clinical relevance of the iTRAQ results, the DEPs S100A8 and LRG1 were selected for further validation with sandwich ELISA in the individual serum specimens of 44 controls, 20 B-NHL patients and 20 T-NHL patients. The mean, SD and distribution of the protein concentrations in each group are shown in Table 3 and Figure 6. The experimental results demonstrated that the serum expression levels of S100A8 and LRG1 were significantly elevated in pediatric NHL compared to healthy children (p < 0.05).
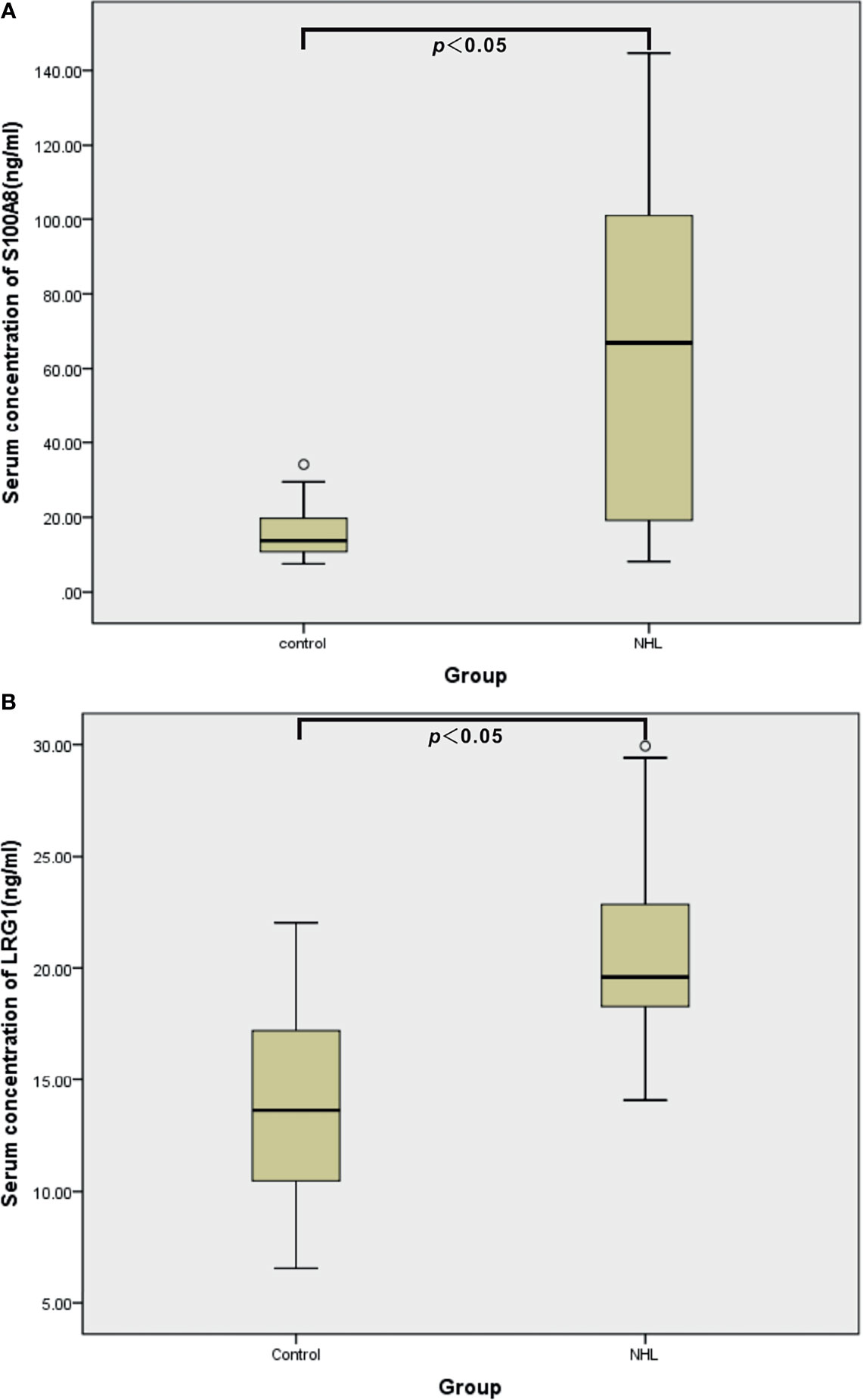
Figure 6 ELISA validation of serum S100A8 (A) and LRG1 (B) in healthy controls (n = 44) and children with NHL (n = 40).
Areas Under the Receiver Operating Characteristic (ROC) Curves (AUCs)
To further estimate the efficacy of serum S100A8 and LRG1 in differentiating children with NHL from healthy controls, ROC curves were generated, as shown in Table 4 and Figure 7. Our results presented unsatisfactory ROC curves of S100A8 and LRG1, which might be ascribed to the small sample size. As a result, the AUCs of S100A8, LRG1, and the combination of S100A8 and LRG1 were 0.873, 0.898 and 0.970, respectively. Serum S100A8 was able to discriminate NHL patients from healthy controls with a cutoff value of 37.880 ng/mL, with a sensitivity and specificity of 72.7% and 100%, respectively. With a cutoff value of 16.316 ng/mL, LRG1 was able to detect NHL with a sensitivity and specificity of 93.2% and 72.7%, respectively. The AUCs for differentiating between the NHL and control groups were 0.873 (95% confidence interval [CI]: 0.790-0.956) for S100A8 and 0.898 (95% CI: 0.816–0.980) for LRG1. Furthermore, the screening efficacy of S100A8 combined with LRG1 was better than that of each marker alone (95% CI: 0.937–1.000). Nevertheless, these results need clinical verification on a large scale.

Table 4 Receiver operating characteristics curves of the DEPs S100A8 and LRG1 in distinguishing pediatric NHL patients from healthy controls individually and in combination.
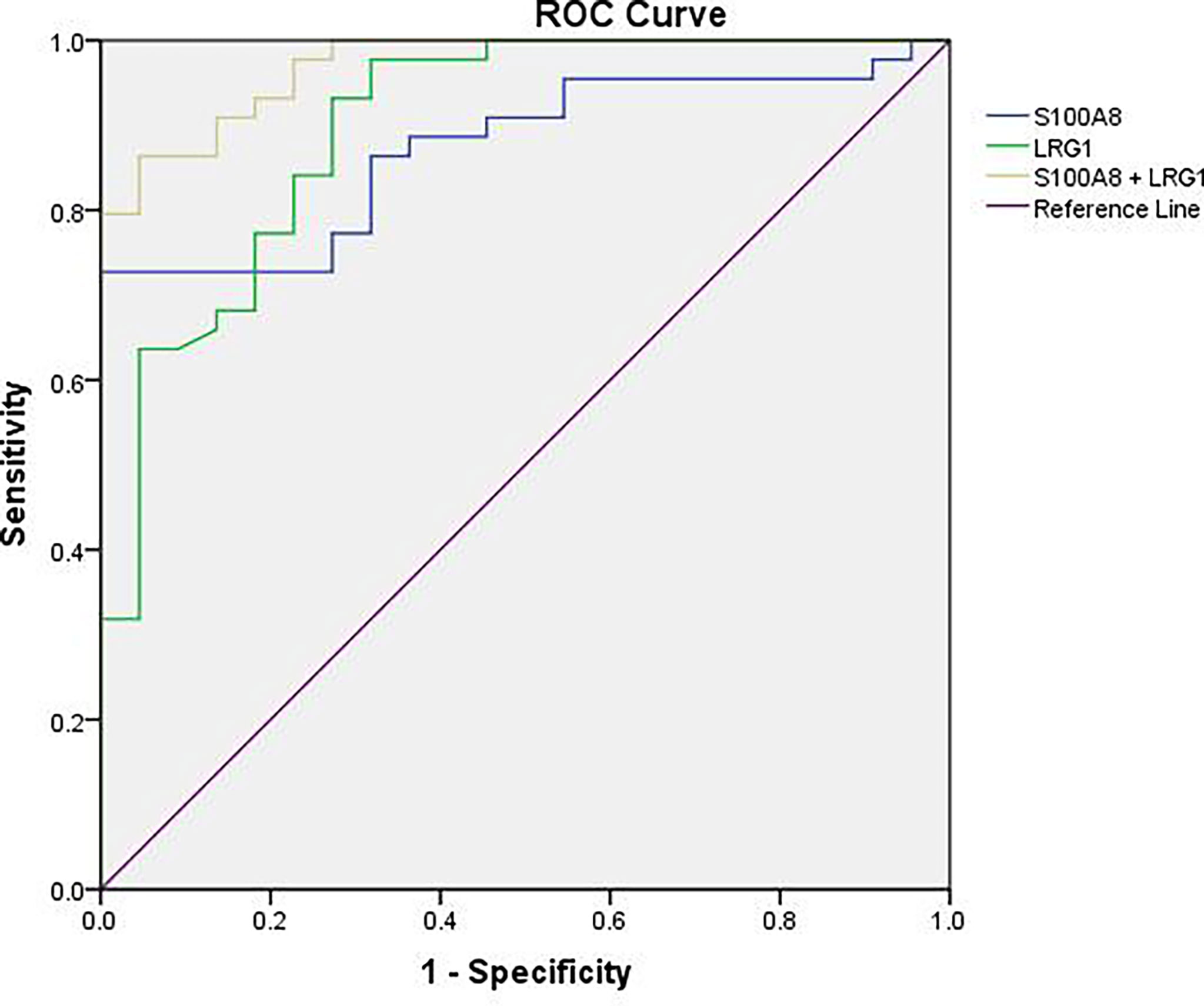
Figure 7 ROC curve analysis for the sensitivity and specificity of S100A8 alone, LRG1 alone, and their combination in the diagnosis of NHL.
Discussion
Pediatric NHL is a heterogeneous group of lymphoid malignancies excluding HL. NHL is the third most common malignant tumor among children aged 1 to 14 years, second only to acute leukemia and central nervous system neoplasms (1). With the marked progress in studies and chemotherapy regimens, the prognosis of pediatric NHL has been significantly improved internationally through the adoption of effective treatment strategies according to different pathological subtypes, with an overall survival rate now exceeding 80% (2). In addition to accurate pathological diagnosis, most studies have shown that the stage of NHL has a significant impact on prognosis. Compared with adult NHL, pediatric NHL is highly invasive and is characterized by a multicenter origin, long-distance dissemination and extranodal infiltration (13). The clinical manifestations of pediatric NHL vary greatly, presenting a highly invasive process. Therefore, most children are already in stage III and IV at the time of initial diagnosis.
In our study all B-NHL cases were BL, and all T-NHL cases were T-LBL. BL is the most common pathological type of pediatric NHL, accounting for 35%~40%, with significantly more males than females being affected. BL is a highly aggressive B-cell NHL and prone to central nervous system (CNS) invasion and early bone marrow metastasis (2, 14). It is reported that the majority (80%) of T-NHL is of T-cell origin, rather than B-cell origin, in children (2). T-LBL generally presents with a mediastinal mass and advanced-stage disease and may involve the bone marrow and, less often, the CNS at diagnosis (4). In this study, most of the children were males, consistent with a previous report (15). Furthermore, Almost all cases were in stage III or IV at the time of initial diagnosis, and there were no stage I cases. It is possible that the children in our study were diagnosed late and in critical condition. In addition, many doctors in China are not well informed about NHL, and early diagnosis needs to be improved. Early diagnosis of NHL can save a number of lives and reduce the physical and economic burden of disease management at advanced stages. Nevertheless, at onset, most patients diagnosed with NHL are in an advanced stage (stages III and IV) because of nonspecific clinical manifestations and the currently limited diagnostic methods (4, 5). The discovery of serum biomarkers that are noninvasive and have high efficacy for pediatric NHL detection can greatly improve the early diagnosis of NHL. For this purpose, the serum proteome of pediatric NHL was quantitatively determined in our research.
Serum is the most easily available specimen in clinical practice. Serum quantitative proteomics is a new technology to screen serum markers of diseases developed in recent years (6, 16). In this study, we aimed to discover useful serum biomarkers of pediatric B-NHL and T-NHL. For this purpose, the serum proteomes of B-NHL, T-NHL and healthy children were globally analyzed by utilizing iTRAQ in combination with 2D LC–MS/MS. The iTRAQ analysis identified 534 proteins, among which 79 and 73 DEPs were identified in the serum of B-NHL and T-NHL patients, respectively, compared with the controls according to our defined criteria. Further analysis showed that 34 and 45 were elevated and decreased, respectively, in children with B-NHL in comparison to those in the control group. Moreover, we identified 45 overexpressed and 28 underexpressed proteins in the T-NHL vs. control comparison. These results indicate that there are significant differences in serum proteins between NHL patients and healthy children, which may be used as candidate markers of NHL.
Furthermore, with the DEPs in B-NHL and T-NHL, IPA analysis revealed that the biological functions of tissue development, metabolic disease and cellular movement were highlighted. These biological functions are closely related to essential tumorigenesis processes. In line with the results of functional analysis, IPA analysis showed multiple pathways to be involved in the DEPs of the B-NHL and T-NHL groups, with the most significantly influenced pathways being acute phase response signaling and the LXR/RXR activation pathway. Acute phase response signaling is a nonspecific physiological and biochemical reaction to tissue damage, infection, inflammation, and cancer. The acute phase response is a significant component part of anticancer responses and is closely related to inflammation (17). Inflammation is a recognized hallmark of cancer that contributes to the initiation and progression of a tumor (18). Moreover, there is growing evidence that inflammation plays an important role in the progression of tumors and survival of patients with cancer (18, 19). The LXR/RXR activation pathway is involved in the modulation of cholesterol metabolism, glucose metabolism, and inflammatory responses (20–22). Numerous studies have suggested that activating LXRs could restrain carcinogenesis and accelerate cancer cell apoptosis, which makes LXRs a potential tumor therapeutic target (23–26). Our results suggest that the acute phase response signaling pathway and LXR/RXR activation pathway might play a role in the tumorigenesis of pediatric NHL. Moreover, it has been reported that aberrant lipid metabolism is one of the main aspects in cancer cells and can affect tumor progression (27, 28). Apolipoprotein A-II (APOA2) was identified as a minimally invasive biomarker for detecting pancreatic cancer, lung cancer and renal cell cancer (29–31). In our research, we found that APOA2 was also overexpressed in NHL in comparison to the control, indicating that lipid metabolism might be abnormal in pediatric NHL. APOA2 may play a critical role in pediatric B-NHL and T-NHL; nevertheless, the specific role of APOA2 in NHL is not completely clear. In the future, the role of APOA2 in pediatric NHL needs to be further studied. Another upregulated protein, YWHAZ, also known as 14-3-3ζ, is commonly overexpressed in a variety of neoplasms and plays a critical role in various cancers, including breast (32), lung (33), prostate (34), hepatocellular (35), and colorectal cancers (36). Emerging evidence has indicated that YWHAZ participates in a variety of important cellular processes, such as cell proliferation, cell cycle progression, apoptosis, migration, and invasion (37).
We performed an extensive and rigorous literature analysis of DEPs in order to evaluate potential biomarkers for pediatric NHL. In this study, S100A8 and LRG1, which were identified using our iTRAQ-based proteomics method and verified using ELISA, might be promising serum markers for pediatric NHL. We found that S100A8 and LRG1 were obviously elevated in pediatric NHL compared with the control. The changes in S100A8 and LRG1 proteins could supply valuable information to diagnose pediatric NHL.
Among the upregulated DEPs, S100A8 is highly overexpressed in multifarious tumors and can promote the initiation and progression of cancers by regulating tumor cell movement, proliferation, differentiation, apoptosis and drug resistance (38–40). Aberrantly overexpressed S100A8 is also related to the metastasis and prognosis of tumors (40). Studies on hematological malignancies have found that the expression levels of S100A8 and S100A9 were markedly increased in acute myeloid leukemia (AML), and high expression of S100A8 was a poor prognostic factor for AML patients (41, 42). Furthermore, S100A8 could inhibit differentiation and maintain the AML immature phenotype (41). S100A8 often heterodimerizes with S100A9 through chemical binding, and serum S100A8/A9 may be potential biomarkers for the treatment response unrelated to inflammation in HL (43). The levels of S100A8/A9 in saliva can help differentiate the subgroups of Sjögren’s syndrome with lymphoma risk (44). A recent report found that S100A8 could promote chemoresistance to adriamycin and vincristine by enhancing autophagy in B-cell lymphoma cells (45).
LRG1, another upregulated protein in NHL serum, was indicated to be involved in various cancers (46–50). Aberrant expression of serum LRG1 was correlated with a number of colonic adenomas (50). Furthermore, the expression level of LRG1 was associated with the localization and tumor volume of colorectal carcinomas and had predictive value for the early diagnosis and prognosis of colorectal cancer combined with other serum markers (47, 51, 52). Accumulating evidence suggests that LRG1 could promote abnormal angiogenesis by modulating endothelial TGF-β signaling but plays a smaller role in normal blood vessel growth (53, 54). Aberrant neovascularization contributes to cancer, so LRG1 might be a potential target for regulating pathological angiogenesis (55). In hematological malignancies, LRG1 was elevated in the serum of pediatric acute lymphoblastic leukemia at the time of diagnosis (8). Moreover, the expression levels of serum LRG1 returned to normal in pediatric B-ALL patients achieving complete remission after induction therapy (56). LRG1 gene silencing promoted cell apoptosis by downregulating antiapoptotic proteins and upregulating proapoptotic proteins in AML KASUMI-1 cells (57).
To date, there has been no evidence available concerning the S100A8 and LRG1 proteins, and abnormal expression of serum S100A8 and LRG1 in pediatric NHL from published proteomics data. Here, our study found that serum levels of S100A8 and LRG1 in NHL group significantly increased compared to control group. ROC curves showed that the combination of S100A8 and LRG1 had higher diagnostic values in screening patients with NHL. These results indicate that S100A8 and LRG1 might be promising biomarkers for pediatric NHL. Hence, it is speculated that S100A8 and LRG1 may contribute to the occurrence and progression of pediatric NHL. However, this study was only a preliminary study with a relatively small sample size. Although, the current study was limited by the relative small sample size, our results were able to prove statistically significant differences between NHL and the controls. Therefore, in future trials, the series of DEPs should be further validated in large-scale samples. In addition, the children with NHL in our study were diagnosed at an advanced stage (III/IV), which may be related to the high heterogeneity and invasiveness of the malignant cells and poor expression ability of children. Further studies using early cases are guaranteed to verify the potential clinical value of identified DEPs.
Conclusion
In conclusion, this is the first study using an iTRAQ-based proteomics approach to screen and identify serum DEPs of pediatric NHL, which could provide potential biomarkers for the early diagnosis, contributing to further understanding the pathogenesis of NHL and providing important experimental data for pediatric NHL. Among the large number of DEPs identified, serum S100A8 and LRG1 could serve as potential biomarkers for NHL diagnosis in the future. Further studies are needed to confirm and validate the DEPs on a large scale.
Data Availability Statement
The raw data of mass spectrometry can be found in the iProx database (accession number: IPX0004164000). Further inquiries can be directed to the corresponding authors.
Ethics Statement
This study followed the Declaration of Helsinki and was approved by the Institutional Ethics Committee of the Department of Medicine of the First Affiliated Hospital of Zhengzhou University. Informed consent was obtained from the parents or guardians pScience Foundation of Henan Province (212300410243rior to study initiation. All serum samples were discarded after clinical use. Written informed consent to participate in this study was provided by the participants’ legal guardian/next of kin.
Author Contributions
Sample collection and data analysis were performed by LC and SY. LC performed the depletion of high-abundance proteins and protein quantification of serum. RY performed iTRAQ labeling and two-dimensional LC-MS/MS. SY performed ELISA validation and statistical analysis. YL and ZZ validated bioinformatics and statistical methods. The first draft of the manuscript was written by RY. All authors contributed to the article and approved the submitted version.
Funding
This research was supported by funding from the Natural Science Foundation of Henan Province (212300410243), the Henan Provincial Science and Technology Research Project (212102310900) and the Henan Province Medical Science and Technology Tackling Program joint co-construction project (LHGJ20210056).
Conflict of Interest
The authors declare that the research was conducted in the absence of any commercial or financial relationships that could be construed as a potential conflict of interest.
Publisher’s Note
All claims expressed in this article are solely those of the authors and do not necessarily represent those of their affiliated organizations, or those of the publisher, the editors and the reviewers. Any product that may be evaluated in this article, or claim that may be made by its manufacturer, is not guaranteed or endorsed by the publisher.
Acknowledgments
The authors thank Yan Yang and Yang Zhang for their excellent technical assistance.
Supplementary Material
The Supplementary Material for this article can be found online at: https://www.frontiersin.org/articles/10.3389/fonc.2022.848286/full#supplementary-material
Additional File 1 | Differentially expressed proteins identified in our study.
Additional File 2 | Protein–protein interaction network analysis of differentially expressed proteins identified in B-NHL compared with the control.
Additional File 3 | Protein–protein interaction network analysis of differentially expressed proteins identified in T-NHL compared with the control.
References
1. Siegel RL, Miller KD, Fuchs HE, Jemal A. Cancer Statistics, 2022. CA Cancer J Clin (2022) 72:7–33. doi: 10.3322/caac.21708
2. Minard-Colin V, Brugières L, Reiter A, Cairo MS, Gross TG, Woessmann W, et al. Non-Hodgkin Lymphoma in Children and Adolescents: Progress Through Effective Collaboration, Current Knowledge, and Challenges Ahead. J Clin Oncol (2015) 33:2963–74. doi: 10.1200/JCO.2014.59.5827
3. Swerdlow SH, Campo E, Pileri SA, Harris NL, Stein H, Siebert R, et al. The 2016 Revision of the World Health Organization Classification of Lymphoid Neoplasms. Blood (2016) 127(20):2375–90. doi: 10.1182/blood-2016-01-643569
4. Cairo MS, Beishuizen A. Childhood, Adolescent and Young Adult Non-Hodgkin Lymphoma: Current Perspectives. Br J Haematol (2019) 185:1021–42. doi: 10.1111/bjh.15764
5. Burkhardt B, Hermiston ML. Lymphoblastic Lymphoma in Children and Adolescents: Review of Current Challenges and Future Opportunities. Br J Haematol (2019) 185(6):1158–70. doi: 10.1111/bjh.15793
6. Keshishian H, Burgess MW, Specht H, Wallace L, Clauser KR, Gillette MA, et al. Quantitative, Multiplexed Workflow for Deep Analysis of Human Blood Plasma and Biomarker Discovery by Mass Spectrometry. Nat Protoc (2017) 12(8):1683–701. doi: 10.1038/nprot.2017.054
7. Cravatt BF, Simon GM, Yates JR 3rd. The Biological Impact of Mass-Spectrometry-Based Proteomics. Nature (2007) 450(7172):991–1000. doi: 10.1038/nature06525
8. Yu R, Zhang J, Zang Y, Zeng L, Zuo W, Bai Y, et al. Itraq-Based Quantitative Protein Expression Profiling of Biomarkers in Childhood B-Cell and T-Cell Acute Lymphoblastic Leukemia. Cancer Manag Res (2019) 11:7047–63. doi: 10.2147/CMAR.S210093
9. Wu WW, Wang G, Baek SJ, Shen RF. Comparative Study of Three Proteomic Quantitative Methods, DIGE, Cicat, and Itraq, Using 2D Gel- or LC-MALDI TOF/TOF. J Proteome Res (2006) 5(3):651–8. doi: 10.1021/pr050405o
10. Wang W, Xie H, Xia B, Zhang L, Hou Y, Li K. Identifying Potential Markers for Monitoring Progression to Ovarian Cancer Using Plasma Label-Free Proteomics. J Cancer (2021) 12(6):1651–9. doi: 10.7150/jca.50733
11. Murphy SB. Classification, Staging and End Results of Treatment of Childhood Non-Hodgkin’s Lymphomas: Dissimilarities From Lymphomas in Adults. Semin Oncol (1980) 7(3):332–9.
12. Chanukuppa V, Taware R, Taunk K, Chatterjee T, Sharma S, Somasundaram V, et al. Proteomic Alterations in Multiple Myeloma: A Comprehensive Study Using Bone Marrow Interstitial Fluid and Serum Samples. Front Oncol (2020) 10:566804. doi: 10.3389/fonc.2020.566804
13. Sandlund JT, Downing JR, Crist WM. Non-Hodgkin’s Lymphoma in Childhood. N Engl J Med (1996) 334(19):1238–48. doi: 10.1056/NEJM199605093341906
14. Woessmann W, Zimmermann M, Meinhardt A, Müller S, Hauch H, Knörr F, et al. Progressive or Relapsed Burkitt Lymphoma or Leukemia in Children and Adolescents After BFM-Type First-Line Therapy. Blood (2020) 135:1124–32. doi: 10.1182/blood.2019003591
15. Suh JK, Gao YJ, Tang JY, Jou ST, Lin DT, Takahashi Y, et al. Clinical Characteristics and Treatment Outcomes of Pediatric Patients With Non-Hodgkin Lymphoma in East Asia. Cancer Res Treat (2020) 52:359–68. doi: 10.4143/crt.2019.219
16. Zhang P, Zhu S, Li Y, Zhao M, Liu M, Gao J, et al. Quantitative Proteomics Analysis to Identify Diffuse Axonal Injury Biomarkers in Rats Using Itraq Coupled LC-MS/MS. J Proteomics (2016) 133:93–9. doi: 10.1016/j.jprot.2015.12.014
17. Gabay C, Kushner I. Acute-Phase Proteins and Other Systemic Responses to Inflammation. N Engl J Med (1999) 340:448–54. doi: 10.1056/NEJM199902113400607
18. Diakos CI, Charles KA, McMillan DC, Clarke SJ. Cancer-Related Inflammation and Treatment Effectiveness. Lancet Oncol (2014) 15:e493–503. doi: 10.1016/S1470-2045(14)70263-3
19. Nakamura K, Smyth MJ. Targeting Cancer-Related Inflammation in the Era of Immunotherapy. Immunol Cell Biol (2017) 95:325–32. doi: 10.1038/icb.2016.126
20. Scott J. The Liver X Receptor and Atherosclerosis. N Engl J Med (2007) 357(21):2195–7. doi: 10.1056/NEJMcibr075951
21. Hong C, Tontonoz P. Liver X Receptors in Lipid Metabolism: Opportunities for Drug Discovery. Nat Rev Drug Discov (2014) 13(6):433–44. doi: 10.1038/nrd4280
22. Zelcer N, Tontonoz P. Liver X Receptors as Integrators of Metabolic and Inflammatory Signaling. J Clin Invest (2006) 116:607–14. doi: 10.1172/JCI27883
23. Lin CY, Gustafsson JÅ. Targeting Liver X Receptors in Cancer Therapeutics. Nat Rev Cancer (2015) 15(4):216–24. doi: 10.1038/nrc3912
24. Wang Q, Wang J, Wang J, Zhang H. Molecular Mechanism of Liver X Receptors in Cancer Therapeutics. Life Sci (2021) 273:119287. doi: 10.1016/j.lfs.2021.119287
25. Pontini L, Marinozzi M. Shedding Light on the Roles of Liver X Receptors in Cancer by Using Chemical Probes. Br J Pharmacol (2021) 178(16):3261–76. doi: 10.1111/bph.15200
26. Ju X, Huang P, Chen M, Wang Q. Liver X Receptors as Potential Targets for Cancer Therapeutics. Oncol Lett (2017) 14(6):7676–80. doi: 10.3892/ol.2017.7259
27. Ray U, Roy SS. Aberrant Lipid Metabolism in Cancer Cells - The Role of Oncolipid-Activated Signaling. FEBS J (2018) 285(3):432–43. doi: 10.1111/febs.14281
28. Visweswaran M, Arfuso F, Warrier S, Dharmarajan A. Aberrant Lipid Metabolism as an Emerging Therapeutic Strategy to Target Cancer Stem Cells. Stem Cells (2020) 38(1):6–14. doi: 10.1002/stem.3101
29. Kato S, Honda K. Use of Biomarkers and Imaging for Early Detection of Pancreatic Cancer. Cancers (Basel) (2020) 12(7):1965. doi: 10.3390/cancers12071965
30. Yoon HI, Kwon OR, Kang KN, Shin YS, Shin HS, Yeon EH, et al. Diagnostic Value of Combining Tumor and Inflammatory Markers in Lung Cancer. J Cancer Prev (2016) 21(3):187–93. doi: 10.15430/JCP.2016.21.3.187
31. Vermaat JS, van der Tweel I, Mehra N, Sleijfer S, Haanen JB, Roodhart JM, et al. Two-Protein Signature of Novel Serological Markers Apolipoprotein-A2 and Serum Amyloid Alpha Predicts Prognosis in Patients With Metastatic Renal Cell Cancer and Improves the Currently Used Prognostic Survival Models. Ann Oncol (2010) 21(7):1472–81. doi: 10.1093/annonc/mdp559
32. Wang W, Zhang L, Wang Y, Ding Y, Chen T, Wang Y, et al. Involvement of Mir-451 in Resistance to Paclitaxel by Regulating YWHAZ in Breast Cancer. Cell Death Dis (2017) 8(10):e3071. doi: 10.1038/cddis.2017.460
33. Chen CH, Chuang SM, Yang MF, Liao JW, Yu SL, Chen JJ. A Novel Function of YWHAZ/β-Catenin Axis in Promoting Epithelial-Mesenchymal Transition and Lung Cancer Metastasis. Mol Cancer Res (2012) 10(10):1319–31. doi: 10.1158/1541-7786.MCR-12-0189
34. Murata T, Takayama K, Urano T, Fujimura T, Ashikari D, Obinata D, et al. 14-3-3ζ, A Novel Androgen-Responsive Gene, Is Upregulated in Prostate Cancer and Promotes Prostate Cancer Cell Proliferation and Survival. Clin Cancer Res (2012) 18(20):5617–27. doi: 10.1158/1078-0432.CCR-12-0281
35. Zhao JF, Zhao Q, Hu H, Liao JZ, Lin JS, Xia C, et al. The ASH1-Mir-375-YWHAZ Signaling Axis Regulates Tumor Properties in Hepatocellular Carcinoma. Mol Ther Nucleic Acids (2018) 11:538–53. doi: 10.1016/j.omtn.2018.04.007
36. Li Y, Wang J, Dai X, Zhou Z, Liu J, Zhang Y, et al. Mir-451 Regulates Foxo3 Nuclear Accumulation Through Ywhaz in Human Colorectal Cancer. Am J Transl Res (2015) 7(12):2775–85.
37. Gan Y, Ye F, He XX. The Role of YWHAZ in Cancer: A Maze of Opportunities and Challenges. J Cancer (2020) 11(8):2252–64. doi: 10.7150/jca.41316
38. Lim SY, Yuzhalin AE, Gordon-Weeks AN, Muschel RJ. Tumor-Infiltrating Monocytes/Macrophages Promote Tumor Invasion and Migration by Upregulating S100A8 and S100A9 Expression in Cancer Cells. Oncogene (2016) 35(44):5735–45. doi: 10.1038/onc.2016.107
39. Allgöwer C, Kretz AL, von Karstedt S, Wittau M, Henne-Bruns D, Lemke J. Friend or Foe: S100 Proteins in Cancer. Cancers (Basel) (2020) 12(8):2037. doi: 10.3390/cancers12082037
40. Lukanidin E, Sleeman JP. Building the Niche: The Role of the S100 Proteins in Metastatic Growth. Semin Cancer Biol (2012) 22(3):216–25. doi: 10.1016/j.semcancer.2012.02.006
41. Laouedj M, Tardif MR, Gil L, Raquil MA, Lachhab A, Pelletier M, et al. S100A9 Induces Differentiation of Acute Myeloid Leukemia Cells Through TLR4. Blood (2017) 129(14):1980–90. doi: 10.1182/blood-2016-09-738005
42. Nicolas E, Ramus C, Berthier S, Arlotto M, Bouamrani A, Lefebvre C, et al. Expression of S100A8 in Leukemic Cells Predicts Poor Survival in De Novo AML Patients. Leukemia (2011) 25(1):57–65. doi: 10.1038/leu.2010.251
43. Şumnu Ş, Mehtap Ö, Mersin S, Toptaş T, Görür G, Gedük A, et al. Serum Calprotectin (S100A8/A9) Levels as a New Potential Biomarker of Treatment Response in Hodgkin Lymphoma. Int J Lab Hematol (2021) 43(4):638–44. doi: 10.1111/ijlh.13559
44. Jazzar AA, Shirlaw PJ, Carpenter GH, Challacombe SJ, Proctor GB. Salivary S100A8/A9 in Sjögren’s Syndrome Accompanied by Lymphoma. J Oral Pathol Med (2018) 47(9):900–6. doi: 10.1111/jop.12763
45. Zhang L, Zhou S, Zhou T, Yuan K, Li X, Tang J. S100A8 Promotes Chemoresistance via Augmenting Autophagy in B−Cell Lymphoma Cells. Oncol Rep (2021) 45(1):151–8. doi: 10.3892/or.2020.7841
46. Kwan YP, Teo M, Lim J, Tan MS, Rosellinny G, Wahli W, et al. LRG1 Promotes Metastatic Dissemination of Melanoma Through Regulating EGFR/STAT3 Signalling. Cancers (Basel) (2021) 13(13):3279. doi: 10.3390/cancers13133279
47. Surinova S, Choi M, Tao S, Schüffler PJ, Chang CY, Clough T, et al. Prediction of Colorectal Cancer Diagnosis Based on Circulating Plasma Proteins. EMBO Mol Med (2015) 7(9):1166–78. doi: 10.15252/emmm.201404873
48. Ramirez-Ardila DE, Ruigrok-Ritstier K, Helmijr JC, Look MP, van Laere S, Dirix L, et al. LRG1 Mrna Expression in Breast Cancer Associates With PIK3CA Genotype and With Aromatase Inhibitor Therapy Outcome. Mol Oncol (2016) 10(8):1363–73. doi: 10.1016/j.molonc.2016.07.004
49. Fahrmann JF, Bantis LE, Capello M, Scelo G, Dennison JB, Patel N, et al. A Plasma-Derived Protein-Metabolite Multiplexed Panel for Early-Stage Pancreatic Cancer. J Natl Cancer Inst (2019) 111(4):372–9. doi: 10.1093/jnci/djy126
50. Ivancic MM, Anson LW, Pickhardt PJ, Megna B, Pooler BD, Clipson L, et al. Conserved Serum Protein Biomarkers Associated With Growing Early Colorectal Adenomas. Proc Natl Acad Sci USA (2019) 116(17):8471–80. doi: 10.1073/pnas.1813212116
51. Surinova S, Radová L, Choi M, Srovnal J, Brenner H, Vitek O, et al. Non-Invasive Prognostic Protein Biomarker Signatures Associated With Colorectal Cancer. EMBO Mol Med (2015) 7(9):1153–65. doi: 10.15252/emmm.201404874
52. Zhong ME, Chen Y, Xiao Y, Xu L, Zhang G, Lu J, et al. Serum Extracellular Vesicles Contain SPARC and LRG1 as Biomarkers of Colon Cancer and Differ by Tumour Primary Location. EBioMedicine (2019) 50:211–23. doi: 10.1016/j.ebiom.2019.11.003
53. Wang X, Abraham S, McKenzie JAG, Jeffs N, Swire M, Tripathi VB, et al. LRG1 Promotes Angiogenesis by Modulating Endothelial TGF-β Signalling. Nature (2013) 499(7458):306–11. doi: 10.1038/nature12345
54. Li Z, Zeng C, Nong Q, Long F, Liu J, Mu Z, et al. Exosomal Leucine-Rich-Alpha2-Glycoprotein 1 Derived From Non-Small-Cell Lung Cancer Cells Promotes Angiogenesis via TGF-β Signal Pathway. Mol Ther Oncolytics (2019) 14:313–22. doi: 10.1016/j.omto.2019.08.001
55. Carmeliet P, Jain RK. Molecular Mechanisms and Clinical Applications of Angiogenesis. Nature (2011) 473(7347):298–307. doi: 10.1038/nature10144
56. Cavalcante Mde S, Torres-Romero JC, Lobo MD, Moreno FB, Bezerra LP, Lima DS, et al. A Panel of Glycoproteins as Candidate Biomarkers for Early Diagnosis and Treatment Evaluation of B-Cell Acute Lymphoblastic Leukemia. Biomark Res (2016) 4:1. doi: 10.1186/s40364-016-0055-6
Keywords: non-Hodgkin’s lymphoma, proteomics, children, isobaric tags for relative and absolute quantification (iTRAQ), serum
Citation: Yu R, Cheng L, Yang S, Liu Y and Zhu Z (2022) iTRAQ-Based Proteomic Analysis Reveals Potential Serum Biomarkers for Pediatric Non-Hodgkin’s Lymphoma. Front. Oncol. 12:848286. doi: 10.3389/fonc.2022.848286
Received: 04 January 2022; Accepted: 21 February 2022;
Published: 17 March 2022.
Edited by:
Xianquan Zhan, Shandong First Medical University, ChinaCopyright © 2022 Yu, Cheng, Yang, Liu and Zhu. This is an open-access article distributed under the terms of the Creative Commons Attribution License (CC BY). The use, distribution or reproduction in other forums is permitted, provided the original author(s) and the copyright owner(s) are credited and that the original publication in this journal is cited, in accordance with accepted academic practice. No use, distribution or reproduction is permitted which does not comply with these terms.
*Correspondence: Zunmin Zhu, zhuzm1964@163.com; Yufeng Liu, lyf6012@163.com