- 1Candiolo Cancer Institute, FPO-IRCCS, Turin, Italy
- 2Department of Oncology, University of Torino, Turin, Italy
- 3Department of Clinical and Biological Sciences, University of Torino, Turin, Italy
- 4Department of Computer Science, University of Torino, Turin, Italy
- 5Department of Medical Sciences, University of Torino, Turin, Italy
- 6Department of Pathology, Azienda Ospedale-Università Padova, Padua, Italy
- 7Department of Medicine (DIMED), University of Padua School of Medicine, Padua, Italy
- 8Medical Oncology, AOU San Luigi Gonzaga, Orbassano (TO), Italy
Background: Advanced and unresectable bone and soft tissue sarcomas (BSTS) still represent an unmet medical need. We demonstrated that the alkylating agent trabectedin and the PARP1-inhibitor olaparib display antitumor activity in BSTS preclinical models. Moreover, in a phase Ib clinical trial (NCT02398058), feasibility, tolerability and encouraging results have been observed and the treatment combination is currently under study in a phase II trial (NCT03838744).
Methods: Differential expression of genes involved in DNA Damage Response and Repair was evaluated by Nanostring® technology, extracting RNA from pre-treatment tumor samples of 16 responder (≥6-month progression free survival) and 16 non-responder patients. Data validation was performed by quantitative real-time PCR, RNA in situ hybridization, and immunohistochemistry. The correlation between the identified candidate genes and both progression-free survival and overall survival was investigated in the publicly available dataset “Sarcoma (TCGA, The Cancer Genome Atlas)”.
Results: Differential RNA expression analysis revealed an 8-gene signature (CDKN2A, PIK3R1, SLFN11, ATM, APEX2, BLM, XRCC2, MAD2L2) defining patients with better outcome upon trabectedin+olaparib treatment. In responder vs. non-responder patients, a significant differential expression of these genes was further confirmed by RNA in situ hybridization and by qRT-PCR and immunohistochemistry in selected experiments. Correlation between survival outcomes and genetic alterations in the identified genes was shown in the TCGA sarcoma dataset.
Conclusions: This work identified an 8-gene expression signature to improve prediction of response to trabectedin+olaparib combination in BSTS. The predictive role of these potential biomarkers warrants further investigation.
Introduction
Bone and soft tissue sarcomas (BSTS) are a wide and heterogeneous family of rare tumors sharing features of mesenchymal origin (1). In advanced stages, when the disease is unresectable or metastatic, prognosis is dismal. Medical treatment may delay progression, with marginal improvement in overall survival (OS) (2–5). This scenario is further complicated by the relative poorness of predictive factors to improve sarcoma patient selection for patient-tailored treatments, with the noteworthy exception of gastrointestinal stromal tumors (6). Hence, in the sarcoma field, there is a huge need to explore innovative therapies, focusing on combinations of cytotoxic compounds, target therapies and immunotherapeutic strategies, to optimize treatment personalization and increase tumor control in terms of mass shrinkage or - at least - sarcoma growth arrest. In recent years, several combinations have been tested (7–10) but, once again, these studies demonstrated promising results only in small subsets of patients. Hence, there is an urgent need to identify predictive biomarkers of tumor response to refine patient selection, according to the concept of precision medicine (11). In this perspective, we focused on the combination of trabectedin, an isoquinoline alkylating agent of marine origin, and the inhibitor of the enzyme poly-(ADP-ribose) polymerase 1 (PARP1) olaparib. Preclinical and clinical data confirmed feasibility and suggested hints of activity in a fraction of the enrolled patients, emphasizing the need to improve patient selection through the identification of specific predictive factors (12, 13). Both drugs under study – trabectedin and olaparib – had already been studied as single agents, looking for predictive factors among the tightly intertwined mechanisms ruled by DNA Damage Response and Repair (DDRR) genes (14–24). However, potential predictive factors of combined trabectedin+olaparib treatment response have not been investigated, so far.
Trabectedin creates DNA adducts by interfering with active transcription, wherein its activity is dependent on transcription-coupled nucleotide excision repair (TC-NER) (25–27). NER defects make tumor cells less sensitive to trabectedin damage and high expression levels of ERCC1 and XPG/ERCC5 (“signs” of a proficient NER machinery) have been described as predictive of better response to trabectedin treatment (14–16, 18). Tumor cells bearing homologous repair deficiency (HRD) are more sensitive to trabectedin-induced cell death, as they cannot recruit the proper machinery to repair the double-strand breaks (DSBs) generated upon trabectedin treatment. Non-homologous end joining (NHEJ) defects, instead, seem to have only a minor effect on trabectedin efficacy (14–16, 28). At present, none of these potential predictive factors of response upon trabectedin treatment has received approval for clinical use.
Differently, PARP1-inhibitors (PARP1i) have been marketed with specific indications with respect to homologous recombination (HR) status, which is considered a predictive biomarker of response to PARP1i (24, 29, 30). The European Medicine Agency has approved olaparib use in ovarian cancer with HRD, while for pancreatic, prostate and breast cancers the indication more strictly refers to BRCA1/2-mutated patients. Hence, BRCA mutational status and HRD are clinical-grade approved predictive biomarkers, to better select patients who might benefit more from PARP1i treatment. Indeed, in cells showing HRD, NHEJ takes action upon DSBs formation, but with respect to HR, considered “error-free”, NHEJ provides DSBs repair with practically no consideration for sequence homology. The error-prone NHEJ promotes accumulation of DNA damage, and its activity is a major driver for PARP1i synthetic lethality in HR-defective cells (31).
The different mechanisms of action of trabectedin and olaparib with respect to DDRR genes imply that finding predictive factors of response to their combined treatment cannot be assumed to be a simple summation. The objective of the present translational, exploratory study, was to look for a potential predictive gene expression signature of response to trabectedin and olaparib in sarcoma patients, taking advantage of tumor specimens derived from patients treated with this combination in the phase Ib TOMAS trial (13).
Materials and methods
Patient-derived samples
Patient samples were all derived from the Phase Ib TOMAS study patient cohort. Only patients treated at or above the third dose level were selected (trabectedin 0.920 mg/m2 q21d, olaparib 200 mg BID). The available material included pre-treatment biopsies or surgical specimens (formalin-fixed, paraffin-embedded; FFPE). All enrolled patients gave written, signed informed consent for the use of tumor samples for biomarker and exploratory analyses. The clinical study protocol was approved by the Institutional Review Board (IRB) and Ethics Committee of each participating center. All study procedures were performed in accordance to the Declaration of Helsinki.
DNA and RNA extraction; DNA data analysis
DNA was extracted from patients’ specimens as previously described (13). DNA purity was checked by NanoDrop™ (Thermo Fisher Scientific, Life Technology, Monza, Italy). DNA concentration was determined by the Qubit dsDNA BR (broad range) and HS (high sensitivity) assay kits (Thermo Fisher Scientific) and the Qubit® 3.0 Fluorometer (Thermo Fisher Scientific). DNA fragmentation was assessed by gel electrophoresis and by 2100 Bioanalyzer Instrument, with High Sensitivity DNA assay Kit (Agilent Technologies, Agilent Technologies, Inc., Santa Clara, California).
Good quality DNA samples underwent whole exome sequencing (WES) using the Twist Bioscience® Human Core Exome (Consensus CDS) + IntegraGen content, for a genomic target of 37 Mb by IntegraGen SA (IntegraGen SA, Evry, France), and Novaseq 6000 sequencer (Illumina) with an average sequencing depth of 135X depth per exome and a coverage >98% at >50X. Genetic discovery analysis was performed by an in-house NGS pipeline (32, 33), constructed for WES analyses of paired cancer genomes in order to call somatic variations, indels and copy number alterations (CNA).
By means of publicly available databases (ClinVar) and PolyPhen-2 prediction tool (34), all benign variants were filtered out from the genetic analysis. Mutations were first looked into in the ClinVar database; if pathogenic, no further analysis was performed. If one mutation was found as being of unknown significance in the ClinVar database, or not described at all, PolyPhen-2 prediction tool was used to inquire its potential detrimental effect on protein function. Lower-quality DNA samples were analyzed with Oncomine-Comprehensive cancer panel v3 (Thermo Fisher Scientific) and meaningful alterations were filtered in with Ion ReporterTM 5.18.2.0 (Thermo Fisher Scientific).
RNA was extracted from FFPE samples with Maxwell® RSC FFPE Kit (Promega Corporation, Madison, WI, USA) and Maxwell® RSC Instrument. RNA purity, concentration and fragmentation were determined using DeNovix DS-11+ Spectrophotometer (DeNovix Inc., Wilmington, DE, USA), Qubit®3.0 Fluorometer (Invitrogen by Life Technologies, Eugene, Oregon, USA) and the Agilent 2100 Bioanalyzer System (Agilent Technologies, Wilmington, DE, USA), respectively.
Nanostring® nCounter assay
Expression of DDRR genes in tumor samples, was determined by NanoString® nCounter Technology (NanoString Technologies, Seattle, WA, USA) by means of the nCounter®Vantage 3D™ RNA DNA Damage and Response Panel. Following manufacturer’s instructions, samples were prepared for hybridization, processed in the Prep Station, counted by the nCounter® Analysis System.
Quantitative real-time polymerase chain reaction and droplet digital absolute qPCR
For Real-time PCR analysis, 1 µg of total RNA was reverse-transcribed into cDNA using SuperScript IV VILO Master Mix (Thermo Fisher Scientific). TaqMan PCR analysis was performed with TaqMan Gene Expression Master Mix by means of ABI PRISM 7900HT System (Applied Biosystems, Monza, Italy). Taqman probes (Thermo Fisher Scientific) were as following: CDKN2A (Hs00923894_m1), APEX2 (Hs00205565_M1). Fluorescence data were automatically converted into Ct (cycle threshold) values. Data export (threshold 0.20) and analysis was performed by Microsoft Office Excel. Expression data were normalized to the geometric mean of housekeeping genes. For housekeeping genes, the Taqman probes (Thermo Fisher Scientific) were as following: B2M (Hs00984230_m1), UBC (Hs00824723_m1), GAPDH (Hs99999905_m1), ACTB (Hs99999903_m1).
The search for CDKN2A gene copy number was carried out by droplet digital PCR (ddPCR) as follows: DNA isolated from FFPE tumor tissues (as described above) was amplified using ddPCR Supermix for Probes (Bio-Rad, Hercules, CA, USA), using CDKN2A and housekeeping genes (EIF2C1, AP3B1, RPP30) probes (Bio-Rad, Segrate, Italy), according to manufacturer’s protocols as described in (35).
In situ hybridization
The RNAscope® Assay was used for in situ hybridization on FFPE tissue following standard protocol procedures (36). Specific preparation and pre-treatment included target retrieval lasting 10-15 minutes and Protease Plus incubation for 30 minutes.
Immunohistochemistry
CDKN2A/p16 immunohistochemistry (IHC), on FFPE tumor tissues, was performed with a BOND-MAX automated staining platform (Leica Biosystems, Buccinasco, Italy), according to standard procedure. The specimens were sectioned at a thickness of 3 μm and stained on glass slides baked at 60°C for 30 minutes. Deparaffinization, rehydration and antigen retrieval were performed by Bond Dewax Solution, Bond Wash Solution, ethanol and Bond ER Solution 1 (prediluted; pH 6.0) antigen retrieval solution (Leica), performed on the BOND-MAX automated slide stainer (Leica) for 30 minutes at 95°C. The ready-to-use primary CDKN2A/p16 primary anti-human antibody (6H12; Leica), was incubated for 20 minutes at room temperature, followed by visualization with the Bond Polymer Refine Red Kit (Leica). The specimens were counter-stained with hematoxylin. Slide fixation was performed with mounting medium and observation under optical microscope (Leica DM750) equipped with Leica ICC50W camera (Leica).
Statistical analyses
The nSolver software v3.0 was used to normalize the number of transcript copies with the geometric mean of 12 housekeeping genes. Log2-fold changes in gene expression were calculated comparing gene expression of samples from non-responder with responder patients. A patient was defined as responder in presence of a progression-free survival (PFS) ≥6 months. Differential expression analysis was performed using nSolver Advanced Analysis software (version 4.0, NanoString Technologies, Seattle, Washington, US), using the Differential Expression module (DE) in default settings. A gene was defined differentially expressed in a significant way, if associated with a p-value <0.05. Volcano plot was generated using the EnhancedVolcano R package v1.8. Volcano plot displays each gene’s -log10(p-value) and log2 fold change with the selected covariate. Highly statistically significant genes fall at the top of the plot above the horizontal lines, and highly differentially expressed genes fall to either side. Horizontal lines indicate various False Discovery Rate (FDR) thresholds or p-value thresholds if there is no adjustment to the p-values. Genes are colored if the resulting p-value is below the given FDR or p-value threshold.
Concerning the analysis of RNA ISH and IHC data, to compare expression levels between the two patient groups, an expert pathologist blinded to the treatment groups evaluated the staining intensity and the percentage of positive cells. Score 1: <25% positive cells, mild intensity staining; score 2: 25%< positive cells<50%, mild intensity staining; score 3: 50%<positive cells<75% strong intensity staining; score 4: positive cells >75%, strong intensity staining. Chi square Test was applied to calculate p value for ISH analysis: Wilcoxon rank-sum test was performed to compare the percentage of p16 positive IHC expression in responder vs. non-responder patients and calculate p-value.
Bioinformatic analyses
We analyzed the genomic data that had been generated for the TOMAS study, to match the RNA expression data, looking for any genetic alteration that could affect DDRR gene function. We broadened our analysis to all known DDRR and related genes and looked both into point mutations and copy number variation alterations.
Analysis of genomic and transcriptomic data of 255 primary sarcoma samples from The Cancer Genome Atlas (TCGA) was performed using CBioPortal v3.6.17 (37), considering the dataset named “Sarcoma (TCGA, PanCancer Atlas)”. 249 soft tissue sarcoma samples were considered for survival analysis, excluding samples lacking complete genomic and expression data, and desmoid tumor samples. A gene was considered altered in a tumor sample if associated with a somatic mutation, a gene copy number alteration, or associated with an expression level higher/lower than two standard deviations (|z-score| > 2) with respect to the mean expression measured in diploid samples. CBioPortal was used also to visualize data of the TCGA sarcoma cohort, for retrieving patient clinical information, gene expression and CNV data. Survival analysis was performed with survival v3.2.13 and survminer v0.4.9 R packages.
Results
Patients’ demographics and analysis of DDRR gene mutations
The characteristics of patients eligible for the analyses are described in Table 1. 32 patients were included in the analysis. Bone sarcomas were a small fraction of the cohort, with only two cases of Ewing’s sarcoma (6%) and one osteoblastic osteosarcoma (3%). Concerning STS, the most prevalent histology was leiomyosarcoma (LMS; n=11), followed by synovial sarcoma (SS; n=5), liposarcomas (LPS; n=5), malignant peripheral nerve sheath tumors (MPNST; n=2) and undifferentiated pleomorphic sarcomas (UPS; n=2). The four remaining histotypes (grouped under the term “other”) included one malignant phyllodes tumor of the breast (MPT), one malignant myoepithelioma of the upper limb, one pleural solitary fibrous tumor and one myxofibrosarcoma of the limb.
Among both responder and non-responder patients, we filtered out all benign or uncertain variants and found a few damaging, pathogenic mutations in DDRR genes. Considering the responder patient cohort (Table 2), patient 10 (TOMAS-10), affected by metastatic uterine LMS, had one TP53 (pathogenic; ClinVar) and one (probably damaging; PolyPhen-2) ERCC2 mutation. Other two TP53 variants were detected in two LMS patients (one uterine and one retroperitoneal LMS); S215N being likely pathogenic/of unknown significance, and I195T being pathogenic, as described in ClinVar for both missense mutations. One “probably damaging” ERCC6 mutation (as predicted by PolyPhen2 tool, being of unknown significance in the ClinVar database) was present in the tumor sample of one uterine LMS patient, and one MLPS patient’s tumor harbored both one pathogenic (ClinVar) PTEN mutation, and one PIK3CA mutation (possibly damaging, according to PolyPhen-2). One probably damaging (PolyPhen-2) PIK3CA mutation was observed also in TOMAS-39 patient, affected by malignant phyllodes tumor (MPT).
Among non-responder patients (Table 3), TP53 mutations were found in two non-responder patients affected by metastatic uterine LMS (C242S and Y205D), both of uncertain pathogenicity according to ClinVar, but probably damaging according to PolyPhen-2. One mutation predicted as “damaging” on BRCA1 protein function (by Polyphen2; of uncertain significance according to ClinVar) was detected in patient TOMAS-29 (metastatic synovial sarcoma of the lower limb). Another patient affected by metastatic synovial sarcoma had a RAD51C missense mutation (pathogenic/likely pathogenic in ClinVar). Patient TOMAS-25 had three deleterious ARID1A indels, while patient TOMAS-26 tumor sample harbored a gain-of-function mutation in the ERBB2 gene, predicted as “possibly damaging” on protein function.
Gene copy number analysis was performed to identify differences among the two groups (Table 4). Dedifferentiated liposarcomas showed MDM2/CDK4 gene amplifications, as already detected by diagnostic cytogenetics (TOMAS-43, TOMAS-48; both responder patients). Significant differences in copy number gain in CDKN2A gene (evaluated in comparison to housekeeping genes) was reported for responder in comparison to non-responder patients (paired Student’s t-test; p=0.038). MYC gene amplification was detected in one responder patient affected by malignant myoepithelioma, and MYC amplification associated with HEY1 amplification (possibly due to close chromosomal location) was identified in patient TOMAS-34 (non-responder patient affected by uterine LMS). Finally, ERBB2 amplification was observed in one case of non-responder UPS of the limb.
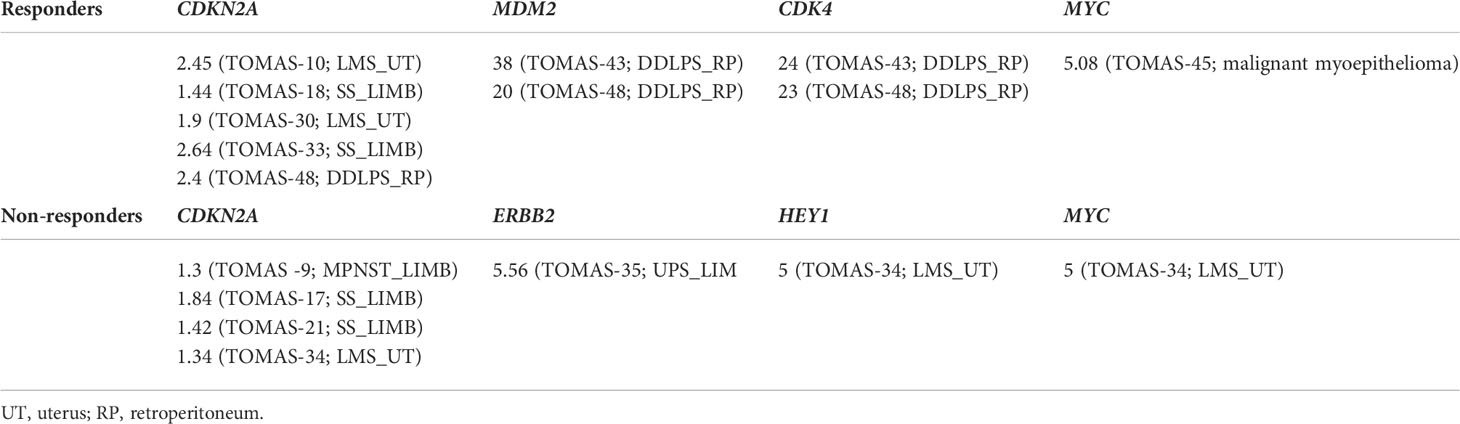
Table 4 Copy number differences in DDRR and related genes among responder and non-responder patients.
Differential expression of DDRR genes among responder and non-responder patients
Thirty-two RNA samples were extracted from FFPE archival tumor tissue from patients subsequently treated with trabectedin and olaparib combination. Significant differential expression levels of DDRR genes were found between the group of 16 responders (PFS ≥ 6 months), and that of 16 non-responders (PFS < 6 months). In detail, the expression of CDKN2A, PIK3R1, SLFN11, ATM, (and POLK) were significantly higher in responders; whilst APEX2, BLM, XRCC2, MAD2L2, and KRAS were significantly higher in non-responders (Figures 1A, B).
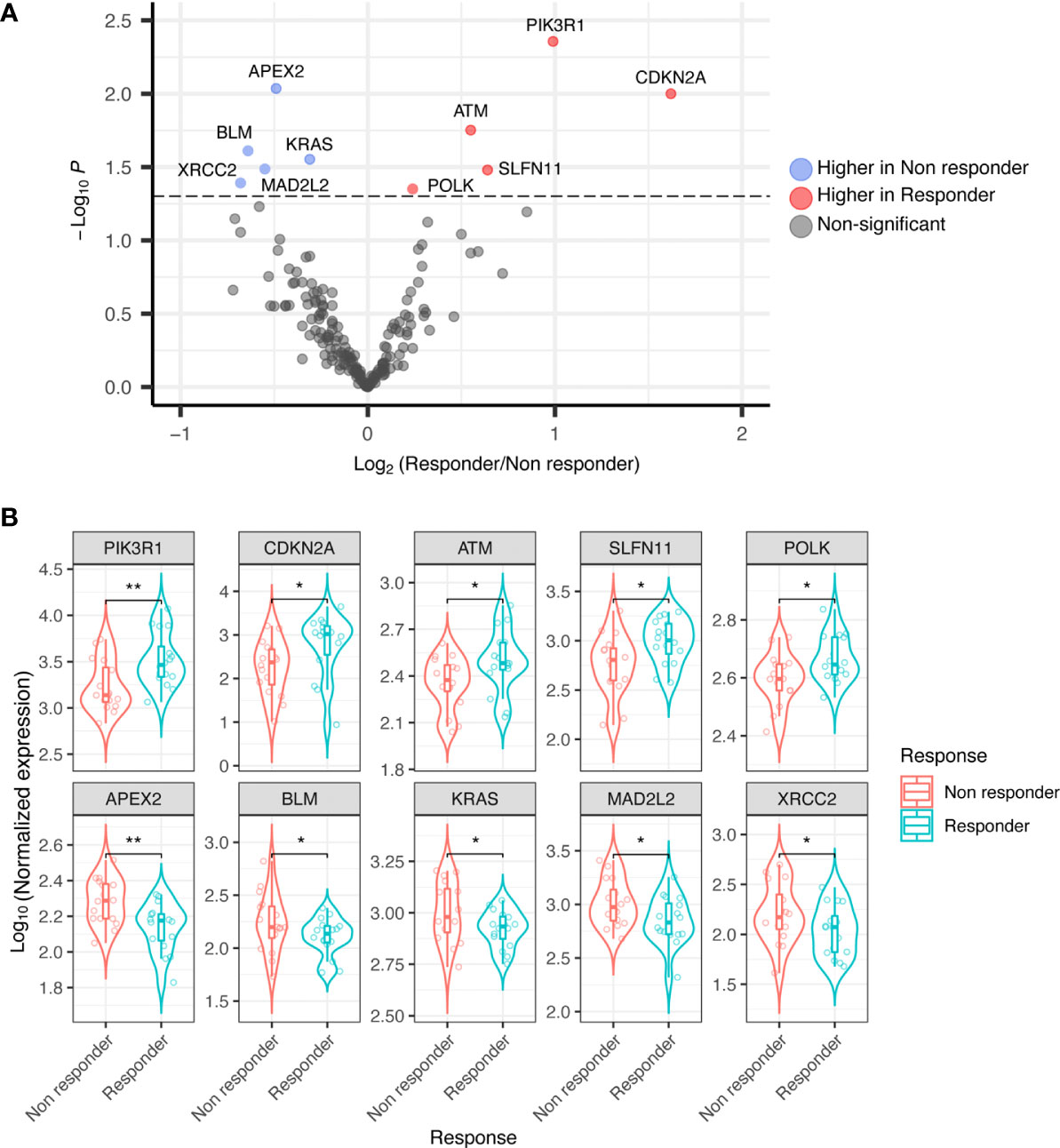
Figure 1 (A) Volcano plot showing differential expression of DDRR genes in responder vs. non-responder patients. (B) Boxplot showing differential expression of DDRR genes in responder vs. non-responder patients, with normalized expression. P-value by Wilcoxon Rank-Sum test. *p<0.05; **p<0.01.
Validation of biomarker expression by RNA-ISH, qRT-PCR and IHC
Differential expression of selected candidate biomarkers and their exact subcellular and tissue localizations were analyzed by RNA-ISH. Specific probes for CDKN2A, PIK3R1, SLFN11, and ATM were more hybridized in tissue slices from tumors of responder patients than in those samples derived from non-responder patients (Figure 2A). APEX2, BLM, XRCC2, and MAD2L2 were less hybridized in tissue slices from responder patient-derived tumors than in those ones from non-responder patients (Figure 2B). A heatmap was generated based on the ISH scores, displaying the differential expression of the eight identified genes among the two patient groups (Figure 2C).
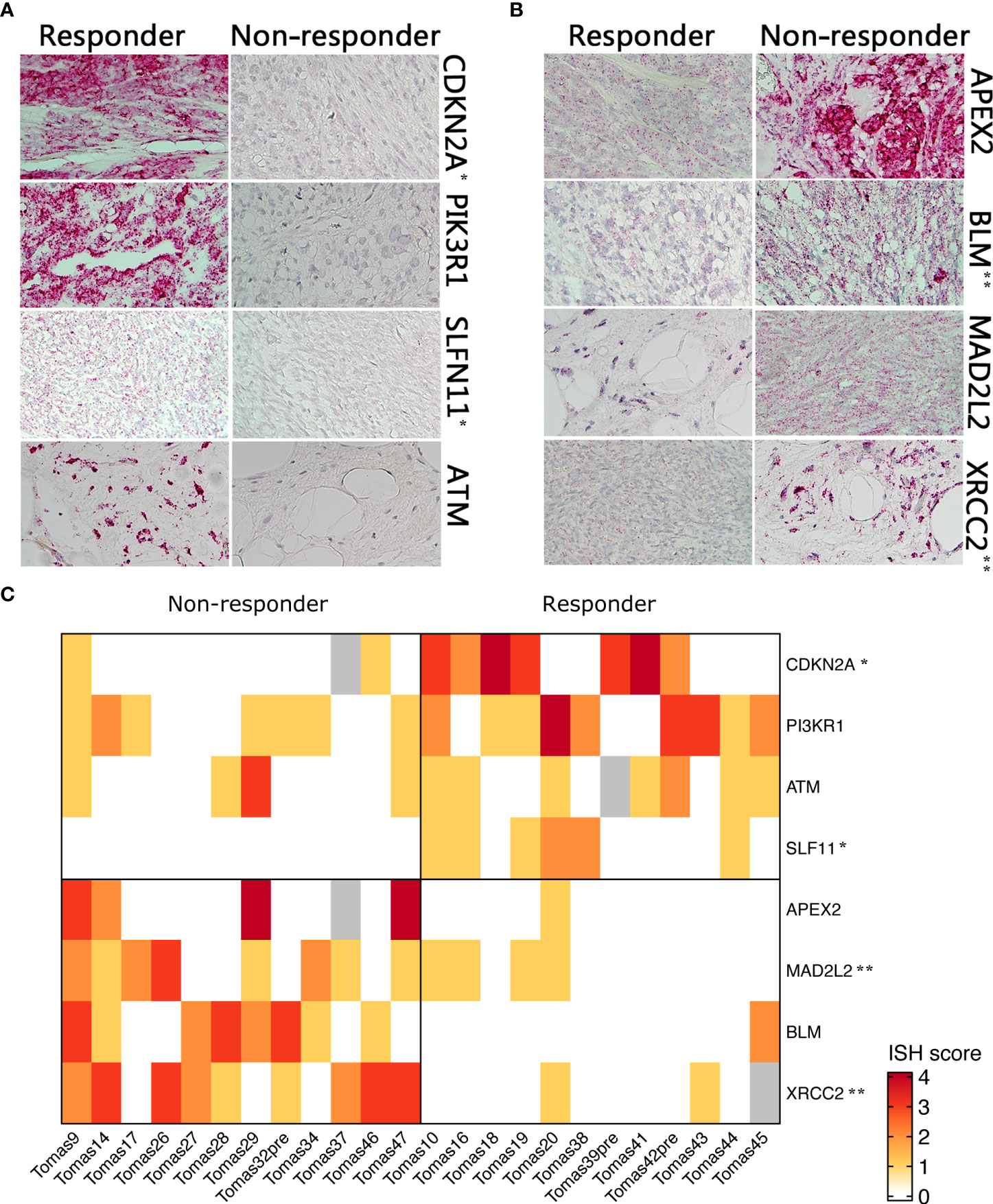
Figure 2 RNA ISH of selected genes in responder vs. non-responder patients. (A) Higher expressed genes in responder patients (B) higher expressed genes in non-responders (C). Heatmap showing differential RNA ISH staining between responder and non-responder patients. ISH score was assigned by an expert pathologist on the basis of staining intensity and percentage of positive cells. P-value was calculated by Chi-square test. *p<0.05; **p<0.01.
The expression levels were further confirmed by qRT-PCR (Figure 3A) and also at the protein level in terms of IHC expression for CDKN2A/p16, where a significant difference was detected between responders and non-responders (p=0.041; Figures 3B, C).
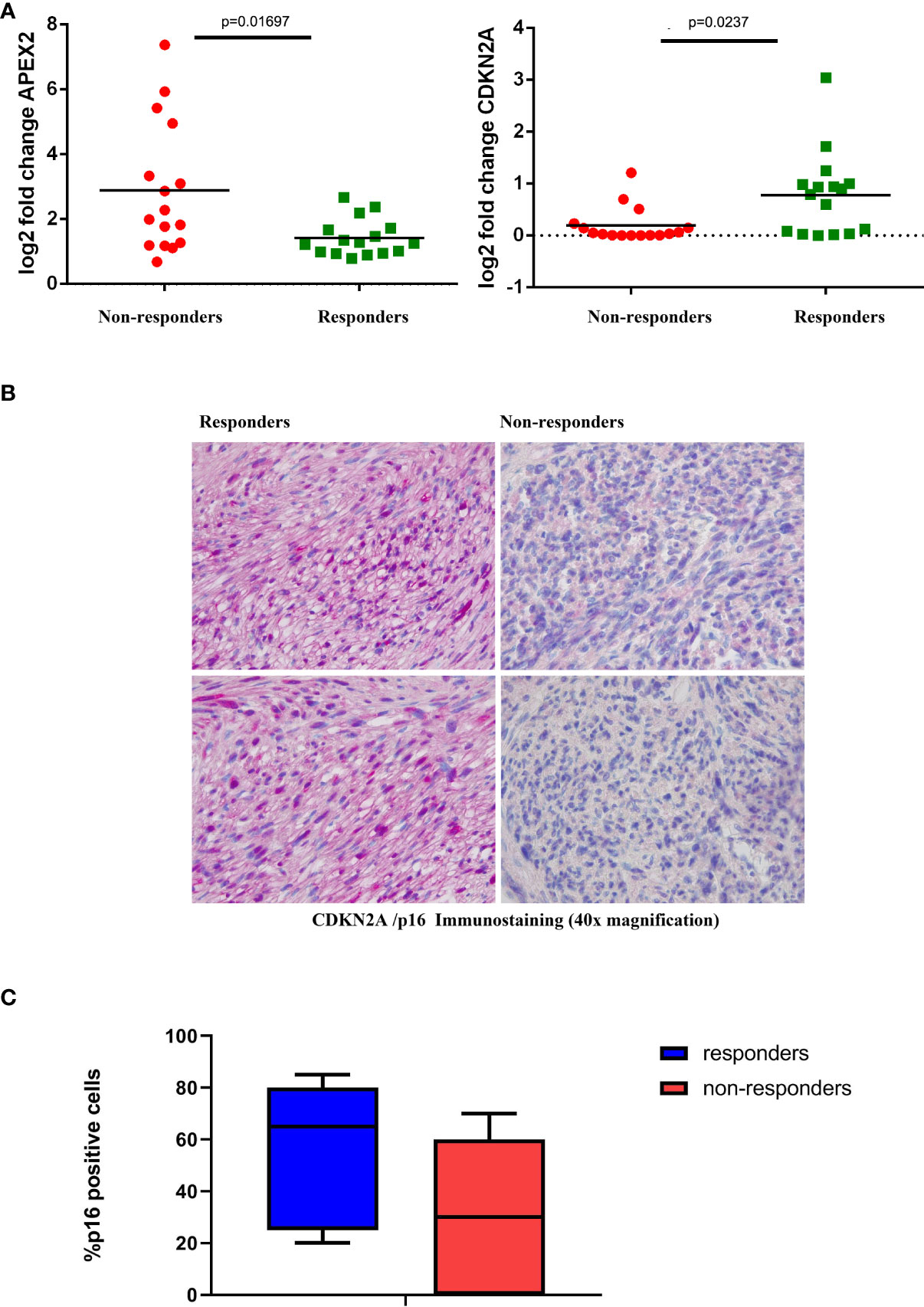
Figure 3 Validation Assays (A) Expression levels of representative genes (CDKN2A, left; and APEX2, right) among responder and non-responder patients. Statistically significant differential expression was shown between the two groups (Wilcoxon rank-sum test). (B) Representative IHC staining of CDKN2A/p16 in tumor samples from responder and non-responder patients. (C) Box plot distribution of CDKN2a/p16 expression level (percentage of IHC positive cells) in responders and non-responders patients.
Correlation of candidate biomarker gene expression levels and overall survival in TCGA sarcoma cohort
The sarcoma dataset was derived from genomic and expression analysis of 255 sarcoma samples from the TCGA sarcoma cohort. 249 samples were selected, being the ones with all data of interest available, and excluding desmoid tumors from the dataset, given their peculiar clinical-pathological behavior (37). The gene characterized by the highest number of genomic or transcriptomic alterations (Figure 4) was CDKN2A (altered in 19% of patients), followed by BLM (altered in 13% of patients), and MAD2L2 (altered in 12% of patients). The most frequent CDKN2A alteration was homo-deletion (n=38, 15% of patients).
We subsequently focused on differences in expression levels of the eight identified candidate genes in the sarcoma cohort of TCGA dataset, to look for any correlation with survival outcomes. We found a significant relation between MAD2L2 (Log-rank; p=0.0017) and BLM (Log-rank; p=0.025) expression levels and OS (Figure 5). The expression levels of the other six genes were not significantly related to OS (Supplementary Figure 1).
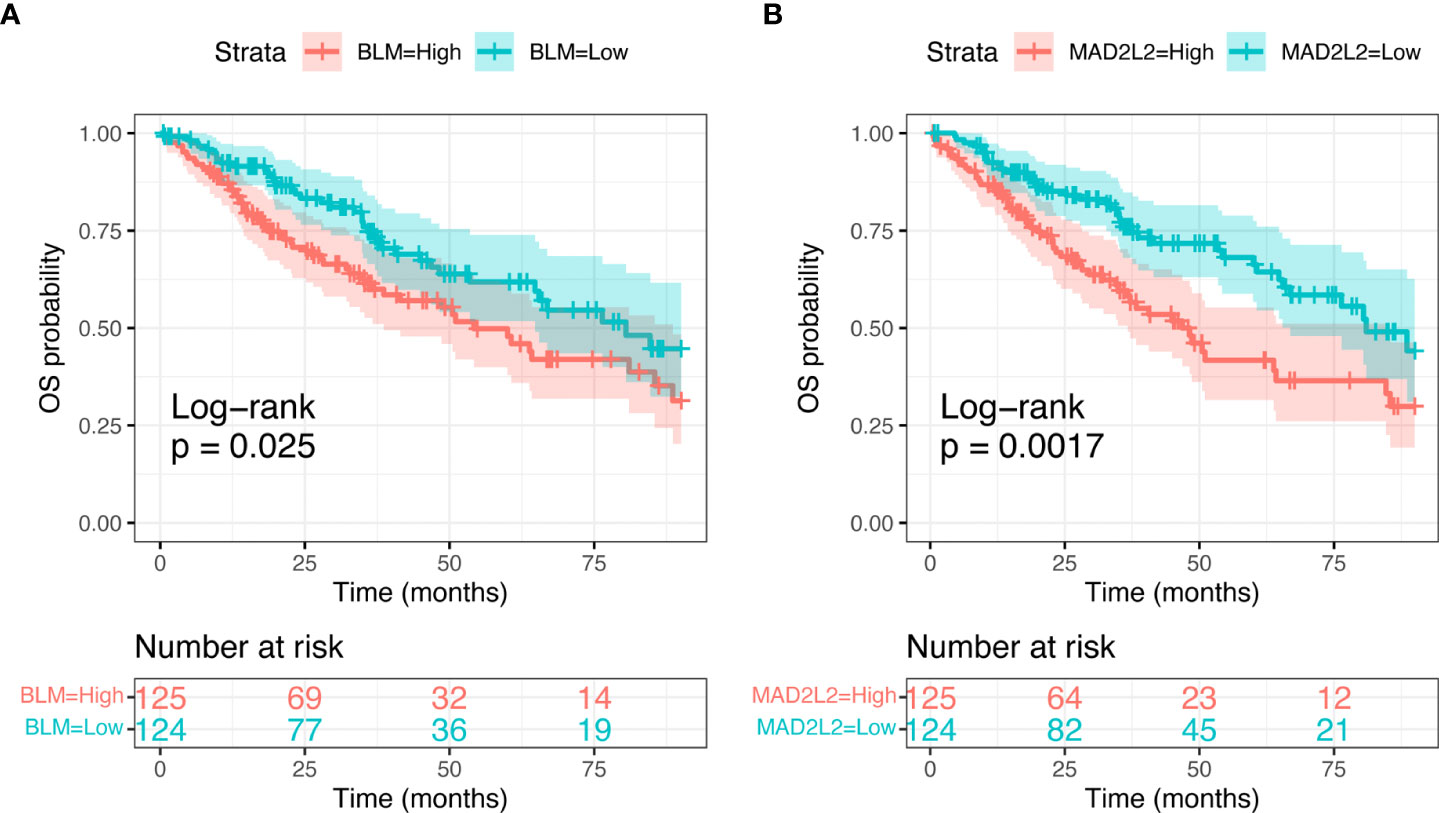
Figure 5 Kaplan-Meier curves showing Overall Sirvival in the sarcoma TCGA cohort, according to selected candidate biomarker genes (BLM, A, and MAD2L2, B).
Discussion
Our work has focused on a relevant translational research question stemming from the clinical results of the phase Ib TOMAS trial (13), asking whether there might be any way to predict response to trabectedin+olaparib treatment in BSTS patients. Of course, the answer to this question is a multi-factorial, poly-genic one, especially considering the low prevalence of BRCA1/2 defects in BSTS (38). Indeed, we identified few differentially expressed DDRR genes, which could provide the basis for a “personalized-medicine” approach to sarcoma treatment with this combination.
We analyzed WES and targeted-panel NGS data of patients from the TOMAS study, for whom RNA expression data were also available (32 patients), looking for any mutation, indel or CNV in DDRR genes, to integrate the expression signature with any mutational input which would not modify expression levels, but could alter gene function as well. Indeed, loss of function gene mutations might have the same effect of reduced gene expression for a given gene, while gain of function could correspond to gene overexpression. Indeed, we observed some relevant DDRR genes mutations, indels and CNVs in both responder and non-responder patients.
We then moved to expression profile analysis and identified a difference in terms of DDRR gene expression between 16 responder and 16 non-responder patients from the phase Ib TOMAS trial. We found an 8-gene signature of differentially expressed DDRR genes which significantly correlates with better outcome in patients treated with trabectedin+olaparib combination, separating our patient population in two groups according to PFS (longer or shorter than 6 months). The differential gene expression data were corroborated with ISH data, confirming expression at the sub-cellular level with RNA in situ hybridization technique, and also at the protein level (e.g. CDKN2A/p16). Our signature emerged from a broad DDRR panel, including 180 genes.
CDKN2A, PIK3R1, SLFN11, ATM were characterized by significantly higher expression levels in responder patients; APEX2, BLM, XRCC2, MAD2L2 displayed instead significantly higher expression levels in non-responder patients. Each gene deserves a separate discussion, being implicated in different aspects of DNA damage response and repair cellular machinery.
CDKN2A is a well-known tumor suppressor gene with a pivotal role in cell cycle control, slowing down G1 to S phase progression. It is involved in DDRR, and its low expression is also a negative prognostic factor across several tumor types (39–43). Similarly, low expression levels of PIK3R1, the gene encoding the regulatory subunit of PIK3CA (p85α), have been associated to poor prognosis, in particular in breast cancer (44). In the TCGA sarcoma cohort dataset, our analyses did not show any significant relationship between CDKN2A and PIK3R1 expression and survival, suggesting that these two genes are unlikely prognostic factors in STS and potentially might be involved in response to the treatment in this case series.
Considering SLFN11, ATM, APEX2, BLM, XRCC2 and MAD2L2 expression, a functional role in response to trabectedin+olaparib treatment might be hypothesized based on their biological roles. SLFN11 and ATM showed higher expression levels in responder patients. SLFN11 had already been associated to PARPi response (45). SLFN11 enhances cancer cell sensitivity to DNA-damaging agents (46), through a peculiar mechanism. Indeed, SLFN11 prevents the synthesis of proteins, which are crucial for cell survival upon significant extents of DNA damage. Namely, SLFN11 downregulates type II RNAs, inducing reduced translation of DDRR genes such as ATM and ATR (47). In this view, ATM higher expression in responder patients seems controversial, because it would lead to a more HR-proficient tumor cell profile in terms of DDRR response. APEX2 is a base-excision repair apurinic/apyrimidinic endonuclease (48). In multiple myeloma cells, it has been described as a key regulator of HR (49). Hence, lower APEX2 expression in responder patients is consistent with HR impairment and better response to trabectedin+olaparib response. What is more, APEX2 has been described as synthetic lethal in cells bearing BRCA2 defects (50). Considering BLM, its role in HR is well-known, both for initiation of HR upon DSBs and for Holliday junction dissolution at the end of the repair process (51). Hence, its lower expression in responder patients could have a direct implication driving sarcoma cells towards a HR-deficient phenotype. XRCC2 is also involved in DSBs repair by HR (52). In our patient population, expression was consistently higher in non-responder patients compared to responders. Given the relevance of HR deficiency for both olaparib and trabectedin mechanism of action, XRCC2 role in resistance to trabectedin+olaparib treatment could be at least partially explained. MAD2L2, instead, has a more prominent role in NHEJ (53). As anticipated, NHEJ does not affect trabectedin efficacy in a relevant way. Theoretically, proficient NHEJ might influence PARPi action, supporting our observation that responder patients show lower levels of MAD2L2 expression. Both XRCC2 and MAD2L2 higher expression was associated with worse survival in the TCGA sarcoma cohort.
Looking for potential DDRR gene function differences, which might not be reflected in expression levels, a few noteworthy mutations have emerged from analysis of WES and targeted-panel NGS data from the TOMAS phase Ib study. Indeed, apart from the expected TP53 mutations, which we detected in our LMS patients, we observed one ERCC2 mutation in one responder patient (Tables 2, 3), who displayed a mutation resulting in a G615W amino acid substitution, predicted as “probably damaging” (PolyPhen2 score of 1). ERCC2 is involved in TC-NER, so a loss of function mutation could represent a “resistance mechanism” to trabectedin. This mutation might have represented our patient’s Achilles’ heel to maintain a sustained response (PFS=10 months). One responder affected by a metastatic myxoid liposarcoma of the lower limb carried a mutation resulting in the R173C PTEN amino acid substitution (TOMAS-38), which is a loss of function mutation. Indeed, PTEN mutations have been described as synthetic lethal with PARPi (54). Among DDRR genes, we also included ERBB2 for its potential effects in DNA damage and repair pathways. We found ERBB2 gene amplification in one patient affected by metastatic UPS of the lower limb, and one gain of function point mutation resulting in the amino acid substitution R678W in a patient affected by metastatic MPNST of the lower limb. ERBB2 amplification has already been reported in UPS (55), as well as ERBB2 gain of function in MPNST (56). The specific R678W substitution, falling into ERBB2 transmembrane domain, confers significant cell survival advantage with respect to wild-type ERBB2 (57). Intriguingly, it has been found that ERBB2 expression affects the repair of specific DNA lesions produced by chemotherapy, linking ERBB2 to the DNA damage and repair response (58).
In conclusion, the response of BSTS patients to trabectedin and olaparib combination correlates with the expression of DDRR genes. CDKN2A, PIK3R1, SLFN11, ATM and APEX2, BLM, XRCC2, MAD2L2 differential expression discriminates responder and non-responder patients. The predictive role of these potential biomarkers warrants further investigation; we will explore this gene signature within data derived from our ongoing TOMAS-2 multicentric, randomized, phase II study.
Data availability statement
The original contributions presented in the study are included in the article/Supplementary Material. Further inquiries can be directed to the corresponding authors.
Ethics statement
The studies involving human participants were reviewed and approved by Institutional review board - Candiolo Cancer Institute, str. prov 142 km 3.95 Candiolo, Italy. The patients/participants provided their written informed consent to participate in this study.
Author contributions
Conceptualization: GGr, YP; Methodology: MLC, GF, UM, EB, YP, GCr; Formal Analysis: MLC, UM, EB, GF, YP, CI, CL, AP, IS; Investigation: AM, MLC, AP, GCh, EM, GGi, SB, LDA, MS, APDT; Visualization: CL, UM, EB, GF, YP; Writing - Original Draft Preparation, AM, YP; Writing - Review & Editing, AM, DS, GGr, GF, YP, MA, DS; Supervision, YP, GGr; Project Administration, YP, GGr; Funding Acquisition, GGr, DS, YP, AB, LDA. All authors have read and agreed to the published version of the manuscript.
Funding
This work was supported by RC 2019 Ministero della Salute; AIRC IG 23104 to GGr, Alleanza contro il cancro-working group Sarcomi, Ricerca Corrente-Reti 2021 RCR 2021 WP8 to YP; FPRC 5x1000 Ministero della Salute 2015 ImGen to GGr and to DS, FPRC 5xmille MIUR 2014 to GGr.; Fondazione per la ricerca sui tumori dell'apparato muscoloscheletrico e rari ONLUS CRT RF = 2016 -0917; AIRC IG 20259 to DS; Ministero della Salute-Ricerca Finalizzata- Giovani Ricercatori GR-2016-02362726 to YP; AIRC 5 per Mille 2018 - ID. 21091 to AB; AIRC IG 2018 - ID.21923 to AB; MIUR BiLiGeCT - Progetto PON ARS01_00492 to AB; EB was the recipient of a PhD fellowship from Department of Medical Sciences, University of Torino (“Dipartimenti di Eccellenza 2018-2022”, Project no. D15D18000410001), F.C. was supported by AIRC fellowship “Volontari Comitato Abruzzo-Molise” Rif. 21173.
Acknowledgments
TOMAS 1b clinical trial is an Italian Sarcoma Group study. The results shown here are in part based upon data generated by the TCGA research network: https://www.cancer.gov/tcga.
Conflict of interest
GGr has received fees for consulting/advisory roles from PharmaMar, Lilly, Novartis, Bayer, and Eisai. LDA received travel grant from PharmaMar and Lilly. MA has received fees for consulting/advisory roles from Bristol- Myers Squibb, Merck, and Roche. AB served in a consulting/advisory role for Illumina and Inivata. AB is cofounder and shareholder of NeoPhore. AB is a member of the NeoPhore scientific advisory board.
The remaining authors declare that the research was conducted in the absence of any commercial or financial relationships that could be construed as a potential conflict of interest.
Publisher’s note
All claims expressed in this article are solely those of the authors and do not necessarily represent those of their affiliated organizations, or those of the publisher, the editors and the reviewers. Any product that may be evaluated in this article, or claim that may be made by its manufacturer, is not guaranteed or endorsed by the publisher.
Supplementary material
The Supplementary Material for this article can be found online at: https://www.frontiersin.org/articles/10.3389/fonc.2022.844250/full#supplementary-material
References
1. Gronchi A, et al. Soft tissue and visceral sarcomas: ESMO–EURACAN–GENTURIS clinical practice guidelines for diagnosis, treatment and follow-up☆. Ann Oncol (2021) 32(11):1348–65. doi: 10.1016/j.annonc.2021.07.006
2. Demetri GD, et al. Efficacy and safety of trabectedin or dacarbazine for metastatic liposarcoma or leiomyosarcoma after failure of conventional chemotherapy: Results of a phase III randomized multicenter clinical trial. J Clin Oncol (2016) 34(8):786–93. doi: 10.1200/JCO.2015.62.4734
3. Schöffski P, et al. Eribulin versus dacarbazine in previously treated patients with advanced liposarcoma or leiomyosarcoma: A randomised, open-label, multicentre, phase 3 trial. Lancet (2016) 387(10028):1629–37. doi: 10.1016/S0140-6736(15)01283-0
4. Judson I, et al. Randomised phase II trial of pegylated liposomal doxorubicin (DOXIL/CAELYX) versus doxorubicin in the treatment of advanced or metastatic soft tissue sarcoma: a study by the EORTC soft tissue and bone sarcoma group. Eur J Cancer (2001) 37(7):870–7. doi: 10.1016/S0959-8049(01)00050-8
5. van der Graaf WT, et al. Pazopanib for metastatic soft-tissue sarcoma (PALETTE): A randomised, double-blind, placebo-controlled phase 3 trial. Lancet (2012) 379(9829):1879–86. doi: 10.1016/S0140-6736(12)60651-5
6. Farag S, Smith MJ, Fotiadis N, Constantinidou A, Jones RL. Revolutions in treatment options in gastrointestinal stromal tumours (GISTs): The latest updates: Revolutions in treatment options in GIST. Curr Treat Options Oncol (2020) 21:1–11. doi: 10.1007/s11864-020-00754-8
7. Hao Z, Wang P. Lenvatinib in management of solid tumors. Oncologist (2020) 25(2):e302–10. doi: 10.1634/theoncologist.2019-0407
8. D'Ambrosio L, et al. Doxorubicin plus dacarbazine, doxorubicin plus ifosfamide, or doxorubicin alone as a first-line treatment for advanced leiomyosarcoma: A propensity score matching analysis from the European organization for research and treatment of cancer soft tissue and bone sarcoma group. Cancer (2020) 126(11):2637–47. doi: 10.1002/cncr.32795
9. Pautier P, et al. Trabectedin in combination with doxorubicin for first-line treatment of advanced uterine or soft-tissue leiomyosarcoma (LMS-02): a non-randomised, multicentre, phase 2 trial. Lancet Oncol (2015) 16(4):457–64. doi: 10.1016/S1470-2045(15)70070-7
10. Müller D, et al. 1669TiP a non-randomized, open-label phase II trial evaluating efficacy and feasibility of combined treatment with trabectedin and nivolumab in patients with metastatic or inoperable soft tissue sarcomas (STS) after failure of an anthracycline-containing regimen. Ann Oncol (2020) 31:S991.doi: 10.1016/annonc/annonc288
11. Chae YK, et al. Path toward precision oncology: Review of targeted therapy studies and tools to aid in defining "Actionability" of a molecular lesion and patient management support. Mol Cancer Ther (2017) 16(12):2645–55. doi: 10.1158/1535-7163.MCT-17-0597
12. Pignochino Y, et al. PARP1 expression drives the synergistic antitumor activity of trabectedin and PARP1 inhibitors in sarcoma preclinical models. Mol Cancer (2017) 16(1):1–15. doi: 10.1186/s12943-017-0652-5
13. Grignani G, et al. Trabectedin and olaparib in patients with advanced and non-resectable bone and soft-tissue sarcomas (TOMAS): an open-label, phase 1b study from the Italian sarcoma group. Lancet Oncol (2018) 19(10):1360–71. doi: 10.1016/S1470-2045(18)30438-8
14. Moura DS, et al. A DNA damage repair gene-associated signature predicts responses of patients with advanced soft-tissue sarcoma to treatment with trabectedin. Mol Oncol (2021) 15(12):3691–705. doi: 10.1002/1878-0261.12996
15. Italiano A, et al. ERCC5/XPG, ERCC1, and BRCA1 gene status and clinical benefit of trabectedin in patients with soft tissue sarcoma. Cancer (2011) 117(15):3445–56. doi: 10.1002/cncr.25925
16. Massuti B, et al. Trabectedin in patients with advanced non-small-cell lung cancer (NSCLC) with XPG and/or ERCC1 overexpression and BRCA1 underexpression and pretreated with platinum. Lung Cancer (2012) 76(3):354–61. doi: 10.1016/j.lungcan.2011.12.002
17. Schöffski P, et al. Predictive impact of DNA repair functionality on clinical outcome of advanced sarcoma patients treated with trabectedin: A retrospective multicentric study. Eur J Cancer (2011) 47(7):1006–12. doi: 10.1016/j.ejca.2011.01.016
18. Moura DS, et al. CUL4A, ERCC5, and ERCC1 as predictive factors for trabectedin efficacy in advanced soft tissue sarcomas (STS): A Spanish group for sarcoma research (GEIS) study. Cancers (2020) 12(5):1128. doi: 10.3390/cancers12051128
19. Pilié PG, Gay CM, Byers LA, O'Connor MJ, Yap TA. PARP inhibitors: extending benefit beyond BRCA-mutant cancers. Clin Cancer Res (2019) 25(13):3759–71. doi: 10.1158/1078-0432.CCR-18-0968
20. Cardnell RJ, et al. Proteomic markers of DNA repair and PI3K pathway activation predict response to the PARP inhibitor BMN 673 in small cell lung cancer. Clin Cancer Res (2013) 19(22):6322–8. doi: 10.1158/1078-0432.CCR-13-1975
21. Michels J, Vitale I, Saparbaev M, Castedo M, Kroemer G. Predictive biomarkers for cancer therapy with PARP inhibitors. Oncogene (2014) 33(30):3894–907. doi: 10.1038/onc.2013.352
22. Mateo J, et al. DNA-Repair defects and olaparib in metastatic prostate cancer. N Engl J Med (2015) 373(18):1697–708. doi: 10.1056/NEJMoa1506859
23. Thomas A, Murai J, Pommier Y. The evolving landscape of predictive biomarkers of response to PARP inhibitors. J Clin Invest (2018) 128(5):1727–30. doi: 10.1172/JCI120388
24. Miller RE, et al. ESMO recommendations on predictive biomarker testing for homologous recombination deficiency and PARP inhibitor benefit in ovarian cancer. Ann Oncol (2020) 31(12):1606–22. doi: 10.1016/j.annonc.2020.08.2102
25. Larsen AK, Galmarini CM, D'Incalci M. Unique features of trabectedin mechanism of action. Cancer Chemother Pharmacol (2016) 77(4):663–71. doi: 10.1007/s00280-015-2918-1
26. D'Incalci M, Galmarini CM. A review of trabectedin (ET-743): a unique mechanism of action. Mol Cancer Ther (2010) 9(8):2157–63. doi: 10.1158/1535-7163.MCT-10-0263
27. Aune GJ, et al. Von Hippel-Lindau–coupled and transcription-coupled nucleotide excision repair–dependent degradation of RNA polymerase II in response to trabectedin. Clin Cancer Res (2008) 14(20):6449–55. doi: 10.1158/1078-0432.CCR-08-0730
28. Tavecchio M, et al. Role of homologous recombination in trabectedin-induced DNA damage. Eur J Cancer (2008) 44(4):609–18. doi: 10.1016/j.ejca.2008.01.003
29. Grignani G, Merlini A, Sangiolo D, D'Ambrosio L, Pignochino Y. Delving into PARP inhibition from bench to bedside and back. Pharmacol Ther (2020) 206:107446. doi: 10.1016/j.pharmthera.2019.107446
30. Kamel D, Gray C, Walia JS, Kumar V. PARP inhibitor drugs in the treatment of breast, ovarian, prostate and pancreatic cancers: an update of clinical trials. Curr Drug Targets (2018) 19(1):21–37. doi: 10.2174/1389450118666170711151518
31. Patel AG, Sarkaria JN, Kaufmann SH. Nonhomologous end joining drives poly (ADP-ribose) polymerase (PARP) inhibitor lethality in homologous recombination-deficient cells. Proc Natl Acad Sci (2011) 108(8):3406–11. doi: 10.1073/pnas.1013715108
32. Corti G, et al. A genomic analysis workflow for colorectal cancer precision oncology. Clin colorectal Cancer (2019) 18(2):91–101. e103. doi: 10.1016/j.clcc.2019.02.008
33. Crisafulli G, et al. Whole exome sequencing analysis of urine trans-renal tumour DNA in metastatic colorectal cancer patients. ESMO Open (2019) 4(6):e000572. doi: 10.1136/esmoopen-2019-000572
34. Adzhubei IA, et al. A method and server for predicting damaging missense mutations. Nat Methods (2010) 7(4):248–9. doi: 10.1038/nmeth0410-248
35. Van Emburgh BO, et al. Acquired RAS or EGFR mutations and duration of response to EGFR blockade in colorectal cancer. Nat Commun (2016) 7:13665–5. doi: 10.1038/ncomms13665
36. Wang F, et al. RNAscope: a novel in situ RNA analysis platform for formalin-fixed, paraffin-embedded tissues. J Mol diagnostics (2012) 14(1):22–9. doi: 10.1016/j.jmoldx.2011.08.002
37. Gao J, et al. Integrative analysis of complex cancer genomics and clinical profiles using the cBioPortal. Sci Signal (2013) 6(269):pl1. doi: 10.1126/scisignal.2004088
38. Gounder MM, et al. Impact of next-generation sequencing (NGS) on diagnostic and therapeutic options in soft-tissue and bone sarcoma. J Clin Oncol (2017) 35(15):11001–1100139. doi: 10.1200/JCO.2017.35.15_suppl.11001
39. Zhao R, Choi BY, Lee M-H, Bode AM, Dong Z. Implications of genetic and epigenetic alterations of CDKN2A (p16INK4a) in cancer. EBioMedicine (2016) 8:30–9. doi: 10.1016/j.ebiom.2016.04.017
40. Monzon J, et al. CDKN2A mutations in multiple primary melanomas. New Engl J Med (1998) 338(13):879–87. doi: 10.1056/NEJM199803263381305
41. Lu VM, et al. The prognostic significance of CDKN2A homozygous deletion in IDH-mutant lower-grade glioma and glioblastoma: a systematic review of the contemporary literature. J Neurooncol (2020) 148(2):221–9. doi: 10.1007/s11060-020-03528-2
42. Foulkes WD, Flanders TY, Pollock PM, Hayward NK. The CDKN2A (p16) gene and human cancer. Mol Med (1997) 3(1):5–20. doi: 10.1007/BF03401664
43. Bui NQ, et al. A clinico-genomic analysis of soft tissue sarcoma patients reveals CDKN2A deletion as a biomarker for poor prognosis. Clin Sarcoma Res (2019) 9:12. doi: 10.1186/s13569-019-0122-5
44. Cizkova M, et al. PIK3R1 underexpression is an independent prognostic marker in breast cancer. BMC Cancer (2013) 13(1):1–15. doi: 10.1186/1471-2407-13-545
45. Lok BH, et al. PARP inhibitor activity correlates with SLFN11 expression and demonstrates synergy with temozolomide in small cell lung cancer. Clin Cancer Res (2017) 23(2):523–35. doi: 10.1158/1078-0432.CCR-16-1040
46. Zoppoli G, et al. Putative DNA/RNA helicase schlafen-11 (SLFN11) sensitizes cancer cells to DNA-damaging agents. Proc Natl Acad Sci (2012) 109(37):15030–5. doi: 10.1073/pnas.1205943109
47. Li M, et al. DNA Damage-induced cell death relies on SLFN11-dependent cleavage of distinct type II tRNAs. Nat Struct Mol Biol (2018) 25(11):1047–58. doi: 10.1038/s41594-018-0142-5
48. Jensen KA, Shi X, Yan S. Genomic alterations and abnormal expression of APE2 in multiple cancers. Sci Rep (2020) 10(1):1–11. doi: 10.1038/s41598-020-60656-5
49. Kumar S, et al. Role of apurinic/apyrimidinic nucleases in the regulation of homologous recombination in myeloma: mechanisms and translational significance. Blood Cancer J (2018) 8(10):1–10. doi: 10.1038/s41408-018-0129-9
50. Mengwasser KE, et al. Genetic screens reveal FEN1 and APEX2 as BRCA2 synthetic lethal targets. Mol Cell (2019) 73(5):885–99.e886. doi: 10.1016/j.molcel.2018.12.008
51. LaRocque JR, et al. Interhomolog recombination and loss of heterozygosity in wild-type and bloom syndrome helicase (BLM)-deficient mammalian cells. Proc Natl Acad Sci (2011) 108(29):11971–6. doi: 10.1073/pnas.1104421108
52. Johnson RD, Liu N, Jasin M. Mammalian XRCC2 promotes the repair of DNA double-strand breaks by homologous recombination. Nature (1999) 401(6751):397–9. doi: 10.1038/43932
53. Boersma V, et al. MAD2L2 controls DNA repair at telomeres and DNA breaks by inhibiting 5′ end resection. Nature (2015) 521(7553):537–40. doi: 10.1038/nature14216
54. Mendes-Pereira AM, et al. Synthetic lethal targeting of PTEN mutant cells with PARP inhibitors. EMBO Mol Med (2009) 1(6-7):315–22. doi: 10.1002/emmm.200900041
55. Becerikli M, et al. Numerical and structural chromosomal anomalies in undifferentiated pleomorphic sarcoma. Anticancer Res (2014) 34(12):7119–27.
56. Holtkamp N, et al. EGFR and erbB2 in malignant peripheral nerve sheath tumors and implications for targeted therapy. Neuro-oncology (2008) 10(6):946–57. doi: 10.1215/15228517-2008-053
57. Pahuja KB, et al. Actionable activating oncogenic ERBB2/HER2 transmembrane and juxtamembrane domain mutations. Cancer Cell (2018) 34(5):792–806. e795. doi: 10.1016/j.ccell.2018.09.010
Keywords: bone and soft tissue sarcomas, predictive biomarkers, DNA damage response and repair genes, trabectedin, olaparib
Citation: Merlini A, Centomo ML, Ferrero G, Chiabotto G, Miglio U, Berrino E, Giordano G, Brusco S, Pisacane A, Maldi E, Sarotto I, Capozzi F, Lano C, Isella C, Crisafulli G, Aglietta M, Dei Tos AP, Sbaraglia M, Sangiolo D, D’Ambrosio L, Bardelli A, Pignochino Y and Grignani G (2022) DNA damage response and repair genes in advanced bone and soft tissue sarcomas: An 8-gene signature as a candidate predictive biomarker of response to trabectedin and olaparib combination. Front. Oncol. 12:844250. doi: 10.3389/fonc.2022.844250
Received: 27 December 2021; Accepted: 26 July 2022;
Published: 30 August 2022.
Edited by:
Alberto Zambelli, Papa Giovanni XXIII Hospital, ItalyReviewed by:
Tomoki Nakamura, Mie University Hospital, JapanYoshiyuki Suehara, Juntendo University, Japan
Copyright © 2022 Merlini, Centomo, Ferrero, Chiabotto, Miglio, Berrino, Giordano, Brusco, Pisacane, Maldi, Sarotto, Capozzi, Lano, Isella, Crisafulli, Aglietta, Dei Tos, Sbaraglia, Sangiolo, D’Ambrosio, Bardelli, Pignochino and Grignani. This is an open-access article distributed under the terms of the Creative Commons Attribution License (CC BY). The use, distribution or reproduction in other forums is permitted, provided the original author(s) and the copyright owner(s) are credited and that the original publication in this journal is cited, in accordance with accepted academic practice. No use, distribution or reproduction is permitted which does not comply with these terms.
*Correspondence: Ymera Pignochino, eW1lcmEucGlnbm9jaGlub0B1bml0by5pdA==; Giovanni Grignani, Z2lvdmFubmkuZ3JpZ25hbmlAaXJjYy5pdA==
†These authors have contributed equally to this work and share first authorship
‡These authors have contributed equally to this work and share last authorship