- 1AMITA Health Saint Joseph Hospital Chicago, Chicago, IL, United States
- 2Department of Epidemiology and Health Statistics, School of Public Health, Anhui Medical University, Hefei, China
- 3Department of Clinical Medicine, School of the First Clinical Medicine, Anhui Medical University, Hefei, China
- 4Gastroenterology, AMITA Health Saint Joseph Hospital Chicago, Chicago, IL, United States
- 5Mercy Internal Medicine Service, Trinity Health of New England, Springfield, MA, United States
- 6Department of Diagnostic Radiology and Nuclear Medicine, Gunma University Graduate School of Medicine, Maebashi, Japan
- 7Department of Orthopedics, Shanghai General Hospital, Shanghai Jiao Tong University School of Medicine, Shanghai Jiao Tong University, Shanghai, China
- 8University of Maryland Medical Center Midtown Campus, Baltimore, MD, United States
- 9College of Medicine, The University of Arizona, Tucson, AZ, United States
- 10Banner-University Medical Center South, Tucson, AZ, United States
- 11Menzies Institute for Medical Research, University of Tasmania, Hobart, TAS, Australia
- 12Department of Radiation Oncology, Mayo Clinic, Rochester, MN, United States
- 13Department of Oncology, The First Affiliated Hospital of Anhui Medical University, Hefei, China
Background: Liver cancer (LC) is well known for its prevalence as well as its poor prognosis. The aberrant expression of lysyl oxidase (LOX) family is associated with liver cancer, but their function and prognostic value in LC remain largely unclear. This study aimed to explore the function and prognostic value of LOX family in LC through bioinformatics analysis and meta-analysis.
Results: The expression levels of all LOX family members were significantly increased in LC. Area under the receiver operating characteristic curve (AUC) of LOXL2 was 0.946 with positive predictive value (PPV) of 0.994. LOX and LOXL3 were correlated with worse prognosis. Meta-analysis also validated effect of LOX on prognosis. Nomogram of these two genes and other predictors was also plotted. There was insufficient data from original studies to conduct meta-analysis on LOXL3. The functions of LOX family members in LC were mostly involved in extracellular and functions and structures. The expressions of LOX family members strongly correlated with various immune infiltrating cells and immunomodulators in LC.
Conclusions: For LC patients, LOXL2 may be a potential diagnostic biomarker, while LOX and LOXL3 have potential prognostic and therapeutic values. Positive correlation between LOX family and infiltration of various immune cells and immunomodulators suggests the need for exploration of their roles in the tumor microenvironment and for potential immunotherapeutic to target LOX family proteins.
Background
Liver cancer (LC) is the sixth most common malignant tumor and the third leading cause of cancer-associated mortality worldwide. Hepatocellular carcinoma (HCC) accounts for 75%-85% of all LC, according to the GLOBOCAN 2020 estimation (1). In east Asia, especially China, a high incidence of HCC was noted, and similarly, the incidence and mortality of LC in developing countries are significantly higher than those in developed countries (2, 3). The variations in the prevalence of LC amongst different populations and regions are attributed to a variety of environmental and genetic factors, such as aflatoxin, alcohol, smoking, chronic hepatitis virus infection, and type 2 diabetes (4–6). Despite significant advances in diagnosis and treatment of LC, including surgical resection, local ablation, liver transplantation, and sorafenib–regorafenib sequential therapy, the prognosis of LC remains poor (6). Based on most recent data, 841,000 new cases and 782,000 deaths of LC around the globe was estimated to occur each year (1). Therefore, it is of great value to explore novel diagnostic and prognostic biomarkers that are sensitive and specific, and to identify potential targets for medications (7).
With the advent of next-generation sequencing (NGS) and other techniques, increasing amount of information has become available for a variety of cancer types and other diseases (8–11). Thus, the mechanisms of cancers, as well as other diseases, have become more widely investigated based on bioinformatic methods, by combining information technology and molecular biology. Bioinformatics methods, such as data-mining, are also widely applied for identification of potential biomarkers as therapeutic targets, as well as diagnostic and prognostic predictors, and to explore the pathogenesis of malignancies at the molecular level (12–14).
As an extracellular enzyme, lysyl oxidase (LOX) oxidatively deaminates specific lysine and hydroxylysine residues to form allysines in the telopeptide domains of the collagen molecule, and thus plays a critical role in covalent cross-link formation in collagen fibrils (15). LOX is highly expressed in tissues containing elastic fibers and fibrillar collagen, such as skin, lung, and the fibrous lamina propria in the small intestine, stomach, and liver (16). In addition to LOX, four LOX-like proteins (LOXL-1, -2, -3, and -4) have also been identified in the LOX family (17–19). Studies have found that the LOX family was involved in carcinogenesis and tumor metastasis, through angiogenesis promotion, formation of mature extracellular matrix at the secondary site, focal adhesion kinase (FAK) activation, and other mechanisms (19–22).
The lysyl oxidase (LOX) family consists of five members: LOX, the first described member of this family, and its four related members called lysyl oxidase-like genes (LOXL1-4). Recent evidence suggests that the LOX family play important roles in liver cancer. LOX secreted by HCC promotes tube formation of endothelial cells through upregulation of VEGF, and overexpressed LOX increases angiogenesis, whereas LOXL1 was found to be increased in liver fibrosis models (23–25). As for LOXL2, its expression level was found to be higher in HCC tissues compared with non-tumor tissue (26). Although LOXL3 has been studied in different types of cancer, studies on its roles in liver cancer are limited (25). LOXL4 was found to increase the risk of invasion and metastasis, promote angiogenesis, and play a role in the immunosuppressive microenvironment in HCC (25, 27, 28)
Although previous studies have investigated the roles of the LOX family in LC, their exact roles and mechanisms, especially for LOXL1 and LOXL3, have yet to be further investigated (25). Previous studies have shown evidence of the potential prognostic values and therapeutic values of LOX family members (25, 26). Thus, online databases were mined to analyze the expression, mutation, function, and immune infiltration of LOX family members in LC, with the goal to determine their potential oncogenic role, as well as their diagnostic and prognostic value in LC.
Results
Differential Expression Levels of LOX Family in LC
All five members of LOX family demonstrated higher expression in liver cancer tumor tissues than normal tissues (Figure 1A and Table 1). These findings were consistent with results from UALCAN, which confirmed that the expression of all LOX family members was statistically significantly higher in tumor tissue (Figure 1B), and from TIMER, which showed higher expression of LOX (P=1.5E-11), LOXL1 (P=2.39E-04), LOXL2 (P=4.02E-25), LOXL3 (P=1.53E-04), and LOXL4 (P=7.15-05). Further analysis of ROC curve showed that AUC of LOXL2 was 0.946 (95%CI:0.915-0.978, with positive predictive value (PPV) of 0.994 and a cutoff value of 1.050 (Figure 2).
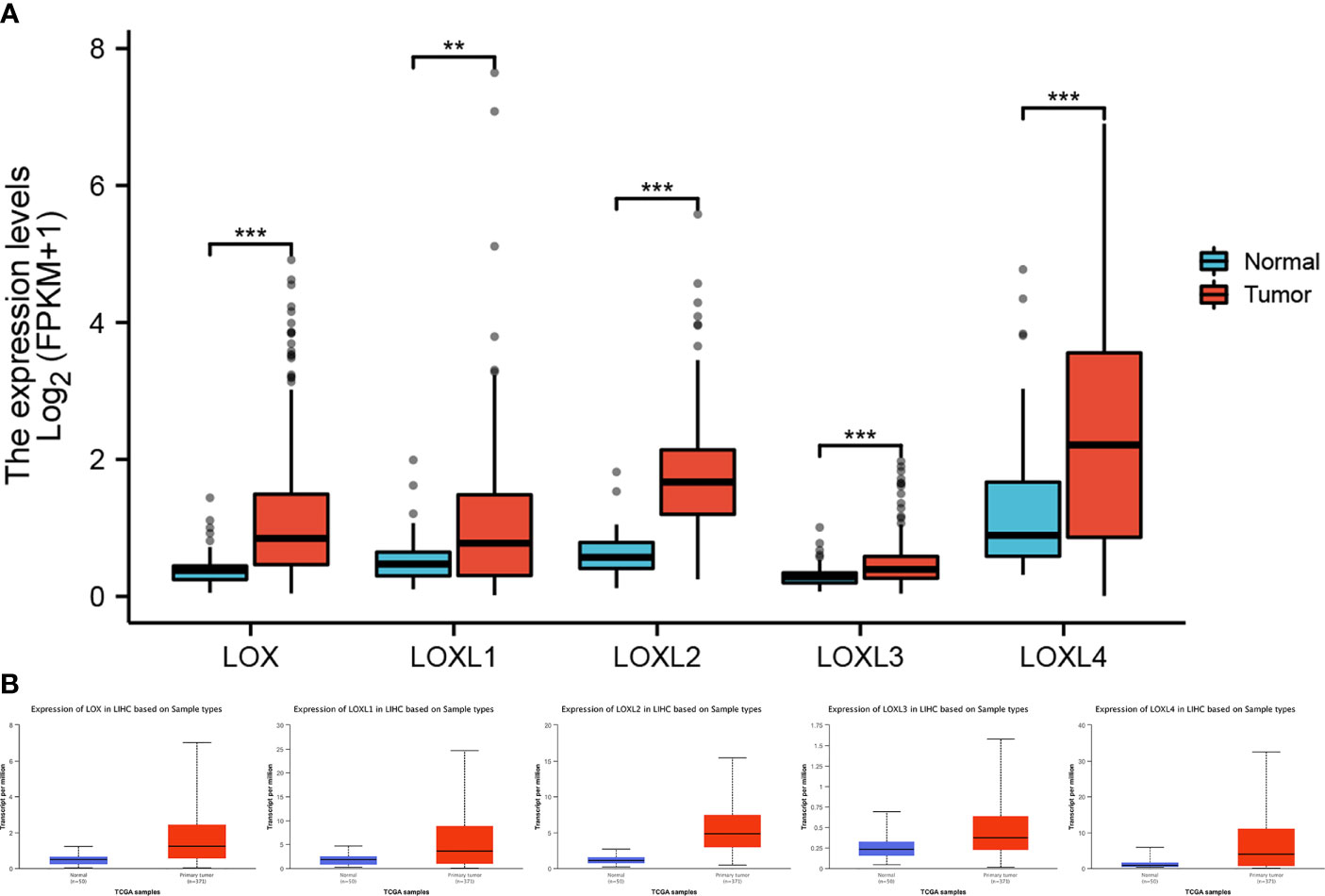
Figure 1 Expression level of LOX family members between normal tissue and tumor tissue in liver cancer. (A) analysis via R software, (B) analysis via ULCAN. ** means P < 0.01, *** means P < 0.001.

Table 1 Expression level of LOX family members between normal tissue and tumor tissue in liver cancer, and overall survival of overexpressing LOX family members in liver cancer.
Prognostic Value of LOX Family in LC
Evaluation of the value of differential expression of LOX family members in LC prognosis found that LOX, LOXL3, and LOXL4 were associated with poor overall survival (OS) (Figure 3A and Table 1). UALCAN was utilized for verification which found that only LOX (P=0.023) and LOXL3 (P=0.031) were associated with poor OS (Figure 3B). Further verification via TIMER also only identified poor prognosis of LOX (P=0.003) and LOXL3 (P=0.023) (Table 1). The combined results indicated that high expression of LOX and LOXL3 was associated with worse OS.
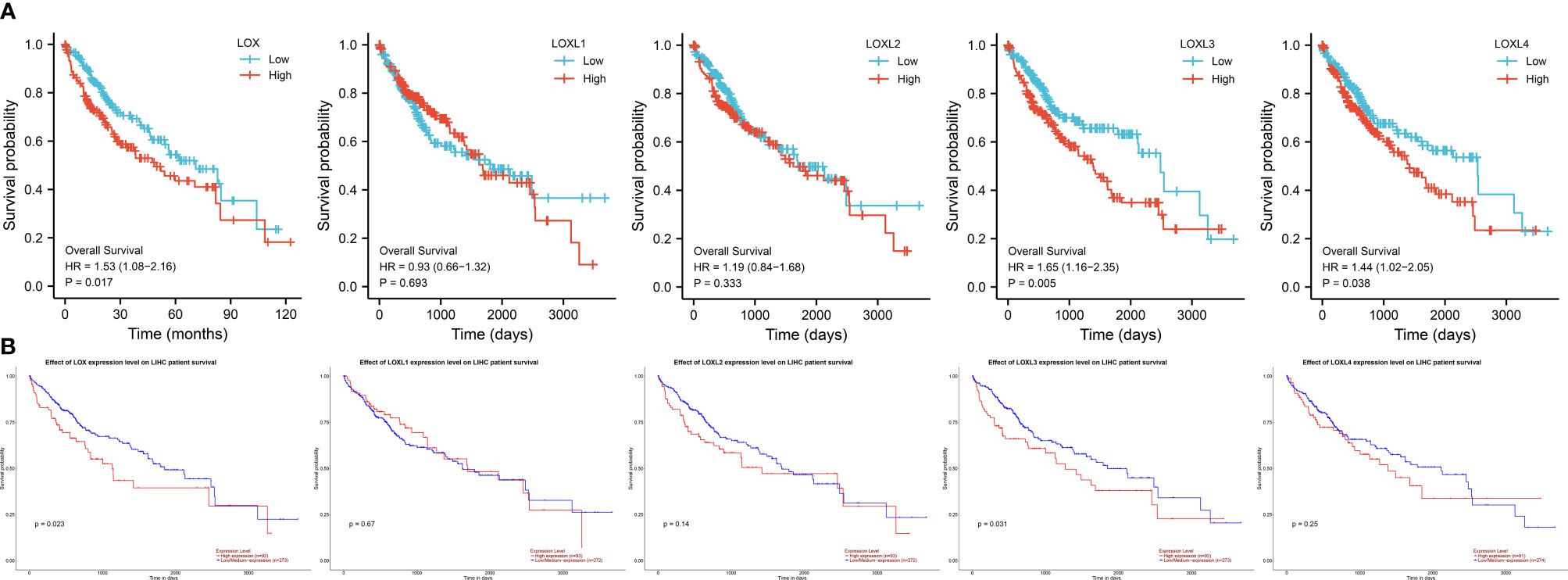
Figure 3 Survival analysis of LOX family members in liver cancer. (A) analysis via R software, (B) analysis via ULCAN.
A nomogram model incorporating the overexpressed LOX family members that were associated with poor prognosis, namely LOX and LOXL3 and other predictors (pathologic stage, histologic grade, AFP (ng/ml), Child-Pugh grade, albumin (g/dl), adjacent hepatic tissue inflammation, vascular invasion, Ishak Fibrosis score, prothrombin time, age, gender, weight) is shown in Figure 4. The C-index of the nomogram was 0.738 (95% CI, 0.697-0.778).
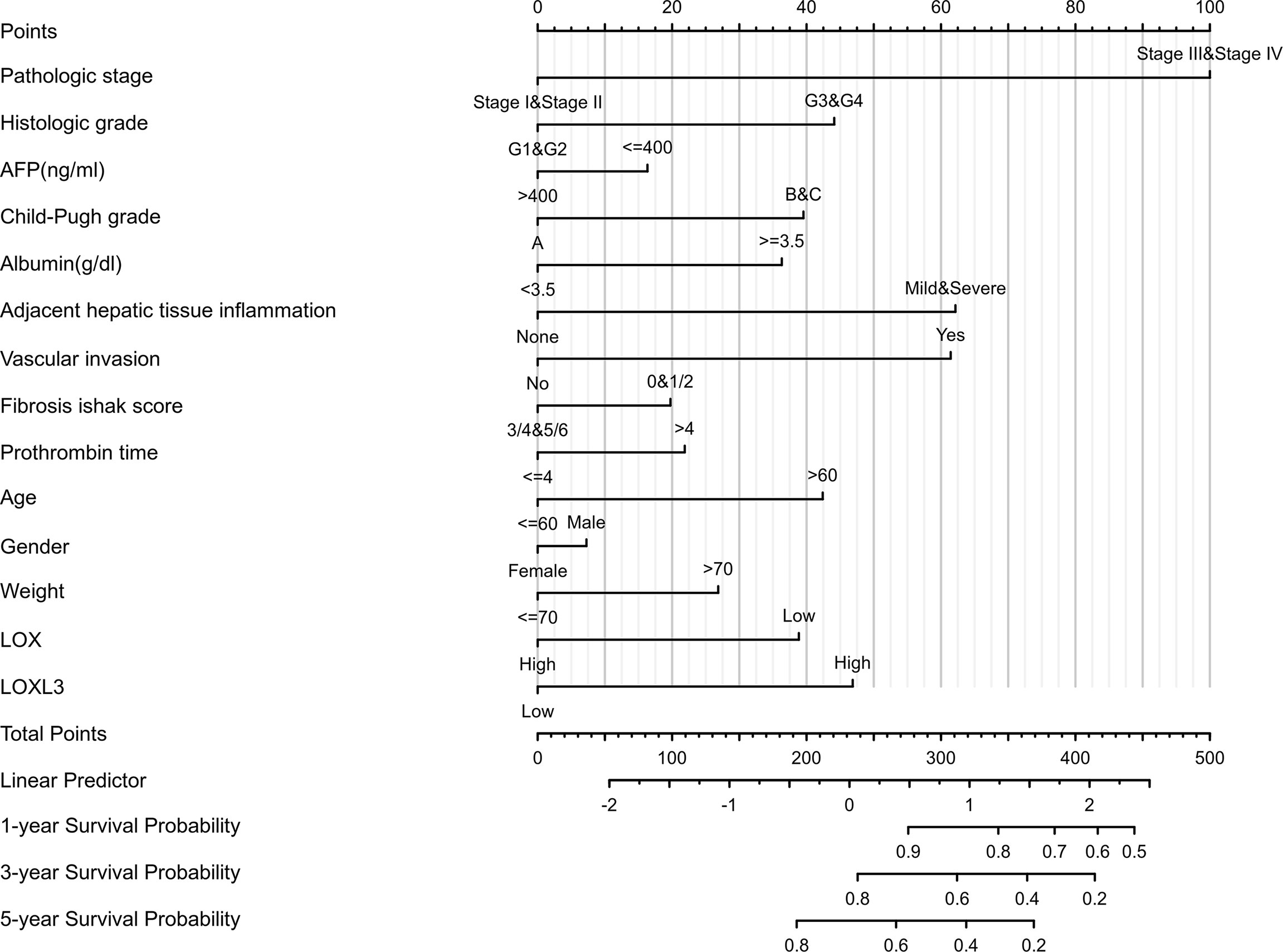
Figure 4 Nomogram for liver cancer based on overexpressed LOX and LOXL3. The nomogram was developed in the cohort, with pathologic stage, histologic grade, AFP (ng/ml), Child-Pugh grade, albumin (g/dl), adjacent hepatic tissue inflammation, vascular invasion, Ishak Fibrosis score, prothrombin time, age, gender, weight. (C-index: 0.738, 95% CI, 0.697-0.778).
Analysis of Genetic Mutations of LOX Family in LC
Next, the genetic alterations of the LOX family in LC patients were evaluated with the cBioPortal online tool. Among 1,066 LC patients, 55 samples had genetic alteration of LOX family members, with a mutation rate of 5.16%. The mutation rate of LOXL2 was the highest (4%) (Figures 5A, B). Using cBioPortal and TIMER online tools, we found significant (p<0.01) and positive correlations amongst LOX family member proteins: LOX with LOXL1, LOXL2, LOXL3, and LOXL4; LOXL1 with LOX, LOXL2, LOXL3, and LOXL4; LOXL2 with LOX, LOXL1, LOXL3, and LOXL4; LOX3 with LOX, LOXL1, LOXL2, and LOXL4; LOX4 with LOX, LOXL1, LOXL2, and LOXL3 (Figures 5C, D).
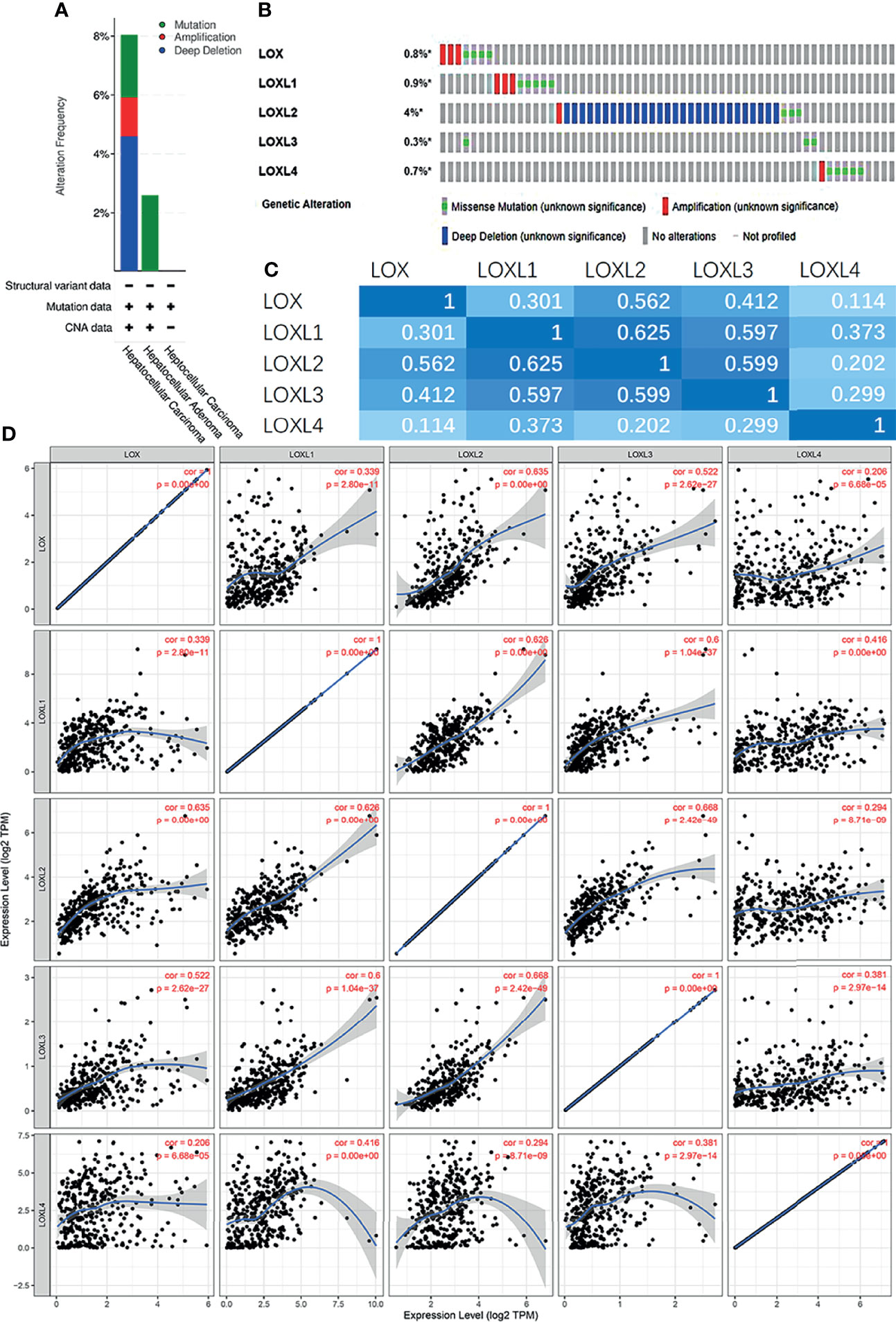
Figure 5 Gene mutation and expression analysis of LOX family members in patients with liver cancer: (A) Genetic alterations of LOX family members in different histopathologic types of liver cancer; (B) Summary of genetic alterations in different expressed LOX family members in liver cancer; (C) Correction between different LOX family members in in liver cancer (cBioPortal); (D) Correction between different LOX family members in in liver cancer (TIMER).
Exploration of Potential Drugs That Are Interacted With LOX Family Members in LC
As LOX and LOXL3 were both found to be overexpressed in LC and associated with worse OS, further exploration of potential interacting drugs was conducted by using Coremine Medical, which identified 30 drugs that were associated with both LOX and LOXL3 in liver neoplasms (Figure 6). The top three drugs were Aminopropionitrile, quinone, and copper.
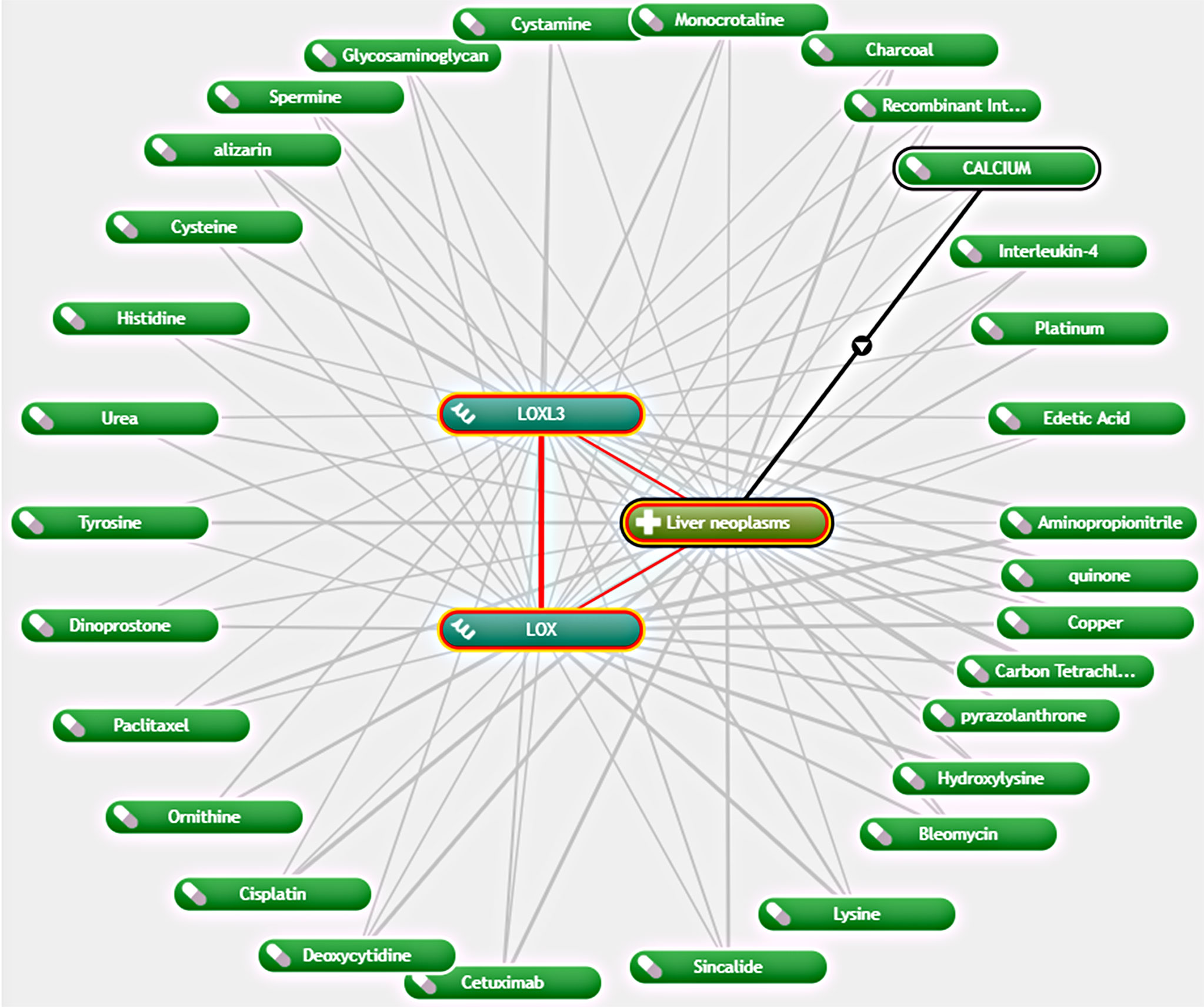
Figure 6 Network of association between LOX and LOXL3 and different drugs in liver neoplasm via Coremine Medical.
Analysis of Interaction of LOX Family Members in Patients With LC
Using the STRING database, PPI network analysis was performed on the differentially expressed LOX family members and 10 proteins (BMP1, ELN, EFEMP2, FBLN5, FN1, MFAP2, MFAP5, PCOLCE, TLL1, TLL2) that significantly interacted with LOX family members to further explore their potential interactions (Figure 7A). The results from GeneMANIA also revealed the function of differentially expressed LOX family members. Their top 20 associated interactors were primarily related to extracellular matrix organization, extracellular structure organization, extracellular matrix, proteinaceous extracellular matrix, extracellular matrix part, extracellular matrix disassembly, and extracellular matrix structural constituent (Figure 7B).
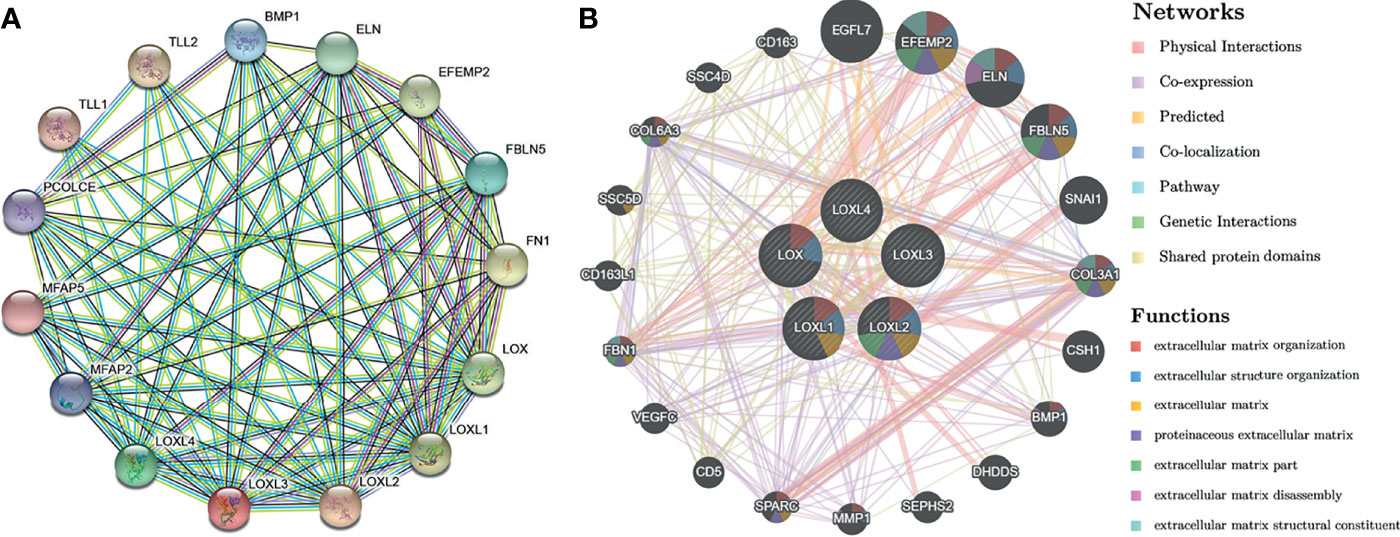
Figure 7 Protein-protein interaction (PPI) network analysis of LOX family members in patients with liver cancer. (A) PPI network of LOX family members and their interactors visualized by STRING; (B) PPI network of LOX family members and their interactors visualized by GeneMANIA.
GO Enrichment and KEGG Pathway Analysis of LOX Family Members in LC
GO enrichment and KEGG pathway analysis of LOX family members and their 20 interactors were conducted by using DAVID. Receptor-mediated endocytosis, extracellular matrix organization, and extracellular matrix disassembly were the top three biological processes that were associated with LOX family members and their interactors (Figure 8A). The extracellular region, proteinaceous extracellular matrix, and extracellular matrix were the top three major cellular components of the target genes (Figure 8B). As for molecular function, scavenger receptor activity, oxidoreductase activity (acting on the CH-NH2 group of donors, oxygen as acceptor), and copper ion binding were the top three functions (Figure 8C). In regard to KEGG pathways, protein digestion and absorption, PI3K-Akt signaling pathway, and ECM-receptor interaction were the top three pathways involved in LC (Figure 8D).
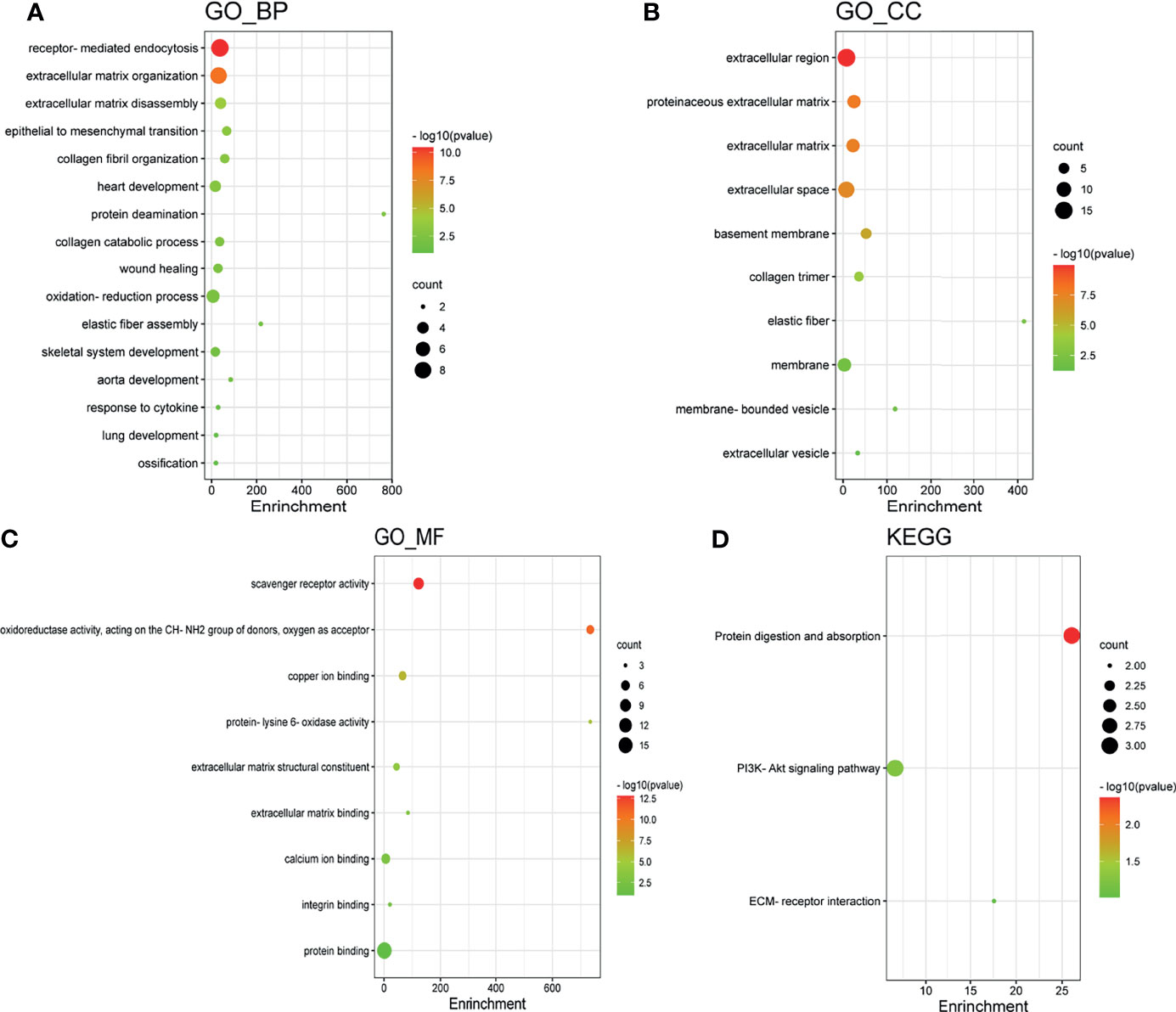
Figure 8 Gene Ontology (GO) enrichment and Kyoto Encyclopedia of Genes and Genomes (KEGG) pathway analysis of LOX family members and their interactors. GO enrichment analysis of target genes based on (A) biological process, (B) cellular component, and (C) molecular function. (D) KEGG pathway enrichment analysis of target genes.
Immune Cell Infiltration of LOX Family Members in LC
The TIMER database was utilized to investigate the association between LOX family members and immune cell infiltration, as immune cell level correlates with the proliferation and progression of cancer cells (Figure 9). The expression of each LOX family member was positively correlated with the infiltration of B cell, CD8+ T cells, CD4+ T cells, macrophages, neutrophils, and dendritic cells (DCs). Among them, Macrophage and CD4+ T Cells demonstrated the strongest positive correlation. In addition, the Cox proportional hazard model showed that B cells (p=0.031), CD8+ T cells (p=0.036), macrophages (p=0.027), and DCs (p=0.004) were significantly associated with adverse clinical outcomes in LC patients (Table 2). The association between immunomodulators and LOX family members with poor prognosis, namely, LOX and LOXL3, were then further explored. The top three immunoinhibitors associated with LOXL3 were CSF1R, HAVCR2, and LGALS9, whilst the top three immunostimulators correlated with LOXL3 were CD86, TNSF13B, and CXCR4. MHCs associated with LOXL3 were HLA-DOA, HLA-DPA1, and HLA-DPB1. As for LOX, TGFB1, TGFBR1, and VTCN1 were the most positively correlated immunoinhibitors. TNFRSF9, CXCR4, and TNFSF15 were the three most positively associated immunostimulators. However, for MHC molecules, their associations were relatively low, with HLA-DQA2, HLA-DOA, and HLA-DPA1 as the top three molecules (Figure 10).
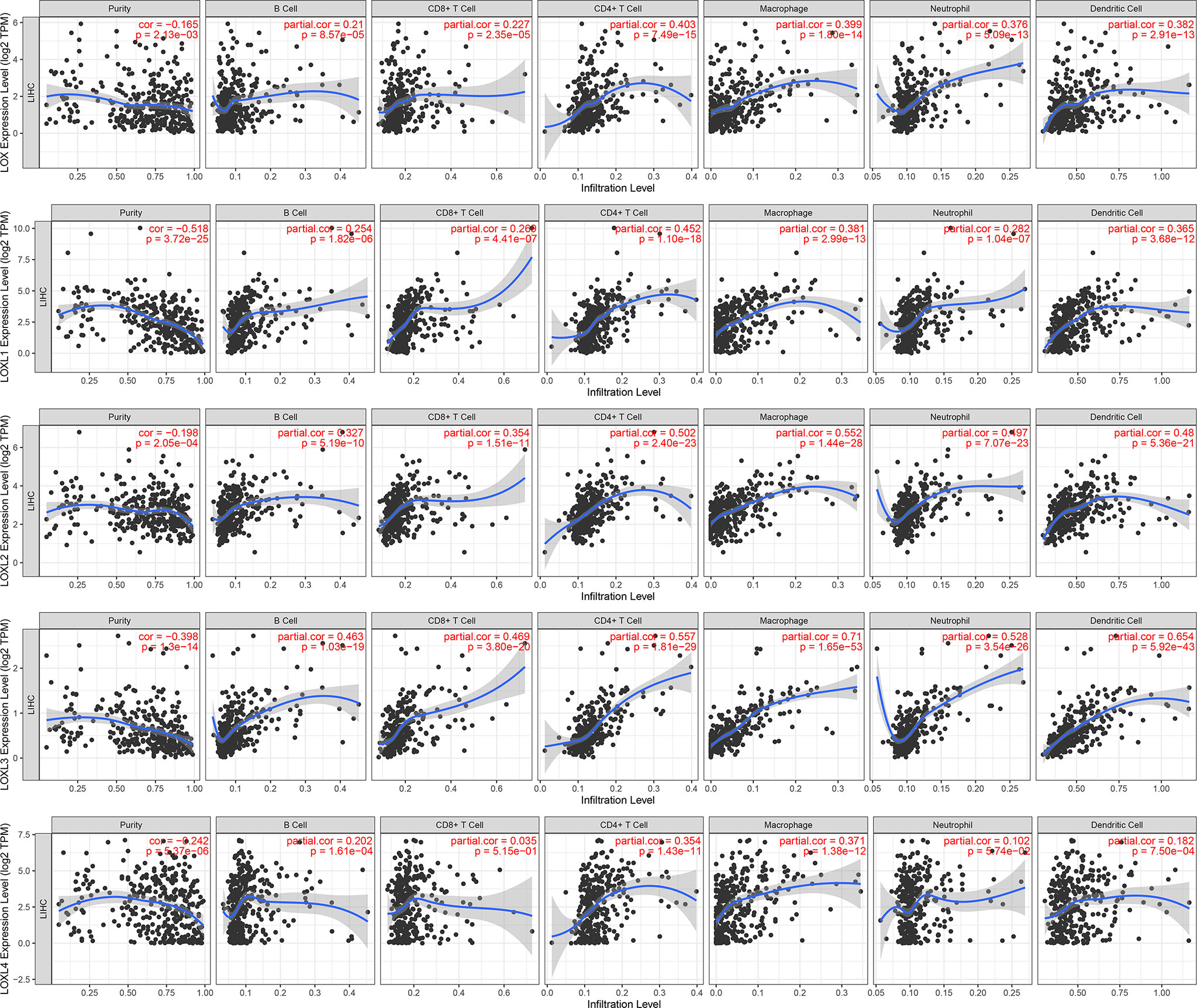
Figure 9 Correlations between differentially expressed LOX family members and immune cell infiltration in liver cancer (TIMER).
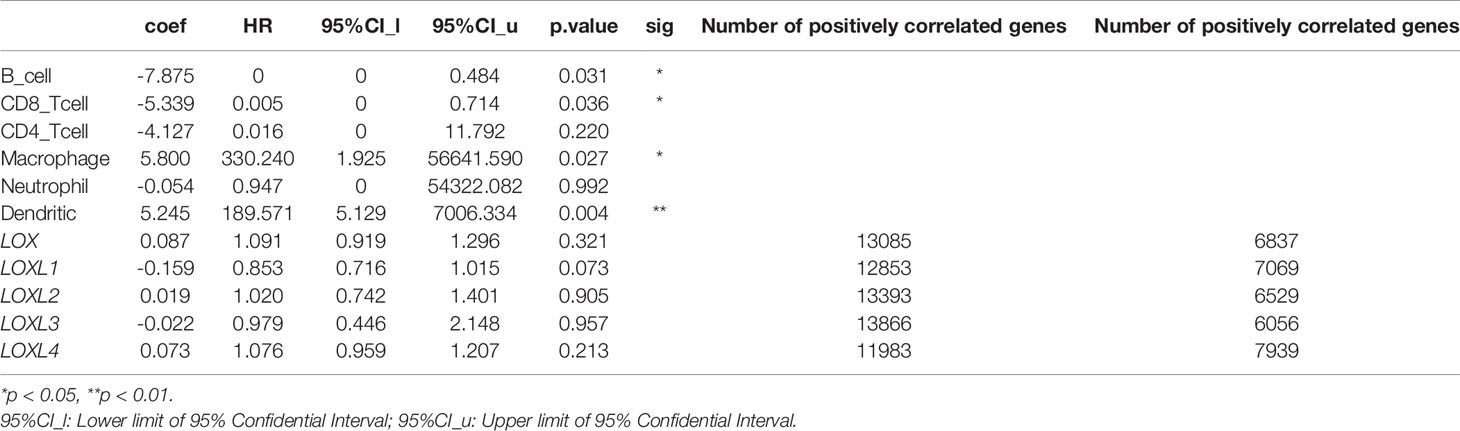
Table 2 The Cox proportional hazard model of LOX family members and six tumor-infiltrating immune cells in liver cancer (TIMER), and Number of genes that are positively and negative correlated with LOX family members.
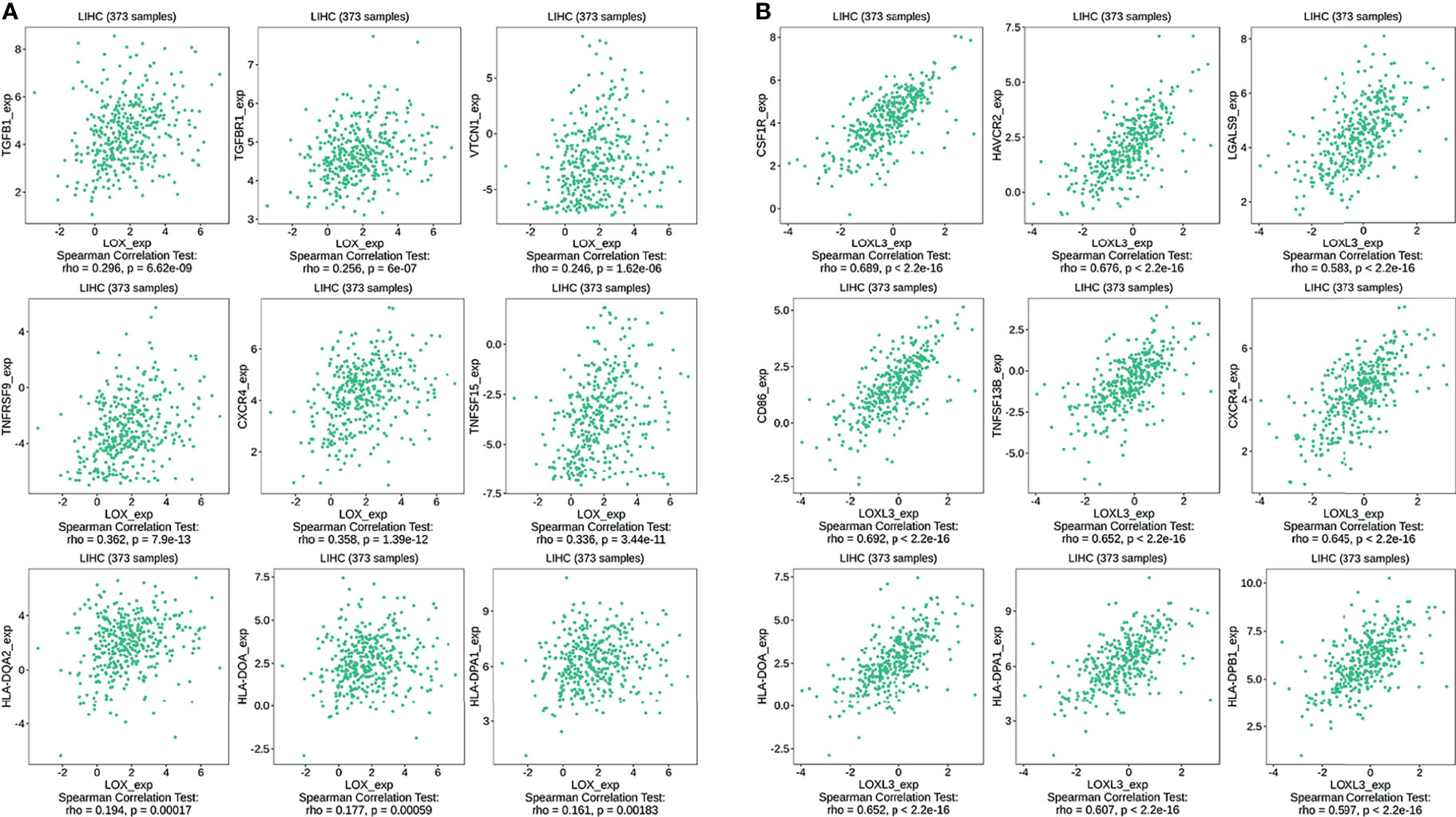
Figure 10 Associations of the LOX and LOXL3 expression level with immunomodulators in LC from TISIDB database. (A) Immunomodulators that are highly correlated with LOX; (B) Immunomodulators that are highly correlated with LOXL3.
Co-Expression Network and GSEA Analysis of Each Member of LOX Family in LC
For each member of LOX family, more genes (dark red dots) are positively correlated than negatively correlated (dark green dots) (Figure 11 and Table 2). GO term annotation of co-expressed genes of each member of LOX family as well as KEGG pathway analysis were shown in Figure 12. These results showed a wide range of influence of LOX family expression network in LC.
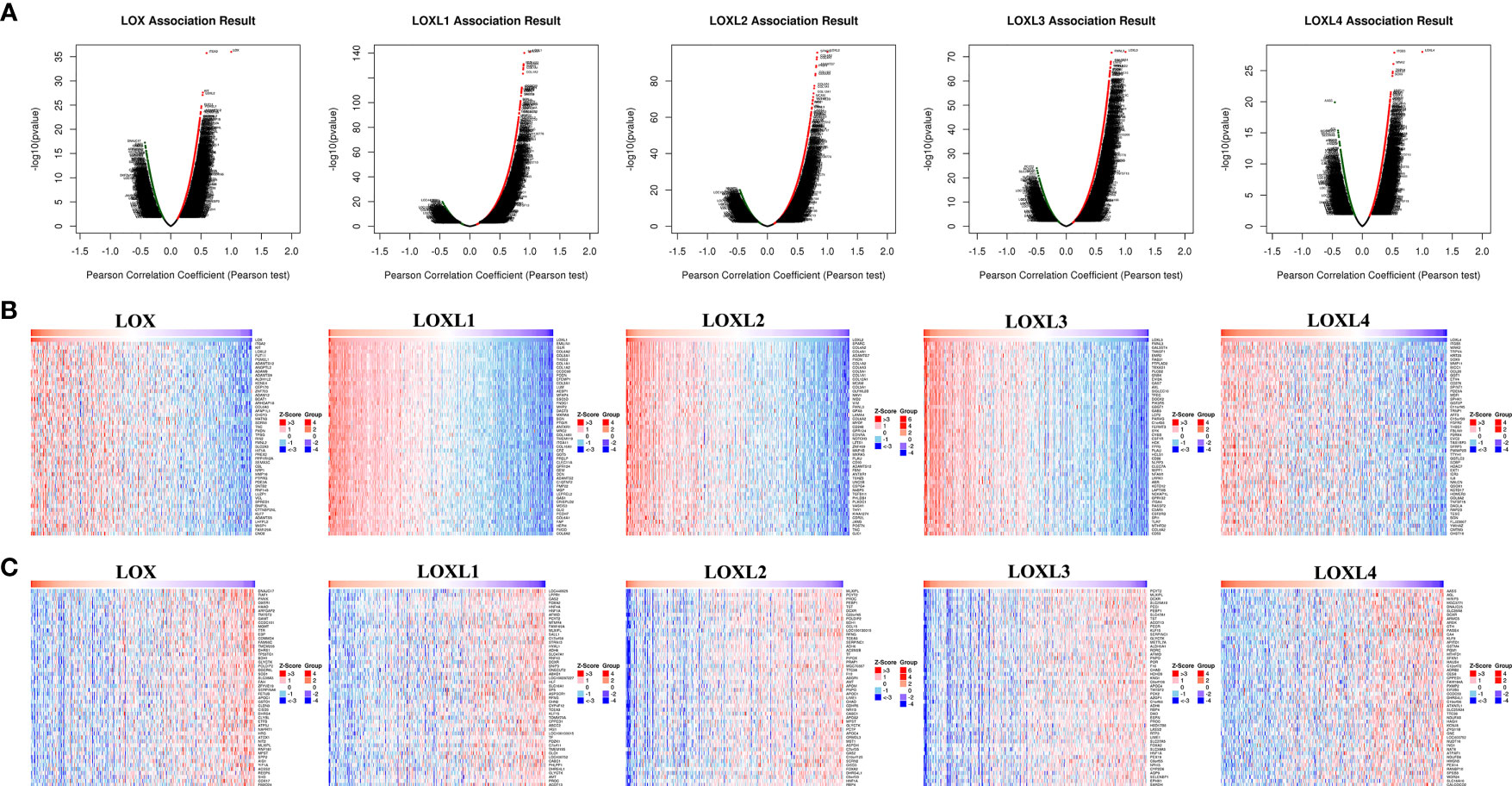
Figure 11 The co‐expression genes with LOX family members in LC from the LinkedOmics database. (A) The whole significantly associated genes with LOX family member distinguished by Pearson test in LC cohort. (B) Top 50 genes positively related to LOX family member in LC showed by heat maps. (C) Top 50 genes negatively related to LOX family member in LC showed by heat maps. Red represents positively linked genes and blue represents negatively linked genes.
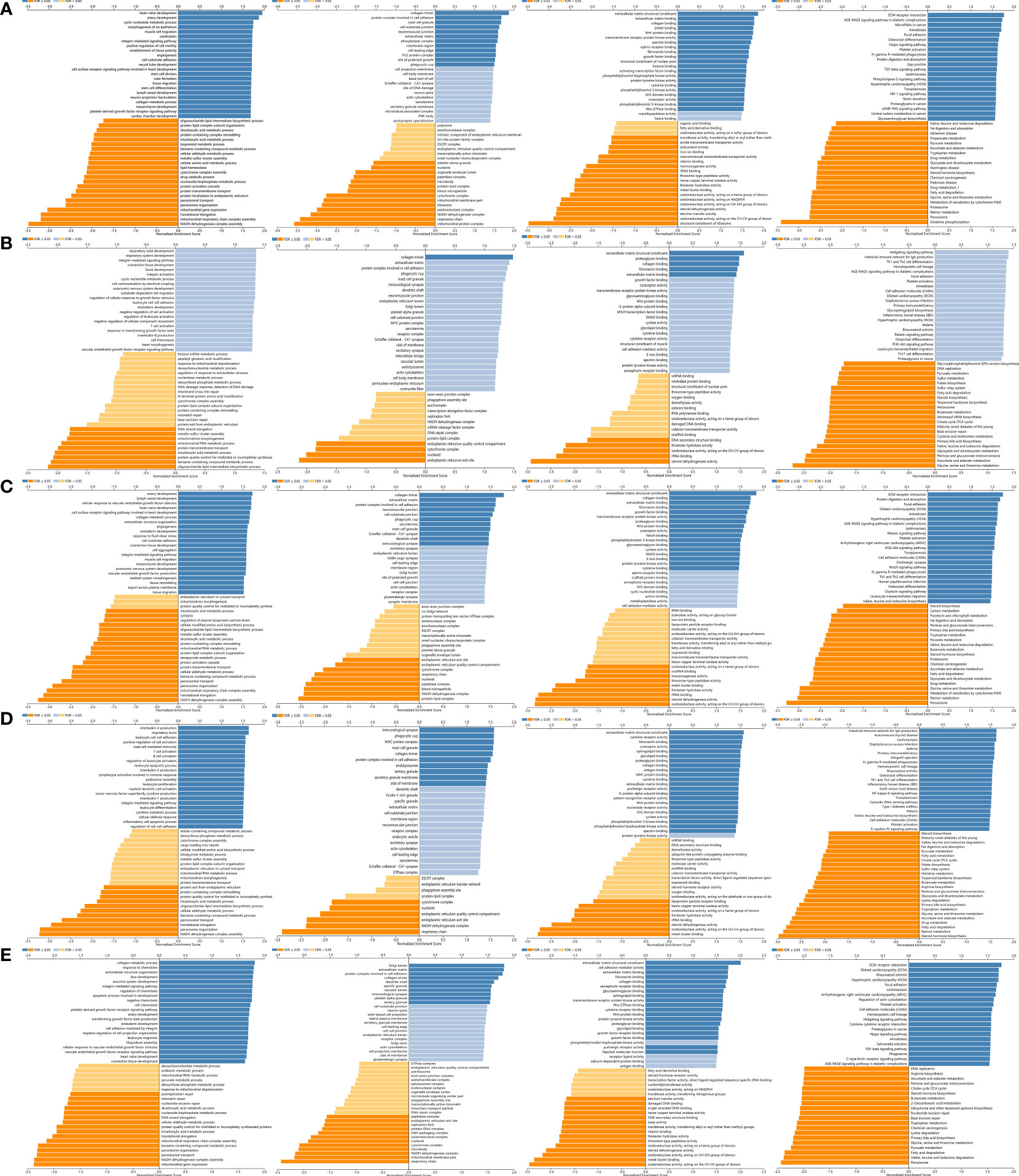
Figure 12 GO annotations and KEGG pathways of LOX family and their associated genes in LC cohort: (A) results of LOX; (B) results of LOXL1; (C) results of LOXL2; (D) results of LOXL3; (E) results of LOXL4.
Meta-Analysis of the Prognosis of LOX, LOXL2 and LOXL4 in LC
Based on the search strategy, four studies (28–31) investigating LOX, LOXL2 and LOXL4 were included for the meta-analysis, while no potential literature on other LOX family members were found. We combined the results of our bioinformatics analysis from the TCGA with those retrieved in the database and obtained the HR values. One study (29) provided results regarding lower expressed LOXL2 compared with higher expressed LOXL2, therefore, the HR was transformed using the formula new HR=e^(-ln HR) to convert the result to the OS of higher expressed LOXL2 compared to lower expressed LOXL2. This resulted in the new HR of 1.761 (95% CI: 1.215-2.551).
The pooled results revealed that overexpression of LOX and LOXL4 were associated with worse OS of LC patients (HR: 1.59, 95% CI: 1.19-2.12, I2 =0%; HR: 1.58, 95% CI: 1.28-1.96, I2 =0%), while the association between overexpression of LOXL2 and OS of LC patients showed no statistical significance (HR: 1.33, 95% CI: 0.99-1.79, I2 =29.5%) (Figure 13). Sensitivity analysis indicated stable results of this meta-analysis.
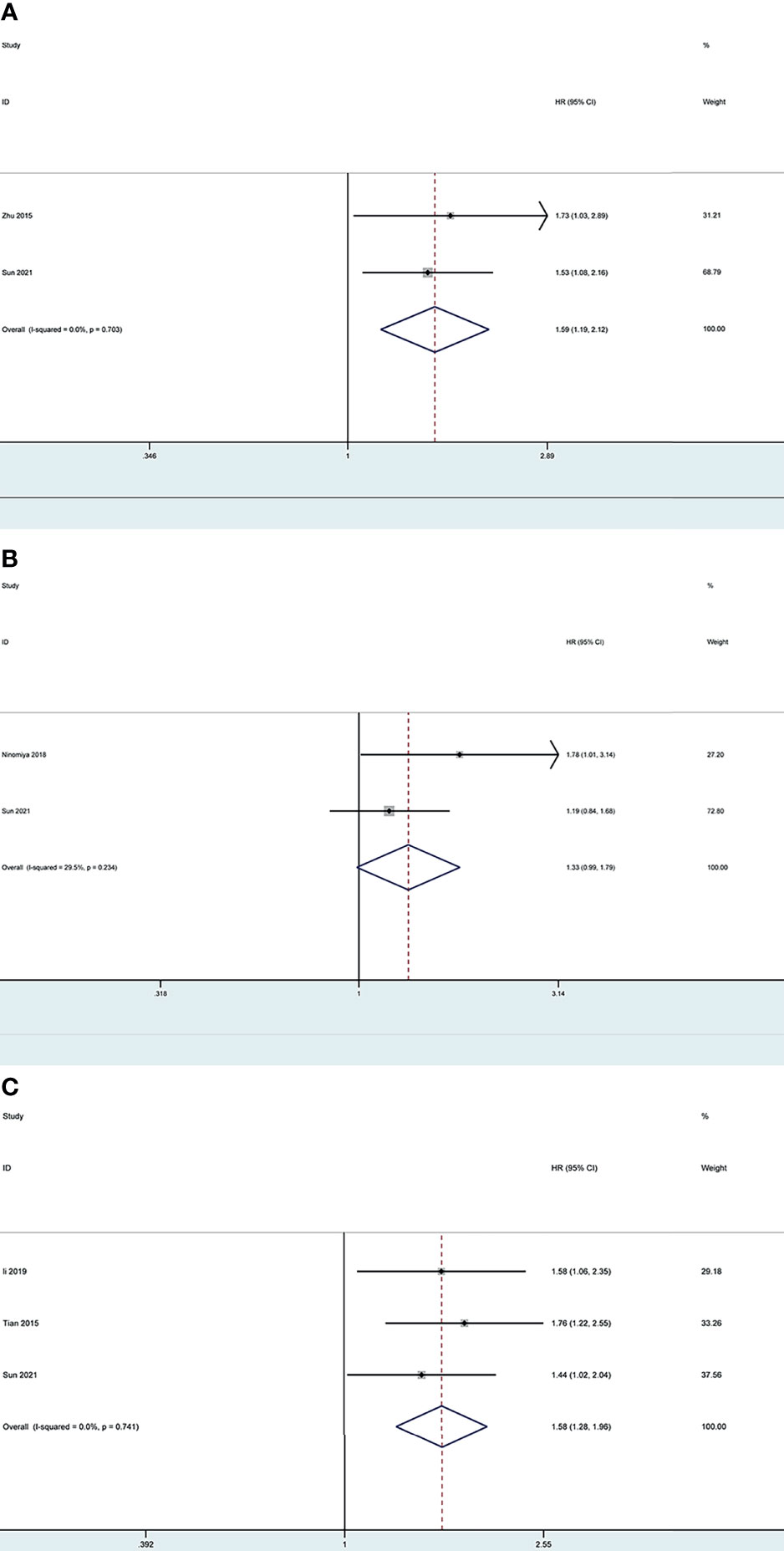
Figure 13 Forest plot of the prognosis of LOX, LOXL2 and LOXL4 for LC patients: (A) Forest plot of overexpressed LOX; (B) Forest plot of overexpressed LOXL2; (C) Forest plot of overexpressed LOXL4.
Discussion
As a common malignancy with the third leading cause of cancer-related mortality (1), LC risk is influenced by various environmental and genetic factors (4–6). Previous studies have demonstrated that LOX is highly expressed in the fibrous lamina propria in the small intestine, stomach, and liver, as well as other tissues that contain elastic fibers and fibrillar collagen (16). LOX, LOXL, LOXL2, LOXL3 and LOXL4 were to be in intracellular locations, perinuclear regions and intranuclear locations, and are secreted to exert their functions, such as extracellular enzyme for initiating covalent cross-link formation in collagen fibrils (15, 19, 32–35). After secretion, LOX family members oxidase crosslink collagen and elastin (19, 36). LOXs were found to be involved in various physiological or pathological pathways, both in extracellular modulation and intracellular signaling (32). Studies have found LOX family members to be involved in carcinogenesis and tumor metastasis by formation of mature extracellular matrix at the secondary site, FAK activation, and promotion of angiogenesis (19–22). Overexpressed LOX was found to promote angiogenesis (23, 25), and expression level of LOXL2 was higher in HCC than in non-tumor tissue (26). LOXL4 was found to increase the risk of invasion and metastasis of LC via various mechanisms such as angiogenesis and through its involvement in creating an immunosuppressive microenvironment (25, 27, 28). However, studies on the roles of LOLX1 and LOXL3 in liver cancer are limited (25). As the role of LOX family members in LC remains inconclusive, this bioinformatic study was conducted to analyze the expression, mutation, prognostic value, and functional enrichment of LOX family in LC.
We found that all five members of LOX family are higher expressed in LC tissues than in the normal tissues and their overexpression are positively correlated with each other, which is consistent with previous findings that the expression of LOX, LOXL2, and LOXL4 are upregulated in HCC (25). A previous study found a 30-fold increase of LOXL1 level in a liver fibrosis model (24). However, the role of LOXL3 in LC was not yet clear (25), and our results provided evidence that not only LOX1 but also LOXL3 is highly expressed in LC. As shown by the cBioPortal analysis, 5.16% of LC patients were found to have genetic mutation of LOX family members, and the mutation rate of LOXL2 was the highest. Further analysis of ROC curve showed that the AUC of LOXL2 was above 0.9 with a PPV of 0.994, indicating its potential role in diagnosis. These findings are consistent with those reported by Wong et al, in which the AUC of LOXL2 to distinguish non-HCC and HCC patients was 0.896 (26). Therefore, LOXL2 is a good candidate for a diagnostic marker in LC, especially HCC.
Based on the analyses through various tools, high expression of LOX and LOXL3 was found to predict worse prognosis. This proves the previous hypothesis of upregulation of the LOX level as a predictive sign for HCC, proposed by Lin et al. (25). LOX gene, located at chromosome 5q23.1, is consist of a variable N-terminal domain and a highly conserved C-terminal domain (25). LOX itself is an extracellular, matrix-embedded protein that plays an essential role in the cross-linking of the collagen fibrils and the deposition of insoluble collagen fibers (37, 38). Previous studies indicated that LOX overexpression induced the Epithelial-Mesenchymal Transition (EMT) (39). In addition, Yang et al. proved that the overexpression of LOX activated the angiogenesis partially through increasing the VEGF and enhancing the tube formation ability of endothelial cells in tumor initiating cells (TICs)-enriched HCC, and LOX inhibitor β-aminopropionitrile (BAPN) reverses the angiogenesis (40). Zhu et al. also found that the proliferative, migratory. and invasive abilities of HCC cells were attenuated, and the expression of vascular endothelial growth factor (VEGF) was decreased by the silencing of LOX, through the p38 mitogen-activated protein kinase (MAPK) signaling pathway (30). LOX3, located at chromosome 2p13.1, plays an important role in remodeling the cross-linking of the structural extracellular matrix (ECM) of fibrotic organs such as the liver (25, 41). It was also shown that higher expression of LOXL3 was regulated by TGF-β in gastric cancer (42). However, studies on the biological function of LOXL3 in HCC are still limited (25, 39, 43). Previous literature on the prognostic role of LOXL3 in LC patients was also minimal. Therefore, meta-analysis on LOXL3 was not conducted. Nevertheless, the result of this analysis added new evidence that LOXL3 could be potentially used a prognostic biomarker in addition to LOX. A nomogram based on LOX, LOXL3, and other predictors were developed which can help predict the mortality risk for an individual LC patient. Moreover, given their negative impacts on the survival in LC patients, LOX and LOXL3 may also serve as potential therapeutic targets. Although the ULCAN and TIMER did not verify the worse prognosis associated with LOXL4, our result based on TCGA data indicated the potential clinical significance of LOXL4 for worse outcome. As part of the LOX family, LOXL4 gene is located at chromosome 10q24.2 (25). The in vitro study suggested that TGF-β might induce LOXL4 upregulation in several different HCC cell lines, and LOXL4 mediated cell-matrix adhesion and cell migration in HCC via upregulation of Src and FAK phosphorylation (43). Although LOXL4 is an important extracellular protein, the HCC cell migration was promoted more by the intracellular LOXL4 (43). In contrast, other study revealed that 5-azacytidine (5-aza-CR)-mediated overexpression of LOXL4 reactivated wild-type p53 and promoted cancer cell death, thus suppressing the development of HCC cancers, which might indicate an improved clinical outcomes of HCC patients (28, 44). These complicated and even contradicting mechanism of LOXL4 in HCC might partially explained the inconsistent findings of its role in HCC prognosis from TCGA, TIMER and ULCAN database.
Further exploration of potential drugs associated with LOX and LOXL3 in LC by using Coremine Medical found 30 drugs. The drug that demonstrated the strongest interaction was aminopropionitrile. β-aminopropionitrile (BAPN), obtained from a natural source, was the first compound found to have inhibitory effect on LOX (45). A previous study suggested the potential therapeutic value of BAPN for liver metastasis in gastric cancer (46). Another animal study demonstrated antifibrotic effect of BPAN through reducing collagen fiber bundles and LOX level, which indicates its potential role in attenuating the development of liver fibrosis (47). It was also found that that BAPN acts by reversing the angiogenesis that was activated by the overexpression of LOX (40). Although quinone and copper were found to be potential interacting drugs, they are more likely to be identified because their own function and roles in the LOX proteins. Quinone is part of the redox cofactor of LOXs, which is a functional group in the catalytic domain of LOX proteins (48). LOX family members also contain a conserved copper-binding site in the C-terminal half of the protein (49). Copper binding to key histidine residues facilitates the formation of quinone-contained redox factor which in turn leads to the oxidase activity (48, 49). Therefore, quinone and copper can be potential research targets in the future to explore any potential practical use or potential use as therapeutic target. In addition, other drugs found through the exploration, such as cetuximab, bleomycin, cisplatin, paclitaxel, were known to have anti-cancer effects in various types of cancer, including LC, and anti-fibrosis effects in other diseases such as pulmonary fibrosis (50–59). Their exact roles and effects in LC may need to be clarified in future studies.
Exploration of the PPI network of LOX family and their top interactors found that these genes are primarily related to extracellular structures and functions. GO enrichment and KEGG pathway analysis of these genes also found they are mostly involved extracellular functions and structures. This is not surprising as it is well known that members of LOX family contribute to structural integrity and increased tensile strength by their catalytic activity, and exert roles in remodeling the cross-linking of the structural extracellular matrix (ECM) of fibrotic organs such as the liver (25). In addition, LOX family members are involved in scavenger receptor activity, oxidoreductase activity, and copper ion binding. Multiple scavenger receptor cysteine-rich (SRCR) domains exist n LOXL2 and LOXL3 (60, 61). As LOX family are copper-dependent amine oxidases (25), it is not unexpected that oxidoreductase activity and copper ion binding are involved. Further analysis via LinkedOmics database also identified a significant amount of co-expressed genes associated with each LOXL family members, and found that these co-expressed genes are also largely involved in extracellular and functions and structures, or participate in human tissues that contain elastic fibers, fibrillar collagen, and organs with a great amount of fibrous lamina propria.
The growth and metastasis of tumor cells depend on a complex tumor microenvironment (TME) (62). TME comprises of cells of hematopoietic origin, such as lymphocytes and myeloid cells, cells of mesenchymal origin, including mesenchymal stem cells, endothelial cells, adipocytes, fibroblasts, and myofibroblasts, and the ECM (63). ECM is a complex network providing structural support, biochemical reagents and biomechanical signals for the growth of cancer cells, and it consists of multiple components, including collagen, integrin, laminin, fibronectin, glycosaminoglycans, matrix metalloproteinases (MMP) and secreted cysteine-rich acidic proteins (64). Further analysis on the relationship of LOX family members and tumor-infiltrating immune cells in LC found positive correlations between the infiltration of B cell, CD8+ T cells, CD4+ T cells, macrophages, neutrophils, and DCs and all LOX family members. Moreover, the infiltration of B cells, CD8+ T cells, macrophages, and DCs were associated with worse outcomes. Immune cell infiltration in HCC under different conditions, such as bile acid-mediated immune cell infiltration (65) and TP53 mutations (66), have been investigated in the past. However, evidence on the associations between LOX family members and tumor-infiltrating immune cells in LC is limited. In addition, immunomodulatory drugs are under development for various conditions and have been approved in recent years for certain tumors such as multiple myeloma (67, 68). Therefore, we explored and identified a list of immunoihibitors, immunostimulators, and MHC molecules that are positively correlated with LOX and LOXL3, the two LOX family members with poor prognosis. These immunomodulators could be potential immunotherapeutic targets. The immune environment is thought to be critical in tumor progression and may even play a crucial role in different treatments for cancers, including chemotherapy, radiotherapy, and especially immunotherapy (69–71). Our findings suggest that there is a significant role of the LOX family in the tumor microenvironment. Therefore, comprehensive studies on the association of tumor-infiltrating immune cells, as well as immunomodulators, and LOX family in LC are needed.
It is well known that tumor heterogeneity relies on the TME, including both the cancer cells themselves and different types of immune cells and the surrounding stroma. TME closely correlates with the response to immunotherapy and the prognosis in multiple cancers (72). TME tends to be involved in the immunosuppression and drug resistance, resulting in less satisfactory responses to immunotherapy. In addition, immune checkpoint blockade (ICB) relies on restoring the function of T cell to eliminate tumors (70). Moreover, as part of the adaptive immune resistance, tumor cells could upregulate the immune checkpoint gene expression to suppress T cell activity that eventually leads to immune escape (73). Thus, our findings of positive association between CD8+ T cells and CD4+ T cells, as well as other immune cells, and LOX family members suggest that ICB and other immunotherapy could have a promising potential in LC treatment as high expression of LOX family members in tumor tissues facilitates immune cells infiltration, which could induce the immune response exerting the antitumor efficiency. It would particularly helpful to investigate compounds target on immunoinhibitors and immunostimulaters identified in our study. As demonstrated in our study immunoinhibitors, CSF1R, HAVCR2, and LGALS9, were found to be associated with LOXL3, while TGFB1, TGFBR1, and VTCN1 correlated with LOX. CSF-1R plays critical roles in regulating tumor-associated macrophages in TME, and targeted inhibition of the CSF-1/CSF-1R signal axis has broad application prospects in immunotherapy of malignant tumors (74). Pexidartinib is an orally administered small-molecule tyrosine kinase inhibitor that selectively inhibits CSF1R, and is currently being assessed for other types of cancer (75). Another kinase inhibitor, Derazantinib, also found to have activity against CSF1R and is under investigation for cholangiocarcinoma (76). Their potential use in LC also deserves further exploration. HAVCR2, also known as TIM-3 and CD366, enhances T cell inhibition and apoptosis and immune-suppressive activity of Tregs (77). Antibodies against HAVCR2 disrupt the binding of the ligands to HAVCR2 are under investigation as a potential combination partner of anti-PD-1/L1 therapy (78). Previous study also found that HAVCR2 receptor limits T-cell responses by interacting with LGALS9 (79). A recent study also demonstrated that chemoradiation could induce increased expression of PD-L1 and LGALS9 in gastric cancer (80), however, whether similar result can be found in LC needs further study. TGF-B1 is a potent inhibitor of T cell growth, partly by inhibiting IL-2 expression and secretion by T cells themselves (81), and interestingly, it can also affect anti-tumor T cell responses by downregulating MHC molecules on the surface of tumor cells. Despite its critical roles, the development of TGFB1-targeting therapies has not been progressed well, probably due to concern of severe toxicities that could arise from blocking tumor suppression exerted by TGF-β1 at early stages of tumorigenesis as TGFB1 exerts potent cytostatic and pro-apoptotic activities in pre-malignant cells (82, 83). In addition, blocking TGFB1 activities on normal cells outside of the TME may also lead to toxicities (82). Nevertheless, certain antibodies are still under investigation. For example, studies on Fresolizumab, a fully human monoclonal IgG4 antibody that neutralizes mature TGFB1, were conducted for malignant pleural mesothelioma, melanoma and renal cell carcinoma (82). Galunisertib, another TGFBR1inhibitor, was found to have 16% of objective responses in glioblastoma patients with no serious treatment-related toxicities (82, 84). Another clinical trial for pancreatic cancer patients showed that combination of chemotherapy (gemcitabine) and galunisertib was associated with increased survival compared to chemotherapy alone (85), and it is now also tested for combination with anti-PD-1 antibodies (82). In addition, a new TGFBR1 kinase inhibitor called vactosertib, currently tested in early-stage clinical trial for several cancer types (83). VTCN1, also known as B7S1, is also a negative regulator of tumor immunity by various mechanisms such as dampening the anti-tumor Th1 responses (86). Recently, an early-stage clinical study of FPA150, an antibody targeted on B7S1 and other the anti-B7x family members, was started for patients with advanced solid tumors to assess preliminary efficacy of FPA150 alone or in combination anti-PD, as well its safety, tolerability, pharmacokinetics, and pharmacodynamics (87). In addition to immunoinhibitors, the immunostimulators CD86, TNSF13B, and CXCR4, were found to be associated with LOXL3, while TNFRSF9, CXCR4, and TNFSF15 correlated with LOX. CD86, also known as B7-2, is an immune checkpoint molecule of B7 family and binds to CD28 and Cytotoxic T-Lymphocyte Antigen 4 (CTLA-4). Interaction of CD86 with CTLA-4 inactivates T lymphocytes, causing the escape of tumor cells from the immune system. Therefore, immunotherapy using CTLA-4 antibodies might promote T cell activation to help eliminating tumor cells (88). Ipilimumab, an CTLA-4 antibody, is currently approved by Food and Drug Administration (FDA) for HCC treatment in combination with nivolumab. In addition, Tremelimumab, fully human immunoglobulin G2 monoclonal antibody directed against CTLA-4, is also under investigation for HCC treatment (89). TNSF14B, also known as B cell-activating factor of the TNF family (BAFF), together with its receptor, BAFFR, are important in early B-cell homeostasis and regulatory T-cell function (90). BAFF inhibitors have been tested for certain diseases. For instance, belimumab, a fully human monoclonal antibody against BAFF, has been shown to have a modest effect for active systemic lupus erythematosus (SLE), and another BAFF inhibitor Blisibimod is also under investigation for SLE (90, 91). Tabalumab is another BAFF inhibitor, and has been evaluated as a combined therapy with bortezomib for multiple myeloma (90). However, the roles of BAFF inhibitors in HCC still yet to be explored. TNFRSF9, also known as CD137, a surface glycoprotein belonging to a member of the tumor necrosis factor receptor superfamily (TNFRSF). It is expressed on activated T cells that have encountered cognate antigen, activated NK cells, and mature DCs (92). Two clinical trials have been being initiated for two anti- TNFRSF9 monoclonal antibodies urelumab (BMS-663513) and utomilumab (PF-05082566) (93, 94). TNFSF15, also called TNF-like molecule 1A (TL1A), is expressed on multiple immune cells such as DCs and B cells. It binds to DR3 receptor, leading to cell apoptosis by activating the caspase cascade through interaction with TRADD and FADD, and the activation of multiple cell survival signaling pathways including NF-kB, STAT3, JNK, p38 MAPK and ERK (95, 96). TNFSF15 can also suppress endothelial cell proliferation and angiogenesis through the binding of DR3, and this was verified in a mouse xenograft tumor model (97, 98). Moreover, TNFSF15 also can be induced in T cells, macrophages, monocytes, and DCs in response to stimulation with immune complexes, Toll-like receptor ligands, inflammatory cytokines, and T-cell receptor activator (99). Current studies mostly focus on the role of TNFSF15 in inflammatory diseases such as SLE and psoriasis (100), its potential roles in HCC yet to be further investigated. Apart from the above immunostimulators, the CXCR4 was associated with both LOX and LOXL3. It is expressed on various pro- and anti-inflammatory immune cells, especially in macrophages and T cells (101). Multiple drugs targeted on CXCR4 have been under investigation (102). AMD3100, also known as plerixafor (Mozobil), was the FDA-approved CXCR4 antagonist used for peripheral blood stem cell transplantation, but its clinical use in LC and other solid tumors is limited due to its poor pharmacokinetics and toxic adverse effects (102, 103). Recently, other CXCR4 antagonists have been developed. For example, BPRCX807 has been experimentally validated in different HCC models (103), and it deserves further investigation. The MHCs, HLA-DOA, HLA-DPA1, and HLA-DPB1, positively associated with LOXL3 all belongs to MHC Class II molecules (104). MHC class II molecules were found to be expressed by antigen-presenting cells, including antigen-presenting cancer-associated fibroblasts (apCAFs) (105, 106). Therefore, these positive association of the MHC Class II molecules might be indirect evidence of apCAFs in HCC, and drugs targeted on these apCAFs might have potential therapeutic values and future studies are needed for further clarification.
This bioinformatic study also acknowledges some limitations: First, as all data were retrieved from online databases, the results still need to be validated with other experiments and cohorts. Second, as this study was mainly aimed at exploring the potential diagnostic, prognostic, and therapeutic values of the LOX family members in LC patients, the details of their mechanisms were not comprehensively explored. Third, most of the samples on the online databases were HCC, therefore their values on other types of LC still need further investigation. Fourth, meta-analysis found that LOXL4 was associated with poor OS, while results from TIMER and UALCAN did not yield the same conclusion. However, only two studies on the survival effect of overexpressed LOXL4 were found, therefore, more studies are urgently needed to validate its effect of the prognosis for LC patients.
Conclusions
This bioinformatics analysis investigated the expression levels, diagnostic and prognostic values, genetic alterations, PPI network, functional enrichment, tumor microenvironment factors, and potential mechanisms of LOX family members in LC. Our results found that all LOX family members are overexpressed in LC tumors, and LOXL2 is good candidate as a diagnostic marker. LOX and LOXL3 are associated with poor prognosis and carry potential as therapeutic targets. The effect of LOXL4 on survival remains equivocal and prompts more studies. The infiltration of a variety of immune cells and a list of immunomodulators were positive correlated with LOX family members. These results highlight the need to explore the roles of LOX family in the tumor microenvironment and their potential as immunotherapeutic targets.
Methods
Analysis of LOX Family Expression Levels
The expression levels of LOX family members between LC and normal tissue were first compared by using the Wilcoxon rank sum test, and visualized by ‘ggplot2’ package of R software version v3.6.3 (The R Foundation for Statistical Computing, 2020). p < 0.05 was considered statistically significant. Data extracted from The Cancer Genome Atlas Liver Hepatocellular Carcinoma (TCGA-LIHC) database (https://portal.gdc.cancer.gov/), and Log2 transformed FPKM (fragments per kilobase exon-model per million reads mapped) were used.
To further verify the expression levels of the 5 members of LOX family between LC tissues and adjoining normal tissues, the difference in transcriptional levels were assessed using students’ t-test through the UALCAN online tool (http://ualcan.path.uab.edu/analysis.html), in which a statistically significant value was defined as p-value < 0.05 (107). These findings were then verified through Tumor Immune Estimation Resource (TIMER) (https://cistrome.shinyapps.io/timer/), an online tool based on data of more than ten-thousand tumors from 32 types of cancer (108, 109).
The optimal discriminate cut-off point between the high and low expression groups was evaluated by the receiver operating characteristic (ROC) curve and area under the curve (AUC) values for overexpressed LOX family members, with data obtained from the TCGA-LIHC database. Log2 transformed FPKM were used for downstream analyses. ROC curve was created by using pROC and ggplot2 packages of R software.
Analysis of Prognostic Value of LOX Family Expression in LC
The prognostic value of LOX family expression was first explored based on the TCGA-LIHC data with Log2 transformed FPKM. We applied the Kaplan-Meier (KM) survival analysis with log-rank test to compare the survival difference between high expression group and low expression group. The KM curves, with p-values and hazard ratio (HR) with 95% confidence interval (CI), were generated by log-rank tests and univariate Cox proportional hazards regression. These calculations were performed using R software with ‘survminer’, and ‘survival’ packages. The results were verified by through the UALCAN online tool (107) and TIMER (108, 109).
A predictive model based on TCGA-LIHC data was also established to predict the mortality risk based on overexpressed members of LOX family and all other potential predictors (110–112). A nomogram using ‘rms’ and ‘survival’ R packages was developed, based on multivariate Cox proportional hazards analysis for predicting the 1,3,5-year overall survival. A graphical representation of potential predicting factors was provided by the nomogram to calculate the risk of mortality for an individual patient. In order to assess the discriminatory performance of the model, C-index was also calculated (112–114).
Analysis of Genetic Mutations of LOX Family in LC
Five datasets, including “TCGA, Firehose Legacy”, “RIKEN, Nat Genet 2012”, “AMC, Hepatology 2014”, “INSERM, Nat Genet 2015”, and “MSK, Clin Cancer Res 2018” were applied to analyze gene mutations of LOX family members via cBioPortal (http://www.cbioportal.org/). cBioPortal is a comprehensive web resource providing visualization, analysis, and download of large-scale cancer genomics data sets (115). The correlation of LOX family members with each other was calculated by analyzing mRNA expressions (RNA sequencing [RNA-seq] version (v.)2 RSEM) in the cBioPortal online tool for Liver Hepatocellular Carcinoma (TCGA, Firehose Legacy). Pearson’s correction was included. TIMER was also used to verify the correlation of LOX family members using the Correlation module (108, 109).
Exploration of Potential Drugs That Are Interacted With LOX Family in LC
Potential drugs that interact with members of the LOX family and demonstrated significant difference in expression and survival between LC and normal tissues were investigated through text mining. Coremine Medical (http://www.coremine.com/medical/) was used to visualize the connections among genes and pathways (116, 117).
Analysis of Interaction of LOX Family Members in LC
Protein-protein interaction (PPI) network analysis was performed on differentially expressed LOX family members and their most significantly interacted proteins via STRING online database (https://string-db.org/) (118) and GeneMANIA (http://www.genemania.org) (119).
GO Enrichment and KEGG Pathway Analysis of LOX Family Members
Functions of LOX family members and their top 20 most associated genes identified from GeneMANIA (119) were analyzed by Gene Ontology (GO) and Kyoto Encyclopedia of Genes and Genomes (KEGG) in the DAVID database (https://david.ncifcrf.gov/summary.jsp) (120, 121). GO enrichment analysis predicted the function based on biological processes (BP), cellular components (CC), and molecular functions (MF), while KEGG analysis determined the related pathways of LOX family members and their associated interactors. The results of GO and KEGG analyses were visualized by the Bioinformatics online tool (http://www.bioinformatics.com.cn) (122, 123). KEGG online web tool (http://www.genome.jp/kegg/), an integrated database for biological interpretation of genome sequences and other large-scale molecular datasets, was also used to verify crucial pathways (123–126).
Immune Cell Infiltration of LOX Family Members in LC
The infiltration of different immune cells and their clinical impact were assessed through TIMER, an online tool for comprehensive molecular characterization of tumor-immune interactions (108, 109). Plots were generated using the Gene module in TIMER, through which we analyzed the correlation between the expression of LOX family members and immune infiltration level in LC. Cutoff value of Cor >0.2 and p<0.05 were used to determine a significant correlation (127, 128). To further explore the interactions between immune system and LOX family members that are associated with poor prognosis, the TISIDB database (http://cis.hku.hk/TISIDB) was used. TISIDB is a web portal for analyzing immune system and tumor interaction, including nearly one thousand reported immune-related anti-tumor genes, etc., and immunological data gathered from seven public databases (129–131). Here, TISIDB was used for exploring the immunomodulators associated with LOX family members in LC.
Association Analysis of Each Member of LOX Family and GSEA Analysis
The LinkedOmics (http://www.linkedomics.org/login.php) is an online tool with multi-omics data from 32 types of cancer based on TCGA (132). LOX family members were screened from the TCGA-LIHC cohort by choosing HiSeq RNA as platform and RNAseq as data type in both search dataset and targe dataset. The genes associated with each member of LOX family member were explored through the LinkFinder module, and the correlation of results was tested by the Pearson correlation coefficient and presented respectively in volcano plot and heat maps. Function module analysis of GO and KEGG pathways were explored by the gene set enrichment analysis (GSEA) in the LinkInterpreter module.
Meta-Analysis of the Prognosis of Overexpressed LOX Family Members in LC
A meta-analysis was performed to verify the results of OS of overexpressed LOX family members in LC. Two authors (S. Mao and Y. Chen) independently searched the potential articles related to LOX family members and LC published until May 2021 via the Cochrane Library, PubMed, Web of Science and CNKI (Chinese National Knowledge Infrastructure). To find all eligible literature, the following search strategy was used: (LOXL1 OR LOXL2 OR LOXL3 OR LOXL4 OR LOX OR lysyl oxidase like 1 OR lysyl oxidase like 2 OR lysyl oxidase like 3 OR lysyl oxidase like 4 OR lysyl oxidase) AND (liver cancer OR hepatocellular carcinoma OR LC OR HCC). Chinese phrases replaced the English terms in the CNKI database. Before conducting this study, we consulted the Preferred Reporting Items declared by the Systematic Review and Meta-Analysis (PRISMA) (133). Then, the strength of associations between LOX family members and OS in LC was evaluated by calculating the combined HRs with the corresponding 95% confidence interval (CI). I2 statistics were used to assess the degree of heterogeneity across the incorporated original studies (134). If I2> 50%, the random-effects model was used to estimate the HR to account for heterogeneity; otherwise, the fixed-effects model was applied (135). In addition, we performed sensitivity analysis by switching between the random-effects model and fixed-effects model and observing for significant differences in the results (136, 137). The above statistical analysis was performed using STATA 15.1. statistical software (Stata Corp., College Station, TX).
Data Availability Statement
The datasets presented in this study can be found in online repositories. The names of the repository/repositories and accession number(s) can be found in the article/supplementary material.
Author Contributions
YZ and CS designed the research study. CS, YC, and SM selected and collected the data. CS, YC, SM, and WG analyzed the data. CS, YC, SM, and NK wrote the manuscript. ZZ participated in the statical analysis. SK, YW, WG, YC, JT, CB, NM, YH, CC, and QZ provided critical opinions and revised the manuscript. All authors read and approved the final manuscript.
Funding
The Training Program Foundation of Youth Scholars by the First Affiliated Hospital of Anhui Medical University (2020kj24).
Conflict of Interest
The authors declare that the research was conducted in the absence of any commercial or financial relationships that could be construed as a potential conflict of interest.
Publisher’s Note
All claims expressed in this article are solely those of the authors and do not necessarily represent those of their affiliated organizations, or those of the publisher, the editors and the reviewers. Any product that may be evaluated in this article, or claim that may be made by its manufacturer, is not guaranteed or endorsed by the publisher.
Acknowledgments
We acknowledge TCGA database for providing their platforms and contributors for uploading their meaningful datasets.
Abbreviations
LC, Liver cancer; HCC, Hepatocellular carcinoma; LOX, lysyl oxidase; LOXL, lysyl oxidase-like; FAK, focal adhesion kinase; BAPN, β-aminopropionitrile; ECM, extracellular matrix; SRCR, scavenger receptor cysteine-rich; TME, tumor microenvironment; ECM, extracellular matrix; MMP, matrix metalloproteinase.
References
1. Sung H, Ferlay J, Siegel RL, Laversanne M, Soerjomataram I, Jemal A, et al. Global Cancer Statistics 2020: GLOBOCAN Estimates of Incidence and Mortality Worldwide for 36 Cancers in 185 Countries. CA Cancer J Clin (2021) 71(3):209–49. doi: 10.3322/caac.21660
2. Tan Z. Recent Advances in the Surgical Treatment of Advanced Gastric Cancer: A Review. Med Sci Monit (2019) 25:3537–41. doi: 10.12659/MSM.916475
3. Wallace MC, Preen D, Jeffrey GP, Adams LA. The Evolving Epidemiology of Hepatocellular Carcinoma: A Global Perspective. Expert Rev Gastroenterol Hepatol (2015) 9(6):765–79. doi: 10.1586/17474124.2015.1028363
4. Chen JG, Zhang SW. Liver Cancer Epidemic in China: Past, Present and Future. Semin Cancer Biol (2011) 21(1):59–69. doi: 10.1016/j.semcancer.2010.11.002
5. Petrizzo A, Caruso FP, Tagliamonte M, Tornesello ML, Ceccarelli M, Costa V, et al. Identification and Validation of HCC-Specific Gene Transcriptional Signature for Tumor Antigen Discovery. Sci Rep (2016) 6:29258. doi: 10.1038/srep29258
6. Niu J, Lin Y, Guo Z, Niu M, Su C. The Epidemiological Investigation on the Risk Factors of Hepatocellular Carcinoma: A Case-Control Study in Southeast China. Med (Baltimore) (2016) 95(6):e2758. doi: 10.1097/MD.0000000000002758
7. Yang JD, Hainaut P, Gores GJ, Amadou A, Plymoth A, Roberts LR. A Global View of Hepatocellular Carcinoma: Trends, Risk, Prevention and Management. Nat Rev Gastroenterol Hepatol (2019) 16(10):589–604. doi: 10.1038/s41575-019-0186-y
8. Zhang G, Kang Z, Mei H, Huang Z, Li H. Promising Diagnostic and Prognostic Value of Six Genes in Human Hepatocellular Carcinoma. Am J Transl Res (2020) 12(4):1239–54.
9. Shirdarreh M, Aziza O, Pezo RC, Jerzak KJ, Warner E. Patients' and Oncologists' Knowledge and Expectations Regarding Tumor Multigene Next-Generation Sequencing: A Narrative Review. Oncologist (2021) 26(8):e1359–71. doi: 10.1002/onco.13783
10. Giunchi F, Franceschini T, Fiorentino M. A Narrative Review of Individualized Treatments of Genitourinary Tumors: Is the Future Brighter With Molecular Evaluations? Transl Androl Urol (2021) 10(3):1553–61. doi: 10.21037/tau-20-1185
11. Levy SE, Myers RM. Advancements in Next-Generation Sequencing. Annu Rev Genomics Hum Genet (2016) 17:95–115. doi: 10.1146/annurev-genom-083115-022413
12. Behjati S, Tarpey PS. What is Next Generation Sequencing? Arch Dis Child Educ Pract Ed (2013) 98(6):236–8. doi: 10.1136/archdischild-2013-304340
13. Li S, Yang R, Sun X, Miao S, Lu T, Wang Y, et al. Identification of SPP1 as a Promising Biomarker to Predict Clinical Outcome of Lung Adenocarcinoma Individuals. Gene (2018) 679:398–404. doi: 10.1016/j.gene.2018.09.030
14. Shen Y, Liu J, Zhang L, Dong S, Zhang J, Liu Y, et al. Identification of Potential Biomarkers and Survival Analysis for Head and Neck Squamous Cell Carcinoma Using Bioinformatics Strategy: A Study Based on TCGA and GEO Datasets. BioMed Res Int (2019) 2019:7376034. doi: 10.1155/2019/7376034
15. Cox TR, Erler JT. Remodeling and Homeostasis of the Extracellular Matrix: Implications for Fibrotic Diseases and Cancer. Dis Model Mech (2011) 4(2):165–78. doi: 10.1242/dmm.004077
16. Fogelgren B, Polgar N, Szauter KM, Ujfaludi Z, Laczko R, Fong KS, et al. Cellular Fibronectin Binds to Lysyl Oxidase With High Affinity and Is Critical for its Proteolytic Activation. J Biol Chem (2005) 280(26):24690–7. doi: 10.1074/jbc.M412979200
17. Stewart GD, Nanda J, Brown DJ, Riddick AC, Ross JA, Habib FK. NO-Sulindac Inhibits the Hypoxia Response of PC-3 Prostate Cancer Cells via the Akt Signalling Pathway. Int J Cancer (2009) 124(1):223–32. doi: 10.1002/ijc.23934
18. Rodriguez HM, Vaysberg M, Mikels A, McCauley S, Velayo AC, Garcia C, et al. Modulation of Lysyl Oxidase-Like 2 Enzymatic Activity by an Allosteric Antibody Inhibitor. J Biol Chem (2010) 285(27):20964–74. doi: 10.1074/jbc.M109.094136
19. Kumari S, Panda TK, Pradhan T. Lysyl Oxidase: Its Diversity in Health and Diseases. Indian J Clin Biochem (2017) 32(2):134–41. doi: 10.1007/s12291-016-0576-7
20. Lucero HA, Ravid K, Grimsby JL, Rich CB, DiCamillo SJ, Maki JM, et al. Lysyl Oxidase Oxidizes Cell Membrane Proteins and Enhances the Chemotactic Response of Vascular Smooth Muscle Cells. J Biol Chem (2008) 283(35):24103–17. doi: 10.1074/jbc.M709897200
21. Erler JT, Giaccia AJ. Lysyl Oxidase Mediates Hypoxic Control of Metastasis. Cancer Res (2006) 66(21):10238–41. doi: 10.1158/0008-5472.CAN-06-3197
22. Miller BW, Morton JP, Pinese M, Saturno G, Jamieson NB, McGhee E, et al. Targeting the LOX/hypoxia Axis Reverses Many of the Features That Make Pancreatic Cancer Deadly: Inhibition of LOX Abrogates Metastasis and Enhances Drug Efficacy. EMBO Mol Med (2015) 7(8):1063–76. doi: 10.15252/emmm.201404827
23. Yang M, Liu J, Wang F, Tian Z, Ma B, Li Z, et al. Lysyl Oxidase Assists Tumorinitiating Cells to Enhance Angiogenesis in Hepatocellular Carcinoma. Int J Oncol (2019) 54(4):1398–408. doi: 10.3892/ijo.2019.4705
24. Zhao W, Yang A, Chen W, Wang P, Liu T, Cong M, et al. Inhibition of Lysyl Oxidase-Like 1 (LOXL1) Expression Arrests Liver Fibrosis Progression in Cirrhosis by Reducing Elastin Crosslinking. Biochim Biophys Acta Mol Basis Dis (2018) 1864(4 Pt A):1129–37. doi: 10.1016/j.bbadis.2018.01.019
25. Lin HY, Li CJ, Yang YL, Huang YH, Hsiau YT, Chu PY. Roles of Lysyl Oxidase Family Members in the Tumor Microenvironment and Progression of Liver Cancer. Int J Mol Sci (2020) 21(24):9751. doi: 10.3390/ijms21249751
26. Wong CC, Tse AP, Huang YP, Zhu YT, Chiu DK, Lai RK, et al. Lysyl Oxidase-Like 2 is Critical to Tumor Microenvironment and Metastatic Niche Formation in Hepatocellular Carcinoma. Hepatology (2014) 60(5):1645–58. doi: 10.1002/hep.27320
27. Tan HY, Wang N, Zhang C, Chan YT, Yuen MF, Feng Y. Lysyl Oxidase-Like 4 Fosters an Immunosuppressive Microenvironment During Hepatocarcinogenesis. Hepatology (2020) 73(6):2326–41. doi: 10.1002/hep.31600
28. Li R, Wang Y, Zhang X, Feng M, Ma J, Li J, et al. Exosome-Mediated Secretion of LOXL4 Promotes Hepatocellular Carcinoma Cell Invasion and Metastasis. Mol Cancer (2019) 18(1):18. doi: 10.1186/s12943-019-0948-8
29. Tian M, Liu W, Jin L, Jiang X, Yang L, Ding Z, et al. LOXL4 Is Downregulated in Hepatocellular Carcinoma With a Favorable Prognosis. Int J Clin Exp Pathol (2015) 8(4):3892–900.
30. Zhu J, Huang S, Wu G, Huang C, Li X, Chen Z, et al. Lysyl Oxidase Is Predictive of Unfavorable Outcomes and Essential for Regulation of Vascular Endothelial Growth Factor in Hepatocellular Carcinoma. Dig Dis Sci (2015) 60(10):3019–31. doi: 10.1007/s10620-015-3734-5
31. Ninomiya G, Yamada S, Hayashi M, Takeda S, Suenaga M, Takami H, et al. Significance of Lysyl Oxidaselike 2 Gene Expression on the Epithelialmesenchymal Status of Hepatocellular Carcinoma. Oncol Rep (2018) 39(6):2664–72. doi: 10.3892/or.2018.6349
32. Wei S, Gao L, Wu C, Qin F, Yuan J. Role of the Lysyl Oxidase Family in Organ Development (Review). Exp Ther Med (2020) 20(1):163–72. doi: 10.3892/etm.2020.8731
33. Molnar J, Fong KS, He QP, Hayashi K, Kim Y, Fong SF, et al. Structural and Functional Diversity of Lysyl Oxidase and the LOX-Like Proteins. Biochim Biophys Acta (2003) 1647(1-2):220–4. doi: 10.1016/S1570-9639(03)00053-0
34. Iturbide A, García de Herreros A, Peiró S. A New Role for LOX and LOXL2 Proteins in Transcription Regulation. FEBS J (2015) 282(9):1768–73. doi: 10.1111/febs.12961
35. Shao B, Zhao X, Liu T, Zhang Y, Sun R, Dong X, et al. LOXL2 Promotes Vasculogenic Mimicry and Tumour Aggressiveness in Hepatocellular Carcinoma. J Cell Mol Med (2019) 23(2):1363–74. doi: 10.1111/jcmm.14039
36. Xiao Q, Ge G. Lysyl Oxidase, Extracellular Matrix Remodeling and Cancer Metastasis. Cancer Microenviron (2012) 5(3):261–73. doi: 10.1007/s12307-012-0105-z
37. López B, González A, Hermida N, Valencia F, de Teresa E, Díez J. Role of Lysyl Oxidase in Myocardial Fibrosis: From Basic Science to Clinical Aspects. Am J Physiol Heart Circ Physiol (2010) 299(1):H1–9. doi: 10.1152/ajpheart.00335.2010
38. Smith-Mungo LI, Kagan HM. Lysyl Oxidase: Properties, Regulation and Multiple Functions in Biology. Matrix Biol (1998) 16(7):387–98. doi: 10.1016/S0945-053X(98)90012-9
39. Ye M, Song Y, Pan S, Chu M, Wang ZW, Zhu X. Evolving Roles of Lysyl Oxidase Family in Tumorigenesis and Cancer Therapy. Pharmacol Ther (2020) 215:107633. doi: 10.1016/j.pharmthera.2020.107633
40. Yang M, Liu J, Wang F, Tian Z, Ma B, Li Z, et al. Lysyl Oxidase Assists Tumor−Initiating Cells to Enhance Angiogenesis in Hepatocellular Carcinoma. Int J Oncol (2019) 54(4):1398–408. doi: 10.3892/ijo.2019.4705
41. Ribeiro AL, Kaid C, Silva PBG, Cortez BA, Okamoto OK. Inhibition of Lysyl Oxidases Impairs Migration and Angiogenic Properties of Tumor-Associated Pericytes. Stem Cells Int (2017) 2017:4972078. doi: 10.1155/2017/4972078
42. Kasashima H, Yashiro M, Okuno T, Miki Y, Kitayama K, Masuda G, et al. Significance of the Lysyl Oxidase Members Lysyl Oxidase Like 1, 3, and 4 in Gastric Cancer. Digestion (2018) 98(4):238–48. doi: 10.1159/000489558
43. Wang N, Zhou X, Tang F, Wang X, Zhu X. Identification of LOXL3-Associating Immune Infiltration Landscape and Prognostic Value in Hepatocellular Carcinoma. Virchows Arch (2021) 479(6):1153–65. doi: 10.1007/s00428-021-03193-4
44. Shao J, Lu J, Zhu W, Yu H, Jing X, Wang YL, et al. Derepression of LOXL4 Inhibits Liver Cancer Growth by Reactivating Compromised P53. Cell Death Differ (2019) 26(11):2237–52. doi: 10.1038/s41418-019-0293-x
45. Madden MZ, Rathmell JC. The Complex Integration of T-Cell Metabolism and Immunotherapy. Cancer Discov (2021) 11(7):1636–43. doi: 10.1158/2159-8290.CD-20-0569
46. Ferreira S, Saraiva N, Rijo P, Fernandes AS. LOXL2 Inhibitors and Breast Cancer Progression. Antioxidants (Basel) (2021) 10(2):312. doi: 10.3390/antiox10020312
47. Li Q, Zhu CC, Ni B, Zhang ZZ, Jiang SH, Hu LP, et al. Lysyl Oxidase Promotes Liver Metastasis of Gastric Cancer via Facilitating the Reciprocal Interactions Between Tumor Cells and Cancer Associated Fibroblasts. EBioMedicine (2019) 49:157–71. doi: 10.1016/j.ebiom.2019.10.037
48. Grau-Bové X, Ruiz-Trillo I, Rodriguez-Pascual F. Origin and Evolution of Lysyl Oxidases. Sci Rep (2015) 5:10568. doi: 10.1038/srep10568
49. Noda K, Kitagawa K, Miki T, Horiguchi M, Akama TO, Taniguchi T, et al. A Matricellular Protein Fibulin-4 Is Essential for the Activation of Lysyl Oxidase. Sci Adv (2020) 6(48):eabc1404. doi: 10.1126/sciadv.abc1404
50. Mohseni R, Arab Sadeghabadi Z, Goodarzi MT, Karimi J. Co-Administration of Resveratrol and Beta-Aminopropionitrile Attenuates Liver Fibrosis Development via Targeting Lysyl Oxidase in CCl4-Induced Liver Fibrosis in Rats. Immunopharmacol Immunotoxicol (2019) 41(6):644–51. doi: 10.1080/08923973.2019.1688829
51. Shah R, Chan KKW. The Impact of Socioeconomic Status on Stage at Presentation, Receipt of Diagnostic Imaging, Receipt of Treatment and Overall Survival in Colorectal Cancer Patients. Int J Cancer (2021) 49(5):1031–43. doi: 10.1002/ijc.33622
52. Viswanadh MK, Agrawal N, Azad S, Jha A, Poddar S, Mahto SK, et al. Novel Redox-Sensitive Thiolated TPGS Based Nanoparticles for EGFR Targeted Lung Cancer Therapy. Int J Pharm (2021) 602:120652. doi: 10.1016/j.ijpharm.2021.120652
53. Conforti F, Davies ER, Calderwood CJ, Thatcher TH, Jones MG, Smart DE, et al. The Histone Deacetylase Inhibitor, Romidepsin, as a Potential Treatment for Pulmonary Fibrosis. Oncotarget (2017) 8(30):48737–54. doi: 10.18632/oncotarget.17114
54. Perrone AM, Ravegnini G, Miglietta S, Argnani L, Ferioli M, De Crescenzo E, et al. Electrochemotherapy in Vulvar Cancer and Cisplatin Combined With Electroporation. Systematic Review and In Vitro Studies. Cancers (Basel) (2021) 13(9):1993. doi: 10.3390/cancers13091993
55. Lee J, You JH, Shin D, Roh JL. Ex Vivo Culture of Head and Neck Cancer Explants in Cell Sheet for Testing Chemotherapeutic Sensitivity. J Cancer Res Clin Oncol (2020) 146(10):2497–507. doi: 10.1007/s00432-020-03306-7
56. Saboormaleki S, Sadeghian H, Bahrami AR, Orafaie A, Matin MM. 7-Farnesyloxycoumarin Exerts Anti-Cancer Effects on a Prostate Cancer Cell Line by 15-LOX-1 Inhibition. Arch Iran Med (2018) 21(6):251–9.
57. Sterzynska K, Klejewski A, Wojtowicz K, Swierczewska M, Nowacka M, Kazmierczak D, et al. Mutual Expression of ALDH1A1, LOX, and Collagens in Ovarian Cancer Cell Lines as Combined CSCs- and ECM-Related Models of Drug Resistance Development. Int J Mol Sci (2018) 20(1):54. doi: 10.3390/ijms20010054
58. Gao Y, Liu T, Liu X, Wu C. Preparation of Paclitaxel-Folic Acid Functionalized Gelatin Grafted Mesoporous Hollow Carbon Nanospheres for Enhancing Antitumor Effects Toward Liver Cancer (SMMC-7721) Cell Lines. J Biomater Appl (2020) 34(8):1071–80. doi: 10.1177/0885328219896457
59. Li Y, Tang T, Lee HJ, Song K. Selective Anti-Cancer Effects of Plasma-Activated Medium and Its High Efficacy With Cisplatin on Hepatocellular Carcinoma With Cancer Stem Cell Characteristics. Int J Mol Sci (2021) 22(8):3956. doi: 10.3390/ijms22083956
60. Csiszar K. Lysyl Oxidases: A Novel Multifunctional Amine Oxidase Family. Prog Nucleic Acid Res Mol Biol (2001) 70:1–32. doi: 10.1016/S0079-6603(01)70012-8
61. Schmelzer CEH, Heinz A, Troilo H, Lockhart-Cairns MP, Jowitt TA, Marchand MF, et al. Lysyl Oxidase-Like 2 (LOXL2)-Mediated Cross-Linking of Tropoelastin. FASEB J (2019) 33(4):5468–81. doi: 10.1096/fj.201801860RR
62. Oliver AJ, Lau PKH, Unsworth AS, Loi S, Darcy PK, Kershaw MH, et al. Tissue-Dependent Tumor Microenvironments and Their Impact on Immunotherapy Responses. Front Immunol (2018) 9:70. doi: 10.3389/fimmu.2018.00070
63. Hanahan D, Coussens LM. Accessories to the Crime: Functions of Cells Recruited to the Tumor Microenvironment. Cancer Cell (2012) 21(3):309–22. doi: 10.1016/j.ccr.2012.02.022
64. Buoncervello M, Gabriele L, Toschi E. The Janus Face of Tumor Microenvironment Targeted by Immunotherapy. Int J Mol Sci (2019) 20(17):4320. doi: 10.3390/ijms20174320
65. Zhang T, Nie Y, Gu J, Cai K, Chen X, Li H, et al. Identification of Mitochondrial-Related Prognostic Biomarkers Associated With Primary Bile Acid Biosynthesis and Tumor Microenvironment of Hepatocellular Carcinoma. Front Oncol (2021) 11:587479. doi: 10.3389/fonc.2021.587479
66. Li W, Wu H, Xu X, Zhang Y. Comprehensive Analysis of Genomic and Immunological Profiles in Chinese and Western Hepatocellular Carcinoma Populations. Aging (Albany NY) (2021) 13(8):11564–94. doi: 10.18632/aging.202853
67. Davis LN, Sherbenou DW. Emerging Therapeutic Strategies to Overcome Drug Resistance in Multiple Myeloma. Cancers (Basel) (2021) 13(7):1686. doi: 10.3390/cancers13071686
68. Maes K, Mondino A, Lasarte JJ, Agirre X, Vanderkerken K, Prosper F, et al. Epigenetic Modifiers: Anti-Neoplastic Drugs With Immunomodulating Potential. Front Immunol (2021) 12:652160. doi: 10.3389/fimmu.2021.652160
69. Pitt JM, Marabelle A, Eggermont A, Soria JC, Kroemer G, Zitvogel L. Targeting the Tumor Microenvironment: Removing Obstruction to Anticancer Immune Responses and Immunotherapy. Ann Oncol (2016) 27(8):1482–92. doi: 10.1093/annonc/mdw168
70. Kalinski P, Talmadge JE. Tumor Immuno-Environment in Cancer Progression and Therapy. Adv Exp Med Biol (2017) 1036:1–18. doi: 10.1007/978-3-319-67577-0_1
71. Roma-Rodrigues C, Mendes R, Baptista PV, Fernandes AR. Targeting Tumor Microenvironment for Cancer Therapy. Int J Mol Sci (2019) 20(4):840. doi: 10.3390/ijms20040840
72. Bagaev A, Kotlov N, Nomie K, Svekolkin V, Gafurov A, Isaeva O, et al. Conserved Pan-Cancer Microenvironment Subtypes Predict Response to Immunotherapy. Cancer Cell (2021) 39(6):845–65.e7. doi: 10.1016/j.ccell.2021.04.014
73. Sanmamed MF, Nie X, Desai SS, Villaroel-Espindola F, Badri T, Zhao D, et al. A Burned-Out CD8+ T-Cell Subset Expands in the Tumor Microenvironment and Curbs Cancer Immunotherapy. Cancer Discov (2021) 11(7):1700–15. doi: 10.1158/2159-8290.CD-20-0962
74. Li HW, Tang SL. Colony Stimulating Factor-1 and its Receptor in Gastrointestinal Malignant Tumors. J Cancer (2021) 12(23):7111–9. doi: 10.7150/jca.60379
75. Vaynrub A, Healey JH, Tap W, Vaynrub M. Pexidartinib in the Management of Advanced Tenosynovial Giant Cell Tumor: Focus on Patient Selection and Special Considerations. Onco Targets Ther (2022) 15:53–66. doi: 10.2147/OTT.S345878
76. Braun S, McSheehy P, Litherland K, McKernan P, Forster-Gross N, Bachmann F, et al. Derazantinib: An Investigational Drug for the Treatment of Cholangiocarcinoma. Expert Opin Investig Drugs (2021) 30(11):1071–80. doi: 10.1080/13543784.2021.1995355
77. Lee J, Han Y, Wang W, Jo H, Kim H, Kim S, et al. Phytochemicals in Cancer Immune Checkpoint Inhibitor Therapy. Biomolecules (2021) 11(8):1107. doi: 10.3390/biom11081107
78. Burugu S, Dancsok AR, Nielsen TO. Emerging Targets in Cancer Immunotherapy. Semin Cancer Biol (2018) 52(Pt 2):39–52. doi: 10.1016/j.semcancer.2017.10.001
79. Anderson AC. Tim-3: An Emerging Target in the Cancer Immunotherapy Landscape. Cancer Immunol Res (2014) 2(5):393–8. doi: 10.1158/2326-6066.CIR-14-0039
80. Petersen SH, Kua LF, Nakajima S, Yong WP, Kono K. Chemoradiation Induces Upregulation of Immunogenic Cell Death-Related Molecules Together With Increased Expression of PD-L1 and Galectin-9 in Gastric Cancer. Sci Rep (2021) 11(1):12264. doi: 10.1038/s41598-021-91603-7
81. Thomas DA, Massagué J. TGF-Beta Directly Targets Cytotoxic T Cell Functions During Tumor Evasion of Immune Surveillance. Cancer Cell (2005) 8(5):369–80. doi: 10.1016/j.ccr.2005.10.012
82. de Streel G, Lucas S. Targeting Immunosuppression by TGF-β1 for Cancer Immunotherapy. Biochem Pharmacol (2021) 192:114697. doi: 10.1016/j.bcp.2021.114697
83. Batlle E, Massagué J. Transforming Growth Factor-β Signaling in Immunity and Cancer. Immunity (2019) 50(4):924–40. doi: 10.1016/j.immuni.2019.03.024
84. Rodon J, Carducci MA, Sepulveda-Sánchez JM, Azaro A, Calvo E, Seoane J, et al. First-In-Human Dose Study of the Novel Transforming Growth Factor-β Receptor I Kinase Inhibitor LY2157299 Monohydrate in Patients With Advanced Cancer and Glioma. Clin Cancer Res (2015) 21(3):553–60. doi: 10.1158/1078-0432.CCR-14-1380
85. Melisi D, Garcia-Carbonero R, Macarulla T, Pezet D, Deplanque G, Fuchs M, et al. Galunisertib Plus Gemcitabine vs. Gemcitabine for First-Line Treatment of Patients With Unresectable Pancreatic Cancer. Br J Cancer (2018) 119(10):1208–14. doi: 10.1038/s41416-018-0246-z
86. Ni L, Dong C. New Checkpoints in Cancer Immunotherapy. Immunol Rev (2017) 276(1):52–65. doi: 10.1111/imr.12524
87. John P, Wei Y, Liu W, Du M, Guan F, Zang X. The B7x Immune Checkpoint Pathway: From Discovery to Clinical Trial. Trends Pharmacol Sci (2019) 40(11):883–96. doi: 10.1016/j.tips.2019.09.008
88. Bolandi N, Derakhshani A, Hemmat N, Baghbanzadeh A, Asadzadeh Z, Afrashteh Nour M, et al. The Positive and Negative Immunoregulatory Role of B7 Family: Promising Novel Targets in Gastric Cancer Treatment. Int J Mol Sci (2021) 22(19):10719. doi: 10.3390/ijms221910719
89. Weinmann A, Galle PR. Role of Immunotherapy in the Management of Hepatocellular Carcinoma: Current Standards and Future Directions. Curr Oncol (2020) 27(Suppl 3):S152–64. doi: 10.3747/co.27.7315
90. Hengeveld PJ, Kersten MJ. B-Cell Activating Factor in the Pathophysiology of Multiple Myeloma: A Target for Therapy? Blood Cancer J (2015) 5(2):e282. doi: 10.1038/bcj.2015.3
91. Navarra SV, Guzmán RM, Gallacher AE, Hall S, Levy RA, Jimenez RE, et al. Efficacy and Safety of Belimumab in Patients With Active Systemic Lupus Erythematosus: A Randomised, Placebo-Controlled, Phase 3 Trial. Lancet (2011) 377(9767):721–31. doi: 10.1016/S0140-6736(10)61354-2
92. Etxeberria I, Glez-Vaz J, Teijeira Á, Melero I. New Emerging Targets in Cancer Immunotherapy: CD137/4-1BB Costimulatory Axis. ESMO Open (2020) 4(Suppl 3):e000733. doi: 10.1136/esmoopen-2020-000733
93. Chester C, Ambulkar S, Kohrt HE. 4-1BB Agonism: Adding the Accelerator to Cancer Immunotherapy. Cancer Immunol Immunother (2016) 65(10):1243–8. doi: 10.1007/s00262-016-1829-2
94. Chu DT, Bac ND, Nguyen KH, Tien NLB, Thanh VV, Nga VT, et al. An Update on Anti-CD137 Antibodies in Immunotherapies for Cancer. Int J Mol Sci (2019) 20(8):1822. doi: 10.3390/ijms20081822
95. Croft M. The Role of TNF Superfamily Members in T-Cell Function and Diseases. Nat Rev Immunol (2009) 9(4):271–85. doi: 10.1038/nri2526
96. Lin WW, Hsieh SL. Decoy Receptor 3: A Pleiotropic Immunomodulator and Biomarker for Inflammatory Diseases, Autoimmune Diseases and Cancer. Biochem Pharmacol (2011) 81(7):838–47. doi: 10.1016/j.bcp.2011.01.011
97. Yang CR, Hsieh SL, Teng CM, Ho FM, Su WL, Lin WW. Soluble Decoy Receptor 3 Induces Angiogenesis by Neutralization of TL1A, a Cytokine Belonging to Tumor Necrosis Factor Superfamily and Exhibiting Angiostatic Action. Cancer Res (2004) 64:1122–9. doi: 10.1158/0008-5472.CAN-03-0609
98. Chew LJ, Pan H, Yu J, Tian S, Huang WQ, Zhang JY, et al. A Novel Secreted Splice Variant of Vascular Endothelial Cell Growth Inhibitor. FASEB J (2002) 16:742–4. doi: 10.1096/fj.01-0757fje
99. Bayry J. Immunology: TL1A in the Inflammatory Network in Autoimmune Diseases. Nat Rev Rheumatol (2010) 6:67–8. doi: 10.1038/nrrheum.2009.263
100. Croft M, Siegel RM. Beyond TNF: TNF Superfamily Cytokines as Targets for the Treatment of Rheumatic Diseases. Nat Rev Rheumatol (2017) 13(4):217–33. doi: 10.1038/nrrheum.2017.22
101. Schottelius M, Herrmann K, Lapa C. In Vivo Targeting of CXCR4-New Horizons. Cancers (Basel) (2021) 13(23):5920. doi: 10.3390/cancers13235920
102. Hu F, Miao L, Zhao Y, Xiao YY, Xu Q. A Meta-Analysis for C-X-C Chemokine Receptor Type 4 as a Prognostic Marker and Potential Drug Target in Hepatocellular Carcinoma. Drug Des Devel Ther (2015) 9:3625–33. doi: 10.2147/DDDT.S86032
103. Song JS, Chang CC, Wu CH, Dinh TK, Jan JJ, Huang KW, et al. A Highly Selective and Potent CXCR4 Antagonist for Hepatocellular Carcinoma Treatment. Proc Natl Acad Sci USA (2021) 118(13):e2015433118. doi: 10.1073/pnas.2015433118
104. Doxiadis GG, Otting N, de Groot NG, Bontrop RE. Differential Evolutionary MHC Class II Strategies in Humans and Rhesus Macaques: Relevance for Biomedical Studies. Immunol Rev (2001) 183:76–85. doi: 10.1034/j.1600-065x.2001.1830106.x
105. Huang M, Dong J. Critical Roles of Balanced T Helper 9 Cells and Regulatory T Cells in Allergic Airway Inflammation and Tumor Immunity. J Immunol Res (2021) 2021:8816055. doi: 10.1155/2021/8816055
106. Geng X, Chen H, Zhao L, Hu J, Yang W, Li G, et al. Cancer-Associated Fibroblast (CAF) Heterogeneity and Targeting Therapy of CAFs in Pancreatic Cancer. Front Cell Dev Biol (2021) 9:655152. doi: 10.3389/fcell.2021.655152
107. Chandrashekar DS, Bashel B, Balasubramanya SAH, Creighton CJ, Ponce-Rodriguez I, Chakravarthi B, et al. UALCAN: A Portal for Facilitating Tumor Subgroup Gene Expression and Survival Analyses. Neoplasia (2017) 19(8):649–58. doi: 10.1016/j.neo.2017.05.002
108. Li T, Fan J, Wang B, Traugh N, Chen Q, Liu JS, et al. TIMER: A Web Server for Comprehensive Analysis of Tumor-Infiltrating Immune Cells. Cancer Res (2017) 77(21):e108–e10. doi: 10.1158/0008-5472.CAN-17-0307
109. Li B, Severson E, Pignon JC, Zhao H, Li T, Novak J, et al. Comprehensive Analyses of Tumor Immunity: Implications for Cancer Immunotherapy. Genome Biol (2016) 17(1):174. doi: 10.1186/s13059-016-1028-7
110. Iasonos A, Schrag D, Raj GV, Panageas KS. How to Build and Interpret a Nomogram for Cancer Prognosis. J Clin Oncol (2008) 26(8):1364–70. doi: 10.1200/JCO.2007.12.9791
111. Balachandran VP, Gonen M, Smith JJ, DeMatteo RP. Nomograms in Oncology: More Than Meets the Eye. Lancet Oncol (2015) 16(4):e173–80. doi: 10.1016/S1470-2045(14)71116-7
112. Liu J, Lichtenberg T, Hoadley KA, Poisson LM, Lazar AJ, Cherniack AD, et al. An Integrated TCGA Pan-Cancer Clinical Data Resource to Drive High-Quality Survival Outcome Analytics. Cell (2018) 173(2):400–16.e11. doi: 10.1016/j.cell.2018.02.052
113. Kramer AA, Zimmerman JE. Assessing the Calibration of Mortality Benchmarks in Critical Care: The Hosmer-Lemeshow Test Revisited. Crit Care Med (2007) 35(9):2052–6. doi: 10.1097/01.CCM.0000275267.64078.B0
114. Pencina MJ, D'Agostino RB. Overall C as a Measure of Discrimination in Survival Analysis: Model Specific Population Value and Confidence Interval Estimation. Stat Med (2004) 23(13):2109–23. doi: 10.1002/sim.1802
115. Gao J, Aksoy BA, Dogrusoz U, Dresdner G, Gross B, Sumer SO, et al. Integrative Analysis of Complex Cancer Genomics and Clinical Profiles Using the Cbioportal. Sci Signal (2013) 6(269):pl1. doi: 10.1126/scisignal.2004088
116. de Leeuw N, Dijkhuizen T, Hehir-Kwa JY, Carter NP, Feuk L, Firth HV, et al. Diagnostic Interpretation of Array Data Using Public Databases and Internet Sources. Hum Mutat (2012) 33(6):930–40. doi: 10.1002/humu.22049
117. Chu H, Zhou X, Liu G, Lv M, Zhou X, Wang Y, et al. Network-Based Detection of Disease Modules and Potential Drug Targets in Intractable Epilepsy. 2014 8th Int Conf Syst Biol (ISB) (2014) 132–40. doi: 10.1145/2554850
118. Szklarczyk D, Gable AL, Lyon D, Junge A, Wyder S, Huerta-Cepas J, et al. STRING V11: Protein-Protein Association Networks With Increased Coverage, Supporting Functional Discovery in Genome-Wide Experimental Datasets. Nucleic Acids Res (2019) 47(D1):D607–13. doi: 10.1093/nar/gky1131
119. Vlasblom J, Zuberi K, Rodriguez H, Arnold R, Gagarinova A, Deineko V, et al. Novel Function Discovery With GeneMANIA: A New Integrated Resource for Gene Function Prediction in Escherichia Coli. Bioinformatics (2015) 31(3):306–10. doi: 10.1093/bioinformatics/btu671
120. Huang da W, Sherman BT, Lempicki RA. Bioinformatics Enrichment Tools: Paths Toward the Comprehensive Functional Analysis of Large Gene Lists. Nucleic Acids Res (2009) 37(1):1–13. doi: 10.1093/nar/gkn923
121. Huang da W, Sherman BT, Lempicki RA. Systematic and Integrative Analysis of Large Gene Lists Using DAVID Bioinformatics Resources. Nat Protoc (2009) 4(1):44–57. doi: 10.1038/nprot.2008.211
122. Zhang MM, Wang D, Lu F, Zhao R, Ye X, He L, et al. Identification of the Active Substances and Mechanisms of Ginger for the Treatment of Colon Cancer Based on Network Pharmacology and Molecular Docking. BioData Min (2021) 14(1):1. doi: 10.1186/s13040-020-00232-9
123. Wang T, Feng Y, Wang H, Huo G, Cai Y, Wang L, et al. The Mechanisms of Sijunzi Decoction in the Treatment of Chronic Gastritis Revealed by Network Pharmacology. Evid Based Complement Alternat Med (2020) 2020:8850259. doi: 10.1155/2020/8850259
124. Kanehisa M, Furumichi M, Tanabe M, Sato Y, Morishima K. KEGG: New Perspectives on Genomes, Pathways, Diseases and Drugs. Nucleic Acids Res (2017) 45(D1):D353–D61. doi: 10.1093/nar/gkw1092
125. Kanehisa M, Sato Y, Furumichi M, Morishima K, Tanabe M. New Approach for Understanding Genome Variations in KEGG. Nucleic Acids Res (2019) 47(D1):D590–D5. doi: 10.1093/nar/gky962
126. Kanehisa M, Sato Y. KEGG Mapper for Inferring Cellular Functions From Protein Sequences. Protein Sci (2020) 29(1):28–35. doi: 10.1002/pro.3711
127. Liu S, Geng R, Lin E, Zhao P, Chen Y. ERBB1/2/3 Expression, Prognosis, and Immune Infiltration in Cutaneous Melanoma. Front Genet (2021) 12:602160. doi: 10.3389/fgene.2021.602160
128. Zhong L, Yang Z, Lei D, Li L, Song S, Cao D, et al. Bromodomain 4 Is a Potent Prognostic Marker Associated With Immune Cell Infiltration in Breast Cancer. Basic Clin Pharmacol Toxicol (2021) 128(1):169–82. doi: 10.1111/bcpt.13481
129. Xu H, Zou R, Li F, Liu J, Luan N, Wang S, et al. MRPL15 is a Novel Prognostic Biomarker and Therapeutic Target for Epithelial Ovarian Cancer. Cancer Med (2021) 10(11):3655–73. doi: 10.1002/cam4.3907
130. He M, Han Y, Cai C, Liu P, Chen Y, Shen H, et al. CLEC10A is a Prognostic Biomarker and Correlated With Clinical Pathologic Features and Immune Infiltrates in Lung Adenocarcinoma. J Cell Mol Med (2021) 25(7):3391–9. doi: 10.1111/jcmm.16416
131. Ding Y, Liu N, Chen M, Xu Y, Fang S, Xiang W, et al. Overexpressed Pseudogene MT1L Associated With Tumor Immune Infiltrates and Indicates a Worse Prognosis in BLCA. World J Surg Oncol (2021) 19(1):133. doi: 10.1186/s12957-021-02231-4
132. Vasaikar SV, Straub P, Wang J, Zhang B. LinkedOmics: Analyzing Multi-Omics Data Within and Across 32 Cancer Types. Nucleic Acids Res (2018) 46(D1):D956–63. doi: 10.1093/nar/gkx1090
133. Page MJ, McKenzie JE, Bossuyt PM, Boutron I, Hoffmann TC, Mulrow CD, et al. The PRISMA 2020 Statement: An Updated Guideline for Reporting Systematic Reviews. J Clin Epidemiol (2021) 134:178–89. doi: 10.1016/j.jclinepi.2021.02.003
134. Higgins JP, Thompson SG. Quantifying Heterogeneity in a Meta-Analysis. Stat Med (2002) 21(11):1539–58. doi: 10.1002/sim.1186
135. DerSimonian R, Laird N. Meta-Analysis in Clinical Trials Revisited. Contemp Clin Trials (2015) 45(Pt A):139–45. doi: 10.1016/j.cct.2015.09.002
136. Borenstein M, Hedges LV, Higgins JP, Rothstein HR. A Basic Introduction to Fixed-Effect and Random-Effects Models for Meta-Analysis. Res Synth Methods (2010) 1(2):97–111. doi: 10.1002/jrsm.12
Keywords: liver cancer, lysyl oxidase, bioinformatic analysis, receiver operating curve, nomogram, prognostic value, immune infiltration
Citation: Sun C, Ma S, Chen Y, Kim NH, Kailas S, Wang Y, Gu W, Chen Y, Tuason JPW, Bhan C, Manem N, Huang Y, Cheng C, Zhou Z, Zhou Q and Zhu Y (2022) Diagnostic Value, Prognostic Value, and Immune Infiltration of LOX Family Members in Liver Cancer: Bioinformatic Analysis. Front. Oncol. 12:843880. doi: 10.3389/fonc.2022.843880
Received: 27 December 2021; Accepted: 08 February 2022;
Published: 04 March 2022.
Edited by:
Alessandro Passardi, Scientific Institute of Romagna for the Study and Treatment of Tumors (IRCCS), ItalyReviewed by:
Shih-Min Hsia, Taipei Medical University, TaiwanCarolina Añazco, Universidad San Sebastián, Chile
Copyright © 2022 Sun, Ma, Chen, Kim, Kailas, Wang, Gu, Chen, Tuason, Bhan, Manem, Huang, Cheng, Zhou, Zhou and Zhu. This is an open-access article distributed under the terms of the Creative Commons Attribution License (CC BY). The use, distribution or reproduction in other forums is permitted, provided the original author(s) and the copyright owner(s) are credited and that the original publication in this journal is cited, in accordance with accepted academic practice. No use, distribution or reproduction is permitted which does not comply with these terms.
*Correspondence: Yanzhe Zhu, ZHJ6aHV5YW56aGVAMTI2LmNvbQ==
†These authors have contributed equally to this work