- 1Department of Cardiothoracic Surgery, The Third Affiliated Hospital of Sun Yat-sen University, Guangzhou, China
- 2Department of Clinical Laboratory, Sun Yat-sen University Cancer Center, State Key Laboratory of Oncology in South China, Collaborative Innovation Center for Cancer Medicine, Guangzhou, China
- 3Department of Microbial and Biochemical Pharmacy, School of Pharmaceutical Sciences, Sun Yat-sen University, Guangzhou, China
Methyltransferase-like 3 (METTL3) is an RNA methyltransferase mediating N6 methyladenosine (m6A) modification. Its role in cancer pathogenesis and progression has attracted increasing attention. However, the immunological role, possible immune mechanism, and clinical significance of METTL3 in esophageal squamous cell carcinoma (ESCC) remain to be confirmed. The Tumor Genome Atlas (TCGA) provided clinical and transcriptome sequencing data for this study (162 tumor tissue samples and 11 normal tissue samples), while the Immunology Database and Analysis Portal (immport, https://www.immport.org/home) provided 2483 immune-related genes. METTL3 was substantially expressed in ESCC and linked to poor prognosis in ESCC, according to the findings. Functional analysis revealed that METTL3 is mainly involved in chromosomal homologous recombination and DNA mismatch repair processes, which could be potential mechanisms for tumor disease development and progression. Analysis on the TISIDB website shows that effector memory CD8 T cells, NK cells, neutrophils and other cells are highly correlated with METTL3 expression. We screened immune genes associated with METTL3 by Spearman’s analysis and performed functional analysis. These immune genes were mostly linked with immune processes, such as cytokine receptors, the MAPK signaling pathway, and natural killer cell-mediated cytotoxicity, indicating that METTL3 is a key molecule in the immune regulation of esophageal cancer. In addition, based on METTL3-related immune genes, we separated the patients into several subgroups and constructed a prognostic prediction model consisting of six immune genes. As an independent prognostic indicator for ESCC, the risk score of this model can be employed. A nomogram was also developed to accurately evaluate individual prognoses based on clinical indicators and risk scores. In summary, this study suggests that METTL3 is not only a potential pathogenic molecule for esophageal carcinogenesis and progression but also a potential biological marker for forecasting ESCC patient prognosis and could serve as a basis for clinical decision making.
Introduction
Esophageal carcinoma (EC) is among the most prevalent upper gastrointestinal tract malignancies worldwide. It is the eighth most frequent cancer worldwide, as well as the sixth leading cause of cancer-related mortality, and the incidence rate is still rising (1–3). In East Asia, especially in China, the incidence rate of EC is very high, and one-third to one-half of EC cases around the world originate in China (4). The most common histological type of EC in China is esophageal squamous cell carcinoma (ESCC), which accounts for 90% of all EC cases (5, 6).
Epigenetics is a field of biology that has rapidly developed in recent years. Its roles in tumor formation and progression have been increasingly recognized (7–9). Epigenetic regulation mechanisms mainly include RNA modification, DNA modification, histone posttranslational modifications, and chromatin remodeling. Epigenetic regulation of gene expression is a dynamic and reversible process (10–12). In biological and pathological processes, the related modifications are crucial for interpreting environmental signals and altering the expression of related genes (13, 14). When these genes are dysregulated, diseases such as ESCC can occur (15–19).
Of more than 170 known RNA modifications, the most widespread mRNA modification, N6-methyladenosine (m6A), has an impact on practically every stage of RNA metabolism involving splicing, decay, export, and translation (20, 21). M6A is dynamically installed and deleted by writer and eraser enzymes, and it acts by recognizing and binding to m6A reader proteins. Methyltransferase-like 3 (METTL3) is the main methyltransferase critical for m6A methylation (22, 23). METTL3 dysregulation has already been widely reported in a wide range of tumor types, such as lung cancer, colorectal cancer and breast cancer (24–28). However, there are still few studies of the immune function and mechanism of METTL3 in ESCC.
In this research, the RNA sequencing data and the clinical information of ESCC patients were acquired from the Cancer Genome Atlas (TCGA). METTL3 gene expression and function (especially immune function) were analyzed. The screened immune-related prognostic genes were also used in consensus clustering analysis and least absolute shrinkage and selection operator (LASSO) regression analysis to build a tumor immune subtype and risk score model to predict ESCC patient prognosis.
Materials and Methods
Acquisition of Data and Tissue Specimens
The TCGA database provides ESCC patient gene expression patterns and clinical data (https://cancergenome.nih.gov/) (29). The dataset included information about 162 tumor tissue samples and 11 normal tissue samples. Additionally, from the Immunology Database and Analysis Portal (IMMPORT, https://www.immport.org/home), 2483 immune-related genes were imported and used for further immunological research (30).
At the Third Affiliated Hospital of Sun Yat-sen University from 2019 to 2021, four pairs of tumor and adjacent tissues were collected. The stage of each sample was confirmed by pathological examination, as stated by the American Joint Commission on Cancer (AJCC) cancer staging manual (8th Edition). For biological research, written informed consent was acquired from the patient or guardian. All of the experiments were authorized by the ethical committee of Sun Yat-sen University’s Third Affiliated Hospital.
Bioinformatic Analysis
Wilcoxon’s signed-rank test was applied for the differential expression analysis of METTL3 and immune cells (using the limma package). The prognostic value of METTL3, the risk score model and the nomogram were expounded by survival analysis (Kaplan-Meier method and log-rank test, using the survival and survminer packages) and receiver operating characteristic (ROC) curve analysis (using the time ROC package). Analysis of whether METTL3 and METTL3-related immune genes were associated with patient prognosis was conducted using Cox regression (univariate and multivariate, using the survival package). The role of METTL3 in ESCC was analyzed using gene set enrichment analysis (GSEA, http://www.gsea-msigdb.org/gsea/index.jsp) (31, 32).
The infiltration level of immune cells was calculated using the CIBERSORT RNA deconvolution algorithm (using the e1071 and preprocessCore packages) (33, 34). Using Pearson’s test, we analyzed the correlation of different immune cells and screened METTL3-related immune genes. In addition, the TISIDB database (http://cis.hku.hk/TISIDB) was utilized to screen immune cells closely linked to METTL3 (35). The biological pathways of METTL3-related genes were further assessed by Gene Ontology (GO) and Kyoto Encyclopedia of Genes and Genomes (KEGG) gene enrichment analyses (using the enrichplot and clusterProfiler packages, respectively) (36–39). The Search Tool for the Retrieval of Interacting Genes/Proteins database (STRING, https://cn.string-db.org/) was used to study gene interactions (40, 41)
The patients with ESCC were divided into different subgroups by consensus clustering analysis (using the ConsensusClusterPlus package) (42, 43). Principal component analysis (PCA) was used to verify the significance of typing (using scatterplot3d packages) (44, 45). The risk score model was created by least absolute shrinkage and selection operator (LASSO) Cox regression analysis (using the glmnet package) (46). A nomogram was generated by combining the risk score with various clinical data (using the RMS package) (47, 48).
Cell Culture
The American Type Culture Collection (ATCC) provided Eca109 cells. Cells were grown in RPMI 1640 medium (Invitrogen, USA). All of the media were mixed with 10% fetal bovine serum (FBS, Gibco, USA), and all of the cells were incubated with 5% CO2 at 37°C.
Establishment of Stable Cell Lines
pcDNA/METTL3 or a vector control was used to establish stable cell lines. Stable Eca109 cell lines that overexpressed METTL3 were generated by lentiviral transduction in the presence of 1 μg/mL polybrene (Sigma, USA). METTL3 stable knockdown involves short hairpin RNA expressed from a lentiviral vector. Lentiviral particles generated by the transfection of the second-generation sh-METTL3 transfer vector plasmid, packaging plasmid psPAX2 and envelope plasmid pMDG.2 (3:2:1) into a lentiviral vector-producing cell line were used to transduce Eca109 cells. Empty vector was used as a control. The cells were transduced in the presence of 1 μg/mL polybrene (Sigma, USA), selected with puromycin, and expanded to produce a stable cell line. The target sequence of the shRNA was as follows: shMETTL3: GCCAAGGAACAATCCATTGTT.
Western Blotting Analysis
Cellular proteins were separated using 10% SDS–PAGE, transferred to polyvinylidene difluoride membranes and probed with antibodies against METTL3 (1:2,000, CST). Anti-rabbit antibodies coupled with horseradish peroxidase (HRP) (1:1,000, BOSTER) were used as secondary antibodies. Specific antibodies were used to measure GAPDH protein levels (1:5,000, CST).
Wound Healing Assay
To generate a confluent monolayer, cells were plated in 60-mm dishes. Then, a 10μl pipette tip was used to scratch the cell monolayer in a straight line. The plate was washed gently twice before being incubated at 37°C with 5% CO2 in 1640 supplemented with 1% FBS. The outcomes were observed at 0 and 48 hours with a microscope. Each test was performed three times.
Cell Migration Assay
Transwell chambers (Corning, USA) were utilized to test cell migration. In the upper chamber, Eca109 cells (1 × 105) were cultured in serum-free media, while in the lower chamber, 20% FBS was added to RPMI 1640 medium. Invasive cells in the lower chambers were fixed with 4% paraformaldehyde and stained with 0.05% crystal violet (Sigma-Aldrich) after being incubated for 24 hours at 37°C. Five random fields of cells were counted under an inverted microscope (Leica DMI4000B, Germany).
Histological Immunohistochemical Assays
At 4°C overnight, tissue slices were treated with primary antibodies against METTL3 (1:50, CST). After washing with PBST, an HRP-conjugated anti-rabbit secondary antibody (1:5,000, BOSTER) was applied to the sections at room temperature for 1 h. Before counterstaining with 10% Mayer’s hematoxylin, the sections were reacted with 3-diaminobenzidine tetrahydrochloride for 10 s. Two experienced pathologists analyzed the IHC results. To quantify the percentage of positively stained cells versus the total number of tumor cells, visual fields (×400 magnification) were selected.
Statistical Analysis
For data analysis and statistics, R software (version 3.6.3; the R Foundation for Statistical Computing) and SPSS software (version 23.0; IBM Corp, New York, USA) were used. Student’s t test or Wilcoxon’s signed-rank test was utilized to perform statistical comparisons between groups. The Kaplan-Meier method and log-rank test were used for survival analysis. Receiver operating characteristic (ROC) curve analysis was used to check performance. Prognostic variables were screened using Cox regression (univariate and multivariate) analysis. For correlation analysis, Pearson’s test was utilized. The CIBERSORTR deconvolution algorithm was utilized to calculate the degree of immune cell infiltration. Consensus clustering analysis was utilized to create subtypes. The risk score model was created using LASSO regression analysis. A P value less than 0.05 was considered significant.
Results
METTL3 Is Overexpressed in ESCC
First, we analyzed the differential expression of METTL3 across cancer datasets. METTL3 expression was considerably higher in liver cancer, cholangiocarcinoma, colon cancer, and other tumor tissues than in normal tissues (*P < 0.05, **P < 0.01, ***P < 0.001 and ****P < 0.0001; -, not significant, Figure 1A). Then, ESCC METTL3 expression was analyzed. The results revealed that ESCC tissues showed a high level of METTL3 expression (P = 7.7e-05, Figure 1B). The same result was found in the paired samples (P = 0.001, Figure 1C). Immunohistochemical staining and western blotting were utilized to examine METTL3 expression in ESCC tissues (n = 4) and normal tissues (n = 4). METTL3 expression was particularly high in tumor tissues (Figures 1D, E).
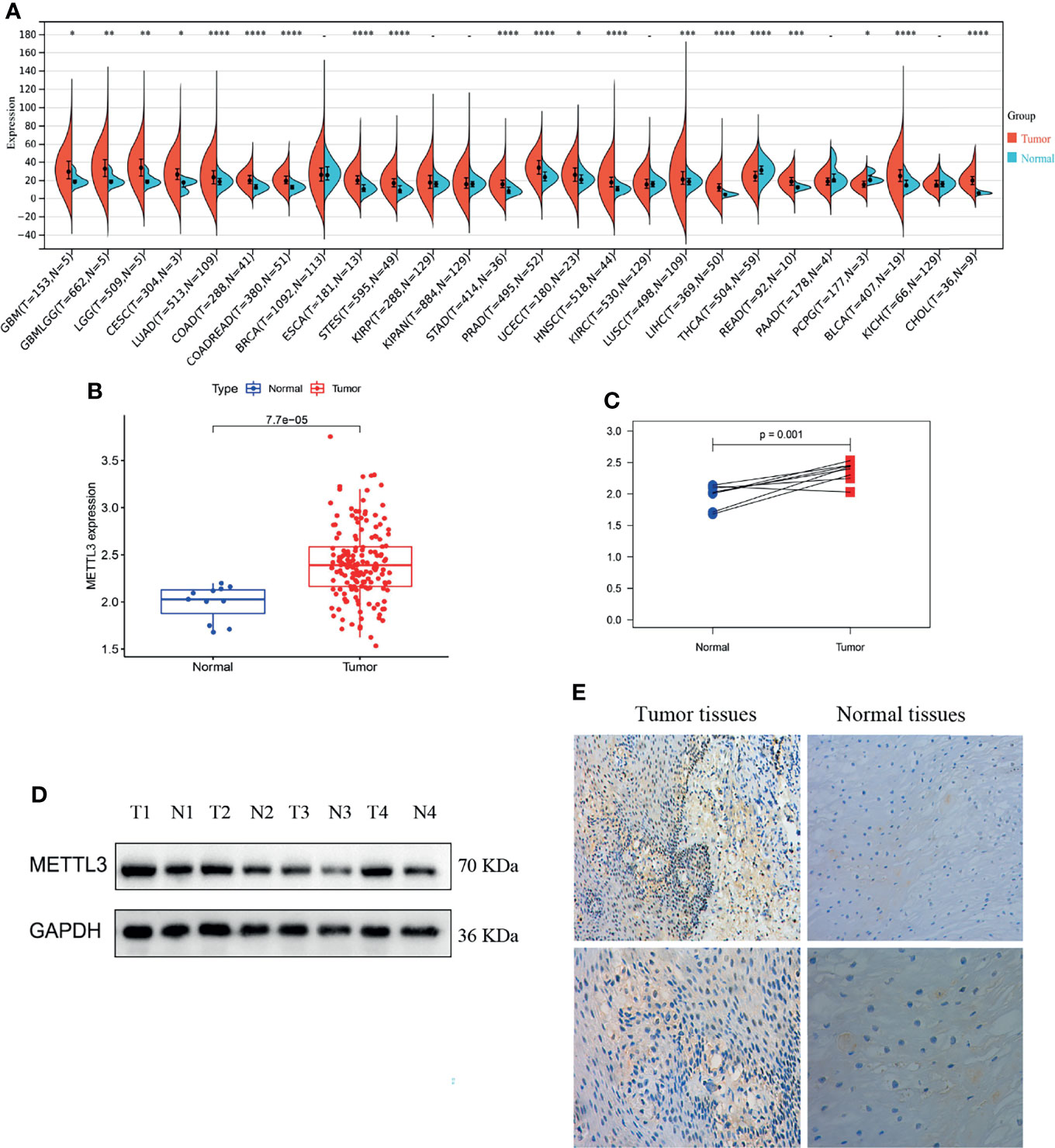
Figure 1 METTL3 was overexpressed in tumor tissues. (A–C) Differential expression of METTL3 between tumor tissues and normal tissues (the normal tissue is represented by blue, whereas the tumor tissue is shown in red; *P < 0.05, **P < 0.01, ***P < 0.001 and ****P < 0.0001. -, not significant). (D, E) Immunohistochemical staining and western blotting of ESCC tissues (n = 4) and adjacent tissues (n = 4).
High METTL3 Expression Can Promote ESCC Progression
Based on the median value of METTL3 expression, ESCC patients were divided into two groups: high expression and low expression. After analyzing the PFS (progression-free survival) of patients, the Kaplan-Meier algorithm was adopted. The findings revealed that the PFS of METTL3-overexpressing patients was markedly lower than that of low METTL3-expressing patients (P = 0.014, Figure 2A), indicating that METTL3-overexpressing patients have a poorer prognosis.
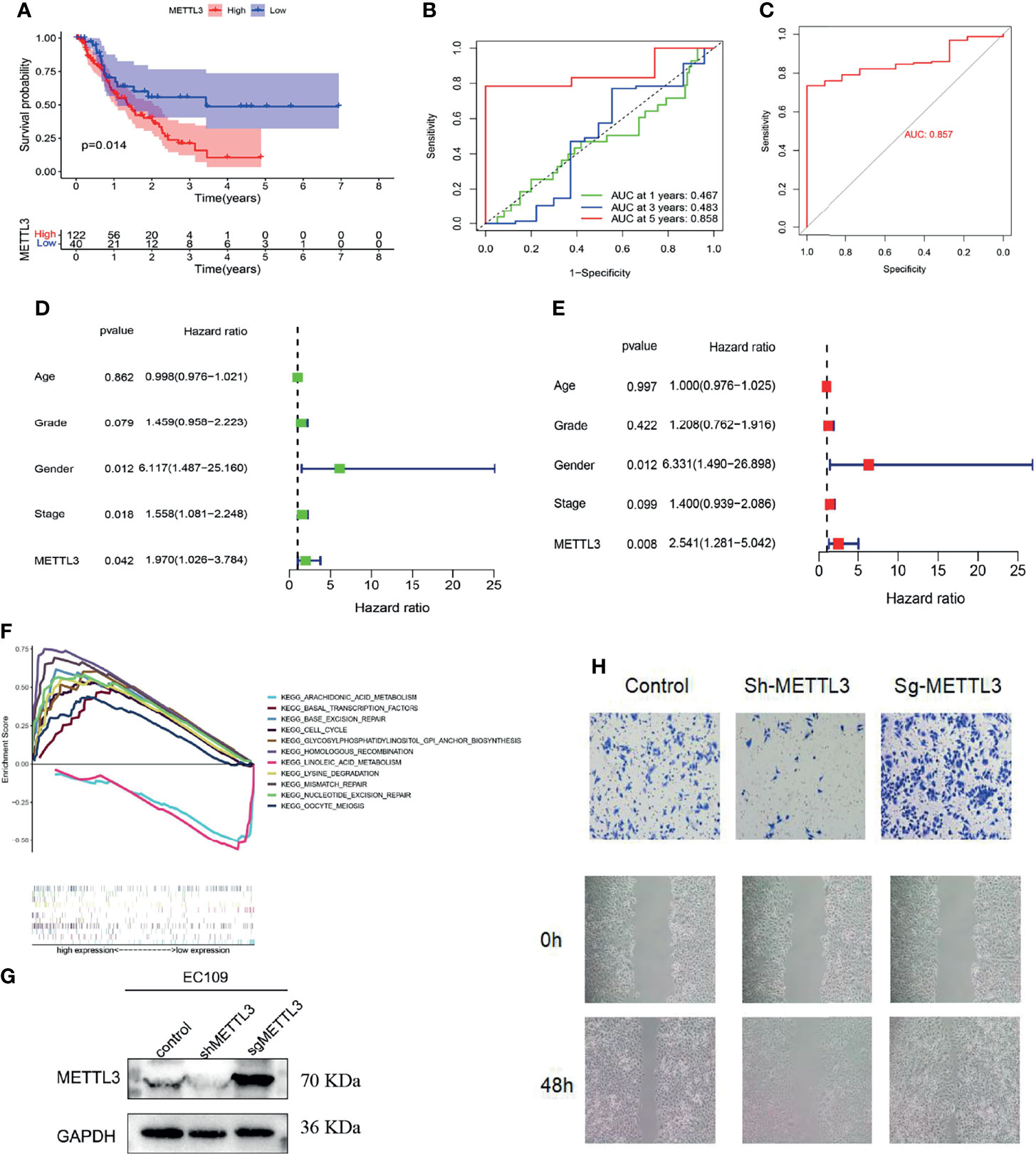
Figure 2 Function and clinical significance of METTL3 in ESCC. (A) PFS analysis of METTL3 in the high and low expression groups (P = 0.014). (B, C) ROC curve analysis to evaluate the prognostic performance (AUC = 0.858) and diagnostic performance (AUC = 0.857) of METTL3 in ESCC. (D, E) Cox regression (univariate and multivariate) analysis indicated that METTL3 could be utilized as a prognostic marker for ESCC (using PFS data, P = 0.008, HR = 2.541). (F) Analysis of METTL3 functionality through GSEA. (G, H) METTL3’s migration ability was identified using wound healing and cell migration assays.
According to ROC curve analysis, METTL3 not only had high accuracy in predicting the 5-year survival of patients with ESCC (area under the curve AUC = 0.858, Figure 2B) but also had important guiding significance for the diagnosis (AUC = 0.857, Figure 2C). Cox regression (univariate and multivariate) analysis also indicated that METTL3 could be utilized as a prognostic marker for ESCC (using PFS data, P = 0.008, HR = 2.541, Figures 2D, E). Furthermore, the gene function differences between the high and low METTL3 expression groups were examined by Gene Set Enrichment Analysis (GSEA). We discovered that METTL3 is primarily implicated in chromosomal homologous recombination and DNA mismatch repair, which could be potential mechanisms for the occurrence and progression of tumor diseases (Figure 2F). The wound healing assay and cell migration assay of Eca109 cells also showed that METTL3 overexpression could promote the migration of ESCC cells, while low METTL3 expression inhibited the occurrence of these conditions (Figures 2G, H).
ESCC Can Be Infiltrated by a Variety of Immune Cells, Some of Which Are METTL3-Related
The infiltration levels of 21 immune cells in tumors were calculated by the CIBERSORT method. The analysis revealed a high degree of macrophage, T cell, and B cell infiltration (Figure 3A). Subsequently, the correlation analysis revealed that immune cells had varying degrees of correlation (Figure 3B). Comparing immune cell levels in the tumor and normal groups, the results showed that plasma cells (P = 0.016), CD8 T cells (P = 0.026), follicular helper T cells (P = 0.005), M0 macrophages (P < 0.001), M1 macrophages (p = 0.008), activated dendritic cells (P < 0.001), resting mast cells (P < 0.001), and neutrophils (P = 0.025) were significantly differentially enriched (Figures 3C, D).
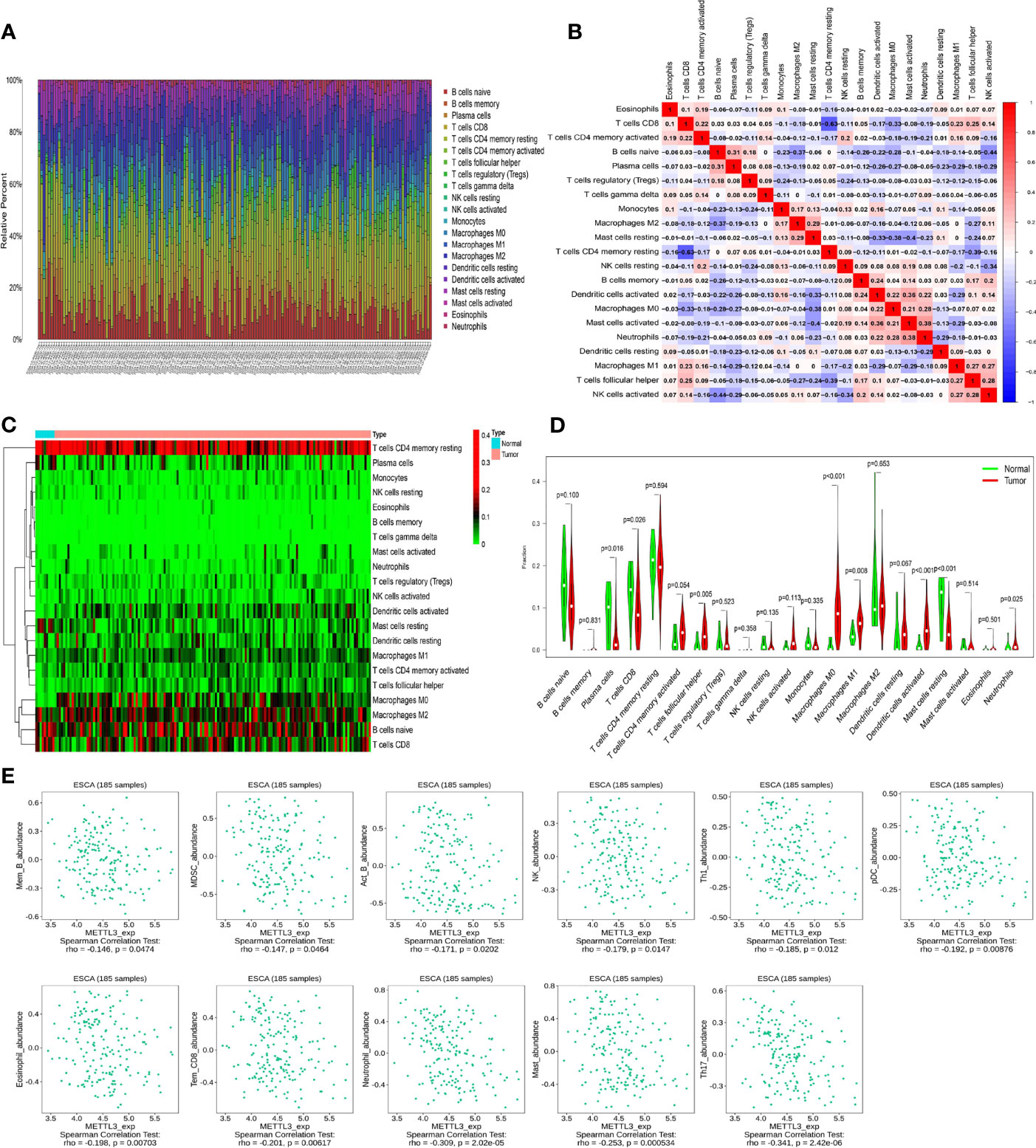
Figure 3 Analysis of immune cell infiltration and METTL3-related immune cells in ESCC. (A) The degree of immune cell infiltration in esophageal cancer (different colors represent different immune cells). (B) Correlation analysis between different immune cells (red and blue represent positive and negative correlations, respectively, and correlation coefficients are expressed numerically). (C, D) The differential enrichment of immune cells in ESCC and normal tissues. (E) Correlation between METTL3 and immune cells.
In addition, immune cells closely linked to METTL3 were screened using the TISIDB database (http://cis.hku.hk/TISIDB). The results revealed that effector memory CD8 T cells (P = 0.00617), NK cells (P = 0.0147), neutrophils (P = 2.02e-05) and other cells were highly correlated with METTL3 expression (Figure 3E), indicating that the METTL3 gene could have a crucial impact on the cellular immune regulation process of ESCC.
METTL3 Has a Critical Function in ESCC Immune Modulation
To understand how the METTL3 gene regulates the immune system in ESCC, we analyzed the correlations between the METTL3 gene and immune genes in the ImmPort database. As a result, 261 highly related immune genes were screened (P < 0.05) (Supplementary Table 1). The Gene Ontology terms (GO terms) of 261 immune genes demonstrated that these genes were mostly expressed in the cytoplasm, related to receptor ligand activity and signal receptor activator activity, and they participated in biological processes such as cytokine secretion, lymphocyte activation and immune effect process regulation (Figures 4A, B). Kyoto Encyclopedia of Genes and Genomes (KEGG) analysis showed that immunomodulatory processes, such as cytokine–cytokine receptor interaction, the MAPK signaling pathway and natural killer cell-mediated cytotoxicity, were also related to these genes (Figures 4C, D).
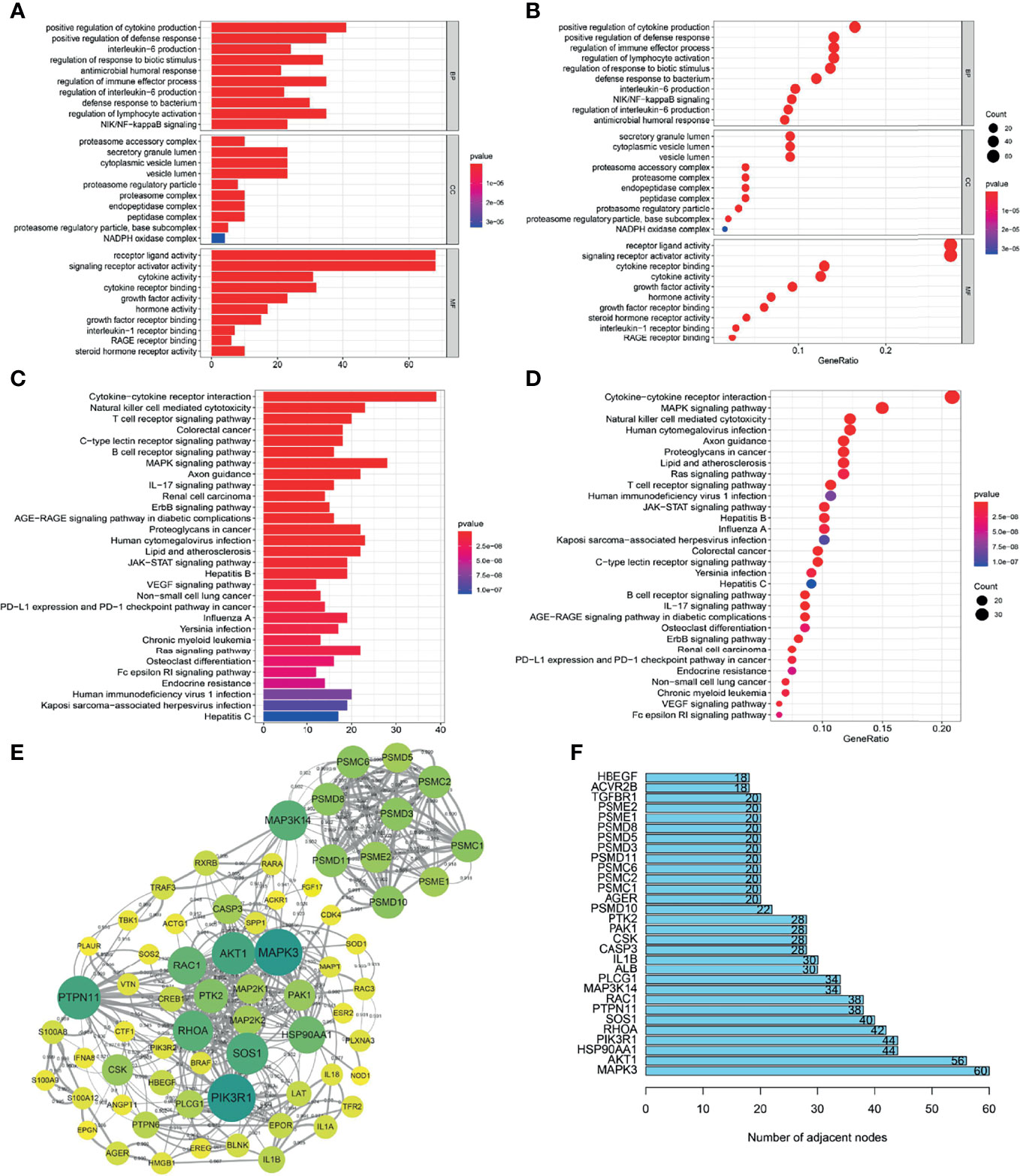
Figure 4 Screening and functional analysis of immune genes highly related to METTL3. (A, B) GO analysis of METTL3-related immune genes. (C, D) KEGG analysis of METTL3-related immune genes. (E) Protein-protein interaction network analysis of METTL3-related immune genes (high confidence 0.700; only some core genes were displayed; the larger the circle, the darker the color, representing the more adjacent nodes). (F) The core genes of the protein-protein interaction network (showing only the top 30).
The STRING website (https://cn.string-db.org/) was applied to examine the protein interaction relationships of these immune genes. We obtained a protein interaction network diagram (Figure 4E). In addition, the number of connection nodes of each protein was counted to clarify the core protein of the protein interaction network. The results showed that MAPK3, AKT1, HSP90AA1, PIK3R1 and RHOA were the most important core genes (Figure 4F). According to the above analysis, METTL3 has a significant impact on the immune regulation of ESCC.
ESCC Can Be Classified Into Three Immune Subtypes With Different Prognoses
We explored the clinical significance of immune genes highly related to METTL3 by Cox univariate analysis and identified 9 immune genes related to prognosis (PSMC6, TBK1, AHNAK, PSMD10, AKT1, JAG2, CTF1, PTH2, RBP2) (Figure 5A). Then, these genes overlapped with the core genes of the protein interaction network, and 6 genes (PSMC6, TBK1, AHNAK, PSMD10, AKT1 and CTF1) were finally obtained (Figure 5B and Supplementary Table 2). Using the ConsensusClusterPlus package and K-means (KM) algorithm, consensus clustering analysis according to the 6 genes was performed. As a result of the expression of the 6 genes, the samples were divided into three distinct subtypes: A, B, and C (Figures 5C–F). Principal component analysis (PCA) showed significantly distinct subtypes (Figure 5G). Overall survival (OS) analysis of the three subtypes showed that patients with types A and B had a better prognosis than those with type C (P = 0.001, Figure 5H). In addition, the thermogram of tumor subtypes showed a correlation between patient survival status and subtype (P < 0.05, Figure 5I). The above analysis showed that tumor typing according to 6 immune genes has guiding significance for patient prognosis evaluation.
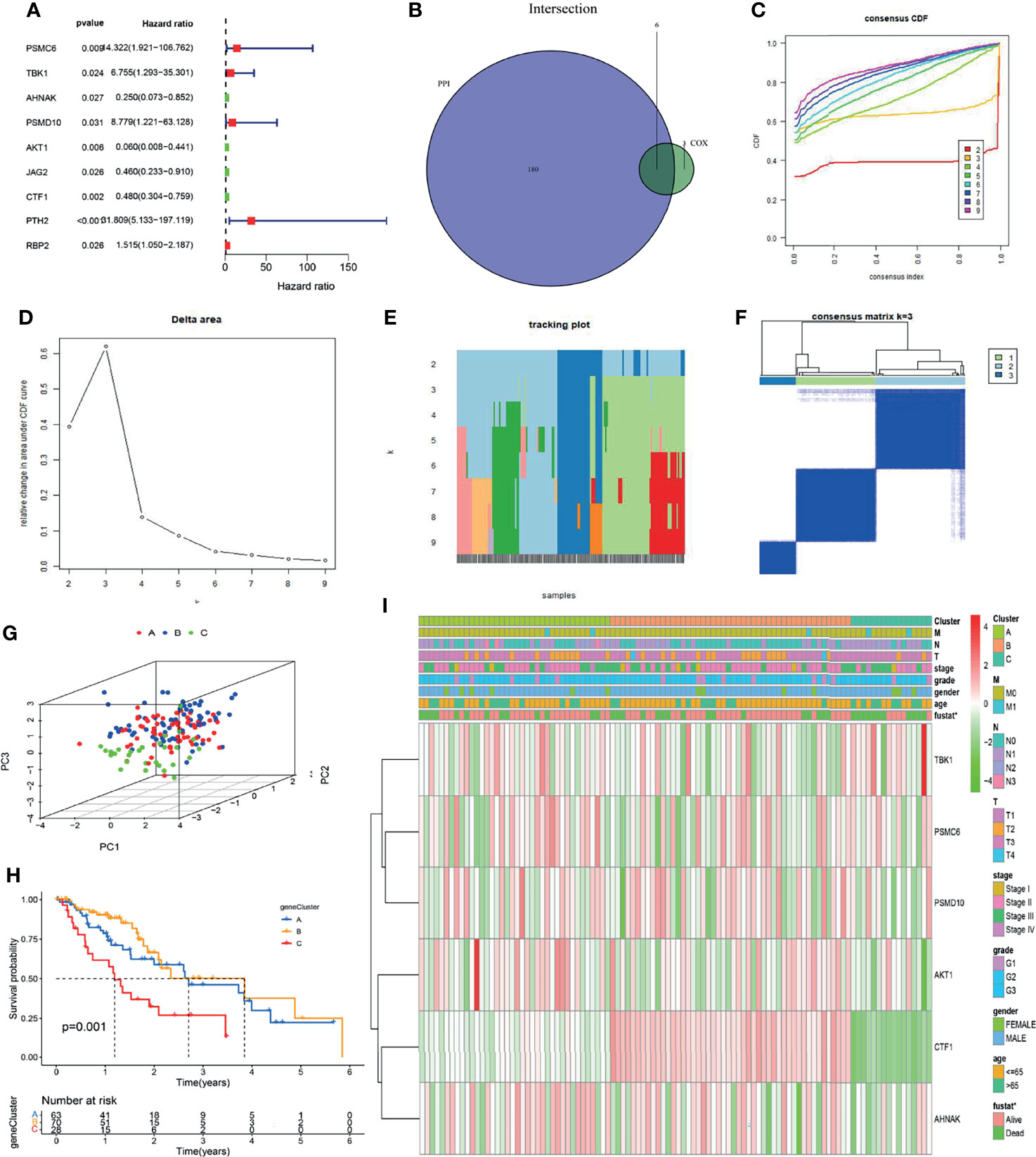
Figure 5 Six immune genes were screened and tumors were classified into three immune subtypes. (A) Using univariate Cox analysis, 9 immunological genes associated with prognosis were identified. (B) The intersection between 180 PPI network core genes and 9 immune genes was selected, and 6 immune genes were screened. (C–F) Based on 6 immune genes, ESCC could be classified into three immune subtypes by consensus clustering analysis. (G) Principal component analysis (PCA) showed that the tumors were well typed. (H) OS analysis of patients with different subtypes (P = 0.001). (I) Gene expression analysis and clinical correlation analysis of different subtypes (*P < 0.05).
The Risk Score Model Was Used to Identify ESCC Patients With Poor Prognosis
Based on the above 6 immune genes related to prognosis, LASSO regression analysis was used to create a risk scoring model that worked best when all 6 immune genes were included in the model. The risk coefficient of each immune-related prognostic gene was calculated, and the risk scoring equation was obtained: risk score = 2.05 * PSMC6 + 0.69 * PSMD10 + 0.38 * TBK1-0.65 * CTF1-1.01 * AHNAK-2.79 * AKT1. The risk scoring model was established (Figures 6A, B).
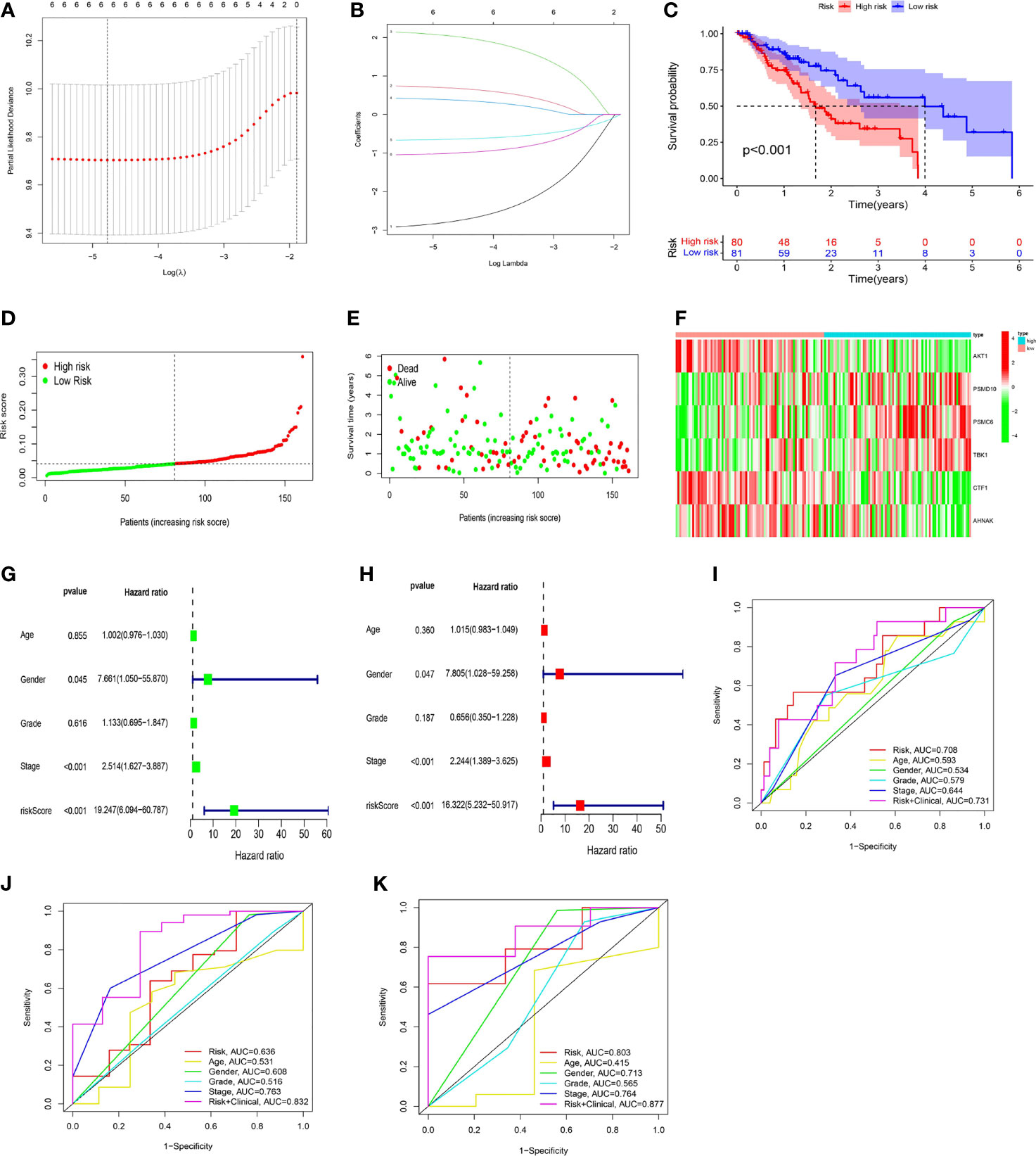
Figure 6 The development of a risk scoring model. (A, B) LASSO regression analysis was used to create a risk scoring model that worked best when all 6 immune genes were included in the model. (C) The low-risk group had a higher overall survival rate than the high-risk group (P < 0.001). (D, E) On the risk curve, a higher risk score indicated a shorter survival time and a higher mortality rate. (F) In the high-risk group, the expression levels of PSMD10, PSMC6, and TBK1 were upregulated, and the expression levels of AKT1, CTF1, and AHNAK were downregulated. (G, H) Cox regression (univariate and multivariate) analysis indicated that gender (P = 0.047, HR = 7.805), stage (P < 0.001, HR = 2.244), and risk score (P < 0.001, HR = 16.322) could be utilized as independent prognostic markers for ESCC patients (using OS data). (I–K) The risk score’s accuracy in predicting prognosis was assessed using ROC curves (I: 1-year; J: 3-year; K: 5-year).
The median risk score was used to categorize ESCC patients into high-risk and low-risk groups. Regarding the overall survival (OS) curve, the low-risk group had a higher survival rate (P < 0.001, Figure 6C). Regarding the risk curve, a higher risk score indicated a shorter survival time and a higher mortality rate (Figures 6D, E). In addition, PSMC6, PSMD10, and TBK1 were highly expressed in the high-risk group, while CTF1, AHNAK, and AKT1 were highly expressed in the low-risk group, according to the gene expression heatmap (Figure 6F).
Cox regression (univariate and multivariate) analysis also indicated that gender (P = 0.047, HR = 7.805), stage (P < 0.001, HR = 2.244), and risk score (P < 0.001, HR = 16.322) could be utilized as prognostic markers for ESCC patients (using OS data, Figures 6G, H). ROC curve analysis showed that, when the risk score was combined with age, gender, grade, stage and other indicators to comprehensively evaluate the prognosis of EC patients, it had high accuracy. The 1-year ROC curve had an AUC of 0.731 (Figure 6I), the 3-year ROC curve had an AUC of 0.832 (Figure 6J), and the 5-year ROC curve had an AUC of 0.877 (Figure 6K), showing a gradual improvement in accuracy.
A Nomogram Can Reliably Predict the Prognosis of ESCC Patients
To more accurately assess the prognosis of patients with ESCC, the risk score and clinical characteristics were used to create a nomogram. The various elements were scored and summed to produce a total score, and patient survival was predicted based on the total score (Figure 7A). In addition, the nomogram’s application ability was validated by calibration curves and ROC curves.
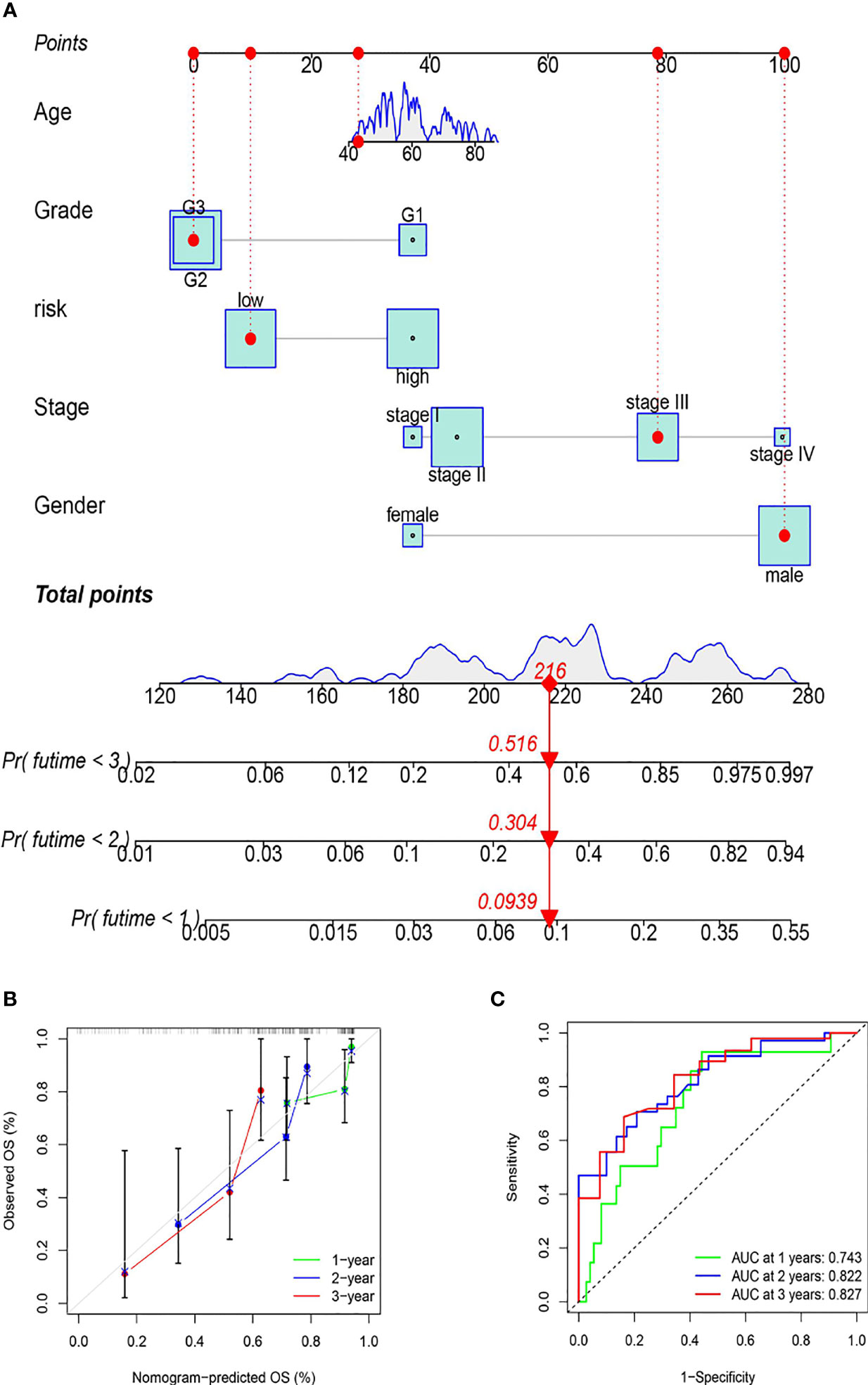
Figure 7 The establishment of the nomogram. (A) Prognostic prediction mechanism of the nomogram (the various elements were scored and summed to produce a total score, and patient survival was predicted based on the total score). (B) The nomogram’s accuracy in predicting prognosis was validated by calibration curves. (C) The nomogram’s accuracy in predicting prognosis was assessed using ROC curve analysis.
As shown by the calibration curves, the prognosis prediction of the nomogram for patients at 1, 2, and 3 years is close to the observation, showing that the model has high accuracy (Figure 7B). As shown by the ROC curves, the 1-year ROC curve had an AUC of 0.743, the 2-year ROC curve had an AUC of 0.822, and the 3-year ROC curve had an AUC of 0.827, proving the high accuracy of the nomogram (Figure 7C). The results of this study revealed that the nomogram can reliably predict the prognosis of ESCC patients.
Discussion
ESCC is an invasive disease with high malignancy potential and a dismal prognosis. The treatment of esophageal cancer mainly includes surgery, radiotherapy, chemotherapy, targeted therapy and so on. Despite recent advances in the treatment of esophageal cancer, the prognosis for esophageal cancer remains relatively poor (49, 50). In recent years, immunotherapy for EC has attracted increasing attention, and various clinical studies have been performed (51, 52). However, immunotherapy for EC always leads to mixed results, partly due to the lack of reliable markers for predicting treatment response (53). In the last several years, an increasing number of studies have been devoted to identifying differential biomarker candidates, especially immune-related genes (54).
Methyltransferase-like 3 (METTL3) is an RNA methyltransferase that mediates N6 methyladenosine (m6A) modification. Its role in cancer pathogenesis and progression has attracted increasing attention (24, 55). However, the immunological role, possible immune mechanism, and clinical significance of METTL3 in esophageal squamous cell carcinoma (ESCC) remain to be confirmed.
Therefore, it is necessary to analyze this topic and understand the immune function and molecular mechanism of METTL3 in esophageal cancer to explore whether METTL3 could become a new biomarker for immunotherapy of esophageal cancer and provide a new basis for immunotherapy and individualized treatment of ESCC patients.
In this investigation, METTL3 expression was shown to be much higher in cancerous tissue than in healthy tissue. METTL3 overexpression predicted a poor prognosis and is an independent prognostic factor. GSEA revealed that METTL3 is mainly involved in chromosome homologous recombination and DNA mismatch repair, which could be potential mechanisms for the occurrence and progression of tumor-related diseases.
Based on CIBERSORT and TISIDB analysis, T lymphocytes, B lymphocytes, and neutrophils had high levels of infiltration in ESCC, and their infiltration was demonstrated to be negatively related to METTL3 expression. METTL3 was linked with immune processes, such as cytokine receptor interaction, the MAPK signaling pathway, and natural killer cell-mediated cytotoxicity, as determined by GO/KEGG functional enrichment analysis.
METTL3-related immune prognostic genes were used to cluster ESCC patients. Survival rates for patients with ESCC in subgroup A and B were considerably greater than for those in categories C, making this finding particularly instructive in such patients’ evaluation and treatment. In addition, we constructed a prognosis prediction model composed of six genes (PSMC6, TBK1, AHNAK, PSMD10, AKT1 and CTF1). Based on the results of univariate and multivariate Cox analyses, the risk score calculated according to the model equation is a good independent survival index. Forecasting patient survival and assessing probable clinical outcomes are both possible uses for the risk score tool. OS analysis and ROC curve analysis also verified that the model had good prediction performance. In addition, the risk score combined with clinical indicators constructed into a nomogram also had superior prognostic predictive power, as verified by the calibration curve and ROC curve analysis.
Among the genes involved in the construction of the prognosis prediction model, PSMC6 encodes the proteasome 26S subunit and is involved in the ATP-dependent degradation of ubiquitinated proteins. Thus, the proteasome participates in cell cycle progression, apoptosis, and DNA damage repair. Studies have shown that PSMC6 is associated with lung adenocarcinoma, breast cancer, pheochromocytoma, low-grade glioma, colorectal melanoma and other diseases (56–59).
TANK-binding kinase 1 (TBK1) is a Ser/Thr kinase with a central role in coordinating the cellular response to invading pathogens and regulating key inflammatory signaling cascades. Some genes play an important role in the antiviral mechanism of cells, such as TBK1, IRGM and so on (60, 61). Studies have shown that TBK1 is also associated with cancers, such as kidney cancer, cervical cancer, and lung cancer (62–64).
AHNAK encodes a large (700 kDa) structural scaffold protein. The protein might play a role in blood-brain barrier formation, cell structure and migration, cardiac calcium channel regulation, and tumor metastasis. Studies have shown that AHNAK is associated with colorectal cancer, ovarian cancer, gastric cancer and other diseases (65–68).
PSMD10 encodes a subunit of the PA700/19S complex, which is a regulatory component of the 26s proteasome and could be involved in protein-protein interactions. Aberrant expression of this gene could play a role in tumorigenesis. Studies have shown that PSMD10 is associated with tumor diseases, such as hepatocellular carcinoma and thyroid cancer (69–72).
Akt serine/threonine kinase 1 (AKT1) encodes a member of the human Akt serine threonine protein kinase family. It can be phosphorylated by phosphoinositide 3 kinase (PI3K) and participate in the AKT/PI3K pathway. It is a key part of many signal transduction pathways and is associated with diseases such as gastric, prostate, breast and ovarian cancers (73–75).
The CTF1 gene encodes a secretory cytokine capable of inducing cardiomyocyte hypertrophy in vitro, and it plays roles in hypertensive heart disease, dilated cardiomyopathy, lung adenocarcinoma and other diseases (76–78).
It is worth mentioning that, while the primary functions of these genes are distinct, we reviewed previous reports that these six genes are associated with cancer patient prognosis. Therefore, the prognostic prediction model relying on these six genes has a high degree of accuracy and reliability.
However, our research still has some shortcomings. First, although we included all esophageal cancer cases (containing 162 tumor tissue samples and 11 normal tissue samples) from the Tumor Genome Atlas (TCGA) database in the study, the number of cases still must be further increased, and we will include Gene Expression Omnibus (GEO), International Cancer Genome Consortium (ICGC) and other databases for further research. Second, although we have performed some experiments to verify the analysis results, more experiments are needed to strengthen the credibility, which will be the focus of our next work, and some experiments have already been performed.
Conclusion
In summary, this study suggests that METTL3 is not only a potential pathogenic molecule for esophageal carcinogenesis and progression but also a potential target for immunotherapy in esophageal cancer. In addition, METTL3 is also a biomarker for forecasting ESCC patient prognosis. The established subtype delineation system and prognostic prediction model can be utilized to predict the prognosis of patients and assess the potential clinical risk. These findings could help to provide a new basis for immunotherapy and individualized treatment of ESCC patients.
Data Availability Statement
The datasets presented in this study can be found in online repositories. The names of the repository/repositories and accession number(s) can be found in the article/Supplementary Material.
Ethics Statement
The studies involving human participants were reviewed and approved by The Third Affiliated Hospital, Sun Yat-sen University. The patients/participants provided their written informed consent to participate in this study
Author Contributions
YZ was a major contributor to all of the experimental work, data analysis, and manuscript writing. SG and YL were involved in the experimental work. FC, YW and YX were involved in the data analysis. JA conceptualized the project, acquired funding, and assisted with the manuscript development. The final manuscript was reviewed and approved by all of the authors.
Funding
The current study was funded by the Natural Science Foundation of Guangdong Province, China (No. 2017A030313118).
Conflict of Interest
The authors declare that the research was conducted in the absence of any commercial or financial relationships that could be construed as a potential conflict of interest.
Publisher’s Note
All claims expressed in this article are solely those of the authors and do not necessarily represent those of their affiliated organizations, or those of the publisher, the editors and the reviewers. Any product that may be evaluated in this article, or claim that may be made by its manufacturer, is not guaranteed or endorsed by the publisher.
Acknowledgments
The patients and researchers who participated in this study are greatly appreciated.
Supplementary Material
The Supplementary Material for this article can be found online at: https://www.frontiersin.org/articles/10.3389/fonc.2022.824190/full#supplementary-material
Abbreviations
EC, esophageal carcinoma; ESCC, esophageal squamous cell carcinoma; METTL3, methyltransferase-like 3; M6A, n6 methyladenosine; TCGA, the Tumor Genome Atlas; IMMPORT, the immunology database and analysis portal; OS, overall survival; PFS, progression-free survival; ROC, receiver operating characteristic; AUC, area under curve; GO, gene ontology; KEGG, Kyoto Encyclopedia of Genes and Genomes; GSEA, gene set enrichment analysis; PCA, principal component analysis; LASSO, the least absolute shrinkage and selection operator; HR, hazard ratio.
References
1. Gupta B, Kumar N. Worldwide Incidence, Mortality and Time Trends for Cancer of the Oesophagus. Eur J Cancer Prev (2017) 26(2):107–18. doi: 10.1097/CEJ.0000000000000249
2. Siegel RL, Miller KD, Jemal A. Cancer Statistics, 2018. CA Cancer J Clin (2018) 68(1):7–30. doi: 10.3322/caac.21442
3. Smyth EC, Lagergren J, Fitzgerald RC, Lordick F, Shah MA, Lagergren P, et al. Oesophageal Cancer. Nat Rev Dis Primers (2017) 3:17048. doi: 10.1038/nrdp.2017.48
4. Chen W, Zheng R, Baade PD, Zhang S, Zeng H, Bray F, et al. Cancer Statistics in China, 2015. CA Cancer J Clin (2016) 66(2):115–32. doi: 10.3322/caac.21338
5. Abnet CC, Arnold M, Wei W-Q. Epidemiology of Esophageal Squamous Cell Carcinoma. Gastroenterology (2018) 154(2):360–73. doi: 10.1053/j.gastro.2017.08.023
6. Bray F, Ferlay J, Soerjomataram I, Siegel RL, Torre LA, Jemal A. Global Cancer Statistics 2018: GLOBOCAN Estimates of Incidence and Mortality Worldwide for 36 Cancers in 185 Countries. CA Cancer J Clin (2018) 68(6):394–424. doi: 10.3322/caac.21492
7. Nebbioso A, Tambaro FP, Dell’Aversana C, Altucci L. Cancer Epigenetics: Moving Forward. PloS Genet (2018) 14(6):e1007362. doi: 10.1371/journal.pgen.1007362
8. Baylin SB, Jones PA. Epigenetic Determinants of Cancer. Cold Spring Harb Perspect Biol (2016) 8(9):a019505. doi: 10.1101/cshperspect.a019505
9. Liu ZX, Li LM, Sun HL, Liu SM. Link Between M6a Modification and Cancers. Front Bioeng Biotechnol (2018) 6:89. doi: 10.3389/fbioe.2018.00089
10. Peixoto P, Cartron PF, Serandour AA, Hervouet E. From 1957 to Nowadays: A Brief History of Epigenetics. Int J Mol Sci (2020) 21(20):7571. doi: 10.3390/ijms21207571
11. Lu C, Thompson CB. Metabolic Regulation of Epigenetics. Cell Metab (2012) 16(1):9–17. doi: 10.1016/j.cmet.2012.06.001
12. Goldman MA. The Epigenetics of the Cell. Genome Biol (2003) 4(3):309. doi: 10.1186/gb-2003-4-3-309
13. Zoghbi HY, Beaudet AL. Epigenetics and Human Disease. Cold Spring Harb Perspect Biol (2016) 8(2):a019497. doi: 10.1101/cshperspect.a019497
14. Elfawy HA, Anupriya S, Mohanty S, Patel P, Ghosal S, Panda PK, et al. Molecular Toxicity of Benzo(a)pyrene Mediated by Elicited Oxidative Stress Infer Skeletal Deformities and Apoptosis in Embryonic Zebrafish. Sci Total Environ (2021) 789:147989. doi: 10.1016/j.scitotenv.2021.147989
15. Lopes N, Correia MP, Henrique R, Jerónimo C. Epigenetic Alterations in Oesophageal Cancer: Expression and Role of the Involved Enzymes. Int J Mol Sci (2020) 21(10):3522. doi: 10.3390/ijms21103522
16. Cao W, Lee H, Wu W, Zaman A, McCorkle S, Yan M, et al. Multi-Faceted Epigenetic Dysregulation of Gene Expression Promotes Esophageal Squamous Cell Carcinoma. Nat Commun (2020) 11(1):3675. doi: 10.1038/s41467-020-17227-z
17. Sahu GR, Nayak BK, Patnaik S, Parija T, Das BR. Rearrangement of P53 Gene With Overexpressed P53 Protein in Primary Cervical Cancer. Oncol Rep (2002) 9(2):433–7. doi: 10.3892/or.9.2.433
18. Nayak BK, Patnaik S, Das BR. Rearrangement of the P53 Gene in Human Breast Tumours. Biochem Biophys Res Commun (1998) 245(2):388–91. doi: 10.1006/bbrc.1998.8444
19. Patnaik S, Nayak BK, Das BR. Rearrangement in the Coding and 5’ Region of P53 Gene in Human Oral Tumors. IUBMB Life (1999) 48(3):305–9. doi: 10.1080/713803524
20. Roundtree IA, Evans ME, Pan T, He C. Dynamic RNA Modifications in Gene Expression Regulation. Cell (2017) 169(7):1187–200. doi: 10.1016/j.cell.2017.05.045
21. Chen XY, Zhang J, Zhu JS. The Role of M6a RNA Methylation in Human Cancer. Mol Cancer (2019) 18(1):103. doi: 10.1186/s12943-019-1033-z
22. Oerum S, Meynier V, Catala M, Tisné C. A Comprehensive Review of M6a/m6Am RNA Methyltransferase Structures. Nucleic Acids Res (2021) 49(13):7239–55. doi: 10.1093/nar/gkab378
23. Fu Y, Dominissini D, Rechavi G, He C. Gene Expression Regulation Mediated Through Reversible M6a RNA Methylation. Nat Rev Genet (2014) 15(5):293–306. doi: 10.1038/nrg3724
24. Cai Y, Feng R, Lu T, Chen X, Zhou X, Wang X. Novel Insights Into the M6a-RNA Methyltransferase METTL3 in Cancer. Biomark Res (2021) 9(1):27. doi: 10.1186/s40364-021-00278-9
25. Zheng W, Dong X, Zhao Y, Wang S, Jiang H, Zhang M, et al. Multiple Functions and Mechanisms Underlying the Role of METTL3 in Human Cancers. Front Oncol (2019) 9:1403. doi: 10.3389/fonc.2019.01403
26. Guo W, Tan F, Huai Q, Wang Z, Shao F, Zhang G, et al. Comprehensive Analysis of PD-L1 Expression, Immune Infiltrates, and M6a RNA Methylation Regulators in Esophageal Squamous Cell Carcinoma. Front Immunol (2021) 12:669750. doi: 10.3389/fimmu.2021.669750
27. Zou J, Zhong X, Zhou X, Xie Q, Zhao Z, Guo X, et al. The M6A Methyltransferase METTL3 Regulates Proliferation in Esophageal Squamous Cell Carcinoma. Biochem Biophys Res Commun (2021) 580:48–55. doi: 10.1016/j.bbrc.2021.05.048
28. Xia TL, Yan SM, Yuan L, Zeng MS. Upregulation of METTL3 Expression Predicts Poor Prognosis in Patients With Esophageal Squamous Cell Carcinoma. Cancer Manag Res (2020) 12:5729–37. doi: 10.2147/CMAR.S245019
29. Wang Z, Jensen MA, Zenklusen JC. A Practical Guide to The Cancer Genome Atlas (TCGA). Methods Mol Biol (2016) 1418:111–41. doi: 10.1007/978-1-4939-3578-9_6
30. Bhattacharya S, Dunn P, Thomas CG, Smith B, Schaefer H, Chen J, et al. ImmPort, Toward Repurposing of Open Access Immunological Assay Data for Translational and Clinical Research. Sci Data (2018) 5:180015. doi: 10.1038/sdata.2018.15
31. Subramanian A, Tamayo P, Mootha VK, Mukherjee S, Ebert BL, Gillette MA, et al. Gene Set Enrichment Analysis: A Knowledge-Based Approach for Interpreting Genome-Wide Expression Profiles. Proc Natl Acad Sci USA (2005) 102(43):15545–50. doi: 10.1073/pnas.0506580102
32. Hung JH, Yang TH, Hu Z, Weng Z, DeLisi C. Gene Set Enrichment Analysis: Performance Evaluation and Usage Guidelines. Brief Bioinform (2012) 13(3):281–91. doi: 10.1093/bib/bbr049
33. Chen B, Khodadoust MS, Liu CL, Newman AM, Alizadeh AA. Profiling Tumor Infiltrating Immune Cells With CIBERSORT. Methods Mol Biol (2018) 1711:243–59. doi: 10.1007/978-1-4939-7493-1_12
34. Newman AM, Liu CL, Green MR, Gentles AJ, Feng W, Xu Y, et al. Robust Enumeration of Cell Subsets From Tissue Expression Profiles. Nat Methods (2015) 12(5):453–7. doi: 10.1038/nmeth.3337
35. Ru B, Wong CN, Tong Y, Zhong JY, Zhong SSW, Wu WC, et al. TISIDB: An Integrated Repository Portal for Tumor-Immune System Interactions. Bioinformatics (2019) 35(20):4200–2. doi: 10.1093/bioinformatics/btz210
36. Gene Ontology Consortium, Blake JA, Dolan M, Drabkin H, Hill DP, Li N, et al. Gene Ontology Annotations and Resources. Nucleic Acids Res (2013) 41(Database issue):D530–5. doi: 10.1093/nar/gks1050
37. The Gene Ontology Consortium. Expansion of the Gene Ontology Knowledgebase and Resources. Nucleic Acids Res (2017) 45(D1):D331–8. doi: 10.1093/nar/gkw1108
38. Kanehisa M, Goto S, Sato Y, Furumichi M, Tanabe M. KEGG for Integration and Interpretation of Large-Scale Molecular Data Sets. Nucleic Acids Res (2012) 40(Database issue):D109–14. doi: 10.1093/nar/gkr988
39. Kanehisa M, Furumichi M, Tanabe M, Sato Y, Morishima K. KEGG: New Perspectives on Genomes, Pathways, Diseases and Drugs. Nucleic Acids Res (2017) 45(D1):D353–61. doi: 10.1093/nar/gkw1092
40. Szklarczyk D, Gable AL, Lyon D, Junge A, Wyder S, Huerta-Cepas J, et al. STRING V11: Protein-Protein Association Networks With Increased Coverage, Supporting Functional Discovery in Genome-Wide Experimental Datasets. Nucleic Acids Res (2019) 47(D1):D607–13. doi: 10.1093/nar/gky1131
41. Szklarczyk D, Gable AL, Nastou KC, Lyon D, Kirsch R, Pyysalo S, et al. The STRING Database in 2021: Customizable Protein-Protein Networks, and Functional Characterization of User-Uploaded Gene/Measurement Sets. Nucleic Acids Res (2021) 49(D1):D605–12. doi: 10.1093/nar/gkaa1074
42. Wilkerson MD, Hayes DN. ConsensusClusterPlus: A Class Discovery Tool With Confidence Assessments and Item Tracking. Bioinformatics (2010) 26(12):1572–3. doi: 10.1093/bioinformatics/btq170
43. Seiler M, Huang CC, Szalma S, Bhanot G. ConsensusCluster: A Software Tool for Unsupervised Cluster Discovery in Numerical Data. OMICS (2010) 14(1):109–13. doi: 10.1089/omi.2009.0083
44. Jolliffe IT, Cadima J. Principal Component Analysis: A Review and Recent Developments. Philos Trans A Math Phys Eng Sci (2016) 374(2065):20150202. doi: 10.1098/rsta.2015.0202
45. Giuliani A. The Application of Principal Component Analysis to Drug Discovery and Biomedical Data. Drug Discov Today (2017) 22(7):1069–76. doi: 10.1016/j.drudis.2017.01.005
46. Li Z, Sillanpää MJ. Overview of LASSO-Related Penalized Regression Methods for Quantitative Trait Mapping and Genomic Selection. Theor Appl Genet (2012) 125(3):419–35. doi: 10.1007/s00122-012-1892-9
47. Balachandran VP, Gonen M, Smith JJ, DeMatteo RP. Nomograms in Oncology: More Than Meets the Eye. Lancet Oncol (2015) 16(4):e173–80. doi: 10.1016/S1470-2045(14)71116-7
48. Iasonos A, Schrag D, Raj GV, Panageas KS. How to Build and Interpret a Nomogram for Cancer Prognosis. J Clin Oncol (2008) 26(8):1364–70. doi: 10.1200/JCO.2007.12.9791
49. Ohashi S, Miyamoto S, Kikuchi O, Goto T, Amanuma Y, Muto M. Recent Advances From Basic and Clinical Studies of Esophageal Squamous Cell Carcinoma. Gastroenterology (2015) 149(7):1700–15. doi: 10.1053/j.gastro.2015.08.054
50. Triantafyllou T, Wijnhoven BPL. Current Status of Esophageal Cancer Treatment. Chin J Cancer Res (2020) 32(3):271–86. doi: 10.21147/j.issn.1000-9604.2020.03.01
51. Zhao Q, Yu J, Meng X. A Good Start of Immunotherapy in Esophageal Cancer. Cancer Med (2019) 8(10):4519–26. doi: 10.1002/cam4.2336
52. Kelly RJ. The Emerging Role of Immunotherapy for Esophageal Cancer. Curr Opin Gastroenterol (2019) 35(4):337–43. doi: 10.1097/MOG.0000000000000542
53. Schizas D, Charalampakis N, Kole C, Mylonas KS, Katsaros I, Zhao M, et al. Immunotherapy for Esophageal Cancer: A 2019 Update. Immunotherapy (2020) 12(3):203–18. doi: 10.2217/imt-2019-0153
54. Baba Y, Nomoto D, Okadome K, Ishimoto T, Iwatsuki M, Miyamoto Y, et al. Tumor Immune Microenvironment and Immune Checkpoint Inhibitors in Esophageal Squamous Cell Carcinoma. Cancer Sci (2020) 111(9):3132–41. doi: 10.1111/cas.14541
55. Zeng C, Huang W, Li Y, Weng H. Roles of METTL3 in Cancer: Mechanisms and Therapeutic Targeting. J Hematol Oncol (2020) 13(1):117. doi: 10.1186/s13045-020-00951-w
56. Hua X, Chen J, Wu L. Identification of Candidate Biomarkers Associated With Apoptosis in Melanosis Coli: GNG5, LPAR3, MAPK8, and PSMC6. Biosci Rep (2019) 39(1):BSR20181369. doi: 10.1042/BSR20181369
57. Zhang M, Wang X, Chen X, Zhang Q, Hong J. Novel Immune-Related Gene Signature for Risk Stratification and Prognosis of Survival in Lower-Grade Glioma. Front Genet (2020) 11:363. doi: 10.3389/fgene.2020.00363
58. Kao TJ, Wu CC, Phan NN, Liu YH, Ta HDK, Anuraga G, et al. Prognoses and Genomic Analyses of Proteasome 26s Subunit, ATPase (PSMC) Family Genes in Clinical Breast Cancer. Aging (Albany NY) (2021) 13(14):17970. doi: 10.18632/aging.203345
59. Zhang JY, Shi KZ, Liao XY, Li SJ, Bao D, Qian Y, et al. The Silence of PSMC6 Inhibits Cell Growth and Metastasis in Lung Adenocarcinoma. BioMed Res Int (2021) 2021:9922185. doi: 10.1155/2021/9922185
60. Zhao W. Negative Regulation of TBK1-Mediated Antiviral Immunity. FEBS Lett (2013) 587(6):542–8. doi: 10.1016/j.febslet.2013.01.052
61. Nath P, Chauhan NR, Jena KK, Datey A, Kumar ND, Mehto S, et al. Inhibition of IRGM Establishes a Robust Antiviral Immune State to Restrict Pathogenic Viruses. EMBO Rep (2021) 22(11):e52948. doi: 10.15252/embr.202152948
62. Cai H, Yan L, Liu N, Xu M, Cai H. IFI16 Promotes Cervical Cancer Progression by Upregulating PD-L1 in Immunomicroenvironment Through STING-TBK1-NF-kB Pathway. BioMed Pharmacother (2020) 123:109790. doi: 10.1016/j.biopha.2019.109790
63. Revach O-Y, Liu S, Jenkins RW. Targeting TANK-Binding Kinase 1 (TBK1) in Cancer. Expert Opin Ther Targets (2020) 24(11):1065–78. doi: 10.1080/14728222.2020.1826929
64. Hu L, Xie H, Liu X, Potjewyd F, James LI, Wilkerson EM, et al. TBK1 Is a Synthetic Lethal Target in Cancer With VHL Loss. Cancer Discov (2020) 10(3):460–75. doi: 10.1158/2159-8290.CD-19-0837
65. Zhao Z, Xiao S, Yuan X, Yuan J, Zhang C, Li H, et al. AHNAK as a Prognosis Factor Suppresses the Tumor Progression in Glioma. J Cancer (2017) 8(15):2924–32. doi: 10.7150/jca.20277
66. Cai Y, Hu Y, Yu F, Tong W, Wang S, Sheng S, et al. AHNAK Suppresses Ovarian Cancer Progression Through the Wnt/β-Catenin Signaling Pathway. Aging (Albany NY) (2021) 13(20):23579–87. doi: 10.18632/aging.203473
67. Shen E, Wang X, Liu X, Lv M, Zhang L, Zhu G, et al. MicroRNA-93-5p Promotes Epithelial-Mesenchymal Transition in Gastric Cancer by Repressing Tumor Suppressor AHNAK Expression. Cancer Cell Int (2020) 20:76. doi: 10.1186/s12935-019-1092-7
68. Cho WC, Jang JE, Kim KH, Yoo BC, Ku JL. SORBS1 Serves a Metastatic Role via Suppression of AHNAK in Colorectal Cancer Cell Lines. Int J Oncol (2020) 56(5):1140–51. doi: 10.3892/ijo.2020.5006
69. Hao P, Yue F, Xian X, Ren Q, Cui H, Wang Y. Inhibiting Effect of MicroRNA-3619-5p/PSMD10 Axis on Liver Cancer Cell Growth. Vivo Vitro Life Sci (2020) 254:117632. doi: 10.1016/j.lfs.2020.117632
70. Liu F, Lou K, Zhao X, Zhang J, Chen W, Qian Y, et al. miR-214 Regulates Papillary Thyroid Carcinoma Cell Proliferation and Metastasis by Targeting Psmd10. Int J Mol Med (2018) 42(6):3027–36. doi: 10.3892/ijmm.2018.3902
71. Li J, Tian F, Li D, Chen J, Jiang P, Zheng S, et al. MiR-605 Represses PSMD10/Gankyrin and Inhibits Intrahepatic Cholangiocarcinoma Cell Progression. FEBS Lett (2014) 588(18):3491–500. doi: 10.1016/j.febslet.2014.08.008
72. Luo T, Fu J, Xu A, Su B, Ren Y, Li N, et al. PSMD10/gankyrin Induces Autophagy to Promote Tumor Progression Through Cytoplasmic Interaction With ATG7 and Nuclear Transactivation of ATG7 Expression. Autophagy (2016) 12(8):1355–71. doi: 10.1080/15548627.2015.1034405
73. Fresno Vara JA, Casado E, de Castro J, Cejas P, Belda-Iniesta C, González-Barón M. PI3K/Akt Signalling Pathway and Cancer. Cancer Treat Rev (2004) 30(2):193–204. doi: 10.1016/j.ctrv.2003.07.007
74. Alwhaibi A, Verma A, Adil MS, Somanath PR. The Unconventional Role of Akt1 in the Advanced Cancers and in Diabetes-Promoted Carcinogenesis. Pharmacol Res (2019) 145:104270. doi: 10.1016/j.phrs.2019.104270
75. Hinz N, Jücker M. Distinct Functions of AKT Isoforms in Breast Cancer: A Comprehensive Review. Cell Commun Signal (2019) 17(1):154. doi: 10.1186/s12964-019-0450-3
76. Robador PA, San José G, Rodríguez C, Guadall A, Moreno MU, Beaumont J, et al. HIF-1-Mediated Up-Regulation of Cardiotrophin-1 Is Involved in the Survival Response of Cardiomyocytes to Hypoxia. Cardiovasc Res (2011) 92(2):247–55. doi: 10.1093/cvr/cvr202
77. Erdmann J, Hassfeld S, Kallisch H, Fleck E, Regitz-Zagrose V. Genetic Variants in the Promoter (G983g>T) and Coding Region (A92T) of the Human Cardiotrophin-1 Gene (CTF1) in Patients With Dilated Cardiomyopathy. Hum Mutat (2000) 16:448. doi: 10.1002/1098-1004(200011)16:5<448::AID-HUMU19>3.0.CO;2-D
Keywords: esophageal squamous cell carcinoma, METTL3, m6A modification, immune infiltration, immunotyping, prognostic model
Citation: Zhou Y, Guo S, Li Y, Chen F, Wu Y, Xiao Y and An J (2022) METTL3 Is Associated With the Malignancy of Esophageal Squamous Cell Carcinoma and Serves as a Potential Immunotherapy Biomarker. Front. Oncol. 12:824190. doi: 10.3389/fonc.2022.824190
Received: 29 November 2021; Accepted: 15 March 2022;
Published: 27 April 2022.
Edited by:
Wencheng Zhang, Tianjin Medical University Cancer Institute and Hospital, ChinaCopyright © 2022 Zhou, Guo, Li, Chen, Wu, Xiao and An. This is an open-access article distributed under the terms of the Creative Commons Attribution License (CC BY). The use, distribution or reproduction in other forums is permitted, provided the original author(s) and the copyright owner(s) are credited and that the original publication in this journal is cited, in accordance with accepted academic practice. No use, distribution or reproduction is permitted which does not comply with these terms.
*Correspondence: Jun An, anjun@mail.sysu.edu.cn
†These authors contributed equally to this work and share first authorship