- Department of Ultrasound, The First Affiliated Hospital of University of Science and Technology of China (USTC), Division of Life Sciences and Medicine, University of Science and Technology of China, Hefei, China
Background: Conventional ultrasound diagnosis of thyroid nodules (TNs) had a high false-positive rate, resulting in many unnecessary fine-needle aspirations (FNAs).
Objective: This study aimed to establish a simple algorithm to reduce unnecessary FNA on TIRADS 4 TNs using different quantitative parameters of ultrasonic elasticity and chi-square automatic interactive detector (CHAID) method.
Methods: From January 2020 to May 2021, 432 TNs were included in the study, which were confirmed by FNA or surgical pathology. Each TN was examined using conventional ultrasound, sound touch elastography, and Shell measurement function. The quantitative parameters E and Eshell were recorded, and the Eshell/E values were calculated for each TN. The diagnostic performance of the quantitative parameters was evaluated using the receiver operating characteristic curves. The CHAID was used to classify and analyze the quantitative parameters, and the prediction model was established.
Results: A total of 226 TNs were malignant and 206 were benign. Eshell and Eshell/E ratio were included in the classification algorithm, which showed a depth of two ramifications (Eshell/E ≤ 0.988 or 0.988–1.043 or >1.043; if Eshell/E ≤ 0.988, then Eshell ≤ 64.0 or 64.0–74.0 or >74.0; if Eshell/E = 0.988–1.043, then Eshell ≤ 66.0 or > 66.0; if Eshell/E >1.043, then Eshell ≤ 69.0 or >69.0). The unnecessary FNAs could have been avoided in 57.3% of the cases using this algorithm.
Conclusion: The prediction model using quantitative parameters had high diagnostic performance; it could quickly distinguish benign lesions and avoid subjective influence to some extent.
Introduction
Thyroid nodules (TNs) have become a prevalent clinical disease. The detection rate of TNs has gradually increased in China, as the mass population has begun to pay attention to physical examination every year and ultrasound technology is constantly updated. Thyroid cancer is a common head and neck malignant tumor (1–3), which has become a hot topic among doctors and patients.
Ultrasound is the preferred method for thyroid examination in clinical practice to assess TNs and cervical lymph nodes (4, 5). In recent years, many TN risk stratification systems (RSSs) have been introduced to express the descriptions of TNs by radiologists relatively objectively, which are based on suspected US features (solid, very hypoechoic, taller-than-wide, extra-thyroidal extension, punctate echogenic foci, and so on) of a TN (6–8). In 2017, the American College of Radiology (ACR) published a white paper on the classification and diagnosis of TNs. The ACR-TIRADS was used to assign points for the suspected US features of a TN. The total points determined the ACR-TIRADS level from TIRADS 1 to TIRADS 5. When the maximum diameter of TIRADS 4 nodule was greater than or equal to 15 mm or a TIRADS 5 nodule was greater than or equal to 10 mm, the fine-needle aspiration (FNA) was needed. The ACR-TIRADS was developed to maximize the identification of malignant tumors using a unified approach, avoiding unnecessary FNA, while not losing cancers avoided among FNAs. Among these, the malignant risk of ACR-TIRADS 4 of TNs was about 5%–20%. This probability span was large, and the false-positive rate was high. In practice, doctors sometimes recommend FNA for patients with TIRADS 4 of TNs because of their anxiety and fear, but the puncture and follow-up of some patients show benign results, which increases the unnecessary burden.
ACR-TIRADS does not include the characteristics of ultrasound elastography (UE). At present, many studies have confirmed the excellent diagnostic performance of UE in thyroid diseases (9–11). Some studies showed that TNs could be differentiated by measuring the internal stiffness of nodules with a sensitivity of 77.2%–85.7% and a specificity of 80.5%–96.0%. The stiffness of benign TNs was lower than that of malignant TNs (12–14).
However, hemorrhage, calcification, and cystic changes may occur in TNs, leading to uneven internal echo, which affects the measurement of TN stiffness using UE. In actual measurements, the internal stiffness of TNs can be quantitatively assessed by sound touch elastography (STE) and peripheral stiffness using the Shell measurement function. Our previous study confirmed that measuring a tissue stiffness of 2 mm around TNs using the Shell measurement function could improve the accuracy of differentiating between malignant and benign TNs (15, 16).
Classification algorithms aim to aid in clinical decision making by incorporating different criteria in a formalized manner. For example, an official and impersonal combination of diagnostic features in the context of a multivariable approach is supposed to improve specificity and reduce variability (17). CHAID is a commonly used decision tree algorithm. Its purpose is to divide the total research population into several relatively homogeneous subpopulations based on certain characteristics (independent variable values) for analysis. Some variables are selected by the decision tree from all independent variables for analysis according to their contribution. Hence, the decision tree can automatically process a large number of independent variables and is more adaptable. CHAID is a nonparametric algorithm; therefore, it is not limited by too many applicable conditions. It has a wider application range and is more suitable for analyzing various complex connections, especially for the analysis of samples with nonlinear correlation or interaction. Hence, it is better than the ordinary statistics model. Some studies applied this algorithm to magnetic resonance imaging and obtained positive results (18–20). Only a few studies applied this algorithm to breast ultrasound research (21). This classification algorithm has not been established for thyroid research yet.
Therefore, this novel study aimed to classify and analyze ultrasound elasticity parameters, select appropriate nudes, combine different parameters, and build a predictive model to objectively assist in the clinical management of TNs and reduce unnecessary FNA on TIRADS 4 nodules.
Materials and Methods
Patient Selection
The ethics committee of the First Affiliated Hospital of the University of Science and Technology of China (USTC) approved this prospective study, and all patients gave informed consent to be included in the study. From January 2020 to May 2021, 1179 consecutive patients with 1463 TNs in the First Affiliated Hospital of USTC were detected by conventional ultrasound. All the TNs were classified according to ACR-TIRADS (7), and then TIRADS 4 TNs were selected. Next, we continued to select the following nodules for this study: solid or <20% cystic; at least 2-mm thyroid tissue around TNs could be measured. All TNs were confirmed by final surgical pathology or FNA.
Ultrasound-guided FNA on TNs was performed by two radiologists having more than 3 years of experience in FNA. According to the Bethesda System for Reporting Thyroid Cytopathology (22), the cytological reports were issued by one of three pathologists with more than 5 years of experience in diagnosing thyroid cytological pathology. All benign or malignant TNs included in the study were confirmed by FNA reports (Bethesda II, V, and VI) or definite histopathological reports. If the FNA report was Bethesda I, another FNA was performed on the same nodule 2 weeks later. TNs whose FNA was reported as Bethesda III or IV and that had no subsequent defined postoperative pathology were excluded. TNs considered benign were included as follows: FNA reports were Bethesda II, and they were followed up for 6 months with the maximum diameter increased by less than 20% and less than 2 mm, or the volume increased by less than 50% on conventional ultrasound (7, 23). All the TNs considered malignant by FNA were further confirmed by histopathology.
Among multiple TNs in the ipsilateral lobe of the thyroid, the ones with the highest risk of malignancy were selected. If the risk was similar, the largest one was selected. Eventually, 364 patients with 432 TNs were included in the study (Figure 1).
Ultrasound Instrument
The ultrasound diagnostic instruments used in this study were a Resona 7 US diagnostic system (Mindray Medical Solutions, Shenzhen, China) and an 11L3 transducer, with the STE function and the Shell measurement software. The Shell software automatically measured the stiffness of the tissue around the target nodules, with a range of 0.5–9 mm and increments of 0.5 mm (Figure 2).
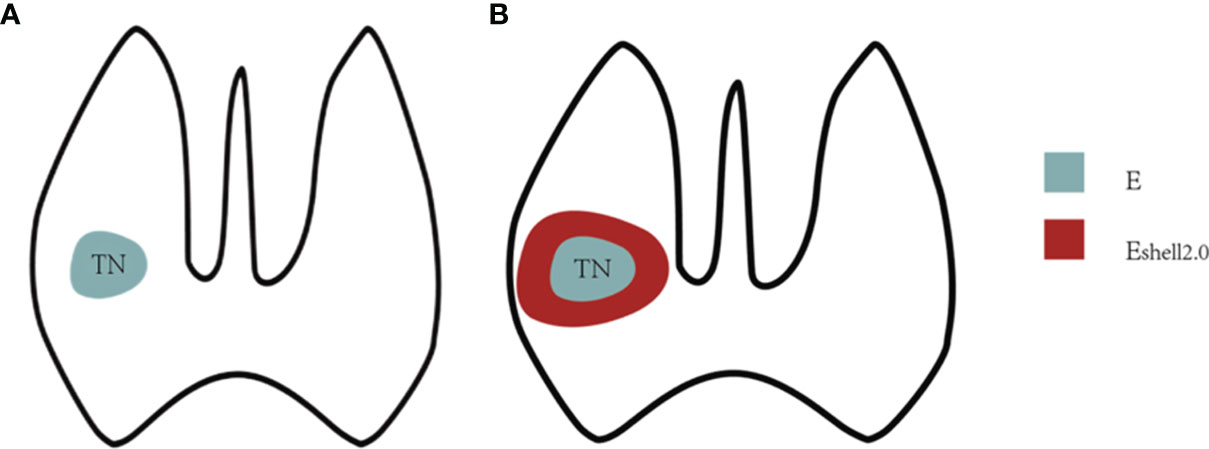
Figure 2 Shell measurement diagram. (A) E value was the interior stiffness of the TN. (B) Eshell value referred to 2 mm-perinodal stiffness of the TN.
Conventional Ultrasound
The ACR-TIRADS classification of all conventional ultrasound images was performed by two radiologists with 10 and 12 years of experience in ultrasound diagnosis, and a consensus was reached after discussion in the case of any disagreement. Neither radiologist had any knowledge of the clinical data of any of the patients.
UE Image Acquisition
First, the longitudinal section of the lateral lobe where the TN was located was selected. The TN was then put in the middle of the region of interest (ROI) to ensure that the ROI included the TN and at least 2 mm of the surrounding thyroid tissue, and the instrument was adjusted to the best state. Then, the edges of the TN were delineated using a tracing method (STE examination), and the internal stiffness of the nodules was measured as the E value. Next, the Shell function was activated, and the stiffness of 2-mm tissue around the nodule was selected to be measured as the Eshell value (Figures 3, 4). The stiffness of each nodule and its periphery was measured three times and averaged. A ratio of the Eshell/E was calculated using the acquired E and Eshell values. The patients were asked to hold their breath so as to reduce the impact of breathing on measurements. All patients were attended by the same radiologist, who had 10 years of experience in ultrasound diagnosis and 5 years in thyroid UE.
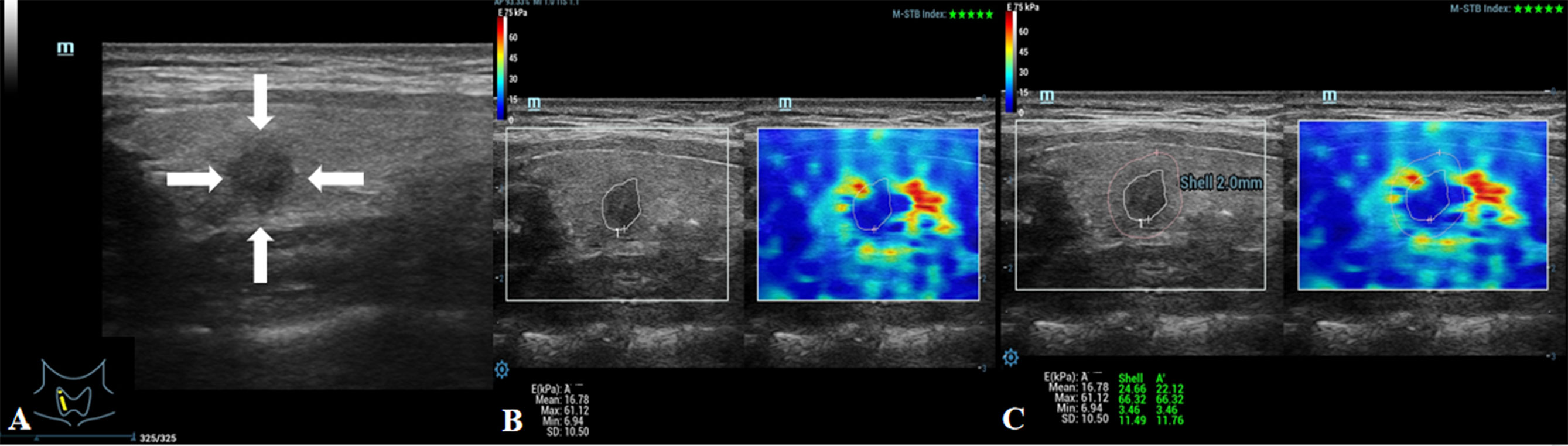
Figure 3 Images showing an ACR-TIRADS 4 TN in a 48-year-old female patient. The pathological diagnosis after surgery was papillary thyroid carcinoma. (A) Conventional ultrasound image of the TN; the arrows point to the TN. (B) E value of the TN was 61.12 kPa. (C) Eshell value of the TN was 66.32 kPa.
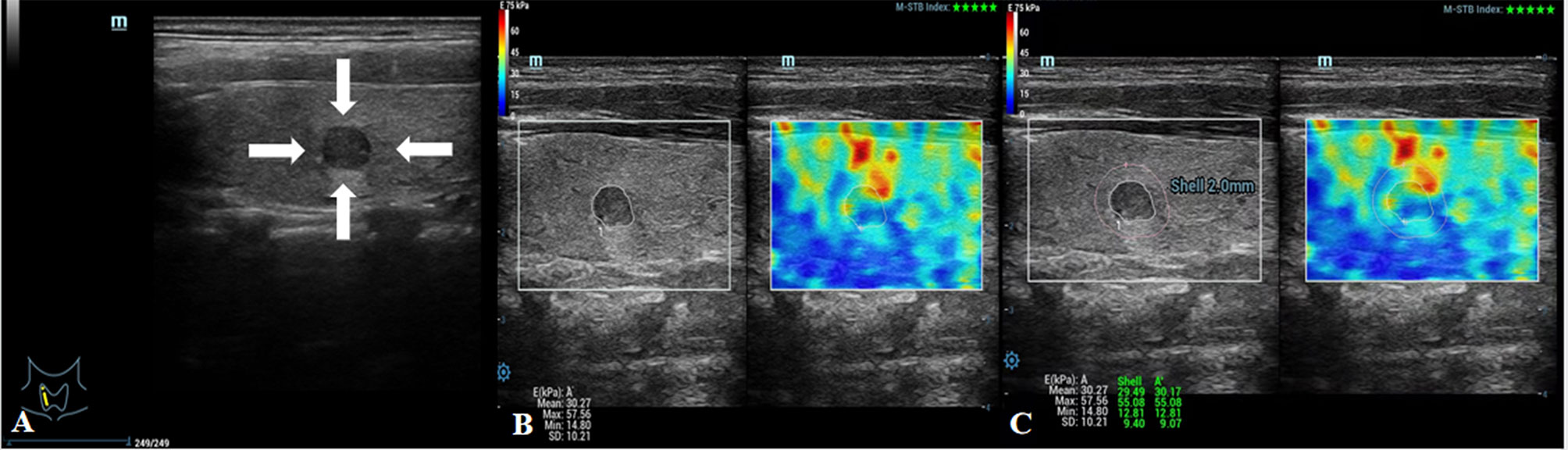
Figure 4 Images showing an ACR-TIRADS 4 TN in a 24-year-old female patient. The FNA diagnosis was a benign TN. (A) Conventional US image of the TN; the arrows point to the TN. (B) E value of the TN was 57.56 kPa. (C) Eshell value of the TN was 55.08 kPa.
Statistical Analysis
The software SPSS 24 (IBM Corp., NY, USA) was used for statistical analysis. Quantitative data were shown as the mean ± SD. Qualitative data were shown as frequencies. The χ2 test and Fisher’s exact probability test were used to compare categorical variables. First, the diagnostic accuracy of the collected quantitative data (E, Eshell, and Eshell/E) was evaluated using the receiver operating characteristic (ROC) curve. Then, the exhaustive chi-squared automatic interaction detection (CHAID) method was used to classify and analyze the elastic data. Based on the chi-square test results, a decision tree was built using the CHAID method. Minimal parent and child node sizes were set to 10 and 5, respectively. The robustness of the classification tree was verified by tenfold cross-validation. The CHAID algorithm automatically calculated the cutoff values of each ramification, and the prediction model was constructed. Therefore, predefined cutoff values were not used. The differences in quantitative parameters were detected with the independent-sample t test. The Z-test was used to compare the areas under ROC curve. A P value < 0.05 was considered to indicate a statistically significant difference.
Results
Conventional Data and US Features
The characteristics of participants are shown in Table 1. The study included 432 TNs in 398 patients; of these, 364 had one single nodule and 34 had one nodule in each lobe. The median age was 48 years (range, 15–79 years); 76.7% (305/398) were women. The size of malignant TNs (maximum diameters were measured using grayscale ultrasound) was significantly smaller than the size of benign TNs (9.73 ± 2.31 vs 12.51 ± 2.41 mm, P < 0.01).
Pathological Diagnosis
A total of 226 TNs were malignant (52.3%) and 206 were benign (47.7%). All the benign TNs had the diagnosis of Bethesda category II and were followed up for 6 months. All the malignant TNs had the diagnosis of Bethesda category IV or V, and every nodule was confirmed by postoperative pathology, which were all papillary thyroid carcinomas.
E and Eshell Values
In malignant TNs, the Eshell values were higher than the E values, and the difference was statistically significant (P < 0.01). In benign TNs, the Eshell values were lower than the E values, and the difference was statistically significant (P < 0.01). Compared with benign TNs, the E values, Eshell values, and Eshell/E values of malignant TNs were higher, and the difference was statistically significant (all P < 0.01) (Table 2).
Diagnostic Performance of Quantitative Parameters
In all TIRADS 4 TNs, Eshell values showed the highest sensitivity (89.8%, P < 0.05), while the Eshell/E values had significantly higher specificity (83.0%, P < 0.05) in differentiating malignant from benign TNs (Table 3). In the ROC curve analysis, the three parameters, the Eshell, E, and Eshell/E values, showed good diagnostic performance [E values: area under the curve (AUC) 0.629, cutoff 69.50, P < 0.05; Eshell values: AUC 0.876, cutoff 64.50, P < 0.05; Eshell/E ratio: AUC 0.890, cutoff 1.005, P < 0.05] (Figure 5).
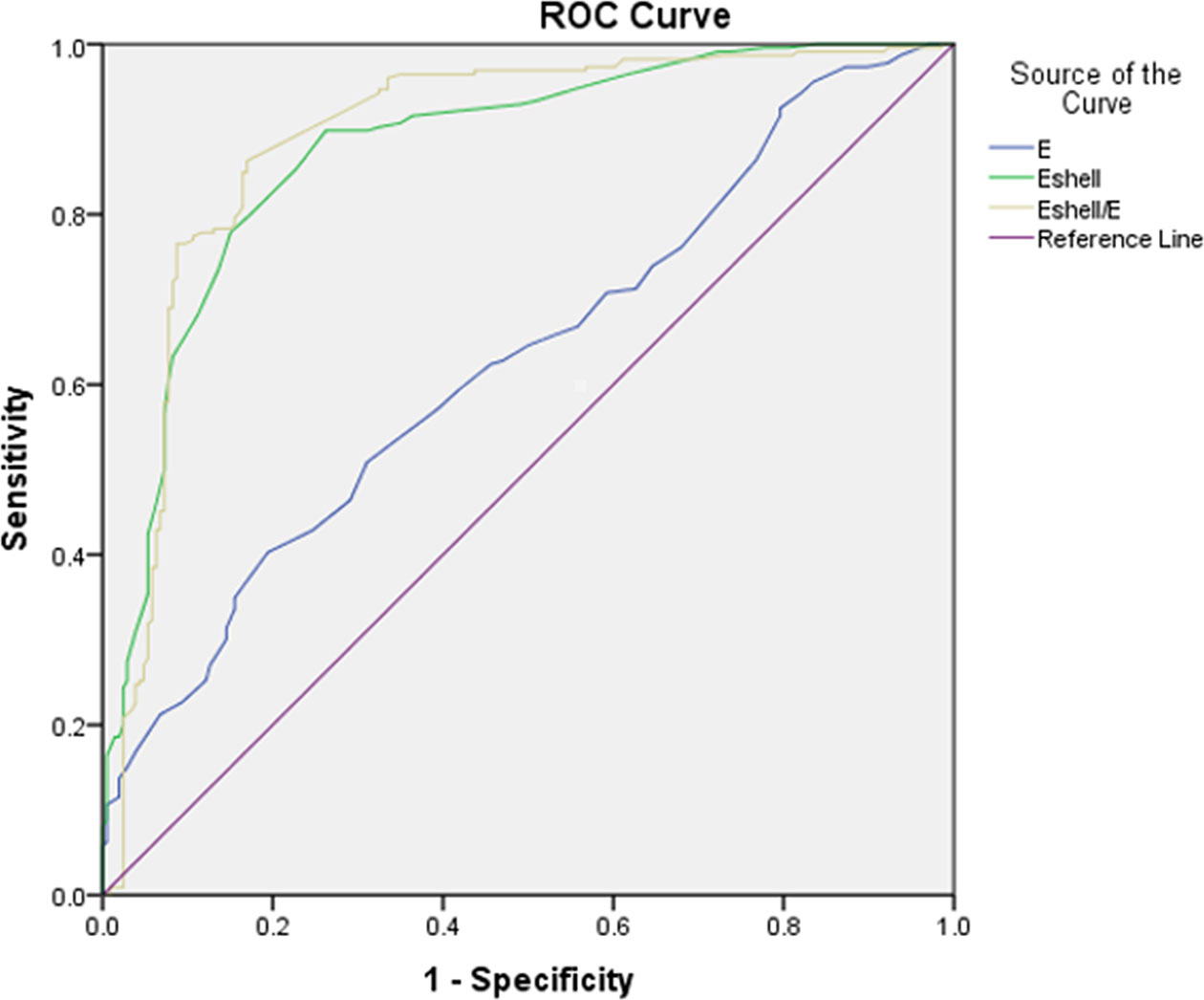
Figure 5 Receiver operating characteristic curves of E, Eshell, and Eshell/E to analyze diagnostic performance (AUC of E = 0.629; AUC of Eshell = 0.876; AUC of Eshell/E = 0.890).
Classification Algorithm
The results of the classification algorithm are shown in Figure 6 and Table 4. The results included the Eshell and Eshell ratio values, but the E values were not included in the algorithm because they did not improve the accuracy of the algorithm. First, the Eshell/E ratio was evaluated: if the Eshell values were ≤0.988, the probability of malignancy was 8.6% (Node 1) and the Eshell values were considered; when its values were ≤64.0, the probability of malignancy dropped to 2.5% (Node 4); when its values were = 64.0–74.0, the probability of malignancy was 25.0% (Node 5); when its values were > 74.0, the probability of malignancy was 57.1% (Node 6). If the Eshell values were 0.988–1.043, the probability of malignancy was 53.3% (Node 2) and the Eshell value was considered; when its values were ≤ 66.0, the probability of malignancy was 23.9% (Node 7); and when its values were ≤66.0, the probability of malignancy was 75.4% (Node 8). If the Eshell/E ratio >1.043, the probability of malignancy was 90.2% (Node 3) and the Eshell values were considered; when its values were ≤ 69.0, the probability of malignancy was 78.0% (Node 9); and when its values were > 69.0, the probability of malignancy was 96.5% (Node 10).
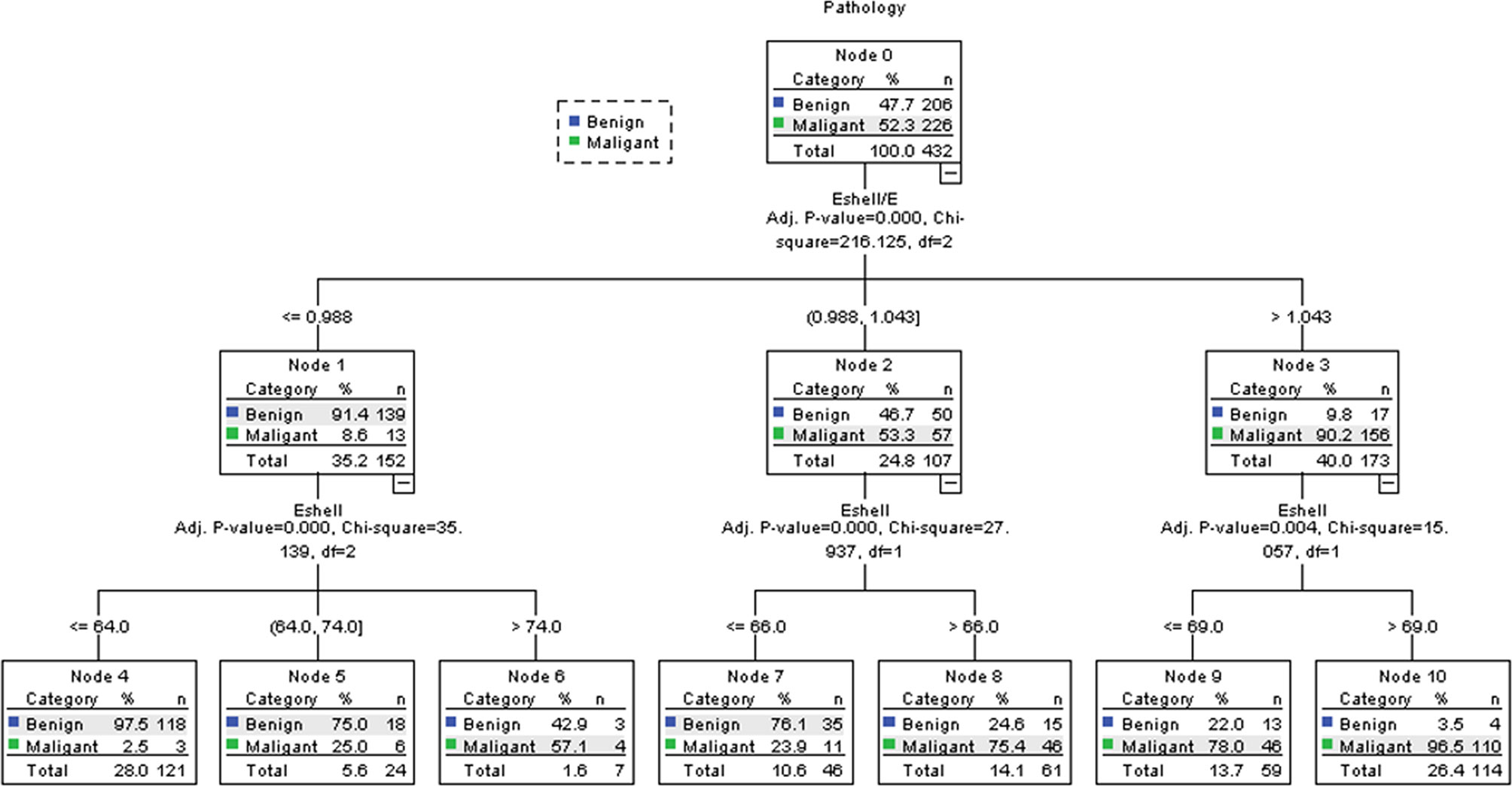
Figure 6 Decision tree of the CHAID. Using the parameters with the highest discriminating power (Eshell and Eshell/E), the research population (Node 0) was further split into child nodes (1–10). After two ramifications, no further discrimination could be achieved. Green tags indicate the percentage of malignant TNs, while blue tags indicate the percentage of benign TNs in each node.
The AUC of the classification algorithm was 0.926 (95% CI 0.901–0.951, P < 0.01), which was significantly higher than the AUC of E (0.629, Z = 10.10, P < 0.01), Eshell (0.0.876, Z = 2.38, P < 0.01), and Eshell/E (0.890, Z = 1.68, P < 0.01). The sensitivity of the classification algorithm was 98.7% (223/226), and the specificity was 57.3% (118/206) (Table 5).
Using this algorithm, 118 out of 206 benign TNs were correctly classified. Hence, the unnecessary FNA could have been avoided in 57.3% of the cases. The algorithm produced three false-negative cases (2.5%).
Discussion
The results of this study showed that a simple prediction model was established by combining the two quantitative indexes (Eshell and Eshell/E) with the classification algorithm, which could accurately distinguish the benign and malignant TNs and reduce the FNA rate of the TIRADS 4 nodules.
At present, several versions of RSSs exist worldwide. Some studies confirmed that ACR-TIRADS had high diagnostic efficiency in differentiating between benign and malignant TNs and a better performance in avoiding unnecessary FNA, but did not have the highest sensitivity among RSSs (16, 24–26). For TIRADS 4 TNs, many FNA results were benign, which increased the unnecessary puncture risk and economic burden for patients (6, 26).
As a relatively new ultrasound technology, the STE had the advantage that it could automatically measure the stiffness of the target using the software to get the quantitative values, and hence the evaluation was relatively objective. A large number of studies confirmed the value of STE in the diagnosis of TNs (27–29).
Most malignant TNs attracted the attention of radiologists when their diameters were small and necessary intervention measures could be taken. Therefore, in our study, the maximum diameters of malignant TNs were significantly smaller than those of benign TNs (P < 0.01). Although the maximum diameter of malignant TNs was smaller than that of benign TNs, their internal stiffness (E) was significantly higher than that of benign TNs (P < 0.01, Table 2).
However, the measurement of internal stiffness of nodules could be subject to errors because the internal stiffness of nodules was often affected by factors such as calcification. The stiffness around the nodules was not affected by these factors. This study confirmed that the internal (E) and peripheral stiffnesses (Eshell) of malignant TNs were higher than those of benign nodules (both P < 0.01). The peripheral stiffness of malignant TNs was higher than their internal stiffness (P < 0.01), which was due to the increase in collagen fiber composition around malignant TNs. In particular, the 2-mm area around the nodules was more abundant in collagen fibers, which increased the peripheral stiffness of malignant TNs. Because of this pathological mechanism, it was meaningful to measure the stiffness around nodules using the STE, which was not affected by the uneven echo inside nodules and hence was more objective (15, 29). On the contrary, benign nodules did not stimulate the proliferation of peripheral fibroblasts, and therefore the peripheral stiffness did not increase. In this study, the peripheral stiffness of benign TNs was lower than the internal stiffness of nodules, which might be due to the fact that this sample only contained TIRADS 4 of TNs, which had a higher proportion of calcification.
If the Eshell/E ratio was higher than 1, the risk of malignancy of TNs was higher; conversely, the possibility of being benign was greater. This study also showed that the Eshell/E ratio of malignant TNs was higher than that of benign TNs, and the difference was statistically significant (P < 0.01). The advantage of Eshell/E over Eshell or E was that it avoided the differences between observers.
The objective of the traditional statistical analysis is to establish an accurate and simple model for the relationship between independent and dependent variables as much as possible. When the relationship between independent and dependent variables is relatively simple, the efficiency of this statistical analysis is higher. However, when the relationship is complex, such as a nonlinear functional relationship, the traditional statistical analysis becomes difficult, its efficiency is low, and the requirements for the analysis are high. According to the purpose of analysis, a decision tree divides the total study population into several relatively homogeneous subpopulations based on certain characteristics, which can handle the complex relationship between independent and dependent variables in a relatively simple and easy way. CHAID is the most basic and simple classification algorithm. Some studies applied the classification algorithm to the diagnosis of breast nodules and proved that similar algorithms could improve the diagnostic accuracy, no matter for breast magnetic resonance or breast ultrasound (20, 21). Kapetas et al. showed that the application of CHAID achieved the highest diagnostic accuracy and the sensitivity of 98.46%. This study also achieved similar results in the diagnosis of thyroid ultrasound. The classification algorithm selected Eshell and Eshell/E among the three quantitative parameters. Compared with individual quantitative parameters, the AUC of the classification algorithm was the highest, with a sensitivity of 98.7% (223/226). Such a high sensitivity ensured that the omission of malignant TNs could be avoided as much as possible in clinical work, which was a prerequisite for us to reduce unnecessary FNA. In Node 4, 118 of 121 TNs were correctly diagnosed, implying that 118 unnecessary FNA were avoided in this sample using this algorithm. At the same time, the probability of malignancy was only 2.5%, which was even lower than the upper limit of malignancy for TIRADS 3 TNs (5%). Therefore, short-term follow-up might be appropriate for these TNs, with a lower risk of missing a large number of thyroid cancers. In Nodes 2, 3, 5−10, the probability of malignancy was more than 23.9%, and therefore the FNA was recommended in all cases.
Therefore, in the face of the clinical management of a large number of TIRADS 4 TNs, the application of a classification algorithm to establish a simple model could quickly and accurately find potentially benign TNs and suggest their follow-up, which helped achieve our goal of reducing FNA.
This study had some limitations. First, as a provincial hospital, many patients were initially diagnosed with thyroid cancer in subordinate hospitals and suggested to come to our hospital for further examination. In addition, some patients with no certain pathological diagnosis (include Bethesda III and IV) were excluded from this study, resulting in some sampling bias. Second, some TNs were discarded because they could not be used to measure the stiffness of the surrounding 2-mm tissue. Finally, this was a single-center study with a relatively small sample size. Further testing of this classification algorithm requires multicenter studies with a large sample size.
Conclusion
In conclusion, a simple classification algorithm was applied to improve the diagnostic performance of ultrasound elastic parameters of TNs, avoiding FNA in 57.3% of nodules.
Data Availability Statement
The raw data supporting the conclusions of this article will be made available by the authors, without undue reservation.
Ethics Statement
The ethics committee of the First Affiliated Hospital of the University of Science and Technology of China (USTC) approved this prospective study. Written informed consent to participate in this study was provided by the participants’ legal guardian/next of kin.
Author Contributions
LX, XL, XY, YC, NH, and LH participated in literature search, data acquisition, data analysis, or data interpretation. XL and LH conceived and designed the study, and critically revised the manuscript, performed the research, wrote the first draft, collected and analyzed the data. XL, NH, and LH participated in paper writing and revised the manuscript. All authors contributed to the article and approved the submitted version.
Conflict of Interest
The authors declare that the research was conducted in the absence of any commercial or financial relationships that could be construed as a potential conflict of interest.
Publisher’s Note
All claims expressed in this article are solely those of the authors and do not necessarily represent those of their affiliated organizations, or those of the publisher, the editors and the reviewers. Any product that may be evaluated in this article, or claim that may be made by its manufacturer, is not guaranteed or endorsed by the publisher.
References
1. Zhu CL, Li SX, Gao X, Zhu GC, Song ML, Gao F. Retrospective Analysis of Thyroid Nodules: Thyroid Cancer Risk Factors in Suzhou, China. Clin Lab (2018) 64(3):333–8. doi: 10.7754/Clin.Lab.2017.170829
2. Bray F, Ferlay J, Soerjomataram I, Siegel RL, Torre LA, Jemal A. Global Cancer Statistics 2018: GLOBOCAN Estimates of Incidence and Mortality Worldwide for 36 Cancers in 185 Countries. CA Cancer J Clin (2018) 68(6):394–424. doi: 10.3322/caac.21492
3. Du LB, Li RH, Ge MH, Wang YQ, Li HZ, Chen WQ, et al. Incidence and Mortality of Thyroid Cancer in China,2008-2012. Chin J Cancer Res (2019) 31(1):144–51. doi: 10.21147/j.issn.1000-9604.2019.01.09
4. Zhou SC, Liu TT, Zhou J, Huang YX, Guo Y, Yu JH, et al. An Ultrasound Radiomics Nomogram for Preoperative Prediction of Central Neck Lymph Node Metastasis in Papillary Thyroid Carcinoma. Front Oncol (2020) 10:1591. doi: 10.3389/fonc.2020.01591
5. Zhang YC, Wu Q, Chen YT, Wang Y. A Clinical Assessment of an Ultrasound Computer-Aided Diagnosis System in Differentiating Thyroid Nodules With Radiologists of Different Diagnostic Experience. Front Oncol (2020) 10:557169. doi: 10.3389/fonc.2020.557169
6. Kwak JY, Han KH, Yoon JH, Moon HJ, Son EJ, Park SH, et al. Thyroid Imaging Reporting and Data System for US Features of Nodules: A Step in Establishing Better Stratification of Cancer Risk. Radiology (2011) 260(3):892–9. doi: 10.1148/radiol.11110206
7. Tessler FN, Middleton WD, Grant EG, Hoang JK, Berland LL, Teefey SA, et al. ACR Thyroid Imaging, Reporting and Data System (TI-RADS): White Paper of the ACR TI-RADS Committee. J Am Coll Radiol (2017) 14(5):587–95. doi: 10.1016/j. jacr.2017.01.046
8. Russ G, Bonnema SJ, Erdogan MF, Durante C, Ngu R, Leenhardt L. European Thyroid Association Guidelines for Ultrasound Malignancy Risk Stratification of Thyroid Nodules in Adults: The EU-TIRADS. Eur Thyroid J (2017) 6(5):225–37. doi: 10.1159/000478927
9. Han DY, Sohn YM, Seo M, Yun SJ, Park WS, Jeon SH, et al. Shear-Wave Elastography in Thyroid Ultrasound: Can Be a Predictor of Extrathyroidal Extension and Cervical Lymph Node Metastasis in Papillary Thyroid Carcinoma? Med (Baltimore) (2020) 99(52):e23654. doi: 10.1097/MD.0000000000023654
10. Sedlackova Z, Herman J, Furst T, Salzman R, Vachutka J, Herman M. Shear Wave Elastography in Diffuse Thyroid Disease. BioMed Pap Med Fac Univ Palacky Olomouc Czech Repub (2021) 165(2):152–6. doi: 10.5507/bp.2020.018
11. Kara T, Ateş F, Durmaz MS, Akyürek N, Durmaz FG, Özbakır B, et al. Assessment of Thyroid Gland Elasticity With Shear-Wave Elastography in Hashimoto’s Thyroiditis Patients. J Ultrasound (2020) 23(4):543–51. doi: 10.1007/s40477-020-00437-y
12. Zhang YF, Xu HX, He Y, Liu C, Guo LH, Liu LN, et al. Virtual Touch Tissue Quantification of Acoustic Radiation Force Impulse: A New Ultrasound Elastic Imaging in the Diagnosis of Thyroid Nodules. PloS One (2012) 7(11):e49094. doi: 10.1371/journal.pone.0049094
13. Sun CY, Lei KR, Liu BJ, Bo XW, Li XL, He YP, et al. Virtual Touch Tissue Imaging and Quantification (VTIQ) in the Evaluation of Thyroid Nodules: The Associated Factors Leading to Misdiagnosis. Sci Rep (2017) 7:41958. doi: 10.1038/srep41958
14. Calvete AC, Mestre JD, Gonzalez JM, Martinez ES, Sala BT, Zambudio AR. Acoustic Radiation Force Impulse Imaging for Evaluation of the Thyroid Gland. J Ultrasound Med (2014) 33(6):1031–40. doi: 10.7863/ultra.33.6.1031
15. Hu L, He NA, Xie L, Ye XJ, Liu X, Pei C, et al. Evaluation of the Perinodular Stiffness Potentially Predicts the Malignancy of the Thyroid Nodules. J Ultrasound Med (2020) 39(11):2183–93. doi: 10.1002/jum.15329
16. Hu L, Liu X, Pei C, Xie L, He NA. Assessment of Perinodular Stiffness in Differentiating Malignant From Benign Thyroid Nodules. Endocrine Connect (2021) 10(5):492–501. doi: 10.1530/EC-21-0034
17. Harper PR. A Review and Comparison of Classification Algorithms for Medical Decision Making. Health Policy (2005) 71(3):315–31. doi: 10.1016/j.healthpol.2004.05.002
18. Baltzer PA, Dietzel M, Kaiser WA. A Simple and Robust Classification Tree for Differentiation Between Benign and Malignant Lesions in MR-Mammography. Eur Radiol (2013) 23(8):2051–60. doi: 10.1007/s00330-013-2804-3
19. Marino MA, Clauser P, Woitek R, Wengert GJ, Kapetas P, Bernathova M, et al. A Simple Scoring System for Breast MRI Interpretation: Does It Compensate for Reader Experience? Eur Radiol (2016) 26(8):2529–37. doi: 10.1007/s00330-015-4075-7
20. Woitek R, Spick C, Schernthaner M, Rudas M, Kapetas P, Bernathova M, et al. A Simple Classification System (the Tree Flowchart) for Breast MRI Can Reduce the Number of Unnecessary Biopsies in MRI-Only Lesions. Eur Radiol (2017) 27(9):3799–809. doi: 10.1007/s00330-017-4755-6
21. Kapetas P, Woitek R, Clauser P, Bernathova M, Pinker K, Helbich TH, et al. A Simple Ultrasound Based Classification Algorithm Allows Differentiation of Benign From Malignant Breast Lesions by Using Only Quantitative Parameters. Mol Imaging Biol (2018) 20(6):1053–60. doi: 10.1007/s11307-018-1187-x
22. Cibas ES, Ali SZ. The 2017 Bethesda System for Reporting Thyroid Cytopathology. Thyroid (2017) 27(11):1341–6. doi: 10.1089/thy.2017.0500
23. Haugen BR, Alexander EK, Bible KC, Doherty GM, Mandel SJ, Nikiforov YE, et al. American Thyroid Association Management Guidelines for Adult Patients With Thyroid Nodules and Differentiated Thyroid Cancer. Thyroid (2016) 26(1):1–133. doi: 10.1089/thy.2015.0020
24. Gao LY, Xi XH, Jiang YX, Yang X, Wang Y, Zhu SL, et al. Comparison Among TIRADS (ACR TI-RADS and KWAKTI-RADS) and 2015 ATA Guidelines in the Diagnostic Efficiency of Thyroid Nodules. Endocrine (2019) 64(1):90–6. doi: 10.1007/s12020-019-01843-x
25. Koc AM, Adıbelli ZH, Erkul Z, Sahin Y, Dilek I. Comparison of Diagnostic Accuracy of ACR-TIRADS, American Thyroid Association (ATA), and EU-TIRADS Guidelines in Detecting Thyroid Malignancy. Eur J Radiol (2020) 133:109390. doi: 10.1016/j.ejrad.2020.109390
26. Hekimsoy İ, Öztürk E, Ertan Y, Orman MN, Kavukçu G, Özgen AG, et al. Diagnostic Performance Rates of the ACR-TIRADS and EU-TIRADS Based on Histopathological Evidence. Diagn Interv Radiol (2021) 27(4):511–8. doi: 10.5152/dir.2021.20813
27. Kim HJ, Kwak MK, Choi IH, Jin SY, Park HK, Byun DW, et al. Utility of Shear Wave Elastography to Detect Papillary Thyroid Carcinoma in Thyroid Nodules: Efficacy of the Standard Deviation Elasticity. Korean J Internal Med (2019) 34(4):850–7. doi: 10.3904/kjim.2016.326
28. Wang F, Chang C, Gao Y, Chen YL, Chen M, Feng LQ. Does Shear Wave Elastography Provide Additional Value in the Evaluation of Thyroid Nodules That Are Suspicious for Malignancy? J Ultrasound Med (2016) 35(11):2397–404. doi: 10.7863/ultra.15.09009
Keywords: decision tree, shear wave elastography, stiffness, thyroid nodule, ultrasound
Citation: Liu X, Xie L, Ye X, Cui Y, He N and Hu L (2022) Evaluation of Ultrasound Elastography Combined With Chi-Square Automatic Interactive Detector in Reducing Unnecessary Fine-Needle Aspiration on TIRADS 4 Thyroid Nodules. Front. Oncol. 12:823411. doi: 10.3389/fonc.2022.823411
Received: 27 November 2021; Accepted: 26 January 2022;
Published: 16 February 2022.
Edited by:
Po-Hsiang Tsui, Chang Gung University, TaiwanReviewed by:
Lei Zhu, Fifth Affiliated Hospital of Wenzhou Medical University, ChinaPietro Locantore, Catholic University of the Sacred Heart, Italy
Zhimin Ding, Southern University of Science and Technology, China
Yijie Dong, Shanghai Jiaotong University School of Medicine, China
Copyright © 2022 Liu, Xie, Ye, Cui, He and Hu. This is an open-access article distributed under the terms of the Creative Commons Attribution License (CC BY). The use, distribution or reproduction in other forums is permitted, provided the original author(s) and the copyright owner(s) are credited and that the original publication in this journal is cited, in accordance with accepted academic practice. No use, distribution or reproduction is permitted which does not comply with these terms.
*Correspondence: Nianan He, aGVuaWFuYW43MUBxcS5jb20=; Lei Hu, ODM1MTAzMTJAcXEuY29t