- 1Perioperative Medicine Research Group, Instituto de Investigación Sanitaria la Fe, Valencia, Spain
- 2Departament de Anesthesiology, Hospital Universitari i Politécnic la Fe, Valencia, Spain
- 3Bioinformatics and Biostatistics Unit, INCLIVA Biomedical Research Institute, Valencia, Spain
- 4Breast Cancer Research Group, Molecular and Cellular Oncolgy Unit, Biomedical Research Institute, INCLIVA, Valencia, Spain
- 5Euro-Periscope: The Onco-Anaesthesiology Research Group (RG) of European Society of Anaesthesiology & Intensive Care (ESA-IC), Brussels, Belgium
- 6Department of Anaesthesiology and Perioperative Medicine, Mater University Hospital, University College Dublin, Dublin, Ireland
Background: Opioid receptors are expressed not only by neural cells in the central nervous system, but also by many solid tumor cancer cells. Whether perioperative opioids given for analgesia after tumor resection surgery might inadvertently activate tumor cells, promoting recurrence or metastasis, remains controversial. We analysed large public gene repositories of solid tumors to investigate differences in opioid receptor expression between normal and tumor tissues and their association with long–term oncologic outcomes.
Methods: We investigated the normalized gene expression of µ, κ, δ opioid receptors (MOR, KOR, DOR), Opioid Growth Factor (OGFR), and Toll-Like 4 (TLR4) receptors in normal and tumor samples from twelve solid tumor types. We carried out mixed multivariable logistic and Cox regression analysis on whether there was an association between these receptors’ gene expression and the tissue where found, i.e., tumor or normal tissue. We also evaluated the association between tumor opioid receptor gene expression and patient disease–free interval (DFI) and overall survival (OS).
Results: We retrieved 8,780 tissue samples, 5,852 from tumor and 2,928 from normal tissue, of which 2,252 were from the Genotype Tissue Expression Project (GTEx) and 672 from the Cancer Genome Atlas (TCGA) repository. The Odds Ratio (OR) [95%CI] for gene expression of the specific opioid receptors in the examined tumors varied: MOR: 0.74 [0.63–0.87], KOR: 1.27 [1.17–1.37], DOR: 1.66 [1.48–1.87], TLR4: 0.29 [0.26–0.32], OGFR: 2.39 [2.05–2.78]. After controlling all confounding variables, including age and cancer stage, there was no association between tumor opioid receptor expression and long–term oncologic outcomes.
Conclusion: Opioid receptor gene expression varies between different solid tumor types. There was no association between tumor opioid receptor expression and recurrence. Understanding the significance of opioid receptor expression on tumor cells remains elusive.
Introduction
Surgery remains a primary treatment for 70% of solid tumors (1) but analgesia after resection is challenging. Pain and nociceptive transmission involve neuronal networks with various receptor types that elicit either activation or suppression of the stimuli (2). Opioids are still the mainstay of postoperative pain management. Their primary site of action is the μ opioid receptor (MOR) which is expressed at various central nervous system (CNS) locations along the pain pathway. Opioid drugs activate MORs to suppress ascending nociception and enhance descending pain inhibition (3, 4). However, one-dimensional reliance on opioid medication has disadvantages. First, MOR is expressed in other tissues such as the brain stem and bowel, leading to undesired side effects such as respiratory depression, nausea, and ileus. Second, repeated, prolonged opioid administration results in hyperalgesia and has been linked to the ongoing problem of opioid dependence (5–8). Third, opioids suppress cell–mediated immunity and directly activate tumor angiogenesis, thereby potentially facilitating residual tumor cell spread (9).
Opioid drugs act as agonists not only at MOR but also at δ–opioid receptors (DOR), and both can be expressed by tumor cells (10). Cancer metastasis and proliferation may be associated with the activation of these opioid receptors through different pathways (11). However, MOR, DOR, and κ opioid receptors (KOR), in addition to opioid growth factor receptor (OGFR) and Toll–like receptor 4 (TLR4), have been shown to promote tumor cell migration (12–14). Previous studies aiming to elucidate the role of these receptors in cancer differ widely in their methodology, e.g., immunohistochemistry (IHC) or nucleic acid polymerase chain reaction (PCR) amplification, as well as in the studied samples, e.g., tissue or cell lines, and in targeted receptors (eTable 1 in the Supplementary Digital Content) (15–24).
The evolution of genetic sequencing technologies and the drive to unravel the mechanisms underlying many human diseases has led to the appearance of large repositories of genetic data such as the Genotype Tissue Expression Project (GTEx) and the Cancer Genome Atlas (TCGA). The TCGA is a National Institute of Health (NIH) sponsored project that aims to discover significant cancer-causing genome alterations in large cohorts of tumors through large-scale genome sequencing (25, 26).
Our study objective was to analyse opioid receptor gene expression in tumors compared to normal tissue and to evaluate the association between this and long-term oncologic outcome, defined as overall survival (OS) and disease-free interval (DFI). Gene expression data was obtained from GTEx and TCGA.
Materials and Methods
In this analysis we followed the recommendations on reporting results from observational studies (STROBE guidelines. https://www.equator-network.org/reporting-guidelines/strobe/).
Population
We analyzed data from normal and tumor tissues from bladder, breast, colon, liver, salivary gland, esophagus, prostate, stomach, thyroid, lung, and kidney tumors.
Data Collection
Unified GTEx and TCGA gene expression data for MOR, DOR, KOR, TLR4, and OGFR genes were obtained using an established technique (27) (Data record 3) for each tissue type. This dataset includes a strict selection of high-quality RNA-Seq samples processed with the same analysis pipeline and corrected for unwanted non-biological variation that affects comparative analyses. In addition, gene expression values were reported in Fragments per Kilobase Million (FPKM) units.
TCGA survival data were downloaded from the TCGA TARGET GTEx dataset deposited in Xenabrowser (https://xenabrowser.net/). We collected DFI and OS. The remaining clinical data for TCGA samples were obtained from TCGABiolinks (28). Information for GTEx individuals was directly downloaded from the GTEx project website (GTEx Analysis Release V8). Clinical information from the different sources and gene expression data in log2 (FPKM+1) scale were formatted and merged.
Definitions
OS is the length of time from either the date of cancer diagnosis or the start of treatment and death from any cause. DFI is the length of time between primary cancer treatment and any signs or symptoms reappearance (29).
Statistical Analysis
We used data of all available patients without formal sample size calculation. Also, as the purpose was to explore a pathophysiological hypothesis, we did not specify any a priori effect size. We reported continuous variables as median and 25th–75th percentiles and categorical variables as numbers and percentages. Distribution was assessed by inspecting quantile–quantile plots, and log-transformation was carried out if the variable distribution violated the normality assumption. Finally, descriptive analyses were performed to summarize patient characteristics.
To assess the association between opioid receptor gene expression and type of tissue, i.e., control versus tumor, we fitted mixed logistic models introducing MOR, KOR, DOR, OGFR, and TLR4 genes as covariables, and primary tumor site as a random effect to consider the variability between different tumor sites. This model was fitted for tumors with data available for every receptor included in the analysis. We fitted a logistic model with all the receptor data available for each tumor type as a sensitivity analysis.
To assess the association between opioid receptor gene expression and DFI and OS, we fitted a mixed Cox model introducing MOR, KOR, DOR, OGFR, and TLR4 genes, age at diagnosis, and cancer stage as covariables. Primary site of tumor was a random effect to consider the variability between different tumor sites.
Statistical significance was set for two–tailed test at P<0.05. No missing values imputation and no correction for multiple comparisons was prespecified: thus, all the findings should be viewed as exploratory. All analyses were performed with R 4.0.3 (The R Foundation for Statistical Computing, www.r-project.org)
Results
We retrieved 8,780 tissue samples: 5,852 from tumor and 2,924 from normal control tissues, of which 2,252 and 672 were from the GTEx and TCGA repository, respectively. Sample characteristics are shown in Table 1. OGFR gene expression was highest while MOR gene expression was lowest, with comparable values between control and tumor samples on every overall gene expression. Median and percentiles values and violin and density plots (Figure 1) show considerable overlap. Opioid receptor expression by tumor primary site is reported in Figure 2.
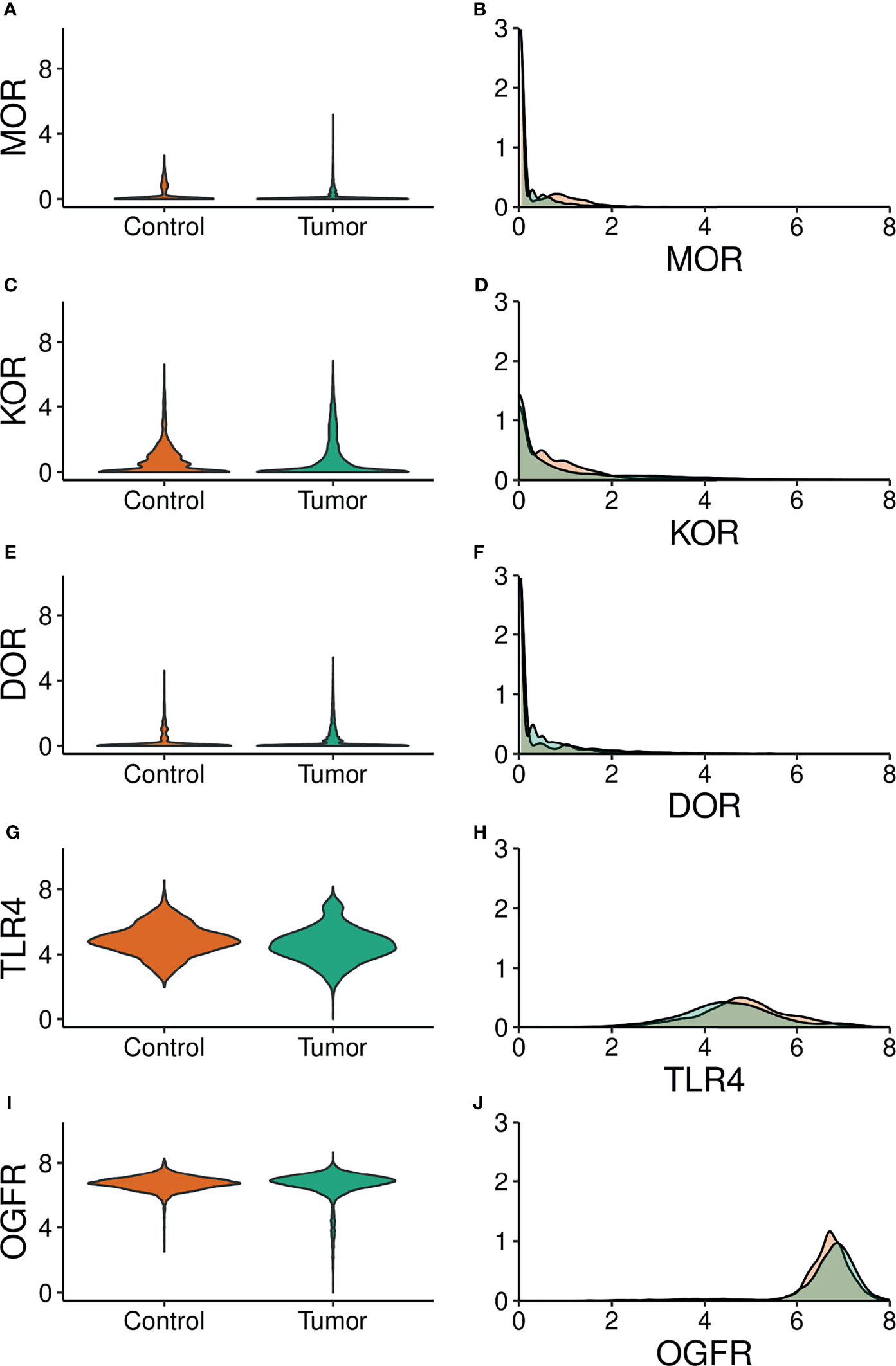
Figure 1 Violin (Left panels) and density (Right panels) plots of the expression of opioid receptor genes. Green: tumor samples. Orange: control samples. MOR, µ opioid receptor; KOR, κ opioid receptor; DOR, δ opioid receptor; TLR4, toll–like receptor; OGFR, opioid growth factor receptor. (A, C, E, G, I) Gene expression (Log scale) is on the y axis. (B, D, F, H, J) Gene expression (Log scale) s on the x axis.
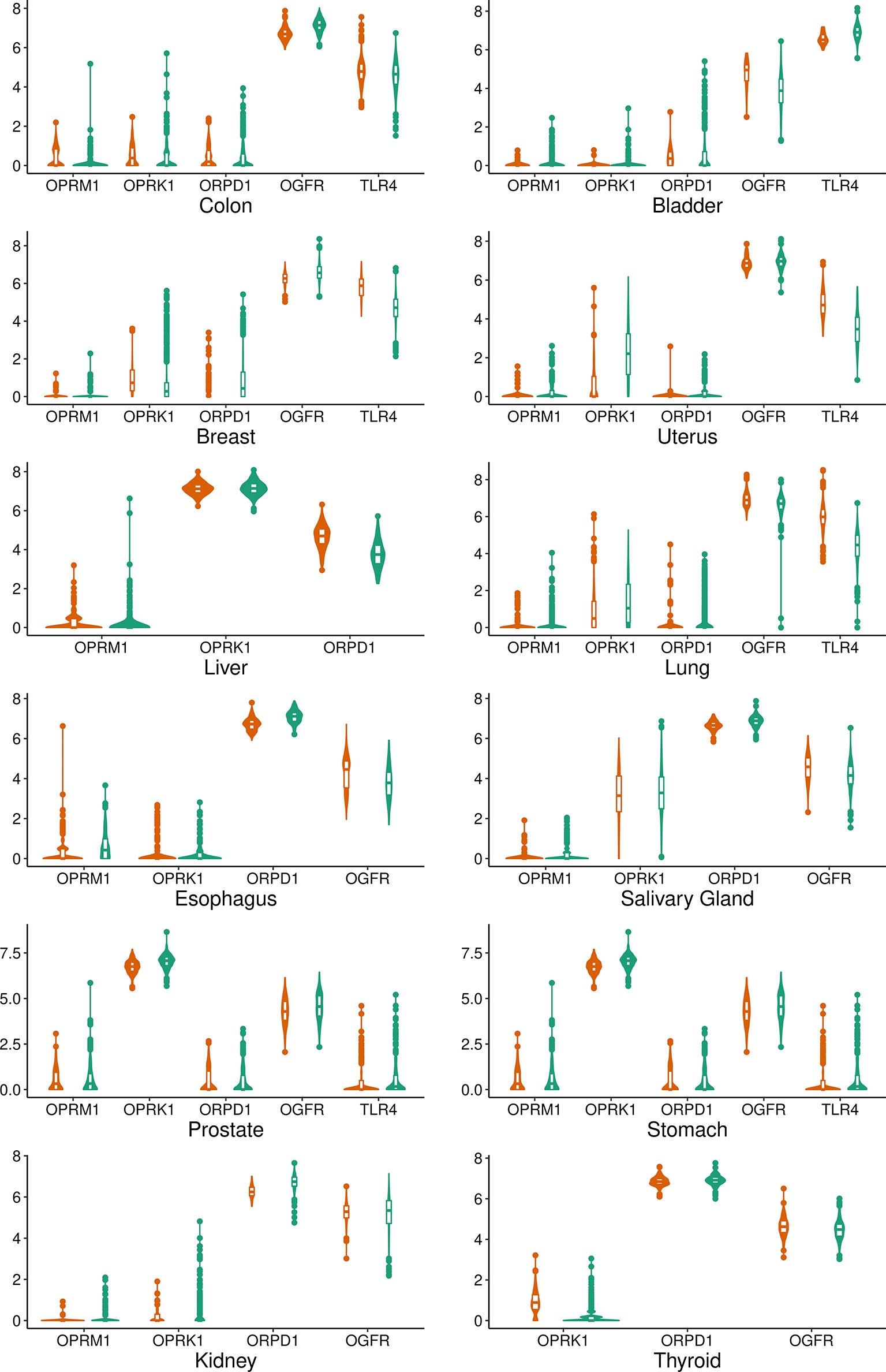
Figure 2 Violin and box plot graphs of the expression of opioid receptors genes by tumor type. Green: tumor samples. Orange: control samples. MOR, µ opioid receptor; KOR, κ opioid receptor; DOR, δ opioid receptor; TLR4, toll–like receptor; OGFR, opioid growth factor receptor.
The logistic model’s estimates are reported in Table 2. Opioid receptors were significantly associated with tumor samples, albeit differently, as some genes associated positively and others negatively. The estimated standard deviation among tumors, i.e. 3.06, is bigger than the largest estimate among the fixed effects, i.e. OGFR estimate 2.39, suggesting considerable effect differences among tumors (Figure 3). Logistic models estimated for each tumor are reported in Figure 4 and show considerable variability among opioid receptor estimates across tumor types.
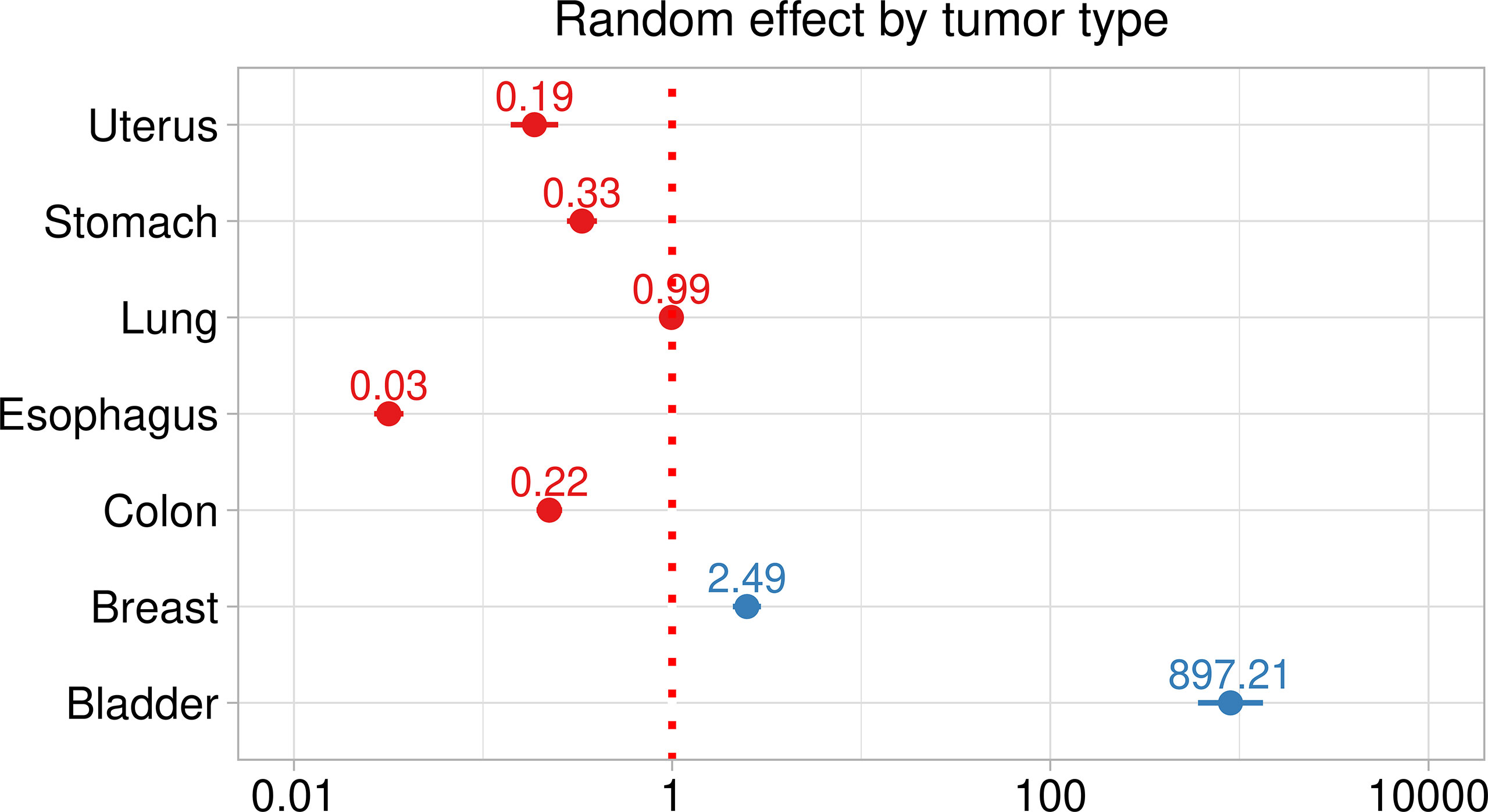
Figure 3 Random effect plot of the mixed logistic model assessing the association between opioid receptors expression and type of tissue. Red dotted line, significance threshold. Dots effect estimates and bar 95% Confidence intervals.
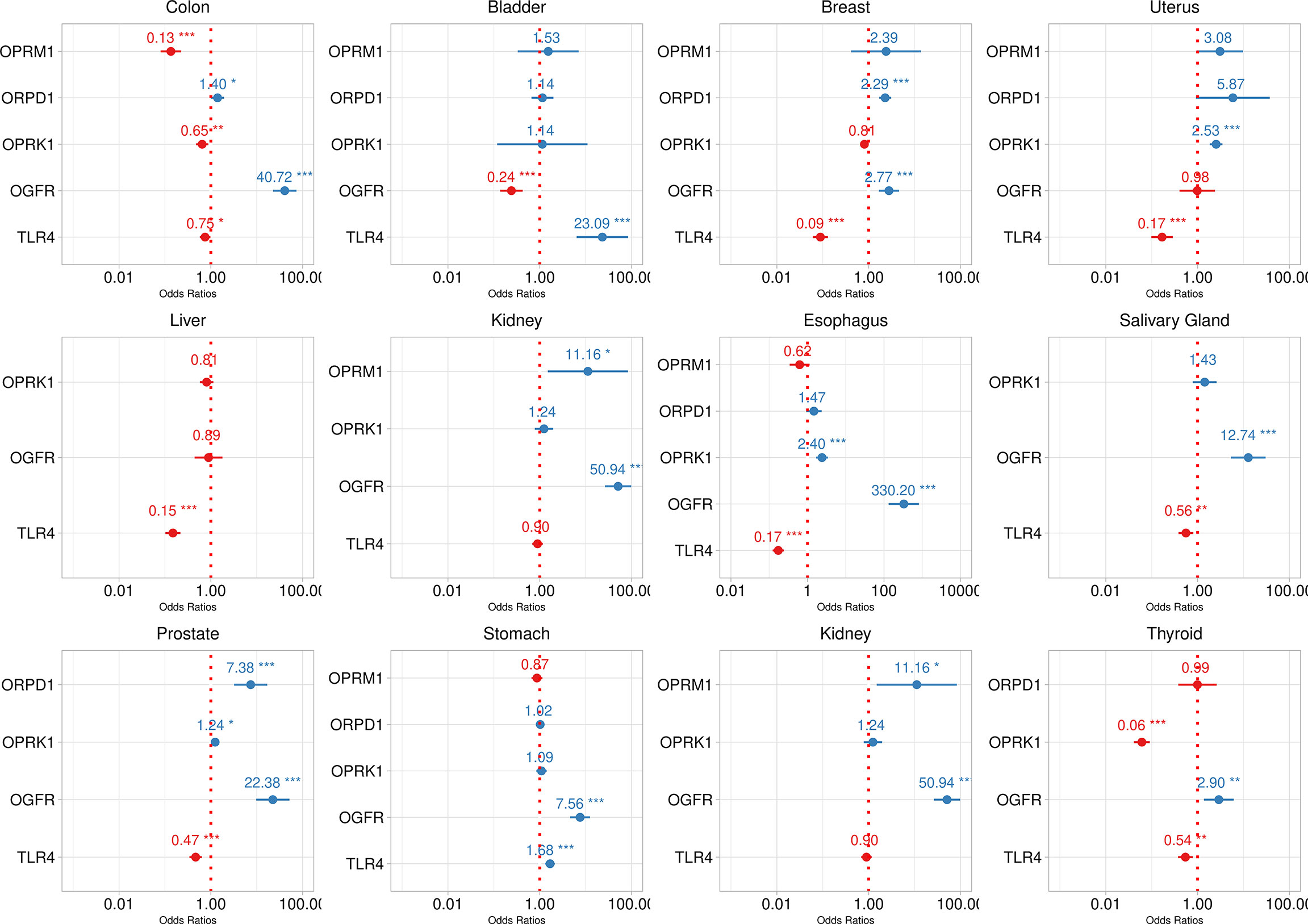
Figure 4 Logistic model fit of opioid receprotrs association with tumor tissue by tumor type. Dotted red line represents no effect. Estimates are reported as red or blue when the odds ratio point estimate is lower or greater than one respectively. Dots are point estimates and bars 95% confidence intervals. Statistical significance is reported as * < 0.05, ** < 0.001, *** < 0.001.
Mixed Cox models estimates for DFI and OS are reported in Table 3. After controlling for age and cancer stage, we found no association overall between opioid receptor expression and long-term outcomes except a weak effect for KOR on OS. The cancer stage is by far the predominant effect in both DFI and OS models as was expected.
Discussion
This study’s’ main findings can be summarized as follows: Firstly, higher or lower opioid receptor gene expression within tumors is variable depending on the specific tumor type; Secondly, single gene expression also varies depending on tumor type; Thirdly, there was no association between opioid gene receptor expression and DFI or OS after controlling for age and tumor stage.
This analysis has several strengths. First, to our knowledge, this is the first analysis of large public genetic databases focusing specifically on tumor opioid receptor expression and their link to cancer outcome. Secondly, we selected normalized data through a previously published meticulous procedure that consistently removes the batch effect from samples. Thirdly, we controlled for confounding bias by performing a time–to–event analysis, including potential confounders such as age and tumor stage. We also assessed the effect of specific cancer types by adding a random effect to the multivariable model to account for the hierarchical structure of the data. Fourthly, we included all previously studied opioid receptors known to be involved in perioperative opioid drug binding in our analysis.
The role of tumor opioid receptor expression on tumor growth and metastasis has generated considerable interest among researchers involved in surgical oncology (1). Because of the pivotal role of opioid analgesics in the perioperative process, it has been speculated that activation of these receptors could cause cancer cells to proliferate, migrate and escape immune control. Our findings add further to previous studies assessing opioid receptor expression in different tumors. Higher MOR expression was found on prostate cancer samples compared to unpaired control tissue (22). Likewise, in a study that compared human lung cancer samples with non-tumor adjacent tissue samples, MOR expression was significantly increased in tumor tissue from patients with metastatic lung cancer had an approximately twofold increase in MOR expression (21).
Higher expression of MOR was associated with tumor tissue in gastric cancer, hepatocellular carcinoma, and colorectal cancer samples (17–20, 24). In contrast, this association of MOR-1 with oncological results was not observed in other tumors. For instance, in esophageal squamous cell carcinoma (ESCC), MOR expression in the cytoplasm was associated with lymph node metastases. However, no link was found between MOR expression and the OS of patients with ESCC (18).
In a retrospective analysis among breast cancer patients, which analyzed the effect of anesthetic technique on MOR expression, the authors found that general anesthesia with opioid analgesia increased MOR expression in the resected tumor compared to anesthetic technique with locoregional analgesia (30), These results support the hypothesis that the opioid receptor genetic footprint varies with tumor type. This is consistent with recent data from a triple-negative breast tumor databank, which analyzed the same receptors as us and found that MOR, OPRD, and OPRK were overexpressed, while TLR4 was downregulated. Furthermore, these authors found that higher doses of intraoperative opioids were associated with somewhat worse oncologic outcomes than patients receiving lower doses during surgery (16). A thorough mapping of different receptors’ expression is important because opposing effects have been described, with some receptor activation having protumor effects while others have potentially tumor suppressing effects. This is even more important since both exogenous and endogenous opioid receptor agonists may play a different role depending on the specific profile of receptor expression, while opioid receptor antagonists such as methylnaltrexone have found to be associated with longer median survival in an unplanned posthoc analysis of two clinical trials (31).
We found no association between opioid receptor expression and long-term outcomes such as DFI and OS. Existing data on this matter are diverging. For example, while some studies found an association between MOR expression in particular and cancer recurrence (18, 19, 22), others did not (16, 24). Indeed, available research on this topic seems to point out that there is no one-size-fits-all explanation to this question. Further investigations on the specific receptor profile of each cancer strain should lay the foundation on whether opioid receptors can be included as oncologic prognostic markers.
Studies that assess opioid receptor expression in cancer rely on immunohistochemistry assay, while quantitative methods such as quantitative real-time polymerase chain reaction (qRT–PCR) are seldom reported, and with different procedures and primers (eTable 1 Supplementary Material) (15, 19, 20). Since there are no consensus guidelines on how to perform these assays (32), we consider that availing of large and validated databases such as the TCGA and GTEx is a powerful tool to draw a genetic footprint of opioid receptors in tumor cells. Furthermore, as our present results suggest, the impact of opioid receptors on cancer cells does not seem to be based on a simple pathway involving an individual receptor overexpression and is probably a more integrated mechanism involving several receptor targets with different effects that can vary depending on specific tumor type.
Furthermore, genetic content within tumors is variable and opioid receptors can present single nucleotide polymorphisms (SNPs). This type of polymorphisms on known oncogenes such as p53 and X-Ray Repair Cross Complementing 3 (XRRCC3) genes have been studied to elucidate their effect on cancer susceptibility with conflicting results to date (33–35). For instance, particular SNPs such as the A118G have been linked to reduced sensitivity to opioid medication in the patient suffering from chronic pain (36, 37) and cancer (19) and even cancer recurrence in specific tumor types and populations (38, 39), Also, TLR4 gene polymorphisms have also been studied and may play a role in proliferation and differentiation and multiple isoforms of receptor subtype resulting from alternative splicing of the pre-mRNA transcript have been identified albeit their functional role has yet to be clarified (40). Investigators are beginning to expand the horizon outside the genetic profile of opioid receptors and to include specific genetic alterations such as Cyclin-Dependent Kinase Inhibitor 2A (CDKN2A) mutations (41), although the influence that specific influence of individual receptor isoforms is still a matter of debate (42).
Several limitations must be acknowledged. For instance, differences in baseline characteristics from GTEx and TCGA repositories may be present. Also, although we controlled for age and cancer stage, the effect of other confounding factors not included in the analysis, such as type of surgery or pathologic stage or opioid agonists or antagonists administration, cannot be ruled out. These parameters could have a modifying effect on the association between opioid receptor expression and long-term cancer outcomes. Furthermore, we also acknowledge that while we assessed all the most common opioid receptor genes, other molecular pathways can be involved in the effect of opioids on cancer growth. Finally, because of the hypothesis-generating purpose of this study, we did not set any a priori effect threshold or multiple comparisons correction; hence some results’ statistical significance and the potential hypothesis derived from them should be confirmed in future trials.
In conclusion, the most common solid tumors express higher opioid receptor genes than normal tissue, but variably depending on the primary tumor analyzed. No association was found between disease-free and overall survival and opioid gene expression after controlling for age and tumor stage. Further studies are warranted to elucidate the specific genetic footprint of opioid receptors in each cancer type and the potential role of gene polymorphisms.
Data Availability Statement
Publicly available datasets were analyzed in this study. This data can be found here: https://www.nature.com/articles/sdata201861#ref-CR22.
Authors Contributions
AB: This author conceived the idea, helped with data acquisition, critical review of the content, and manuscript preparation. SZ: This author coded the data acquisition from the repositories, provided a critical review of the content, and helped with manuscript preparation. IG-C: This author provided a critical review of the content, and helped with manuscript preparation. PE: This author provided a critical review of the content, and helped with manuscript preparation. MA: This author provided a critical review of the content, and helped with manuscript preparation. DB: This author provided a critical review of the content, and helped with manuscript preparation. OD-C: This author provided a critical review of the content, and helped with manuscript preparation. GM: This author conceived the idea, helped with data acquisition, critical review of the content, and manuscript preparation. All authors contributed to the article and approved the submitted version.
Conflict of Interest
OD-C: Received payment for educational talks and scientific conferences from MSD (Merck Sharp & Dohme, Inc.).
The remaining authors declare that the research was conducted in the absence of any commercial or financial relationships that could be construed as a potential conflict of interest.
Publisher’s Note
All claims expressed in this article are solely those of the authors and do not necessarily represent those of their affiliated organizations, or those of the publisher, the editors and the reviewers. Any product that may be evaluated in this article, or claim that may be made by its manufacturer, is not guaranteed or endorsed by the publisher.
Supplementary Material
The Supplementary Material for this article can be found online at: https://www.frontiersin.org/articles/10.3389/fonc.2022.801411/full#supplementary-material
References
1. Sullivan R, Alatise OI, Anderson BO, Audisio R, Autier P, Aggarwal A, et al. Global Cancer Surgery: Delivering Safe, Affordable, and Timely Cancer Surgery. Lancet Oncol (2015) 16:1193–224. doi: 10.1016/S1470-2045(15)00223-5
2. Peirs C, Seal RP. Neural Circuits of Pain: Recent Advances and Current Perspectives. Science (2016) 354:578–84. doi: 10.1126/science.aaf8933
3. Stein C. The Control of Pain in Peripheral Tissue by Opioids. N Engl J Med (1995) 332(25):1685–90. doi: 10.1056/NEJM199506223322506
4. Waldhoer M, Bartlett SE, Whistler JL. Opioid Receptors. Annu Rev Biochem (2004) 73:953–90. doi: 10.1146/annurev.biochem.73.011303.073940
5. Hollmann MW, Rathmell JP, Lirk P. Optimal Postoperative Pain Management: Redefining the Role for Opioids. Lancet (2019) 393(10180):1483–5. doi: 10.1016/S0140-6736(19)30854-2
6. Brat GA, Agniel D, Beam A, Yorkgitis B, Bicket M, Homer M, et al. Postsurgical Prescriptions for Opioid Naive Patients and Association With Overdose and Misuse: Retrospective Cohort Study. BMJ (2018) 360:j5790. doi: 10.1136/bmj.j5790
7. Neuman MD, Bateman BT, Wunsch H. Inappropriate Opioid Prescription After Surgery. Lancet (2019) 393:1547–57. doi: 10.1016/S0140-6736(19)30428-3
8. Makary MA, Overton HN, Wang P. Overprescribing is Major Contributor to Opioid Crisis. BMJ (2017) 359:j4792. doi: 10.1136/bmj.j4792
9. Boland JW, McWilliams K, Ahmedzai SH, Pockley AG. Effects of Opioids on Immunologic Parameters That are Relevant to Anti-Tumour Immune Potential in Patients With Cancer: A Systematic Literature Review. Br J Cancer (2014) 111:866–73. doi: 10.1038/bjc.2014.384
10. Wigmore T, Farquhar-Smith P. Opioids and Cancer: Friend or Foe? Curr Opin Support Palliat Care (2016) 10:109–18. doi: 10.1097/SPC.0000000000000208
11. Sekandarzad MW, Van Zundert AAJ, Lirk PB, Doornebal CW, Hollmann MW. Perioperative Anesthesia Care and Tumor Progression. Anesth Analg (2017) 124:1697–708. doi: 10.1213/ANE.0000000000001652
12. Kim JY, Ahn HJ, Kim JK, Kim J, Lee SH, Chae HB. Morphine Suppresses Lung Cancer Cell Proliferation Through the Interaction With Opioid Growth Factor Receptor: An In Vitro and Human Lung Tissue Study. Anesth Analg (2016) 123:1429–36. doi: 10.1213/ANE.0000000000001293
13. Liao SJ, Zhou YH, Yuan Y, Li D, Wu FH, Wang Q, et al. Triggering of Toll-Like Receptor 4 on Metastatic Breast Cancer Cells Promotes αvβ3-Mediated Adhesion and Invasive Migration. Breast Cancer Res Treat (2012) 133:853–63. doi: 10.1007/s10549-011-1844-0
14. Matzner P, Sorski L, Shaashua L, Elbaz E, Lavon H, Melamed R, et al. Perioperative Treatment With the New Synthetic TLR-4 Agonist GLA-SE Reduces Cancer Metastasis Without Adverse Effects. Int J Cancer (2016) 138:1754–64. doi: 10.1002/ijc.29885
15. Jorand R, Biswas S, Wakefield DL, Tobin SJ, Golfetto O, Hilton K, et al. Molecular Signatures of Mu Opioid Receptor and Somatostatin Receptor 2 in Pancreatic Cancer. Mol Biol Cell (2016) 27:3659–72. doi: 10.1091/mbc.E16-06-0427
16. Montagna G, Gupta HV, Hannum M, Tan KS, Lee J, Scarpa JR, et al. Intraoperative Opioids are Associated With Improved Recurrence-Free Survival in Triple-Negative Breast Cancer. Br J Anaesth (2021) 126:367–76. doi: 10.1016/j.bja.2020.10.021
17. Nylund G, Pettersson A, Bengtsson C, Khorram-Manesh A, Nordgren S, Delbro DS. Functional Expression of μ-Opioid Receptors in the Human Colon Cancer Cell Line, HT-29, and Their Localization in Human Colon. Dig Dis Sci (2008) 53:461–6. doi: 10.1007/s10620-007-9897-y
18. Zhang YF, Xu QX, Liao LD, Xu XE, Wu JY, Wu ZY, et al. Association of Mu-Opioid Receptor Expression With Lymph Node Metastasis in Esophageal Squamous Cell Carcinoma. Dis Esophagus (2015) 28:196–203. doi: 10.1111/dote.12165
19. Yao YS, Yao RY, Zhuang LK, Qi WW, Lv J, Zhou F, et al. MOR1 Expression in Gastric Cancer: A Biomarker Associated With Poor Outcome. Clin Transl Sci (2015) 8:137–42. doi: 10.1111/cts.12246
20. Chen DT, Pan JH, Chen YH, Xing W, Yan Y, Yuan YF, et al. The Mu-Opioid Receptor is a Molecular Marker for Poor Prognosis in Hepatocellular Carcinoma and Represents a Potential Therapeutic Target. Br J Anaesth (2019) 122:e157–67. doi: 10.1016/j.bja.2018.09.030
21. Singleton PA, Mirzapoiazova T, Hasina R, Salgia R, Moss J. Increased μ-Opioid Receptor Expression in Metastatic Lung Cancer. Br J Anaesth (2014) 113:i103–8. doi: 10.1093/bja/aeu165
22. Zylla D, Gourley BL, Vang D, Jackson S, Boatman S, Lindgren B, et al. Opioid Requirement, Opioid Receptor Expression, and Clinical Outcomes in Patients With Advanced Prostate Cancer. Cancer (2013) 119:4103–10. doi: 10.1002/cncr.28345
23. Zhang H, Sun M, Zhou D, Gorur A, Sun Z, Zeng W, et al. Increased Mu-Opioid Receptor Expression is Associated With Reduced Disease-Free and Overall Survival in Laryngeal Squamous Cell Carcinoma. Br J Anaesth (2020) 125:722–9. doi: 10.1016/j.bja.2020.07.051
24. Díaz-Cambronero O, Mazzinari G, Giner F, Belltall A, Ruiz-Boluda L, Marqués-Marí A, et al. Mu Opioid Receptor 1 (MOR-1) Expression in Colorectal Cancer and Oncological Long-Term Outcomes: A Five-Year Retrospective Longitudinal Cohort Study. Cancers (Basel) (2020) 12(1):134. doi: 10.3390/cancers12010134
25. Grossman RL, Heath AP, Ferretti V, Varmus HE, Lowy DR, Kibbe WA, et al. Toward a Shared Vision for Cancer Genomic Data. N Engl J Med (2016) 375:1109–12. doi: 10.1056/nejmp1607591
26. Tomczak K, Czerwińska P, Wiznerowicz M. The Cancer Genome Atlas (TCGA): An Immeasurable Source of Knowledge. Contemp Oncol (Pozn) (2015) 19:A68–77. doi: 10.5114/wo.2014.47136
27. Wang Q, Armenia J, Zhang C, Penson AV, Reznik E, Zhang L, et al. Data Descriptor: Unifying Cancer and Normal RNA Sequencing Data From Different Sources. Sci Data (2018) 5:180061. doi: 10.1038/sdata.2018.61
28. Colaprico A, Silva TC, Olsen C, Garofano L, Cava C, Garolini D, et al. TCGAbiolinks: An R/Bioconductor Package for Integrative Analysis of TCGA Data. Nucleic Acids Res (2016) 44:e71. doi: 10.1093/nar/gkv1507
29. Buggy DJ, Freeman J, Johnson MZ, Leslie K, Riedel B, Sessler DI, et al. Systematic Review and Consensus Definitions for Standardised Endpoints in Perioperative Medicine: Postoperative Cancer Outcomes. Br J Anaesth (2018) 121:38–44. doi: 10.1016/j.bja.2018.03.020
30. Levins KJ, Prendeville S, Conlon S, Buggy DJ. The Effect of Anesthetic Technique on µ-Opioid Receptor Expression and Immune Cell Infiltration in Breast Cancer. J Anesth (2018) 32:792–6. doi: 10.1007/s00540-018-2554-031
31. Janku F, Johnson LK, Karp DD, Atkins JT, Singleton PA, Moss J. Treatment With Methylnaltrexone is Associated With Increased Survival in Patients With Advanced Cancer. Ann Oncol (2016) 27:2032–8. doi: 10.1093/annonc/mdw317
32. Du KN, Feng L, Newhouse A, Mehta J, Lasala J, Mena GE, et al. Effects of Intraoperative Opioid Use on Recurrence-Free and Overall Survival in Patients With Esophageal Adenocarcinoma and Squamous Cell Carcinoma. Anesth Analg (2018) 127:210–6. doi: 10.1213/ANE.0000000000003428
33. Kuang J, Yan X, Genders AJ, Granata C, Bishop DJ. An Overview of Technical Considerations When Using Quantitative Real-Time PCR Analysis of Gene Expression in Human Exercise Research. PloS One (2018) 13(5):1–27. doi: 10.1371/journal.pone.0196438
34. Manuguerra M, Saletta F, Karagas MR, Berwick M, Veglia F, Vineis P, et al. XRCC3 and XPD/ERCC2 Single Nucleotide Polymorphisms and the Risk of Cancer: A HuGE Review. Am J Epidemiol (2006) 164:297–302. doi: 10.1093/aje/kwj189
35. Chen X, Liu F, Li B, Wei YG, Yan LN, Wen TF. P53 Codon 72 Polymorphism and Liver Cancer Susceptibility: A Meta-Analysis of Epidemiologic Studies. World J Gastroenterol (2011) 17:1211–8. doi: 10.3748/wjg.v17.i9.1211
36. Kasai S, Ikda K. Pharmacogenomics of the Human µ-Opioid Receptor Pharmacogenomics. Pharmacogenomics (2011) 12:1305–20. doi: 10.2217/pgs.11.68
37. Janicki PK, Schuler G, Francis D, Bohr A, Gordin V, Jarzembowski T, et al. A Genetic Association Study of the Functional A118G Polymorphism of the Human μ-Opioid Receptor Gene in Patients With Acute and Chronic Pain. Anesth Analg (2006) 103:1011–7. doi: 10.1213/01.ane.0000231634.20341.88
38. Bortsov AV, Millikan RC, Belfer I, Boortz-Marx RL, Arora H, Mclean SA. μ-Opioid Receptor Gene A118G Polymorphism Predicts Survival in Patients With Breast Cancer. Anesthesiology (2012) 116:896–902. doi: 10.1097/ALN.0b013e31824b96a1
39. Wang S, Li Y, Liu XD, Zhao CX, Yang KQ. Polymorphism of A118G in μ-Opioid Receptor Gene is Associated With Risk of Esophageal Squamous Cell Carcinoma in a Chinese Population. Int J Clin Oncol (2013) 18:666–9. doi: 10.1007/s10147-012-0441-5
40. Regan PM, Langford D, Khalili K. Regulation and Functional Implications of Opioid Receptor Splicing in Opioid Pharmacology and HIV Pathogenesis. J Cell Physiol (2016) 231(5):976–85. doi: 10.1002/jcp.25237
41. Connolly JG, Tan KS, Mastrogiacomo B, Dycoco J, Caso R, Jones GD, et al. Intraoperative Opioid Exposure, Tumour Genomic Alterations, and Survival Differences in People With Lung Adenocarcinoma. Br J Anaesth (2021) 127:75–84. doi: 10.1016/j.bja.2021.03.030
Keywords: opioid receptors, perioperative opioid, cancer, surgery, neoplasm, tumor, immunohistochemistry
Citation: Belltall A, Zúñiga-Trejos S, Garrido-Cano I, Eroles P, Argente-Navarro MP, Buggy DJ, Díaz-Cambronero O and Mazzinari G (2022) Solid Tumor Opioid Receptor Expression and Oncologic Outcomes: Analysis of the Cancer Genome Atlas and Genotype Tissue Expression Project. Front. Oncol. 12:801411. doi: 10.3389/fonc.2022.801411
Received: 25 October 2021; Accepted: 17 February 2022;
Published: 10 March 2022.
Edited by:
Kalpna Gupta, University of California, Irvine, United StatesReviewed by:
Mellar Pilgrim Davis, Geisinger Health System, United StatesJamie Elmawieh, Gustave Roussy Cancer Campus, France
Copyright © 2022 Belltall, Zúñiga-Trejos, Garrido-Cano, Eroles, Argente-Navarro, Buggy, Díaz-Cambronero and Mazzinari. This is an open-access article distributed under the terms of the Creative Commons Attribution License (CC BY). The use, distribution or reproduction in other forums is permitted, provided the original author(s) and the copyright owner(s) are credited and that the original publication in this journal is cited, in accordance with accepted academic practice. No use, distribution or reproduction is permitted which does not comply with these terms.
*Correspondence: Amparo Belltall, YmVsbHRhbGxhbXBhcm9AZ21haWwuY29t