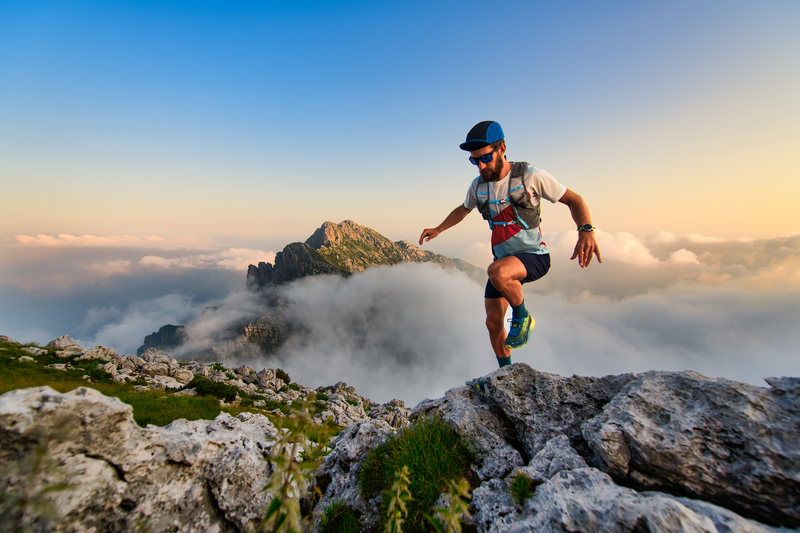
95% of researchers rate our articles as excellent or good
Learn more about the work of our research integrity team to safeguard the quality of each article we publish.
Find out more
ORIGINAL RESEARCH article
Front. Oncol. , 28 June 2022
Sec. Cancer Metabolism
Volume 12 - 2022 | https://doi.org/10.3389/fonc.2022.781686
This article is part of the Research Topic Rising Stars in Cancer Metabolism 2022 View all 10 articles
ATP-binding cassette (ABC) transporters are a large superfamily of membrane proteins that facilitate the translocation of heterogeneous substrates. Studies indicate that ABC transporters may play important roles in various carcinomas. However, the correlation between ABC transporters and immunomodulation in thyroid carcinoma (TC), as well as the prognoses for this disease, is poorly understood.TC data from The Cancer Genome Atlas (TCGA) database were used to identify prognostic hallmark ABC transporters associated with immune cell infiltration patterns via multiple bioinformatic analyses. Thereafter, quantitative real-time polymerase chain reaction (qRT-PCR) was performed to validate the expression of these selected hallmark ABC transporters in both TC and para-cancerous thyroid tissues. Of a total of 49 ABC transporters, five (ABCA8, ABCA12, ABCB6, ABCB8, and ABCC10) were identified as hallmark ABC transporters. All five were differentially expressed in TC and associated with the relapse-free survival rates of patients with TC. Immunoregulation by these five hallmark ABC transporters involved the modulation of various aspects of immune cell infiltration, such as hot or cold tumor subsets and the abundances of infiltrating immune cells, as well as specific immunomodulators and chemokines. Besides the diverse significantly correlated factors, the five hallmark ABC transporters and correlated genes were most highly enriched in plasma membrane, transporter activity, and transmembrane transport of small molecules. In addition, many chemicals, namely bisphenol A and vincristine, affected the expression of these five transporters. The qRT-PCR results of collected TC and para-cancerous thyroid tissues were consistent with those of TCGA. The findings in this study may reveal the role played by these five hallmark ABC transporters in regulating immune cell infiltration patterns in TC as well as the molecular mechanisms underlying their functions, leading to a better understanding of their potential prognostic and immunotherapeutic values.
Thyroid carcinoma (TC) is the most common endocrine malignancy worldwide, and its global incidence rate has been growing over the last three decades. Before the 1990s, the incidence of TC in the United States was relatively stable at approximately 5/100,000. However, its incidence had tripled (15/100,000) by 2014 (1). The incidence of TC in Canada from 2012–2016 was reportedly 17.4/100,000, a figure closely similar to that of the United States (2). Such dramatic increases in the incidence of TC have also been observed in many other countries, including 29 European countries and China (3, 4). According to Global Cancer Statistics, 586,202 new TC cases were reported worldwide in 2020, amounting to an incidence of 13.2/100,000 (5). However, the mortality rate of TC has remained relatively low and stable (<1/100,000); (1–4, 6). The etiology of TC remains unclear. Exposure to ionizing radiation during childhood is considered to be risk factor that is most and closely associated with TC (7). Moreover, other factors, such as chromosomal (genetic) alterations and obesity, are reportedly associated with the occurrence and development of TC (8, 9).
Based on histological features, TC is mainly divided into the following four types: papillary thyroid carcinoma (PTC); follicular thyroid carcinoma (FTC); anaplastic thyroid carcinoma (ATC); and medullary thyroid carcinoma (MTC); (10). PTC represents the most common differentiated subtype of TC, and its incidence has reportedly increased over the past decade (11). Most PTC patients receive favorable prognoses involving 10-year survival rates ranging from 93–97% (12, 13). The 10-year survival rates for FTC and MTC are 85% and 75%, respectively, and thus worse than that for PTC (12). ATC begets the worst prognosis, with a 10-year survival rate of 14% and a median survival of six months (12, 14). Although the majority of TCs, which remain indolent, are associated with an innocuous clinical course, some cases manifest aggressive behavioral patterns, such as metastasis and recurrence, resulting in poorer prognoses. The recurrence rate of PTC following conventional treatment is reportedly as high as 28% (15). Metastasis in TC, which mostly involves the cervical lymph system, acts as an unfavorable factor which leads to poor prognoses (16). The 8th edition of the American Joint Committee on Cancer (AJCC) tumor-node-metastasis (TNM) staging system identifies sex, age, N classification, pathological subtype, and radioactive iodine avidity as some of the major factors affecting the prognosis of PTC (17). In addition, the 10-year cancer-specific survival rate for IVb stage PTC patients over 55 years old presenting with extensive extrathyroidal extension is reportedly 33.3% (18). The limited number of alternative therapeutic strategies that have been used against surgically inoperable and radioiodine-refractory TC have not been successful at improving the survival of TC patients. Therefore, development of new intervention strategies against TC are felt to be warranted.
The tumor microenvironment (TME), which is composed of the extracellular matrix, stromal cells, immune cells, and some secreted factors, plays an important role in TC (19). Of these, tumor immune cell infiltration has been demonstrated to be closely associated with TC progression and prognosis (20, 21). Tumors may be categorized as immunologically hot or cold types, which definitions are based on the degree of immune infiltration in the TME (22). The hot type is characterized by high tumor immunity, indicating association with a stronger immune response and a better survival outcome. By contrast, the TME of a cold tumor is much more immunosuppressive, responding poorly to treatment (23). Various immune cells that infiltrate tumors may either accelerate or decelerate tumor progression, depending on population and activation status (24). Tumor-promoting immune cells, including dendritic cells (DCs), macrophages, and monocytes, may promote tumor growth, metastasis, and drug resistance in the TME, whereas antitumor immune cells, namely B cells, natural killer (NK) cells, and CD8+ T cells, suppress tumor cell proliferation, invasion, adhesion, and metastasis. DCs, which play a key role in antigen presentation and cytokine secretion, are increased in TC (25). In TC, infiltrating DCs activate T cells and NK cells via tumor antigen presentation. Moreover, these infiltrating DCs are known to produce some immunosuppressive cytokines that inhibit immune responses (26). Tumor-associated macrophages (TAMs), which originate from monocytic precursors, infiltrate into the tumor stroma, and facilitate macrophage polarization from the antitumor M1 phenotype to the tumor-promoting M2 phenotype, thereby aggravating TC growth and metastasis (27–29). Monocytes that differentiate in the bone marrow are mainly responsible for inflammation. Increased monocyte infiltration in a mouse TC model promotes tumor progression by elevating immune-related gene and cytokine expression (30). These results show that immunosuppressive cells in the TME of TC can strengthen the ability of tumor cells to fight immune response, thereby enhancing immune escape. Therefore, investigating immune cell infiltration in relation to regulatory mechanisms of TC may be vital for developing new immunotherapeutic strategies that improve TC patient outcomes.
ATP-binding cassette (ABC) transporters are a large superfamily of membrane proteins that acquire energy from ATP hydrolysis to facilitate the translocation of heterogeneous substrates (31). A total of 49 human ABC transporters are grouped into seven distinct subfamilies as follows: ABCA; ABCB; ABCC; ABCD; ABCE; ABCF; and ABCG (32). All ABC transporters are composed of transmembrane domains (TMDs) and nucleotide-binding domains (NBDs); (33). ATP hydrolysis which occurs at the NBDs, induces conformational changes in TMDs, which, in turn, facilitate inward or outward transportation of specific substrates across the membrane (34). ABC transporters, which are ubiquitous, have been found to be associated with diverse biochemical and physiological processes, such as maintenance of cellular environments, protection from harmful materials, and modulation of drug kinetics (35–37). ABC transporters reportedly play vital roles in numerous carcinomas (38). For example, ABCG1, which is overexpressed in clear cell renal cell carcinoma, has been found to be associated with overall patient survival, indicating its potential as a diagnostic and prognostic biomarker in clear cell renal cell carcinoma (39). ABCB1 and ABCG2 reportedly play a critical role in the prevention of chemo-resistant liver cancer stem cell death in hepatocellular carcinoma (40). Differentially expressed ABCC2 and ABCC5 are considered as diagnostic biomarkers of lung adenocarcinoma, while ABCC2, ABCC6, and ABCC8 are reportedly associated with its prognosis (40). Many ABC transporters which are differentially expressed between colorectal cancer (CRC) and non-neoplastic control tissues, may be linked to both the onset and treatment outcomes of CRC (41). The functions of ABC transporters known to be involved in immunity against infection and cancer have been summarized and reviewed, providing a broader understanding of the effects of ABC transporters on immunity to viruses and tumors (42). However, the prognostic roles and immune-related mechanisms of ABC transporters in TC remain unclear.
In this study, we aim to predict the prognostic implications and immune cell infiltration related features of ABC transporters by performing comprehensive analyses followed by validation via RT-PCR. Here, we attempt to provide a deeper insight into the immune cell infiltration patterns seen in TC as well as to identify some potential prognostic and immunotherapeutic targets in TC.
High-throughput RNA sequencing (RNA-seq) data of 502 TC and 58 normal thyroid tissues obtained from The Cancer Genome Atlas (TCGA) database were considered as the public data source for the purposes of this study. The RNA-seq data in a fragments per kilobase per million format were converted into a transcript per million (TPM) reads format and log2 transformed. The clinical features of TC patients obtained from TCGA dataset are summarized (Table 1).
A total of 45 TC patients who received surgical therapy at the Shengjing Hospital of China Medical University were selected for the study. The exclusion and inclusion criteria were similar to those of a previously reported study of ours (43). Both TC and para-cancerous thyroid tissues were collected. A para-cancerous thyroid tissue is defined as a tissue situated at least 2 cm far away from the TC area, as confirmed without TC cells by pathologists. Based on postoperative pathological diagnoses, all included TC tissue specimens were the PTC histological type. Informed consent was obtained from all patients. This study was approved by the Ethics Committee of Shengjing Hospital of China Medical University. The clinical features of all collected patients were summarized (Table 1). All specimens were immediately stored until needed for total RNA extraction, qRT-PCR and hematoxylin-eosin staining.
We compared the expression levels of all ABC transporters known to be active in TC as well as in normal thyroid tissues, that were available in the public TCGA database. R software, version 3.6.3, with the ggplot2 package (version 3.3.3), was used for this comparison. Correlation between the expression levels of individual genes and prognoses was analyzed via the online database Kaplan–Meier plotter (44). To analyze the relapse-free survival (RFS), TC patient samples from TCGA were split into two groups by the relative expression levels of individual genes and assessed by a Kaplan-Meier survival plot. The best performing threshold was considered as the best cutoff value. The hazard ratio (HR) with 95% confidence intervals (CIs) and log rank P-value of individual genes were performed. Differentially expressed, prognostic ABC transporters were selected as hallmark transporters for further analysis.
Immune and stromal scores via ESTIMATE (Estimation of STromal and Immune cells in MAlignant Tumor tissues using Expression data) were used to calculate the levels of infiltrating immune and stromal cells (45). The ESTIMATE score is equal to the sum of immune and stromal scores. The abundance of 24 immune cell type in different kinds of tumors can be estimated using gene expression levels obtained from datasets, such as RNA-seq and microarray data, which are calculated via the Immune Cell Abundance Identifier (ImmuCellAI); (46). Therefore, the abundance of tumor-infiltrating immune cells in TC and normal thyroid tissues was determined and compared using ImmuCellAI. The correlation between the expression levels of hallmark ABC transporters and the abundance of gene markers of immune cells infiltrating TC tissues were adjusted for corresponding tumor purity and assessed using Tumor IMmune Estimation Resource 2.0 (TIMER 2.0); (47–49). In addition, immunomodulators and chemokines were compared against the expression of each hallmark ABC transporter using an integrated repository portal for tumor–immune system interactions (TISIDB), in order to analyze the correlation between them (50).
LinkedOmics is a publicly available platform that includes multi-omics data of 32 TCGA cancer types, and supports multi-omics analysis in a cancer type or pan-cancer analysis. (51). In the LinkFinder modules of LinkedOmics, genes and microRNAs (miRNAs), that were significantly associated with each hallmark ABC transporter, were analyzed statistically using Pearson’s correlation coefficient and presented in both volcano plots and heat maps. In the LinkInterpreter modules of LinkedOmics, transcription factor (TF) targets, that were significantly associated with each hallmark ABC transporter, were enriched through Gene Set Enrichment Analysis. The rank criterion was P-value < 0.05, the minimum number of genes (Size) was 3, and the simulations was 500. The GeneMANIA prediction algorithm is an interface and a large database that can be utilized to analyze gene functions and build an interaction network (52). We predicted the functions of hallmark ABC transporters and 100 resultant closely associated genes. Thereafter, a regulation network was constructed for visualization.
We chose FunRich software (version 3.1.3) to perform enrichment analysis of these five hallmark ABC transporters with the top 100 genes that were closely related to them. Prediction of the functional enrichment of these genes was based on four aspects: cellular component; molecular function; biological process; and biological pathway (53). Gene set analysis of these five hallmark ABC transporters involved in cancer-related pathway activities was performed in GSCALite (54). The following cancer-related pathways were included: TSC/mTOR; RTK; RAS/MAPK; PI3K/AKT; hormone ER; hormone AR; epithelial–mesenchymal transition (EMT); DNA damage response; cell cycle; and apoptosis pathways.
The Comparative Toxicogenomics Database (CTD, version 16548) provides manually curated information regarding chemical–gene, chemical–disease, and gene–disease relationships. This information helps understand the effects exerted by environmental factors on human health (55). The interaction between these five hallmark ABC transporters and chemicals in TC was inferred via curated chemical–gene and chemical–disease associations.
The prognostic value of these five hallmark ABC transporters in TC patients was determined via receiver operating characteristic (ROC) curve analysis. ROC analysis was performed using R software (version 3.6.3) with the pROC (version 1.17.0.1) and ggplot2 (version 3.3.3) packages. The area under the curve (AUC) ranges between 0.5 and 1, with an AUC value closer to 1 indicating a better prognostic effect, particularly a longer RFS (AUCs of 0.5–0.7; 0.7–0.9; and AUC > 0.9 indicate low accuracy; moderate accuracy; and high accuracy, respectively).
Total RNA was extracted from tissues using Trizol (Takara, Dalian, China), and cDNA was synthesized via reverse transcription using a PrimeScript™ RT reagent Kit with gDNA Eraser (Takara, Dalian, China). Thereafter, qRT-PCR analysis was performed on a Roche LightCycler 480 II system using a TB Green®Premix Ex Taq™II kit (Takara, Dalian, China), according to the manufacturers’ protocols. Primer sequences are listed (Table 2). The housekeeping gene glyceraldehyde-3-phosphate dehydrogenase (GAPDH) was used as an internal control. The relative expression of target genes was determined using the 2-ΔΔ CT method, which was similar to that of a previously reported study of ours (43).
Paraffin-fixed sample sections with 3 μm thick were prepared. Briefly, slides were dewaxed and rehydrated, then nuclei were stained with hematoxylin and cytoplasm was stained with eosin. After dehydrating, slides were mounted with neutral balsam. The images were photographed by a microscope.
Student’s t-tests or Wilcoxon rank-sum tests were used to compare between the levels of gene expression in TC and normal tissues. Log-rank test and the Kaplan–Meier method were used to depict survival curves. Pearson’s correlation coefficient was selected to analyze significantly correlated genes. Spearman’s rank correlation coefficient was used to analyze the results of immune-associated analyses. Statistical significance was set at P < 0.05.
To determine the expression and significance of ABC transporters in TC, we compared the transcription levels of all ABC transporters in TC and normal thyroid tissues obtained from the TCGA database. We also analyzed the correlation between the expression levels of all ABC transporters and TC prognoses using the Kaplan–Meier plotter. An integrated comparison indicated that five ABC transporters, namely ABCA8, ABCA12, ABCB6, ABCB8, and ABCC10, were differentially expressed. In addition, these five were also significantly associated with the RFS of TC. As such, these five were considered as hallmark ABC transporters (P < 0.05; Figure 1). Among these hallmark genes, ABCA12 and ABCC10 showed significantly higher expression in TC and were associated with a worse RFS as well, indicating that these played a prominent role in promoting TC progression. Moreover, ABCA8, ABCB6, and ABCB8 showed significantly lower expression levels in TC, in addition to being associated with a worse RFS, demonstrating that these three mainly functioned as inhibitors of TC progression. Therefore, we subsequently analyzed these five hallmark ABC transporters in order to elucidate the molecular mechanisms underlying the role played by them in TC progression.
Figure 1 The expression levels and prognostic values of five hallmark ABC transporters in TC. (A–E) ABCA8, ABCB6, and ABCB8 mRNA expression was downregulated in TC compared with that in normal tissues, while that of ABCA12 and ABCC10 was upregulated. (F–J) Correlation between expression of the five hallmark ABC transporters and prognoses for TC. *P < 0.05, ***P < 0.001.
Since immunomodulation plays a vital role in TC progression, we investigated the correlation between immune cell infiltration and the expression levels of the five selected hallmark ABC transporters in TC. Firstly, we evaluated enrichment differences between immune, stromal, and ESTIMATE scores based on the expression of the five hallmark ABC transporters, respectively. The results revealed that the expression levels of all five hallmark ABC transporters were associated with at least one of the immune, stromal, and ESTIMATE scores of TC obtained from TCGA database (Figures 2A-E). Especially, both ABCB6 and ABCB8 were related to all three types of scores. Thereafter, we investigated the differences between the infiltration of 24 immune cell types in TC and normal thyroid tissues using ImmuCellAI. Among them, a total of 16 types of immune cells were discovered to be differentially infiltrated between TC and normal thyroid tissues, indicating that they may be performing immunoregulatory functions in the progression of TC. These results showed that the abundances of cytotoxic T cells (Tc), type 1 T regulatory cells (Tr1), regulatory T cells (Treg), mucosal-associated invariant T cells, DCs, macrophages, and monocytes in TC tissues were higher than those in normal thyroid tissues. Meanwhile, the abundances of T helper 1 cells (Th1), Th2, follicular helper T cells (Tfh), central memory T cells (Tcm), B cells, NK cells, gamma delta (γδ) T cells (Tgd), CD4+ T cells, and CD8+ T cells in TC tissues were decreased (Figure 2F). Thereafter, we used TIMER 2.0 to explore the correlation between the expression levels of hallmark ABC transporters and the abundances of gene markers of differentially infiltrated immune cells in TC. All five hallmark ABC transporters were associated with Th2 cells, Tcm cells, Treg cells, monocytes, and DCs. Of all the expression levels of these transporters, the expression level that was most significantly negatively correlated with the abundance of Tregs (rho = −0.305, P < 0.001) was that of ABCA8. In addition, the results showed that ABCA8 expression was associated with most gene markers of infiltrated immune cells, except those of Th1 cells and monocytes. In addition to being the expression level that was most positively correlated with DCs (rho = 0.654, P < 0.001), the expression of ABCA12 was correlated with nearly all infiltrated immune cells, except M1 macrophages. ABCB6 expression, which showed the most positive correlation with CD8+ T cells (rho = 0.493, P < 0.001), appeared to be negatively correlated with most gene markers, except with NK. ABCB8 expression, which showed the most negative correlation with DCs (rho = −0.364, P < 0.001), showed no correlation with Th2 cells, Tfh cells, or NK cells. ABCC10 expression showed the highest positive correlation with the abundance of M2 macrophages and most gene markers, except with that of NK cells and DCs (rho = 0.284, P < 0.001); (Table 3; Figure 2G).
Figure 2 Immune-associated analysis of the hallmark ABC transporters in TC. (A–E) Correlation between the expression levels of hallmark ABC transporters and immune, stromal, and ESTIMATE scores. (F) Differential abundances of tumor-infiltrating immune cells between TC and normal thyroid tissues. (G) Correlation between the expression levels of hallmark ABC transporters and the abundances of tumor-infiltrating immune cells, which were most associated with each hallmark ABC transporters. *P < 0.05, **P <0.01, ***P < 0.001. ns, not significant..
Moreover, we analyzed the relationship between immunomodulators and the expression levels of hallmark ABC transporters using TISIDB (Figures 3A-C). Of all five expression levels, that of ABCA8 showed the most negative correlation with VTCN1 (rho = −0.369, P < 0.001) as well as the most positive correlation with KDR (rho = 0.467, P < 0.001). ABCA12 expression had the most positive correlation with VTCN1 (rho = 0.755, P < 0.001) and the most negative correlation with KDR (rho = −0.540, P < 0.001). ABCB6 expression showed a weak to moderate negative correlation with most immunomodulators, particularly CD274 (rho = −0.550, P < 0.001), TGFBR1 (rho = −0.546, P < 0.001), and TNFSF18 (rho = −0.545, P < 0.001). Similarly, the expression levels of ABCB6, and ABCB8 showed a weak to moderate negative correlation with most immunomodulators, particularly TGFBR1 (rho = −0.559, P < 0.001) and TNFSF15 (rho = −0.518, P < 0.001). Moreover, the highest positive correlation with TNFRSF25 (rho = 0.499, P < 0.001) was shown by ABCC10 expression. Finally, we analyzed the association between the five hallmark ABC transporters and 41 types of chemokine ligands and 18 types of receptors (Figures 3D, E). The results showed that ABCA8 expression had the most positive correlation with CCL14 (rho = 0.359, P < 0.001) and the most negative correlation with CXCL17 (rho = −0.406, P < 0.001). ABCA12 expression showed a moderate to strong positive correlation with most ligands and receptors, particularly CCL20 (rho = 0.684, P < 0.001), CXCL5 (rho = 0.670, P < 0.001), CXCL16 (rho = 0.638, P < 0.001), and CCR9 (rho = 0.431, P < 0.001). ABCB6 expression showed a weak to moderate negative correlation with most ligands and receptors, including CXCL17 (rho = −0.546, P < 0.001), CXCL5 (rho = −0.496, P < 0.001), CCL20 (rho = −0.496, P < 0.001), and CCR8 (rho = −0.446, P < 0.001). The expression level which was most positively correlated with CCL14 (rho = 0.273, P < 0.001) and most negatively correlated with CXCL5 (rho = −0.387, P < 0.001) was that of ABCB8. ABCC10 showed the most positive correlation with CXCL14 (rho = 0.345, P < 0.001). Considered together, these results revealed that the expression of these five hallmark ABC transporters was closely correlated with immune cell infiltration patterns and immunoregulation in TC.
Figure 3 Immunomodulators and chemokines-associated analysis of the hallmark ABC transporters in TC. (A–C) Correlation between the expression levels of hallmark ABC transporters and immunomodulators in TC. (D, E) Correlation between the expression levels of hallmark ABC transporters and chemokine ligands and receptors in TC.
To explore the molecular mechanisms underlying the regulation of immune cell infiltration in TC by the five hallmark ABC transporters, we identified significantly correlated genes, using the LinkFinder module of LinkedOmics and visualized them in the form of heatmaps and volcano plots. The results showed that the genes which were most significantly positively correlated with the regulation of immune cell infiltration by ABCA8, ABCA12, ABCB6, ABCB8, and ABCC10 were platelet endothelial aggregation receptor 1 (PEAR1; rho = 0.604, P < 0.001), V-set domain-containing T cell activation inhibitor 1 (VTCN1; rho = 0.754, P < 0.001), microtubule associated protein 1 light chain 3 alpha (MAP1LC3A; rho = 0.694, P < 0.001), chromosome 2 open reading frame 7 (C2orf7; rho = 0.782, P < 0.001), and zinc finger protein 513 (ZNF513; rho = 0.682, P < 0.001), respectively (Figure 4). In addition, the genes that were most significantly negatively correlated with the regulation of immune cell infiltration by ABCA8, ABCA12, ABCB6, ABCB8, and ABCC10 were abhydrolase domain-containing protein 12 (ABHD12; rho = −0.523, P < 0.001), BTB domain containing 11 (BTBD11; rho = −0.687, P < 0.001), calpastatin (CAST; rho = −0.687, P < 0.001), lysine demethylase 5B (KDM5B; rho = −0.727, P < 0.001), and mitochondrial ribosomal protein S35 (MRPS35; rho = −0.589, P < 0.001), respectively (Figure 4).
Figure 4 Correlation of differentially expressed genes with five hallmark ABC transporters in TC. (A, D, G, J, M) Differentially expressed genes, which were correlated with five hallmark ABC transporters, were analyzed by Pearson test, and visualized in form of volcano plots. (B, E, H, K, N) The top 50 genes, which were positively correlated with five hallmark ABC transporters, were visualized in form of heatmaps. (C, F, I, L, O) The top 50 genes, which were negatively correlated with five hallmark ABC transporters, were also visualized in form of heatmaps.
It is widely accepted that miRNAs and TFs are key regulators of gene expression. Therefore, we also identified the miRNAs and TF targets that were significantly correlated with the five hallmark ABC transporters, using the LinkFinder and LinkInterpreter modules of LinkedOmics. The results of correlated miRNAs were also present in the form of heatmaps and volcano plots. The miRNAs most significantly positively correlated with ABCA8, ABCA12, ABCB6, ABCB8, and ABCC10 were hsa-mir-145 (rho = 0.454, P < 0.001), hsa-mir-934 (rho = 0.677, P < 0.001), hsa-mir-204 (rho = 0.517, P < 0.001), hsa-mir-22 (rho = 0.538, P < 0.001), and hsa-mir-187 (rho = 0.391, P < 0.001), respectively (Figure 5). Moreover, the miRNAs that were most significantly negatively correlated with ABCA8, ABCA12, ABCB6, ABCB8, and ABCC10 were hsa-mir-203 (rho = −0.432, P < 0.001), hsa-mir-1179 (rho = −0.573, P < 0.001), hsa-mir-21 (rho = −0.445, P < 0.001), hsa-mir-146b (rho = −0.493, P < 0.001), and hsa-mir-874 (rho = −0.355, P < 0.001), respectively (Figure 5). In addition, the TF targets that were most correlated with ABCA8, ABCA12, ABCB6, ABCB8, and ABCC10 were V$LXR_DR4_Q3, V$NFKB_C, V$ELK1_02, V$ELK1_02, and V$PAX8_01, respectively (Table 4).
Figure 5 Correlation of differentially expressed miRNAs with five hallmark ABC transporters in TC. (A, D, G, J, M) Differentially expressed miRNAs, which were correlated with five hallmark ABC transporters, were analyzed by Pearson test, and visualized in form of volcano plots. (B, E, H, K, N) The top 50 miRNAs, which were positively correlated with five hallmark ABC transporters, were visualized in form of heatmaps. (C, F, I, L, O) The top 50 miRNAs, which were negatively correlated with five hallmark ABC transporters, were also visualized in form of heatmaps.
Table 4 Correlations of the expression of the five hallmark ABC transporters with the abundance of gene markers of immune cell infiltration in TC.
To identify the molecular mechanisms underlying the regulation of immune cell infiltration by hallmark ABC transporters more precisely, we constructed a gene regulation network and conducted functional enrichment analysis of the five hallmark ABC transporters and a 100 of the genes that were most correlated with them, using GeneMANIA and Funrich. The network revealed that these genes were closely associated with transmembrane transporter activity and regulation of lipid transport (Figure 6). The enrichment analyses indicated that the most highly enriched cellular components were the plasma membrane (53.7%) and lysosomes (26.3%); (Figure 7A). Transporter activity (37.6%) and auxiliary transport protein activity (10.9%) were the most highly enriched molecular functions (Figure 7B). The biological processes of genes were most highly enriched in transport (49.5%); (Figure 7C). Some biological pathways, including transmembrane transport of small molecules (46.3%) and ABC family protein-mediated transport (40.7%), were also most highly enriched (Figure 7D). In addition, cancer process and cancer-related pathway enrichment analyses of these hallmark ABC transporters were performed and visualized using GSCALite. The results showed that all five hallmark ABC transporters were involved in regulating these cancer-related pathways (Figure 7E).
Figure 6 The constructed gene regulation network of five hallmark ABC transporters and 100 genes, which were most highly correlated with five hallmark ABC transporters.
Figure 7 Functional and pathway enrichment analysis of the five hallmark ABC transporters in TC. (A) The most enriched cellular components of correlated genes of the five hallmark ABC transporters. (B) The most enriched molecular functions of correlated genes of the five hallmark ABC transporters. (C) The most enriched biological processes of correlated genes of the five hallmark ABC transporters. (D) The most enriched biological pathways of correlated genes of the five hallmark ABC transporters. (E) The cancer related pathway enrichment of the five hallmark ABC transporters.
Since hallmark ABC transporters were closely associated with the transmembrane transport of small molecules, we screened out TC-associated chemicals and inferred their association with these five hallmark ABC transporters, using the CTD database. The results showed that multiple types of chemicals, including bisphenol A and vincristine, affected the expression or mutagenesis of these hallmark ABC transporters (Table 5). These findings provided important information regarding the nature of interaction between chemicals and hallmark ABC transporters and their effects on the progression of TC.
To evaluate the prognostic value of these five hallmark ABC transporters, ROC curves were generated using the expression data of TC and normal thyroid tissues obtained from TCGA (Figure 8A). The AUCs and 95% CI values of these five hallmark ABC transporters were calculated (Table 6). Among the five hallmark ABC transporters, ABCA8 showed the highest relative accuracy while that of ABCC10 appeared to be the lowest. These results indicated the considerable potential shown by these five hallmark ABC transporters for predicting the RFS of TC.
Figure 8 Prognostic prediction value and validation of the five hallmark ABC transporters in TC. (A) The ROC curves of the five hallmark ABC transporters in TC. (B) Relative mRNA expression of the five hallmark ABC transporters in TC and normal thyroid tissues. FPR, false positive rate; TPR, true positive rate. *P < 0.05.
Representative hematoxylin and eosin staining images of both collected TC and para-cancerous thyroid tissues were performed (Supplemental Figure 1). To validate the bioinformatic analyses of these five hallmark ABC transporters in TC, we performed qRT-PCR tests to examine their transcriptional expression in both TC and para-cancerous thyroid tissues. The results indicated that the expression levels of ABCA12 and ABCC10 in TC were higher compared to those in para-cancerous thyroid tissue, while the expression levels of ABCA8, ABCB6, and ABCB8 in TC were significantly lower (P <0.05; Figure 8B). These results were consistent with those obtained from the public via TCGA database.
It has become increasingly evident that ABC transporters play important roles in the immunomodulation of tumors. For example, multidrug resistance protein 1 (MDR1), encoded by ABCB1, is expressed in cytotoxic T lymphocytes and NK cells, and mediates immune responses (56). A high level of the MDR1+ immune cell infiltrate, mostly comprising M2 macrophages, was confirmed as an independent prognostic factor associated with poor prognoses for epithelial ovarian cancer (57). ABCA1 regulates the immune sensitivity of osteosarcoma cells. Moreover, the ABCB1: ABCA1 ratio was reportedly upregulated in osteosarcoma cells and positively correlated with a higher probability of relapse (58). ABCA8 expression was downregulated in stomach adenocarcinoma and was positively associated with six types of infiltrated immune cells, particularly M2 macrophages (59). Major histocompatibility complex class I (MHC-I) molecules play a vital role in immune surveillance as well as in the presentation of antigen peptides on the cell surface (60). The heterodimer of transporter associated with antigen processing (TAP) transports antigenic peptides and provides peptides to MHC-I molecules (61, 62). TAP blockade in DCs reportedly impaired classic MHC-I presentation for CD8+ T cell priming (63). Moreover, low TAP1/TAP2 expression led to the overexpression and efficient presentation of the antigen preprocalcitonin in lung carcinoma, as recognized via tumor-specific cytotoxic T lymphocytes (64). ABCC8 is considered a prognostic risk factor which is closely related to the LUAD microenvironment (65). Single-nucleotide polymorphisms (SNPs) in TAP1 and TAP2 affected their expression and were associated with cervical cancer in the Chinese Han population (66). ABCD3 expression, which is considered as an independent prognostic factor of CRC, was decreased in CRC tissues and associated with the overall survival of CRC patients (67).
Previous studies have demonstrated that altered expression levels of some ABC transporters were associated with their molecular roles in TC. ABCA9 expression was upregulated by hsa_circ_0039411 sponging miR-1179, leading to enhanced oncogenic properties in PTC (68). ABCB5 expression was reported to be associated with larger tumor size in PTC (69). Moreover, in the solid variant of PTC, high ABCC1 expression was associated with larger tumor size, while high ABCG2 expression was correlated with lymphovascular invasion (70). ABCG2 expression was also found to be closely related to the induction of EMT in PTC (71). High ABCC2 expression was observed in advanced stage MTC (72). In ATC, high expression levels of ABCB1, ABCC1 and ABCG2 observed in several cell lines and tissues, were associated with cancer drug resistance (73). However, the prognostic and immunotherapeutic value of ABC transporters in TC has not been well characterized. Therefore, the current study aimed to explore the prognostic and immune related value of ABC transporters in TC, leading to the provision of new directions and targets for its treatment.
In this study, data of TC and normal thyroid tissues from TCGA were analyzed to screen out hallmark ABC transporters, which are differentially expressed and associated with the prognosis for TC. Based on the results, ABCA8, ABCA12, ABCB6, ABCB8, and ABCC10 were selected as hallmark ABC transporters in TC. Of these, the expression of ABCA8, ABCB6, and ABCB8 was down-regulated in TC, compared with those in normal thyroid tissues, while that of ABCA12 and ABCC10 was up-regulated. Usually, up-regulation of oncogenes and down-regulation of anti-oncogenes affect many behavior patterns of malignant tumor cells, including metastasis and immune resistance. Our results revealed that ABCA8, ABCB6, and ABCB8 may inhibit the malignant progression of TC. Conversely, ABCA12 and ABCC10 may promote the occurrence and growth of TC. Since these are differentially expressed in TC and closely associated with the prognoses for TC, we selected these five as hallmark ABC transporters in TC for subsequent analyses.
It is increasingly becoming evident that immunotherapies play a vital role in tumor treatment, wherein the efficacy of immunomodulation depends mainly on immune cell infiltration. Based on our results, the five hallmark ABC transporters exert a variety of effects on immune cell infiltration in TC. Firstly, the expression of five hallmark ABC transporters was associated with two different TC subsets (hot or cold), indicating that the five hallmark ABC transporters may be useful for converting immunosuppressive (cold) TCs into immunosensitive (hot) ones. Moreover, our results showed that all five hallmark ABC transporters were associated with Th2 cells, Tcm cells, Treg cells, monocytes, and DCs, which revealed that their roles in immune cell infiltration-related immunoregulation were similar. In addition, the specificity of the transporters in regulating Th1 cells, Tfh cells, CD8+ T cells, NK cells, M1 macrophages, and B cells demonstrated the diversity of their roles. Our results also indicated that multiple immunomodulators, such as KDR, MHC molecules, VTCN1, CD274, TGFBR1, TNFSF18, and TNFSF15, were closely associated with these five hallmark ABC transporters. KDR, also known as vascular endothelial growth factor receptor 2 (VEGFR2), is the main receptor of VEGF signaling (74). Activation of KDR, which promotes endothelial cell mitogenesis and vascular permeability, plays a vital role in the induction of tumor angiogenesis (75). The VEGF-KDR signaling pathway was found to play an immunosuppressive role in TME and immune effector cell activity (76). MHC molecule expression is known to mediate immune escape mechanisms in tumors (77). VTCN1, also termed B7-H4, reportedly inhibits T cell proliferation and cytokine secretion, thereby negatively regulating T cell immune response, and positively regulating immune escape (78). CD274, also commonly referred to as programmed cell death 1 ligand 1 (PD-L1), is a ligand of PD-1, which is expressed on many immune cells. The PD-1/PD-L1 axis, which is exploited by tumor cells, may inhibit immune response and block immune cell activation (78). Cancer cells generate multiple factors, including TGF-β1, to create an immune inhibitory environment and evade T cell surveillance (79, 80). As the irreplaceable receptor of TGF-β1, TGFBR1, which is observed in different tumor types, participates in tumor immunological reactions (81). Tumor necrosis factor (TNF) superfamily ligands, such as TNFSF15 and TNFSF18, exert diverse modulatory effects by influencing immune responses and impacting immune cells (82–84). Moreover, chemokine–chemokine receptor interactions regulate immune cell recruitment into tumors and the stimulation of immune response (85). Our results demonstrated that the immune regulation by hallmark ABC transporters was partly mediated via chemokine ligands and receptors.
The presence of complex regulatory networks that affect almost all molecular processes at both intracellular as well as TME levels, is well known. The results of the analysis conducted on correlated genes, miRNAs, and TF targets showed that each hallmark ABC transporters possessed a unique feature and a distinctive regulatory pattern. The constructed regulation network and enrichment of these five transporters showed that the genes, which were most correlated with the five hallmark ABC transporters, were closely associated with transmembrane transporter activity and ABC family protein-mediated transport. In addition, multiple cancer processes and cancer-related pathways, including RTK, RAS/MAPK, PI3K/AKT, and EMT, were partly activated or suppressed by these five hallmark ABC transporters. These findings indicated that these five hallmark ABC transporters may modulate immune cell infiltration patterns by altering the expression levels of correlated genes, regulating transmembrane transporter activity, and influencing cancer-related pathways. However, the exact regulatory mechanisms have not yet been fully elucidated and further discovery and validation are felt to be required.
The known risk factors for TC include female sex, obesity, smoking status, radioactive iodine exposure history, and family genetic history (86–88). However, these recognized causative factors do not fully explain the increasing incidence of TC. Recent studies have demonstrated the influence of anthropogenic environmental chemical factors on TC (89). For example, individuals with higher cadmium exposure were observed to be more susceptible to TC (90). Environmental radiation exposure, such as that due to the Chernobyl accident, led to radiation dose-associated DNA double-strand breaks, subsequently resulting in PTC growth (91). Artificial light at night has also been found to be positively associated with TC incidence (92). Exposure to multiple essential microelements, including manganese and strontium, were positively associated with capsular invasion, multifocality, and tumor stage of PTC (93). Bisphenol A altered endocrine function and partly facilitated EMT in PTC (94). In this study, we focused on elucidating the associations between TC-associated chemicals and the five hallmark ABC transporters. We indicated that these chemicals exerted significant effects on the expression of these five hallmark ABC transporters in TC. The methylation and mRNA expression levels of these five hallmark ABC transporters were mostly affected by bisphenol A and vincristine. The findings of the present study are consistent with those of previous studies. Therefore, more attention should be paid to regulating the impact of chemical factors on TC.
Moreover, to verify the results of bioinformatic analyses, we performed qRT-PCR to detect the mRNA expression of these five hallmark ABC transporters in collected TC and para-cancerous thyroid tissues. The qRT-PCR results were entirely consistent with our TCGA analysis. In the future, we expect to directly confirm probable mechanisms underlying the regulation of immune cell infiltration patterns in TC by these five hallmark ABC transporters, via in vitro and in vivo experiments.
Our study was affected by several limitations. Firstly, in our effort to elucidate the specific roles of hallmark ABC transporters in modulating TC prognosis and progression, we analyzed the data of all TCs obtained from the TCGA database, without differentiating between its pathological subtypes. Secondly, compared with PTC, the incidence rates of MTC and ATC are very low. As such, it is relatively difficult to collect ATC and MTC case data for qRT-PCR analysis. Therefore, only PTC and para-cancerous thyroid tissues could be collected and used for experimental validation.
The findings of this study indicate that five hallmark ABC transporters (ABCA8, ABCA12, ABCB6, ABCB8, and ABCC10) are strongly associated with immunomodulation in TC, as well as with the prognoses for TC. In addition, factors, as well as chemicals and regulatory networks, that are significantly correlated with these five hallmark ABC transporters in TC, are elucidated. These findings may help better understand the molecular mechanisms underlying the role played by these hallmark ABC transporters in TC. The findings of this study also indicate that these hallmark ABC transporters may help enhance prognostic prediction and enable the development of effective immunotherapies against TC.
The original contributions presented in the study are included in the article/Supplementary Material. Further inquiries can be directed to the corresponding author.
The studies involving human participants were reviewed and approved by the Ethics Committee of Shengjing Hospital of China Medical University. The patients/participants provided their written informed consent to participate in this study.
Conceptualization, LW and ZL; Methodology, LW, XS and JH; Investigation, LW and XS; Resources, LW, XS and JH; Writing – Original Draft Preparation, LW; Writing – Review & Editing, XS, JH and ZL; Supervision, ZL. All authors read and approved the final manuscript.
This work was supported by the National Natural Science Foundation of China (Grant number: 81672644), the Young Scholar Support Program 2018 of China Medical University (Grant number: QGZD2018061), and the 345 Talent Project of Shengjing Hospital of China Medical University (50A; 30).
The authors declare that the research was conducted in the absence of any commercial or financial relationships that could be construed as a potential conflict of interest.
All claims expressed in this article are solely those of the authors and do not necessarily represent those of their affiliated organizations, or those of the publisher, the editors and the reviewers. Any product that may be evaluated in this article, or claim that may be made by its manufacturer, is not guaranteed or endorsed by the publisher.
We would like to thank Dr. Yang Fan (Shengjing Hospital of China Medical University) for her critical reading of the manuscript.
The Supplementary Material for this article can be found online at: https://www.frontiersin.org/articles/10.3389/fonc.2022.781686/full#supplementary-material
Supplementary Figure 1 | Representative hematoxylin and eosin staining images of both collected TC and para-cancerous thyroid tissues. (A) Representative hematoxylin and eosin staining image of para-cancerous thyroid tissues. (B) Representative hematoxylin and eosin staining image of PTC tissues. Magnification 200×.
1. Roman BR, Morris LG, Davies L. The Thyroid Cancer Epidemic 2017 Perspective. Curr Opin Endocrinol Diabetes Obes (2017) 24(5):332–6. doi: 10.1097/MED.0000000000000359
2. Ellison LF, Bushnik T. Changing Trends in Thyroid Cancer Incidence in Canada: A Histologic Examination 1992 to 2016. Health Rep (2020) 31(1):15–25. doi: 10.25318/82-003-x202000100002-eng
3. Dal Maso L, Tavilla A, Pacini F, Serraino D, van Dijk BAC, Chirlaque MD, et al. Survival of 86,690 Patients With Thyroid Cancer: A Population-Based Study in 29 European Countries From EUROCARE-5. Eur J Cancer (2017) 77:140–52. doi: 10.1016/j.ejca.2017.02.023
4. Dal Maso L, Panato C, Franceschi S, Serraino D, Buzzoni C, Busco S, et al. The Impact of Overdiagnosis on Thyroid Cancer Epidemic in Italy,1998-2012. Eur J Cancer (2018) 94:6–15. doi: 10.1016/j.ejca.2018.01.083
5. Sung H, Ferlay J, Siegel RL, Laversanne M, Soerjomataram I, Jemal A, et al. Global Cancer Statistics 2020: GLOBOCAN Estimates of Incidence and Mortality Worldwide for 36 Cancers in 185 Countries. CA Cancer J Clin (2021) 71(3):209–49. doi: 10.3322/caac.21660
6. Vaccarella S, Franceschi S, Bray F, Wild CP, Plummer M, Dal Maso L. Worldwide Thyroid-Cancer Epidemic? The Increasing Impact of Overdiagnosis. N Engl J Med (2016) 375(7):614–7. doi: 10.1056/NEJMp1604412
7. Bogovic Crncic T, Ilic Tomas M, Girotto N, Grbac Ivankovic S. Risk Factors for Thyroid Cancer: What Do We Know So Far? Acta Clin Croat (2020) 59(Suppl 1):66–72. doi: 10.20471/acc.2020.59.s1.08
8. Asa SL, Mete O. Oncocytic Change in Thyroid Pathology. Front Endocrinol (Lausanne) (2021) 12:678119. doi: 10.3389/fendo.2021.678119
9. Franchini F, Palatucci G, Colao A, Ungaro P, Macchia PE, Nettore IC. Obesity and Thyroid Cancer Risk: An Update. Int J Environ Res Public Health (2022) 19(3):1116. doi: 10.3390/ijerph19031116
10. Fagin JA, Wells SA Jr. Biologic and Clinical Perspectives on Thyroid Cancer. N Engl J Med (2016) 375(11):1054–67. doi: 10.1056/NEJMra1501993
11. Carling T, Udelsman R. Thyroid Cancer. Annu Rev Med (2014) 65:125–37. doi: 10.1146/annurev-med-061512-105739
12. Hundahl SA, Fleming ID, Fremgen AM, Menck HR. A National Cancer Data Base Report on 53,856 Cases of Thyroid Carcinoma Treated in the U.S. 1985-1995 [See Commetns]. Cancer (1998) 83(12):2638–48. doi: 10.1002/(sici)1097-0142(19981215)83:12<2638::aid-cncr31>3.0.co;2-1
13. Ito Y, Miyauchi A, Kihara M, Fukushima M, Higashiyama T, Miya A. Overall Survival of Papillary Thyroid Carcinoma Patients: A Single-Institution Long-Term Follow-Up of 5897 Patients. World J Surg (2018) 42(3):615–22. doi: 10.1007/s00268-018-4479-z
14. Nachalon Y, Stern-Shavit S, Bachar G, Shvero J, Limon D, Popovtzer A. Aggressive Palliation and Survival in Anaplastic Thyroid Carcinoma. JAMA Otolaryngol Head Neck Surg (2015) 141(12):1128–32. doi: 10.1001/jamaoto.2015.2332
15. Grogan RH, Kaplan SP, Cao H, Weiss RE, Degroot LJ, Simon CA, et al. A Study of Recurrence and Death From Papillary Thyroid Cancer With 27 Years of Median Follow-Up. Surgery (2013) 154(6):1436–46. doi: 10.1016/j.surg.2013.07.008
16. Maksimovic S, Jakovljevic B, Gojkovic Z. Lymph Node Metastases Papillary Thyroid Carcinoma and Their Importance in Recurrence of Disease. Med Arch (2018) 72(2):108–11. doi: 10.5455/medarh.2018.72.108-111
17. Perrier ND, Brierley JD, Tuttle RM. Differentiated and Anaplastic Thyroid Carcinoma: Major Changes in the American Joint Committee on Cancer Eighth Edition Cancer Staging Manual. CA Cancer J Clin (2018) 68(1):55–63. doi: 10.3322/caac.21439
18. Lee YK, Kim D, Shin DY, Lee CR, Lee EJ, Kang SW, et al. The Prognosis of Papillary Thyroid Cancer With Initial Distant Metastasis is Strongly Associated With Extensive Extrathyroidal Extension: A Retrospective Cohort Study. Ann Surg Oncol (2019) 26(7):2200–9. doi: 10.1245/s10434-019-07314-x
19. Ferrari SM, Fallahi P, Galdiero MR, Ruffilli I, Elia G, Ragusa F, et al. Immune and Inflammatory Cells in Thyroid Cancer Microenvironment. Int J Mol Sci (2019) 20(18):4413. doi: 10.3390/ijms20184413
20. Ahn J, Jin M, Song E, Ryu YM, Song DE, Kim SY, et al. Immune Profiling of Advanced Thyroid Cancers Using Fluorescent Multiplex Immunohistochemistry. Thyroid (2021) 31(1):61–7. doi: 10.1089/thy.2020.0312
21. Cameselle-Garcia S, Abdulkader-Sande S, Sanchez-Ares M, Rodriguez-Carnero G, Garcia-Gomez J, Gude-Sampedro F, et al. PD-L1 Expression and Immune Cells in Anaplastic Carcinoma and Poorly Differentiated Carcinoma of the Human Thyroid Gland: A Retrospective Study. Oncol Lett (2021) 22(1):553. doi: 10.3892/ol.2021.12814
22. Too NSH, Ho NCW, Adine C, Iyer NG, Fong ELS. Hot or Cold: Bioengineering Immune Contextures Into In Vitro Patient-Derived Tumor Models. Adv Drug Deliv Rev (2021) 175:113791. doi: 10.1016/j.addr.2021.05.001
23. Galon J, Bruni D. Approaches to Treat Immune Hot, Altered and Cold Tumours With Combination Immunotherapies. Nat Rev Drug Discov (2019) 18(3):197–218. doi: 10.1038/s41573-018-0007-y
24. Wu T, Dai Y. Tumor Microenvironment and Therapeutic Response. Cancer Lett (2017) 387:61–8. doi: 10.1016/j.canlet.2016.01.043
25. Hilly O, Koren R, Raz R, Rath-Wolfson L, Mizrachi A, Hamzany Y, et al. The Role of S100-Positive Dendritic Cells in the Prognosis of Papillary Thyroid Carcinoma. Am J Clin Pathol (2013) 139(1):87–92. doi: 10.1309/AJCPAKYDO56NKMYZ
26. Scouten WT, Francis GL. Thyroid Cancer and the Immune System: A Model for Effective Immune Surveillance. Expert Rev Endocrinol Metab (2006) 1(3):353–66. doi: 10.1586/17446651.1.3.353
27. Ryder M, Ghossein RA, Ricarte-Filho JC, Knauf JA, Fagin JA. Increased Density of Tumor-Associated Macrophages is Associated With Decreased Survival in Advanced Thyroid Cancer. Endocr Relat Cancer (2008) 15(4):1069–74. doi: 10.1677/ERC-08-0036
28. Kim S, Cho SW, Min HS, Kim KM, Yeom GJ, Kim EY, et al. The Expression of Tumor-Associated Macrophages in Papillary Thyroid Carcinoma. Endocrinol Metab (Seoul) (2013) 28(3):192–8. doi: 10.3803/EnM.2013.28.3.192
29. Fang W, Ye L, Shen L, Cai J, Huang F, Wei Q, et al. Tumor-Associated Macrophages Promote the Metastatic Potential of Thyroid Papillary Cancer by Releasing CXCL8. Carcinogenesis (2014) 35(8):1780–7. doi: 10.1093/carcin/bgu060
30. Park S, Zhu J, Altan-Bonnet G, Cheng SY. Monocyte Recruitment and Activated Inflammation are Associated With Thyroid Carcinogenesis in a Mouse Model. Am J Cancer Res (2019) 9(7):1439–53.
31. Locher KP. Mechanistic Diversity in ATP-Binding Cassette (ABC) Transporters. Nat Struct Mol Biol (2016) 23(6):487–93. doi: 10.1038/nsmb.3216
32. Dean M, Hamon Y, Chimini G. The Human ATP-Binding Cassette (ABC) Transporter Superfamily. J Lipid Res (2001) 42(7):1007–17.
33. Thomas C, Tampe R. Structural and Mechanistic Principles of ABC Transporters. Annu Rev Biochem (2020) 89:605–36. doi: 10.1146/annurev-biochem-011520-105201
34. Theodoulou FL, Kerr ID. ABC Transporter Research: Going Strong 40 Years on. Biochem Soc Trans (2015) 43(5):1033–40. doi: 10.1042/BST20150139
35. Sarkadi B, Homolya L, Szakacs G, Varadi A. Human Multidrug Resistance ABCB and ABCG Transporters: Participation in a Chemoimmunity Defense System. Physiol Rev (2006) 86(4):1179–236. doi: 10.1152/physrev.00037.2005
36. Chai AB, Ammit AJ, Gelissen IC. Examining the Role of ABC Lipid Transporters in Pulmonary Lipid Homeostasis and Inflammation. Respir Res (2017) 18(1):41. doi: 10.1186/s12931-017-0526-9
37. Amawi H, Sim HM, Tiwari AK, Ambudkar SV, Shukla S. ABC Transporter-Mediated Multidrug-Resistant Cancer. Adv Exp Med Biol (2019) 1141:549–80. doi: 10.1007/978-981-13-7647-4_12
38. Theile D, Wizgall P. Acquired ABC-Transporter Overexpression in Cancer Cells: Transcriptional Induction or Darwinian Selection? Naunyn Schmiedebergs Arch Pharmacol (2021) 394(8):1621–32. doi: 10.1007/s00210-021-02112-3
39. Meng F, Xiao Y, Xie L, Liu Q, Qian K. Diagnostic and Prognostic Value of ABC Transporter Family Member ABCG1 Gene in Clear Cell Renal Cell Carcinoma. Channels (Austin) (2021) 15(1):375–85. doi: 10.1080/19336950.2021.1909301
40. Yin W, Xiang D, Wang T, Zhang Y, Pham CV, Zhou S, et al. The Inhibition of ABCB1/MDR1 or ABCG2/BCRP Enables Doxorubicin to Eliminate Liver Cancer Stem Cells. Sci Rep (2021) 11(1):10791. doi: 10.1038/s41598-021-89931-9
41. Hlavata I, Mohelnikova-Duchonova B, Vaclavikova R, Liska V, Pitule P, Novak P, et al. The Role of ABC Transporters in Progression and Clinical Outcome of Colorectal Cancer. Mutagenesis (2012) 27(2):187–96. doi: 10.1093/mutage/ger075
42. Thurm C, Schraven B, Kahlfuss S. ABC Transporters in T Cell-Mediated Physiological and Pathological Immune Responses. Int J Mol Sci (2021) 22(17):9186. doi: 10.3390/ijms22179186
43. Wang L, Huang Y, Liu C, Guo M, Ma Z, He J, et al. Deltex3 Inhibits Epithelial Mesenchymal Transition in Papillary Thyroid Carcinoma via Promoting Ubiquitination of XRCC5 to Regulate the AKT Signal Pathway. J Cancer (2021) 12(3):860–73. doi: 10.7150/jca.48141
44. Nagy A, Munkacsy G, Gyorffy B. Pancancer Survival Analysis of Cancer Hallmark Genes. Sci Rep (2021) 11(1):6047. doi: 10.1038/s41598-021-84787-5
45. Yoshihara K, Shahmoradgoli M, Martinez E, Vegesna R, Kim H, Torres-Garcia W, et al. Inferring Tumour Purity and Stromal and Immune Cell Admixture From Expression Data. Nat Commun (2013) 4:2612. doi: 10.1038/ncomms3612
46. Miao YR, Zhang Q, Lei Q, Luo M, Xie GY, Wang H, et al. ImmuCellAI: A Unique Method for Comprehensive T-Cell Subsets Abundance Prediction and its Application in Cancer Immunotherapy. Adv Sci (Weinh) (2020) 7(7):1902880. doi: 10.1002/advs.201902880
47. Danaher P, Warren S, Dennis L, D'Amico L, White A, Disis ML, et al. Gene Expression Markers of Tumor Infiltrating Leukocytes. J Immunother Cancer (2017) 5:18. doi: 10.1186/s40425-017-0215-8
48. Li T, Fu J, Zeng Z, Cohen D, Li J, Chen Q, et al. TIMER2.0 for Analysis of Tumor-Infiltrating Immune Cells. Nucleic Acids Res (2020) 48(W1):W509–14. doi: 10.1093/nar/gkaa407
49. Lin T, Zhang E, Mai PP, Zhang YZ, Chen X, Peng LS. CXCL2/10/12/14 are Prognostic Biomarkers and Correlated With Immune Infiltration in Hepatocellular Carcinoma. Biosci Rep (2021) 41(6):BSR20204312. doi: 10.1042/BSR20204312
50. Ru B, Wong CN, Tong Y, Zhong JY, Zhong SSW, Wu WC, et al. TISIDB: An Integrated Repository Portal for Tumor-Immune System Interactions. Bioinformatics (2019) 35(20):4200–2. doi: 10.1093/bioinformatics/btz210
51. Vasaikar SV, Straub P, Wang J, Zhang B. LinkedOmics: Analyzing Multi-Omics Data Within and Across 32 Cancer Types. Nucleic Acids Res (2018) 46(D1):D956–63. doi: 10.1093/nar/gkx1090
52. Warde-Farley D, Donaldson SL, Comes O, Zuberi K, Badrawi R, Chao P, et al. The GeneMANIA Prediction Server: Biological Network Integration for Gene Prioritization and Predicting Gene Function. Nucleic Acids Res (2010) 38(Web Server issue):W214–220. doi: 10.1093/nar/gkq537
53. Pathan M, Keerthikumar S, Ang CS, Gangoda L, Quek CY, Williamson NA, et al. FunRich: An Open Access Standalone Functional Enrichment and Interaction Network Analysis Tool. Proteomics (2015) 15(15):2597–601. doi: 10.1002/pmic.201400515
54. Liu CJ, Hu FF, Xia MX, Han L, Zhang Q, Guo AY. GSCALite: A Web Server for Gene Set Cancer Analysis. Bioinformatics (2018) 34(21):3771–2. doi: 10.1093/bioinformatics/bty411
55. Davis AP, Grondin CJ, Johnson RJ, Sciaky D, Wiegers J, Wiegers TC, et al. Comparative Toxicogenomics Database (CTD): Update 2021. Nucleic Acids Res (2021) 49(D1):D1138–43. doi: 10.1093/nar/gkaa891
56. Chen ML, Sun A, Cao W, Eliason A, Mendez KM, Getzler AJ, et al. Physiological Expression and Function of the MDR1 Transporter in Cytotoxic T Lymphocytes. J Exp Med (2020) 217(5):e20191388. doi: 10.1084/jem.20191388
57. Badmann S, Heublein S, Mayr D, Reischer A, Liao Y, Kolben T, et al. M2 Macrophages Infiltrating Epithelial Ovarian Cancer Express MDR1: A Feature That May Account for the Poor Prognosis. Cells (2020) 9(5):1224. doi: 10.3390/cells9051224
58. Belisario DC, Akman M, Godel M, Campani V, Patrizio MP, Scotti L, et al. ABCA1/ABCB1 Ratio Determines Chemo- and Immune-Sensitivity in Human Osteosarcoma. Cells (2020) 9(3):647. doi: 10.3390/cells9030647
59. Guo Y, Wang ZW, Su WH, Chen J, Wang YL. Prognostic Value and Immune Infiltrates of ABCA8 and FABP4 in Stomach Adenocarcinoma. BioMed Res Int (2020) 2020:4145164. doi: 10.1155/2020/4145164
60. Van Kaer L. Major Histocompatibility Complex Class I-Restricted Antigen Processing and Presentation. Tissue Antigens (2002) 60(1):1–9. doi: 10.1034/j.1399-0039.2002.600101.x
61. Ritz U, Seliger B. The Transporter Associated With Antigen Processing (TAP): Structural Integrity, Expression, Function, and its Clinical Relevance. Mol Med (2001) 7(3):149–58.
62. Parcej D, Tampe R. ABC Proteins in Antigen Translocation and Viral Inhibition. Nat Chem Biol (2010) 6(8):572–80. doi: 10.1038/nchembio.410
63. Barbet G, Nair-Gupta P, Schotsaert M, Yeung ST, Moretti J, Seyffer F, et al. TAP Dysfunction in Dendritic Cells Enables Noncanonical Cross-Presentation for T Cell Priming. Nat Immunol (2021) 22(4):497–509. doi: 10.1038/s41590-021-00903-7
64. El Hage F, Durgeau A, Mami-Chouaib F. TAP Expression Level in Tumor Cells Defines the Nature and Processing of MHC Class I Peptides for Recognition by Tumor-Specific Cytotoxic T Lymphocytes. Ann N Y Acad Sci (2013) 1283:75–80. doi: 10.1111/j.1749-6632.2012.06777.x
65. Wang Z, Xu H, Zhu L, He T, Lv W, Wu Z. Establishment and Evaluation of a 6-Gene Survival Risk Assessment Model Related to Lung Adenocarcinoma Microenvironment. BioMed Res Int (2020) 2020:6472153. doi: 10.1155/2020/6472153
66. Yang J, Liu W, Yan Z, Li C, Liu S, Yang X, et al. Polymorphisms in Transporter Associated With Antigen Presenting are Associated With Cervical Intraepithelial Neoplasia and Cervical Cancer in a Chinese Han Population. HLA (2021) 98(1):23–36. doi: 10.1111/tan.14333
67. Zhang Y, Zhang Y, Wang J, Yang J, Yang G. Abnormal Expression of ABCD3 is an Independent Prognostic Factor for Colorectal Cancer. Oncol Lett (2020) 19(5):3567–77. doi: 10.3892/ol.2020.11463
68. Yang Y, Ding L, Li Y, Xuan C. Hsa_circ_0039411 Promotes Tumorigenesis and Progression of Papillary Thyroid Cancer by miR-1179/ABCA9 and miR-1205/MTA1 Signaling Pathways. J Cell Physiol (2020) 235(2):1321–9. doi: 10.1002/jcp.29048
69. Karas Zella MA, Sebastiao APM, Collaco LM, Ogata DC, Cecchetti G, Bartolomei IJP, et al. Prognostic Significance of CD133 and ABCB5 Expression in Papillary Thyroid Carcinoma. Eur J Histochem (2020) 64(4):3143. doi: 10.4081/ejh.2020.3143
70. Ohashi R, Kawahara K, Namimatsu S, Okamura R, Igarashi T, Sugitani I, et al. Expression of MRP1 and ABCG2 is Associated With Adverse Clinical Outcomes of Papillary Thyroid Carcinoma With a Solid Component. Hum Pathol (2017) 67:11–7. doi: 10.1016/j.humpath.2017.03.012
71. Mato E, Gonzalez C, Moral A, Perez JI, Bell O, Lerma E, et al. ABCG2/BCRP Gene Expression is Related to Epithelial-Mesenchymal Transition Inducer Genes in a Papillary Thyroid Carcinoma Cell Line (TPC-1). J Mol Endocrinol (2014) 52(3):289–300. doi: 10.1530/JME-14-0051
72. Ruggeri RM, Sciacchitano S, Vitarelli E, Trimarchi F, Barresi G, Trovato M. Immunoexpression of Multidrug-Resistance Protein 2 and Cyclooxygenase 2 in Medullary Thyroid Carcinomas. Arch Pathol Lab Med (2006) 130(7):1014–9. doi: 10.5858/2006-130-1014-IOMPAC
73. Abbasifarid E, Sajjadi-Jazi SM, Beheshtian M, Samimi H, Larijani B, Haghpanah V. The Role of ATP-Binding Cassette Transporters in the Chemoresistance of Anaplastic Thyroid Cancer: A Systematic Review. Endocrinology (2019) 160(8):2015–23. doi: 10.1210/en.2019-00241
74. Shibuya M. Vascular Endothelial Growth Factor Receptor-2: Its Unique Signaling and Specific Ligand, VEGF-E. Cancer Sci (2003) 94(9):751–6. doi: 10.1111/j.1349-7006.2003.tb01514.x
75. Apte RS, Chen DS, Ferrara N. VEGF in Signaling and Disease: Beyond Discovery and Development. Cell (2019) 176(6):1248–64. doi: 10.1016/j.cell.2019.01.021
76. Fukumura D, Kloepper J, Amoozgar Z, Duda DG, Jain RK. Enhancing Cancer Immunotherapy Using Antiangiogenics: Opportunities and Challenges. Nat Rev Clin Oncol (2018) 15(5):325–40. doi: 10.1038/nrclinonc.2018.29
77. Shklovskaya E, Rizos H. MHC Class I Deficiency in Solid Tumors and Therapeutic Strategies to Overcome it. Int J Mol Sci (2021) 22(13):6741. doi: 10.3390/ijms22136741
78. Wang JY, Wang WP. B7-H4, a Promising Target for Immunotherapy. Cell Immunol (2020) 347:104008. doi: 10.1016/j.cellimm.2019.104008
79. Andersson J, Tran DQ, Pesu M, Davidson TS, Ramsey H, O'Shea JJ, et al. CD4+ FoxP3+ Regulatory T Cells Confer Infectious Tolerance in a TGF-Beta-Dependent Manner. J Exp Med (2008) 205(9):1975–81. doi: 10.1084/jem.20080308
80. Peng L, Yuan XQ, Zhang CY, Ye F, Zhou HF, Li WL, et al. High TGF-Beta1 Expression Predicts Poor Disease Prognosis in Hepatocellular Carcinoma Patients. Oncotarget (2017) 8(21):34387–97. doi: 10.18632/oncotarget.16166
81. Wang J, Xiang H, Lu Y, Wu T. Role and Clinical Significance of TGFbeta1 and TGFbetaR1 in Malignant Tumors (Review). Int J Mol Med (2021) 47(4):55. doi: 10.3892/ijmm.2021.4888
82. Sag D, Ayyildiz ZO, Gunalp S, Wingender G. The Role of TRAIL/DRs in the Modulation of Immune Cells and Responses. Cancers (Basel) (2019) 11(10):1469. doi: 10.3390/cancers11101469
83. Valatas V, Kolios G, Bamias G. TL1A (TNFSF15) and DR3 (TNFRSF25): A Co-Stimulatory System of Cytokines With Diverse Functions in Gut Mucosal Immunity. Front Immunol (2019) 10:583. doi: 10.3389/fimmu.2019.00583
84. Vanamee ES, Faustman DL. On the TRAIL of Better Therapies: Understanding TNFRSF Structure-Function. Cells (2020) 9(3):764. doi: 10.3390/cells9030764
85. Vilgelm AE, Richmond A. Chemokines Modulate Immune Surveillance in Tumorigenesis, Metastasis, and Response to Immunotherapy. Front Immunol (2019) 10:333. doi: 10.3389/fimmu.2019.00333
86. Kitahara CM, Preston DL, Neta G, Little MP, Doody MM, Simon SL, et al. Occupational Radiation Exposure and Thyroid Cancer Incidence in a Cohort of U.S. Radiologic Technologists 1983-2013. Int J Cancer (2018) 143(9):2145–9. doi: 10.1002/ijc.31270
87. An SY, Kim SY, Oh DJ, Min C, Sim S, Choi HG. Obesity is Positively Related and Tobacco Smoking and Alcohol Consumption are Negatively Related to an Increased Risk of Thyroid Cancer. Sci Rep (2020) 10(1):19279. doi: 10.1038/s41598-020-76357-y
88. Lee JH, Youn S, Jung S, Kim K, Chai YJ, Chung YS, et al. A National Database Analysis for Factors Associated With Thyroid Cancer Occurrence. Sci Rep (2020) 10(1):17791. doi: 10.1038/s41598-020-74546-3
89. Marotta V, Malandrino P, Russo M, Panariello I, Ionna F, Chiofalo MG, et al. Fathoming the Link Between Anthropogenic Chemical Contamination and Thyroid Cancer. Crit Rev Oncol Hematol (2020) 150:102950. doi: 10.1016/j.critrevonc.2020.102950
90. Zhang Q, Jiang C, Li H, Zhang C, Wu H, Huang F. Effect of the Interaction Between Cadmium Exposure and CLOCK Gene Polymorphisms on Thyroid Cancer: A Case-Control Study in China. Biol Trace Elem Res (2020) 196(1):86–95. doi: 10.1007/s12011-019-01904-2
91. Morton LM, Karyadi DM, Stewart C, Bogdanova TI, Dawson ET, Steinberg MK, et al. Radiation-Related Genomic Profile of Papillary Thyroid Carcinoma After the Chernobyl Accident. Science (2021) 372(6543):eabg2538. doi: 10.1126/science.abg2538
92. Zhang D, Jones RR, James P, Kitahara CM, Xiao Q. Associations Between Artificial Light at Night and Risk for Thyroid Cancer: A Large US Cohort Study. Cancer (2021) 127(9):1448–58. doi: 10.1002/cncr.33392
93. Hu MJ, He JL, Tong XR, Yang WJ, Zhao HH, Li GA, et al. Associations Between Essential Microelements Exposure and the Aggressive Clinicopathologic Characteristics of Papillary Thyroid Cancer. Biometals (2021) 34(4):909–21. doi: 10.1007/s10534-021-00317-w
Keywords: thyroid carcinoma, ATP-binding cassette transporters, prognosis, immune cell infiltration, immunomodulation
Citation: Wang L, Sun X, He J and Liu Z (2022) Identification and Validation of Prognostic Related Hallmark ATP-Binding Cassette Transporters Associated With Immune Cell Infiltration Patterns in Thyroid Carcinoma. Front. Oncol. 12:781686. doi: 10.3389/fonc.2022.781686
Received: 04 October 2021; Accepted: 01 June 2022;
Published: 28 June 2022.
Edited by:
Nadia Judith Jacobo-Herrera, Instituto Nacional de Ciencias Médicas y Nutrición Salvador Zubirán (INCMNSZ), MexicoReviewed by:
Gabriela Figueroa González, Universidad Nacional Autónoma de México, MexicoCopyright © 2022 Wang, Sun, He and Liu. This is an open-access article distributed under the terms of the Creative Commons Attribution License (CC BY). The use, distribution or reproduction in other forums is permitted, provided the original author(s) and the copyright owner(s) are credited and that the original publication in this journal is cited, in accordance with accepted academic practice. No use, distribution or reproduction is permitted which does not comply with these terms.
*Correspondence: Zhen Liu, bGl1emhlbjE5NzNAYWxpeXVuLmNvbQ==
Disclaimer: All claims expressed in this article are solely those of the authors and do not necessarily represent those of their affiliated organizations, or those of the publisher, the editors and the reviewers. Any product that may be evaluated in this article or claim that may be made by its manufacturer is not guaranteed or endorsed by the publisher.
Research integrity at Frontiers
Learn more about the work of our research integrity team to safeguard the quality of each article we publish.