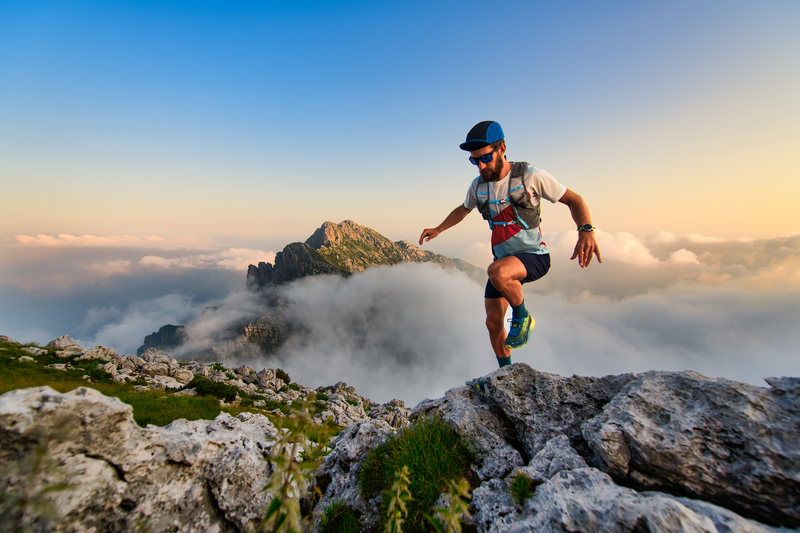
94% of researchers rate our articles as excellent or good
Learn more about the work of our research integrity team to safeguard the quality of each article we publish.
Find out more
ORIGINAL RESEARCH article
Front. Oncol. , 18 January 2023
Sec. Cancer Imaging and Image-directed Interventions
Volume 12 - 2022 | https://doi.org/10.3389/fonc.2022.1082454
Background: Whole-lesion histogram analysis can provide comprehensive assessment of tissues by calculating additional quantitative metrics such as skewness and kurtosis; however, few studies have evaluated its value in the differential diagnosis of lung lesions.
Purpose: To compare the diagnostic performance of conventional diffusion-weighted imaging (DWI), intravoxel incoherent motion (IVIM) magnetic resonance imaging (MRI) and diffusion kurtosis imaging (DKI) in differentiating lung cancer from focal inflammatory lesions, based on whole-lesion volume histogram analysis.
Methods: Fifty-nine patients with solitary pulmonary lesions underwent multiple b-values DWIs, which were then postprocessed using mono-exponential, bi-exponential and DKI models. Histogram parameters of the apparent diffusion coefficient (ADC), true diffusivity (D), pseudo-diffusion coefficient (D*), and perfusion fraction (f), apparent diffusional kurtosis (Kapp) and kurtosis-corrected diffusion coefficient (Dapp) were calculated and compared between the lung cancer and inflammatory lesion groups. Receiver operating characteristic (ROC) curves were constructed to evaluate the diagnostic performance.
Results: The ADCmean, ADCmedian, Dmean and Dmedian values of lung cancer were significantly lower than those of inflammatory lesions, while the ADCskewness, Kappmean, Kappmedian, KappSD, Kappkurtosis and Dappskewness values of lung cancer were significantly higher than those of inflammatory lesions (all p < 0.05). ADCskewness (p = 0.019) and Dmedian (p = 0.031) were identified as independent predictors of lung cancer. Dmedian showed the best performance for differentiating lung cancer from inflammatory lesions, with an area under the ROC curve of 0.777. Using a Dmedian of 1.091 × 10-3 mm2/s as the optimal cut-off value, the sensitivity, specificity, positive predictive value and negative predictive value were 69.23%, 85.00%, 90.00% and 58.62%, respectively.
Conclusions: Whole-lesion histogram analysis of DWI, IVIM and DKI parameters is a promising approach for differentiating lung cancer from inflammatory lesions, and Dmedian shows the best performance in the differential diagnosis of solitary pulmonary lesions.
Lung cancer has been the most common cancer in China, and is the leading cause of cancer-related deaths in both China and United States (1). In clinical practice, detection of lung lesions mainly relies on computed tomography (CT) due to its short-time scanning and high resolution of density. As a preferred imaging technique for detecting lung lesions, CT imaging can capture the important features of lesions in terms of morphology, boundary, tissue density and enhancement. However, given that lung cancer and some benign lesions (e.g., inflammatory lesions) usually have overlapped features on CT, it is occasionally difficult to distinguish lung cancer from benign lesions. Although CT-guided transthoracic lung biopsy has been widely used for pathologic diagnosis of lung lesions, this technique is invasive and not suitable for patients with poor lung function. Thus, it is essential to develop non-invasive and valuable tools for the accurate diagnosis of lung lesions. Early diagnosis and differentiation of pulmonary lesions will help to select the optimal therapeutic strategy and avoid some unnecessary treatments.
In recent years, diffusion-weighted imaging (DWI), a non-invasive magnetic resonance imaging (MRI) technique, has shown potential diagnostic value for lung lesions. Previous studies have demonstrated that the apparent diffusion coefficient (ADC), a parameter calculated from DWI data based on a mono-exponential model, can be used to distinguish malignant and benign pulmonary lesions (2, 3), and may also help characterize the subtype of lung cancer (2). However, the ADC value is also affected by tissue microcirculation perfusion. Compared with conventional DWI, intravoxel incoherent motion (IVIM) MR imaging allows separate calculation of diffusion and perfusion parameters based on a bi-exponential model (4). Additionally, another advanced diffusion model, diffusion kurtosis imaging (DKI), has been a new topic of growing interest in radiology and enables characterization of non-Gaussian water diffusion behavior (5). Considering that analyses using mono- and bi-exponential DWI models and DKI model provide information on different aspects of tissue microstructure, it is necessary to explore and compare their roles in the differentiation of solitary pulmonary lesions, and thus helping to select the optimal imaging parameter for lesion diagnosis. In fact, several studies have been conducted to address this issue (3, 6, 7), but did not yield consistent and conclusive results. It should be noted that most of previous studies analyzed the diffusion MR imaging parameters based on single-section (usually selected the slice with the largest diameter of lung lesions) regions of interest (ROIs) rather than whole-lesion volumes. Traditional single-section ROI analysis cannot capture the heterogeneity within the whole lesions, which may lead to subjective bias and possible sampling error of measurement.
Whole-lesion histogram analysis, a method that can provide comprehensive microstructural information of lesions by calculating additional quantitative metrics (such as skewness and kurtosis), has been increasingly applied in cancer imaging researches. This volumetric analysis can address internal lesion heterogeneity, and has better interobserver reproducibility and higher diagnostic accuracy compared with single-section ROI analysis (8, 9). Quantitative whole-lesion histogram analysis has been used to differentiate histological grades of rectal cancer (10) and gastric cancer (11), to distinguish benign and malignant breast lesions (12), and to assess response to combined chemotherapy and radiation therapy (CRT) in patients with rectal cancer (8). However, to our knowledge, studies using whole-lesion histogram analysis of mono- and bi-exponential DWI and DKI for differentiation of lung lesions are limited. Thus, the present study aimed to evaluate the diagnostic performance of conventional ADC, IVIM and DKI metrics in differentiating primary lung cancer from focal inflammatory lesions, by using histogram analysis derived from whole-lesion volumes.
This prospective single-center study was approved by the Institutional Review Board of The First Affiliated Hospital of Henan University. Written informed consent was obtained from each patient prior to inclusion. From February 2022 to September 2022, consecutive patients who met the following inclusion criteria were recruited: (1) pulmonary solitary lesions detected by initial CT examinations, and the maximum diameter of the lesion was greater than or equal to 15 mm; (2) the pulmonary lesions could be clearly visualized on subsequent MR imaging; (3) the solid component accounted for more than 50% of the whole lesion; and (4) no radiochemotherapy or targeted drug therapy was performed prior to MRI examinations. The exclusion criteria were: (1) CT images showed ground glass foci; (2) poor physical condition that led to intolerance to MRI examinations; (3) contraindication to MR scanning, such as claustrophobia and placement of artificial cardiac pacemaker; and (4) poor MR imaging quality that made it impossible to perform data post-processing.
CT-guided transthoracic needle biopsy or surgery was performed within 10 days after MRI examinations. Diagnosis of primary lung cancer was confirmed by pathological results, while diagnosis of inflammatory lesions was confirmed by either pathological results or radiological follow-up more than one month after anti-inflammatory treatment (manifested as the disappearance or obvious regression of the lesions).
Seventy-four consecutive patients were included in this study, and 15 patients were excluded due to the following reasons: (1) other types of space-occupying lung lesions, including low-grade mucoepidermoid carcinoma (n = 1), atypical hyperplasia (n = 1), low-grade intraepithelial neoplasia (n = 1), lymphoma (n = 1), and hamartoma (n = 2); (2) contraindication to MR scanning (n = 4); and (3) poor imaging quality (n = 5). Thus, the MR imaging data of lung lesions in the remaining 59 patients (27 males and 32 females; mean age 57.8 ± 9.9, range from 39 to 79 years) were used for final analyses.
Within one week after the lesions were detected by CT, all patients underwent MR imaging using a 1.5-T MRI scanner (MAGNETOM Sempra, Siemens Healthcare, Erlangen, Germany) with a 13-channel body coil. First, conventional axial T1-weighted, as well as axial and coronal T2-weighted imaging sequences were acquired. Then, conventional DWI, IVIM and DKI images were obtained using multiple b-values. Detailed MR imaging parameters are shown in Table 1.
MRI data analysis was performed using the Medical Imaging Interaction Toolkit (MITK) Workbench. Multiple b-values DWI data were post-processed using mono-exponential (b-values = 0 and 800 s/mm2), bi-exponential IVIM (b-values = 0, 10, 20, 30, 50, 80, 150, 300, 500, 800, and 1000 s/mm2) and DKI (b-values = 0, 500, 1000, 1500, and 2000 s/mm2) models. Subsequently, the values of the ADC, IVIM parameters [true molecular diffusion coefficient (D), pseudo-diffusion coefficient (D*), and perfusion fraction (f)] and DKI parameters [apparent diffusional kurtosis (Kapp) and kurtosis-corrected diffusion coefficient (Dapp)] were calculated. The calculation equations and the meanings for ADC, IVIM and DKI parameters have been described in detail in previous studies (3, 13).
For further histogram analyses, ROIs were manually delineated layer by layer on ADC maps by referring to the conventional axial T2-weighted images, and areas with vessels, cavity and necrosis within the lesions were excluded, thus generating a three-dimensional volume of interest (VOI). The ROIs were automatically copied from the ADC maps to the corresponding IVIM and DKI parametric maps. Then, histogram analyses of these quantitative MR parameters were performed based on the information extracted from each voxel within the entire VOI. The calculated histogram parameters included: (1) mean; (2) median; (3) standard deviation (SD); (4) skewness, which measures the degree of histogram asymmetry around the mean; and (5) kurtosis, which measures the histogram sharpness.
Two of the authors (JXL and BLW, radiologists with five and seven years of experience in chest imaging, respectively) who were blinded to the histopathological results independently performed data analysis.
Statistical analyses were performed using SPSS version 21.0 (IBM Corp, Armonk, NY) and MedCalc version 19.2.0 (MedCalc, Mariakerke, Belgium) software. Between-group differences in demographic and clinical data, as well as in all histogram parameters were determined using chi-square test, two-sample t-test and Mann-Whitney U-test. We calculated the intraclass correlation coefficient (ICC) by using a two-way random-effects model to evaluate the consistency and reliability of the same histogram parameter between the two independent observers (< 0.40, poor agreement; 0.40–0.59, fair agreement; 0.60–0.74, good agreement; and ≥ 0.75, excellent agreement). For histogram metrics showing significant between-group differences, univariate and multivariate logistic regression analyses were performed to determine the independent predictors of lung cancer. Furthermore, we used receiver operating characteristic (ROC) curves to assess the diagnostic performance of each histogram parameter in differentiating lung cancer from inflammatory lesions, and identified the corresponding optimal cut-off value. Then, the area under the ROC curve (AUC), sensitivity, specificity, positive predictive value (PPV) and negative predictive value (NPV) were calculated. The DeLong test (14) was used to compare the AUC values of different histogram metrics, and the Benjamin–Hochberg false discovery rate was used to correct for multiple comparisons (15). A p value less than 0.05 was considered to indicate statistical significance.
According to the pathological results or radiological follow-up after anti-inflammatory treatment, the included 59 patients were divided into two groups: the lung cancer group (n = 39) and the inflammatory lesion group (n = 20). The demographic and clinical characteristics of the two patient groups are shown in Table 2. There were no significant differences in age, sex, lesion size and lesion location between the lung cancer and inflammatory lesion groups (all p > 0.05).
As show in Table 3, all the ADC, D, D*, f, Kapp and Dapp histogram parameters of pulmonary lesions showed excellent or good interobserver agreement, with an ICC range from 0.678 to 0.894.
Compared to inflammatory lesions, lung cancer had significantly lower ADCmean, ADCmedian, Dmean and Dmedian values, and showed significantly higher ADCskewness, Kappmean, Kappmedian, KappSD, Kappkurtosis and Dappskewness values (all p < 0.05) (Table 4). No significant differences in ADCSD, ADCkurtosis, DSD, Dkurtosis, Dskewness, Kappskewness, Dappmean, Dappmedian, DappSD and Dappkurtosis values were observed between the lung cancer and inflammatory lesion groups (all p > 0.05). We also did not find significant between-group differences in all D* and f histogram metrics (all p > 0.05). Representative cases of lung cancer and inflammatory lesions are shown in Figures 1, 2, respectively.
Figure 1 A 56-year-old female diagnosed with adenocarcinoma of the left lower lobe. (A) ADC map; (B) D map; (C) D* map; (D) f map (E) Dapp map; (F) Kapp map; (G) microscopic image of H&E staining (original magnification, ×200); (H) an example diagram of the histogram.
Figure 2 A 52-year-old male diagnosed with lung abscess of the left lower lobe. (A) ADC map; (B) D map; (C) D* map; (D) f map (E) Dapp map; (F) Kapp map.
As shown in Table 5, univariate logistic regression analysis revealed that ADCmean, ADCmedian, ADCskewness, Dmedian, Kappmean, Kappmedian, KappSD, Kappkurtosis and Dappskewness were statistically significant variables in the evaluation of lung cancer (all p < 0.05). Further multivariate logistic regression analysis showed that ADCskewness (odds ratio [OR] = 7.061, p = 0.019) and Dmedian (OR = 0.044, p = 0.031) were independent predictors of lung cancer.
Figure 3 shows the ROC curves of different histogram parameters for differentiating malignant lung cancers from benign inflammatory lesions, and the measurements of ROC analyses are shown in Table 6. ROC curve analysis revealed that Dmedian showed the best performance for differentiating lung cancer from inflammatory lesions, with an area under the ROC curve of 0.777 (95% confidence interval [CI]: 0.660-0.984). Using a Dmedian of 1.091 × 10-3 mm2/s as the optimal cut-off value, the sensitivity, specificity, PPV and NPV were 69.23%, 85.00%, 90.00% and 58.62%, respectively. The AUC values of ADCmean, ADCmedian and ADCskewness, Dmean, Kappmean, Kappmedian, KappSD, Kappkurtosis and Dappskewness were 0.673 (95% CI: 0.536-0.810), 0.681 (95% CI: 0.544-0.818), 0.678 (95% CI: 0.517-0.840), 0.685 (95% CI: 0.543-0.826), 0.673 (95% CI: 0.532-0.814), 0.686 (95% CI: 0.546-0.826), 0.715 (95% CI: 0.586-0.845), 0.711 (95% CI: 0.577-0.846), and 0.721 (95% CI: 0.571-0.870), respectively. Further pairwise comparisons of ROC curves did not yield significant differences in AUCs (all p > 0.05). ADCskewness had the highest sensitivity (92.31%) and ADCmean showed the highest specificity (95.00%) in differentiating lung cancer from inflammatory lesions.
Figure 3 ROC curves of the quantitative histogram metrics for differentiating lung cancer from inflammatory lesions.
Table 6 ROC analysis results of whole-lesion histogram parameters for distinguishing lung cancer from benign lung lesions.
Using whole-lesion volume histogram analysis, the present study suggests that histogram metrics of DWI, IVIM and DKI parameters can objectively provide the overall histologic information of lung lesions and are clinically feasible and of value in differentiating lung cancer from benign inflammatory lesions. Our study also found significant between-group differences in histogram features of multiple magnetic resonance diffusion parameters, indicating that lung cancer has different histological features compared with inflammatory lesions. Furthermore, of all the histogram metrics, Dmedian showed the best diagnostic performance in differentiating lung cancer from benign inflammatory lesions, and ADCskewness and Dmedian were identified as independent predictors of lung cancer. Our findings confirmed the potential value of histogram analysis of quantitative MRI parameters in the diagnosis of lung lesions.
An important finding of our study was that lung cancer showed lower ADCmean, ADCmedian, Dmean and Dmedian values compared to inflammatory lesions. Both the ADC and D are diffusion-related parameters, and prior study has demonstrated a strong negative association between tumor cellularity and the ADC value (16). Thus, given that malignant tumors usually have rapid cell proliferation and high cell density and malignant tumor cells have large nuclei and little cytoplasm, lower ADC and D histogram metrics (mean and median) in lung cancer may be attributed to higher cellularity and reduced extracellular space that leads to a more pronounced restriction of diffusional motion of water molecules. Using single-section ROI method and whole-lesion histogram analysis, prior studies have consistently demonstrated significantly lower ADCmean and Dmean values in malignant lung tumors than benign lesions (3, 6, 7, 9, 13, 17–19). In contrast, another study based on whole-lesion histogram analysis revealed significantly higher ADCmean and ADCmedian values in lung cancer compared with benign inflammatory lesions (20). This opposite result may be attributed to the selection of different benign lung lesions, as they included lung cancer and specific infectious lesions (pulmonary abscesses and mycobacterium infections) for comparison. Overall, our findings of reduced ADCmean and ADCmedian in lung cancer relative to inflammatory lesions are compatible with these previous studies.
Another important finding of our study was that lung cancer had significantly higher values in most of the Kapp histogram metrics (mean, median, SD and kurtosis) compared to inflammatory lesions. DKI detects non-Gaussian interactions of water molecules within tissue environments, and the additional parameter derived from DKI model, Kapp, may reflect the heterogeneity and irregularity of cellular microstructure, as well as the amounts of interfaces within cellular tissues (5). Thus, the possible reason for the higher histogram metrics of DKI parameters in lung cancer is that the cellular structure and tissue environment of lung cancer are more complex than those of inflammatory lesions, leading to more significant non-Gaussian distribution in lung cancer. Similarly, higher mean Kapp value in malignant pulmonary tumors than in benign lesions has been demonstrated by previous studies using single-section ROI analyses (3, 21). However, another study found that the Kapp of malignant tumors was lower than that of benign lesions, although the difference was not statistically significant (7). Inconsistency of previous research results may be associated with differences in the selection of b-values, the inclusion criteria of lung lesions and the chosen of analytical methods. Notably, most of previous studies only used single-slice ROI method to compare differences in DKI parameters between malignant and benign lung lesions, and did not consider the important aspects of texture and heterogeneity in the whole lesions. Considering that whole-lesion histogram analysis allows for a more comprehensive, intuitive and accurate assessment of the microscopic motion of water molecules in tissues compared to traditional single-section ROI method, our findings may provide more reliable biomarkers for the differential diagnosis of lung lesions.
Additionally, we found that both the ADCskewness and Dappskewness values of lung cancer showed a “positive skewness” pattern and were significantly higher than that of inflammatory lesions, which is consistent with a recent study (13). Skewness reflects the degree of asymmetry of the data, and the skewness of the normal distribution is 0. A “positive skewness” pattern of the ADC and Dapp means that more data are distributed to the left of the mean value. Higher ADCskewness and Dappskewness values in lung cancer might be due to its higher cell density and more pixels had lower ADC and Dapp values.
Both the quantitative parameters D* and f derived from IVIM are related to perfusion. In IVIM theory, D* is considered to be proportional to the blood velocity and capillary segment length, while f reflects the blood perfusion of the tissue and is related to blood volume (4, 22). Differences in D* and f between malignant and benign lung lesions have been inconsistent in previous studies using single-section ROI analyses. Some studies found no significant differences in both D* and f values between lung cancer and benign lesions (7, 23, 24), while others revealed significant higher D* or lower f values in lung cancer compared to benign lesions (6, 25, 26). For whole-lesion histogram features, in line with all prior whole-lesion histogram studies (9, 13), we also did not find significant differences in any histogram metrics of D* and f between lung cancer and benign inflammatory lesions, suggesting that D* and f histogram characteristics cannot reflect the characteristics of lung lesions well and have relatively limited value in the differential diagnosis of pulmonary lesions. Two possible reasons may account for the non-significant differences in D* and f between lung cancer and inflammatory lesions. First, both our findings and those of previous studies revealed that measurement consistency of D* and f among different observers were relatively low (3, 13), and prior studies also found that D* had a relatively large SD (23, 26), indicating poor reproducibility of these two parameters. Second, malignant lung tumors have rich neovascularization due to the high expression of vascular endothelial growth factor, which may theoretically increase blood supply of tumor tissues (27); however, malignant tumors tend to have immature new blood vessels with increased microvascular permeability and relatively insufficient veins or lymphatic vessels to drain the excess leaking fluid, which may result in compression of tumoral vessels and lead to reduced blood perfusion in the tissues. Thus, non-significant difference in D* and f values between lung cancer and benign lesions may be due to the combined effect of the two factors.
Further ROC analyses suggested that whole-lesion quantitative histogram analysis of conventional DWI, IVIM and DKI are clinically feasible and of potential value in differentiating lung cancer from inflammatory lesions. We found that ADCskewness had the highest sensitivity and ADCmean achieved the highest specificity in differentiating lung cancer from inflammatory lesions. This finding means that ADCskewness, a metric that reflects the higher cellular density in malignant tumors, has a relatively better performance in the diagnosis of lung cancer; while ADCmean, a metric that measures the overall diffusion and perfusion information of lesions, shows a better performance in identifying inflammatory lesions. Moreover, our study revealed that Dmedian had the highest AUC in differentiating lung cancer from inflammatory lesions, and further multivariate logistic regression analysis found that the Dmedian was an independent predictor of lung cancer. These findings suggest that Dmedian may act as a reliable biomarker for differentiating lung cancer from inflammatory lesions, and highlight the superiority of the whole-lesion volume histogram analysis in the differential diagnosis of solitary lung lesions. A recent meta-analysis also confirmed that D had the best performance in the differential diagnosis between malignant and benign solitary pulmonary lesions (28). Unlike ADC that is sensitive to both the diffusion of water molecules and microcirculation perfusion, D reflects only the pure diffusion of water molecules by removing the effect of perfusion portion and can achieve a more accurate assessment of the restricted movements of water molecules in tissues. Malignant tumors have high cell density and reduced extracellular space; thus, the low diffusion coefficient detected in lung cancer may be mainly related to pure molecular diffusion rather than perfusion.
This study has several limitations. First, the sample size included was small, resulting in a small number of lung lesions available for analysis. We also did not evaluate the diagnostic performance of whole-lesion histogram analysis of DWI, IVIM and DKI in distinguishing subtypes of lung cancer due to a small sample size. Future studies with larger sample sizes are needed to verify our findings and to further assess the role of DWI, IVIM and DKI histogram features in the differential diagnosis of pathological types of lung cancer. Second, we only included lung lesions with a maximum diameter ≥ 15 mm for histogram analysis, which may lead to selection bias. Third, some inflammatory lesions were confirmed based on long-term follow-up rather than by pathological examinations, which may lead to inaccurate results to some extent.
In summary, the results of our study suggest that whole-lesion quantitative histogram analysis of DWI, IVIM and DKI parameters provides a useful tool for differentiating lung cancer from inflammatory lesions, and Dmedian may act as a potential biomarker for the differential diagnosis of solitary pulmonary lesions.
The original contributions presented in the study are included in the article/supplementary material. Further inquiries can be directed to the corresponding authors.
The studies involving human participants were reviewed and approved by the Institutional Review Board of The First Affiliated Hospital of Henan University. The patients/participants provided their written informed consent to participate in this study.
JZ, ZW, JL and BW contributed to the conception and design of the study. JL, BW, ZH, YZ, SZ, SG, SX, XW and TT contributed to literature search, data analysis and data interpretation. JL and BW contributed to the drafting of the manuscript. JZ, ZW and JL obtained funding to support this work. JZ and BW critically revised the manuscript. All authors approved the final version of the manuscript.
This study was supported by the Postgraduate Cultivating Innovation and Quality Improvement Action Plan of Henan University (SYLY2022156), the Henan Province Medical Science and Technology Research Project (LHGJ20210565) and the Scientific Research Project of Hubei Provincial Health Commission (WJ2023M066).
The authors declare that the research was conducted in the absence of any commercial or financial relationships that could be construed as a potential conflict of interest.
All claims expressed in this article are solely those of the authors and do not necessarily represent those of their affiliated organizations, or those of the publisher, the editors and the reviewers. Any product that may be evaluated in this article, or claim that may be made by its manufacturer, is not guaranteed or endorsed by the publisher.
1. Xia C, Dong X, Li H, Cao M, Sun D, He S, et al. Cancer statistics in China and united states 2022: profiles, trends, and determinants. Chin Med J (Engl). (2022) 135(5):584–90. doi: 10.1097/cm9.0000000000002108
2. Shen G, Jia Z, Deng H. Apparent diffusion coefficient values of diffusion-weighted imaging for distinguishing focal pulmonary lesions and characterizing the subtype of lung cancer: a meta-analysis. Eur Radiol (2016) 26(2):556–66. doi: 10.1007/s00330-015-3840-y
3. Zheng Y, Li J, Chen K, Zhang X, Sun H, Li S, et al. Comparison of conventional DWI, intravoxel incoherent motion imaging, and diffusion kurtosis imaging in differentiating lung lesions. Front Oncol (2021) 11:815967. doi: 10.3389/fonc.2021.815967
4. Le Bihan D, Breton E, Lallemand D, Aubin ML, Vignaud J, Laval-Jeantet M. Separation of diffusion and perfusion in intravoxel incoherent motion MR imaging. Radiology (1988) 168(2):497–505. doi: 10.1148/radiology.168.2.3393671
5. Rosenkrantz AB, Padhani AR, Chenevert TL, Koh DM, De Keyzer F, Taouli B, et al. Body diffusion kurtosis imaging: Basic principles, applications, and considerations for clinical practice. J Magn Reson Imaging (2015) 42(5):1190–202. doi: 10.1002/jmri.24985
6. Deng Y, Li X, Lei Y, Liang C, Liu Z. Use of diffusion-weighted magnetic resonance imaging to distinguish between lung cancer and focal inflammatory lesions: A comparison of intravoxel incoherent motion derived parameters and apparent diffusion coefficient. Acta Radiol (2016) 57(11):1310–7. doi: 10.1177/0284185115586091
7. Wan Q, Deng YS, Lei Q, Bao YY, Wang YZ, Zhou JX, et al. Differentiating between malignant and benign solid solitary pulmonary lesions: Are intravoxel incoherent motion and diffusion kurtosis imaging superior to conventional diffusion-weighted imaging? Eur Radiol (2019) 29(3):1607–15. doi: 10.1007/s00330-018-5714-6
8. Nougaret S, Vargas HA, Lakhman Y, Sudre R, Do RK, Bibeau F, et al. Intravoxel incoherent motion-derived histogram metrics for assessment of response after combined chemotherapy and radiation therapy in rectal cancer: Initial experience and comparison between single-section and volumetric analyses. Radiology (2016) 280(2):446–54. doi: 10.1148/radiol.2016150702
9. Yuan M, Zhong Y, Zhang YD, Yu TF, Li H, Wu JF. Volumetric analysis of intravoxel incoherent motion imaging for assessment of solitary pulmonary lesions. Acta Radiol (2017) 58(12):1448–56. doi: 10.1177/0284185117698863
10. Peng Y, Tang H, Meng X, Shen Y, Hu D, Kamel I, et al. Histological grades of rectal cancer: whole-volume histogram analysis of apparent diffusion coefficient based on reduced field-of-view diffusion-weighted imaging. Quant. Imaging Med Surg (2020) 10(1):243–56. doi: 10.21037/qims.2019.11.17
11. Zhang Y, Chen J, Liu S, Shi H, Guan W, Ji C, et al. Assessment of histological differentiation in gastric cancers using whole-volume histogram analysis of apparent diffusion coefficient maps. J Magn Reson Imaging (2017) 45(2):440–9. doi: 10.1002/jmri.25360
12. Li T, Hong Y, Kong D, Li K. Histogram analysis of diffusion kurtosis imaging based on whole-volume images of breast lesions. J Magn Reson Imaging (2020) 51(2):627–34. doi: 10.1002/jmri.26884
13. Zhu Q, Ren C, Xu JJ, Li MJ, Yuan HS, Wang XH. Whole-lesion histogram analysis of mono-exponential and bi-exponential diffusion-weighted imaging in differentiating lung cancer from benign pulmonary lesions using 3 T MRI. Clin Radiol (2021) 76(11):846–53. doi: 10.1016/j.crad.2021.07.003
14. DeLong ER, DeLong DM, Clarke-Pearson DL. Comparing the areas under two or more correlated receiver operating characteristic curves: a nonparametric approach. Biometrics (1988) 44(3):837–45. doi: 10.2307/2531595
15. Genovese CR, Lazar NA, Nichols T. Thresholding of statistical maps in functional neuroimaging using the false discovery rate. Neuroimage (2002) 15(4):870–8. doi: 10.1006/nimg.2001.1037
16. Yin Y, Sedlaczek O, Muller B, Warth A, Gonzalez-Vallinas M, Lahrmann B, et al. Tumor cell load and heterogeneity estimation from diffusion-weighted MRI calibrated with histological data: An example from lung cancer. IEEE Trans Med Imaging (2018) 37(1):35–46. doi: 10.1109/tmi.2017.2698525
17. Çakır Ç., Gençhellaç H, Temizöz O, Polat A, Şengül E, Duygulu G. Diffusion weighted magnetic resonance imaging for the characterization of solitary pulmonary lesions. Balkan. Med J (2015) 32(4):403–9. doi: 10.5152/balkanmedj.2015.15663
18. Çakmak V, Ufuk F, Karabulut N. Diffusion-weighted MRI of pulmonary lesions: Comparison of apparent diffusion coefficient and lesion-to-spinal cord signal intensity ratio in lesion characterization. J Magn Reson Imaging (2017) 45(3):845–54. doi: 10.1002/jmri.25426
19. Liang J, Li J, Li Z, Meng T, Chen J, Ma W, et al. Differentiating the lung lesions using intravoxel incoherent motion diffusion-weighted imaging: a meta-analysis. BMC Cancer (2020) 20(1):799. doi: 10.1186/s12885-020-07308-z
20. Usuda K, Iwai S, Yamagata A, Iijima Y, Motono N, Matoba M, et al. Whole-lesion apparent diffusion coefficient histogram analysis: Significance for discriminating lung cancer from pulmonary abscess and mycobacterial infection. Cancers (Basel). (2021) 13(11):2720. doi: 10.3390/cancers13112720
21. Das SK, Yang DJ, Wang JL, Zhang C, Yang HF. Non-Gaussian diffusion imaging for malignant and benign pulmonary nodule differentiation: a preliminary study. Acta Radiol (2017) 58(1):19–26. doi: 10.1177/0284185116639763
22. Le Bihan D. Intravoxel incoherent motion perfusion MR imaging: A wake-up call. Radiology (2008) 249(3):748–52. doi: 10.1148/radiol.2493081301
23. Yuan M, Zhang YD, Zhu C, Yu TF, Shi HB, Shi ZF, et al. Comparison of intravoxel incoherent motion diffusion-weighted MR imaging with dynamic contrast-enhanced MRI for differentiating lung cancer from benign solitary pulmonary lesions. J Magn Reson Imaging (2016) 43(3):669–79. doi: 10.1002/jmri.25018
24. Wan Q, Deng YS, Zhou JX, Yu YD, Bao YY, Lei Q, et al. Intravoxel incoherent motion diffusion-weighted MR imaging in assessing and characterizing solitary pulmonary lesions. Sci Rep (2017) 7:43257. doi: 10.1038/srep43257
25. Wang LL, Lin J, Liu K, Chen CZ, Liu H, Lv P, et al. Intravoxel incoherent motion diffusion-weighted MR imaging in differentiation of lung cancer from obstructive lung consolidation: comparison and correlation with pharmacokinetic analysis from dynamic contrast-enhanced MR imaging. Eur Radiol (2014) 24(8):1914–22. doi: 10.1007/s00330-014-3176-z
26. Zhou SC, Wang YJ, Ai T, Huang L, Zhu TT, Zhu WZ, et al. Diagnosis of solitary pulmonary lesions with intravoxel incoherent motion diffusion-weighted MRI and semi-quantitative dynamic contrast-enhanced MRI. Clin Radiol (2019) 74(5):409.e407–409.e416. doi: 10.1016/j.crad.2018.12.022
27. Yi CA, Lee KS, Kim EA, Han J, Kim H, Kwon OJ, et al. Solitary pulmonary nodules: dynamic enhanced multi-detector row CT study and comparison with vascular endothelial growth factor and microvessel density. Radiology (2004) 233(1):191–9. doi: 10.1148/radiol.2331031535
Keywords: diffusion-weighted imaging, intravoxel incoherent motion, diffusion kurtosis imaging, magnetic resonance imaging, lung lesions, histogram analysis
Citation: Li J, Wu B, Huang Z, Zhao Y, Zhao S, Guo S, Xu S, Wang X, Tian T, Wang Z and Zhou J (2023) Whole-lesion histogram analysis of multiple diffusion metrics for differentiating lung cancer from inflammatory lesions. Front. Oncol. 12:1082454. doi: 10.3389/fonc.2022.1082454
Received: 28 October 2022; Accepted: 21 December 2022;
Published: 18 January 2023.
Edited by:
Manisha Aggarwal, Johns Hopkins University, United StatesReviewed by:
Liangliang Meng, Chinese People’s Liberation Army General Hospital, ChinaCopyright © 2023 Li, Wu, Huang, Zhao, Zhao, Guo, Xu, Wang, Tian, Wang and Zhou. This is an open-access article distributed under the terms of the Creative Commons Attribution License (CC BY). The use, distribution or reproduction in other forums is permitted, provided the original author(s) and the copyright owner(s) are credited and that the original publication in this journal is cited, in accordance with accepted academic practice. No use, distribution or reproduction is permitted which does not comply with these terms.
*Correspondence: Zhixue Wang, V2FnWmhpWHVlOTE3QDEyNi5jb20=; Jun Zhou, emhvdWp1bnpuQDEyNi5jb20=
†These authors have contributed equally to this work and share first authorship
Disclaimer: All claims expressed in this article are solely those of the authors and do not necessarily represent those of their affiliated organizations, or those of the publisher, the editors and the reviewers. Any product that may be evaluated in this article or claim that may be made by its manufacturer is not guaranteed or endorsed by the publisher.
Research integrity at Frontiers
Learn more about the work of our research integrity team to safeguard the quality of each article we publish.