Erratum: Analysis of the regulatory mechanisms of prognostic immune factors in thyroid cancer
- 1Department of Pediatric Surgery, Jingzhou Central Hospital, Jingzhou Hospital Affiliated to Yangtze University, Jingzhou, Hubei, China
- 2Department of Anesthesiology, Jingzhou Central Hospital, Jingzhou Hospital Affiliated to Yangtze University, Jingzhou, Hubei, China
- 3Department of Clinical Nutrition, Sinopharm Dongfeng General Hospital, Hubei University of Medicine, Shiyan, Hubei, China
Objective: To explore the regulatory mechanism of immune prognostic factors in thyroid cancer.
Methods: Based on the TCGA database and GEO database, this study used bioinformatics methods to study the potential regulatory mechanism of thyroid cancer prognosis, analyzed the differentially expressed genes and differential miRNAs between thyroid cancer and normal paracancerous tissues by R software, and constructed lasso risk factors. The immune prognostic factors of thyroid cancer were obtained from the model, and the miRDB website was used to predict the possibility of differential miRNA target binding of the immune prognostic factors and correlation analysis was performed, and finally verified by cell experiments.
Results: There were 1413 differentially expressed genes between thyroid cancer and normal paracancerous tissues, among which 21 immune-related genes were prognostic factors with significant differences in expression; lasso risk model obtained AKAP12, APOC1, TIMP3, ADAMTS9, ANK2, HTRA3, SYNDIG1 , ADAMTS5 and DACT1 were nine prognostic factors. A total of 58 differential miRNAs were found in thyroid cancer tissues and non-cancerous tissues. The possibility of differential miRNA targeting and binding of immune prognostic factors on the miRDB website and cell experiments was analyzed.
Conclusions: The potential miRNA regulatory mechanism of immune prognostic factors in thyroid cancer has been explored.
Introduction
Thyroid cancer is a common endocrine malignancy (1, 2). Although THCA has a low mortality rate and a relatively good prognosis, the existing therapeutic modalities for locally advanced or recurrent metastatic THCA are still ineffective in improving the prognosis of patients. Therefore, new therapeutic modalities targeting the molecular mechanisms of thyroid carcinogenesis and progression are still being explored.
Recent studies have revealed that normal cells must possess the intrinsic characteristics of tumor cells to develop into cancer cells; most importantly, tumor cells can evade antitumor immune responses and form tumor foci (3). Antitumor immunity is an essential mechanism for the host’s inhibition of tumorigenesis. However, tumor cells can evade the body’s immune attack, survive, and proliferate to form tumor foci (4, 5). Tumor cells can play a crucial role in disease development through different immune mechanisms. Immune-related genes have been shown to play a role in cancer development (6–8) and, in some studies, can also can predict the prognosis of cancer patients and as therapeutic targets (9–12). In addition to being an effective prognostic biomarker by constructing the immune prognostic features of cancer using bioinformatics, it is also a promising and novel therapeutic target. Wang et al. (13) found that an immune-related prognostic signature consisting of SLC10A2, FGF2, CCL28, NDRG1, ESM1, UCN, UTS2, and TRDC could effectively predict 3- and 5-year overall survival rates. Heterogeneous expression of immune-related genes can be used as a prognostic factor, but the regulatory mechanism for the heterogeneous expression of immune factors is unknown.
Micro ribonucleic acids (miRNAs) are conserved endogenous short-stranded noncoding ribonucleic acid (RNA) molecules consisting of 21-23 nucleotides. miRNAs have been shown to target multiple messenger RNAs (mRNAs) and are involved in almost every biological process (14, 15). In recent years, studies targeting the role of miRNAs in cancer based on miRNA expression profiles have increased annually. Many miRNAs are down- or up-regulated in human cancers and act as oncogenic or intra-cancer suppressors (16). In ovarian cancer, many miRNAs regulate the epithelial-mesenchymal transition program (17). At the level of molecular mechanisms, miR-125a and miR-125b can regulate immune cell development and function, thus acting as tumor suppressors or promoters (18).
In this study, a total of nine prognostic immune factors, AKAP12, APOC1, TIMP3, ADAMTS9, ANK2, HTRA3, SYNDIG1, ADAMTS5, and DACT1, were obtained by screening differentially-expressed genes and differential miRNAs using LASSO analysis and analyzing the differential miRNA. The target-binding relationship between differential miRNAs and prognostic immune factors was also analyzed. We validated them using correlation analysis to find the regulatory mechanism of immune prognostic factors in THCA.
Materials and methods
Data sources
The data sources for this study were the TCGA and GEO databases. Those databases included RNA sequencing (RNAseq) data and clinicopathological information from 510 THCA samples; mRNA data from 58 normal tissues adjacent to the cancer were obtained. The tissue from 29 THCA patients and five non-THCA patients was obtained from the GEO database GSE103996 dataset miRNA expression profile. Immune-related genes was got from the Immunology Database and Analysis Portal database (https://www.immport.org).
Differential expression analysis
Differential expression genes(DEGs) and differentially-expressed miRNAs of THCA tissues and paraneoplastic tissues were analyzed differentially using the R package limma package, where differentially-expressed genes were screened at p < 0.05, |log2FC|>1, and differentially-expressed miRNAs were screened at p < 0.05, |log2FC|>1, and represented in volcano and heat maps using Venn diagrams to take the intersection of the screened DEGs with immune-related genes.
Prognostic analysis
The transcriptional expression profile data and information of thyroid cancer patients obtained from the TCGA database were plotted using Kaplan–Meier curves with R software. P-values and hazard ratios with 95% confidence intervals (CI) were derived using a log-rank test and univariate Cox regression; the data were presented as forest plots.
The prognostic signature model
The relationship between prognostic immune-related gene expression and overall survival (OS) was assessed using LASSO COX analysis. the prognostic risk prediction model for THCA was based on the LASSO risk score calculation formula. patients with THCA were divided into high-risk and low-risk groups. KM curves were plotted to compare OS in the high-risk and low-risk fractions. receiver operating characteristic survival analysis was performed using the R package SURVIVAL; decision curve analysis was performed using the risk model decision analysis (rmda) package. The relationship between risk score models and tumor immune infiltrating cells was also observed.
Single gene expression analysis
RNAseq data in level 3 HTSeq-FPKM format or miRNAseq data in level 3 BCGSC miRNA Profiling from the TCGA THCA (thyroid cancer) project. The miRNAseq data in fragments per kilobase per million (FPKM) or reads per million mapped reads (RPM) format was log2-transformed; data significance was p < 0.05; *, p < 0.05; **, p < 0.01; ***, and p < 0.001.
Target gene miRNA prediction and correlation analysis
Target gene miRNA prediction was performed using the microRNA target prediction database (miRDB) website (http://mirdb.org/). Transcriptional profiles and miRNA expression profile data were obtained from the TCGA database. Correlation plots between the two were implemented using the R ggstatsplot package. Spearman’s correlation analysis can perform correlations between quantitative variables, which were statistically significant with a p-value less than 0.05.
Cell culture and RT-qPCR
RT-qPCR assays for human normal thyroid cell line Nthy-ori 3-1 and human THCA cell line ACT-1 were purchased from the American Type Culture Collection. All cell lines were cultured in RPMI-1640 containing 10% fetal bovine serum (FBS, Gibco), 1% penicillin/streptomycin, and maintained in a 37°C, 5% CO2 incubator. Total RNA was extracted from each group of cells using TRIzol reagent (Invitrogen, Carlsbad, CA) following the manufacturer’s guidelines. RNA was synthesized into cDNA using Primescript RT Reagent Kit (Takara, Dalian, China). cDNA amplification and quantification were performed on an Applied Biosystems 7500 instrument using SYBR Green mix (Takara). The relative expression of mRNA was calculated using the 2-ΔΔCt method, and the experiment was repeated three times independently for each sample. miRNA was used as an internal reference for U6, and glyceraldehyde-3-phosphate dehydrogenase (GAPDH) was used as an internal reference for genes. The PCR primers are shown in Table 1.
Statistical analysis
Cellular tests were performed using the IBM Statistical Package for the Social Sciences (SPSS) 24.0 and GraphPad Prism8.0.1 software to process the data. Normally distributed measures were expressed as (x ± s), and an independent samples t-test was used to compare the two parts. A one-way analysis of variance can comparison between multiple parts, and Tukey’s multiple comparisons test was used for post hoc tests. P was a two-sided test, and differences were statistically significant at p < 0.05.
Results
Immunoprognostic analysis of differentially significant genes in thyroid cancer
We obtained transcriptomic data from 510 thyroid cancer patients and 58 paraneoplastic tissues from the TCGA database for differential gene analysis; a total of 676 up-regulated genes and 737 downregulated genes were obtained by |log2FC|>1 with p < 0.05. Figure 1A shows the volcano plot, and Figure 1B shows the heat map. We then obtained 264 identical genes by taking the intersection of the DEGs and immune gene sets (Figure 1C). We performed a prognostic analysis of these 264 genes based on individual gene expression using both one-way Cox and log-rank tests. We obtained a total of FIBIN, LT8D2, GHR, ANTXR1, BMP2, FMOD, CDH3, TREM2, AKAP12, APOC1, ANXA1, TIMP3, ADAMTS9 ANK2, HTRA3, TNFRSF12A, SYNDIG1, ASXL3, SPOCK2, ADAMTS5, and DACT1 21 prognostic genes with significantly different immune-related expression (Figure 1D).
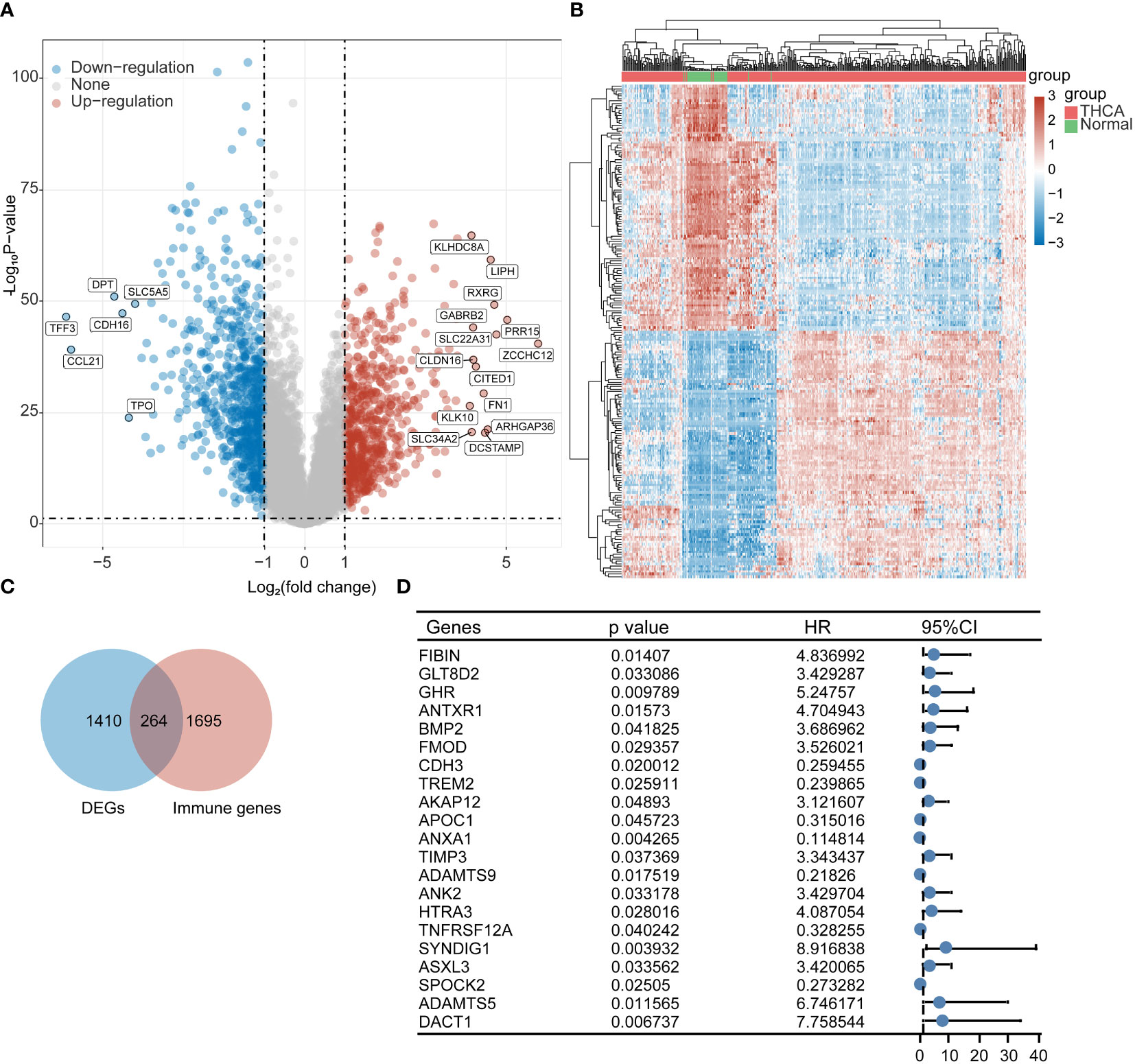
Figure 1 Screening of immune-related prognostic genes in DEGs. (A) Volcano plot, (B) Heat map, (C) Wayne plot, and (D) 21 differential genes with prognostic value.
Immunogenic prognostic model construction
To further explore the clinical value of significant differences in immune-related expression, prognostic features were established for 21 immune-related genes based on LASSO Cox analysis (Figures 2A, B). Using risk score Riskscore = (0.0819)*FIBIN+(0.0058)*AKAP12+(-0.0814)*APOC1+(0.1218)*TIMP3+(-0.1791)*ADAMTS9+(0.0335)*ANK2+(0.3115)*HTRA3+(0.2709)*SYNDIG1+(0.0237)*ADAMTS5+(0.1317)*DACT1, THCA patients were divided into high and low-risk groups (Figure 2C); Survival status is shown in Figure 6D. The figure shows the difference in survival between the high and low-risk parts (p = 0.00613). Prognostic survival was predicted for 1, 3, and 5 years; the model showed good sensitivity (Figure 6E). In summary, nine prognostic factors were obtained for AKAP12, APOC1, TIMP3, ADAMTS9, ANK2, HTRA3, SYNDIG1, ADAMTS5, and DACT1.
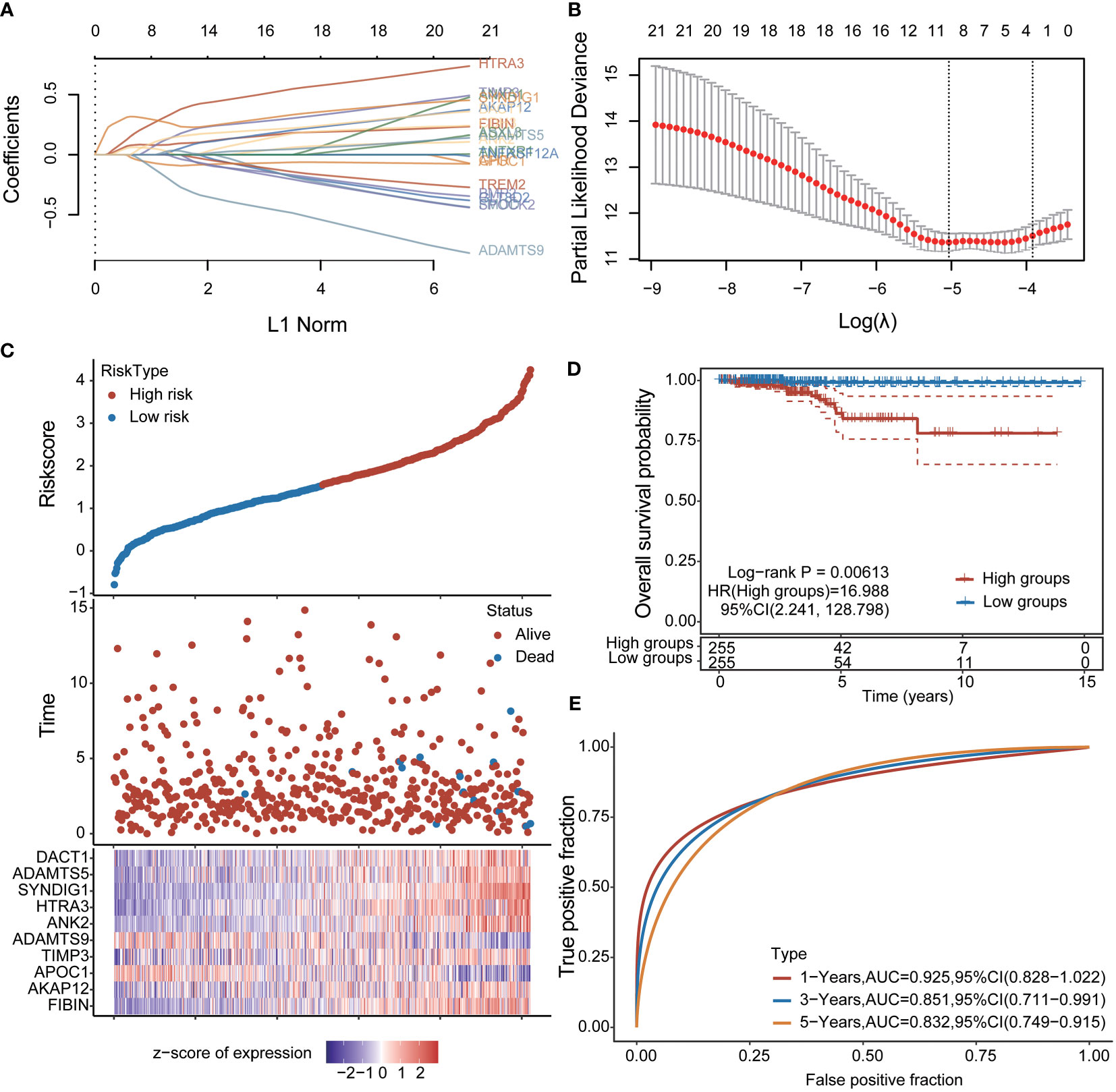
Figure 2 Construction of prognostic features of immune-related genes in thyroid cancer (A, B) distribution of LASSO coefficients of 21 pivot genes, the adjustment parameter λ.min= 0.0065 is obtained, and the vertical black dotted line in B defines the optimal λ value; (C) Thyroid cancer Risk score (Riskscore) distribution of patients, survival status and duration of thyroid cancer patients (D) KM survival curves of high and low-risk groups; (E): ROC curves of 1 year, 3 years and 5 years.
Significant differences exist in miRNAs regulating the heterogeneous expression of prognostic factors
To understand the heterogeneous expression mechanism of immune prognostic factors in thyroid cancer and adjacent tissues, we introduced the miRNA transcriptome information of thyroid cancer and non-cancer tissues into the GSE103996 dataset of the GEO database, and obtained 58 differential miRNAs through analysis. Among them, 21 were up-regulated and 37 were down-regulated. The heat map is shown in Figure 3B. We then screened for miRNAs that bind to postimmune factors, and their targeting relationships are shown in the Sankey diagram in Figure 3C.
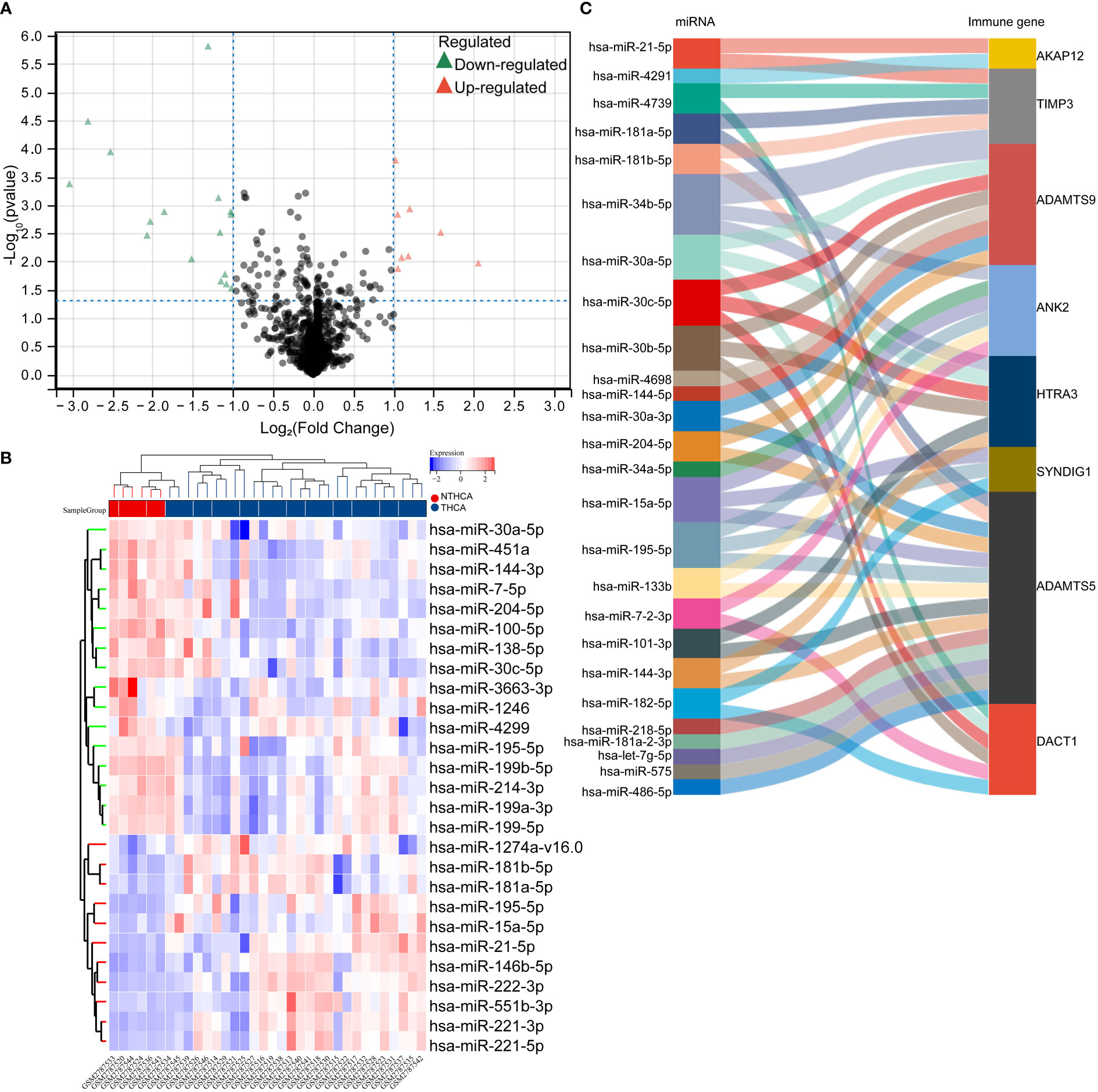
Figure 3 Targeted regulation of differentially significant miRNAs that regulate heterogeneous expression of prognostic factors. (A, B) volcano plot of differentially significantly expressed miRNAs in the GSE103996 dataset, heat map; (C) Sankey plot of miRNAs that target predicted binding to prognostic factors.
Targeted binding miRNA expression to prognostic factors and prognosis
We verified the expression of miRNAs that bind to prognostic immune factors in thyroid cancer tissues and paracancerous tissues in the THCAGA database as follows: miR-21-5p, miR-181a-5p, miR-181b-5p, miR-34a-5p, miR-15-5p, miR-182-5p, and miR-181-2-3p were significantly up-regulated tissues. miR-34b-5p, miR-30c-5p, miR-34b-5p, miR-144-5p, miR-30a-3p, miR-204-5p, miR-195-5p, miR-133b, miR-7-2-3p, miR-101-3p, miR-144-3p, miR-218-5p, let-7g-5p, and miR-486-5p were significantly downregulated in tumor tissues. miR-4793, miR-4698, and miR-575 were not significantly changed (Figures 4A–Y).
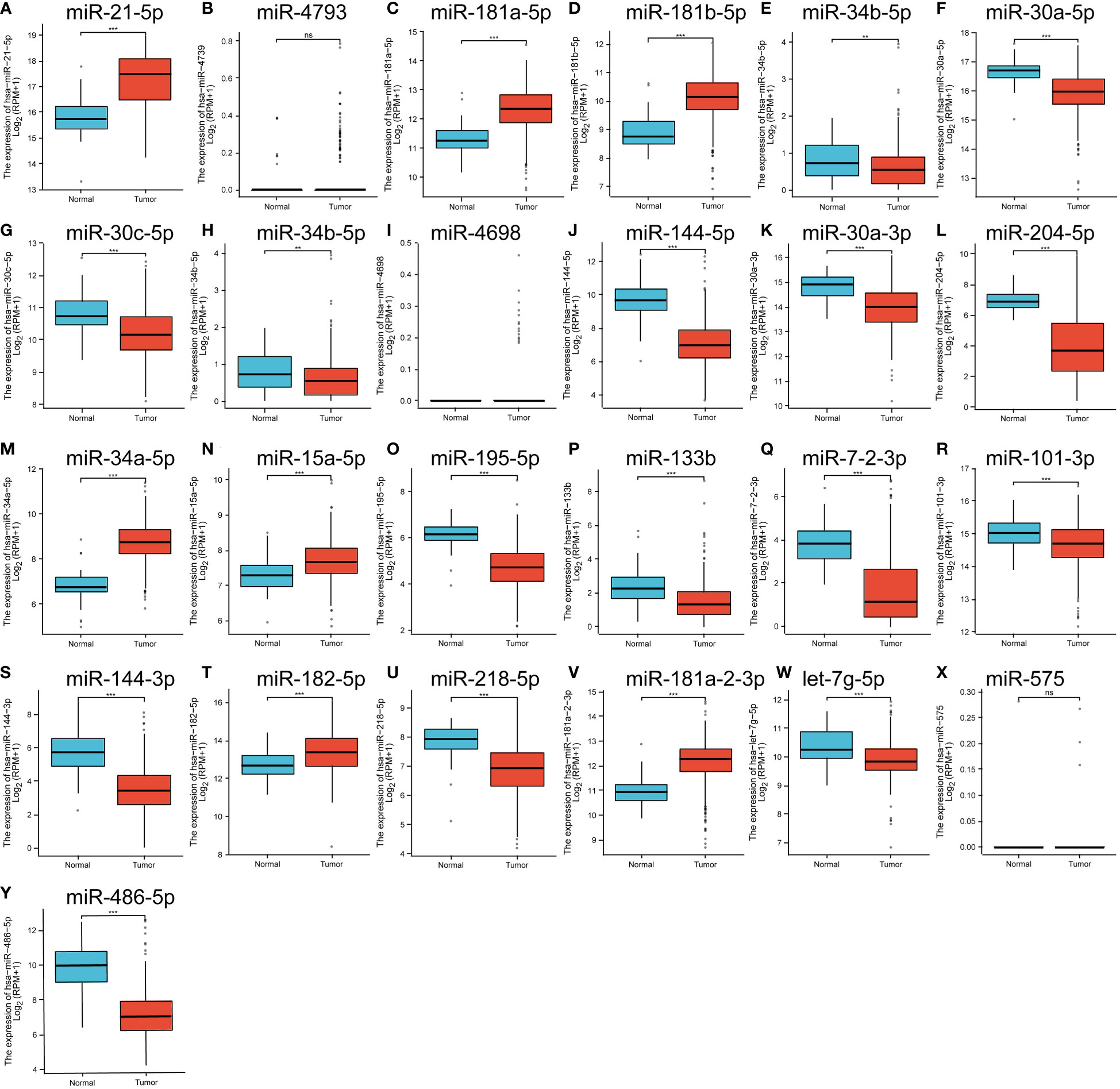
Figure 4 (A–Y) is the expression of significantly different miRNAs in the GEO database that have a targeted binding relationship with the prognostic factors in the TCGA database. ns means no statistical significance, ** means p<0.01, *** means p<0.00.1.
Subsequently, we verified the prognostic impact of the expression profiles of these miRNAs on THCA. The results showed that among these significantly different miRNAs, only miR-181-2-3p significantly impacted the prognosis of THCA (Figures 5A–V).
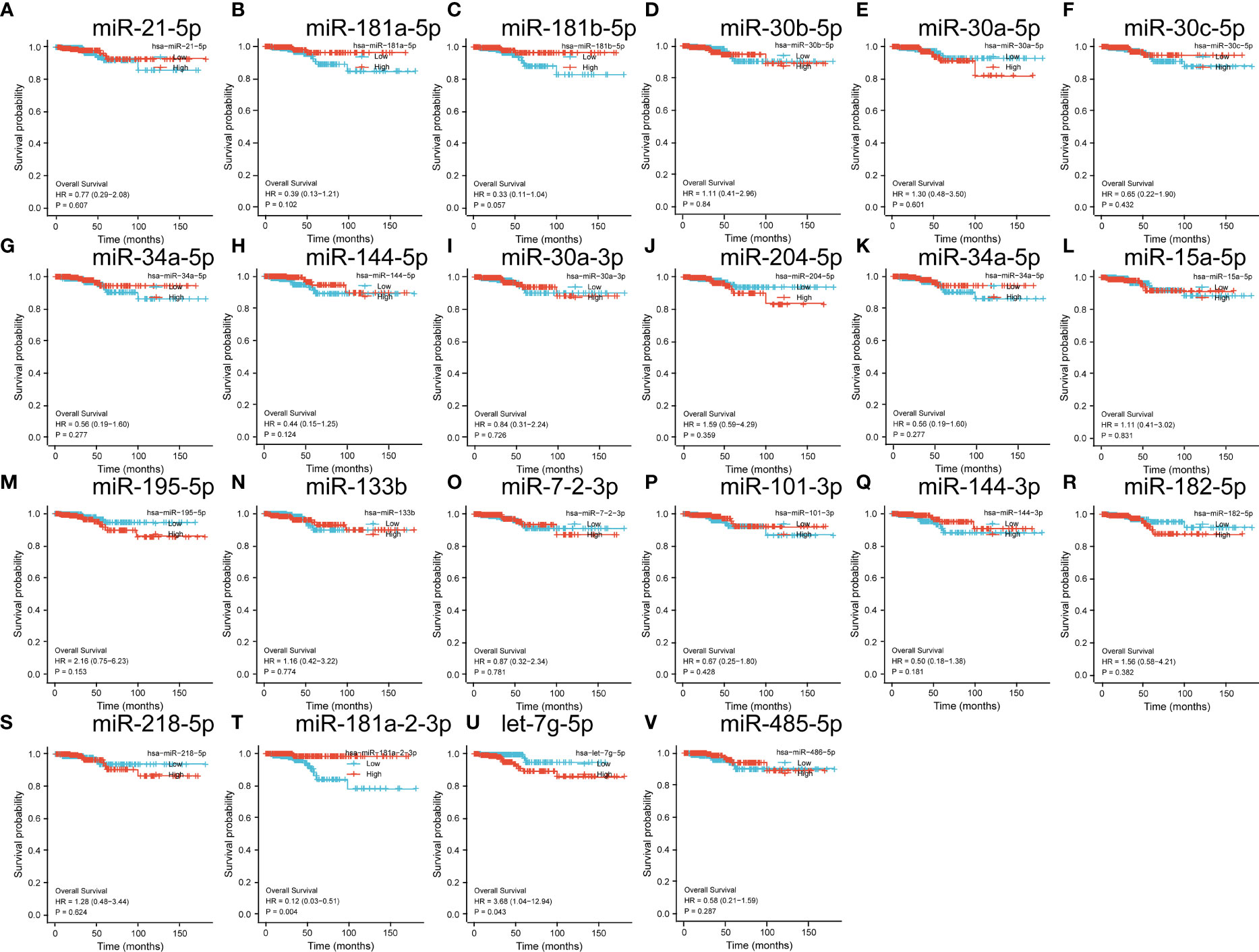
Figure 5 (A–V) is a significantly different miRNA in the GEO database that has a target binding relationship with a prognostic factor and the impact on the prognosis of thyroid cancer.
Correlation between miRNA and prognostic immune factors
To further validate the mechanisms of miRNA regulation involved in the expression of prognostic factors, we analyzed the targeted regulatory relationships between miRNAs and prognostic factors. The results are shown in Figure 6. The figure shows the following: ADMTS5 was significantly and negatively correlated with miR-15a-5p, miR-101-3p, miR-181a-5p, and miR-181b-5p. ADMTS9 was significantly and negatively correlated with miR-30a-5p, miR-30a-3p, miR-30c-5p, and miR-144-5p. AKAP12 was significantly and negatively correlated with miR-21-5p. ANK2 was significantly and negatively correlated with miR-15a-5p and miR-34a-5p. DACT1 was significantly and negatively associated with miR-182-5p. SYNDIG1 was significantly and negatively related to miR-15a-5p and miR-182-5p. TIMP3 was significantly and negatively related to miR-21-5p, miR-181a-5p, and miR-181b-5p.
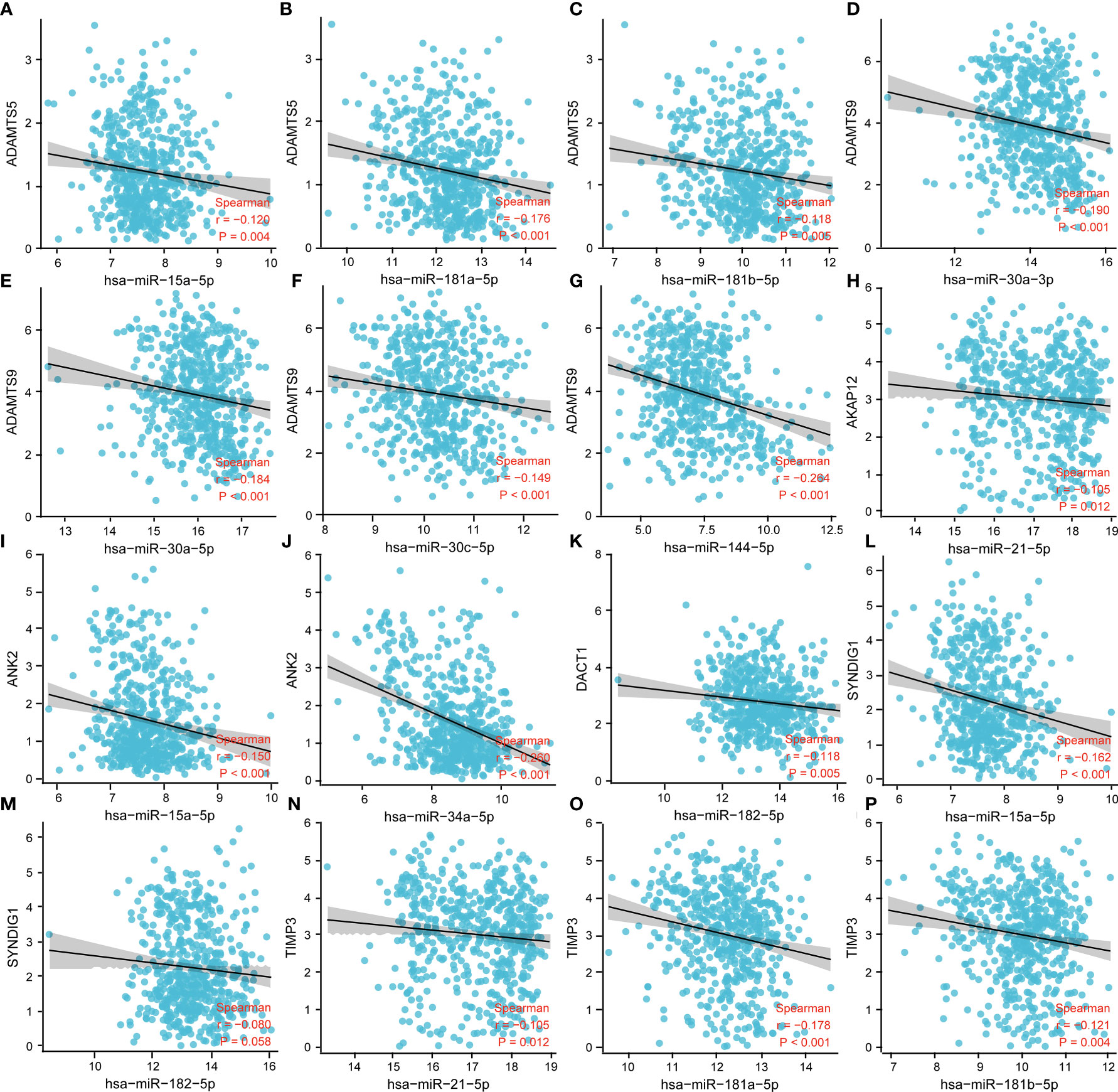
Figure 6 Correlation analysis between miRNA and prognostic immune factors. (A–C) ADMTS5 with miR-15a-5p, miR-101-3p, miR-181a-5p, miR-181b-5p; (D–G) ADMTS9 with miR-30a-5p, miR-30a-3p, miR-30c-5p, miR-144-5p; (H) AKAP12 with miR-21-5p. (I, J) ANK2 with miR-15a-5p, miR-34a-5p; (K) DACT1 with miR-182-5p; (L, M) SYNDIG1 with miR-15a-5p, miR-182-5p; and (N–P) TIMP3 with miR-21-5p, miR-181a-5p, and miR-181b-5p. P < 0.05 was statistically significant.
Correlation of experimental cellular miRNAs with target genes
First, we verified the expression of 9 immune-related prognostic factors, AKAP12, APOC1, TIMP3, ADAMTS9, ANK2, HTRA3, SYNDIG1, ADAMTS5 and DACT1, in 11 thyroid cancer cell lines in the CCLE database. Eleven thyroid cancer cell lines expressed to varying degrees (Figures 7A–I). We verified the expression of immunoprognostic factors in the cells, as shown in Figure 8A. Compared with the normal human thyroid cell line Nthy-ori 3-1, the human THCA cell line ACT-1 showed significant upregulation of ADAMTS9, APOC1, and miR-15a-5p. AKAP12, TIMP3, ANK2, HTRA3, SYNDIG1, ADAMTS5, DACT1, miR-30c-5p, and miR-34b-5p showed significant down-regulation (Figure 7A). We then compared the correlation of miR-15a-5p, miR-30c-5p, and miR-34b-5p with their target genes and found that miR-15a-5p showed a significant negative correlation with ADAMTS5, ANK2, and SYNDIG1. miR-30c-5p and miR-34b-5p showed a significant negative correlation with ADAMTS9 (Figure 8B).
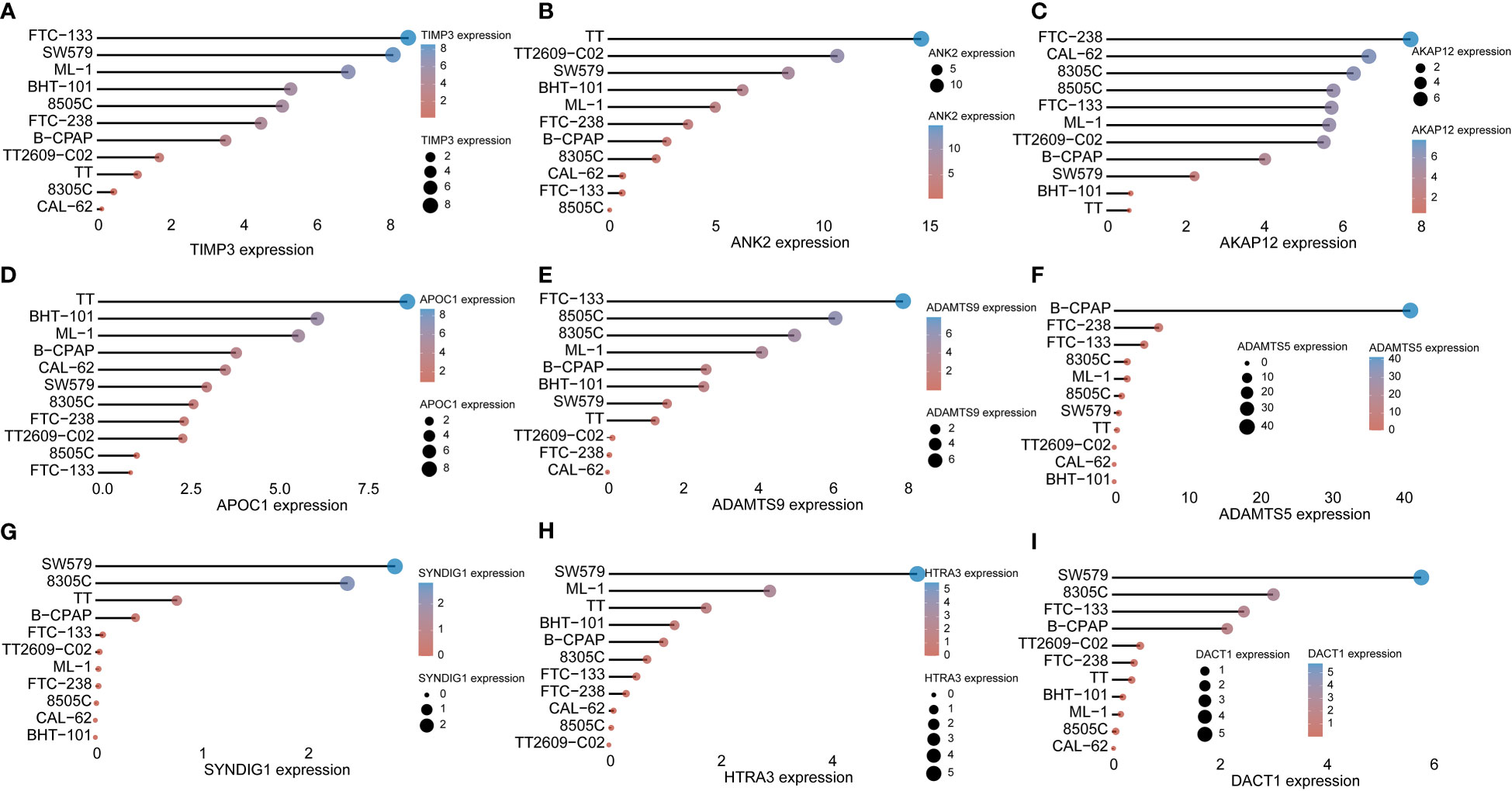
Figure 7 Immune prognostic factors expressed in CCLE data Verification (A-I) AKAP12, APOC1, TIMP3, ADAMTS9, ANK2, HTRA3, SYNDIG1, ADAMTS5, and DACT1 Nine immune-related prognostic factors in TT, BHT-101, ML-1, B-CPAP, the expression of 11 thyroid cancer cell lines including CAL-62, SW579, 8305C, FTC-238, TT2609-CO2, 8505C, and FTC-133.
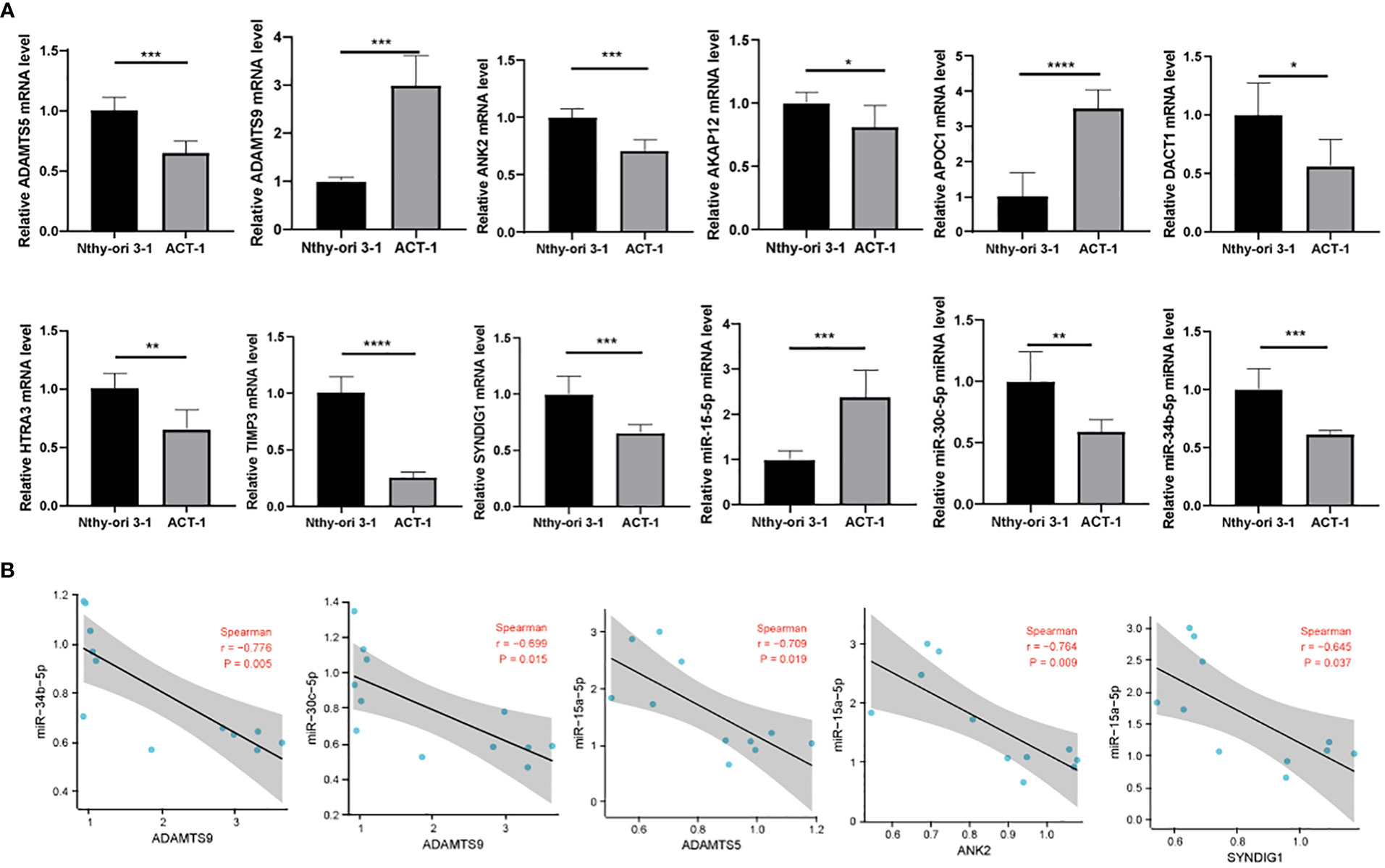
Figure 8 Correlation of experimental cellular miRNAs with target genes. (A) RT-qPCR detection of ADAMTS5, ADAMTS9, ANK2, AKAP12, APOC1, DACT1, HTRA3, TIMP3, SYNDIG1, miR-15a-5p, miR-30c-5p, and miR-34b-5p expression levels; (B) Correlation analysis of miR-15a-5p, miR-30c-5p, and miR-34b-5p and their target genes. * means p <0.05; ** means p < 0.01; *** means p < 0.001; **** means p < 0.0001.
Discussion
Tumor immunity is associated with proto-oncogene activation and tumor immune escape; therefore, understanding tumor-associated immune genes is crucial for tumor immunity research. In recent years, THCA immune studies have focused primarily on developing immune prognostic models (19–21), and less research has been involved in the regulatory mechanisms of prognostic immune factors. This study mainly analyzed the relationship between immune prognostic factors and miRNAs in THCA. We provided a theoretical basis for further understanding of the immune gene regulation mechanism of THCA and a reference for assessing the prognosis of THCA by analyzing the correlation between the two.
This study analyzed the relationship between immune genes and prognosis using THCAGA and immunoinformatics databases. Twenty-one genes with significant differences in the immune prognosis of THCA were screened, and nine prognostic immune factors were identified by constructing a LASSO risk model (AKAP12, APOC1, TIMP3, ADAMTS9, ANK2, HTRA3, SYNDIG1, ADAMTS5, and DACT1). This risk model predicted OS after five years with high accuracy (AUC = 0.832).
The nine immune genes in the model have biological characteristics and are related to the clinical prognosis of tumor patients. AKAP12 is an A-kinase scaffold protein with a characteristic binding structural domain of the protein kinase A regulatory subunit. AKAP12 deletion is associated with increased cancer susceptibility (22). AKAP1 expression is associated with APOC1 overexpression, promotes tumor progression, and has a poorer prognosis for patient survival (23). TIMP3 is a metalloproteinase that belongs to the TIMP family. It has a high affinity for proteoglycans in the ECM and its broadest range of substrates, including all MMPs, ADAMs (a disintegrin and metalloproteinase), and ADAMTSs (ADAMs with thrombospondin motifs) (24).
TIMP-3 has been shown to have anti-metastatic effects by inhibiting matrix metalloproteinases and members of the ADAM family and down-regulating angiogenesis (25). ADAMTS5 and ADAMTS9 belong to the ADAMTS (disintegrin-like and metalloproteinase with platelet abasicin motif) proteins. They are extracellular zinc metalloproteinases that play an essential role in extracellular matrix assembly and degradation, connective tissue structure, angiogenesis generation, and cell migration (26). ADAMTSL5 plays a role in maintaining the function of critical oncogenic signaling pathways, suggesting that it may act as a significant regulator of tumorigenicity and drug resistance (27). adamts9 regulates cancer cell growth and metastasis (28) and is associated with patient survival (29).
ANK2 has a high mutation frequency in some cancers (30) and silencing of ANK2 expression reduces the growth and invasion of cancer cell type. HTRA3 has been implicated as a tumor suppressor in cancer progression in several cancer types, HTRA3 expression is negatively correlated with adaptive immune cell abundance (T helper cell 17 cells) and positively correlated with innate immune cells (natural killer cells, macrophages, etc.); abundance is positively correlated (31). SYNDIG1 is a prognostic immune factor in diffuse large B-cell lymphoma and breast cancer (32, 33). DACT1 belongs to the DACT (Disheveled-associated antagonist of β-catenin) family and is a methylation biomarker for DACT1 in esophageal squamous cell carcinoma (34).
The above data suggest that the nine immune genes in our constructed risk model can exist as prognostic factors in some tumors, reflecting the accuracy of our constructed risk model from the side.
In many studies, the regulatory mechanisms of prognostic factors remain unclear. Based on this starting point, this study analyzed the correlation between immune prognostic factors and miRNAs through TCGA and GEO databases to find the miRNA mechanism of immune prognostic factors in thyroid cancer species.
It has been shown that miRNAs can regulate the target genes involved in the prognosis of tumor patients. For example, in nasopharyngeal carcinoma, the negative correlation between miRNA-19a-3p and PDCD5 expression levels, miRNA-19a-3p targeting to suppress PDCD5 expression, miRNA-19a-3p levels correlated with N classification and clinical stage of nasopharyngeal carcinoma patients, and PDCD5 levels correlated with T classification, pathological grade, and clinical stage. Survival analysis showed that the expression of high levels of miRNA-19a-3p or low levels of PDCD5 had a poorer prognosis in patients with nasopharyngeal carcinoma (35).
Our study identified 16 groups of miRNAs that showed negative correlations with prognostic immune factors, with specific target-binding relationships confirmed in other cancers. For example, TIMP3 was downregulated in cervical cancer and was related to poor prognosis in cervical cancer patients, and miR-21-5p target binding to TIMP3 modulated the development of cervical cancer (36). In gastric cancer, the miR-21-5p binding relationship with TIMP3 is related to drug resistance in gastric cancer (37). In prostate cancer, the miR-181b-5p-TIMP3 axis regulates prostate cancer proliferation, migration, and invasive ability (38).
This study also found that by constructing a miRNA regulatory network with certain selected candidate factors (39–42), we could provide a new approach to cancer diagnosis and treatment. The miRNA regulatory network of prognostic immune factors was screened. We also validated at the cellular level that miR-15a-5p showed a significant negative correlation with ADAMTS5, ANK2, and SYNDIG1. miR-30c-5p and miR-34b-5p showed a significant negative correlation with ADAMTS9, but no functional gene. However, no further validation of the functional role of the genes was performed, which will be the direction of our later research.
In summary, nine immunoprognostic factors (AKAP12, APOC1, TIMP3, ADAMTS9, ANK2, HTRA3, SYNDIG1, ADAMTS5, and DACT1) were screened as biomolecular markers for predicting THCA. A prognostic assessment model and risk score system were constructed to predict THCA 5-year OS rates with high and low-risk groups using risk scores. The 5-year OS rate of THCA was predicted with high accuracy. By constructing a prognostic immune factor miRNA regulatory network, we can provide a theoretical basis for the prognostic immune regulatory mechanism of THCA.
Data availability statement
The original contributions presented in the study are included in the article/supplementary material. Further inquiries can be directed to the corresponding author.
Author contributions
YT writing the article, writing the revision of article, collect data. TX writing the article, designing the methods, analysis and interpretation. XS writing the article, writing the revision of article, designing the methodology. All authors contributed to the article and approved the submitted version.
Conflict of interest
The authors declare that the research was conducted in the absence of any commercial or financial relationships that could be construed as a potential conflict of interest.
Publisher’s note
All claims expressed in this article are solely those of the authors and do not necessarily represent those of their affiliated organizations, or those of the publisher, the editors and the reviewers. Any product that may be evaluated in this article, or claim that may be made by its manufacturer, is not guaranteed or endorsed by the publisher.
References
1. Bray F, Ferlay J, Soerjomataram I, Siegel RL, Torre LA, Jemal A, et al. Global cancer statistics 2018: GLOBOCAN estimates of incidence and mortality worldwide for 36 cancers in 185 countries. CA Cancer J Clin (2018) 68(6):394–424. doi: 10.3322/caac.21492
2. Franchini F, Palatucci G, Colao A, Ungaro P, Macchia PE, Nettore IC, et al. Obesity and thyroid cancer risk: An update. Int J Environ Res Public Health (2022) 19(3):1116. doi: 10.3390/ijerph19031116
3. Pulendran B, Davis MM. The science and medicine of human immunology. Science (2020) 369(6511). doi: 10.1126/science.aay4014
4. St Paul M, Ohashi PS. The roles of CD8(+) T cell subsets in antitumor immunity. Trends Cell Biol (2020) 30(9):695–704. doi: 10.1016/j.tcb.2020.06.003
5. Ikeda H, Togashi Y. Aging, cancer, and antitumor immunity. Int J Clin Oncol (2022) 27(2):316–22. doi: 10.1007/s10147-021-01913-z
6. Oliva M, Spreafico A, Taberna M, Alemany L, Coburn B, Mesia R, et al. Immune biomarkers of response to immune-checkpoint inhibitors in head and neck squamous cell carcinoma. Ann Oncol (2019) 30(1):57–67. doi: 10.1093/annonc/mdy507
7. Akbulut H. Immune gene therapy of cancer. Turk J Med Sci (2020) 50(SI-2):1679–90. doi: 10.3906/sag-2005-327
8. Hogg SJ, Beavis PA, Dawson MA, Johnstone RW. Targeting the epigenetic regulation of antitumour immunity. Nat Rev Drug Discovery (2020) 19(11):776–800. doi: 10.1038/s41573-020-0077-5
9. Sun S, Guo W, Wang Z, Wang X, Zhang G, Zhang H, et al. Development and validation of an immune-related prognostic signature in lung adenocarcinoma. Cancer Med (2020) 9(16):5960–75. doi: 10.1002/cam4.3240
10. Shen S, Wang G, Zhang R, Zhao Y, Yu H, Wei Y, et al. Development and validation of an immune gene-set based prognostic signature in ovarian cancer. EBioMedicine (2019) 40:318–26. doi: 10.1016/j.ebiom.2018.12.054
11. Kong Y, Feng ZC, Zhang YL, Liu XF, Ma Y, Zhao ZM, et al. Identification of immune-related genes contributing to the development of glioblastoma using weighted gene Co-expression network analysis. Front Immunol (2020) 11:1281. doi: 10.3389/fimmu.2020.01281
12. Xu H, Zhang A, Han X, Li Y, Zhang Z, Song L, et al. ITGB2 as a prognostic indicator and a predictive marker for immunotherapy in gliomas. Cancer Immunol Immunother (2022) 71(3):645–60. doi: 10.1007/s00262-021-03022-2
13. Wang J, Yu S, Chen G, Kang M, Jin X, Huang Y, et al. A novel prognostic signature of immune-related genes for patients with colorectal cancer. J Cell Mol Med (2020) 24(15):8491–504. doi: 10.1111/jcmm.15443
14. Annese T, Tamma R, De Giorgis M, Ribatti D. microRNAs biogenesis, functions and role in tumor angiogenesis. Front Oncol (2020) 10:581007. doi: 10.3389/fonc.2020.581007
15. Chauhan N, Dhasmana A, Jaggi M, Chauhan SC, Yallapu MM. miR-205: A potential biomedicine for cancer therapy. Cells (2020) 9(9):1957. doi: 10.3390/cells9091957
16. Ali Syeda Z, Langden SSS, Munkhzul C, Lee M, Song SJ. Regulatory mechanism of MicroRNA expression in cancer. Int J Mol Sci (2020) 21(5):1723. doi: 10.3390/ijms21051723
17. Ghafouri-Fard S, Shoorei H, Taheri M. miRNA profile in ovarian cancer. Exp Mol Pathol (2020) 113:104381. doi: 10.1016/j.yexmp.2020.104381
18. Wang JK, Wang Z, Li G. MicroRNA-125 in immunity and cancer. Cancer Lett (2019) 454:134–45. doi: 10.1016/j.canlet.2019.04.015
19. Gan X, Guo M, Chen Z, Li Y, Shen F, Feng J, et al. Development and validation of a three-immune-related gene signature prognostic risk model in papillary thyroid carcinoma. J Endocrinol Invest (2021) 44(10):2153–63. doi: 10.1007/s40618-021-01514-7
20. Lin R, Fogarty CE, Ma B, Li H, Ni G, Liu X, et al. Identification of ferroptosis genes in immune infiltration and prognosis in thyroid papillary carcinoma using network analysis. BMC Genomics (2021) 22(1):576. doi: 10.1186/s12864-021-07895-6
21. Qin R, Li C, Wang X, Zhong Z, Sun C. Identification and validation of an immune-related prognostic signature and key gene in papillary thyroid carcinoma. Cancer Cell Int (2021) 21(1):378. doi: 10.1186/s12935-021-02066-9
22. Hayashi M, Nomoto S, Kanda M, Okamura Y, Nishikawa Y, Yamada S, et al. Identification of the a kinase anchor protein 12 (AKAP12) gene as a candidate tumor suppressor of hepatocellular carcinoma. J Surg Oncol (2012) 105(4):381–6. doi: 10.1002/jso.22135
23. Li YL, Wu LW, Zeng LH, Zhang ZY, Wang W, Zhang C, et al. ApoC1 promotes the metastasis of clear cell renal cell carcinoma via activation of STAT3. Oncogene (2020) 39(39):6203–17. doi: 10.1038/s41388-020-01428-3
24. Fan D, Kassiri Z. Biology of tissue inhibitor of metalloproteinase 3 (TIMP3), and its therapeutic implications in cardiovascular pathology. Front Physiol (2020) 11:661. doi: 10.3389/fphys.2020.00661
25. Rai GP, Baird SK. Tissue inhibitor of matrix metalloproteinase-3 has both anti-metastatic and anti-tumourigenic properties. Clin Exp Metastasis (2020) 37(1):69–76. doi: 10.1007/s10585-019-10017-y
26. Mead TJ, Apte SS. ADAMTS proteins in human disorders. Matrix Biol (2018) 71-72:225–39. doi: 10.1016/j.matbio.2018.06.002
27. Arechederra M, Bazai SK, Abdouni A, Sequera C, Mead TJ, Richelme S, et al. ADAMTSL5 is an epigenetically activated gene underlying tumorigenesis and drug resistance in hepatocellular carcinoma. J Hepatol (2021) 74(4):893–906. doi: 10.1016/j.jhep.2020.11.008
28. Chen L, Tang J, Feng Y, Li S, Xiang Q, He X, et al. ADAMTS9 is silenced by epigenetic disruption in colorectal cancer and inhibits cell growth and metastasis by regulating Akt/p53 signaling. Cell Physiol Biochem (2017) 44(4):1370–80. doi: 10.1159/000485534
29. Du W, Wang S, Zhou Q, Li X, Chu J, Chang Z, et al. ADAMTS9 is a functional tumor suppressor through inhibiting AKT/mTOR pathway and associated with poor survival in gastric cancer. Oncogene (2013) 32(28):3319–28. doi: 10.1038/onc.2012.359
30. Jeong S, Park YJ, Yun W, Lee ST, Choi JR, Suh C, et al. Genetic heterogeneity and prognostic impact of recurrent ANK2 and TP53 mutations in mantle cell lymphoma: A multi-centre cohort study. Sci Rep (2020) 10(1):13359. doi: 10.1038/s41598-020-70310-9
31. Ji C, Sun LS, Xing F, Niu N, Gao HL, Dai JW, et al. HTRA3 is a prognostic biomarker and associated with immune infiltrates in gastric cancer. Front Oncol (2020) 10:603480. doi: 10.3389/fonc.2020.603480
32. Mu S, Shi D, Ai L, Fan F, Peng F, Sun C, et al. International prognostic index-based immune prognostic model for diffuse Large b-cell lymphoma. Front Immunol (2021) 12:732006. doi: 10.3389/fimmu.2021.732006
33. Cui Q, Tang J, Zhang D, Kong D, Liao X, Ren J, et al. A prognostic eight-gene expression signature for patients with breast cancer receiving adjuvant chemotherapy. J Cell Biochem (2019). doi: 10.1002/jcb.29550
34. Guo YL, Shan BE, Guo W, Dong ZM, Zhou Z, Shen SP, et al. Aberrant methylation of DACT1 and DACT2 are associated with tumor progression and poor prognosis in esophageal squamous cell carcinoma. J BioMed Sci (2017) 24(1):6. doi: 10.1186/s12929-016-0308-6
35. Zhong JH, Zhong JJ, Shi YN, Hu XM, Xu MJ, Guo Z, et al. Prognostic potentials of miRNA-19a-3p and PDCD5 in nasopharynx carcinoma. Eur Rev Med Pharmacol Sci (2020) 24(21):11114–9. doi: 10.26355/eurrev_202011_23598
36. Gao Y, Zou T, Liang W, Zhang Z, Qie M. Long non-coding RNA HAND2-AS1 delays cervical cancer progression via its regulation on the microRNA-21-5p/TIMP3/VEGFA axis. Cancer Gene Ther (2021) 28(6):619–33. doi: 10.1038/s41417-020-00243-y
37. Chen J, Zhou C, Li J, Xiang X, Zhang L, Deng J, et al. miR215p confers doxorubicin resistance in gastric cancer cells by targeting PTEN and TIMP3. Int J Mol Med (2018) 41(4):1855–66. doi: 10.3892/ijmm.2018.3405
38. Xie X, Sun FK, Huang X, Wang CH, Dai J, Zhao JP, et al. A circular RNA, circSMARCA5, inhibits prostate cancer proliferative, migrative, and invasive capabilities via the miR-181b-5p/miR-17-3p-TIMP3 axis. Aging (Albany NY) (2021) 13(15):19908–19. doi: 10.18632/aging.203408
39. Ran X, Luo J, Zuo C, Huang Y, Sui Y, Cen J, et al. Developing metabolic gene signatures to predict intrahepatic cholangiocarcinoma prognosis and mining a miRNA regulatory network. J Clin Lab Anal (2022) 36(1):e24107. doi: 10.1002/jcla.24107
40. Gao L, Zhou L, Huang X. Identification of novel kinase-transcription factor-mRNA-miRNA regulatory network in nasopharyngeal carcinoma by bioinformatics analysis. Int J Gen Med (2021) 14:7453–69. doi: 10.2147/IJGM.S327657
41. Yang T, Miao X, Bai Z, Tu J, Shen S, Niu H, et al. A novel mRNA-miRNA regulatory Sub-network associated with prognosis of metastatic clear cell renal cell carcinoma. Front Oncol (2020) 10:593601. doi: 10.3389/fonc.2020.593601
Keywords: thyroid cancer, immune gene, prognosis, miRNA, targeted combination
Citation: Tian Y, Xie T and Sun X (2022) Analysis of the regulatory mechanisms of prognostic immune factors in thyroid cancer. Front. Oncol. 12:1059591. doi: 10.3389/fonc.2022.1059591
Received: 01 October 2022; Accepted: 24 November 2022;
Published: 14 December 2022.
Edited by:
Ye Wang, The Second Affiliated Hospital of Medical College of Qingdao University, ChinaReviewed by:
Hao Shen, Huazhong University of Science and Technology, ChinaMeifang Xiao, Hainan Maternal and Child Health Hospital, China
Copyright © 2022 Tian, Xie and Sun. This is an open-access article distributed under the terms of the Creative Commons Attribution License (CC BY). The use, distribution or reproduction in other forums is permitted, provided the original author(s) and the copyright owner(s) are credited and that the original publication in this journal is cited, in accordance with accepted academic practice. No use, distribution or reproduction is permitted which does not comply with these terms.
*Correspondence: Xue Sun, hbsysxue@163.com
†These authors share first authorship