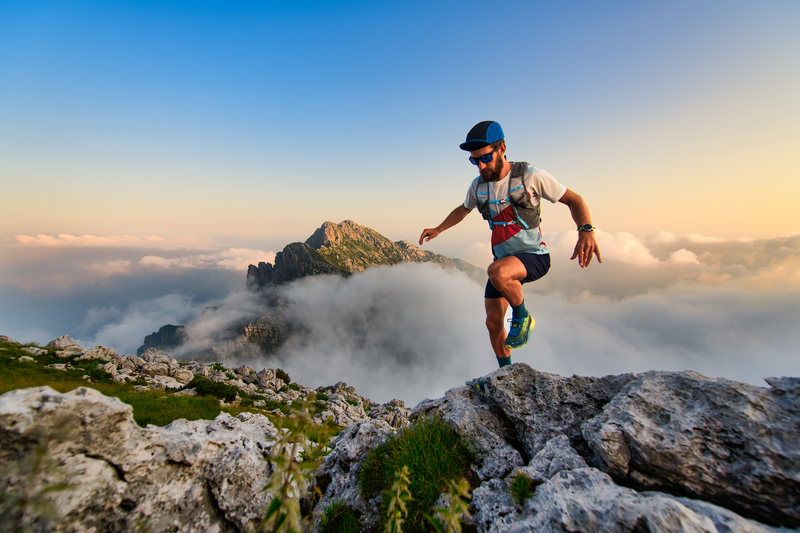
94% of researchers rate our articles as excellent or good
Learn more about the work of our research integrity team to safeguard the quality of each article we publish.
Find out more
ORIGINAL RESEARCH article
Front. Oncol. , 08 December 2022
Sec. Molecular and Cellular Oncology
Volume 12 - 2022 | https://doi.org/10.3389/fonc.2022.1055648
This article is part of the Research Topic Molecular Regulation of Tumor Cells Migration and Metastatic Growth View all 11 articles
Background: Ovarian cancer (OC) is a fatal gynecological tumor with high mortality and poor prognosis. Yet, its molecular mechanism is still not fully explored, and early prognostic markers are still missing. In this study, we assessed carcinogenicity and clinical significance of family with sequence similarity 83 member D (FAM83D) in ovarian cancer by integrating single-cell RNA sequencing (scRNA-seq) and a prognostic model.
Methods: A 10x scRNA-seq analysis was performed on cells from normal ovary and high-grade serous ovarian cancer (HGSOC) tissue. The prognostic model was constructed by Lasso-Cox regression analysis. The biological function of FAM83D on cell growth, invasion, migration, and drug sensitivity was examined in vitro in OC cell lines. Luciferase reporter assay was performed for binding analysis between FAM83D and microRNA-138-5p (miR-138-5p).
Results: Our integrative analysis identified a subset of malignant epithelial cells (C1) with epithelial-mesenchymal transition (EMT) and potential hyperproliferation gene signature. A FAM83D+ malignant epithelial subcluster (FAM83D+ MEC) was associated with cell cycle regulation, apoptosis, DNA repair, and EMT activation. FAM83D resulted as a viable prognostic marker in a prognostic model that efficiently predict the overall survival of OC patients. FAM83D downregulation in SKOV3 and A2780 cells increased cisplatin sensitivity, reducing OC cell proliferation, migration, and invasion. MiR-138-5p was identified to regulate FAM83D’s carcinogenic effect in OC cells.
Conclusions: Our findings highlight the importance of miR-138 -5p/FAM83D/EMT signaling and may provide new insights into therapeutic strategies for OC.
Ovarian cancer (OC) is one of the most common fatal malignant tumors in women. Despite notable progress in surgery, targeted therapy, chemotherapy, and neoadjuvant chemotherapy, the 5-year overall survival rate of OC patients is still unsatisfactory owing to a high rate of chemoresistance, recurrence, and distant metastasis (1). Moreover, accurate prediction of early-stage OC remains challenging due to profound genetic heterogeneity, which limits the reproducible prognostic classifications (2). Therefore, there is a pressing requirement to comprehensively elucidate the complicated molecular properties and heterogeneity of OC development to explore new biomarkers for predicting prognosis.
In recent years, bulk analysis of gene expression patterns has often been used to explore prognostic markers of cancer (3–5). It measures the average expression level of individual genes across, allowing us to understand the differences in gene expression between samples. In addition, the whole-genome analysis of OC by multiple methods, including copy number variation and methylation level of key genes, have also been used to identify operable markers (6). However, there is still a problem of low accuracy of the screened markers in multi-sample validation and therefore cannot be applied in clinical practice. A meta-analysis has shown that the accuracy of most models in the new data set was lower than the validation set provided in their publications (7). As a result, the studies rely on bulk sample analysis, blurring the molecular markers of different cell subsets, thus hindering the identification of the precise molecular mechanism of OC. Fortunately, emerging single-cell technologies could address these limitations by providing powerful ways to probe genetic and functional heterogeneity at the single-cell level (8). The latest single-cell RNA sequencing (scRNA-seq) studies depicted a landscape of OC, advancing our understanding of its molecular mechanisms (9, 10). Still, there are only a few studies on prognostic markers based on scRNA-seq from OC.
Herein, we performed 10x scRNA-seq to analyze the transcriptomic profiles of 13890 cells from the normal ovary and OC tissues. We revealed a cluster of malignant epithelial cells with epithelial-mesenchymal transition (EMT) and potential hyperproliferation gene signature and constructed a robust prediction model including 10-EMT-related genes. Among them, a family with sequence similarity 83 member D (FAM83D+) malignant epithelial cells were significantly associated with multiple carcinogenic pathways. Furthermore, we discovered that FAM83D promotes OC cell progression and cisplatin resistance, and confirmed that miR-138-5p could directly target FAM83D and notably restrain the progress of OC cells by bioinformatics analysis and experiments.
In this study, tissue from one patient with HGSOC and one patient with normal ovary were used for single cell suspension preparation. Fresh specimens were collected at the time of surgical excision under the supervision of a qualified pathologist. Tissues were transported using MACS Tissue Storage Solution (MACS, Cat. no.130-100-008F) on ice to preserve viability. Then we washed the tissues 2–3 times with phosphate buffered saline (PBS; Hyclone, Cat. no. SH30256.01) and minced them on ice. We used the Tumor Dissociation Kit (MACS, Cat. no.130-095-929) to digest the human tissues gently to generate single-cell suspensions. The OC and control tissues were dissociated at 37°C with a shaking speed of 30 rpm for about six minutes. Then, we collected the dissociated cells to digest sufficiently with 0.25% trypsin (Gibco, Cat. no.25200056) for about two minutes. Cell suspensions were filtered using a 40μm nylon cell strainer (Falcon, Cat. no. 352340), and red blood cells were removed by red blood cell lysis buffer (Solarbio). Single-cell suspensions were stained with AO/PI fluorescent dyes (Logos Biosystems, Cat. no. LB F23001) to check viability with LUNA (Logos Biosystems, Cat. no. LUNA-STEM). Then we diluted them to approximately 1 × 106 cells/ml with PBS containing 0.02% BSA for single-cell sequencing. Cells were loaded according to the standard protocol of the Chromium single cell 3′ kit, capturing 5,000 cells to 10,000 cells/chip position (V3 chemistry). Libraries for scRNA-seq were generated using the 10x Genomics Chromium platform and sequenced on an Illumina Novaseq 6000 system. Finally, a gene-barcode matrix containing barcoded cells and gene expression counts was generated.
The matrices of samples were combined and treated using Seurat V3 (11). Low quality cells (< 300 genes/cell, < 3 cells/gene, > 20% mitochondrial genes) were removed. The find clusters function and t-distributed Stochastic Neighbor Embedding (tSNE) function of the Seurat software package were performed for data clustering, and the visualization of major clusters.The estimation algorithm was conducted to calculate each cell’s immune and stromal scores using the estimate package (12).The initial copy number variations (CNV) of each region was inferred by the infer-CNV package (13). The overall average was used as a baseline. The CNV of all cells was counted by the expression level of scRNA-seq data, and the cut-off value was 0.1. Finally, denoising was performed to produce CNV profiles. The single-cell trajectory was studied by the monocle2 package (14). The “differential gene test” function was used to count differentially expressed genes in the pseudo-time of cluster cell transformation. To probe the interactions between different clusters of the malignant epithelium cells, Cellchat (15) was used.
TCGA database and Oncomine database were exploited to detect FAM83D expression. Kaplan-Meier (KM) plotter was used to assess the prognostic value of FAM83D in 1656 patients with OC (http://www.kmplot.com). In order to investigate the biological function in OC, Gene Sets gained from the MSigDB database were used to perform Gene Set Enrichment Analysis (GSEA) (16), GSVA enrichment analysis (17), the enriched Kyoto Encyclopedia of Genes, Genomes (KEGG), and Gene Ontology (GO) analysis. Three miRNA-mRNA predictors (Starbase, TargetScan, and miRDB) were used to predict the miRNAs regulating FAM83D.
The 153 EMT gene signatures were collected from GOBP_EPITHELIAL_TO_MESENCHYMAL_TRANSITION gene set (http://www.gsea-msigdb.org/gsea/msigdb/index.jsp). GSE18520 (adjusted P < 0.001 and | logFC | > 2) identified 41 differentially expressed EMT genes. A total of 276 OC samples from GSE9891 were used as a training cohort. A total of 374 TCGA OC samples were used as test cohorts. Univariate Cox regression was used to screen prognostic genes among the 41 genes in the training set. Furthermore, the most useful prognostic markers were found by the lasso Cox regression model. Finally, 10 EMT gene features with non-zero coefficients were selected by R package glmnet (18), and the best lambda was determined by 10x cross-validation.
Human OC cell lines (SKOV3, A2780, OVCAR3, CAOV3, and HEY) and ovarian surface epithelium (OSE) cells were cultured according to a previous protocol (19). Cells were transfected with 100 nmol/L FAM83D siRNA2 and siRNA3 or control siRNA (JTS scientific) by Lipofectamine 3000 (Invitrogen).
Cells were lysed with RIPA buffer (Thermo Fisher Scientific, Waltham, MA, USA). The protein concentration was detected by a BCA protein assay kit (Beyotime Biotechnology, Jiangsu, China) and separated by SDS-PAGE (Gene Molecular Biotech Inc, Shanghai, China). The primary antibodies were used: anti-FAM83D (1:1000, Biorbyt), GAPDH, Vimentin, E-cadherin, and N-cadherin (1:1000, CST). Anti-GAPDH antibody was used as the loading control.
Total RNA was extracted by TRIzol reagent (Invitrogen, Calsbad, CA) and reversely transcribed using the ReverTraAceqPCR RT kit (Toyobo, Shanghai, China). The qRT-PCR was performed using SYBR Premix DimerEraser (Perfect Real Time) kit (TaKaRa Bio Inc) on an ABI 7500 Real-Time PCR system (Applied Biosystems, Foster City, USA) under the following conditions: 10 min at 95°C, followed by 40 cycles at 95°C for 15 sec and 60°C for 60 sec. Data were analyzed using the -2ΔΔct method and the expression of GAPDH was used as normalization control. All primer sequences used are summarized in Table S1.
Three thousand cells were seeded in 96-well plates for 24, 48, or 72 h. After each time point, 10μL of sterile Cell Counting Kit-8 (CCK-8; Dojindo, Rockville, MD, USA) was added to each well and incubated for another 2 h at 37°C. The absorbance was determined at 450 nm (Tecan, Switzerland).
A2780 and SKOV3 transfected cells were exposed to 8μg/ml and 10μg/ml cisplatin, respectively. After 24 hours, apoptosis cells were measured by annexin V-FITC and propidium iodide (PI) kit (BD Biosciences, San Jose, California, USA). BD flow cytometry was used to analyze the double-stained cells.
Wound-healing assay was applied to assess cell migration ability. Briefly, 1×106 cells were seeded on 6-well plates for 24h. Then, a line was drawn using a marker on the bottom of the dish, and a sterile 20-μl pipet tip was used to scratch three separate wounds through the cells (moving perpendicular to the line). The cells were gently rinsed twice with PBS to remove floating cells and incubated in 3 ml of medium. Images of the scratches were taken using an inverted microscope at 0 and 72 h of incubation. The scratch area was measured by image J software. The percentage of wound healing is the ratio of 72h migrated area (scratch area at 0h - scratch area at 72h) to 0h area. Transwell assays were used to evaluate cell migration and invasion ability. The cells were plated into the upper chamber of uncoated or matrigel-coated transwell chambers. The procedure was the same as previously described (19).
The miRNA-mimics were used to upregulate miR-138-5p (GenePharma, Shanghai, China). The overexpression plasmid (GenePharma) pcDNA3.1-FAM83D was constructed to upregulate the expression of FAM83D. The empty vector served as a negative control. Puromycin (GeneCHEM) was used to screen the stably transfected cell lines.
The FAM83D 3’-UTR fragment containing wildtype or the mutated miR-138-5p binding sites was amplified and subcloned into the pmirGLO Luciferase vector. OC cells were co-transfected with pmirGLO-wildtype/mutant FAM83D 3’-UTR and mimics, and relative luciferase activity was analyzed by the Dual-Luciferase Reporter assay system (TRAN, China).
All experiments were conducted for at least three independent times. The statistical differences between experimental groups were detected by the student’s t test. All data were presented as the means ± SDs based on three independent samples. Graphpad prism 8.0.1 software and R 4.1.0 were used for statistical analyses. Statistical significance was indicated by *p < 0.05.
To investigate OC heterogeneity, we performed 10x scRNA-seq of one HGSOC and one normal ovary. After data preprocessing including quality control and removal of low-quality cells, 6235 OC cells and 7655 normal ovary cells were analyzed. According to the expression of known markers, these cells were clustered into six groups: C0 and C1 (epithelial cluster), C2 (T cell cluster), C3 (macrophage cluster), C4 (mesenchyme cluster), and C5 (B cell cluster) (Figures 1A, B). Then, we delineated the distinctive gene signatures of each cluster and presented the top 5 significant differential expression genes (Figure 1C). The clusters were evaluated by annotation enrichment analysis of GO terms and KEGG pathway. C0 and C1 were characterized by cell junction, RNA regulation pathways, and cell cycle. The three clusters of immune cells C2, C3 and C5 showed gene enrichment for immune-related pathways. C4, mesenchymal cells were found to be strongly associated with focal adhesion, and extracellular matrix (ECM)-receptor interaction (Figures S1A, B). Consistent with immune scores and stromal scores, mesenchymal cells (C4) showed a higher stromal score and immune cells (C2, C3, and C5) exhibited a higher immune score (Figure 1D). In addition, large-scale copy number variation (CNV) helped to identify malignant clusters. The results showed the CNV level of C1 was significantly higher than other cell types (Figure 1E). This data suggested that OC originated from the epithelium and that OC is highly heterogeneous and consists of various cell types.
Figure 1 Single-cell expression profile of OC and normal ovary. (A) OC cell clusters from 10x Genomics scRNA-seq analysis visualized by UMAP. The number and percentage of cells for each cell type were summarized in the right panel. (B) Violin diagram showing the expression of marker genes in different cell types. (C) The dot map shows the top 5 genes in each cell type. (D) Violin plots show estimates of immune scores and stromal scores for different cell types. (E) The heatmap displayed large-scale CNVs of C0-C5. The red color represents a high CNV level and the blue represents a low CNV level.
We focused on the malignant features and molecular characteristics of C0 and C1 epithelial cells. We found that normal tissue is mainly composed of C0. In contrast, the tumor tissue is dominated by C1 (Figure 2A). Pseudo-time analysis was performed during tumor development to stratify tumor cells. Along the trajectory of normal to the tumor, the pseudo-time diagram illustrated the differentiation process from C0 to C1, which confirmed that C1 represents an advanced stage of epithelial cell differentiation of OC (Figure 2B).
Figure 2 Distinct molecular characteristics of epithelial cells in OC and construction of EMT gene set risk factor model. (A) The proportion of different cell types in different samples. (B) The developmental pseudo-time of epithelial cells inferred by analysis with Monocle2. (C) GSVA analysis for C0 and C1. (D) The forest plot of the association between 10 gene signature levels and overall survival in the training cohort. HR, 95% CI, and P values were determined by univariate Cox regression analysis. (E) A nomogram for predicting the 1-, 3- and 5-year OS for OC patients. (F) Calibration curves for nomogram. The light grey line indicates the ideal reference line where predicted probabilities would match the observed survival rates. The red dots are calculated by bootstrapping and represent the performance of the nomogram. The closer the solid red line is to the light grey line, the more accurately the model predicts survival. (G) The ROC curves of the diagnostic performance of the model. (H) Risk score distribution, survival status, and gene expression profile of patients in high-risk score group and low-risk score group in the training dataset. (I) Kaplan Meier curve of OS in a high-risk group and low-risk group of the training dataset.
Then, we identified genes whose expression patterns were drastically altered throughout differentiation and divided them into three subgroups according to their dynamic expression along the trajectory (Figure S2A). KEGG and GO analysis of these dynamically expressed genes illustrated that C1 was enriched in many carcinogenic pathways, such as DNA replication, signal transduction by p53 class mediator, TGF-beta signaling pathway, and Focal adhesion (Figures S2B, C, cluster 1 and 3). GSVA showed that C1 was more enriched in carcinogenic terms of cell cycle and invasion, such as EPITHELIAL_MESENCHYMAL_TRANSITION (EMT), E2F_TARGETS, etc. (Figure 2C). Of note, the expression of key epithelial markers (CDH1) of EMT progression was found to be downregulated or fluctuated, while the mesenchymal marker (CDH2) was increased, indicating dynamic changes in EMT are crucial during OC progression (Figures S2D, E). In brief, the above results display dynamic gene expression profiling during OC progress and suggest that C1 is a malignant epithelial cluster with excessive activation of EMT and proliferation-related pathways.
To probe into the clinical application of the EMT gene set, we used clinical data of 285 OC patients in GSE9891 to search for genes with significant prognostic significance among 41 genes (Figure S2F). The prognosis-related genes via univariate Cox analysis are presented in Figure 2D. FAM83D, PHLDB2, HMGA2, SFRP2, TGFB2, TGFBR2, MCRIP1, and DDX5 were risk genes for the prognosis of OC patients, while ELL3 and FOXA2 were protective genes. Then, we constructed a RiskScore model using the above 10 genes with the method of LASSO regression analysis (Figure S2G). The risk score for each OC patient was calculated using the following formula: ELL3× (-0.99548) + FAM83D × (0.39404) + PHLDB2× (0.11511) + HMGA2 × (0.10286) + SFRP2× (0.05705) + FOXA2× (-0.47186) + TGFB2× (0.17726) + TGFBR2× (0.36395) + MCRIP1× (0.4506) + DDX5× (0.27404). A nomogram with 10 gene signatures was constructed to evaluate the 1-, 3- and 5-year survival rates of OC patients (Figure 2E). Subsequently, the calibration curve of the nomogram showed that the predicted survival was in good agreement with the actual survival of the OC cohort (Figure 2F). In addition, the AUC for the OS was 0.722 (Figure 2G). To verify the accuracy of our model, KM survival curves were plotted in the GSE9891 cohort and TCGA cohort, respectively. According to the median RiskScore, patients were divided into low-risk and high-risk groups. We found that the higher the RiskScore, the higher the mortality rate (Figure 2H and Figure S2H). Besides, the KM survival curve showed that there were significant differences in prognosis between the two groups (p < 0.0001 in the GSE9891 cohort, Figure 2I; p = 0.018 in the TCGA cohort, Figure S2I).
In order to portray the essential characteristics of malignant epithelial cells, the C1 cluster was further subdivided into 9 different subgroups based on the Umap graph (Figure 3A). Firstly, we used cellchat to study the communication network among 9 subclusters. The data showed that S5 had a significant cell ratio in C1 and closely interacted with other subclusters, indicating its leading role in OC (Figure 3B). We further explored the biological functions of different subclusters through GSVA analysis (Figure S3A). As shown in Figures 3C; S5 was enriched in multiple HALLMARK pathways compared with other subclusters, such as G2M_CHECKPOINT, E2F_TARGETS, APOPTOSIS, DNA_REPAIR, and P53_PATHWAY. Hence, we deduced that S5 dominates the carcinogenic role in OC. Furthermore, we detected the expression of RiskScore model genes in S5. Interestingly, FAM83D was the only overlapping gene among the top 100 genes of S5 and RiskScore model genes (Figure 3D). It is worth mentioning that S5 specifically highly expressed FAM83D (Figures S3B, 3E). Thus, we named S5 as FAM83D+ malignant epithelial cells (FAM83D+ MEC). Taken together, these data suggest that FAM83D+ MEC has a crucial role in OC progression.
Figure 3 Malignant epithelial marker FAM83D overexpression is associated with a poor prognosis in OC. (A) The tSNE plot of nine subgroups generated from malignant epithelial cells. (B) The circle chart showed the number and intensity of interaction between different cell subgroups. The size of the circle is proportional to the number of cells in cell subgroups. (C) GSVA analysis score of S0-S8 in hallmark pathway. (D) The Venn diagram shows the intersection gene of S5 top100 Markers and riskscore model. (E) Positive expression ratio of FAM83D in S0-S8. (F) qRT-PCR and Western blot analysis of FAM83D expression in the ovarian cell lines and the normal ovarian cell line OSE. The right panel, the level of proteins was quantified by gray analysis. *P < 0.05 vs. OSE cells (t-test, N = 3). (G) The expression levels of FAM83D between OC and adjacent tissues in the Oncomine database. (H) The GSEA shows the high FAM83D group was associated with the G2M checkpoint and WANG_CISPLATIN_RESPONSE_AND_XPC_UP in the TCGA OC dataset. (I) Kaplan–Meier plots indicate the overall survival and progression-free survival for OC patients categorized by FAM83D expression. (J) FAM83D was related to survival in different subgroups (grade, stage, etc.) of OC patients.
Then, we analyzed the expression of FAM83D in cell lines and tissues. qRT-PCR and Western blot showed that FAM83D is significantly upregulated in HEY and SKOV3 cell lines (Figure 3F). Moreover, the Oncomine database showed that FAM83D was more highly expressed in OC tissues than in adjacent control (p < 0.001) (Figure 3G). Besides, the TCGA database demonstrated that FAM83D was considerably elevated in various cancers, including OC, BRCA, PAAD, and UCEC (Figure S3C).
To further study the role of FAM83D in OC progression, subjects in the TCGA OC dataset were divided into two groups based on the median value of FAM83D. GSEA showed that the high FAM83D group was related to the G2M checkpoint and WANG_CISPLATIN_RESPONSE_AND_XPC_UP (Figure 3H). In GSE45553, the expression of FAM83D in the cisplatin-resistant group was higher than cisplatin-sensitive group (p = 0.0002) (Figure S3D). These results indicate that FAM83D might regulate cell proliferation and induce cisplatin resistance.
Subsequently, we focused on the prognosis effect of FAM83D in 1656 OC patients. KM displayed that high FAM83D expression was significantly relevant to poor OS (p=4.5e-06) and progression-free survival (p=0.00018) in OC (Figure 3I). We also determined the relationship between FAM83D and clinical features by Forest plot. In clinical stages analysis, patients with high FAM83D had a poor prognosis in the stageIII/IV (hazard ratio: 1.42; 95% CI: 1.13-1.78) or gradeIII (hazard ratio:1.38; 95%CI: 1.06-1.79). Furthermore, in the chemotherapy analysis, high FAM83D expression of patients treated with platina or taxol was related to poor survival (Figure 3J). Taken together, these results suggested that FAM83D is an indicator of poor prognosis of OC.
To further examine the role of FAM83D, we constructed FAM83D knockdown cell lines by transfecting siRNA duplexes (Figures S4A, B). FAM83D knockdown inhibited the proliferation capacity and was more sensitive to cisplatin toxicity (A2780-siCON: IC50 = 5.443μg/ml, A2780-siFAM83D: IC50 = 2.647μg/ml; SKOV3-siCON: IC50 = 14.31μg/ml, SKOV3-siFAM83D: IC50 = 7.763μg/ml) (Figures 4A, B). Moreover, cisplatin-induced apoptosis cells were significantly increased in FAM83D knockdown cells (p = 0.0001 in A2780; p= 0.016 in SKOV3) (Figure 4C). These results suggest that FAM83D knockdown increases the cisplatin sensitivity of OC cells.
Figure 4 FAM83D deficiency inhibits the proliferation and promoted cisplatin sensitivity. (A) CCK8 assays for proliferation rates in SKOV3 and HEY cells transfected with siCON and siFAM83D. (B) Cisplatin sensitivity in SKOV3 and HEY cells transfected with siCON and siFAM83D. (C) Apoptotic cells detected by flow cytometry in siCON and siFAM83D treated or untreated cells with cisplatin. siCON, cells transfected with siRNA negative control; siFAM83D, cells transfected with FAM83D siRNA. ns, no significance, *P < 0.05, **P < 0.01 and ***P < 0.001.
In order to study the role of FAM83D on the migration and invasion of OC cells, Scratch and Transwell experiments were carried out. Scratch assay revealed that the number of migrated cells was decreased in FAM83D knockdown cells (p < 0.05, Figure 5A). Transwell showed FAM83D knockdown reduced migration and invasion ability of SKOV3 and HEY (p < 0.01, Figure 5B). Therefore, FAM83D was considered to be an irritation to OC migration and invasion.
Figure 5 FAM83D knockdown suppresses cell migration, invasion, and EMT in HEY and SKOV3 cells. (A) Migratory ability by scratch wound healing assay. The closure areas were quantified by comparison with the original wound area by Image J. Scale bar: 500 μm. The migration activity is expressed as mean ± SEM. *p < 0.05 vs. cells transfected with siCON cells (t-test, N = 3). (B) Representative images of migration and invasion were detected by transwell assay in SKOV3 and HEY cells transfected with siCON and siFAM83D cell groups. Scale bar: 200 μm. **p < 0.01 vs. cells transfected with siCON cells, ***p < 0.001 vs. cells transfected with siCON cells (t-test, N = 3). (C) GSEA analysis of the relationship between FAM83D and EMT. (D) The protein expression of GAPDH, E-cadherin, N-cadherin, and vimentin detected by Western blot in SKOV3 and HEY cells transfected with siCON and siFAM83D. The right panel, the level of proteins was quantified by gray analysis. *P < 0.05, ** P< 0.01 and *** P< 0.001 vs. cells transfected with siCON cells (t-test, N = 3). (E) The mRNA expression of GAPDH, E-cadherin, N-cadherin, and vimentin were detected by qRT-PCR in SKOV3 and HEY cells transfected with siCON and siFAM83D. *p < 0.05 vs. cells transfected with siCON cells, **p < 0.01 vs. cells transfected with siCON cells, ***p < 0.001 vs. cells transfected with siCON cells (t-test, N = 3).
GSEA analysis showed FAM83D was related to EMT (Figure 5C). Western blot (Figure 5D) and qRT-PCR (Figure 5E) showed that FAM83D knockdown increases the expression of E-cadherin (epithelial marker), and decreases the expression of N-cadherin and vimentin (mesenchymal markers), indicating that FAM83D knockdown could inhibit EMT, thus inhibiting OC progression. These results suggest that FAM83D induces the migration and invasion of OC cells by EMT.
Three databases (TargetScan, miRDB, and Starbase) were used to forecast possible miRNAs targeting the FAM83D 3’-UTR (Figure 6A), and miR-138-5p was identified.
Figure 6 FAM83D is a novel target of miR-138-5p in OC cells. (A) Bioinformatics analysis predicted that FAM83D was the target of miR-138-5p. (B) Schematic representation of the FAM83D 3’ -UTR containing the binding site for miR-138-5p. (C) The dual luciferase reporter assay confirmed that FAM83D is the direct target gene of miR-138-5p. ns, no significance, *P < 0.05, **P < 0.01 and ***P < 0.001 vs. cells transfected with NC mimics cells (t-test, N = 3) (D) Western Blot and qRT-PCR analysis of FAM83D expression in SKOV3 and HEY cells transfected with miR-138-5p mimics or NC mimics for 48 h The protein levels were quantified by grey analysis and showed in the right panel. **P < 0.01 and ***P < 0.001 vs. cells transfected with NC mimics cells (t-test, N = 3). (E) Growth curve of HEY and SKOV3 cells upon transfection with NC mimics and miR-138-5p mimics examined by CCK8 assay. *P < 0.05 vs. cells transfected with NC mimics cells (t-test, N = 3). (F) Scratch wound healing showed the migratory capacities of HEY and SKOV3 cells upon transfection with NC mimics and miR-138-5p mimics. Scale bar: 500 μm. **P < 0.01 vs. cells transfected with NC mimics cells (t-test, N = 3). (G) Cell migration and invasion ability were measured by Transwell assays. Scale bar: 200 μm. **P < 0.01 and ***P < 0.001 vs. cells transfected with NC mimics cells (t-test, N = 3). (H) Western blot and qRT-PCR analysis of the expression of EMT markers. The protein levels were quantified by grey analysis and showed in the right panel. *P < 0.05, **P < 0.01 and ***P < 0.001 vs. cells transfected with NC mimics cells (t-test, N = 3).
In order to confirm whether FAM83D was a target of miR-138-5p, we constructed luciferase vectors containing the miR-138-5p binding site wild-type or mutated FAM83D 3 ‘-UTR (Figure 6B). The luciferase reporter assay suggested that miR-138-5p mimics notably reduced the luciferase activity of FAM83D-wt in SKOV3 and HEY cells (p < 0.05, respectively; Figure 6C). Western blot and qRT-PCR confirmed that miR-138-5p mimics decrease the expression of FAM83D in SKOV3 and HEY cells (Figure 6D). The above results showed that FAM83D was a novel target of miR-138-5p in OC cells.
To determine the role of miR-138-5p in OC, we overexpressed the expression of miR-138-5p in OC cells by transfection mimics. CCK8 confirmed that the miR-138-5p overexpression observably restrained cell proliferation in SKOV3 and HEY cells (Figure 6E). Scratch and Transwell assay indicated that miR-138-5p overexpression remarkably restrained the migration and invasion ability of SKOV3 and HEY cells (Figures 6F, G, respectively). Meanwhile, miR-138-5p overexpression increased E-cadherin and inhibited N-cadherin and vimentin, suggesting miR-138-5p inhibited the EMT pathway (Figure 6H). Therefore, these results confirmed that miR-138-5p inhibited OC cells’ migration, invasion, and EMT.
To confirm whether miR-138-5p restrains the migration and invasion of OC cells by regulating FAM83D, the JLV-Puro-FAM83D expression plasmid was used to construct FAM83D overexpression OSE and SKOV3 cell lines (OSEFAM83D-OE and SKOV3FAM83D-OE) for rescue experiment. MiR-138-5p mimics observably inhibited the expression of FAM83D in OSEFAM83D-OE and SKOV3FAM83D-OE cells (Figures 7A, B). Scratch (Figures 7C and S5A) and Transwell assays (Figure 7D) confirmed that the FAM83D overexpression significantly promoted cell migration, while miR-138-5p mimics weakened the ability of cell migration in OSEFAM83D-OE and SKOV3FAM83D-OE cells. The result of CCK8 demonstrated miR-138-5p mimics could abrogate the facilitation of FAM83D in cell proliferation (Figure S5B).
Figure 7 Overexpression of miR-138-5p reverses the facilitation of FAM83D on OC cell progression and EMT. (A, B) qRT-PCR and Western blot analysis of FAM83D expression in OSEFAM83D-OE and SKOV3FAM83D-OE cells transfected with miR-138-5p mimics. The protein levels were quantified by grey analysis and showed in the lower panel. *P < 0.05, **P < 0.01 and ***P < 0.001 (test =3). (C) The closure areas in the scratch wound healing assays were shown by Image J **P < 0.01 and ***P < 0.001 (test =3). (D) Migration of OSEFAM83D-OE and SKOV3FAM83D-OE cells transfected with miR-138-5p mimics were determined by Transwell assay. Scale bar: 200 μm. **P < 0.01 and ***P < 0.001 (test =3). (E, F) qRT-PCR and Western blot analysis of EMT markers in OSEFAM83D-OE and SKOV3FAM83D-OE cells transfected with miR-138-5p mimics. The protein levels were quantified by grey analysis and showed in the right panel. *P < 0.05, **P < 0.01 and ***P < 0.001 (test =3) NC, cells infected with the JLV-Puro-FAM83D expression plasmid negative control; FAM83D-OE, cells infected with the JLV-Puro-FAM83D expression plasmid.
Furthermore, overexpression of FAM83D markedly inhibited the expression of E-cadherin and enhanced the expression of N-cadherin and vimentin, whereas miR-138-5p mimics reversed the role of FAM83D on EMT (Figures 7E, F). These results confirmed that miR-138-5p could inhibit EMT phenotype partially by targeting FAM83D, and miR-138-5p/FAM83D/EMT axis is conducive to the progression of OC cells.
OC is a heterogeneous disease with distinct genetic and molecular features (20). Therefore, accurate prognoses and efficacious therapies are urgently needed. A combination of scRNA-seq technology and bioinformatics methods has shown to be an efficient tool for us to identify key genes associated with OC and get a prefound understanding of its pathogenesis and heterogeneity. Consistent with them (21, 22), we confirmed the great heterogeneity of OC. We identified different cell types of OC, including two epithelial cell types, mesenchyme cells, macrophages, B cells, and T cells. Two groups of epithelial cells accounted for about 80% of the total cells, which supports that OC mostly originates from the epithelium (23). Through trajectory analysis and CNV analysis (24), we found that malignant epithelial cells (C1) highly expressed the malignant marker PAX8 and genes related to DNA replication pathways and WNT, KRAS, and other carcinogenic pathways. It has been proven that dysregulated ribosomal organisms occur in the progress of most spontaneous cancers (25). Intriguingly, in this study, the overall upregulation of ribosomal biogenesis-related genes was found to be accompanied by the deterioration of ovarian epithelial cells (Figures S1A, B). This suggested that ribosomal biogenesis has a critical role the progression of OC. Moreover, our data revealed multiple subpopulations of epithelial cells, particularly a predominant subgroup (FAM83D+ MEC). FAM83D is a microtubule-associated protein that can induce polar ejection forces and mitotic progression (26). Previous studies have confirmed that high expression of FAM83D in various cancers and the upregulation of FAM83D may be related to cancer progression (27–29). Consistently, we found FAM83D+ MEC exhibited mostly ligand-receptor pairs with other clusters and was associated with multiple carcinogenic pathways, including DNA replication and repair, and P53 pathways. Altogether, attention to the molecular characteristics of epithelial cell subsets contributed to our understanding of OC.
EMT can transform epithelial phenotypic characteristics into aggressive and metastatic characteristics, resulting in poor prognosis (30–32). Recently, more studies have shown that cells resistant to carboplatin and/or paclitaxel have a mesenchymal phenotype, indicating that EMT is a key factor in treating drug sensitivity (33–35). Recently, a single-cell transcriptional analysis confirmed the activation of EMT, enabling OC cells to obtain additional chemoresistance and metastasis (21). In this study, trajectory analysis of scRNA-seq data was conducted to characterize EMT changes during OC development. Consistent with previous studies, our results showed that the EMT-related pathway was activated during C0 to C1 differentiation. Previous studies have reported that additional ablation of CDH1 (E-cadherin) induces the persistence of OC diffusion and ascites accumulation, enhances peritoneal metastasis, and leads to poor prognosis (36, 37). Furthermore, CDH2 (N-cadherin) has been reported to enhance the adhesion to organotypic meso mimetic cultures and peritoneal explant and increase invasive and migratory properties (38). Herein, the expression of CDH1 was downregulated, and CDH2 was upregulated in the malignant epithelial clusters. In summary, our results strongly suggested that malignant epithelial cells have the molecular characteristics of excessive EMT.
Considerable evidence suggests that the heterogeneity of OC is the main reason for poor prognosis. The traditional clinicopathological indicators, e.g., tumor size, vascular infiltration, and TNM stage, cannot meet the current needs for predicting the individual prognosis (39, 40). Therefore, screening prognostic markers that can fully represent biological characteristics is of huge value in the individualized prevention and treatment of OC patients. In this study of OC, we identified malignant epithelial cells with EMT signatures using 10x single-cell technology. Furthermore, combined with GEO and TCGA databases, we constructed a RiskScore prognostic model. The AUC, nomogram, and KM curves were performed to demonstrate the model’s effectiveness in predicting OC risk. Furthermore, we demonstrated the carcinogenic effect of FAM83D, a key gene in the model, in vitro, indicating FAM83D is related to poor prognosis. Similarly, another EMT-related five-gene panel, including FAM83D, can predict the prognosis of HCC reliably and independently (41). In conclusion, we integrated single-cell sequencing and public databases to construct a model that may function as a powerful prognostic indicator for OC patients and demonstrated the clinical value of FAM83D, which may be a therapeutic target for OC.
There are few reports on the transcriptional regulation of FAM83D. Recently, studies have demonstrated that microRNA (miRNA) offers a novel sight to study the regulation mechanism of cancer (42–44). We used bioinformatics methods to find that miR-138-5p could target FAM83D. A previous study showed that miR-138-5p represses breast cancer cell invasion, migration, and EMT through targeting regulation of RHBDD1 (45). Another study suggested that miR-138-5p is a vital regulator of chemoresistance in colorectal cancer cells by acting on the NFIB-Snail1 axis (46). MiR-138-5p regulates the tumor microenvironment to inhibit NSCLC cells by targeting PD-L1/PD-1 (47). Herein, we confirmed that miR-138-5p directly binds to the FAM83D sequence through luciferase reporter gene assay. MiR-138-5p overexpression could decrease the mRNA and protein levels of FAM83D. We further studied the biological significance of miR-138-5p in OC in vitro and confirmed that the ectopic expression of miR-138-5p remarkably repressed the migration, invasion, and EMT of OC cells. Taken together, we confirmed that miR-138-5p inhibit OC progression by targeting FAM83D.
ScRNA-seq uncovered new molecular features of OC malignant epithelial cells. Moreover, a robust RiskScore prognostic model was constructed combined with public databases. Through integrated analysis, FAM83D was identified as an indicator of poor prognosis of OC and its carcinogenic role in OC cells was further confirmed in vitro. In addition, we discovered that miR-138-5p could regulate FAM83D expression, which could be a novel target for OC therapy. Our work clarified the molecular characteristics of OC malignant epithelial cells based on scRNA-seq, thus providing clinical guidance for the prognosis and treatment of OC patients.
The datasets presented in this study can be found in online repositories. The names of the repository/repositories and accession number(s) can be found in the article/Supplementary Material.
JL, XZ, and WY conceived and designed the study. JL and ZFL carried out all experiments and wrote the manuscript. HZ and JG participated in bioinformatics analysis. ZBL supported the study. YG, XZ, and WY supervised the study. CY, XZ and WY revised the manuscript. All authors contributed to the article and approved the submitted version.
The study is supported by the National Natural Science Foundation of China (No. 81672838, No. 82002739), Beijing Municipal Science and Technology Commission (No. Z181100001718193), Beijing Natural Science Foundation (No. 7212039) and Beijing Municipal Administration of Hospitals Clinical Medicine Development of Special Funding (No. XMLX201705).
The authors declare that the research was conducted in the absence of any commercial or financial relationships that could be construed as a potential conflict of interest.
All claims expressed in this article are solely those of the authors and do not necessarily represent those of their affiliated organizations, or those of the publisher, the editors and the reviewers. Any product that may be evaluated in this article, or claim that may be made by its manufacturer, is not guaranteed or endorsed by the publisher.
The Supplementary Material for this article can be found online at: https://www.frontiersin.org/articles/10.3389/fonc.2022.1055648/full#supplementary-material
1. Siegel RL, Miller KD, Fuchs HE, Jemal A. Cancer statistics, 2021. CA: Cancer J Clin (2021) 71(1):7–33. doi: 10.3322/caac.21654
2. Geistlinger L, Oh S, Ramos M, Schiffer L, LaRue RS, Henzler CM, et al. Multiomic analysis of subtype evolution and heterogeneity in high-grade serous ovarian carcinoma. Cancer Res (2020) 80(20):4335–45. doi: 10.1158/0008-5472.Can-20-0521
3. Wang R, Dang M, Harada K, Han G, Wang F, Pool Pizzi M, et al. Single-cell dissection of intratumoral heterogeneity and lineage diversity in metastatic gastric adenocarcinoma. Nat Med (2021) 27(1):141–51. doi: 10.1038/s41591-020-1125-8
4. Wang X, Li X, Lin F, Sun H, Lin Y, Wang Z, et al. The lnc-Ctslp8 upregulates Ctsl1 as a competitive endogenous rna and promotes ovarian cancer metastasis. J Exp Clin Cancer Res: CR (2021) 40(1):151. doi: 10.1186/s13046-021-01957-z
5. Norquist BM, Harrell MI, Brady MF, Walsh T, Lee MK, Gulsuner S, et al. Inherited mutations in women with ovarian carcinoma. JAMA Oncol (2016) 2(4):482–90. doi: 10.1001/jamaoncol.2015.5495
6. Patch AM, Christie EL, Etemadmoghadam D, Garsed DW, George J, Fereday S, et al. Whole-genome characterization of chemoresistant ovarian cancer. Nature (2015) 521(7553):489–94. doi: 10.1038/nature14410
7. Waldron L, Haibe-Kains B, Culhane AC, Riester M, Ding J, Wang XV, et al. Comparative meta-analysis of prognostic gene signatures for late-stage ovarian cancer. J Natl Cancer Institute (2014) 106(5):dju049. doi: 10.1093/jnci/dju049
8. Olbrecht S, Busschaert P, Qian J, Vanderstichele A, Loverix L, Van Gorp T, et al. High-grade serous tubo-ovarian cancer refined with single-cell rna sequencing: Specific cell subtypes influence survival and determine molecular subtype classification. Genome Med (2021) 13(1):111. doi: 10.1186/s13073-021-00922-x
9. Hornburg M, Desbois M, Lu S, Guan Y, Lo AA, Kaufman S, et al. Single-cell dissection of cellular components and interactions shaping the tumor immune phenotypes in ovarian cancer. Cancer Cell (2021) 39(7):928–44.e6. doi: 10.1016/j.ccell.2021.04.004
10. Hu Z, Artibani M, Alsaadi A, Wietek N, Morotti M, Shi T, et al. The repertoire of serous ovarian cancer non-genetic heterogeneity revealed by single-cell sequencing of normal fallopian tube epithelial cells. Cancer Cell (2020) 37(2):226–42.e7. doi: 10.1016/j.ccell.2020.01.003
11. Butler A, Hoffman P, Smibert P, Papalexi E, Satija R. Integrating single-cell transcriptomic data across different conditions, technologies, and species. Nat Biotechnol (2018) 36(5):411–20. doi: 10.1038/nbt.4096
12. Yoshihara K, Shahmoradgoli M, Martínez E, Vegesna R, Kim H, Torres-Garcia W, et al. Inferring tumour purity and stromal and immune cell admixture from expression data. Nat Commun (2013) 4:2612. doi: 10.1038/ncomms3612
13. Patel AP, Tirosh I, Trombetta JJ, Shalek AK, Gillespie SM, Wakimoto H, et al. Single-cell rna-seq highlights intratumoral heterogeneity in primary glioblastoma. Sci (New York NY) (2014) 344(6190):1396–401. doi: 10.1126/science.1254257
14. Trapnell C, Cacchiarelli D, Grimsby J, Pokharel P, Li S, Morse M, et al. The dynamics and regulators of cell fate decisions are revealed by pseudotemporal ordering of single cells. Nat Biotechnol (2014) 32(4):381–6. doi: 10.1038/nbt.2859
15. Jin S, Guerrero-Juarez CF, Zhang L, Chang I, Ramos R, Kuan CH, et al. Inference and analysis of cell-cell communication using cellchat. Nat Commun (2021) 12(1):1088. doi: 10.1038/s41467-021-21246-9
16. Yu G, Wang LG, Han Y, He QY. Clusterprofiler: An r package for comparing biological themes among gene clusters. Omics: J Integr Biol (2012) 16(5):284–7. doi: 10.1089/omi.2011.0118
17. Hänzelmann S, Castelo R, Guinney J. Gsva: Gene set variation analysis for microarray and rna-seq data. BMC Bioinf (2013) 14:7. doi: 10.1186/1471-2105-14-7
18. Friedman J, Hastie T, Tibshirani R. Regularization paths for generalized linear models Via coordinate descent. J Stat Softw (2010) 33(1):1–22. doi: 10.18637/jss.v033.i01
19. Zhao H, Gao Y, Chen Q, Li J, Ren M, Zhao X, et al. Rad51ap1 promotes progression of ovarian cancer Via tgf-β/Smad signalling pathway. J Cell Mol Med (2021) 25(4):1927–38. doi: 10.1111/jcmm.15877
20. Handley KF, Sims TT, Bateman NW, Glassman D, Foster KI, Lee S, et al. Classification of high-grade serous ovarian cancer using tumor morphologic characteristics. JAMA Netw Open (2022) 5(10):e2236626. doi: 10.1001/jamanetworkopen.2022.36626
21. Izar B, Tirosh I, Stover EH, Wakiro I, Cuoco MS, Alter I, et al. A single-cell landscape of high-grade serous ovarian cancer. Nat Med (2020) 26(8):1271–9. doi: 10.1038/s41591-020-0926-0
22. Xu J, Fang Y, Chen K, Li S, Tang S, Ren Y, et al. Single-cell rna sequencing reveals the tissue architecture in human high-grade serous ovarian cancer. Clin Cancer Res (2022) 28(16):3590–602. doi: 10.1158/1078-0432.Ccr-22-0296
23. Bell DA. Origins and molecular pathology of ovarian cancer. Modern Pathol (2005) 18 Suppl 2:S19–32. doi: 10.1038/modpathol.3800306
24. Manasa P, Sidhanth C, Krishnapriya S, Vasudevan S, Ganesan TS. Oncogenes in high grade serous adenocarcinoma of the ovary. Genes Cancer (2020) 11(3-4):122–36. doi: 10.18632/genesandcancer.206
25. Pelletier J, Thomas G, Volarević S. Ribosome biogenesis in cancer: New players and therapeutic avenues. Nat Rev Cancer (2018) 18(1):51–63. doi: 10.1038/nrc.2017.104
26. Fulcher LJ, He Z, Mei L, Macartney TJ, Wood NT, Prescott AR, et al. Fam83d directs protein kinase Ck1α to the mitotic spindle for proper spindle positioning. EMBO Rep (2019) 20(9):e47495. doi: 10.15252/embr.201847495
27. Wang D, Han S, Peng R, Wang X, Yang XX, Yang RJ, et al. Fam83d activates the Mek/Erk signaling pathway and promotes cell proliferation in hepatocellular carcinoma. Biochem Biophys Res Commun (2015) 458(2):313–20. doi: 10.1016/j.bbrc.2015.01.108
28. Yang XX, Ma M, Sang MX, Zhang XY, Zou NY, Zhu SC. Knockdown of Fam83d enhances radiosensitivity in coordination with irradiation by inhibiting emt Via the Akt/Gsk-3β/Snail signaling pathway in human esophageal cancer cells. OncoTargets Ther (2020) 13:4665–78. doi: 10.2147/ott.S245681
29. Yin C, Lin X, Wang Y, Liu X, Xiao Y, Liu J, et al. Fam83d promotes epithelial-mesenchymal transition, invasion and cisplatin resistance through regulating the Akt/Mtor pathway in non-Small-Cell lung cancer. Cell Oncol (Dordrecht) (2020) 43(3):395–407. doi: 10.1007/s13402-020-00494-9
30. Liang H, Zhao X, Wang C, Sun J, Chen Y, Wang G, et al. Correction to: Systematic analyses reveal long non-coding rna (Ptaf)-mediated promotion of emt and invasion-metastasis in serous ovarian cancer. Mol Cancer (2020) 19(1):173. doi: 10.1186/s12943-020-01296-1
31. Jinesh GG, Brohl AS. Classical epithelial-mesenchymal transition (Emt) and alternative cell death process-driven blebbishield metastatic-witch (Bmw) pathways to cancer metastasis. Signal Transduction Targeted Ther (2022) 7(1):296. doi: 10.1038/s41392-022-01132-6
32. Sadrkhanloo M, Entezari M, Orouei S, Ghollasi M, Fathi N, Rezaei S, et al. Stat3-emt axis in tumors: Modulation of cancer metastasis, stemness and therapy response. Pharmacol Res (2022) 182:106311. doi: 10.1016/j.phrs.2022.106311
33. Zhu X, Shen H, Yin X, Long L, Xie C, Liu Y, et al. Mir-186 regulation of Twist1 and ovarian cancer sensitivity to cisplatin. Oncogene (2016) 35(3):323–32. doi: 10.1038/onc.2015.84
34. Zhang J, Guan W, Xu X, Wang F, Li X, Xu G. A novel homeostatic loop of sorcin drives paclitaxel-resistance and malignant progression Via Smad4/Zeb1/Mir-142-5p in human ovarian cancer. Oncogene (2021) 40(30):4906–18. doi: 10.1038/s41388-021-01891-6
35. Huang Y, Hong W, Wei X. The molecular mechanisms and therapeutic strategies of emt in tumor progression and metastasis. J Hematol Oncol (2022) 15(1):129. doi: 10.1186/s13045-022-01347-8
36. Shi M, Whorton AE, Sekulovski N, Paquet M, MacLean JA, Song Y, et al. Inactivation of Trp53, pten, Rb1, and/or Cdh1 in the ovarian surface epithelium induces ovarian cancer transformation and metastasis. Biol Reprod (2020) 102(5):1055–64. doi: 10.1093/biolre/ioaa008
37. Lin HW, Fu CF, Chang MC, Lu TP, Lin HP, Chiang YC, et al. Cdh1, Dlec1 and Sfrp5 methylation panel as a prognostic marker for advanced epithelial ovarian cancer. Epigenomics (2018) 10(11):1397–413. doi: 10.2217/epi-2018-0035
38. Klymenko Y, Johnson J, Bos B, Lombard R, Campbell L, Loughran E, et al. Heterogeneous cadherin expression and multicellular aggregate dynamics in ovarian cancer dissemination. Neoplasia (New York NY) (2017) 19(7):549–63. doi: 10.1016/j.neo.2017.04.002
39. Jacobs IJ, Menon U, Ryan A, Gentry-Maharaj A, Burnell M, Kalsi JK, et al. Ovarian cancer screening and mortality in the uk collaborative trial of ovarian cancer screening (Ukctocs): A randomised controlled trial. Lancet (London England) (2016) 387(10022):945–56. doi: 10.1016/s0140-6736(15)01224-6
40. Zhu JW, Charkhchi P, Akbari MR. Potential clinical utility of liquid biopsies in ovarian cancer. Mol Cancer (2022) 21(1):114. doi: 10.1186/s12943-022-01588-8
41. Zhu G, Xia H, Tang Q, Bi F. An epithelial-mesenchymal transition-related 5-gene signature predicting the prognosis of hepatocellular carcinoma patients. Cancer Cell Int (2021) 21(1):166. doi: 10.1186/s12935-021-01864-5
42. Quirico L, Orso F, Cucinelli S, Paradzik M, Natalini D, Centonze G, et al. Mirna-guided reprogramming of glucose and glutamine metabolism and its impact on cell Adhesion/Migration during solid tumor progression. Cell Mol Life Sci: CMLS (2022) 79(4):216. doi: 10.1007/s00018-022-04228-y
43. Patel M, Wang Y, Bartom ET, Dhir R, Nephew KP, Matei D, et al. The ratio of toxic-to-Nontoxic mirnas predicts platinum sensitivity in ovarian cancer. Cancer Res (2021) 81(15):3985–4000. doi: 10.1158/0008-5472.Can-21-0953
44. Cho JG, Kim SW, Lee A, Jeong HN, Yun E, Choi J, et al. Microrna-dependent inhibition of Wee1 controls cancer stem-like characteristics and malignant behavior in ovarian cancer. Mol Ther Nucleic Acids (2022) 29:803–22. doi: 10.1016/j.omtn.2022.08.028
45. Zhao C, Ling X, Li X, Hou X, Zhao D. Microrna-138-5p inhibits cell migration, invasion and emt in breast cancer by directly targeting Rhbdd1. Breast Cancer (Tokyo Japan) (2019) 26(6):817–25. doi: 10.1007/s12282-019-00989-w
46. Xu W, Chen B, Ke D, Chen X. Microrna-138-5p targets the nfib-Snail1 axis to inhibit colorectal cancer cell migration and chemoresistance. Cancer Cell Int (2020) 20:475. doi: 10.1186/s12935-020-01573-5
Keywords: ovarian cancer, single-cell RNA sequencing, bioinformatics, prognostic signature, FAM83D
Citation: Li J, Li Z, Gao Y, Zhao H, Guo J, Liu Z, Yin C, Zhao X and Yue W (2022) Integrating single-cell RNA sequencing and prognostic model revealed the carcinogenicity and clinical significance of FAM83D in ovarian cancer. Front. Oncol. 12:1055648. doi: 10.3389/fonc.2022.1055648
Received: 28 September 2022; Accepted: 22 November 2022;
Published: 08 December 2022.
Edited by:
Vasiliki Gkretsi, European University Cyprus, CyprusReviewed by:
Martyna Filipska, Fundació Institut Mar d’Investigacions Mèdiques (IMIM), SpainCopyright © 2022 Li, Li, Gao, Zhao, Guo, Liu, Yin, Zhao and Yue. This is an open-access article distributed under the terms of the Creative Commons Attribution License (CC BY). The use, distribution or reproduction in other forums is permitted, provided the original author(s) and the copyright owner(s) are credited and that the original publication in this journal is cited, in accordance with accepted academic practice. No use, distribution or reproduction is permitted which does not comply with these terms.
*Correspondence: Wentao Yue, eXVld3RAY2NtdS5lZHUuY24=; Xiaoting Zhao, emhhb194aWFvdGluZ0BjY211LmVkdS5jbg==; Chenghong Yin, eWluY2hoQGNjbXUuZWR1LmNu
Disclaimer: All claims expressed in this article are solely those of the authors and do not necessarily represent those of their affiliated organizations, or those of the publisher, the editors and the reviewers. Any product that may be evaluated in this article or claim that may be made by its manufacturer is not guaranteed or endorsed by the publisher.
Research integrity at Frontiers
Learn more about the work of our research integrity team to safeguard the quality of each article we publish.