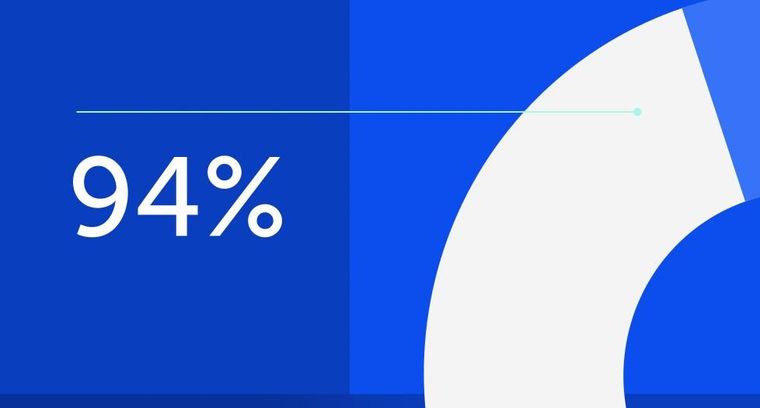
94% of researchers rate our articles as excellent or good
Learn more about the work of our research integrity team to safeguard the quality of each article we publish.
Find out more
MINI REVIEW article
Front. Oncol., 24 November 2022
Sec. Cancer Genetics
Volume 12 - 2022 | https://doi.org/10.3389/fonc.2022.1051487
This article is part of the Research TopicRising Stars in Cancer Genetics 2022View all 4 articles
Cancer-specific alternatively spliced events (ASE) play a role in cancer pathogenesis and can be targeted by immunotherapy, oligonucleotide therapy, and small molecule inhibition. However, identifying actionable ASE targets remains challenging due to the uncertainty of its protein product, structure impact, and proteoform (protein isoform) function. Here we argue that an integrated multi-omics profiling strategy can overcome these challenges, allowing us to mine this untapped source of targets for therapeutic development. In this review, we will provide an overview of current multi-omics strategies in characterizing ASEs by utilizing the transcriptome, proteome, and state-of-art algorithms for protein structure prediction. We will discuss limitations and knowledge gaps associated with each technology and informatics analytics. Finally, we will discuss future directions that will enable the full integration of multi-omics data for ASE target discovery.
Alternative splicing is an integral transcriptional process that enables the production of multiple isoforms from a single gene (1, 2). The process is guided by the combinatorial inclusion and exclusion of exons or intron flanking regions (Figure 1A). Several pan-cancer studies of splicing-associated variants have suggested that cancer-specific isoform switches are part of the oncogenic process and contribute to the functional transformation of cancer cells (3–6). In cancer, these splicing-associated mutations can be either a trans-acting variant, which results in broad splicing change, or a cis-acting variant, which results in localized splicing change in a single gene (7). Examples of trans-acting variants include splicing factor (e.g., SF3B1, SRSF2, U2AF1, ZRSR2) mutations in both blood cancers (8–11) and solid tumors (12–15), and examples of the cis-acting splicing variants of cancer drivers include genome rearrangement, such as DUX4-ERG (16), point mutations in the splicing motifs of TP53 (17). Together, this splicing-associated genetic evidence suggests that alternative splicing events (ASEs) are important actors of the cancer oncogenic process (18) and can influence the cancer etiology in many situations.
Figure 1 A holistic multi-omics approach to understanding therapeutic targets in cancer. (A) Central dogma of DNA-to-RNA followed by RNA splicing and protein translation. (B) Multi-omics technologies can be applied to study alternative splicing events, including High-throughput sequencing to profile the RNA transcript (Left-Most). Mass spectrometry technologies to profile the protein (Middle-Left). Protein structure can be predicted (Middle-Right). Functional screening such as siRNA or CRISPR can be applied (Right-Most). (C) RNA sequencing technologies can reveal the alternative splicing pattern (Left-Most). Mass spectrometry technologies can validate the protein product (Middle-Left). Structure prediction can identify the impact of alternative splicing (Middle-Right). Functional screening with oligonucleotide or CRISPR to screen for the functional effect of the target (Right-Most). (D) Examples of therapeutic strategies to target alternative splicing include splicing-derived neoantigens for immunotherapy, splicing-associated therapeutic vulnerability, splicing factor inhibitors, and small molecular inhibition to overcome drug resistance. Created with BioRender.com.
Cancer-specific isoforms are RNA transcript isoforms exclusively expressed in cancer cells. Because they originate from somatic events (or from genes regulated by a cancer-cell epigenetic program), they are typically not expressed in normal tissue (19, 20). While these cancer isoform switches can be utilized as a biomarker for the clinical monitoring of adult malignancies (21, 22), several therapeutic strategies are available for targeting these alternative splicing events. Four major strategies include:
1. Chemotherapeutic inhibition of splicing factors, such as Sudemycin or other small molecules targeting SF3B1 (23–27).
2. Oligonucleotide therapy to activate or inhibit certain splicing events. Whereas splice-switching oligonucleotides (SSOs) can block access of the RNA splicing machinery to a splice site (28), a decoy RNA binding sequence can be used to inhibit the splicing factor activity by competing with its capacity for RNA binding (29).
3. Identification of oncogene with splicing-associated therapeutic vulnerability (See Figure 1D). Example of this includes MYC-driven cancers, such as neuroblastoma (30), lymphoma (31), and triple-negative breast cancer (32).
4. Immunotherapy targeting of neoantigen derived from cancer-specific ASEs (See Figure 1D). For example, neoantigens derived from these splicing alterations (7) can be used for mRNA vaccines (33), and surface antigens derived from cancer-specific isoforms can be targeted by CAR-T cell engineering, such as FN1 ED-B variant (34).
Given their therapeutic potential, identifying novel ASEs by high-throughput methods is critical to discovering new therapeutic targets for treating cancer patients.
Currently, the mining of therapeutic targets is often limited to short-read RNA sequencing analysis of gene-level data without isoform considerations. This can limit the discovery process to a small number of targets, not considering either the protein isoform or its functional consequences. Thus, we posit that integrative multi-omics profiling will be necessary for the effective prioritization of ASE events as therapeutic targets as well as to understand their role in reprogramming the molecular network for cancer drug resistance (Figures 1B, C). These technologies include:
1. Short-and-long-read RNA sequencing for discovery and validation of novel transcript.
2. Mass spectrometry technologies to validate the protein isoform product.
3. Structure prediction of the protein isoform to identify small molecular binding sites.
4. Functional prediction of proteoform alterations and target validation by functional screening.
Through these technologies we will be able to prioritize and discover effective therapeutic strategies to target ASEs (Figure 1D). In this review, we will provide an overview of the different multi-omics technologies, bioinformatics algorithms, and existing knowledge gaps in the field of ASE therapeutic discovery.
Bulk RNA sequencing profiling is now a routine part of consortium projects and many clinical trials with ample opportunities for data mining. There are two library preparation protocols for RNA sequencing: 1) The polyadenylated (poly-A) protocol and 2) the Ribosome-depleted protocol (Total RNAseq). The poly-A method selects RNA transcripts with oligo (dT) primers (35), which effectively enriches mature RNA transcripts while eliminating transcripts lacking the poly-A RNA track added to the 3’ end, such as rRNA and most noncoding RNAs. Alternatively, the total RNAseq protocol performs ribosome depletion followed by sequencing, which retains both mature and immature transcripts without significant bias to the transcript direction.
There are several bioinformatics methods for analyzing short-read RNA sequencing data. For transcript-level bioinformatics analysis, the relative proportion of the full-length mRNA isoform can be estimated by RNAseq reads aligned to a given reference genome. The analysis can be divided into alignment-based algorithms and pseudo-alignment algorithms. Alignment-based algorithms include RSEM (36) and HISAT (37). RSEM aligns the reads directly onto the transcript sequence by considering the uncertainty of read mapping and then calculates a maximum likelihood abundance using the expectation-maximization algorithm (36). HISAT optimizes the indexing scheme by the Burrows-Wheeler transform algorithm and the Ferragina-Manzini index (37). More recently, pseudo alignment tools, such as Kallisto (38) and salmon (39), have gained in popularity. These algorithms perform alignment-free isoform quantification, providing orders of magnitude faster processing without sacrificing accuracy (40).
However, these strategies assume a complete reference of transcript isoform annotation, and missing transcripts may impact the final estimation (41). Another significant problem with transcript-level estimation is that RNA sequencing coverage bias can also impact the algorithmic performance (42). Therefore, alternative approaches are needed to complement the transcription estimation by examining local splicing changes in junction reads. Junctions are sequencing reads mapped to the genome reference that spans between two or more exons. Junction reads are critical in determining the exon usage pattern, and derived statistics can be applied to infer cancer-specific splicing events. One typical statistic for inferring these junction-associated changes is the percent spliced in (Ψ) score (43), which represents the exon inclusion percentage. Tools utilizing this strategy includes MISO (44), rMATS (45), MAJIQ (46), and SplAdder (47). Other algorithms for determining alternative splicing include LeafCutter (48) and Splicing Deficiency (49), which leverage the intron coverage to infer transcript usage and splicing dysregulation. These tools provide an additional alternative for detecting unannotated splicing events. Nonetheless, there are limitations to this type of approach. Ambiguity in the RNA mapping (such as repetitive regions) can impact junction coverage and result in a missed opportunity for identifying novel targetable isoforms. To overcome this junction coverage problem, long-read RNA sequencing or single-module RNA sequencing (50, 51) can be applied to capture the complete assembly of the transcript isoform and their alternative splice sites. There are two approaches for long-read sequencing: Pacific Biosciences’ single-molecular real-time sequencing and Oxford Nanopore Technologies’ nanopore sequencing. These technologies enable an average read length of > 30kb and can go up to 50kb in many situations. However, long-read sequencing technologies are largely limited with low sequencing depth (52). Fragmentation and pore-blocking may also occur in transcripts with high RNA modifications, resulting in a truncated transcript biased to the 3’ or 5’ end. Hence, a joint characterization of the short-read and long-read RNA sequencing may be an optimal strategy for characterizing novel transcripts. For example, long-read RNA sequencing data can guide the transcript assembly of the short-read RNA sequencing data. Moreover, this strategy can be enhanced by single-molecule barcoding, which simplifies the transcript assembly, facilitating new transcript identification and bulk RNA sequencing analyses (53, 54). Altogether, additional algorithm development to integrate both datasets will be necessary.
While the RNA-based sequencing approach can identify novel transcripts, a significant portion (~2000) of the novel RNA isoforms lack proof of generating a protein product (55, 56). Therefore, proteogenomics, a strategy of integrating RNA sequencing with mass spectrometry data, is needed to confirm these protein products. There are several software workflows for the preparation and analysis of proteogenomic data (57–59). Generally, these tools are used to build custom protein databases based on nucleotide sequences for use in proteomics experiments. For example, RNAseq can identify alternative splicing events at the transcript level that, when translated, do not appear in standard protein databases (e.g., UniProt/SwissProt). Once assembled, these custom databases can be appended to standard (canonical protein) search databases, enabling the identification and quantification of non-standard peptides and proteins (e.g., splice variants, single nucleotide polymorphisms, gene fusions).
Numerous efforts have been made to resolve isoform-level protein quantification in human tissues (60–63). For example, Lau et al. (62) used an RNAseq-guided method to generate a customized database of tissue-specific splicing junctions, which was searched against ~80 million public mass spectra. Hundreds of splicing events were identified for each tissue, including alternative 3’ and 5’ splice sites, mutually exclusive exons, intron retention, and skipped exons. In particular, the authors revealed significantly higher usage of alternative splicing events in the heart and testis. In another example, Kahles et al. describe analyses of alternate splicing in 8,705 cancer patients across 32 cancer types from The Cancer Genome Atlas (7). Of note, they focused on 63 patients from breast cancer and ovarian serous cystadenocarcinoma with proteomics data from the Clinical Proteomics Tumor Analysis Consortium (CPTAC) and validated neojunctions using proteomics data multiplexed with tandem mass tag (TMT) technology. Although they were able to validate the protein potential for over two-thirds (43/63) of the junction, more than a third of the junctions remained unvalidated. This is likely due to the limitation of the TMT-library technology, which requires pooling of samples into a single multiplexed experiment and leads to difficulties in identifying low-abundance proteins (64).
Despite high splicing diversity reported by transcriptomic studies (65, 66), in which >85% of genes have transcribed multiple non-trivial isoforms, proteogenomic studies tend to focus on a single protein isoform per gene or lack the resolution to identify alternative proteoforms (60, 61). This discrepancy can be partially explained by processes such as micro-RNA-based degradation (67) or nonsense-mediated decay (68), but there are also technical challenges that restrict the detectability of isoforms. For example, trypsin is one of the most used enzymes for digesting proteins into peptides prior to LC-MS/MS. However, the ends of exons are enriched for trypsin digestion sites (i.e., lysine and arginine) (69), and the use of trypsin can digest peptides that would otherwise span the exon-exon junction that’s critical for making the splicing determination. Combining chymotrypsin with trypsin has partially alleviated this issue and resulted in increased junction peptide detection by >30% (69). Alternatively, the mass spectrometry machine can be programmed to monitor the specific mass range of the peptide supporting the proteoform. This targeted mass spectrometry strategy can enhance the proteomics identification of splice variants (70, 71). Moreover, a custom proteogenomic database built based on high-quality long-read sequencing transcripts can provide an improved custom database for mass spectrometry analysis (72, 73).
A superior understanding of an exon’s structural properties and functional features of the encoded protein segment will be necessary to optimize therapeutic targets of alternatively spliced exons. Here, we review several computational methods and studies that attempted to perform functional characterization of protein isoforms. ASEs are often enriched in intrinsically disordered regions (IDRs) (74), regions lacking a well-defined tertiary structure. These disordered regions contain linear motifs and post-translational modification sites (PTMs), which are highly conserved in protein-protein interactions (74). IDRs are important in maintaining cellular functions (75), play a role in several human diseases (76, 77), and have become promising candidates for rational drug design efforts. Thus, the prediction of IDR in these novel spliced isoforms is a critical component of the effective drug design. The IDR prediction architectures are divided into three categories:
1. Sequence scoring functions, using the additive and weighted functions to process the input protein sequences and sequence-derived information, such as FoldIndex (78) and IUPred3 (79).
2. Machine learning-based models trained from experimentally characterized IDRs, such as DisEMBL (80), DISOPRED3 (81), SPOT-Disorder2 (82), and flDPnn (83).
3. Meta-predictors, using multiple disorder predictions as inputs to generate the new disorder prediction, such as metaPrDOS (84), MobiDB-lite (85), and DisCoP (86).
Additionally, conserved binding motifs are often coded in tissue-specific alternatively spliced exons, which results in the tissue-specific rewiring of protein-protein interaction networks (74, 87). More recent work has suggested the potential for these protein-protein interactions to drive oncogenic transformation (5). Consequently, understanding the protein-rewiring by alternatively spliced membrane receptors, such as G protein-coupled receptors (GPCRs) (88–90), a major class of druggable receptors for cancer therapy (91), will be critical for future drug design efforts. GPCRs are a superfamily of proteins affected by various post-translational modifications (92) and alternative splicing (93) and are recognized for their role in cancer development, progression, and metastasis (94–96). Receptors, such as GPCRs, undergo significant conformational changes upon binding to their targets, which add to the structural and functional heterogeneity of their disordered regions, further intensified by cancer-related mutations (96). For example, the unique isoforms and their IDRs (detected mainly in the 3rd intracellular loop and the C-terminal tail) are typically enriched in tissue-specific isoforms, resulting in tissue-specific protein-protein interactions (90), including the GPCRs (97). Notably, the IDRs in the C-terminal of GPCRs are proposed to have a high potential for new target-binding partners (98). The importance of the N-terminal for the activation of two distinct GPCR isoforms was also highlighted (99). Given the richness of the conformational rearrangements and expression patterns across tissues, the GPCR isoforms represent new targets for developing drugs with improved tissue selectivity (93). Currently, there are a number of computational algorithms for modeling GPCR structure and ligand binding sites and their binding affinities, such as GPCR-I-TASSER (100), Computational Profiling for GPCRs (CPG) (101), GPCR_LigandClassify (102), and an unnamed method by Seo et al. (103), and conformation-specific thermostabilizing mutations predictive tool LiticonDesign (104). GPCR models include PRECOG (105), a machine learning algorithm that can model the coupling of GPCR isoforms and the G-protein, which can be leveraged as a target by Designer Receptors Exclusively Activated by Designer Drugs (106). Regardless, algorithms that fully consider the GPCR proteoform diversity is limited and remains a major knowledge gap in the field of GPCR drug discovery.
Another functional impact of alternative splicing is its effect on post-translational modification (107). PTMs are widely distributed in the protein universe, which include phosphorylation, ubiquitination, SUMOylation, lipidation, and glycosylation. These PTMs influence protein function by regulating protein cleavage, linkage, and cross-linking. Understanding the splicing impact on PTMs will enhance our ability to prioritize targetable protein regions. For example, splice variants that result in drug resistance include, BCL-ABL (108), FGFR (109), and HER2 (110). In recent years, several computational methods have been developed to predict PTMs in a whole protein sequence, such as MusiteDeep (111), iAcet-Sumo (112), GPS 5.0 (113). Although several high-throughput methods have been developed to identify the PTMs, this remains challenging and experimental validation of the PTMs by mass spectrometry is necessary to confirm the computational predictions. Therefore, a joint multi-omics profiling of splicing events and its post-translational signals will be critical in guiding future directions in targeted therapy, especially oncogenic kinase signaling. An alternative PTM modification is by glycolipid on glycosylphosphatidylinositol (GPI) anchor proteins. GPI-anchor proteins are membrane proteins and link to proteins to the outer face of the plasma membrane in eukaryotic cells, making them ideal targets for therapeutic intervention, including CAR T cell engineering. For example, GPI-anchor protein under consideration by CAR T cell include CEA (114), GPC1 (115), GPC2 (116), GPC3 (117), FOLR2 (118), and Mesothelin (119). While several predictors have been designed specifically for GPI-anchor predictions, such as GPI-SOM (120), FragAnchor (121), and PredGPI (122), no studies have performed a systematic analysis of cancer-specific protein isoform that may have gained a novel GPI-anchor. Thus, this represents a tremendous opportunity to uncover additional targets for therapeutic targeting.
Alternative splicing is one of the major contributors to protein variation and diversity, which provide cancer cells the cellular plasticity to survive stress (123). With the availability of CPTAC data, emerging studies are investigating a multi-omics approach for integrated splice variants discovery but are limited to the purpose of disease monitoring and prognosis determination (124, 125). Here, our scientific predicate (in this review) is that cancer-specific alternative splicing event is an untapped source of targets for therapeutic intervention, and a complete multi-omics characterization will enable the discovery, validation, and prioritization of the ASE targets. 1) we propose that the joint analysis of long and short RNA sequencing will enable a comprehensive search for novel splice variants in which long RNA sequencing is more suited for identifying novel isoforms, and short-read RNA sequencing will be able to estimate transcript abundance. Genotype-Tissue Expression (GTEx) project (60, 126, 127) can also be utilized as a normal tissue reference for the therapeutic discovery process. Significant multi-omics data has been generated by the GTEx community, which includes short-read RNA sequencing (17364 samples), Oxford Nanopore Long-read sequencing (88 samples), and TMT mass spectrometry proteomics data (32 samples). Jointly these data will provide a valuable normal control that enhances the therapeutic candidate selection process of cancer-restricted isoform variant while reducing unwanted adverse effects during therapy. 2) we propose that proteogenomic integration of RNA seq and mass spectrometry will enable the validation of the protein product derived from the aberrant splicing event. 3) with tremendous therapeutic interest in immunotherapy, kinase inhibition, and GPCR drug response, we propose that a structural understanding of gaining and losing PTM and IDR domains by alternative splicing will be necessary to prioritize important proteoforms with the spliced exon. Thus, protein structure annotation followed by mass spectrometry validation will enable the joint prediction and validation of new post-translational modification sites on the novel proteoform. Together, the development of these new technologies and integrative pipelines will enable us to leverage multi-omics data for therapeutic discovery.
Several challenges in each omics need to be considered in future development. 1) for both long read sequencing and mass spectrometry analysis, obtaining deep coverage remains costly in terms of time and money. Additionally, technical challenges with coverage bias will also need to be addressed. To overcome this issue, the harmonization of different datasets may enhance our ability to discover novel targets. The assumption is a recurring candidate, while suboptimal for detection in certain settings, can then be rescreened by informatics processing or through a targeted mass spectrometry or re-sequencing effort. 2) algorithm prediction of proteoform function will need to be validated by experiments. While we have proposed that mass spectrometry data may be one mechanism to validate the PTMs, the oncogenic role of the region remains to be validated. For trans-acting variants in splicing factors, such as mutations in SF3B1, a genetic mouse model will be necessary to evaluate its effect on malignant transformation (128). For cis-acting variants and therapeutic vulnerabilities, high-throughput functional screening of alternative splicing events, such as CRISPR (129) and shRNA (130), can be performed in different cancer cell lines. These models will evaluate phenotypes associated with proliferative gains or cancer-killing effects in the presence of other oncogenic drivers. Of note, current high-throughput genetic screening of adult cancers DepMap (131), Sanger (132), as well as in pediatric cancer (133) are restricted to gene-level knockouts. Thus, to fully comprehend the functional role of splice variants in cancer, high throughput CRISPR and shRNA libraries that target junctions will need to be developed in future studies.
Cancer-derived isoforms remains a dark matter in the context of cancer therapy. New omics dataset and structure prediction strategies will be critical for understanding the splicing function as well as optimizing therapeutic development priorities. Additional technologies, such as machine learning, will be essential to automate the integrative discovery platform from RNA-to-Protein-to-Structure-to-Function. In summary, the complete integration of these omics technologies will surely be necessary to facilitate the next generation of small molecule design, immunotherapeutic development, and to overcome therapeutic relapse and drug resistance.
TS, BZ, YL, HW, LW, BM, PS, and AK contributed to the writing and review of the manuscript. TS, PS, and AK contributed to the conception of the review paper. All authors contributed to the article and approved the submitted version.
The study was funded by American Cancer Society IRG-21-145-25, Moffitt Cancer Center Quantitative Science/Team Science, Moffitt Cancer Center Department of Biostatistics and Bioinformatics Pilot Award. This work has been supported in part by the Biostatistics and Bioinformatics Shared Resource at the H. Lee Moffitt Cancer Center & Research Institute, an NCI designated Comprehensive Cancer Center (P30-CA076292).
TS reports a patent for EBD CAR US20220267425A1.
The remaining authors declare that the research was conducted in the absence of any commercial or financial relationships that could be construed as a potential conflict of interest.
All claims expressed in this article are solely those of the authors and do not necessarily represent those of their affiliated organizations, or those of the publisher, the editors and the reviewers. Any product that may be evaluated in this article, or claim that may be made by its manufacturer, is not guaranteed or endorsed by the publisher.
1. Lee Y, Rio DC. Mechanisms and regulation of alternative pre-mRNA splicing. Annu Rev Biochem (2015) 84:291–323. doi: 10.1146/annurev-biochem-060614-034316
2. Nilsen TW, Graveley BR. Expansion of the eukaryotic proteome by alternative splicing. Nature (2010) 463:457–63. doi: 10.1038/nature08909
3. David JK, Maden SK, Weeder BR, Thompson RF, Nellore A. Putatively cancer-specific exon-exon junctions are shared across patients and present in developmental and other non-cancer cells. NAR Cancer 2 (2020) 2:zcaa001. doi: 10.1093/narcan/zcaa001
4. Climente-Gonzalez H, Porta-Pardo E, Godzik A, Eyras E. The functional impact of alternative splicing in cancer. Cell Rep (2017) 20:2215–26. doi: 10.1016/j.celrep.2017.08.012
5. Kahraman A, Karakulak T, Szklarczyk D, von Mering C. Pathogenic impact of transcript isoform switching in 1,209 cancer samples covering 27 cancer types using an isoform-specific interaction network. Sci Rep (2020) 10:14453. doi: 10.1038/s41598-020-71221-5
6. Shiraishi Y, Okada A, Chiba K, Kawachi A, Omori I, Mateos RN, et al. Systematic identification of intron retention associated variants from massive publicly available transcriptome sequencing data. Nat Commun (2022) 13:5357. doi: 10.1038/s41467-022-32887-9
7. Kahles A, Lehmann KV, Toussaint NC, Huser M, Stark SG, Sachsenberg T, et al. Comprehensive analysis of alternative splicing across tumors from 8,705 patients. Cancer Cell (2018) 34:211–24.e6. doi: 10.1016/j.ccell.2018.07.001
8. Yoshida K, Sanada M, Shiraishi Y, Nowak D, Nagata Y, Yamamoto R, et al. Frequent pathway mutations of splicing machinery in myelodysplasia. Nature (2011) 478:64–9. doi: 10.1038/nature10496
9. Rossi D, Bruscaggin A, Spina V, Rasi S, Khiabanian H, Messina M, et al. Mutations of the SF3B1 splicing factor in chronic lymphocytic leukemia: association with progression and fludarabine-refractoriness. Blood (2011) 118:6904–8. doi: 10.1182/blood-2011-08-373159
10. Patnaik MM, Lasho TL, Finke CM, Hanson CA, Hodnefield JM, Knudson RA, et al. Spliceosome mutations involving SRSF2, SF3B1, and U2AF35 in chronic myelomonocytic leukemia: prevalence, clinical correlates, and prognostic relevance. Am J Hematol (2013) 88:201–6. doi: 10.1002/ajh.23373
11. Zhang SJ, Rampal R, Manshouri T, Patel J, Mensah N, Kayserian A, et al. Genetic analysis of patients with leukemic transformation of myeloproliferative neoplasms shows recurrent SRSF2 mutations that are associated with adverse outcome. Blood (2012) 119:4480–5. doi: 10.1182/blood-2011-11-390252
12. Suzuki H, Kumar SA, Shuai S, Diaz-Navarro A, Gutierrez-Fernandez A, De Antonellis P, et al. Recurrent noncoding U1 snRNA mutations drive cryptic splicing in SHH medulloblastoma. Nature (2019) 574:707–11. doi: 10.1038/s41586-019-1650-0
13. Furney SJ, Pedersen M, Gentien D, Dumont AG, Rapinat A, Desjardins L, et al. SF3B1 mutations are associated with alternative splicing in uveal melanoma. Cancer Discovery (2013) 3:1122–9. doi: 10.1158/2159-8290.CD-13-0330
14. Maguire SL, Leonidou A, Wai P, Marchio C, Ng CK, Sapino A, et al. SF3B1 mutations constitute a novel therapeutic target in breast cancer. J Pathol (2015) 235:571–80. doi: 10.1002/path.4483
15. Heimberger AB, Hlatky R, Suki D, Yang D, Weinberg J, Gilbert M, et al. Prognostic effect of epidermal growth factor receptor and EGFRvIII in glioblastoma multiforme patients. Clin Cancer Res (2005) 11:1462–6. doi: 10.1158/1078-0432.CCR-04-1737
16. Zhang J, McCastlain K, Yoshihara H, Xu B, Chang Y, Churchman ML, et al. Jude Children's research hospital-Washington university pediatric cancer genome, deregulation of DUX4 and ERG in acute lymphoblastic leukemia. Nat Genet (2016) 48:1481–9. doi: 10.1038/ng.3691
17. Escobar-Hoyos LF, Penson A, Kannan R, Cho H, Pan CH, Singh RK, et al. Altered RNA splicing by mutant p53 activates oncogenic RAS signaling in pancreatic cancer. Cancer Cell (2020) 38:198–211.e8. doi: 10.1016/j.ccell.2020.05.010
18. Cherry S, Lynch KW. Alternative splicing and cancer: insights, opportunities, and challenges from an expanding view of the transcriptome. Genes Dev (2020) 34:1005–16. doi: 10.1101/gad.338962.120
19. Urbanski LM, Leclair N, Anczukow O. Alternative-splicing defects in cancer: Splicing regulators and their downstream targets, guiding the way to novel cancer therapeutics. Wiley Interdiscip Rev RNA (2018) 9:e1476. doi: 10.1002/wrna.1476
20. Zaidi SK, Frietze SE, Gordon JA, Heath JL, Messier T, Hong D, et al. Bivalent epigenetic control of oncofetal gene expression in cancer. Mol Cell Biol (2017) 37:23. doi: 10.1128/MCB.00352-17
21. Vitting-Seerup K, Sandelin A. The landscape of isoform switches in human cancers. Mol Cancer Res (2017) 15:1206–20. doi: 10.1158/1541-7786.MCR-16-0459
22. Chang A, Chakiryan NH, Du D, Stewart PA, Zhang Y, Tian Y, et al. Proteogenomic, epigenetic, and clinical implications of recurrent aberrant splice variants in clear cell renal cell carcinoma. Eur Urol (2022) 82(4):354–62. doi: 10.1016/j.eururo.2022.05.021
23. Lagisetti C, Palacios G, Goronga T, Freeman B, Caufield W, Webb TR. Optimization of antitumor modulators of pre-mRNA splicing. J Med Chem (2013) 56:10033–44. doi: 10.1021/jm401370h
24. Kaida D, Motoyoshi H, Tashiro E, Nojima T, Hagiwara M, Ishigami K, et al. Spliceostatin a targets SF3b and inhibits both splicing and nuclear retention of pre-mRNA. Nat Chem Biol (2007) 3:576–83. doi: 10.1038/nchembio.2007.18
25. Eskens FA, Ramos FJ, Burger H, O'Brien JP, Piera A, de Jonge MJ, et al. Phase I pharmacokinetic and pharmacodynamic study of the first-in-class spliceosome inhibitor E7107 in patients with advanced solid tumors. Clin Cancer Res (2013) 19:6296–304. doi: 10.1158/1078-0432.CCR-13-0485
26. Hasegawa M, Miura T, Kuzuya K, Inoue A, Won Ki S, Horinouchi S, et al. Identification of SAP155 as the target of GEX1A (Herboxidiene), an antitumor natural product. ACS Chem Biol (2011) 6:229–33. doi: 10.1021/cb100248e
27. Wu G, Fan L, Edmonson MN, Shaw T, Boggs K, Easton J, et al. Inhibition of SF3B1 by molecules targeting the spliceosome results in massive aberrant exon skipping. RNA (2018) 24:1056–66. doi: 10.1261/rna.065383.117
28. Bauman J, Jearawiriyapaisarn N, Kole R. Therapeutic potential of splice-switching oligonucleotides. Oligonucleotides (2009) 19:1–13. doi: 10.1089/oli.2008.0161
29. Denichenko P, Mogilevsky M, Clery A, Welte T, Biran J, Shimshon O, et al. Specific inhibition of splicing factor activity by decoy RNA oligonucleotides. Nat Commun (2019) 10:1590. doi: 10.1038/s41467-019-09523-0
30. Singh S, Quarni W, Goralski M, Wan S, Jin H, Van de Velde LA, et al. Targeting the spliceosome through RBM39 degradation results in exceptional responses in high-risk neuroblastoma models. Sci Adv (2021) 7:eabj5405. doi: 10.1126/sciadv.abj5405
31. Koh CM, Bezzi M, Low DH, Ang WX, Teo SX, Gay FP, et al. MYC regulates the core pre-mRNA splicing machinery as an essential step in lymphomagenesis. Nature (2015) 523:96–100. doi: 10.1038/nature14351
32. Hsu TY, Simon LM, Neill NJ, Marcotte R, Sayad A, Bland CS, et al. The spliceosome is a therapeutic vulnerability in MYC-driven cancer. Nature (2015) 525:384–8. doi: 10.1038/nature14985
33. Pardi N, Hogan MJ, Porter FW, Weissman D. mRNA vaccines - a new era in vaccinology. Nat Rev Drug Discovery (2018) 17:261–79. doi: 10.1038/nrd.2017.243
34. Wagner J, Wickman E, Shaw TI, Anido AA, Langfitt D, Zhang J, et al. Antitumor effects of CAR T cells redirected to the EDB splice variant of fibronectin. Cancer Immunol Res (2021) 9:279–90. doi: 10.1158/2326-6066.CIR-20-0280
35. Wang Z, Gerstein M, Snyder M. RNA-Seq: a revolutionary tool for transcriptomics. Nat Rev Genet (2009) 10:57–63. doi: 10.1038/nrg2484
36. Li B, Dewey CN. RSEM: accurate transcript quantification from RNA-seq data with or without a reference genome. BMC Bioinf (2011) 12:323. doi: 10.1186/1471-2105-12-323
37. Kim D, Langmead B, Salzberg SL. HISAT: a fast spliced aligner with low memory requirements. Nat Methods (2015) 12:357–60. doi: 10.1038/nmeth.3317
38. Bray NL, Pimentel H, Melsted P, Pachter L. Near-optimal probabilistic RNA-seq quantification. Nat Biotechnol (2016) 34:525–7. doi: 10.1038/nbt.3519
39. Patro R, Duggal G, Love MI, Irizarry RA, Kingsford C. Salmon provides fast and bias-aware quantification of transcript expression. Nat Methods (2017) 14:417–9. doi: 10.1038/nmeth.4197
40. Teng M, Love MI, Davis CA, Djebali S, Dobin A, Graveley BR, et al. A benchmark for RNA-seq quantification pipelines. Genome Biol (2016) 17:74. doi: 10.1186/s13059-016-0940-1
41. Ma C, Kingsford C. Detecting, categorizing, and correcting coverage anomalies of RNA-seq quantification. Cell Syst (2019) 9:589–99.e7. doi: 10.1016/j.cels.2019.10.005
42. Love MI, Hogenesch JB, Irizarry RA. Modeling of RNA-seq fragment sequence bias reduces systematic errors in transcript abundance estimation. Nat Biotechnol (2016) 34:1287–91. doi: 10.1038/nbt.3682
43. Venables JP, Klinck R, Bramard A, Inkel L, Dufresne-Martin G, Koh C, et al. Identification of alternative splicing markers for breast cancer. Cancer Res (2008) 68:9525–31. doi: 10.1158/0008-5472.CAN-08-1769
44. Katz Y, Wang ET, Airoldi EM, Burge CB. Analysis and design of RNA sequencing experiments for identifying isoform regulation. Nat Methods (2010) 7:1009–15. doi: 10.1038/nmeth.1528
45. Shen S, Park JW, Lu ZX, Lin L, Henry MD, Wu YN, et al. rMATS: robust and flexible detection of differential alternative splicing from replicate RNA-seq data. Proc Natl Acad Sci U S A (2014) 111:E5593–601. doi: 10.1073/pnas.1419161111
46. Vaquero-Garcia J, Barrera A, Gazzara MR, Gonzalez-Vallinas J, Lahens NF, Hogenesch JB, et al. A new view of transcriptome complexity and regulation through the lens of local splicing variations. Elife (2016) 5:e11752. doi: 10.7554/eLife.11752
47. Kahles A, Ong CS, Zhong Y, Ratsch G. SplAdder: identification, quantification and testing of alternative splicing events from RNA-seq data. Bioinformatics (2016) 32:1840–7. doi: 10.1093/bioinformatics/btw076
48. Li YI, Knowles DA, Humphrey J, Barbeira AN, Dickinson SP, Im HK, et al. Annotation-free quantification of RNA splicing using LeafCutter. Nat Genet (2018) 50:151–8. doi: 10.1038/s41588-017-0004-9
49. Bai B, Hales CM, Chen PC, Gozal Y, Dammer EB, Fritz JJ, et al. U1 small nuclear ribonucleoprotein complex and RNA splicing alterations in alzheimer's disease. Proc Natl Acad Sci U.S.A. (2013) 110:16562–7. doi: 10.1073/pnas.1310249110
50. Byrne A, Beaudin AE, Olsen HE, Jain M, Cole C, Palmer T, et al. Nanopore long-read RNAseq reveals widespread transcriptional variation among the surface receptors of individual b cells. Nat Commun (2017) 8:16027. doi: 10.1038/ncomms16027
51. Karlsson K, Linnarsson S. Single-cell mRNA isoform diversity in the mouse brain. BMC Genomics (2017) 18:126. doi: 10.1186/s12864-017-3528-6
52. Amarasinghe SL, Su S, Dong X, Zappia L, Ritchie ME, Gouil Q. Opportunities and challenges in long-read sequencing data analysis. Genome Biol (2020) 21:30. doi: 10.1186/s13059-020-1935-5
53. Tilgner H, Jahanbani F, Blauwkamp T, Moshrefi A, Jaeger E, Chen F, et al. Comprehensive transcriptome analysis using synthetic long-read sequencing reveals molecular co-association of distant splicing events. Nat Biotechnol (2015) 33:736–42. doi: 10.1038/nbt.3242
54. Gupta I, Collier PG, Haase B, Mahfouz A, Joglekar A, Floyd T, et al. Single-cell isoform RNA sequencing characterizes isoforms in thousands of cerebellar cells. Nat Biotechnol (2018) 36(12):1197–202. doi: 10.1038/nbt.4259
55. Adhikari S, Nice EC, Deutsch EW, Lane L, Omenn GS, Pennington SR, et al. A high-stringency blueprint of the human proteome. Nat Commun (2020) 11:5301. doi: 10.1038/s41467-020-19045-9
56. Omenn GS, Lane L, Lundberg EK, Overall CM, Deutsch EW. Progress on the HUPO draft human proteome: 2017 metrics of the human proteome project. J Proteome Res (2017) 16:4281–7. doi: 10.1021/acs.jproteome.7b00375
57. Mani DR, Krug K, Zhang B, Satpathy S, Clauser KR, Ding L, et al. Cancer proteogenomics: current impact and future prospects. Nat Rev Cancer (2022) 22:298–313. doi: 10.1038/s41568-022-00446-5
58. Ruggles KV, Krug K, Wang X, Clauser KR, Wang J, Payne SH, et al. Methods, tools and current perspectives in proteogenomics. Mol Cell Proteomics (2017) 16:959–81. doi: 10.1074/mcp.MR117.000024
59. Sheynkman GM, Johnson JE, Jagtap PD, Shortreed MR, Onsongo G, Frey BL, et al. Using galaxy-p to leverage RNA-seq for the discovery of novel protein variations. BMC Genomics (2014) 15:703. doi: 10.1186/1471-2164-15-703
60. Jiang L, Wang M, Lin S, Jian R, Li X, Chan J, et al. A quantitative proteome map of the human body. Cell (2020) 183:269–83.e19. doi: 10.1016/j.cell.2020.08.036
61. Wang D, Eraslan B, Wieland T, Hallstrom B, Hopf T, Zolg DP, et al. A deep proteome and transcriptome abundance atlas of 29 healthy human tissues. Mol Syst Biol (2019) 15:e8503. doi: 10.15252/msb.20188503
62. Lau E, Han Y, Williams DR, Thomas CT, Shrestha R, Wu JC, et al. Splice-Junction-Based mapping of alternative isoforms in the human proteome. Cell Rep (2019) 29:3751–65.e5. doi: 10.1016/j.celrep.2019.11.026
63. Liu Y, Gonzalez-Porta M, Santos S, Brazma A, Marioni JC, Aebersold R, et al. Impact of alternative splicing on the human proteome. Cell Rep (2017) 20:1229–41. doi: 10.1016/j.celrep.2017.07.025
64. Pappireddi N, Martin L, Wuhr M. A review on quantitative multiplexed proteomics. Chembiochem (2019) 20:1210–24. doi: 10.1002/cbic.201800650
65. Wang ET, Sandberg R, Luo S, Khrebtukova I, Zhang L, Mayr C, et al. Alternative isoform regulation in human tissue transcriptomes. Nature (2008) 456:470–6. doi: 10.1038/nature07509
66. Djebali S, Davis CA, Merkel A, Dobin A, Lassmann T, Mortazavi A, et al. Landscape of transcription in human cells. Nature (2012) 489:101–8. doi: 10.1038/nature11233
67. Bartel DP. MicroRNAs: target recognition and regulatory functions. Cell (2009) 136:215–33. doi: 10.1016/j.cell.2009.01.002
68. Chang YF, Imam JS, Wilkinson MF. The nonsense-mediated decay RNA surveillance pathway. Annu Rev Biochem (2007) 76:51–74. doi: 10.1146/annurev.biochem.76.050106.093909
69. Wang X, Codreanu SG, Wen B, Li K, Chambers MC, Liebler DC, et al. Detection of proteome diversity resulted from alternative splicing is limited by trypsin cleavage specificity. Mol Cell Proteomics (2018) 17:422–30. doi: 10.1074/mcp.RA117.000155
70. Uzozie AC, Aebersold R. Advancing translational research and precision medicine with targeted proteomics. J Proteomics (2018) 189:1–10. doi: 10.1016/j.jprot.2018.02.021
71. Lin TT, Zhang T, Kitata RB, Liu T, Smith RD, Qian WJ, et al. Mass spectrometry-based targeted proteomics for analysis of protein mutations. Mass Spectrom Rev (2021):e21741. doi: 10.1002/mas.21741
72. Li Y, Wang X, Cho JH, Shaw TI, Wu Z, Bai B, et al. JUMPg: An integrative proteogenomics pipeline identifying unannotated proteins in human brain and cancer cells. J Proteome Res (2016) 15:2309–20. doi: 10.1021/acs.jproteome.6b00344
73. Miller RM, Jordan BT, Mehlferber MM, Jeffery ED, Chatzipantsiou C, Kaur S, et al. Enhanced protein isoform characterization through long-read proteogenomics. Genome Biol (2022) 23:69. doi: 10.1186/s13059-022-02624-y
74. Buljan M, Chalancon G, Eustermann S, Wagner GP, Fuxreiter M, Bateman A, et al. Tissue-specific splicing of disordered segments that embed binding motifs rewires protein interaction networks. Mol Cell (2012) 46:871–83. doi: 10.1016/j.molcel.2012.05.039
75. Davey NE. The functional importance of structure in unstructured protein regions. Curr Opin Struct Biol (2019) 56:155–63. doi: 10.1016/j.sbi.2019.03.009
76. Lee KH, Zhang P, Kim HJ, Mitrea DM, Sarkar M, Freibaum BD, et al. C9orf72 dipeptide repeats impair the assembly, dynamics, and function of membrane-less organelles. Cell (2016) 167:774–788.e17. doi: 10.1016/j.cell.2016.10.002
77. Zou H, Pan T, Gao Y, Chen R, Li S, Guo J, et al. Pan-cancer assessment of mutational landscape in intrinsically disordered hotspots reveals potential driver genes. Nucleic Acids Res (2022) 50:e49. doi: 10.1093/nar/gkac028
78. Prilusky J, Felder CE, Zeev-Ben-Mordehai T, Rydberg EH, Man O, Beckmann JS, et al. FoldIndex: a simple tool to predict whether a given protein sequence is intrinsically unfolded. Bioinformatics (2005) 21:3435–8. doi: 10.1093/bioinformatics/bti537
79. Erdos G, Pajkos M, Dosztanyi Z. IUPred3: prediction of protein disorder enhanced with unambiguous experimental annotation and visualization of evolutionary conservation. Nucleic Acids Res (2021) 49:W297–303. doi: 10.1093/nar/gkab408
80. Linding R, Jensen LJ, Diella F, Bork P, Gibson TJ, Russell RB. Protein disorder prediction: implications for structural proteomics. Structure (2003) 11:1453–9. doi: 10.1016/j.str.2003.10.002
81. Jones DT, Cozzetto D. DISOPRED3: precise disordered region predictions with annotated protein-binding activity. Bioinformatics (2015) 31:857–63. doi: 10.1093/bioinformatics/btu744
82. Hanson J, Paliwal KK, Litfin T, Zhou Y. SPOT-Disorder2: Improved protein intrinsic disorder prediction by ensembled deep learning. Genomics Proteomics Bioinf (2019) 17:645–56. doi: 10.1016/j.gpb.2019.01.004
83. Hu G, Katuwawala A, Wang K, Wu Z, Ghadermarzi S, Gao J, et al. flDPnn: Accurate intrinsic disorder prediction with putative propensities of disorder functions. Nat Commun (2021) 12:4438. doi: 10.1038/s41467-021-24773-7
84. Ishida T, Kinoshita K. Prediction of disordered regions in proteins based on the meta approach. Bioinformatics (2008) 24:1344–8. doi: 10.1093/bioinformatics/btn195
85. Necci M, Piovesan D, Dosztanyi Z, Tosatto SCE. MobiDB-lite: fast and highly specific consensus prediction of intrinsic disorder in proteins. Bioinformatics (2017) 33:1402–4. doi: 10.1093/bioinformatics/btx015
86. Oldfield CJ, Fan X, Wang C, Dunker AK, Kurgan L. Computational prediction of intrinsic disorder in protein sequences with the disCoP meta-predictor. Methods Mol Biol (2020) 2141:21–35. doi: 10.1007/978-1-0716-0524-0_2
87. Buljan M, Chalancon G, Dunker AK, Bateman A, Balaji S, Fuxreiter M, et al. Alternative splicing of intrinsically disordered regions and rewiring of protein interactions. Curr Opin Struct Biol (2013) 23:443–50. doi: 10.1016/j.sbi.2013.03.006
88. Gwon Y, Maxwell BA, Kolaitis RM, Zhang P, Kim HJ, Taylor JP. Ubiquitination of G3BP1 mediates stress granule disassembly in a context-specific manner. Sci 372 (2021) 372(6549):eabf6548. doi: 10.1126/science.abf6548
89. Marti-Solano M, Crilly SE, Malinverni D, Munk C, Harris M, Pearce A, et al. Combinatorial expression of GPCR isoforms affects signalling and drug responses. Nature (2020) 587:650–6. doi: 10.1038/s41586-020-2888-2
90. Fonin AV, Darling AL, Kuznetsova IM, Turoverov KK, Uversky VN. Multi-functionality of proteins involved in GPCR and G protein signaling: making sense of structure-function continuum with intrinsic disorder-based proteoforms. Cell Mol Life Sci (2019) 76:4461–92. doi: 10.1007/s00018-019-03276-1
91. Lappano R, Maggiolini M. G Protein-coupled receptors: novel targets for drug discovery in cancer. Nat Rev Drug Discovery (2011) 10:47–60. doi: 10.1038/nrd3320
92. Zhang B, Li S, Shui W. Post-translational modifications of G protein-coupled receptors revealed by proteomics and structural biology. Front Chem (2022) 10:843502. doi: 10.3389/fchem.2022.843502
93. Markovic D, Challiss RA. Alternative splicing of G protein-coupled receptors: physiology and pathophysiology. Cell Mol Life Sci (2009) 66:3337–52. doi: 10.1007/s00018-009-0093-4
94. Chaudhary PK, Kim S. An insight into GPCR and G-proteins as cancer drivers. Cells (2021) 10. doi: 10.3390/cells10123288
95. Gad AA, Balenga N. The emerging role of adhesion GPCRs in cancer. ACS Pharmacol Transl Sci (2020) 3:29–42. doi: 10.1021/acsptsci.9b00093
96. Schoneberg T, Liebscher I. Mutations in G protein-coupled receptors: Mechanisms, pathophysiology and potential therapeutic approaches. Pharmacol Rev (2021) 73:89–119. doi: 10.1124/pharmrev.120.000011
97. Venkatakrishnan AJ, Flock T, Prado DE, Oates ME, Gough J, Madan Babu M. Structured and disordered facets of the GPCR fold. Curr Opin Struct Biol (2014) 27:129–37. doi: 10.1016/j.sbi.2014.08.002
98. Guillien M, Mouhand A, Fournet A, Gontier A, Marti Navia A, Cordeiro TN, et al. Structural insights into the intrinsically disordered GPCR c-terminal region, major actor in arrestin-GPCR interaction. Biomolecules (2022) 12. doi: 10.3390/biom12050617
99. Schihada H, Klompstra TM, Humphrys LJ, Cervenka I, Dadvar S, Kolb P, et al. Isoforms of GPR35 have distinct extracellular n-termini that allosterically modify receptor-transducer coupling and mediate intracellular pathway bias. J Biol Chem (2022) 298:102328. doi: 10.1016/j.jbc.2022.102328
100. Zhang J, Yang J, Jang R, Zhang Y. GPCR-I-TASSER: A hybrid approach to G protein-coupled receptor structure modeling and the application to the human genome. Structure (2015) 23:1538–49. doi: 10.1016/j.str.2015.06.007
101. de Felice A, Aureli S, Limongelli V. Drug repurposing on G protein-coupled receptors using a computational profiling approach. Front Mol Biosci (2021) 8:673053. doi: 10.3389/fmolb.2021.673053
102. Ahmed M, Hasani HJ, Kalyaanamoorthy S, Barakat K. GPCR_LigandClassify.py; a rigorous machine learning classifier for GPCR targeting compounds. Sci Rep (2021) 11:9510. doi: 10.1038/s41598-021-88939-5
103. Seo S, Choi J, Ahn SK, Kim KW, Kim J, Choi J, et al. Prediction of GPCR-ligand binding using machine learning algorithms. Comput Math Methods Med (2018) 2018:6565241. doi: 10.1155/2018/6565241
104. Jana S, Ghosh S, Muk S, Levy B, Vaidehi N. Prediction of conformation specific thermostabilizing mutations for class a G protein-coupled receptors. J Chem Inf Model (2019) 59:3744–54. doi: 10.1021/acs.jcim.9b00175
105. Singh G, Inoue A, Gutkind JS, Russell RB, Raimondi F. PRECOG: PREdicting COupling probabilities of G-protein coupled receptors. Nucleic Acids Res (2019) 47:W395–401. doi: 10.1093/nar/gkz392
106. Urban DJ, Roth BL. DREADDs (designer receptors exclusively activated by designer drugs): chemogenetic tools with therapeutic utility. Annu Rev Pharmacol Toxicol (2015) 55:399–417. doi: 10.1146/annurev-pharmtox-010814-124803
107. Niklas KJ, Bondos SE, Dunker AK, Newman SA. Rethinking gene regulatory networks in light of alternative splicing, intrinsically disordered protein domains, and post-translational modifications. Front Cell Dev Biol (2015) 3:8. doi: 10.3389/fcell.2015.00008
108. Berman E, Jhanwar S, Hedvat C, Arcila ME, Wahab OA, Levine R, et al. Resistance to imatinib in patients with chronic myelogenous leukemia and the splice variant BCR-ABL1(35INS). Leuk Res (2016) 49:108–12. doi: 10.1016/j.leukres.2016.08.006
109. Sahadevan K, Darby S, Leung HY, Mathers ME, Robson CN, Gnanapragasam VJ. Selective over-expression of fibroblast growth factor receptors 1 and 4 in clinical prostate cancer. J Pathol (2007) 213:82–90. doi: 10.1002/path.2205
110. Yochum ZA, Villaruz LC. Alternative splicing of HER2: a novel mediator of EGFR TKI resistance. Transl Lung Cancer Res (2020) 9:1606–12. doi: 10.21037/tlcr-20-618
111. Wang D, Liu D, Yuchi J, He F, Jiang Y, Cai S, et al. MusiteDeep: a deep-learning based webserver for protein post-translational modification site prediction and visualization. Nucleic Acids Res (2020) 48:W140–6. doi: 10.1093/nar/gkaa275
112. Yang Y, Wang H, Ding J, Xu Y. iAcet-sumo: Identification of lysine acetylation and sumoylation sites in proteins by multi-class transformation methods. Comput Biol Med (2018) 100:144–51. doi: 10.1016/j.compbiomed.2018.07.006
113. Wang C, Xu H, Lin S, Deng W, Zhou J, Zhang Y, et al. GPS 5.0: An update on the prediction of kinase-specific phosphorylation sites in proteins. Genomics Proteomics Bioinf (2020) 18:72–80. doi: 10.1016/j.gpb.2020.01.001
114. Cha SE, Kujawski M, Yazaki PJ, Brown C, Shively JE. Tumor regression and immunity in combination therapy with anti-CEA chimeric antigen receptor T cells and anti-CEA-IL2 immunocytokine. Oncoimmunology (2021) 10:1899469. doi: 10.1080/2162402X.2021.1899469
115. Kato D, Yaguchi T, Iwata T, Katoh Y, Morii K, Tsubota K, et al. GPC1 specific CAR-T cells eradicate established solid tumor without adverse effects and synergize with anti-PD-1 ab. Elife (2020) 9. doi: 10.7554/eLife.49392
116. Heitzeneder S, Bosse KR, Zhu Z, Zhelev D, Majzner RG, Radosevich MT, et al. GPC2-CAR T cells tuned for low antigen density mediate potent activity against neuroblastoma without toxicity. Cancer Cell (2022) 40:53–69.e9. doi: 10.1016/j.ccell.2021.12.005
117. Shi D, Shi Y, Kaseb AO, Qi X, Zhang Y, Chi J, et al. Chimeric antigen receptor-Glypican-3 T-cell therapy for advanced hepatocellular carcinoma: Results of phase I trials. Clin Cancer Res (2020) 26:3979–89. doi: 10.1158/1078-0432.CCR-19-3259
118. Rodriguez-Garcia A, Lynn RC, Poussin M, Eiva MA, Shaw LC, O'Connor RS, et al. CAR-T cell-mediated depletion of immunosuppressive tumor-associated macrophages promotes endogenous antitumor immunity and augments adoptive immunotherapy. Nat Commun (2021) 12:877. doi: 10.1038/s41467-021-20893-2
119. Le Q, Castro S, Tang T, Loeb AM, Hylkema T, McKay CN, et al. Therapeutic targeting of mesothelin with chimeric antigen receptor T cells in acute myeloid leukemia. Clin Cancer Res (2021) 27:5718–30. doi: 10.1158/1078-0432.CCR-21-1546
120. Fankhauser N, Maser P. Identification of GPI anchor attachment signals by a kohonen self-organizing map. Bioinformatics (2005) 21:1846–52. doi: 10.1093/bioinformatics/bti299
121. Poisson G, Chauve C, Chen X, Bergeron A. FragAnchor: a large-scale predictor of glycosylphosphatidylinositol anchors in eukaryote protein sequences by qualitative scoring. Genomics Proteomics Bioinf (2007) 5:121–30. doi: 10.1016/S1672-0229(07)60022-9
122. Pierleoni A, Martelli PL, Casadio R. PredGPI: a GPI-anchor predictor. BMC Bioinf (2008) 9:392. doi: 10.1186/1471-2105-9-392
123. Paronetto MP, Passacantilli I, Sette C. Alternative splicing and cell survival: from tissue homeostasis to disease. Cell Death Differ (2016) 23:1919–29. doi: 10.1038/cdd.2016.91
124. Wu Q, Feng L, Wang Y, Mao Y, Di X, Zhang K, et al. Multi-omics analysis reveals RNA splicing alterations and their biological and clinical implications in lung adenocarcinoma. Signal Transduct Target Ther (2022) 7:270. doi: 10.1038/s41392-022-01098-5
125. Sherill-Rofe D, Raban O, Findlay S, Rahat D, Unterman I, Samiei A, et al. Multi-omics data integration analysis identifies the spliceosome as a key regulator of DNA double-strand break repair. NAR Cancer (2022) 4:zcac013. doi: 10.1093/narcan/zcac013
126. Glinos DA, Garborcauskas G, Hoffman P, Ehsan N, Jiang L, Gokden A, et al. Transcriptome variation in human tissues revealed by long-read sequencing. Nature (2022) 608:353–9. doi: 10.1038/s41586-022-05035-y
127. G.T. Consortium. The GTEx consortium atlas of genetic regulatory effects across human tissues. Science (2020) 369:1318–30. doi: 10.1126/science.aaz1776
128. Obeng EA, Chappell RJ, Seiler M, Chen MC, Campagna DR, Schmidt PJ, et al. Physiologic expression of Sf3b1(K700E) causes impaired erythropoiesis, aberrant splicing, and sensitivity to therapeutic spliceosome modulation. Cancer Cell (2016) 30:404–17. doi: 10.1016/j.ccell.2016.08.006
129. Gonatopoulos-Pournatzis T, Wu M, Braunschweig U, Roth J, Han H, Best AJ, et al. Genome-wide CRISPR-Cas9 interrogation of splicing networks reveals a mechanism for recognition of autism-misregulated neuronal microexons. Mol Cell (2018) 72:510–24.e12. doi: 10.1016/j.molcel.2018.10.008
130. Fuchs A, Riegler S, Ayatollahi Z, Cavallari N, Giono LE, Nimeth BA, et al. Targeting alternative splicing by RNAi: from the differential impact on splice variants to triggering artificial pre-mRNA splicing. Nucleic Acids Res (2021) 49:1133–51. doi: 10.1093/nar/gkaa1260
131. Tsherniak A, Vazquez F, Montgomery PG, Weir BA, Kryukov G, Cowley GS, et al. Defining a cancer dependency map. Cell (2017) 170:564–76.e16. doi: 10.1016/j.cell.2017.06.010
132. Behan FM, Iorio F, Picco G, Goncalves E, Beaver CM, Migliardi G, et al. Prioritization of cancer therapeutic targets using CRISPR-Cas9 screens. Nature (2019) 568:511–6. doi: 10.1038/s41586-019-1103-9
Keywords: proteomics, transcriptomics, alternative splicing, biomarker, therapeutic discovery
Citation: Shaw TI, Zhao B, Li Y, Wang H, Wang L, Manley B, Stewart PA and Karolak A (2022) Multi-omics approach to identifying isoform variants as therapeutic targets in cancer patients. Front. Oncol. 12:1051487. doi: 10.3389/fonc.2022.1051487
Received: 22 September 2022; Accepted: 07 November 2022;
Published: 24 November 2022.
Edited by:
Erik Wiemer, Erasmus Medical Center, NetherlandsReviewed by:
Abdullah Kahraman, University of Applied Sciences and Arts Northwestern Switzerland, SwitzerlandCopyright © 2022 Shaw, Zhao, Li, Wang, Wang, Manley, Stewart and Karolak. This is an open-access article distributed under the terms of the Creative Commons Attribution License (CC BY). The use, distribution or reproduction in other forums is permitted, provided the original author(s) and the copyright owner(s) are credited and that the original publication in this journal is cited, in accordance with accepted academic practice. No use, distribution or reproduction is permitted which does not comply with these terms.
*Correspondence: Timothy I. Shaw, dGltb3RoeS5zaGF3QG1vZmZpdHQub3Jn
†These authors have contributed equally to this work
Disclaimer: All claims expressed in this article are solely those of the authors and do not necessarily represent those of their affiliated organizations, or those of the publisher, the editors and the reviewers. Any product that may be evaluated in this article or claim that may be made by its manufacturer is not guaranteed or endorsed by the publisher.
Research integrity at Frontiers
Learn more about the work of our research integrity team to safeguard the quality of each article we publish.