- Department of Radiation Oncology, National Cancer Center/National Clinical Research Center for Cancer/Cancer Hospital, Chinese Academy of Medical Sciences and Peking Union Medical College, Beijing, China
Purpose: This study proposes a method to identify plans that failed patient-specific quality assurance (QA) and attempts to establish a criterion to prescreen treatment plans for patient-specific QA measurements with independent Monte Carlo dose calculations.
Materials and methods: Patient-specific QA results measured with an ArcCHECK diode array of 207 patients (head and neck: 25; thorax: 61; abdomen: 121) were retrospectively analyzed. All patients were treated with the volumetric modulated arc therapy (VMAT) technique and plans were optimized with a Pinnacle v16.2 treatment planning system using an analytical algorithm-based dose engine. Afterwards, phantom verification plans were designed and recalculated by an independent GPU-accelerated Monte Carlo (MC) dose engine, ArcherQA. Moreover, sensitivity and specificity analyzes of gamma passing rates between measurements and MC calculations were carried out to show the ability of MC to monitor failing plans (ArcCHECK 3%/3 mm,<90%), and attempt to determine the appropriate threshold and gamma passing rate criterion utilized by ArcherQA to prescreen treatment plans for ArcCHECK measurements. The receiver operator characteristic (ROC) curve was also utilized to characterize the performance of different gamma passing rate criterion used by ArcherQA.
Results: The thresholds for 100% sensitivity to detect plans that failed patient-specific QA by independent calculation were 97.0%, 95.4%, and 91.0% for criterion 3%/3 mm, 3%/2 mm, and 2%/2 mm, respectively, which corresponded to specificities of 0.720, 0.528, and 0.585, respectively. It was shown that the 3%/3 mm criterion with 97% threshold for ArcherQA demonstrated perfect sensitivity and the highest specificity compared with other criteria, which may be suitable for prescreening treatment plans treated with the investigated machine to implement measurement-based patient-specific QA of patient plans. In addition, the area under the curve (AUC) calculated from ROC analysis for criterion 3%/3 mm, 3%/2 mm, and 2%/2 mm used by ArcherQA were 0.948, 0.924, and 0.929, respectively.
Conclusions: Independent dose calculation with the MC-based program ArcherQA has potential as a prescreen treatment for measurement-based patient-specific QA. AUC values (>0.9) showed excellent classification accuracy for monitoring failing plans with independent MC calculations.
1 Introduction
The accuracy of radiotherapy is crucial to its therapeutic effects on patients. Patient-specific QA is an important standard process to identify discrepancies between the dose calculated by a treatment planning system (TPS) and that delivered by the treatment machine (1–3). For two-dimensional (2D) or three-dimensional (3D) conformal radiation therapy, the shaped fields are relatively simple to deliver and are commissioned closely to an accurate TPS model. With the introduction of intensity-modulated radiotherapy (IMRT) and volumetric modulated arc radiotherapy (VMAT), the complexity of plans has increased markedly with the different degree of modulation (4, 5). Hence, robust dosimetric verification of small fields is required to validate whether the small-field modeling is sufficiently accurate and close to the delivered dose (6). Moreover, dynamic treatments (movable multi-leaf collimator, gantry or variable intensity during beam delivering) also need to accurately verify whether or not IMRT or VMAT plans are physically achievable (3). Therefore, pretreatment patient-specific QA of treatment plans has been recommended by many reports and professional organizations to ensure safety and to find any possible clinically related errors (7–9).
Patient-specific QA can be divided into two categories: measurement-based QA and software calculation. There are many methods for measurement-based patient-specific QA, such as point dose measurements, planar dose measurements, or three-dimensional measurements with numerous devices, like ion chambers (10), films (11), diode arrays (12), or electronic portal imaging devices (EPIDs) (13), etc. However, these measurement processes are known to be time-consuming and tedious, while occupying precious machine time. Software calculation is another common approach for patient-specific QA by exporting DICOM files (14, 15) from TPS or log files (16), etc., from a treatment machine to independent programs to recalculate dose or monitor unit (MU) with different algorithms. Apparently, automated software calculation is a more effective way for patient-specific QA compared to measurements. It was reported that independent computer calculations might take the place of measurements by analyzing point dose data using a statistical process control (17). (Sochi et al.) analyzed 100 IMRT plans and concluded that an in-house independent dose calculation algorithm performed better than film measurements in predicting the plan disagreement (18). (Cry et al.) showed that the independent calculation was more sensitive in detecting failing plans than measurement-based QA (19). The good performance of independent calculation may be because the independent calculation is able to decouple the TPS errors and machine QA (18). It was reported that few TPS errors could be discovered by measurement-based patient-specific QA (20, 21) and most problems came from the delivering system, which should be settled by machine QA (22). Hence, it is debated whether or not measurement-based patient-specific QA should be replaced with independent calculation (23, 24). In a recent AAPM report, TG 219 published in October 2021, it was recommended to implement a secondary dose calculation or an MU check for every IMRT/VMAT plan (25). Nevertheless, measurement-based patient-specific QA is still widely utilized as a standard in most radiation therapy institutions and there are limitations for software to find problems in beam delivery before patient treatment.
This study proposes a method for monitoring plans that fail patient-specific QA with independent dose calculation and attempts to establish a criterion to prescreen treatment plans for patient-specific QA measurements. This proposed method combines the advantages of independent calculation for high accuracy, high efficiency, and no treatment machine time occupation with the merits of measurements to validate beam delivery before patient treatment and avoid serious errors previously reported by the New York Times (26). To exclude the impact of management of heterogeneities by different algorithms (27), independent dose calculations were carried out using phantom verification plans to increase detection sensitivity for failing plans measured with a phantom. An ArcCHECK (Sun Nuclear Corporation, Melbourne, USA) diode array was utilized for measurement-based dose verification. Numerous in-house or commercial programs have been developed for independent dose calculation with either analytical algorithm or MC code (25). It is well known that the MC method is regarded as the gold standard in dose calculation. Several commercial vendors provide MC-based independent dose calculation software, such as SciMoCa (Scientific RT GmbH, Munich, Germany), VERIQA (PTW Freiburg, Germany), and ArcherQA (Wisdom Technology Company Limited, Hefei, China). ArcherQA is a GPU-accelerated MC dose engine that provides 3D dose calculation and specific beam modeling (28). Accuracy and high speed of ArcherQA have been demonstrated in several publications (29, 30). In this study, ArcherQA was applied to perform independent dose calculations and attempt to detect failing plans from the ArcCHECK measurements.
2 Materials and methods
2.1 Patient and plan information
This study was approved by the institutional review ethical board and informed consent was waived. A total of 207 patients (head and neck: 25; thorax: 61; abdomen: 121) were enrolled retrospectively in this study. All patients were treated with a 6-MV photon beam delivered by an Elekta Versa HD accelerator (Elekta AB, Stockholm, Sweden) in flattering filter-free mode. The VMAT technique was used for optimization with the Pinnacle TPS (version 16.2, Philips Healthcare, Eindhoven, Netherlands). The dose calculation algorithm was set as an adaptive convolve dose engine in the TPS.
2.2 Prescreening treatment plan workflow for patient-specific QA measurements with independent MC calculations
The workflow for prescreening treatment plans with MC is shown in Figure 1. Patient plans were initially optimized with the TPS. Phantom verification plans were then designed by recalculating dose distribution with the phantom image in the TPS and exported to ArcherQA to recalculate the dose distribution with the MC algorithm. Then, 3D gama analysis was carried out between doses computed with the TPS and MC. If the gamma passing rate of a plan is higher than a specific threshold, it means that the plan is acceptable (pass) for treatment; otherwise, further measurement is needed to validate the plan.
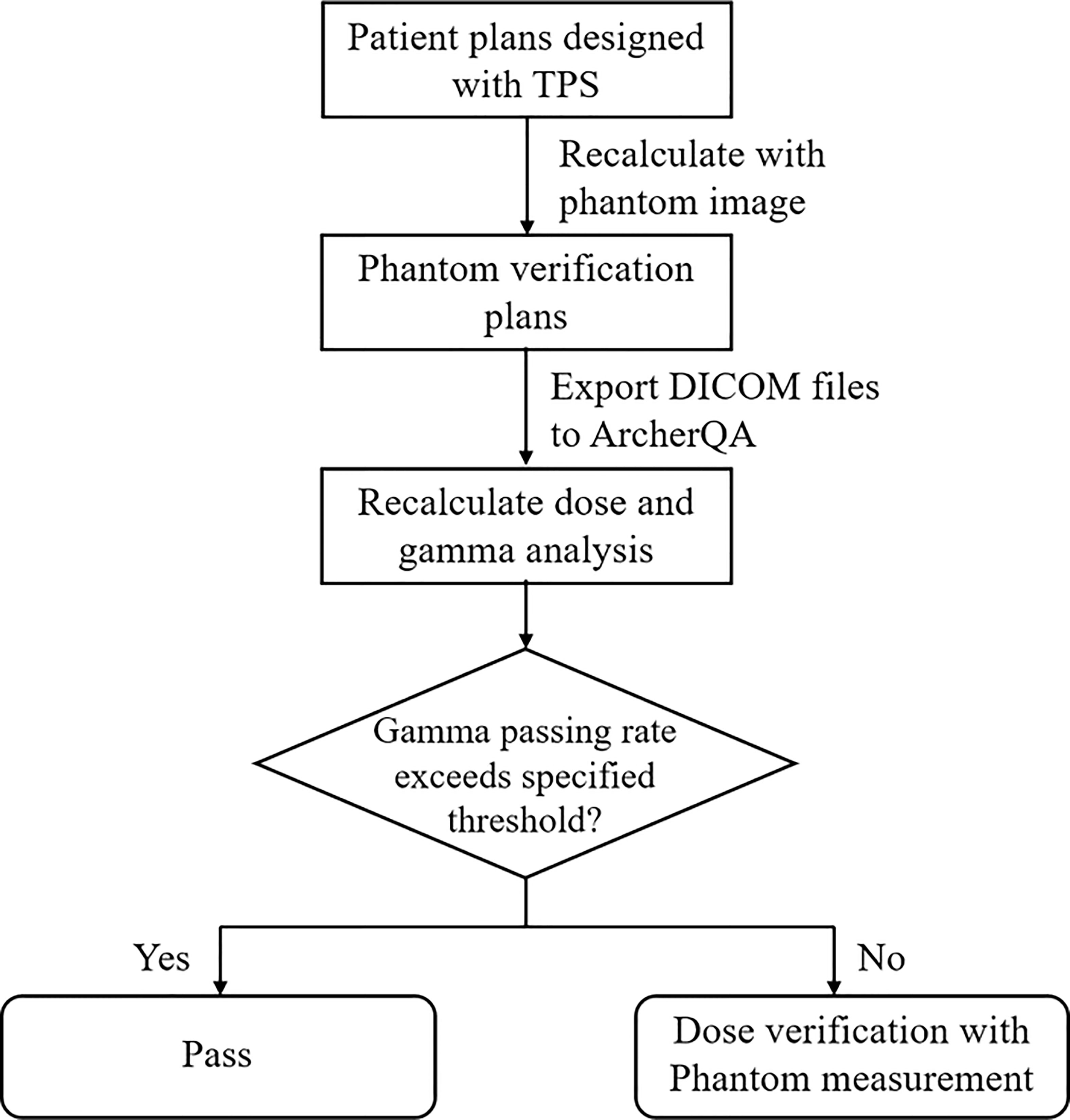
Figure 1 The workflow of pre-screening treatment plans for patient-specific QA measurement with ArcherQA computed with the TPS and MC. If the gamma passing rate of a plan is higher than a specific threshold, it means that the plan is acceptable (pass) for treatment; otherwise, further measurement is needed to validate the plan.
2.3 Phantom measurements
Each patient plan was recalculated on a 3D diode array ArcCHECK phantom (Sun Nuclear Corporation, Melbourne, USA) with a dose grid of 0.2 cm × 0.2 cm × 0.2 cm. The measurement data was collected with SNC patient software (version 8.2, Sun Nuclear Corporation, Melbourne, USA). The gamma passing rate was computed for evaluating dose discrepancies between the TPS and phantom measurements. The accelerator Elekta Versa HD in this study was a relatively old machine commissioned and installed in 2016 before publication of AAPM TG-218 report (31). Therefore, a dose difference of 3% and distance-to-agreement of 3 mm were chosen as the criterion with a threshold of 10% (following AAPM report TG 119) (32). A gamma passing rate lower than 90% was recognized as failing.
2.4 Independent MC dose calculation
2.4.1 Software
The commercial MC dose engine ArcherQA (Wisdom Technology Company Limited, Hefei, China) was utilized for independent dose calculation. Electron–photon coupled transport was simulated with MC code accelerated by GPU (29). ArcherQA can perform 3D dose calculations and provide specific beam modeling and commissioning for users. The software was installed in a standard PC (Configuration: Intel Core i7–11700 @2.5 GHz, RAM 24 G, GPU NVIDIA TITAN V; Memory 12G). The calculation time was approximately one min for a head and neck dual arc VMAT plan.
2.4.2 Beam modeling and phantom verification
Specific beam modeling was carried out for the Elekta Versa HD accelerator using data measured with a water tank phantom (PDD, profiles, output factors, etc.). The details for beam modeling was introduced before (28, 29). The modeling was also commissioned and validated with phantom measurement results. Forty-two phantom verification plans were recalculated with ArcherQA and the RT dose files were imported to the SNC patient software to compare with ArcCHECK measurement results. The commissioning aimed to improve the gamma passing rates between ArcherQA and ArcCHECK measurement for all these plans.
2.4.3 Calculation
All 207 phantom verification plans (RT plan, RT structure, RT dose, and CT image) were imported to ArcherQA to recalculate the dose distribution with the MC algorithm. 3D gamma analysis was carried out for comparison of doses calculated by the TPS and MC algorithm with variable criteria: 3%/3 mm, 3%/2 mm and 2%/2 mm (threshold = 10%).
2.5 Criterion for selecting treatment plans for measurement
Plans with gamma passing rates lower than the specified threshold calculated by ArcCHECK measurements or MC calculations were labeled as positive; otherwise, they were labeled as negative. For ArcCHECK measurements, the threshold was 90% under the 3%/3 mm criterion. For the MC calculation, variable thresholds were characterized with different criteria (3%/3 mm, 3%/2 mm, and 2%/2 mm). The classification accuracy was evaluated with sensitivity and specificity analyzes (19, 33–35). All plans can be divided into four categories labeled by ArcCHECK measurements and ArcherQA calculation: true positive (TP), false positive (FP), false negative (FN) and true negative (TN) as illustrated in Figure 2. Sensitivity was defined as the proportion of failing plans labeled by ArcCHECK measurements that were properly recognized as positive by MC calculation Specificity was the proportion of negative (passing) plans labeled by ArcCHECK measurements that were properly recognized as negative by MC calculation . The values of sensitivity or specificity can range from 0 to 1, and a sensitivity or specificity value close to one demonstrates high classification performance.
The threshold to select treatment plans for patient-specific QA measurements was defined as the minimum gamma passing rate to detect failing plans with 100% sensitivity. Moreover, appropriate criteria (3%/3 mm, 3%/2 mm, or 2%/2 mm) were selected with highest specificity when 100% sensitivity was achieved.
The ROC curve was used in evaluating the performance across different criteria (19, 33, 34). This value is plotted as the true positive rate (sensitivity) varies with false positive rate (1–specificity). The AUC was calculated to characterize the performance of the classifier. Generally, AUC values range from 0.5 to 1 (0.5 represents a random classification) and an AUC value close to one indicates a perfect classifier.
3 Results
3.1 MC beam modeling and phantom verification results
After commissioning, the average differences between measurements and ArcherQA calculations for profiles of different field size and angles were within ±2%. The average gamma passing rates between MC calculations and measurements for these 42 phantom verification plans were 3%/3 mm, 99.57% ± 0.64% and 3%/2 mm, 97.85% ± 1.71%, which was significantly higher than the average gamma passing rate between the TPS and ArcCHECK of 96.72% ± 3.44% (p< 0.001). These results demonstrate the high accuracy of the MC calculations.
3.2 Correlation analysis between ArcCHECK measurements and MC calculations
Correlation analysis between gamma passing rates calculated with measurements and those calculated with MC (variable criteria) of the 207 phantom verification plans were performed. There were 14 failing plans indicated by ArcCHECK measurements with a failing proportion of 6.76% in all plans.
The average gamma passing rate for ArcCHECK measurements (3%/3 mm) was 95.82% ± 3.5%, which was significantly different from the MC calculation, with 97.14% ± 3.16% for the 3%/3 mm criterion (p< 0.001), 94.38% ± 4.69% for the 3%/2 mm criterion (p< 0.001), and 90.52% ± 6.07% for the 2%/2 mm criterion (p< 0.001). The correlation analysis between gamma passing rates for ArcCHECK measurements and those obtained with MC calculations with the regression method is shown in Figure 3. Adjusted R2 values were 0.508, 0.494, and 0.498 for criterion 3%/3 mm, 3%/2 mm, and 2%/2 mm with MC calculations, respectively. Pearson’s r was calculated to be 0.714, 0.704, and 0.707 for criterion 3%/3 mm, 3%/2 mm, and 2%/2 mm with MC calculations, respectively. These results shown a strong correlation (>0.7) between measurement and MC-calculated gamma passing rates (36).
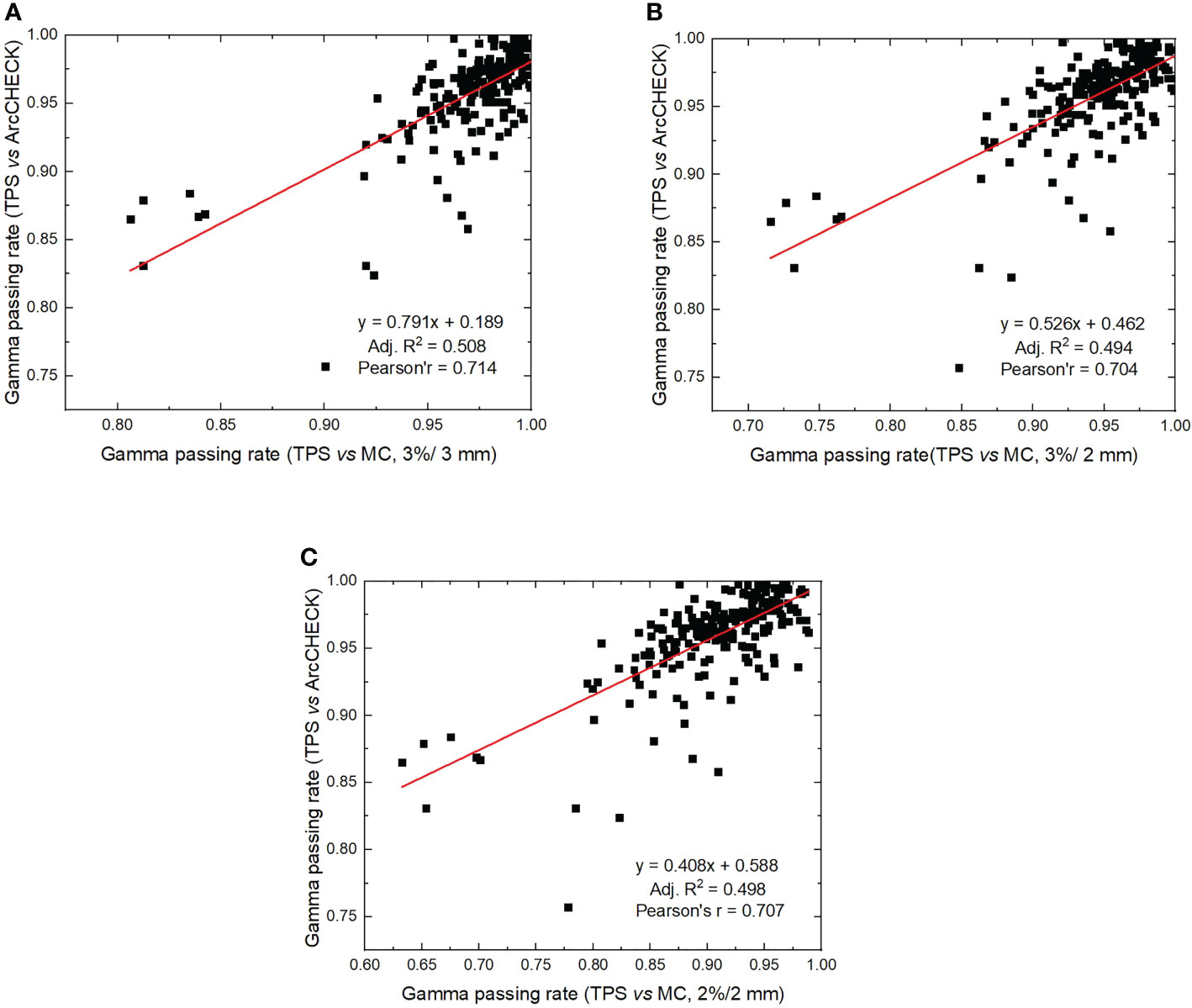
Figure 3 Correlation analysis between gamma passing rates calculated by ArcCHECK measurements (TPS vs measurements) and gamma passing rates calculated by ArcherQA (TPS vs MC). (A) 3%/3 mm criterion, (B) 3%/2 mm criterion, (C) 2%/2 mm criterion).
3.3 Criterion
The sensitivity and specificity for detecting failing plans measured with the phantom by independent MC calculation is shown in Figure 4. For 100% sensitivity, the thresholds were 97.0%, 95.4%, and 91.0% for criterion 3%/3 mm, 3%/2 mm, and 2%/2 mm with MC, respectively, which corresponds to specificities of 0.720, 0.528, and 0.585, respectively. For the MC calculation, the 3%/3 mm criterion showed the highest specificity compared with the other two criteria when 100% sensitivity was achieved. The ROC curves for variable criteria are plotted in Figure 5 and the AUC indexes were 0.948, 0.924, and 0.929 for the 3%/3 mm, 3%/2 mm, and 2%/2 mm criterion calculated with MC, respectively. It is shown that all criteria indicate excellent classification accuracy with AUC >0.9. Hence, the 3%/3 mm criterion with 97% threshold may be suitable for prescreening treatment plans with the investigated machine for patient-specific measurement-based QA.
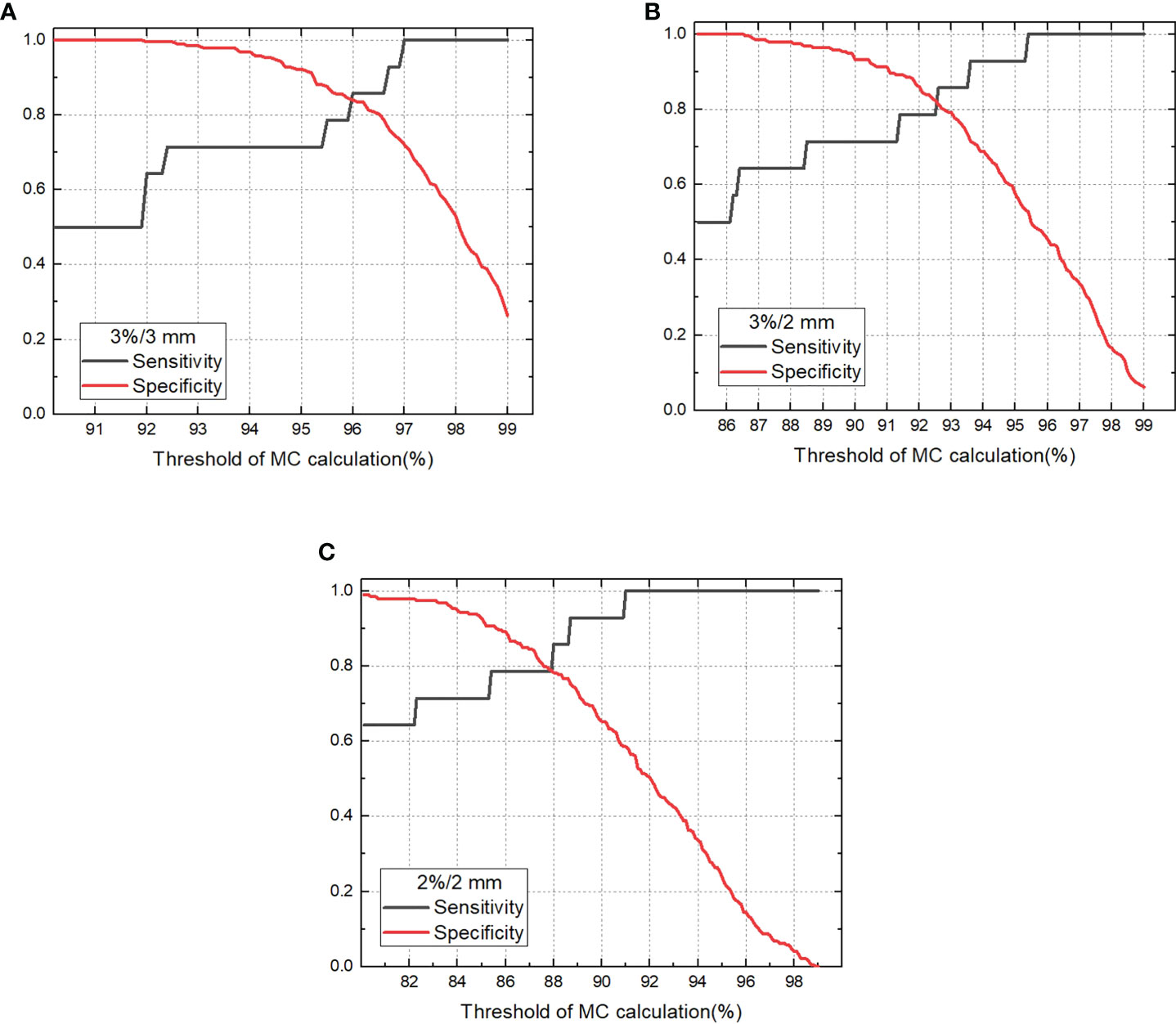
Figure 4 Sensitivity and specificity for detecting unacceptable plans vary with threshold for gamma passing rate of MC calculation (A). 3%/3 mm criterion, (B) 3%/2 mm criterion, (C) 2%/2 mm criterion).
3.4 Validation in clinic
The criterion was tested in clinic for one month. 110 clinical treatment plans were measured with ArcCHECK as well as calculated with ArcherQA using the 3%/3 mm criterion and 97% threshold. As a consequence, one plan failed with the ArcCHECK measurement and 33 plans failed MC calculations. The plan that failed the ArcCHECK measurement was also labeled as a failing plan by the MC calculation, which showed a 100% sensitivity for detecting failing plans with MC calculations. Hence, if the MC calculation was implemented for prescreening treatment plans, approximately 385 min of machine time and labor force can be saved per month for one machine without considering phantom set up time.
4 Discussions
Patient-specific QA of treatment plans has been recommended for all IMRT or VMAT plans. Practically, patient-specific QA can be implemented for all plans or sampled for selected plans (37, 38). For the sampling method, the selection criteria were either totally random or based on plenty of selection criteria, such as machine availability, plan complexity, economic factors, physicist time or preference, etc. (37). So far, there is no uniform selection criterion or explicit guidelines for radiation therapy centers to screen patients for patient-specific QA (39, 40). Measurement-based and calculation-based methods are two main strategies for the patient-specific QA of patient plans. This study attempted to establish a selection criterion to prescreen treatment plans for the measurement-based patient-specific QA of patient plans with independent MC calculations, combining advantages of both a measurement-based method and software calculations. The proposed method is more efficient than delivering all patient plans for phantom verification and can still monitor delivery problems for those plans with inferior passing rates identified by MC calculation. Furthermore, it is in accordance with recommendations in AAPM TG 218 or TG 219 that an independent dose calculation could be an effective supplement to measurement-based patient-specific QA, other than replacing it (25, 31, 32).
The prerequisite to effectively monitor failing measurement plans is the accurate modeling of independent dose calculation. If the modeling of the independent calculation is more accurate than the TPS, especially for small fields or penumbral toe and tails (23), it can be more effective for independent calculation to detect TPS errors. The MC algorithm is commonly utilized in radiation dose calculation, implementing random method in the numerical simulation of interactions between particles (41, 42). ArcherQA is a commercial MC-based dose engine that mainly simulates electron or photon transport and their interactions with other primary or secondary particles (28–30). ArcherQA was evaluated with several treatment machines and validated MC codes. The differences for the percent depth doses and axial profiles were within ±3% and ±2%, respectively, compared with the benchmarked MC code EGSnrc for the Varian TrueBeam accelerator (43). With helical tomotherapy, the gamma passing rates were 99.7%, 98.5%, and 97.2% for the prostate, lung case, and head and neck cases, respectively, compared to GEANT4 (30). In this study, beam modeling was first calibrated using water tank phantom measurements and then validated with clinical plans by ArcCHECK phantom measurements. The average gamma passing rate between ArcherQA and ArcCHECK measurements (3%/3 mm) of 42 validated plans was 99.57% ± 0.64%, which was significantly higher than the average gamma passing rate between TPS and ArcCHECK of 96.72% ± 3.44% (p< 0.001). These results shown good modeling accuracy for MC compared to the TPS, and also a prerequisite to increase accuracy for predicting ArcCHECK results with ArcherQA
With the proposed selection method in this study, it is still crucial to determine the criterion of suitable dose differences, distance-to-agreement for gamma analysis with MC, and rational threshold for passing plans. In this study, 3%/3 mm criterion with 97% threshold was determined a suitable criterion for prescreening treatment plans with the investigated accelerator. Meanwhile, if using 97% threshold for 3%/3 mm criterion, 32.85% of patients need further measurements predicted by MC, which is a relatively reasonable proportion in selecting patients for patient-specific QA. Actually, 100% sensitivity is a relatively conservative attempt to detect all failing plans indicated by phantom measurements. As reported, 80% sensitivity might also be acceptable in clinical practice (19). The criterion was also validated in clinic with 110 treatment plans; one failed plan indicated with ArcCHECK was detected by the ArcherQA calculation, which illustrated a sensitivity of 100%. The plan failing the ArcCHECK measurement was a bilateral breast radiation VMAT plan with very high complexity and modulation. It was solved by decreasing the maximum leaf-motion speed from 1.0 cm/deg to 0.5 cm/deg, and the modified plan finally passed the ArcherQA calculation as well as the ArcCHECK measurement. This was an example for ArcherQA to monitor failed plans in clinical practice.
For the measurement, it was reported that ArcCHECK also had limitations for dependencies on beam angle, direction, field size, etc. (44, 45) It was reported that some failing plans shown by ArcCHECK could be actually acceptable (passing) as indicated by ion chamber measurements (46). This may explain why a few failing plans measured with ArcCHECK have a relatively high gamma passing rate (maximum 96.9%, 3%/3 mm criterion) calculated with MC. These plans might be actually acceptable plans that were classified as failing plans due to the measurement limitations of ArcCHECK. Nevertheless, the feasibility of ArcCHECK for patient-specific QA has been demonstrated to be acceptable in many reports (44, 45) and is widely used in clinic. The accuracy of ArcCHECK is not the main concern in this study. This study mainly aimed to monitor failing plans measured with ArcCHECK with independent dose calculation and to provide a criterion to select patients for measurements. The reasonable criterion and threshold appear to vary with treatment machine, measurement device, TPS, beam modeling, and commissioning of the MC algorithm, which need to be investigated case by case. Despite the many merits of independent dose calculation, it is still recommended to implement measurement-based patient-specific QA for hypo-fractionation, SRS, or SBRT plans for safety and strict requirements for delivering (25, 31). If the sampling method of patient-specific QA of treatment plans is utilized, independent dose calculation might be considered as an effective approach to prescreen treatment plans.
5 Conclusions
The feasibility of independent dose calculations with the MC-based program ArcherQA for detecting failing plans with measurement-based patient-specific QA was demonstrated. A strong correlation (>0.7) between the gamma passing rate calculated with measurements and that calculated by MC were indicated. Meanwhile, AUC values (>0.9) showed excellent classification accuracy for monitoring failed plans with independent MC calculation. Furthermore, 100% sensitivity was achieved to detect failing plans with independent MC calculation using different criteria and the 3%/3 mm criterion with 97% threshold showed the highest specificity. This criterion and threshold may be suitable for prescreening treatment plans with the investigated machine to carry out further measurement-based QA. With supplementary independent dose calculation, patient-specific QA of patient plan procedures could be more efficient and potentially more reliable.
Data availability statement
The raw data supporting the conclusions of this article will be made available by the authors, without undue reservation.
Ethics statement
This retrospective study was approved by the review board of Cancer Hospital, Chinese Academy of Medical Sciences, and informed consent was waived.
Author contributions
YX and KZ: conception design, collected the data, data analysis. ZL and WR: dose verification with phantom measurements. BL and XM: dose calculation with software. JD and KM: supervised the study. All authors contributed to the article and approved the submitted version.
Funding
This work is supported by the National Natural Science Foundation of China (Grant No.11875320) and the Beijing Nova Program (Z201100006820058).
Acknowledgments
We thank Prof. Dr. X. George Xu, Prof. Dr. Xi Pei and Dr. Bo Cheng from University of Science and Technology of China and Anhui Wisdom Technology Company Limited for support in software and beam modelling.
Conflict of interest
The authors declare that the research was conducted in the absence of any commercial or financial relationships that could be construed as a potential conflict of interest.
Publisher’s note
All claims expressed in this article are solely those of the authors and do not necessarily represent those of their affiliated organizations, or those of the publisher, the editors and the reviewers. Any product that may be evaluated in this article, or claim that may be made by its manufacturer, is not guaranteed or endorsed by the publisher.
References
1. Schreibmann E, Dhabaan A, Elder E, Fox T. Patient-specific quality assurance method for VMAT treatment delivery. Med Phys (2009) 36(10):4530–5. doi: 10.1118/1.3213085
2. Li J, Zhang X, Li J, Jiang R, Sui J, Chan MF, et al. Impact of delivery characteristics on dose delivery accuracy of volumetric modulated arc therapy for different treatment sites. J Radiat Res (2019) 60(5):603–11. doi: 10.1093/jrr/rrz033
3. Ezzell GA, Galvin JM, Low D, Palta JR, Rosen I, Sharpe MB, et al. IMRT subcommitte; AAPM radiation therapy committee. Guidance document on delivery, treatment planning, and clinical implementation of IMRT: report of the IMRT subcommittee of the AAPM radiation therapy committee. Med Phys (2003) 30(8):2089–115.
4. Bortfeld T. IMRT: A review and preview. Phys Med Biol 51(13):R363–79. doi: 10.1088/0031-9155/51/13/R21
5. Xu Y, Xu YJ, Men K, Xiao JP, Dai JR. Application of piecewise VMAT technique to whole-brain radiotherapy with simultaneous integrated boost for multiple metastases. Radiat Oncol (2022) 17(1):86. doi: 10.1186/s13014-022-02059-6
6. Webb LK, Inness EK, Charles PH. A comparative study of three small-field detectors for patient specific stereotactic arc dosimetry. Australas Phys Eng Sci Med (2018) 41(1):217–23. doi: 10.1007/s13246-018-0622-2
7. Hartford AC, Palisca MG, Eichler TJ, Beyer DC, Devineni VR, Ibbott GS, et al. American Society for therapeutic radiology and oncology (ASTRO) and American college of radiology (ACR) practice guidelines for intensity-modulated radiation therapy (IMRT). Int J Radiat Oncol Biol Phys (2009) 73(1):9–14. doi: 10.1016/j.ijrobp.2008.04.049
8. Gregoire V, Mackie TR, de Neve W, Gospodarowicz M, Purdy JA, van Herk M, et al. ICRU report 83: Prescribing, recording, and reporting intensity-modulated photon beam therapy (IMRT). J ICRU. (2010) 10(1):1–107 Oxford University Press.
9. Low DA, Moran JM, Dempsey JF, Dong L, Oldham M. Dosimetry tools and techniques for IMRT. Med Phys (2011) 38(3):1313–38. doi: 10.1118/1.3514120
10. Heng VJ, Serban M, Seuntjens J, Renaud MA. Ion chamber and film-based quality assurance of mixed electron-photon radiation therapy. Med Phys (2021) 48(9):5382–95. doi: 10.1002/mp.15081
11. Marrazzo L, Zani M, Pallotta S, Arilli C, Casati M, Compagnucci A, et al. GafChromic® EBT3 films for patient specific IMRT QA using a multichannel approach. Physica Med (2015) 31(8):1035–42. doi: 10.1016/j.ejmp.2015.08.010
12. Xu Q, Huynh K, Nie W, Rose MS, Chawla AK, Choe KS, et al. Implementing and evaluating a high-resolution diode array for patient-specific quality assurance of robotic brain stereotactic radiosurgery/radiotherapy. J Appl Clin Med Phys (2022) 23(5):e13569. doi: 10.1002/acm2.13569
13. Bakhtiari M, Kumaraswamy L, Bailey DW, de Boer S, Malhotra HK, Podgorsak MB. Using an EPID for patient-specific VMAT quality assurance. Med Phys (2011) 38(3):1366–73. doi: 10.1118/1.3552925
14. Campos GFP, Souto ACS, Lencart JB, Cunha LPT, Dias AG. Development of an independent MU calculation software for radiotherapy treatments with stereotactic cones. J Appl Clin Med Phys (2022) 23(4):e13542. doi: 10.1002/acm2.13542
15. Lorenz F, Killoran JH, Wenz F, Zygmanski P. An independent dose calculation algorithm for MLC-based stereotactic radiotherapy. Med Phys (2007) 34(5):1605–14. doi: 10.1118/1.2717385
16. Katsuta Y, Kadoya N, Fujita Y, Shimizu E, Majima K, Matsushita H, et al. Log file-based patient dose calculations of double-arc VMAT for head-and-neck radiotherapy. Phys Med (2018) 48:6–10. doi: 10.1016/j.ejmp.2018.03.006
17. Pawlicki T, Yoo S, Court LE, McMillan SK, Rice RK, Russell JD, et al. Moving from IMRT QA measurements toward independent computer calculations using control charts. Radiother Oncol (2008) 89(3):330–7. doi: 10.1016/j.radonc.2008.07.002
18. Siochi RA, Huang Y, Bayouth J. Assessment of an in-house independent phantom dose calculation algorithm for IMRT QA. Med Phys (2008) 35:2944–4. doi: 10.1118/1.2962735
19. Kry SF, Glenn MC, Peterson CB, Branco D, Mehrens H, Steinmann A, et al. Independent recalculation outperforms traditional measurement-based IMRT QA methods in detecting unacceptable plans. Med Phys (2019) 46(8):3700–8. doi: 10.1002/mp.13638
20. Yan G, Liu C, Simon TA, Peng LC, Fox C, Li JG. On the sensitivity of patient-specific IMRT QA to MLC positioning errors. J Appl Clin Med Phys (2009) 10(1):120–8. doi: 10.1120/jacmp.v10i1.2915
21. Kry SF, Molineu A, Kerns JR, Faught AM, Huang JY, Pulliam KB, et al. Institutional patient-specific IMRT QA does not predict unacceptable plan delivery. Int J Radiat Oncol Biol Phys (2014) 90(5):1195–201. doi: 10.1016/j.ijrobp.2014.08.334
22. Klein EE, Hanley J, Bayouth J, Yin FF, Simon W, Dresser S, et al. Task group 142, American association of physicists in medicine. task group 142 report: Quality assurance of medical accelerators. Med Phys (2009) 36(9):4197–212. doi: 10.1118/1.3190392
23. Siochi RA, Molineu A, Orton CG. Point/Counterpoint. patient-specific QA for IMRT should be performed using software rather than hardware methods. Med Phys (2013) 40(7):070601. doi: 10.1118/1.4794929
24. Smith JC, Dieterich S, Orton CG. Point/counterpoint. it is still necessary to validate each individual IMRT treatment plan with dosimetric measurements before delivery. Med Phys (2011) 38(2):553–5. doi: 10.1118/1.3512801
25. Zhu TC, Stathakis S, Clark JR, Feng W, Georg D, Holmes SM, et al. Report of AAPM task group 219 on independent calculation-based dose/MU verification for IMRT. Med Phys (2021) 48(10):e808–29. doi: 10.1002/mp.15069
26. Bogdanich W. As technology surges, radiation safeguards lag. New York: The New York Times (2010). Available at: http://www.nytimes.com/2010/01/27/us/27radiation.html?pagewanted=all&_r=0.
27. Fogliata A, Vanetti E, Albers D, Brink C, Clivio A, Knöös T, et al. On the dosimetric behaviour of photon dose calculation algorithms in the presence of simple geometric heterogeneities: comparison with Monte Carlo calculations. Phys Med Biol (2007) 52(5):1363–85. doi: 10.1088/0031-9155/52/5/011
28. Xu XG, Liu ty, Su L, Du X, Riblett M, Ji W, et al. ARCHER, a new Monte Carlo software tool for emerging heterogeneous computing environments. Ann Nucl Energy (2015) 82:2–9. doi: 10.1016/j.anucene.2014.08.062
29. Yao XU. Method of virtual source modeling for external photon radiotherapy and its clinical application in dose checking [Ph.D. thesis]. Anhui: University of Science and Technology of China (2021).
30. Su L, Yang Y, Bednarz B, Sterpin E, Du X, Liu T, et al. ARCHERRT - a GPU-based and photon-electron coupled Monte Carlo dose computing engine for radiation therapy: software development and application to helical tomotherapy. Med Phys (2014) 41(7):071709. doi: 10.1118/1.4884229
31. Miften M, Olch A, Mihailidis D, Moran J, Pawlicki T, Molineu A, et al. Tolerance limits and methodologies for IMRT measurement-based verification QA: Recommendations of AAPM task group no. 218. Med Phys (2018) 45(4):e53–83. doi: 10.1002/mp.12810
32. Ezzell GA, Burmeister JW, Dogan N, LoSasso TJ, Mechalakos JG, Mihailidis D, et al. IMRT commissioning: Multiple institution planning and dosimetry comparisons, a report from AAPM task group 119. Med Phys (2009) 36(11):5359–73. doi: 10.1118/1.3238104
33. Carlone M, Cruje C, Rangel A, McCabe R, Nielsen M, Macpherson M. ROC analysis in patient specific quality assurance. Med Phys (2013) 40(4):042103. doi: 10.1118/1.4795757
34. Hasse K, Scholey J, Ziemer BP, Natsuaki Y, Morin O, Solberg TD, et al. Use of receiver operating curve analysis and machine learning with an independent dose calculation system reduces the number of physical dose measurements required for patient-specific quality assurance. Int J Radiat Oncol Biol Phys (2021) 109(4):1086–95. doi: 10.1016/j.ijrobp.2020.10.035
35. Li J, Wang L, Zhang X, Liu L, Li J, Chan MF, et al. Machine learning for patient-specific quality assurance of VMAT: Prediction and classification accuracy. Int J Radiat Oncol Biol Phys (2019) 105(4):893–902. doi: 10.1016/j.ijrobp.2019.07.049
36. Katsuta Y, Kadoya N, Fujita Y, Shimizu E, Matsunaga K, Sawada K, et al. Patient-specific quality assurance using Monte Carlo dose calculation and elekta log files for prostate volumetric-modulated arc therapy. Technol Cancer Res Treat (2017) 16(6):1220–5. doi: 10.1177/1533034617745250
37. Chan GH, Chin LCL, Abdellatif A, Bissonnette JP, Buckley L, Comsa D, et al. Survey of patient-specific quality assurance practice for IMRT and VMAT. J Appl Clin Med Phys (2021) 22(7):155–64. doi: 10.1002/acm2.13294
38. Pan Y, Yang R, Zhang S, Li J, Dai J, Wang J, et al. National survey of patient specific IMRT quality assurance in China. Radiat Oncol (2019) 14(1):69. doi: 10.1186/s13014-019-1273-5
39. Das IJ, Cheng CW, Watts RJ, Ahnesjö A, Gibbons J, Li XA, et al. TG-106 of the therapy physics committee of the AAPM. accelerator beam data commissioning equipment and procedures: Rreport of the TG-106 of the therapy physics committee of the AAPM. Med Phys (2008) 35(9):4186–215.
40. Jacqmin DJ, Bredfeldt JS, Frigo SP, Smilowitz JB. Implementation of the validation testing in MPPG 5.a "Commissioning and QA of treatment planning dose calculations-megavoltage photon and electron beams". J Appl Clin Med Phys (2017) 18(1):115–27.
41. Rogers DW, Faddegon BA, Ding GX, Ma CM, We J, Mackie TR. BEAM: A Monte Carlo code to simulate radiotherapy treatment units. Med Phys (1995) 22(5):503–24. doi: 10.1118/1.597552
42. Ma CM, Mok E, Kapur A, Pawlicki T, Findley D, Brain S, et al. Clinical implementation of a Monte Carlo treatment planning system. Med Phys (1999) 26(10):2133–43. doi: 10.1118/1.598729
43. Adam DP, Liu T, Caracappa PF, Bednarz BP, Xu XG. New capabilities of the Monte Carlo dose engine ARCHER-RT: Clinical validation of the Varian TrueBeam machine for VMAT external beam radiotherapy. Med Phys (2020) 47(6):2537–49. doi: 10.1002/mp.14143
44. Chaswal V, Weldon M, Gupta N, Chakravarti A, Rong Y. Commissioning and comprehensive evaluation of the ArcCHECK cylindrical diode array for VMAT pretreatment delivery QA. J Appl Clin Med Phys (2014) 15(4):4832. doi: 10.1120/jacmp.v15i4.4832
45. Feygelman V, Zhang G, Stevens C, Nelms BE. Evaluation of a new VMAT QA device, or the "X" and "O" array geometries. J Appl Clin Med Phys (2011) 12(2):3346. doi: 10.1120/jacmp.v12i2.3346
Keywords: patient-specific QA, dosimetric verification, Monte Carlo, independent dose verification, ArcherQA
Citation: Xu Y, Zhang K, Liu Z, Liang B, Ma X, Ren W, Men K and Dai J (2022) Treatment plan prescreening for patient-specific quality assurance measurements using independent Monte Carlo dose calculations. Front. Oncol. 12:1051110. doi: 10.3389/fonc.2022.1051110
Received: 22 September 2022; Accepted: 19 October 2022;
Published: 07 November 2022.
Edited by:
James Chow, University of Toronto, CanadaReviewed by:
RenMing Zhong, Sichuan University, ChinaRuijie Yang, Peking University Third Hospital, China
Copyright © 2022 Xu, Zhang, Liu, Liang, Ma, Ren, Men and Dai. This is an open-access article distributed under the terms of the Creative Commons Attribution License (CC BY). The use, distribution or reproduction in other forums is permitted, provided the original author(s) and the copyright owner(s) are credited and that the original publication in this journal is cited, in accordance with accepted academic practice. No use, distribution or reproduction is permitted which does not comply with these terms.
*Correspondence: Jianrong Dai, dai_jianrong@cicams.ac.cn; Kuo Men, menkuo@cicams.ac.cn
†These authors have contributed equally to this work and share first authorship