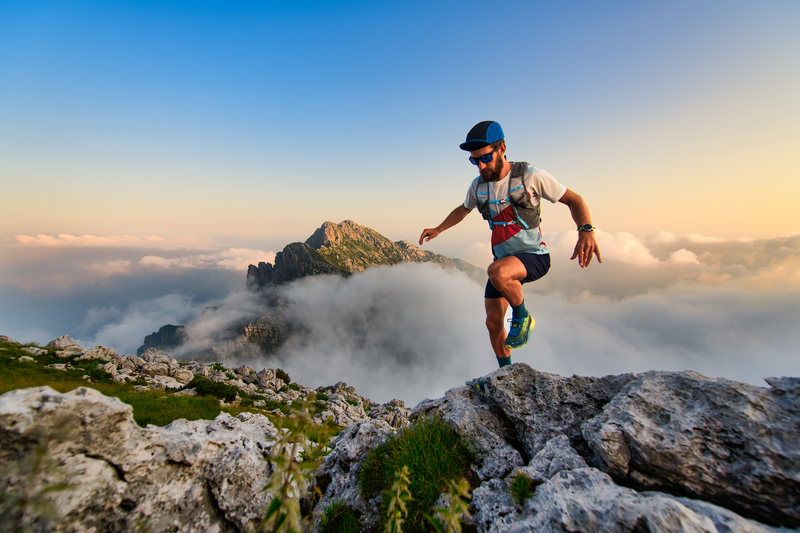
94% of researchers rate our articles as excellent or good
Learn more about the work of our research integrity team to safeguard the quality of each article we publish.
Find out more
ORIGINAL RESEARCH article
Front. Oncol. , 08 November 2022
Sec. Cancer Imaging and Image-directed Interventions
Volume 12 - 2022 | https://doi.org/10.3389/fonc.2022.1021570
This article is part of the Research Topic Incorporation of Reporting and Data Systems into Cancer Radiology View all 8 articles
Purposes: This study aimed to establish a predictive model of microvascular invasion (MVI) in hepatocellular carcinoma (HCC) by contrast-enhanced computed tomography (CT), which relied on a combination of machine learning approach and imaging features covering Liver Imaging and Reporting and Data System (LI-RADS) features.
Methods: The retrospective study included 279 patients with surgery who underwent preoperative enhanced CT. They were randomly allocated to training set, validation set, and test set (167 patients vs. 56 patients vs. 56 patients, respectively). Significant imaging findings for predicting MVI were identified through the Least Absolute Shrinkage and Selection Operator (LASSO) logistic regression method. Predictive models were performed by machine learning algorithm, support vector machine (SVM), in the training set and validation set, and evaluated in the test set. Further, a combined model adding clinical findings to the radiologic model was developed. Based on the LI-RADS category, subgroup analyses were conducted.
Results: We included 116 patients with MVI which were diagnosed through pathological confirmation. Six imaging features were selected about MVI prediction: four LI-RADS features (corona enhancement, enhancing capsule, non-rim aterial phase hyperehancement, tumor size) and two non-LI-RADS features (internal arteries, non-smooth tumor margin). The radiological feature with the best accuracy was corona enhancement followed by internal arteries and tumor size. The accuracies of the radiological model and combined model were 0.725–0.714 and 0.802–0.732 in the training set, validation set, and test set, respectively. In the LR-4/5 subgroup, a sensitivity of 100% and an NPV of 100% were obtained by the high-sensitivity threshold. A specificity of 100% and a PPV of 100% were acquired through the high specificity threshold in the LR-M subgroup.
Conclusion: A combination of LI-RADS features and non-LI-RADS features and serum alpha-fetoprotein value could be applied as a preoperative biomarker for predicting MVI by the machine learning approach. Furthermore, its good performance in the subgroup by LI-RADS category may help optimize the management of HCC patients.
Hepatocellular carcinoma (HCC) represents the third leading cause of cancer-related mortality worldwide and remains a growing tendency (1). Microvascular invasion (MVI) indicates the frequent recurrence, extrahepatic metastases, and poor prognosis after surgical resection and transplantation in HCC patients (2–4). It is critical to detect the presence of MVI at the time of the diagnosis of liver lesion, which impact the choice involving wide margin hepatectomy or combined with intraoperative electron radiotherapy, or liver transplantation, or tumor ablation (3–6). Thus, MVI urgently requires preoperative prediction to apply the optimal therapeutic strategy, rather than being diagnosed through microscopic examination of a pathological specimen after hepatectomy (7, 8). Adequate number of sampling sites (NUSS) and sampling location affect the detection rate of MVI. Chen et al. suggested that at least four to eight NUSS within paracancerous parenchyma ≤1 cm were needed for assessing MVI according to tumor size (9). Thus, pathological biopsy is likely to increase false-negative rates before surgery due to sampling errors and number of NUSS.
With increasing attention to MVI, serum markers (i.e., AFP) and imaging features as non-invasive examinations before surgery, such as internal arteries and tumor margin, have been used to predict MVI (10–12). However, prior studies showed heterogeneous standards and varied accuracies, which require further research (10–12). The Liver Imaging Reporting and Data System (LI-RADS) has been recognized for HCC diagnosis (13). Regarding its advantages of standardized criteria and being easy to generalize, few studies have also attempted to explore the relationship between LI-RADS features and MVI in HCC. For example, ancillary features of LI-RADS including absence of nodule-in-nodule architecture, coronal enhancement, mosaic architecture, and LR-M features including marked diffusion restriction and rim arterial enhancement have been reported as predictive factors of MVI (12, 14–16). These studies are mainly derived from magnetic resonance imaging (MRI) features, whereas there was shortage of computed tomography (CT)-driven results (12, 14–19). Additional studies are needed to determine whether predictive MRI features are still applicable for CT. Compared with MRI, CT has some advantages which are shorter scanning time and lower cost, more stability, and not being limited by the presence of metal objects implanted in the human body. Therefore, research of CT deserves further study. This may also help in expanding the power of LI-RADS features in clinical decision making and obtaining predictive models of MVI based on standardized CT imaging features.
Machine learning has been used to extract radiomics features and develop corresponding models for MVI prediction (20–22). Although models with radiomics features presented good performance, the interpretability and generalizability limit clinical application. The machine learning approach could identify complicated interactions among predictive factors and be able to avoid overfitting as well as improve the performance of the model (23, 24). However, it is underused in interpretable imaging features. The combination of interpretable imaging features and machine learning method may find functional information and aid in the identification of MVI.
By machine learning algorithms, our purpose is to establish and evaluate visibly standardized CT feature-based models for MVI detection. Additionally, subgroup analyses are used to evaluate model performance for risk stratification of HCC patients according to LI-RADS category.
The single-center study was approved by the ethical committees of the Beijing Youan Hospital, Capital Medical University, and complied with the ethical guidelines of the Declaration of Helsinki. Owing to the retrospective nature of our study, the institutional review board waived the patients’ informed consent.
Consecutive adult patients were recruited from January 2015 to December 2021. A total of 1,581 patients were screened according to the selection criteria, which were as follows: 1) diagnosed by histological evaluation in accordance with the American Association for the Study of Liver Diseases (AASLD) or European Association for the Study of the Liver (EASL) guidelines for HCC (25); 2) underwent enhanced CT examinations within 1 month before surgery; 3) patients whose state of MVI was evaluated by histological results. The excluded criteria included the following: 1) any preoperative treatment; 2) macrovascular or biliary duct invasion; 3) positive resection margin through pathological assessment; 4) extrahepatic propagation or spontaneous tumor rupture; 5) absence of quality imaging information. A flowchart for subjects is provided in Figure 1. Finally, we selected 279 patients, who were randomly allocated to training set, validation set, and test set through a 6:2:2 split (training set: 167 patients, validation set and test set: both 56 patients).
A contrast-enhanced multiphase CT examination was performed on a 64-multidetector CT scanner (GE LightSpeed VCT, USA). Intravenous administration of an iodine contrast agent (370 mg I/ml of iopromide 370, Schering, Berlin, German) with a dose of 1.5 ml/kg was provided at a rate of 3 ml/s using the automatic bolus-tracking program. The experienced radiology technician controlled the celiac artery attenuation and placed the region of interest in the abdominal aorta. The trigger threshold level-based SmartPrep contrast was set at 100 Hounsfield units. The contrast-enhanced CT scanning protocol is summarized in the Supplemental Table 1.
Three board-certified gastrointestinal radiologists independently and retrospectively reviewed the enhanced CT images, who were informed the diagnosis of HCC, but unaware of other outcomes of pathologic examinations and clinical information. For multifocal HCC, the lesion with the largest diameter was selected for assessment. According to LI-RADS version 2018 (13), reviewers determined the imaging characteristics of tumors: major features (non-rim arterial phase hyperenhancement (APHE), non-peripheral washout, tumor size, and enhancing capsule), ancillary features (corona enhancement, nodule-in-nodule architecture, mosaic architecture, blood products in mass), and LR-M features (targetoid features including rim APHE and peripheral washout and delayed enhancement, intratumor necrosis). Moreover, non-LI-RADS features based on previous studies were also evaluated: tumor number, internal arteries, non-smooth tumor margin, peritumoral hypoattenuating halo, and tumor–liver difference. Supplemental Table 2 shows the definition of imaging features in detail. The LI-RADS category was also allocated to tumor lesions. When there was a difference of opinion about the evaluation of any imaging characteristic, a second review was done until agreement was reached.
The clinical characteristics included age, gender, etiology of chronic liver disease, Child–Pugh grade, and blood variables (shown in Table 1).
Feature selection could affect learning accuracy and result in comprehensibility. We applied the Least Absolute Shrinkage and Selection Operator (LASSO) for imaging feature selection. Support vector machine (SVM) was used to develop the machine learning model for MVI prediction in HCC. In addition, continuous blood signatures were translated into categorical variables and compared via chi-squared test or Fisher’s exact test (with p < 0.05). Demographic variables were compared by chi-squared test or Student t test (p < 0.05). Then, we found the significant clinical variables based on logistic regression (p < 0.05) which were combined with radiologic features to develop combined model.
Minimization of the empirical error penalized by a regularization term, namely, totality of the empirical error (loss term) and regularization term (penalty term), is applied to construct a sparse learning model for feature selection (24). The LASSO logistic regression algorithm is frequently used as the standard sparse regression approach on the basis of the regularization framework. The regularization parameter λ is the balance between the loss term and the penalty term (26).
We standardized features with the z score. The value of regularization parameter λ was selected via 10-fold cross-validation in the training set, which resulted in the sparsest model remaining within one standardized error of the minimum loss. Imaging features were selected which yielded non-zero coefficients (27). The multicollinearity of the significant attributes were evaluated using the variation inflation factor (VIF<5).
We developed radiological predictive model using SVM and optimized hyperparameters through learning curves and grid searches in the training set and validation set. Furthermore, a combined model using features in the radiologic model with a serum alpha-fetoprotein (AFP) value was also used in the training set and the validation set and assessed in the test set.
The sensitivity, specificity, accuracy, positive predictive value (PPV), and negative predictive value (NPV) of the MVI were compared using the McNemar test. Areas under the curve (AUC) of two models for MVI detection were compared by the Delong test. Moreover, we made the decision curve analysis (DCA) counting the net benefits under different threshold probabilities, which indicated the clinical relevance of the prediction model (28). The calibration curve was used to evaluate the goodness of models.
We defined three thresholds from the results of the training set: Youden’s index, high specificity threshold (95% specificity), and high sensitivity threshold (95% sensitivity). Performance matrices were evaluated according to three thresholds of MVI probabilities in the test set, namely, the whole set, LR 4/5 group, and LR-M group. Statistical analyses were performed using Python ver. 3.8 and SPSS software ver. 26.0. Inter-reader agreement among three observers for the CT features was assessed by a Fleiss’ kappa value of ≤0.20 which means poor level, 0.21–0.40 which is fair level, 0.41–0.60 which is moderate level, 0.61–0.80 which is substantial level, and ≥0.80 which means nearly best level.
A total of 279 patients were enrolled in this study (Table 1). One hundred sixty-seven patients, 56 patients, and 56 patients constituted the training set, validation set, and test set, which involved 70 (41.9%), 23 (41.1%), and 23 (41.1%) patients with presence of MVI, respectively (p = 0.990). The clinical variables were similar among the training set, validation set, and test set (p = 0.278–0.97). Between MVI-positive patients and MVI-negative patients, clinical factors including AFP and gender were significantly different in the training set and the test set, separately. Many radiologic features were also different between the MVI-positive group and MVI-negative group in the training, validation, and test sets which are shown in detail in Table 1.
Six radiological features were selected via the LASSO logistic regression approach with the optimal λ (λ = 0.0129). There are four LI-RADS features (corona enhancement, enhancing capsule, non-rim aterial phase hyperehancement, tumor size) and two non-LI-RADS features (internal arteries, non-smooth tumor margin) (Figure 2). Misclassification errors and coefficients are shown in Figure 3. The VIF values of those features ranged 1.10–1.49 (all <5), which were proved without multicollinearity. Their Fleiss’ kappa values ranged from 0.331-0.974 (listed in Supplementary Table 3). At the aspect of blood signatures and demographic variables, we only found that AFP was related to MVI using multivariate logistic regression in the training set (p < 0.05) (Supplementary Table 4).
Figure 2 Graphical illustration of the selected features. Corona enhancement (A). Internal arteries (B). Non-smooth margin (C). Enhancing capsule (D). Non-rim AP hyperenhancement (E).
Figure 3 Imaging feature selection for MVI identification in the training set by LASSO logistic regression. (A) Selection of tuning λ in the LASSO model through 10-fold cross-validation. Vertical black lines define the best values of λ that provide the best fit. (B) LASSO coefficient profiles of all imaging features for MVI presence. Vertical black lines are plotted at the value selected in (A). Imaging features with non-zero coefficients are indicated. MVI, microvascular invasion; LASSO, Least Absolute Shrinkage and Selection Operator.
A radiological model using the SVM approach that integrated corresponding radiologic predictors was built and optimized in the training set and validation set, which presented an AUC and accuracy of 0.795/0.793 and 0.725/0.714, respectively (Supplementary Table 5). The AUC and accuracy of the radiological model in the test model were 0.775 and 0.714 (Table 2). The comparison predictive performance between radiological model and combined model which was added with AFP presented no significant difference among the three sets (AUC: p = 0.08–0.569, accuracy: p = 0.125–1.000, sensitivity: p = 0.065–1.000, specificity: p = 0.625–1.000). However, the combined model obtained a better benefit than the radiological model for MVI probability examination by DCA (Figure 4 and Supplementary Figure 1). Notably, a calibration curve of the combined model demonstrated a better calibration than that of the radiologic model, which indicated a general underestimation of MVI risk in HCC (Figure 4). We also conducted subgroup analyses using both the radiological model and combined model in the test set. The combined model maintained good performance in both the LR-4/5 and LR-M groups rather than radiological model (Table 2).
Figure 4 Predictive performance of the radiological model and combined model for MVI prediction in HCC patients in the test set (A–C). Receiver operating characteristic (ROC) curves of radiological model and combined model in the test set (A). Decision curve graphics (DCA) of the radiological model and combined model in the test set (B). Calibration curve graphics of predicted risk based on the radiological model and combined model in the test set (C).
Furthermore, the Youden index, high-sensitivity threshold, and high-specificity threshold of the combined model were calculated as 0.59, 0.27026, and 0.6954 in the training set, respectively. Based on the Youden index, the sensitivity, specificity, PPV, NPV, and accuracy of the test set were 52.2%, 84.8%, 70.6%, 71.8%, and 71.4%, respectively (Figure 5). In the LR-4/5 subgroup, a sensitivity of 100% and an NPV of 100% were obtained through the high-sensitivity threshold. In the LR-M subgroup, a specificity of 100% and a PPV of 100% were acquired from the high-specificity threshold.
Figure 5 Charts exhibit performance metrics of the combined model in the (A) whole test set, (B) LR-4/5 subgroup, and (C) LR-M subgroup. PPV, positive predictive value; NPV, negative predictive value. Ninety-five percent sensitivity threshold and high specificity threshold of combined model were 0.27026 and 0.6954, respectively.
The predictive power of variables derived from the combined model is shown in Figure 6. The highest accuracy variable was corona enhancement. Features with the highest sensitivity was enhancing capsule followed by a non-smooth tumor margin and internal arteries. AFP presented the highest specificity which was followed by corona enhancement.
Figure 6 Feature predictive performance for predicting MVI in the test set. AP, arterial phase; PPV, positive predictive value; NPV, negative predictive value.
With the accumulation of knowledge about the prognostic implication of MVI in HCC, it becomes more clinically significant, especially accurate preoperative prediction. Our study selected optimal features including four LI-RADS features (corona enhancement, enhancing capsule non-rim aterial phase hyperehancement, tumor size) and two non-LI-RADS features (internal arteries, non-smooth tumor margin) and AFP. Predictive models of MVI were developed by the SVM approach. Both radiological model and combined model with AFP presented good accuracy and an AUC of MVI prediction in the test set (AUC: 0.775 vs. 0.778, accuracy: 0.714 vs. 0.732, respectively). However, the combined model showed relatively robust accuracy during subgroup analyses in the test set. DCA and the calibration curve also supported the combined model with good performance for MVI prediction.
Based on our prior knowledge and previous literature about MVI and liver tumor (22, 29, 30), we also selected SVM as the classifier and found its good performance of MVI prediction in HCC. Similar to previous studies (10, 11, 31), the serum AFP level is related to the prevalence of MVI. The combined model through adding AFP to the radiological model improved predictive performance, which was reported by previous studies (14, 32, 33). Although we did not find a significant difference between radiological model and combined model, the combined model with AFP recognized MVI false-negative patients predicted by the radiological model in LR-M patients (2 (66.7%)). Therefore, we inferred that it may result from a limited sample size. Notably, Yu et al. also reported that AFP was considered as a suitable biomarker for MVI false-negative patients in conventional pathological protocols (34).
The predictive model that is to be transportable into clinical practice is the final purpose, which is the benchmark indicator assessing its clinical power. Prior studies demonstrated that wide resection (>1 cm), anatomical resection, and intraoperative electron radiotherapy could improve the survival rate of HCC patients along with MVI (35–37). Although LR-M features are not typical features of HCC, HCC with LR-M features have been found to have a significant association with poor prognosis and aggressive behavior (38, 39). To prioritize the allocation of MVI risk probability in LR-M category patients, we defined the 95% specificity threshold in the training set. In the LR-M subgroup, the high specificity threshold could get a specificity of 100% and a PPV of 100%, which may contribute to those high risk for MVI for further treatment. The high-sensitivity threshold could yield an NPV of 100% in the LR-4/5 subgroup. The low-risk patients could avoid biopsy and be considered as the potentially eligible patients for liver transplant.
Regarding LI-RADS features, we selected corona enhancement and major features. Corona enhancement is an LI-RADS ancillary feature for MVI prediction in HCC, which is consistent with previous findings of enhanced MRI (12, 14). Prior studies showed that the reduction in portal flow caused by microscopic tumor thrombin blocking tiny portal vein branches around the tumor caused compensatory hyperperfusion in the AP, which led to corona enhancement (40, 41). Some radiomics studies about MVI prediction focus on the value of the peritumoral area (21, 42). Corona enhancement was the highest accuracy feature which indicates the importance of the peritumoral area. Major LI-RADS features included the enhancing capsule and non-rim enhancement in AP and tumor size in our model. The enhancing capsule and enhancement pattern have remained controversial currently. Some studies suggested that the radiological capsule (22, 23, 43, 44) was a predictor of MVI presence. However, a meta-analysis about enhanced MRI features revealed that the enhancing capsule was not a predictor of MVI (12). Our study was consistent with Xu et al. (22), who obtained a capsule with high sensitivity and low accuracy of MVI prediction, similar to non-rim enhancement in the arterial phase. Wei et al. also reported a typical enhancement pattern which was an independent factor of MVI presence. However, Zhou et al. and Hong et al. showed that rim enhancement in AP or LR-M features was a marker for MVI prediction (12, 32). Thus, those two features need more external data to assess their power of MVI identification. In addition, we also discovered a link between tumor size and MVI. The presence of MVI was also associated with bigger tumor size (>5 cm) according to enhanced MRI (DOR: 5.2 (3.0–9.0) by pooled analysis of inconsistent data (12). Large tumor size was also shown in prior research to be a reliable indicator of MVI based on pathologic results (45, 46), and it was also thought to be a predictor of a poor prognosis (46). A further predictor of vasculature enclosing tumor cluster (VETC) style in HCC was larger tumor size (>5 cm) (47, 48). VETC, a potent pathological style that affects prognosis, was linked to MVI’s frequent existence (48).
We found two non-LIRADS characteristics including internal arteries and a non-smooth margin. Internal arteries were revealed to be the feature with the high sensitivity in our study. Internal arteries predicted on enhanced MRI could be a separate determinant of MVI, according to Jiang et al. (14). Macrotrabecular massive hepatocellular carcinoma, which is regarded as an aggressive form of HCC and commonly has MVI and a poor prognosis, was associated with internal arteries (49–51). Studies also show that internal arteries may serve as a radiogenomic marker for cell growth and matrix penetration (52). The non-smooth margin exhibited a significant relationship with MVI, along with the highest NPV among predictors in our study. The outcome is the same as enhanced MRI (14). Research has shown that tumors with a non-smooth margin were mainly presented in three types of tumors: single nodular type with extranodular growth, confluent multinodular type, and invasive type, which displayed high prevalence of MVI (53). They have suggested that anatomic resection should be employed to reduce the recurrence.
In addition, our study did not find nodule-in-nodule architecture related to MVI, which is different to previous enhanced MRI research (16). The proportion of patients with nodule-in-nodule architecture presented an obvious difference (our study: 1 (0.4%) patients vs. Wei et al.: 49 (44.1%) patients), which may affect the result (16). Similarly, the different ratios of patients with multifocality tumor between our study and Jiang et al.’s study may lead to inconsistent results of MVI prediction (our study: 13 (4.7%) patients vs. 125 (39%) patients) (12).
Our study has some limitations. Firstly, it was a retrospective study whose bias may inevitably exist. Future studies could perform a multicenter prospective study to validate our results. Secondly, our study included mainly HBV-related patients, which may be limitedly applied in patients by other etiologies. More studies are required to explore the application of the model. Thirdly, a gender difference was found between MVI-positive patients and MVI-negative patients in the training set and test set. However, gender did not drive the outcome that no difference exists between correctly and incorrectly predicted MVI patients. Fourth, we did not compare the performance of enhanced CT-based versus enhanced MRI-based LI-RADS features about MVI identification head by head, and further study on the comparison is required in the future. Finally, our research presented no significantly different accuracy and AUC between the radiological model and combined model. More studies with larger samples should be further conducted.
In conclusion, a combination of four LI-RADS features (tree major features, corona enhancement) and two non-LI-RADS features (internal arteries, non-smooth margin) and AFP could be applied as preoperative biomarkers for predicting MVI through a machine learning approach. Furthermore, its good performance in the subgroup by the LI-RADS category may help optimize the management of HCC patients.
The raw data supporting the conclusions of this article will be made available by the authors, without undue reservation.
The studies involving human participants were reviewed and approved by the ethical committees of the Beijing Youan Hospital, Capital Medical University. Written informed consent for participation was not required for this study in accordance with the national legislation and the institutional requirements.
XY: conceptualization, data curation, formal analysis, investigation, software. Methodology, visualization, original draft writing. GS: software, review, and editing. JL: review and editing, validation. BL: methodology, validation. CC: validation. DZ: formal analysis, supervision, review and editing, HL: conceptualization, formal analysis, supervision, review and editing. All authors contributed to the article and approved the submitted version.
This work was supported by the National Natural Science Foundation of China (grant no. 61936013) and the Beijing Natural Science Foundation (7212051).
The authors declare that the research was conducted in the absence of any commercial or financial relationships that could be construed as a potential conflict of interest.
All claims expressed in this article are solely those of the authors and do not necessarily represent those of their affiliated organizations, or those of the publisher, the editors and the reviewers. Any product that may be evaluated in this article, or claim that may be made by its manufacturer, is not guaranteed or endorsed by the publisher.
The Supplementary Material for this article can be found online at: https://www.frontiersin.org/articles/10.3389/fonc.2022.1021570/full#supplementary-material
Supplementary Figure 1 | Predictive performance of radiological model and combined model for MVI prediction in HCC patients in the training set and validation set (A–F). Receiver operating characteristic (ROC) curves of radiological model and combined model in the (A) training set, (B) validation set. Decision curve graphics (DCA) of radiological model and combined model in the training (C) and validation set (D). Calibration curve graphics by tertiles of predicted risk based on the radiological model and combined model in training set (E) and validation set (F). HCC, hepatocellular carcinoma; MVI, microvascular invasion.
1. Forner A, Reig M, Bruix J. Hepatocellular carcinoma. Lancet (London England) (2018) 391:1301–14. doi: 10.1016/s0140-6736(18)30010-2
2. Lim KC, Chow PK, Allen JC, Chia GS, Lim M, Cheow PC, et al. Microvascular invasion is a better predictor of tumor recurrence and overall survival following surgical resection for hepatocellular carcinoma compared to the Milan criteria. Ann Surg (2011) 254:108–13. doi: 10.1097/SLA.0b013e31821ad884
3. Mazzaferro V, Llovet JM, Miceli R, Bhoori S, Schiavo M, Mariani L, et al. Predicting survival after liver transplantation in patients with hepatocellular carcinoma beyond the Milan criteria: a retrospective, exploratory analysis. Lancet Oncol (2009) 10:35–43. doi: 10.1016/s1470-2045(08)70284-5
4. Roayaie S, Blume IN, Thung SN, Guido M, Fiel MI, Hiotis S, et al. A system of classifying microvascular invasion to predict outcome after resection in patients with hepatocellular carcinoma. Gastroenterology (2009) 137:850–5. doi: 10.1053/j.gastro.2009.06.003
5. Hirokawa F, Hayashi M, Miyamoto Y, Asakuma M, Shimizu T, Komeda K, et al. Outcomes and predictors of microvascular invasion of solitary hepatocellular carcinoma. Hepatol Res (2014) 44:846–53. doi: 10.1111/hepr.12196
6. Beaufrère A, Caruso S, Calderaro J, Poté N, Bijot JC, Couchy G, et al. Gene expression signature as a surrogate marker of microvascular invasion on routine hepatocellular carcinoma biopsies. J Hepatol (2022) 76:343–52. doi: 10.1016/j.jhep.2021.09.034
7. Pommergaard HC, Rostved AA, Adam R, Thygesen LC, Salizzoni M, Gómez Bravo MA, et al. Vascular invasion and survival after liver transplantation for hepatocellular carcinoma: a study from the European liver transplant registry. HPB (2018) 20:768–75. doi: 10.1016/j.hpb.2018.03.002
8. Poté N, Cauchy F, Albuquerque M, Cros J, Soubrane O, Bedossa P, et al. Contribution of virtual biopsy to the screening of microvascular invasion in hepatocellular carcinoma: A pilot study. Liver Int (2018) 38:687–94. doi: 10.1111/liv.13585
9. Chen L, Chen S, Zhou Q, Cao Q, Dong Y, Feng S, et al. Microvascular invasion status and its survival impact in hepatocellular carcinoma depend on tissue sampling protocol. Ann Surg Oncol (2021) 28:6747–57. doi: 10.1245/s10434-021-09673-w
10. Lei Z, Li J, Wu D, Xia Y, Wang Q, Si A, et al. Nomogram for preoperative estimation of microvascular invasion risk in hepatitis b virus-related hepatocellular carcinoma within the Milan criteria. JAMA Surg (2016) 151:356–63. doi: 10.1001/jamasurg.2015.4257
11. McHugh PP, Gilbert J, Vera S, Koch A, Ranjan D, Gedaly R. Alpha-fetoprotein and tumour size are associated with microvascular invasion in explanted livers of patients undergoing transplantation with hepatocellular carcinoma. HPB (2010) 12:56–61. doi: 10.1111/j.1477-2574.2009.00128.x
12. Hong SB, Choi SH, Kim SY, Shim JH, Lee SS, Byun JH, et al. MRI Features for predicting microvascular invasion of hepatocellular carcinoma: A systematic review and meta-analysis. Liver Cancer (2021) 10:94–106. doi: 10.1159/000513704
13. American College of Radiology. CT/MRI liver imaging reporting and data system v2018 core. America: American College of Radiology (2018). Available at: https://www.acr.org/Clinical-Resources/Reporting-and-Data-Systems/LI-RADS/CT-MRI-LI-RADS-v2018.
14. Jiang H, Wei J, Fu F, Wei H, Qin Y, Duan T, et al. Predicting microvascular invasion in hepatocellular carcinoma: A dual-institution study on gadoxetate disodium-enhanced MRI. Liver Int (2022) 42:1158–72. doi: 10.1111/liv.15231
15. Liang X, Shi S, Gao T. Preoperative gadoxetic acid-enhanced MRI predicts aggressive pathological features in LI-RADS category 5 hepatocellular carcinoma. Clin Radiol (2022) 77(9):708–16. doi: 10.1016/j.crad.2022.05.018
16. Wei H, Jiang H, Liu X, Qin Y, Zheng T, Liu S, et al. Can LI-RADS imaging features at gadoxetic acid-enhanced MRI predict aggressive features on pathology of single hepatocellular carcinoma? Eur J Radiol (2020) 132:109312. doi: 10.1016/j.ejrad.2020.109312
17. Yang J, Jiang H, Xie K, Bashir MR, Wan H, Huang J, et al. Profiling hepatocellular carcinoma aggressiveness with contrast-enhanced ultrasound and gadoxetate disodium-enhanced MRI: An intra-individual comparative study based on the liver imaging reporting and data system. Eur J Radiol (2022) 154:110397. doi: 10.1016/j.ejrad.2022.110397
18. Wei H, Yang T, Chen J, Duan T, Jiang H, Song B. Prognostic implications of CT/MRI LI-RADS in hepatocellular carcinoma: State of the art and future directions. Liver Int (2022) 42(10):2131–44. doi: 10.1111/liv.15362
19. Yang H, Han P, Huang M, Yue X, Wu L, Li X, et al. The role of gadoxetic acid-enhanced MRI features for predicting microvascular invasion in patients with hepatocellular carcinoma. Abdominal Radiol (New York) (2022) 47:948–56. doi: 10.1007/s00261-021-03392-2
20. Yang Y, Fan W, Gu T, Yu L, Chen H, Lv Y, et al. Radiomic features of multi-ROI and multi-phase MRI for the prediction of microvascular invasion in solitary hepatocellular carcinoma. Front Oncol (2021) 11:756216. doi: 10.3389/fonc.2021.756216
21. Chong HH, Yang L, Sheng RF, Yu YL, Wu DJ, Rao SX, et al. Multi-scale and multi-parametric radiomics of gadoxetate disodium-enhanced MRI predicts microvascular invasion and outcome in patients with solitary hepatocellular carcinoma ≤ 5 cm. Eur Radiol (2021) 31(7):4824–38. doi: 10.1007/s00330-020-07601-2
22. Xu X, Zhang HL, Liu QP, Sun SW, Zhang J, Zhu FP, et al. Radiomic analysis of contrast-enhanced CT predicts microvascular invasion and outcome in hepatocellular carcinoma. J Hepatol (2019) 70:1133–44. doi: 10.1016/j.jhep.2019.02.023
23. Yan Y, Zhou Q, Zhang M, Liu H, Lin J, Liu Q, et al. Integrated nomograms for preoperative prediction of microvascular invasion and lymph node metastasis risk in hepatocellular carcinoma patients. Ann Surg Oncol (2020) 27:1361–71. doi: 10.1245/s10434-019-08071-7
24. Li X, Wang Y, Ruiz R. A survey on sparse learning models for feature selection. IEEE Trans Cybernetics (2022) 52:1642–60. doi: 10.1109/tcyb.2020.2982445
25. Sun SW, Liu QP, Xu X, Zhu FP, Zhang YD, Liu XS. Direct comparison of four presurgical stratifying schemes for prediction of microvascular invasion in hepatocellular carcinoma by gadoxetic acid-enhanced MRI. J Magn Reson Imaging (2020) 52:433–47. doi: 10.1002/jmri.27043
26. Tibshirani R. Regression shrinkage and selection Via the lasso. Journal of the Royal Statistical Society. Series B (Methodological) (1996) 58:267–88. doi: 10.1111/j.2517-6161.1996.tb02080.x
27. Yu Y, Fan Y, Wang X, Zhu M, Hu M, Shi C, et al. Gd-EOB-DTPA-enhanced MRI radiomics to predict vessels encapsulating tumor clusters (VETC) and patient prognosis in hepatocellular carcinoma. Eur Radiol (2022) 32:959–70. doi: 10.1007/s00330-021-08250-9
28. Kerr KF, Brown MD, Zhu K, Janes H. Assessing the clinical impact of risk prediction models with decision curves: Guidance for correct interpretation and appropriate use. J Clin Oncol (2016) 34:2534–40. doi: 10.1200/jco.2015.65.5654
29. Chen Y, Xia Y, Tolat PP, Long L, Jiang Z, Huang Z, et al. Comparison of conventional gadoxetate disodium-enhanced MRI features and radiomics signatures with machine learning for diagnosing microvascular invasion. AJR Am J Roentgenol (2021) 216(6):1510–20. doi: 10.2214/AJR.20.23255
30. He M, Hu Y, Wang D, Sun M, Li H, Yan P, et al. Value of CT-based radiomics in predicating the efficacy of anti-HER2 therapy for patients with liver metastases from breast cancer. Front Oncol (2022) 12:852809. doi: 10.3389/fonc.2022.852809
31. Notarpaolo A, Layese R, Magistri P, Gambato M, Colledan M, Magini G, et al. Validation of the AFP model as a predictor of HCC recurrence in patients with viral hepatitis-related cirrhosis who had received a liver transplant for HCC. J Hepatol (2017) 66:552–9. doi: 10.1016/j.jhep.2016.10.038
32. Zhou H, Sun J, Jiang T, Wu J, Li Q, Zhang C, et al. A nomogram based on combining clinical features and contrast enhanced ultrasound LI-RADS improves prediction of microvascular invasion in hepatocellular carcinoma. Front Oncol (2021) 11:699290. doi: 10.3389/fonc.2021.699290
33. Liu B, Zeng Q, Huang J, Zhang J, Zheng Z, Liao Y, et al. IVIM using convolutional neural networks predicts microvascular invasion in HCC. Eur Radiol (2022) 32(10):7185–95. doi: 10.1007/s00330-022-08927-9
34. Yu HM, Wang K, Feng JK, Lu L, Qin YC, Cheng YQ, et al. Image-matching digital macro-slide-a novel pathological examination method for microvascular invasion detection in hepatocellular carcinoma. Hepatol Int (2022) 16(2):381–95. doi: 10.1007/s12072-022-10307-w
35. Shi C, Zhao Q, Liao B, Dong Z, Wang C, Yang J, et al. Anatomic resection and wide resection margin play an important role in hepatectomy for hepatocellular carcinoma with peritumoural micrometastasis. ANZ J Surg (2019) 89:E482–e486. doi: 10.1111/ans.15396
36. Chen ZH, Zhang XP, Feng JK, Li LQ, Zhang F, Hu YR, et al. Actual long-term survival in hepatocellular carcinoma patients with microvascular invasion: a multicenter study from China. Hepatol Int (2021) 15:642–50. doi: 10.1007/s12072-021-10174-x
37. Wu YL, Zhai Y, Li M, Cai JQ, Ma P, Wang LM, et al. Long-term outcome of centrally located hepatocellular carcinomas treated by radical resection combined with intraoperative electron radiotherapy (IOERT). Front Oncol (2022) 12:773301. doi: 10.3389/fonc.2022.773301
38. Jeon SK, Joo I, Lee DH, Lee SM, Kang HJ, Lee KB, et al. Combined hepatocellular cholangiocarcinoma: LI-RADS v2017 categorisation for differential diagnosis and prognostication on gadoxetic acid-enhanced MR imaging. Eur Radiol (2019) 29:373–82. doi: 10.1007/s00330-018-5605-x
39. Choi SH, Lee SS, Park SH, Kim KM, Yu E, Park Y, et al. And prognosis of primary liver cancers at gadoxetic acid-enhanced MRI. Radiology (2019) 290:388–97. doi: 10.1148/radiol.2018181290
40. Choi JY, Lee JM, Sirlin CB. CT and MR imaging diagnosis and staging of hepatocellular carcinoma: part II. extracellular agents, hepatobiliary agents, and ancillary imaging features. Radiology (2014) 273:30–50. doi: 10.1148/radiol.14132362
41. Matsui O, Kobayashi S, Sanada J, Kouda W, Ryu Y, Kozaka K, et al. Hepatocelluar nodules in liver cirrhosis: hemodynamic evaluation (angiography-assisted CT) with special reference to multi-step hepatocarcinogenesis. Abdominal Imaging (2011) 36:264–72. doi: 10.1007/s00261-011-9685-1
42. Renzulli M, Mottola M, Coppola F, Cocozza MA, Malavasi S, Cattabriga A, et al. Automatically extracted machine learning features from preoperative CT to early predict microvascular invasion in HCC: The role of the zone of transition (ZOT). Cancers (Basel) (2022) 14(7):1816. doi: 10.3390/cancers14071816
43. Zhao H, Hua Y, Dai T, He J, Tang M, Fu X, et al. Development and validation of a novel predictive scoring model for microvascular invasion in patients with hepatocellular carcinoma. Eur J Radiol (2017) 88:32–40. doi: 10.1016/j.ejrad.2016.12.030
44. Meng XP, Wang YC, Zhou JY, Yu Q, Lu CQ, Xia C, et al. Comparison of MRI and CT for the prediction of microvascular invasion in solitary hepatocellular carcinoma based on a non-radiomics and radiomics method: Which imaging modality is better? J Magn Reson Imaging (2021) 54(2):526–36. doi: 10.1002/jmri.27575
45. Rungsakulkij N, Mingphruedhi S, Suragul W, Tangtawee P, Muangkaew P, Aeesoa S. Platelet-to-Lymphocyte ratio and Large tumor size predict microvascular invasion after resection for hepatocellular carcinoma. Asian Pacific J Cancer Prev (2018) 19:3435–41. doi: 10.31557/apjcp.2018.19.12.3435
46. Pawlik TM, Delman KA, Vauthey JN, Nagorney DM, Ng IO, Ikai I, et al. Tumor size predicts vascular invasion and histologic grade: Implications for selection of surgical treatment for hepatocellular carcinoma. Liver Transplant (2005) 11:1086–92. doi: 10.1002/lt.20472
47. Liu PH, Hsu CY, Hsia CY, Lee YH, Su CW, Huang YH, et al. Prognosis of hepatocellular carcinoma: Assessment of eleven staging systems. J Hepatol (2016) 64:601–8. doi: 10.1016/j.jhep.2015.10.029
48. Renne SL, Woo HY, Allegra S, Rudini N, Yano H, Donadon M, et al. Vessels encapsulating tumor clusters (VETC) is a powerful predictor of aggressive hepatocellular carcinoma. Hepatol (Baltimore Md) (2020) 71:183–95. doi: 10.1002/hep.30814
49. Rhee H, Cho ES, Nahm JH, Jang M, Chung YE, Baek SE, et al. Gadoxetic acid-enhanced MRI of macrotrabecular-massive hepatocellular carcinoma and its prognostic implications. J Hepatol (2021) 74:109–21. doi: 10.1016/j.jhep.2020.08.013
50. Martin JD, Fukumura D, Duda DG, Boucher Y, Jain RK. Reengineering the tumor microenvironment to alleviate hypoxia and overcome cancer heterogeneity. Cold Spring Harbor Perspect Med (2016) 6(12):a027094. doi: 10.1101/cshperspect.a027094
51. Calderaro J, Couchy G, Imbeaud S, Amaddeo G, Letouzé E, Blanc JF, et al. Histological subtypes of hepatocellular carcinoma are related to gene mutations and molecular tumour classification. J Hepatol (2017) 67:727–38. doi: 10.1016/j.jhep.2017.05.014
52. Segal E, Sirlin CB, Ooi C, Adler AS, Gollub J, Chen X, et al. Decoding global gene expression programs in liver cancer by noninvasive imaging. Nat Biotechnol (2007) 25:675–80. doi: 10.1038/nbt1306
Keywords: hepatocellular carcinoma, microvascular invasion, liver imaging reporting and data system, machine learning, contrast enhanced computed tomography
Citation: Yang X, Shao G, Liu J, Liu B, Cai C, Zeng D and Li H (2022) Predictive machine learning model for microvascular invasion identification in hepatocellular carcinoma based on the LI-RADS system. Front. Oncol. 12:1021570. doi: 10.3389/fonc.2022.1021570
Received: 17 August 2022; Accepted: 19 October 2022;
Published: 08 November 2022.
Edited by:
Pilar López-Larrubia, Spanish National Research Council (CSIC), SpainReviewed by:
Yan Zhou, Tianjin Third Central Hospital, ChinaCopyright © 2022 Yang, Shao, Liu, Liu, Cai, Zeng and Li. This is an open-access article distributed under the terms of the Creative Commons Attribution License (CC BY). The use, distribution or reproduction in other forums is permitted, provided the original author(s) and the copyright owner(s) are credited and that the original publication in this journal is cited, in accordance with accepted academic practice. No use, distribution or reproduction is permitted which does not comply with these terms.
*Correspondence: Daobing Zeng, ZGFvX3plbmdAYWxpeXVuLmNvbQ==; Hongjun Li, bGlob25nanVuMDAxMTNAY2NtdS5lZHUuY24=
Disclaimer: All claims expressed in this article are solely those of the authors and do not necessarily represent those of their affiliated organizations, or those of the publisher, the editors and the reviewers. Any product that may be evaluated in this article or claim that may be made by its manufacturer is not guaranteed or endorsed by the publisher.
Research integrity at Frontiers
Learn more about the work of our research integrity team to safeguard the quality of each article we publish.