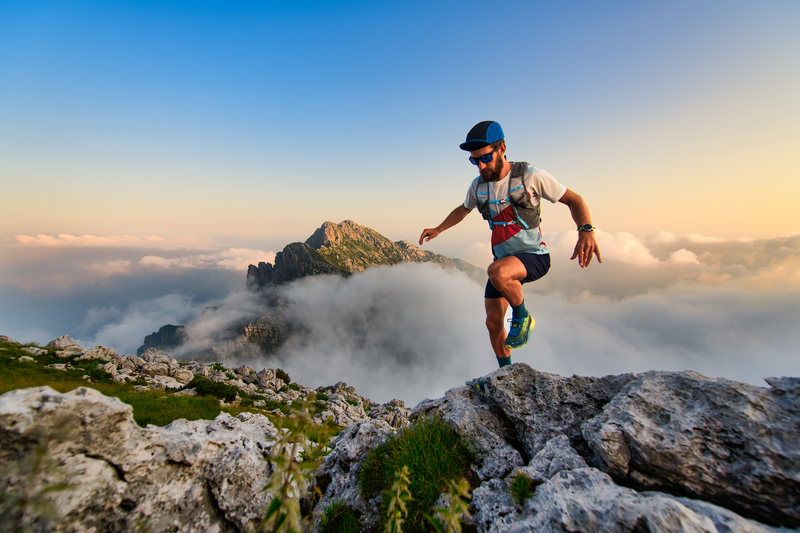
95% of researchers rate our articles as excellent or good
Learn more about the work of our research integrity team to safeguard the quality of each article we publish.
Find out more
ORIGINAL RESEARCH article
Front. Oncol. , 09 December 2022
Sec. Pharmacology of Anti-Cancer Drugs
Volume 12 - 2022 | https://doi.org/10.3389/fonc.2022.1017041
This article is part of the Research Topic Emerging strategies for cancer therapy targeting ferroptosis View all 9 articles
Purpose: Triple-negative breast cancer (TNBC) is an aggressive tumor with poor prognosis, it has higher recurrence and metastatic rates than other breast cancer subtypes. This study aims to investigate biomarkers and potential targets for TNBC related to ferroptosis through data mining and bioinformatics analysis. The findings may provide new insights for treating TNBC.
Methods: The TNBC patients’ data from the Cancer Genome Atlas (TCGA) database were extracted for differential expression and prognosis analysis. Consensus genes obtained by intersecting differential expressed and ferroptosis-related genes was used to establish the prognostic model by the univariate and multivariate Cox analyses. Besides, TNBC data from the Gene Expression Omnibus (GEO) database was used to confirm the reliability of the prognosis model. Moreover, clinical information was analyzed by multifactorial independent analysis to identify independent prognostic factors. The expression of genes constituting the prognostic model was further validated using the Human Protein Atlas (HPA) database. Finally, the Comparative Toxicogenomic Data (CTD) database was used to explore possible treatment drugs for TNBC.
Results: We obtained 13,245 differential expressed genes, and 177 consensus genes. 98 genes with prognostic implication were obtained by univariable Cox. Then, a prognostic model including 12 ferroptosis-related genes was constructed by multivariable Cox. The area under curve (AUC) value of the prognostic model for TNBC was 0.82. The GEO database validated that the model (AUC = 0.77) could predict the patient outcomes. The staining results of 10 out of 12 prognostic model genes in HPA database showed that their expression was consistent with our predictions. Clinical risk analysis indicated that risk score of patients could act as an independent prognostic factor. Finally, six drugs that may have interaction with 12 ferroptosis-related genes were obtained using the CTD database.
Conclusion: The prognostic model composed of 12 ferroptosis-related genes could predict the prognosis of TNBC patients, and seven genes (ASNS, LAMP2, CAV1, DPP4, HELLS, TF, ZFP69B) could be potential new therapeutic targets for TNBC, and two drugs (1-methyl-3-isobutylxanthine, rosiglitazone) could act as potential therapeutic drugs for the treatment of TNBC.
Breast cancer is one of the most frequent malignancies and the common cause of cancer death in women (1). Breast cancer is a heterogeneous tumor with histological morphological and genomic differences. Based on genomic differences, it can be divided into molecular subtypes such as HER2, Lumina A, Lumina B, and triple-negative breast cancer (TNBC) (2). In TNBC, progesterone receptor (PR), estrogen receptor (ER) and human epidermal growth-factor receptor 2 (HER2) are all negatively expressed (3). Thus, conventional targeted therapies are ineffective in patients with TNBC. Compared with those of other breast cancer subtypes, TNBC patients have normally shorter survival time, higher risk of recurrence and invasion with the extension of treatment time and the development of drug resistance (4). In recent years, the platinum containing regimen has been the most studied and effective therapy for TNBC patients (4), and neoadjuvant chemotherapy has been considered as the preferred approach for the majority of the patients with early-stage TNBC due to the ability of increasing their prognosis and survival (5). However, Poggio et al. found that the platinum-based neoadjuvant chemotherapy was associated with a worse hematological toxicity (6). With the development of bioinformatics theory and emerging study of ferroptosis in cancer research area, clinical researchers gradually turned their focus to novel ferroptosis-related targets for TNBC treatment.
Ferroptosis is a new non-programmed apoptosis death process discovered by chemical screening (7). It is mainly caused by mitochondrial lipid peroxidation via iron-dependent accumulation of reactive oxygen species (ROS) (7). In recent years, induction of ferroptosis has appeared as a prospective therapeutic choice, especially for those malignancies that are resistant to conventional treatments (8). For examples, Ding et al. used breast cancer cells (MDA-MB-231, SUM159, BT-574) and found that DMOCPTL directly bound to the glutathione peroxidase 4 (GPX4) to up-regulate early growth response 1 (EGR1) in TNBC cells, leading to apoptosis of mitochondrial mediator and further inhibiting breast tumor growth (9). Song et al. used breast cancer cell lines (HS578t and MDA-MB-231) and found that the expression of GPX4 was increased in gefitinib-resistant cells, and when GPX4 was silenced, the expression of intracellular malignant malonaldehyde (MDA) and ROS increased and the expression of glutathione (GSH) decreased, which promoted the ferroptosis of cells, and then increased the sensitivity of cells to gefitinib (10). Wen et al. found that 18β-glycyrrhetinic acid (GA) promoted the production of ROS and reactive nitrogen species (RNS) by activating inducible nitric oxide synthase (iNOS), and then suppressed the activity of GSH and GPX4 by deactivating nicotinamide adenine dinucleotide phosphate oxidase (NADPH), thereby aggravating lipid peroxidation and triggering ferroptosis in breast cancer cells (MDA-MB-231) (11). Therefore, it is a new idea to regulate tumor progression by regulating ferroptosis.
Bioinformatics can be used to pre-screen differentially expressed genes and further construct a prognostic model, in which we can find the potential biomarkers related to the survival and prognosis of patients and discover the potential novel targets associated with tumors, providing additional support for research into disease mechanisms and new drugs. In this study, based on the public TNBC profiles from the Cancer Genome Atlas (TCGA), the Gene Expression Omnibus (GEO) database, and the clinical TNBC samples from the University of California Santa Cruz (UCSC) databases, we explored the potential oncogenesis and progression mechanisms of ferroptosis in TNBC from the perspectives of gene expression, prognostic model, biological pathways, immune infiltrations and target drugs. We believe this study offers novel characteristic biomarkers and medications for TNBC, broadens the clinical significance of the Cox model from prognosis prediction to precise therapy, and provides the researchers a new way of integrated bioinformatic analysis on predicting potential ferroptosis-related targets and drugs of TNBC.
1,222 cases of breast cancer (including 113 normal cases and 1,109 tumor cases) were obtained from TCGA database (https://portal.gdc.cancer.gov/) and the clinical and phenotype data of breast cancer were downloaded from UCSC database (http://xena.ucsc.edu/). GSE31519 dataset was downloaded from GEO database (https://www.ncbi.nlm.nih.gov/geo/) and the ferroptosis-related genes were obtained from the FerrDB data (http://www.zhounan.org/ferrdb/current/) for subsequent analysis and verification.
122 cases related to TNBC from 1109 cases of breast cancer were screened out by the clinical and phenotypic data from UCSC database. And 62 cases related to TNBC from GSE31519 dataset were screened out by the clinical information of GEO dataset.
TNBC data from the TCGA database were differentially analyzed and visualized using R version 4.05 (https://www.R-project.org, R Foundation for Statistical Computing, Vienna, Austria). Among them, the “limma” package was applied to obtain differentially expressed genes with adjusted P-value < 0.05, and |logFC| > 0, and the “ggrepel” and “ggplots2” packages were used to draw and visualize the volcano plot of the differential genes. Adjusted P-value took in account the false discovery rate (FDR), could help reduce the number of false positives.
The genes related to ferroptosis from the FerrDB database were intersected with the differential genes to obtain consensus genes, and the expression matrix of the consensus genes was extracted. Survival analysis was performed on the consensus gene using R. Using adjusted P-value (FDR) < 0.05 as the screening condition, the “survival” package was used to analyze OS survival data and obtain ferroptosis-related genes with prognostic value.
Using the “survival” and “survminer” packages, the multivariate Cox analysis was performed on the ferroptosis-related genes with prognostic value. And then a prognostic model was established. Among the genes constituting the prognostic model, the genes with adjusted P-value (FDR) < 0.05 could exist as independent prognostic factors for TNBC. The risk scores of patients were obtained according to the expression and the correlation coefficients of the prognostic genes. And then the patients were separated into two risk groups (high and low) by the median of the risk scores, the survival rates of the two groups were also calculated. The ROC curve was drawn to verify the reliability of the prognostic model by “timeROC” package. The GEO data was utilized to verify the dependability of the prognostic model, and the “Pathology” and “Tissue” modules in the Human Protein Atlas (HPA) database (https://www.proteinatlas.org) were used to further confirm the protein expression of the genes.
For the purpose of further exploring the reliability of the prognosis model, the clinical data (Age, Stage, T, N, M and Risk Score of the model) from UCSC database were sorted, and the “survival” and “surviviner” packages were used for univariate and multivariate Cox analysis. Among them, the clinical factors with adjusted P-value (FDR) < 0.05 could exist as independent prognostic factors.
To explore the specific functions and mechanisms of ferroptosis-related genes in TNBC patients, the Gene Ontology (GO) and Kyoto Encyclopedia of Genes and Genomes (KEGG) analysis were acted on the expression matrices of the consensus genes by utilizing the “clusterProfiler”, “org.Hs.eg.db”, “enrichplot” and “ggplot2” packages. The GO functional annotations and KEGG pathways associated with the consensus genes were screened out with adjusted P-value (q-value) < 0.05, which was also calculated by taking the FDR into account to reduce the number of false positives.
To investigate the immune infiltration of cases, TNBC data from TCGA were immunized using the “ggplot2”, “reshape2”, “ggpubr” and “dplyr” packages with “ Cibersort.R “ data and 22 immune cell types (LM22) as background files (12). Among them, the Permutations coefficient was 1000, and the CIBERSORT output value RMSE was normalized to one to explore the different immune infiltration between the normal group and the tumor group (12).
To further study the role of genes, the “Discover” function of the Comparative Toxicogenomics Database (CTD) database (http://ctdbase.org/) was used to explore the compounds that interact with the genes constituting the prognostic model. And the compounds were arranged by “Interaction Count”. Compounds with Interaction count value greater than or equal to 45 were screened out as the examples of potential compounds related to TNBC treatment.
Figure 1 shows the flowchart of the study in the identification of ferroptosis-related prognostic model related to the diagnosis and treatment of TNBC. In this study, the differentially expressed mRNAs (diff-mRNAs) were first screened out by the TNBC-related data from the TCGA database and the ferroptosis-related genes (ferrDB) were downloaded from the FerrDB database. Then, the consensus genes were obtained by the intersection of diff-mRNAs and ferrDB. Subsequently, the signal pathways and biological functions associated with the consensus genes were analyzed through the KEGG and GO databases respectively. Meantime, a prognostic model with the ability to predict the patients’ survival was constructed based on the consensus genes through univariate and multivariate Cox analyses and further validated using the dataset derived from GEO database. After that, the abnormal expressions of the prognostic model-related key genes were also verified in the HPA database and the clinic prognostic factors were analyzed using multifactorial independent analysis. Moreover, the immune infiltration of the immune cells in the normal and tumor samples related to TNBC was further evaluated and compared with each other via the CIBERSORT analysis tool. Finally, the potential drugs interacted with the model-related key genes were mined in the CTD database.
235 relevant cases from the TCGA database (containing 113 normal cases, 122 TNBC cases) were performed for differential analysis. A total of 13,245 significant genes were obtained, of which 6,636 genes were down-regulated and 6,879 genes were up-regulated in expression (Figure 2).
Figure 2 Volcano map of differentially expressed genes from TCGA. Red dots indicate up-regulated genes, blue dots indicate down-regulated genes.
A total of 259 ferroptosis-related genes were acquired from the FerrDB database, and 177 consensus genes were obtained by intersecting them with the differentially expressed genes obtained above. By univariate Cox analysis of consensus genes, 98 genes associated with the prognosis of TNBC patients were acquired. Subsequently, 29 prognostic genes were identified with adjusted P-value < 0.001 for subsequent multivariate Cox analysis (Table 1).
According to the results of KEGG pathway enrichment and GO functional analysis, 117 consensus gene of the TNBC sample mainly enriched in ferroptosis, autophagy-animal, chemical carcinogenesis-reactive oxygen species pathways (Figure 3A), response to oxidative stress, cellular response to chemical stress, cellular response to oxidative stress etc. in BP, and melanosome, pigment granule, secondary lysosome etc. in CC, ubiquitin protein ligase binding, ubiquitin-like protein ligase binding, antioxidant activity etc. in MF (Figure 3B). The top five most statistically significant (smallest adjusted P-value) pathways and functions were further demonstrated for gene enrichment, including ferroptosis, autophagy-animal (Figure 3C) and response to oxidative stress and cellular response to oxidative stress (Figure 3D), etc.
Figure 3 KEGG pathway and GO function analysis of consensus genes. (A) Histogram of KEGG pathway enrichment analysis. (B) Histogram of GO functional enrichment analysis. (C) Conceptual diagram of the gene network of the top five KEGG significantly enriched pathways. (D) Conceptual diagram of the gene network of the top five GO significantly enriched functions.
Multivariate Cox analysis was performed on the above 29 genes to obtained 12 genes correlated with the prognosis of TNBC patients (Table 2), and the prognosis model was composed of them, including CDKN2A, TF, DPP4, LAMP2, ASNS, HMGB1, ZFP69B, ELAVL1, HELLS, CAV1, PSAT1 and SLC7A11 (Figure 4). Among them, CDKN2A, LAMP2, ASNS, HMGB1, ZFP69B, ELAVL1, HELLS, PSAT1 and SLC7A11 were up-regulated and TF, DPP4 and CAV1 were down-regulated in TNBC compared with normal tissues (Table 2). In addition, CDKN2A, TF, DPP4, LAMP2, ASNS, HMGB1, ZFP69B could serve as independent prognostic genes in this prognostic model (adjusted P-value < 0.05, Figure 4). Patients Risk score = (0.361879*CDKN2A) + (-0.17576*TF) + (-0.32256*DPP4) + (0.723853*LAMP2) + (-0.60588*ASNS) + (0.622896*HMGB1) + (0.556995*ZFP69B) + (-0.91682*ELAVL1) + (-0.39918*HELLS) + (0.228499*CAV1) + (0.229658*PSAT1) + (0.226121*SLC7A11). The risk status showed the number of patient deaths in the high-risk group was greater than that in the low-risk group (Figure 5A). In addition, the survival curves illustrated the survival rates of the low-risk group was remarkably higher than that of the high-risk group (3-year survival rate was 83.69% in high-risk group, 95% CI = 75.57% - 92.70%, and 89.30% in low-risk group, 95% CI = 82.90%- 96.20%; 5-year survival rate was 55.42% in high-risk group, 95% CI = 43.01% -71.40% and 87.00% in low-risk group, 95% CI = 79.40%- 95.20%) (adjusted P = 6.73e-08, Figure 5B). The ROC curve with an AUC value of 0.82 indicated that the prognostic model could forecast the prognosis of TNBC patients well (Figure 5C).
Figure 4 Risk forest plot of 12 prognostic genes. “*” represents adjusted P-value < 0.05, “**” represents adjusted P-value < 0.01.
Figure 5 The construction and the validation of the prognostic model for TNBC patients. The data on the left were from TCGA data and the data on the right were from GEO data. From top to bottom were the risk score of patients, patient survival status, expression profiles of the 12 prognosis-related genes (A, D), Kaplan-Meier curves for the high-risk and low-risk groups (B, E) and the ROC curves (C, F).
The prognostic model was further validated by the gene expression and the clinical data from the TNBC data in the GEO dataset GSE31519. In accordance with the TCGA data, there was an increase in the number of patient deaths as the risk score increased (Figure 5D). Besides, due to the less clear of the expression pattern between low- and high-risk samples in Figure 5D, their detailed characteristics, including the survival status, average survival time, tumor grade, lymph node meta, and the mean/median expression levels of 12 prognostic genes, were summarized in Supplementary Table 1, which clearly showed the consistent results derived from different patient samples stored in TCGA and GSE databases respectively on the whole. The survival curve displayed that the patient’s survival rate was higher in the low-risk group than that in the high-risk group (Figure 5E). And the ROC curve (AUC = 0.77) indicated that the prognostic model in the validation set also forecasted the prognosis of TNBC patients well, indicating the reliability and independence of the prognostic model (Figure 5F).
Figure 6 illustrated the staining of ductal carcinoma tissue for the genes constituting the prognostic model in the HPA database, and the left side denotes the normal group and the right side represents the tumor group. There were no associated proteins were detected in the tissue sections for DPP4 (date not shown) and no tissue sections for SLC7A11. The results of the HPA data showed that the tissue-stained sections for the remaining 10 prognostic model genes (CDKN2A, TF, ZFP69B, PSAT1, HMGB1, ELAVL1, ASNS, LAMP2, CAV1, HELLS) were consistent with our predictions (Figure 6). The HPA database only has sections of tissue classified according to pathological features, while TNBC is predominantly invasive ductal carcinoma (13), so we chose sections of ductal carcinoma tissue for this exploration. The staining of these tissue sections in the HPA database also demonstrated the accuracy of our computational predictions.
Figure 6 Staining of 10 out of 12 prognostic genes in ductal carcinoma tissue sections from the HPA database. In each set, normal tissue sections are shown on the left and tumor tissue sections are shown on the right.
Using the clinical data downloaded from the TCGA and UCSC databases, we conducted a multifactorial independent analysis to assess their clinical risk factors. The results (Table 3) showed that the adjusted P-value for the Risk Score in the multi-factor analysis was less than 0.05, indicating that the Risk Score value could exist as an independent prognostic factor. The adjusted P-value for the Risk Score in the univariate analysis was greater than 0.05, which might be due to the multicollinearity caused by the influence of confounding factors (HR value including 1). In addition, the clinical risk factor N (Node, referring to the lymph node metastasis of the tumor) had an adjusted P-value of less than 0.05 in both multifactorial as well as univariate analyses, and its HR was greater than 1, suggesting that it may play a notable role in the tumor progression of TNBC patients.
Based on the immune infiltration of 22 immune cells in the 113 normal cases, 122 TNBC cases, we could see that the tumor group of the TNBC data had significantly lower proportions of T cells regulatory (Tregs), T cells CD8, T cells CD4 naïve, T cells gamma delta, T cells CD4 memory resting, and eosinophils (P-value < 0.01), while the proportions of B cells memory, neutrophils, B cells naïve, monocytes, macrophages M1, and plasma cells were notably increased (P- value < 0.001) (Figure 7).
Figure 7 Immune infiltration composition of the 22 immune cells in the cases was predicted by the CIBERSORT algorithm for the 113 normal cases, 122 TNBC cases. Green denotes the normal group and the red denotes the tumor group. “*” represents adjusted P-value < 0.05, “**” represents adjusted P-value < 0.01, “***” represents adjusted P-value < 0.005, “****” represents adjusted P-value < 0.001.
2442 compounds with interaction value ranged from 1 to 50 were obtained from the prediction of CTD (Supplementary Table 2). Table 4 listed the top six compounds interacting with the key genes: decitabine (Figure 8A), dexamethasone, lipopolysaccharides, iron, 1-methyl-3-isobutylxanthine (Figure 8B), rosiglitazone (Figure 8C). Among them, decitabine, dexamethasone had an interaction value of 50, lipopolysaccharides, iron had an interaction value of 46 and 1-methyl-3-isobutylxanthine, rosiglitazone had an interaction value of 45, these compounds may have a potential effect on TNBC.
Figure 8 Compounds with potential therapeutic effect on key genes. (A) decitabine (B) 1-methyl-3-isobutylxanthine (C) rosiglitazone. The structures of the compounds with potential therapeutic value from the CTD database were redrawn by Indraw after taking the reference of those shown in the PubChem database.
TNBC is the most aggressive molecular subtype of breast cancer and it is highly heterogeneous in terms of the tumor microenvironment. Despite advance in the treatment, the prognosis of TNBC patients remains poor (14). Therefore, it is very important to explore potential therapeutic or therapeutic-promoting biomarkers and drugs. This study is one of the pioneer studies to investigate the prognosis of TNBC from the perspective of ferroptosis. In this study, we used TCGA and UCSC databases and their molecular characteristics to screen out relevant cases (113 normal cases and 122 TNBC cases), and performed differential analysis (13,245 differentially expressed genes). Subsequently, 177 consensus gene were obtained by intersecting the differentially expressed genes with the ferroptosis-related genes. Using consensus genes and univariate Cox, 98 consensus gene with prognostic value were screened out. Finally, using multivariate Cox and consensus genes with adjusted P-value < 0.01, we successfully constructed a prognostic model composed of ferroptosis-related genes (the AUC was 0.82). We used the relevant dataset GSE31519 of GEO database to confirm the prognostic model (the AUC was 0.77), and also used the HPA database to validate the expression of the genes constituting the prognostic model. Since TNBC and ductal carcinoma are not identical, ductal carcinoma tissue sections cannot fully represent TNBC data, which might be the reason for the absence of DPP4 protein staining in the sections. And the results indicated that the expressions of the genes were basically consistent with our prediction by calculation.
We checked the relevant literature to explore the expression and the possible mechanisms in ferroptosis related genes (Tables 5, 6). As shown in Table 5, experimental studies have shown that CDKN2A, PSAT1, HMGB1, ELAVL1, and SLC7A11 were up-regulated in TNBC, ASNS and LAMP2 were up-regulated in breast cancer and CAV1 was down-regulated in breast cancer, and HELLS was up-regulated in other tumors. In addition, computational prediction indicated that DPP4 was down-regulated in breast cancer, TF was down-regulated in other tumors and ZFP69B was up-regulated in other tumors. The expression trends of all these genes were aligning with our models. Thus, all these studies demonstrated the reliability of our computational predictions.
It could be seen from Table 6 that CDKN2A, PSAT1, SLC7A11, ASNS, LAMP2, CAV1, ZFP69B and ELAVL1 may contribute to the process of ferroptosis in cells through the “antioxidant defence”; TF may contribute to the process of ferroptosis in cells through the “iron toxicity”; HMGB1 and DPP4 may participate in the process of ferroptosis in cells through the “lipid peroxidation”; HELLS may contribute to the process of ferroptosis in cell through “iron toxicity” and “lipid peroxidation”. The mechanism of these genes in ferroptosis could further help the treatment of TNBC from the perspective of ferroptosis.
From the above, it could be seen that the abnormal expression of ASNS, LAMP2, CAV1, DPP4 in triple-negative breast cancer and HELLS, TF, ZFP69B in breast cancer have not been experimentally verified, and these genes could exist as potential treatment biomarkers of TNBC. In addition, the possible ferroptosis mechanisms of CDKN2A, PSAT1, SLC7A11, LAMP2, CAV1, and HMGB1 in TNBC and ASNS, ZFP69B, ELAVL1, TF, HELLS, and DPP4 in breast cancer have not been reported. The expression of these genes in TNBC and the possible mechanism of ferroptosis deserve further exploration, which will supply new scientific guidance for the treatment of TNBC patients.
In order to further explore the role of drugs interacting with genes in TNBC, we also queried the literature related to drugs (Table 7). As shown in Table 7, decitabine, rosiglitazone and 1-methyl-3-isobutylxanthine (Figure 8) could inhibit the development of tumor cells. In the process of inducing ferroptosis in tumor cells, decitabine may play the role of “antioxidant defence” and “lipid peroxidation”; rosiglitazone may play the role of “lipid peroxidation”; 1-methyl-3 -Isobutylxanthine may play a role through “antioxidant defence”. These studies may provide help for drug-induced ferroptosis in TNBC cells.
As for dexamethasone, iron and lipopolysaccharide, studies have illustrated that the three can promote the development of tumors. For example, studies have shown that dexamethasone induced the expression of Krüppel-like factor 5 (KLF5) in a time- and dose-dependent manner, thereby reducing the sensitivity of TNBC to the chemotherapeutic drugs paclitaxel and cisplatin (51); excess iron could upregulate the expression of the inflammatory cytokine interleukin-6 (IL-6), which in turn triggered Janus kinase 2 (JAK2)/signal transducer and activator of transcription 3 (STAT3) signaling, ultimately leading to epithelial-mesenchymal transition (EMT) and migration of TNBC cells (52); lipopolysaccharide could up-regulate the expression of protein kinase (TOPK) in breast cancer cells, and then induce the migration and invasion of breast cancer (53).
At the same time, we are also interested in the pathways and biological function enriched in prognostic model genes, so as to lay a foundation for further exploration of the mechanism of TNBC-related ferroptosis. Han et al. showed that overexpression of ATF4 could increase protein synthesis, leading to oxidative stress and cell death (54), and that activation of ATF4 could trigger the transcription of SLC7A11 (19). Nah et al. indicated that phosphorylated CAV1 could trigger autophagy by binding to the Beclin 1 (BECN1)/VPS34 complex under oxidative stress (55). And the BECN1 could be phosphorylated under the stimulation of AMPK, then the phosphorylated BECN1 could promote ferroptosis by regulating the cysteine and glutamate anti-transporter system (56). These studies suggested that autophagy, oxidative stress and post-translational modifications played important roles in ferroptosis, which were consistent with the KEGG pathway and GO functions of “autophagy”, “oxidative stress” and “ubiquitin ligase binding” enriched by consensus genes in the sample data. Therefore, further exploration of pathways and functions could better explore the role of ferroptosis in TNBC, and provide new ideas and guidance for the treatment of TNBC.
The tumor immune microenvironment played a notable role in the mechanisms of drug resistance, and the response of TNBC to drugs was mainly controlled through the acquisition of signals from the tumor immune microenvironment (57). Studies have shown that substances in the tumor microenvironment interacted with the genes. For example. Wang et al. showed that interferon gamma (IFNγ) discharged from CD8 T cells reduced the expression of SLC7A11 and SLC3A2, inhibited the uptake of cystine by tumor cells, which in turn enhanced ferroptosis -specific lipid peroxidation in cancer cells, and increased ferroptosis contributed to the anti-tumor effect of immunotherapy (58). Xu et al. indicated that GPX4 could inhibit lipid peroxidation and ferroptosis in Treg cells, which in turn maintained the activation of Treg cell (59). In addition, some studies have shown that drugs could also alter the components of the tumor immune microenvironment. Bunt et al. illustrated that rosiglitazone in combination with gemcitabine could regulate T-cell populations by increasing circulating CD8+ T cells and intra-tumor CD4+ cells and CD8+ T cells, thereby reducing the progression and metastasis of pancreatic tumor, and further enhancing cell apoptosis (60). Grader-Beck et al. indicated that 1-methyl-3-isobutylxanthine increased endogenous cAMP, thereby inhibiting CD3 and CD28 mediated T cell activation and cytokine production (61). These studies demonstrated that ferroptosis could be promoted by altering the tumor microenvironment. Therefore, understanding the ferroptosis caused by changes in TNBC tumor immune infiltration through various pathways could further help us to explore the treatment of TNBC from the perspective of ferroptosis.
There are a few limitations in this study. The TNBC data were from the publicly available TCGA, GEO and UCSC databases, which means that the samples included in this study were not strictly randomized, and this may increase the sample error in statistics. Besides, only the top six out of 2442 compounds targeting to the genes of Cox model were analyzed as examples, the rest of them remains to be further investigated. In addition, the partial outcomes obtained by prognostic genes, models, signaling pathways and drugs related to ferroptosis, which provided the potential predictability of this study, need further experimental research and clinical verification. However, our study systematically explored the ferroptosis-related prognostic genes, their correlated drugs, immune infiltration and biological functional mechanisms in TNBC, which may provide new insights into ferroptosis-based targeted therapy for this cancer.
In this study, we successfully constructed a prognostic model, which could well predict the prognosis of patients (AUC = 0.82). Seven genes (ASNS, LAMP2, CAV1, DPP4, HELLS, TF, ZFP69B) could be present as potential therapeutic biomarkers for TNBC. Two drugs (rosiglitazone and 1-methyl-3-isobutyl-cryptoxanthine) could be used as potential therapeutic agents for TNBC. The possible ferroptosis mechanisms of seven genes and two drugs could contribute to the induction of ferroptosis in TNBC cells, and supply help for the treatment of TNBC.
The original contributions presented in the study are included in the article/Supplementary Materials. Further inquiries can be directed to the corresponding authors.
ZH, WC, NX, and BL contributed to the design and conception of the study. NX and YL did information retrieval and analysis. NX, BL and ST wrote the manuscript. NX and CY created tables and figures. ZH, WC and BL guided manuscript writing and revised the manuscript. ZH provided financial support. All authors contributed to the article and approved the submitted version.
This work was supported by the Key Discipline Construction Project of Guangdong Medical University (4SG22004G) and the Innovation Project of Guangdong Graduate Education (2020JGXM053).
All authors declare that the research was conducted in the absence of any commercial or financial relationships that could be construed as a potential conflict of interest.
All claims expressed in this article are solely those of the authors and do not necessarily represent those of their affiliated organizations, or those of the publisher, the editors and the reviewers. Any product that may be evaluated in this article, or claim that may be made by its manufacturer, is not guaranteed or endorsed by the publisher.
The Supplementary Material for this article can be found online at: https://www.frontiersin.org/articles/10.3389/fonc.2022.1017041/full#supplementary-material
1. Siegel RL, Miller KD, Fuchs HE, Jemal A. Cancer statistics. CA Cancer J Clin (2022) 72:7–33. doi: 10.3322/caac.21708
2. Goldhirsch A, Winer EP, Coates AS, Gelber RD, Piccart-Gebhart M, Thürlimann B, et al. Personalizing the treatment of women with early breast cancer: Highlights of the st gallen international expert consensus on the primary therapy of early breast cancer 2013. Ann Oncol (2013) 24:2206–23. doi: 10.1093/annonc/mdt303
3. Wolff AC, Hammond ME, Hicks DG, Dowsett M, McShane LM, Allison KH, et al. Recommendations for human epidermal growth factor receptor 2 testing in breast cancer: American society of clinical Oncology/College of American pathologists clinical practice guideline update. J Clin Oncol (2013) 31(31):3997–4013. doi: 10.1200/jco.2013.50.9984
4. Elghazaly H, Rugo HS, Azim HA, Swain SM, Arun B, Aapro M, et al. Cancer conference ( BGICC ) consensus and recommendations for the management of triple-negative breast cancer. Cancers (Basel) (2021) 13(9): 2262. doi: 10.3390/cancers13092262
5. Harbeck N, Gluz O. Neoadjuvant therapy for triple negative and HER2-positive early breast cancer. Breast (2017) 34:S99–S103. doi: 10.1016/j.breast.2017.06.038
6. Poggio F, Bruzzone M, Ceppi M, Pondé NF, La Valle G, Del Mastro L, et al. Platinum-based neoadjuvant chemotherapy in triple-negative breast cancer: a systematic review and meta-analysis. Ann Oncol (2018) 29(7):1497–508. doi: 10.1093/annonc/mdy127
7. Mou Y, Wang J, Wu J, He D, Zhang C, Duan C, et al. Ferroptosis, a new form of cell death: Opportunities and challenges in cancer. J Hematol Oncol (2019) 12:34. doi: 10.1186/s13045-019-0720-y
8. Su Y, Zhao B, Zhou L, Zhang Z, Shen Y, Lv H, et al. Ferroptosis, a novel pharmacological mechanism of anti-cancer drugs. Cancer Lett (2020) 483:127–36. doi: 10.1016/j.canlet.2020.02.015
9. Ding Y, Chen X, Liu C, Ge W, Wang Q, Hao X, et al. Identification of a small molecule as inducer of ferroptosis and apoptosis through ubiquitination of GPX4 in triple negative breast cancer cells. J Hematol Oncol (2021) 14:19. doi: 10.1186/s13045-020-01016-8
10. Song X, Wang X, Liu Z, Yu Z. Role of GPX4-mediated ferroptosis in the sensitivity of triple negative breast cancer cells to gefitinib. Front Oncol (2020) 10:597434. doi: 10.3389/fonc.2020.597434
11. Wen Y, Chen H, Zhang L, Wu M, Zhang F, Yang D, et al. Glycyrrhetinic acid induces oxidative/nitrative stress and drives ferroptosis through activating NADPH oxidases and iNOS, and depriving glutathione in triple-negative breast cancer cells. Free Radic Biol Med (2021) 173:41–51. doi: 10.1016/j.freeradbiomed.2021.07.019
12. Chen B, Khodadoust MS, Liu CL, Newman AM, Alizadeh AA. Profiling tumor infiltrating immune cells with CIBERSORT. Methods Mol Biol (2018) 1711:243–59. doi: 10.1007/978-1-4939-7493-1_12
13. Liao HY, Zhang WW, Sun JY, Li FY, He ZY, Wu SG. The clinicopathological features and survival outcomes of different histological subtypes in triple-negative breast cancer. J Cancer (2018) 9:296–303. doi: 10.7150/jca.22280
14. Vagia E, Mahalingam D, Cristofanilli M. The landscape of targeted therapies in TNBC. Cancers (Basel) (2020) 12:916. doi: 10.3390/cancers12040916
15. Yoon EC, Wilson P, Zuo T, Pinto M, Cole K, Harigopal M. High frequency of p16 and SOX10 coexpression but not androgen receptor expression in triple-negative breast cancers. Hum Pathol (2020) 102:13–22. doi: 10.1016/j.humpath.2020.06.004
16. Metcalf S, Dougherty S, Kruer T, Hasan N, Biyik R, Lindsey S, et al. Selective loss of phosphoserine aminotransferase 1 ( PSAT1 ) suppresses migration , invasion , and experimental metastasis in triple negative breast cancer. Clin Exp Metastasis (2020) 37:187–97. doi: 10.1007/s10585-019-10000-7
17. Lee HJ, Kim A, Song IH, Park IA, Yu JH, Ahn JH, et al. Cytoplasmic expression of high mobility group B1 ( HMGB1 ) is associated with tumor-infiltrating lymphocytes ( TILs ) in breast cancer. Pathol Int (2016) 66:202–9. doi: 10.1111/pin.12393
18. Mehta M, Basalingappa K, Griffith JN, Andrade D. HuR silencing elicits oxidative stress and DNA damage and sensitizes human triple-negative breast cancer cells to radiotherapy. Oncotarget (2016) 7:64820–35. doi: 10.18632/oncotarget.11706
19. Bai X, Ni J, Beretov J, Wasinger VC, Wang S, Zhu Y, et al. Redox biology activation of the eIF2 α / ATF4 axis drives triple-negative breast cancer radioresistance by promoting glutathione biosynthesis. Redox Biol (2021) 43:101993. doi: 10.1016/j.redox.2021.101993
20. Qin C, Yang X, Zhan Z. High expression of asparagine synthetase is associated with poor prognosis of breast cancer in chinese population. Cancer Biother Radiopharm (2020) 35:581–5. doi: 10.1089/cbr.2019.3295
21. Damaghi M, Tafreshi NK, Lloyd MC, Sprung R, Estrella V, Wojtkowiak JW, et al. Chronic acidosis in the tumour microenvironment selects for overexpression of LAMP2 in the plasma membrane. Nat Commun (2015) 6: 8752. doi: 10.1038/ncomms9752
22. Witkiewicz AK, Dasgupta A, Sotgia F, Mercier I, Pestell RG, Sabel M, et al. An absence of stromal caveolin-1 expression predicts early tumor recurrence and poor clinical outcome in human breast cancers. Am J Pathol (2009) 174:2023–34. doi: 10.2353/ajpath.2009.080873
23. Hou X, Yang L, Wang K. HELLS , a chromatin remodeler is highly expressed in pancreatic cancer and downregulation of it impairs tumor growth and sensitizes to cisplatin by reexpressing the tumor suppressor. Cancer Med (2021) 10:350–64. doi: 10.1002/cam4.3627
24. Choy T, Wang C, Phan NN, Dang H, Anuraga G, Liu Y-H, et al. Identification of dipeptidyl peptidase ( DPP ) family genes in clinical breast cancer patients via an integrated bioinformatics approach. Diagnostics (2021) 11(7): 1204. doi: 10.3390/diagnostics11071204
25. Hong X, Roh W, Sullivan RJ, Wong KHK, Wittner BS, Guo H, et al. The lipogenic regulator srebp2 induces transferrin in circulating melanoma cells and suppresses ferroptosis. Cancer Discovery (2021) 11:678–95. doi: 10.1158/2159-8290.CD-19-1500
26. Zheng X, Wang X, Zheng L, Zhao H, Li W, Wang B, et al. Construction and analysis of the tumor-specific mRNA–miRNA–lncRNA network in gastric cancer. Front Pharmacol (2020) 11:1112. doi: 10.3389/fphar.2020.01112
27. Zhang P, Gao K, Zhang L, Sun H, Zhao X, Liu Y, et al. CRL2-KLHDC3 E3 ubiquitin ligase complex suppresses ferroptosis through promoting p14 ARF degradation. Cell Death Differ (2022) 29:758–71. doi: 10.1038/s41418-021-00890-0
28. Jin H, Hong S, Kim J, Jang S, Kim Y. Knock-down of PSAT1 enhances sensitivity of NSCLC cells to glutamine-limiting conditions. Anticancer Res (2019) 39:6723–30. doi: 10.21873/anticanres.13887
29. Harding HP, Zhang Y, Zeng H, Novoa I, Lu PD, Calfon M, et al. An integrated stress response regulates amino acid metabolism and resistance to oxidative stress national institute of environmental health sciences. Mol Cell (2003) 11:619–33. doi: 10.1016/s1097-2765(03)00105-9
30. Lee N, Carlisle AE, Peppers A, Park SJ, Doshi MB, Spears ME, et al. Xct-driven expression of gpx4 determines sensitivity of breast cancer cells to ferroptosis inducers. Antioxidants (2021) 10:1–12. doi: 10.3390/antiox10020317
31. Ye J, Kumanova M, Hart LS, Sloane K, Zhang H, Panis DN, et al. The GCN2-ATF4 pathway is critical for tumour cell survival and proliferation in response to nutrient deprivation. EMBO J (2010) 29:2082–96. doi: 10.1038/emboj.2010.81
32. Saha T. LAMP2A overexpression in breast tumors promotes cancer cell survival via chaperone-mediated autophagy. Autophagy (2012) 8:1643–56. doi: 10.4161/auto.21654
33. Sotgia F, Martinez-outschoorn UE, Howell A, Pestell RG, Pavlides S, Lisanti MP. Caveolin-1 and cancer metabolism in the tumor Microenvironment : Markers , models , and mechanisms. Annu Rev Pathol (2012) 7:423–67. doi: 10.1146/annurev-pathol-011811-120856
34. Dixon SJ, Patel D, Welsch M, Skouta R, Lee E, Hayano M, et al. Pharmacological inhibition of cystine-glutamate exchange induces endoplasmic reticulum stress and ferroptosis. Elife (2014) 3:e02523. doi: 10.7554/eLife.02523
35. Lin Z, Song J, Gao Y, Huang S. Redox biology hypoxia-induced HIF-1 α / lncRNA-PMAN inhibits ferroptosis by promoting the cytoplasmic translocation of ELAVL1 in peritoneal dissemination from gastric cancer. Redox Biol (2022) 52:102312. doi: 10.1016/j.redox.2022.102312
36. Jiang Y, Mao C, Yang R, Yan B, Shi Y, Liu X, et al. EGLN1/c-myc induced lymphoid-specific helicase inhibits ferroptosis through lipid metabolic gene expression changes. Theranostics (2017) 7:3293–305. doi: 10.7150/thno.19988
37. Liu L, Liu S, Luo H, Chen C, Zhang X, He L, et al. GPR30-mediated HMGB1 upregulation in CAFs induces autophagy and tamoxifen resistance in ERα -positive breast cancer cells. Aging (Albany NY) (2021) 13:16178–97. doi: 10.18632/aging.203145
38. Wen Q, Liu J, Kang R, Zhou B, Tang D. Biochemical and biophysical research communications the release and activity of HMGB1 in ferroptosis. Biochem Biophys Res Commun (2019) 510:278–83. doi: 10.1016/j.bbrc.2019.01.090
39. Fan J, Li Y, Levy RM, Fan JJ, David J, Vodovotz Y, et al. Hemorrhagic shock induces NAD(P)H oxidase activation in neutrophils: role of HMGB1-TLR4 signaling. J Immunol (2007) 178:6573–80. doi: 10.4049/jimmunol.178.10.6573
40. Xie Y, Zhu S, Song X, Xie Y, Zhu S, Song X, et al. The tumor suppressor p53 limits ferroptosis by blocking DPP4 activity. Cell Rep (2017) 20:1692–704. doi: 10.1016/j.celrep.2017.07.055
41. Yu J, Qin B, Moyer AM, Nowsheen S, Liu T, Qin S, et al. DNA Methyltransferase expression in triple-negative breast cancer predicts sensitivity to decitabine. J Clin Invest (2018) 128:2376–88. doi: 10.1172/JCI97924
42. Nakajima W, Miyazaki K, Sakaguchi M, Asano Y, Ishibashi M, Kurita T, et al. Epigenetic priming with decitabine augments the therapeutic effect of cisplatin on triple-negative breast cancer cells through induction of proapoptotic factor NOXA. Cancers (Basel) (2022) 14:248. doi: 10.3390/cancers14010248
43. Ohshima Hosoyama S, Davare MA, Hosoyama T, Nelon LD, Keller C. Bortezomib stabilizes NOXA and triggers ROS-associated apoptosis in medulloblastoma. J Neurooncol (2011) 105(3):475–83. doi: 10.1007/s11060-011-0619-0
44. Brglez V, Pucer A, Pungerčar J, Lambeau G, Petan T. Secreted phospholipases A2are differentially expressed and epigenetically silenced in human breast cancer cells. Biochem Biophys Res Commun (2014) 445:230–5. doi: 10.1016/j.bbrc.2014.01.182
45. Mody M, Dharker N, Bloomston M, Wang PS, Chou FS, Glickman TS, et al. Rosiglitazone sensitizes MDA-MB-231 breast cancer cells to anti-tumour effects of tumour necrosis factor-α, CH11 and CYC202. Endocr Relat Cancer (2007) 14:305–15. doi: 10.1677/ERC-06-0003
46. Ishay-ronen D, Diepenbruck M, Kiran R, Kalathur R, Wang J, Hess C, et al. Gain fat-lose Metastasis : Converting invasive breast cancer cells into adipocytes inhibits cancer metastasis. Cancer Cell (2019) 35:17–32.e6. doi: 10.1016/j.ccell.2018.12.002
47. Lv P, Wang W, Cao Z, Zhao D, Zhao G, Li D, et al. Fsk and IBMX inhibit proliferation and proapoptotic of glioma stem cells via activation of cAMP signaling pathway. J Cell Biochem (2019) 120:321–31. doi: 10.1002/jcb.27364
48. Crumpton TL, Seidler FJ, Slotkin TA. Generation of reactive oxygen species by xanthine derivatives in MDA-MB-231 human breast cancer cells. Breast Cancer Res Treat (2001) 66:143–6. doi: 10.1023/a:1010603816003
49. Lee HD, Lee W, Roh E, Seo C, Son J, Lee SH, et al. Manassantin a inhibits cAMP-induced melanin production by down-regulating the gene expressions of MITF and tyrosinase in melanocytes. Exp Dermatol (2011) 20:761–3. doi: 10.1111/j.1600-0625.2011.01296.x
50. Hill HZ, Li W, Xin P, Mitchell DL. Melanin : A two edged Sword? Pigment Cell Res (1997) 10:158–61. doi: 10.1111/j.1600-0749.1997.tb00478.x
51. Li Z, Dong J, Zou T, Du C, Li S, Chen C, et al. Dexamethasone induces docetaxel and cisplatin resistance partially through up-regulating krüppel-like factor 5 in triple-negative breast cancer. Oncotarget (2017) 8:11555–65. doi: 10.18632/oncotarget.14135
52. Cheng M, Liu P, Xu LX. Iron promotes breast cancer cell migration via IL-6/JAK2/STAT3 signaling pathways in a paracrine or autocrine IL-6-rich inflammatory environment. J Inorg Biochem (2020) 210:111159. doi: 10.1016/j.jinorgbio.2020.111159
53. Seol MA, Park JH, Jeong JH, Lyu J, Han SY, Oh SM. Role of TOPK in lipopolysaccharide-induced breast cancer cell migration and invasion. Oncotarget (2017) 8:40190–203. doi: 10.18632/oncotarget.15360
54. Han J, Back SH, Hur J, Lin Y, Gildersleeve R, Shan J, et al. ER-stress-induced transcriptional regulation increases protein synthesis leading to cell death. Nat Cell Biol (2013) 15:481–90. doi: 10.1038/ncb2738
55. Nah J, Yoo S, Jung S, Jeong E, Park M, Kaang B, et al. Phosphorylated CAV1 activates autophagy through an interaction with BECN1 under oxidative stress. Cell Death Dis (2017) 8:e2822. doi: 10.1038/cddis.2017.71
56. Kang R, Zhu S, Zeh HJ, Klionsky DJ, Tang D. BECN1 is a new driver of ferroptosis. Autophagy (2018) 14(12):2173–5. doi: 10.1080/15548627.2018.1513758
57. Deepak KGK, Vempati R, Nagaraju GP, Dasari VR, Nagini S, Rao DN, et al. Tumor microenvironment: Challenges and opportunities in targeting metastasis of triple negative breast cancer. Pharmacol Res (2020) 153:104683. doi: 10.1016/j.phrs.2020.104683
58. Wang W, Green M, Choi JE, Kennedy PD, Johnson JK, Liao P, et al. CD8+ T cells regulate tumour ferroptosis during cancer immunotherapy. Nature (2019) 569:270–4. doi: 10.1038/s41586-019-1170-y
59. Xu C, Sun S, Johnson T, Qi R, Zhang S, Zhang J, et al. The glutathione peroxidase Gpx4 prevents lipid peroxidation and ferroptosis to sustain treg cell activation and suppression of antitumor immunity. Cell Rep (2021) 35:109235. doi: 10.1016/j.celrep.2021.109235
60. Bunt SK, Mohr AM, Bailey JM, Grandgenett PM, Hollingsworth MA. Rosiglitazone and gemcitabine in combination reduces immune suppression and modulates T cell populations in pancreatic cancer. Cancer Immunol Immunother CII (2013) 62:225–36. doi: 10.1007/s00262-012-1324-3
Keywords: triple-negative breast cancer, ferroptosis, prognostic model, immune infiltration, drug prediction
Citation: Xu N, Li B, Liu Y, Yang C, Tang S, Cho WC and Huang Z (2022) Ferroptosis and triple-negative breast cancer: Potential therapeutic targets. Front. Oncol. 12:1017041. doi: 10.3389/fonc.2022.1017041
Received: 11 August 2022; Accepted: 10 November 2022;
Published: 09 December 2022.
Edited by:
Yanqing Liu, Columbia University, United StatesReviewed by:
Xiaolu Zhu, Columbia University, United StatesCopyright © 2022 Xu, Li, Liu, Yang, Tang, Cho and Huang. This is an open-access article distributed under the terms of the Creative Commons Attribution License (CC BY). The use, distribution or reproduction in other forums is permitted, provided the original author(s) and the copyright owner(s) are credited and that the original publication in this journal is cited, in accordance with accepted academic practice. No use, distribution or reproduction is permitted which does not comply with these terms.
*Correspondence: Zunnan Huang, em5faHVhbmdAZ2RtdS5lZHUuY24=; William C. Cho, Y2hvY3NAaGEub3JnLmhr
†These authors have contributed equally to this work
Disclaimer: All claims expressed in this article are solely those of the authors and do not necessarily represent those of their affiliated organizations, or those of the publisher, the editors and the reviewers. Any product that may be evaluated in this article or claim that may be made by its manufacturer is not guaranteed or endorsed by the publisher.
Research integrity at Frontiers
Learn more about the work of our research integrity team to safeguard the quality of each article we publish.