- 1Department of Organ Transplantation, Qilu Hospital, Cheeloo College of Medicine, Shandong University, Jinan, Shandong, China
- 2Department of General Surgery, The Second Hospital of Shandong University, Jinan, Shandong, China
- 3Department of General Surgery, Qilu Hospital, Cheeloo College of Medicine, Shandong University, Jinan, Shandong, China
Objective: Colorectal cancer (CRC) is a common type of malignant tumor of the digestive tract. Tumor mutation burden (TMB) is a potential prognostic indicator of numerous malignant tumors. This study investigated the prognostic value of TMB in CRC.
Methods: This study analyzed the clinical and somatic mutation data of patients with CRC from the Memorial Sloan Kettering Cancer Center (MSKCC) and The Cancer Genome Atlas (TCGA) cohorts. The genetic landscape was visualized using the maftools package in R software. Survival curves were constructed using the Kaplan–Meier method, and Cox regression analysis was performed to confirm that TMB is an independent prognostic indicator. A nomogram was developed to construct the prognostic model, which was evaluated using the C-index, calibration curve, and decision curve analysis.
Results: In patients with CRC, APC mutations indicated longer overall survival (OS), whereas KRAS mutations indicated shorter OS. For all included patients, there was no significant difference in the OS between the TMB-high and TMB-low groups. For patients with KRAS mutations, the OS in the TMB-high group was longer than that in the TMB-low group. Cox regression analysis showed that TMB was an independent prognostic factor in CRC patients with KRAS mutations. This explains the good accuracy of the nomogram prognostic model using TMB and indicates its good prospect in clinical applications.
Conclusions: A high TMB indicates better prognosis in CRC patients with KRAS mutations, thus confirming the value of TMB in clinical applications.
Introduction
Colorectal cancer (CRC) is the third most prevalent malignant tumor worldwide, with high morbidity and mortality (1). Although scientific and clinical advances in its early detection and surgery have increased the 5-year survival rates to 90% and 71% for localized and regionalized CRC, respectively, the 5-year survival rate for metastatic CRC remains low at 14% (2). Routine treatments for CRC currently include surgery, radiotherapy, and chemotherapy (3). In patients with advanced tumors, radiotherapy and chemotherapy elicit numerous side effects owing to their low specificity and their cytotoxicity toward growing and dividing cells (4). Cancer immunotherapy, which involves harnessing the immune system to attack the cancer, is a promising approach to the treatment of CRC (2). However, many patients nonetheless have poor therapeutic outcomes during clinical treatment. There is, therefore, a clear need to identify predictive biomarkers to guide CRC treatment.
Tumor mutation burden (TMB) is defined as the total number of somatic coding errors, base substitutions, and indel mutations found per million bases of DNA, and it can effectively estimate both the mutational and neoantigen loads (5, 6). TMB was first identified as a latent biomarker for immune checkpoint inhibitors (ICIs) in melanoma (7), and it has since been suggested that a high TMB is related to the effectiveness of ICIs. There are indeed indications that TMB could more broadly be a useful indicator of the efficiency of immunotherapy (8, 9). Additionally, TMB is a potential prognostic indicator, although the relationship between TMB and the prognosis of patients with tumors is still a matter of debate. Ballman et al. (10) and Riviere et al. (11) reported that a high TMB was intimately associated with longer survival. Samstein et al. (12) reported no correlation between a high TMB and a more favorable prognosis in patients with advanced cancers who were not treated with ICIs. However, a high TMB has been reported to indicate a worse prognosis for thyroid carcinoma (13), head and neck squamous cell carcinoma (14), and intrahepatic cholangiocarcinoma (15). There have only been a few reports to date on the correlation between TMB and the survival of patients with CRC.
In this study, we analyzed the somatic mutation data and clinical characteristics of patients with CRC in the Memorial Sloan Kettering Cancer Center (MSKCC) and The Cancer Genome Atlas (TCGA) cohorts and determined the most common mutations in driver genes in CRC. The association between TMB and the prognosis of patients with CRC was then explored in terms of the different driver gene mutations, and it was found that TMB is an independent prognostic factor for CRC patients with KRAS mutations.
Materials and methods
Data collection and processing
The clinical information and somatic mutation data of 1,099 patients with CRC from the MSKCC cohort (16) (https://www.cbioportal.org/study/summary?id=crc_msk_2017) and 556 patients with CRC from TCGA cohort (https://portal.gdc.cancer.gov/) were included. All included data were from patients with CRC confirmed by biopsy or operation resection. If the MSKCC database contained data from multiple samples from the same patient, only the most recent data were used. Data with missing clinical information were excluded from the corresponding analyses. TMB was defined as the number of somatic, non-silent, protein-coding mutations in the coding regions per megabase (mut/Mb). The genetic landscape was visualized using the maftools package in R software. Ethics approval was not required for the study. The datasets used and analyzed in this study are publicly available, and the clinicopathological information, including age, sex, TMB, primary tumor location, and stage at diagnosis, was reviewed retrospectively.
Survivability and Cox regression analysis
Based on a TMB cutoff of 10 mut/Mb (17, 18), a total of 1,376 patients with TMB ≤ 10 mut/Mb were assigned into the TMB-low category, while 278 patients with TMB > 10 mut/Mb (one patient lacked TMB data) were included into the TMB-high category. Survival curves were constructed according to the Kaplan–Meier method, and differences between the curves were examined using a log-rank test. A p-value <0.05 was considered statistically significant. Cox regression analysis was then performed to predict whether TMB could be used as an independent prognostic indicator for CRC (674 CRC patients with KRAS mutations and complete clinical information were included).
Construction and validation of the nomogram
To maximize the statistical power and minimize bias in the analysis, propensity score matching was performed with a 7:3 ratio. The following covariates that might affect the prognostic outcomes were included: sex, age, primary tumor location, stage at diagnosis, and TMB. The training and validation groups were analyzed using the chi-square test. The predictive accuracy and the discrimination of the nomogram were assessed using the concordance index (C-index) and decision curve analysis (DCA). A calibration curve was used to compare the degree of agreement between the predicted probabilities of the nomogram and the actual observations.
Statistical analysis
Statistical analyses were performed using IBM Corporation SPSS version 25.0 software. The survival, rms, and stdca.R packages in R (version 4.1.0) were used for the analysis of the prognostic model, and the curves were drawn using the ggplot2 package in R. Statistical significance was set at p < 0.05 in our analyses.
Results
Cohort characteristics
In this study, the MSKCC and TCGA cohorts included 1,099 and 556 patients with CRC, respectively. We used the 341- (IMPACT341) and 410-gene (IMPACT410) panels for the majority of the patients in the MSKCC cohort. Compared to the most recent 468-gene panel (IMPACT468), the unsequenced genes in the earlier versions were assumed to be wild type or non-mutated. Patients in TCGA cohort were examined using whole-exome sequencing (WES), which is considered the gold standard for the calculation of TMB values. The clinical data in this study included age (<60 and ≥60 years), sex (female/male), TMB [low (≤ 10 mut/Mb) and high (>10 mut/Mb)], primary tumor location (left or right), and stage at diagnosis (I–IV). The baseline clinicopathological features of the study cohort and the number of participants with missing data for each variable of interest are listed in Table 1.
Landscape of genetic mutation profiles in CRC
The gene mutation profiles of 1,099 and 556 patients with CRC (1,134 CRC samples) from the MSKCC and TCGA cohorts, respectively, were analyzed using maftools in R software (Figures 1A, B). In brief, these mutations were further classified according to their categories, which were primarily missense mutations. Single nucleotide polymorphisms were more common than insertions or deletions. In terms of single nucleotide variations (SNVs), C>T was the most common in both the MSKCC and TCGA cohorts. The somatic mutation characteristics of the two cohorts were highly similar. The number of variants per sample in TCGA cohort was higher than that of the MSKCC cohort due to TCGA mutation data being examined using WES. The top 10 mutated genes in the MSKCC cohort were APC (75%), TP53 (73%), KRAS (44%), PIK3CA (20%), SMAD4 (15%), MLL2 (9%), FBXW7 (13%), BRAF (12%), TCF7L2 (11%), and SOX9 (9%) (Figure 1A), while the top 10 mutated genes in TCGA cohort were TTN (49%), APC (73%), MUC16 (27%), TP53 (59%), SYNE1 (28%), KRAS (42%), FAT4 (23%), RYR2 (19%), OBSCN (21%), and PIK3CA (27%) (Figure 1B). Thus, both cohorts overlapped in terms of mutations of APC, KRAS, PIK3CA, and TP53.
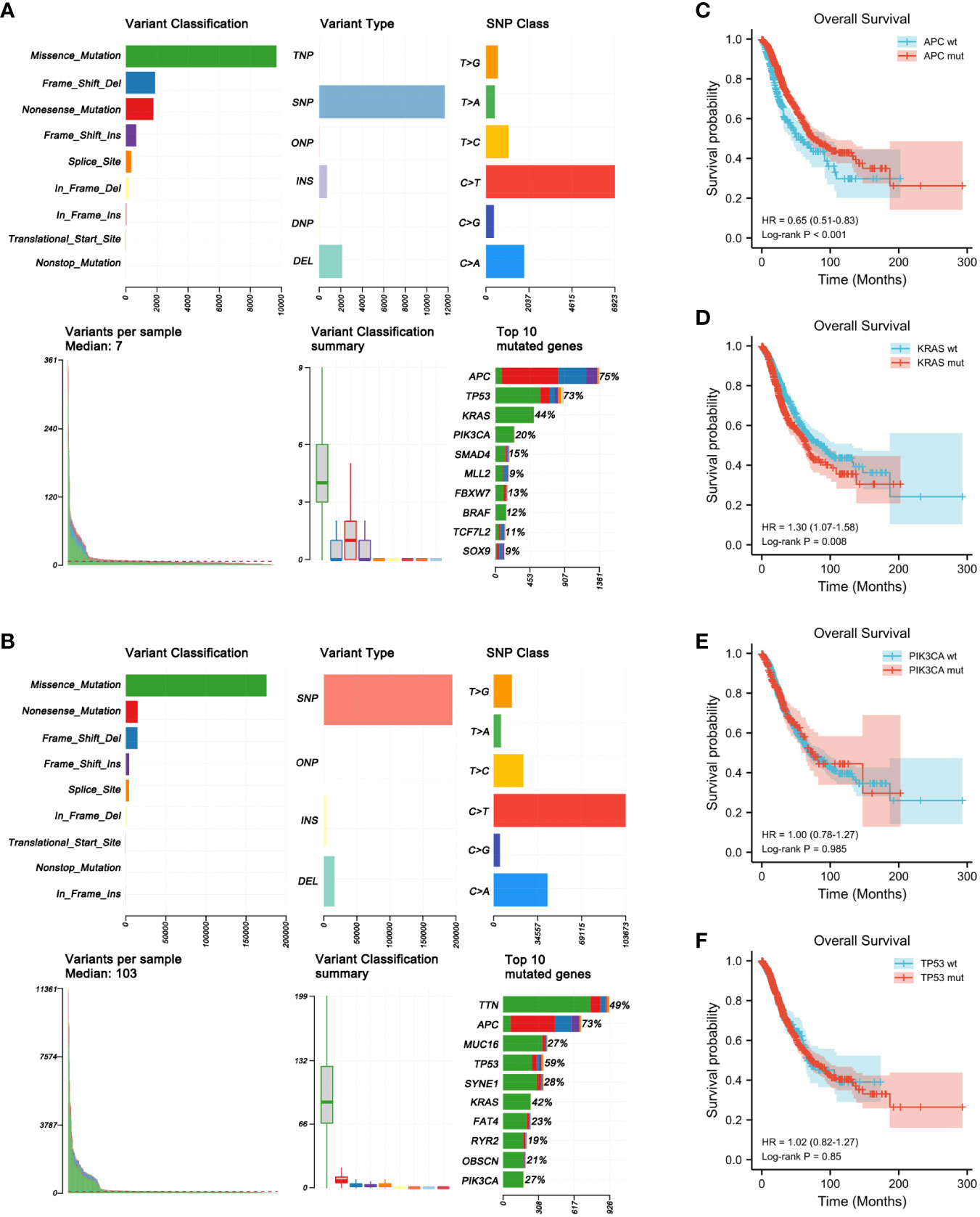
Figure 1 Genome-wide mutation profiling of patients with colorectal cancer (CRC). (A, B) Cohort summary plots for the Memorial Sloan Kettering Cancer Center (MSKCC) (A) and The Cancer Genome Atlas (TCGA) (B) cohorts displaying the distribution of variants according to variant classification, type, and single nucleotide variation (SNV) class. The bottom panel (from left to right) indicates the mutation load for each sample and variant classification type. A stacked bar plot shows the top 10 mutated genes. (C–F) Kaplan–Meier analysis showing the impact of gene mutations on the overall survival (OS) of patients with CRC (1,653 patients from the MSKCC and TCGA cohorts with complete OS data). (C) CRC patients with APC mutations had longer OS than those with wild-type APC (HR = 0.65, p < 0.001). (D) CRC patients with KRAS mutations had shorter OS than those with wild-type KRAS (HR = 1.30, p = 0.008). (E, F) PIK3CA (HR = 1.00, p = 0.985) (E) and TP53 (HR = 1.02, p = 0.850) (F) mutations had no obvious effect on the OS of patients with CRC.
Prognostic value of the mutant genes
To determine the prognostic significance of the frequently mutated genes in CRC, we performed survival analysis using the Kaplan–Meier method with the log-rank test. We divided the patients into two groups (wild-type and mut-type) according to their gene mutation status (Table 2). Survival analysis indicated that CRC patients with APC mutations had longer overall survival (OS) than those with wild-type APC [hazard ratio (HR) = 0.65, p < 0.001] (Figure 1C). CRC patients with KRAS mutations had worse prognosis (HR = 1.30, p = 0.008) than those with wild-type KRAS (Figure 1D). PIK3CA and TP53 mutations had no obvious effect on the prognosis of patients with CRC (PIK3CA: HR = 1.00, p = 0.985; TP53: HR = 1.02, p = 0.850) (Figures 1E, F).
Prognostic impact of TMB
Subsequently, the prognostic value of TMB was investigated. The median TMB of the participants was 5.1 mut/Mb (range = 0.2–336.8 mut/Mb). Using a TMB cutoff of 10 mut/Mb, we stratified the patients into TMB-high (≥10 mut/Mb) and TMB-low (<10 mut/Mb). For the entire cohort of CRC patients with complete TMB and OS data in this study, no significant disparity in OS was observed between the TMB-high and TMB-low groups (HR = 0.82, p = 0.151) (Figure 2A). We then analyzed the survival predictive value of TMB in CRC patients with specific somatic mutation backgrounds. APC mutation did not affect the survival predictive value of TMB, as there was no obvious disparity in the OS data between the TMB-high and TMB-low groups (APC mutation: HR = 0.86, p = 0.372; wild-type APC: HR = 0.66, p = 0.106) (Figures 2B, C). For patients with KRAS mutations, the OS was longer in the TMB-high group than that in the TMB-low group (HR = 0.58, p = 0.013) (Figure 2D), whereas there was no difference in the OS of patients with wild-type KRAS (HR = 1.05, p = 0.797) (Figure 2E). PIK3CA mutation did not affect the survival predictive value of TMB, as there was no significant difference in the OS data between the TMB-high and the TMB-low group (PIK3CA mutation: HR = 0.75, p = 0.256; PIK3CA wild type: HR = 0.84, p = 0.327) (Figures 2F, G). In CRC patients with TP53 mutations, a similar OS was observed in the TMB-high and TMB-low groups (HR = 0.92, p = 0.628) (Figure 2H). In patients with wild-type TP53, the TMB-high group tended to have longer OS than the TMB-low group (HR = 0.66, p = 0.091) (Figure 2I). Since the number of patients reaching the expected events in the TMB-high group was too small, the p-value obtained was not significant. Overall, a high TMB indicated better prognosis in CRC patients with KRAS mutations. The analysis result were listed in Table 3.
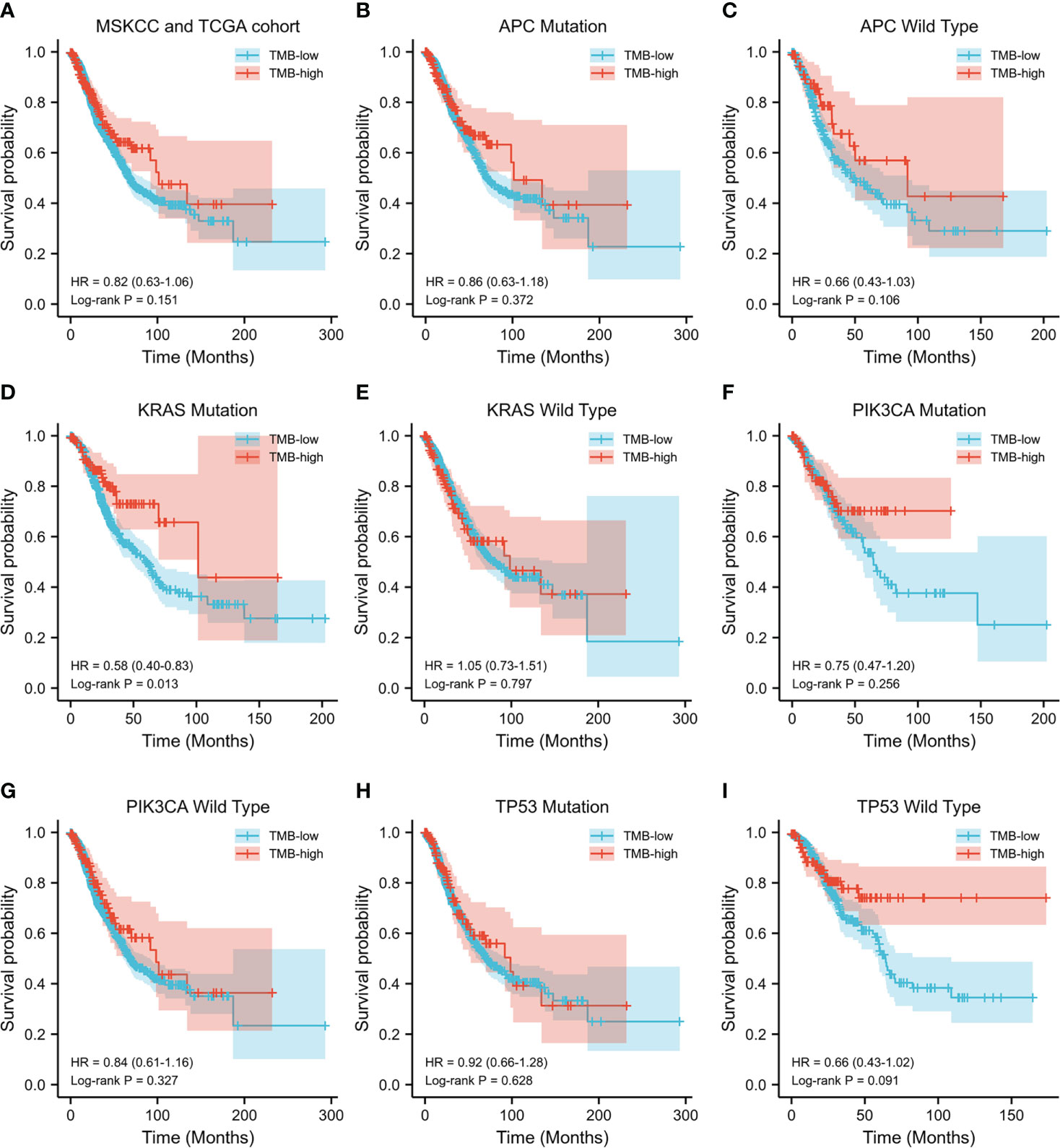
Figure 2 Evaluation of the tumor mutation burden (TMB) to predict the prognosis of patients with colorectal cancer (CRC). (A) Kaplan–Meier curve showing the impact of TMB on the overall survival (OS) of all CRC patients with complete TMB and OS data [1,652 patients from the Memorial Sloan Kettering Cancer Center (MSKCC) and The Cancer Genome Atlas (TCGA) cohorts] in this study (HR = 0.82, p = 0.151). (B–I) Kaplan–Meier analysis showing the impact of TMB on the OS of CRC patients with APC mutations (HR = 0.86, p = 0.372) (B), wild-type APC (HR = 0.66, p = 0.106) (C), KRAS mutations (HR = 0.58, p = 0.013) (D), wild-type KRAS (HR = 1.05, p = 0.797) (E), PIK3CA mutations (HR = 0.75, p = 0.256) (F), wild-type PIK3CA (HR = 0.84, p = 0.327) (G), TP53 mutations (HR = 0.92, p = 0.628) (H), and wild-type TP53 (HR = 0.66, p = 0.091) (I).
Cox regression analysis of CRC patients with KRAS mutations
To identify the independent markers with prognostic significance, we performed a multivariate Cox regression analysis to explore the correlation between OS and specific factors, including age, sex, primary tumor location, and stage at diagnosis, in CRC patients with KRAS mutations. In total, 674 patients with complete clinical information were included in the analysis. The results of the analysis suggested that TMB was an independent prognostic predictor for CRC patients with KRAS mutations (HR = 0.60, 95% CI = 0.38–0.94, p = 0.026). Additionally, age (<60 and ≥60 years) was also an independent prognostic factor for CRC patients with KRAS mutations (HR = 1.39, 95% CI = 1.03–1.87, p = 0.030) (Table 4).
Construction of a prognostic model
To increase the predictive prognostic value of TMB, we established a prognostic model for CRC patients with KRAS mutations. A total of 674 cases with complete clinical information were included in this analysis, of which 485 were randomly assigned to the training group and 189 to the validation group (Table 5). There was no significant difference between the training and validation cohorts for any of the included variables. We established a predictive nomogram model based on the clinical characteristics of the patients in the training group. The total scores for CRC patients with KRAS mutations can be computed to estimate the 1-, 3-, and 5-year survival rates, which would assist clinicians in assessing the risk of these patients in clinical practice (Figure 3). The survival model was assessed using the C-index and calibration plots. The C-index of the nomogram was 0.594 (95% CI = 0.566–0.622), and the calibration curve showed high consistency in the predicted outcomes of the survival model in both the training and validation cohorts (Figures 4A, B). The results of the DCA showed that the nomogram had good accuracy in predicting the OS of CRC patients with KRAS mutations (Figures 4C, D).
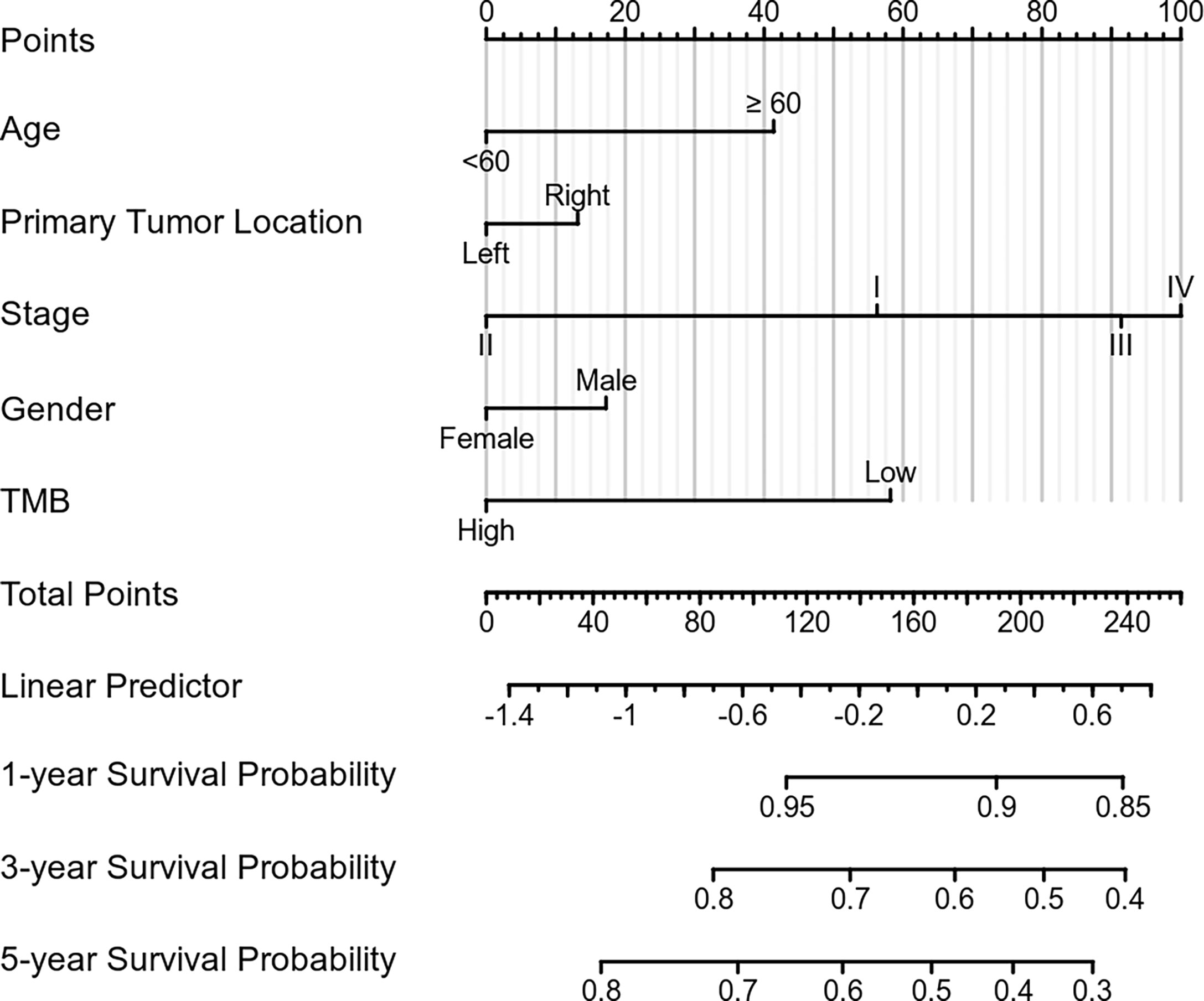
Figure 3 Construction of the prognostic nomogram. Predicted 1-, 3-, and 5-year survival rates in colorectal cancer (CRC) patients with KRAS mutations based on our nomogram, which included age, sex, tumor mutation burden, primary tumor location, and stage at diagnosis (C-index = 0.594, 95% CI = 0.566–0.622).
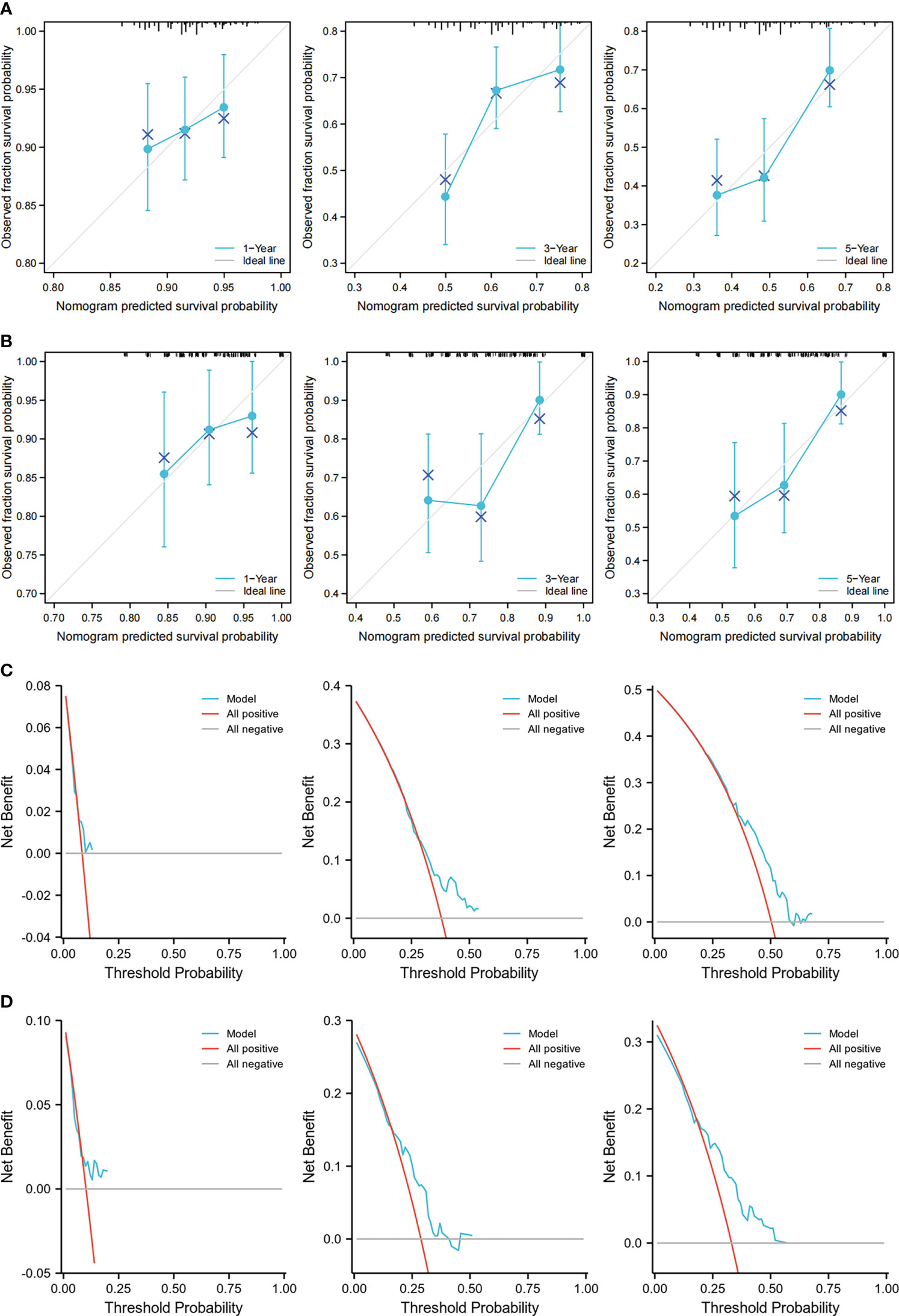
Figure 4 Calibration curves and decision curve analysis (DCA) of the nomogram. (A, B) Calibration curves showing the observation and prediction results of the 1-, 3-, and 5-year survival rates of patients in the training group (C-index = 0.594, 95% CI = 0.566–0.622) (A) and those in the validation group (C-index = 0.635, 95% CI = 0.582–0.688) (B). (C, D) DCA of the nomogram for the 1-, 3-, and 5-year survival prediction of patients in the training group (C-index = 0.594, 95% CI = 0.566–0.622) (C) and those in the validation group (C-index = 0.635, 95% CI = 0.582–0.688) (D).
Discussion
TMB has recently been used to predict the outcome of ICI therapy, acting as an emerging indicator in several cancers to differentiate patients with various malignancies who may derive benefit from immunotherapy (19, 20). TMB has also been reported to be a prognostic predictor in certain types of cancer, such as cutaneous melanoma (21) and bladder cancer (22). TMB can be used as an independent indicator to assess the likelihood of patients with CRC responding well to ICI treatment (23); however, the prognostic value of TMB in CRC remains unclear. Our study illustrated, for the first time, the role of TMB in predicting the survival likelihood of patients with CRC involving KRAS mutation. Firstly, the clinical features and gene mutation data of 1,655 patients with CRC were obtained from the MSKCC and TCGA databases. Of these, 1,376 patients with TMB ≤ 10 mut/Mb were classified into the TMB-low category, while 278 patients with TMB > 10 mut/Mb were assigned into the TMB-high category (one patient lacked TMB data). No significant difference in OS was observed between the included CRC patients in the TMB-high and TMB-low groups in this study. Therefore, a more in-depth analysis is required.
Mutations in driver genes have been shown to play a central role in cancer development (24), determining the malignant behavior of tumors and affecting patient survival. In this study, we investigated the gene mutation profiles of 1,655 patients with CRC from the MSKCC and TCGA cohorts. We found that APC, KRAS, PIK3CA, and TP53 were the most common mutated genes in both cohorts. Furthermore, Kaplan–Meier analysis showed that CRC patients with APC mutations had longer OS than those with wild-type APC. In contrast, KRAS mutations were associated with poor prognosis. Previous studies have suggested that inactivating mutations or deletion of the APC tumor suppressor gene are an early event in the development of CRC (25). The detection of APC gene mutations is useful for the early diagnosis and personalized treatment of CRC. KRAS is a member of the RAS family of genes and is associated with human tumors. KRAS mutations are significantly associated with distant metastasis of CRC and indicate poor prognosis (26).
Several factors are known to influence the prognosis of patients, including psychosocial, socioeconomic, and clinical parameters, and these parameters are routinely used to optimize treatment outcomes, limit risks, and personalize therapeutic strategies (27). TNM assessment continues to be the gold standard for tumor classification (28). Recent studies have indicated that, in CRC, KRAS mutations are related to suppressed immune pathways and that patients with KRAS mutations have altered expression levels of several immune-related genes (29). However, the expression levels of these genes are not routinely assayed in clinical practice, which limits their prognostic application. Our findings indicate that, in patients with KRAS mutations, those in the TMB-high group had longer OS than those in the TMB-low group. Multivariate Cox regression analysis showed that TMB was an independent prognostic factor for CRC patients with KRAS mutations, but not for those with APC mutations.
KRAS mediates numerous signal transduction pathways and plays critical regulatory roles during cell proliferation. KRAS mutations lead to abnormal cell proliferation and oncogenic transformation, promote cancer metastasis, and increase the resistance of several cancer types, including CRC, to chemotherapy and epidermal growth factor receptor (EGFR)-targeted therapy (30). Recent studies have shown that KRAS mutations are associated with poor prognosis in CRC (31–33), which may be related to its accelerated metastatic characteristic (34) and its resistance to chemotherapy and cetuximab (35). KRAS mutations can interact with the IL-22 pathway and enhance tumor cell proliferation (36). Additionally, studies have shown that all KRAS codon 12 alterations and p.G13D mutations are associated with poor prognosis in patients with CRC (37). When combined with chemotherapy, bevacizumab is the most effective first-line treatment for CRC patients with metastasis and KRAS mutations (38, 39). Immunotherapy for patients with KRAS mutations has been reported in recent years, but no clear effects on the outcomes have been observed (40). However, KRAS mutations are associated with high microsatellite instability in CRC (41), suggesting sensitivity to ICI therapy (42). This, in turn, suggests that KRAS-mutant CRC is a unique type of cancer, differing in terms of the treatment scheme and prognosis. A high TMB indicates that the tumor expresses more neoantigens and a greater probability that the immune system will recognize the cancer cells. This may explain why patients with high TMB have better prognosis (43).
In patients with CRC undergoing surgical resection, the prognosis and management are based on the TNM classification. Although the T and N stages are still the main prognostic factors in the current analysis, prognostic factors unrelated to the TNM stage may also affect the prognosis of patients. A nomogram is an easy-to-use prognostic model that helps clinicians in evaluating the prognosis of patients and making clinical decisions. To improve the prognostic value of TMB in CRC patients with KRAS mutations, we divided the enrolled patients into the training and validation groups. A nomogram was constructed based on the clinical characteristics of the training group. The validation group was used to evaluate the accuracy of the nomogram. This study is the first to construct a prognostic model for CRC patients with KRAS mutations. Establishing a nomogram prognostic model greatly improves the clinical value of TMB. Moreover, it is useful for clinicians when evaluating the prognosis of CRC patients with KRAS mutations and making patient-tailored clinical decisions.
Conclusion
In conclusion, KRAS-mutant CRC is associated with poor prognosis and requires special prognostic indicators. With the application of immunotherapy and targeted therapy, somatic mutations and TMB have become the commonly used data in the clinic, which makes TMB more valuable for prognostic prediction. In this study, we analyzed the somatic mutation and TMB data of patients with CRC and found that TMB was an independent prognostic factor in those with KRAS mutations. Our study does, however, have some limitations. This is a retrospective study using data of patients with CRC obtained from a publicly accessible database, which reduced the power of proof. Since the collection of large quantities of CRC case data is time- and resource-intensive, we first opted to use public data for retrospective analysis. Although the conclusions of this study have some limitations, our findings indicate that there is ample merit in collecting the data of patients with CRC from clinical studies to further verify the prognostic value of TMB in those with KRAS mutations.
Data availability statement
The datasets presented in this study can be found in online repositories. The names of the repository/repositories and accession number(s) can be found below: TCGA cohort (https://portal.gdc.cancer.gov/) and MSKCC cohort (https://www.cbioportal.org/study/summary?id=crc_msk_2017).
Author contributions
All authors listed have made a substantial, direct, and intellectual contribution to the work, and approved it for publication.
Funding
This study was supported by the Shandong Natural Science Foundation (no. ZR2021MH339).
Conflict of interest
The authors declare that the research was conducted in the absence of any commercial or financial relationships that could be construed as a potential conflict of interest.
Publisher’s note
All claims expressed in this article are solely those of the authors and do not necessarily represent those of their affiliated organizations, or those of the publisher, the editors and the reviewers. Any product that may be evaluated in this article, or claim that may be made by its manufacturer, is not guaranteed or endorsed by the publisher.
References
1. Bray F, Ferlay J, Soerjomataram I, Siegel RL, Torre LA, Jemal A. Global cancer statistics 2018: GLOBOCAN estimates of incidence and mortality worldwide for 36 cancers in 185 countries. CA Cancer J Clin (2018) 68(6):394–424. doi: 10.3322/caac.21492
2. Lichtenstern CR, Ngu RK, Shalapour S, Karin M. Immunotherapy, inflammation and colorectal cancer. Cells (2020) 9(3):618. doi: 10.3390/cells9030618
3. Schmoll HJ, Van Cutsem E, Stein A, Valentini V, Glimelius B, Haustermans K, et al. ESMO consensus guidelines for management of patients with colon and rectal cancer. A personalized approach to clinical decision making. Ann Oncol (2012) 23(10):2479–516. doi: 10.1093/annonc/mds236
4. Garg MB, Lincz LF, Adler K, Scorgie FE, Ackland SP, Sakoff JA. Predicting 5-fluorouracil toxicity in colorectal cancer patients from peripheral blood cell telomere length: A multivariate analysis. Br J Cancer (2012) 107(9):1525–33. doi: 10.1038/bjc.2012.421
5. Zhang C, Li Z, Qi F, Hu X, Luo J. Exploration of the relationships between tumor mutation burden with immune infiltrates in clear cell renal cell carcinoma. Ann Transl Med (2019) 7(22):648. doi: 10.1186/s12967-019-1805-7
6. Chan TA, Yarchoan M, Jaffee E, Swanton C, Quezada SA, Stenzinger A, et al. Development of tumor mutation burden as an immunotherapy biomarker: utility for the oncology clinic. Ann Oncol (2019) 30(1):44–56. doi: 10.1093/annonc/mdy495
7. Snyder A, Makarov V, Merghoub T, Yuan J, Zaretsky JM, Desrichard A, et al. Genetic basis for clinical response to CTLA-4 blockade in melanoma. N Engl J Med (2014) 371(23):2189–99. doi: 10.1056/NEJMoa1406498
8. Ott PA, Bang YJ, Piha-Paul SA, Razak ARA, Bennouna J, Soria JC, et al. T-Cell-Inflamed gene-expression profile, programmed death ligand 1 expression, and tumor mutational burden predict efficacy in patients treated with pembrolizumab across 20 cancers: KEYNOTE-028. J Clin Oncol (2019) 37(4):318–27. doi: 10.1200/JCO.2018.78.2276
9. Marabelle A, Fakih M, Lopez J, Shah M, Shapira-Frommer R, Nakagawa K, et al. Association of tumour mutational burden with outcomes in patients with advanced solid tumours treated with pembrolizumab: Prospective biomarker analysis of the multicohort, open-label, phase 2 KEYNOTE-158 study. Lancet Oncol (2020) 21(10):1353–65. doi: 10.1016/S1470-2045(20)30445-9
10. Ballman KV. Biomarker: Predictive or prognostic. J Clin Oncol (2015) 33(33):3968–71. doi: 10.1200/JCO.2015.63.3651
11. Riviere P, Goodman AM, Okamura R, Barkauskas DA, Whitchurch TJ, Lee S, et al. High tumor mutational burden correlates with longer survival in immunotherapy-naïve patients with diverse cancers. Mol Cancer Ther (2020) 19(10):2139–45. doi: 10.1158/1535-7163.MCT-20-0161
12. Samstein RM, Lee CH, Shoushtari AN, Hellmann MD, Shen R, Janjigian YY, et al. Tumor mutational load predicts survival after immunotherapy across multiple cancer types. Nat Genet (2019) 51(2):202–6. doi: 10.1038/s41588-018-0312-8
13. Xie Z, Li X, Lun Y, He Y, Wu S, Wang S, et al. Papillary thyroid carcinoma with a high tumor mutation burden has a poor prognosis. Int Immunopharmacol (2020) 89(Pt B):107090. doi: 10.1016/j.intimp.2020.107090
14. Zhang L, Li B, Peng Y, Wu F, Li Q, Lin Z, et al. The prognostic value of TMB and the relationship between TMB and immune infiltration in head and neck squamous cell carcinoma: A gene expression-based study. Oral Oncol (2020) 110:104943. doi: 10.1016/j.oraloncology.2020.104943
15. Zhang R, Li Q, Fu J, Jin Z, Su J, Zhang J, et al. Comprehensive analysis of genomic mutation signature and tumor mutation burden for prognosis of intrahepatic cholangiocarcinoma. BMC Cancer (2021) 21(1):112. doi: 10.1186/s12885-021-07788-7
16. Yaeger R, Chatila WK, Lipsyc MD, Hechtman JF, Cercek A, Sanchez-Vega F, et al. Clinical sequencing defines the genomic landscape of metastatic colorectal cancer. Cancer Cell (2018) 33(1):125–36:e3. doi: 10.1016/j.ccell.2017.12.004
17. Valero C, Lee M, Hoen D, Zehir A, Berger MF, Seshan VE, et al. Response rates to anti-PD-1 immunotherapy in microsatellite-stable solid tumors with 10 or more mutations per megabase. JAMA Oncol (2021) 7(5):739–43. doi: 10.1001/jamaoncol.2020.7684
18. Sha D, Jin Z, Budczies J, Kluck K, Stenzinger A, Sinicrope FA. Tumor mutational burden as a predictive biomarker in solid tumors. Cancer Discov (2020) 10(12):1808–25. doi: 10.1158/2159-8290.CD-20-0522
19. Hugo W, Zaretsky JM, Sun L, Song C, Moreno BH, Hu-Lieskovan S, et al. Genomic and transcriptomic features of response to anti-PD-1 therapy in metastatic melanoma. Cell (2016) 165(1):35–44. doi: 10.1016/j.cell.2016.02.065
20. Carbone DP, Reck M, Paz-Ares L, Creelan B, Horn L, Steins M, et al. First-line nivolumab in stage IV or recurrent non-Small-Cell lung cancer. N Engl J Med (2017) 376(25):2415–26. doi: 10.1056/NEJMoa1613493
21. Kang K, Xie F, Mao J, Bai Y, Wang X. Significance of tumor mutation burden in immune infiltration and prognosis in cutaneous melanoma. Front Oncol (2020) 10:573141. doi: 10.3389/fonc.2020.573141
22. Lv J, Zhu Y, Ji A, Zhang Q, Liao G. Mining TCGA database for tumor mutation burden and their clinical significance in bladder cancer. Biosci Rep (2020) 40(4): BSR20194337. doi: 10.1042/BSR20194337
23. Schrock AB, Ouyang C, Sandhu J, Sokol E, Jin D, Ross JS, et al. Tumor mutational burden is predictive of response to immune checkpoint inhibitors in MSI-high metastatic colorectal cancer. Ann Oncol (2019) 30(7):1096–103. doi: 10.1093/annonc/mdz134
24. Raskov H, Søby JH, Troelsen J, Bojesen RD, Gögenur I. Driver gene mutations and epigenetics in colorectal cancer. Ann Surg (2020) 271(1):75–85. doi: 10.1097/SLA.0000000000003393
25. Müller MF, Ibrahim AE, Arends MJ. Molecular pathological classification of colorectal cancer. Virchows Arch (2016) 469(2):125–34. doi: 10.1007/s00428-016-1956-3
26. Huang D, Sun W, Zhou Y, Li P, Chen F, Chen H, et al. Mutations of key driver genes in colorectal cancer progression and metastasis. Cancer Metastasis Rev (2018) 37(1):173–87. doi: 10.1007/s10555-017-9726-5
27. Law WL, Choi HK, Lee YM, Ho JW. The impact of postoperative complications on long-term outcomes following curative resection for colorectal cancer. Ann Surg Oncol (2007) 14(9):2559–66. doi: 10.1245/s10434-007-9434-4
28. Greene FL, Stewart AK, Norton HJ. A new TNM staging strategy for node-positive (stage III) colon cancer: An analysis of 50,042 patients. Ann Surg (2002) 236(4):416–21; discussion 421. doi: 10.1097/00000658-200210000-00003
29. Liu J, Huang X, Liu H, Wei C, Ru H, Qin H, et al. Immune landscape and prognostic immune-related genes in KRAS-mutant colorectal cancer patients. J Transl Med (2021) 19(1):27. doi: 10.1186/s12967-020-02638-9
30. Van Schaeybroeck S, Kalimutho M, Dunne PD, Carson R, Allen W, Jithesh PV, et al. ADAM17-dependent c-MET-STAT3 signaling mediates resistance to MEK inhibitors in KRAS mutant colorectal cancer. Cell Rep (2014) 7(6):1940–55. doi: 10.1016/j.celrep.2014.05.032
31. Uhlig J, Cecchini M, Sheth A, Stein S, Lacy J, Kim HS. Microsatellite instability and KRAS mutation in stage IV colorectal cancer: Prevalence, geographic discrepancies, and outcomes from the national cancer database. J Natl Compr Canc Netw (2021) 19:307–18. doi: 10.6004/jnccn.2020.7619
32. Scott A, Goffredo P, Ginader T, Hrabe J, Gribovskaja-Rupp I, Kapadia MR, et al. The impact of KRAS mutation on the presentation and prognosis of non-metastatic colon cancer: An analysis from the national cancer database. J Gastrointest Surg (2020) 24:1402–10. doi: 10.1007/s11605-020-04543-4
33. Formica V, Sera F, Cremolini C, Riondino S, Morelli C, Arkenau HT, et al. KRAS and BRAF mutations in stage II and III colon cancer: A systematic review and meta-analysis. J Natl Cancer Inst (2022) 114:517–27. doi: 10.1093/jnci/djab190
34. Nash GM, Gimbel M, Shia J, Nathanson DR, Ndubuisi MI, Zeng ZS, et al. KRAS mutation correlates with accelerated metastatic progression in patients with colorectal liver metastases. Ann Surg Oncol (2010) 17:572–8. doi: 10.1245/s10434-009-0605-3
35. Di Fiore F, Blanchard F, Charbonnier F, Le Pessot F, Lamy A, Galais MP, et al. Clinical relevance of KRAS mutation detection in metastatic colorectal cancer treated by cetuximab plus chemotherapy. Br J Cancer (2007) 96:1166–9. doi: 10.1038/sj.bjc.6603685
36. McCuaig S, Barras D, Mann EH, Friedrich M, Bullers SJ, Janney A, et al. The interleukin 22 pathway interacts with mutant KRAS to promote poor prognosis in colon cancer. Clin Cancer Res (2020) 26:4313–25. doi: 10.1158/1078-0432.CCR-19-1086
37. Taieb J, Le Malicot K, Shi Q, Penault-Llorca F, Bouché O, Tabernero J, et al. Prognostic value of BRAF and KRAS mutations in MSI and MSS stage III colon cancer. J Natl Cancer Inst (2017) 109(5):djw272. doi: 10.1093/jnci/djw272
38. Benvenuti S, Sartore-Bianchi A, Di Nicolantonio F, Zanon C, Moroni M, Veronese S, et al. Oncogenic activation of the RAS/RAF signaling pathway impairs the response of metastatic colorectal cancers to anti-epidermal growth factor receptor antibody therapies. Cancer Res (2007) 67(6):2643–8. doi: 10.1158/0008-5472.CAN-06-4158
39. You XH, Jiang YH, Fang Z, Sun F, Li Y, Wang W, et al. Chemotherapy plus bevacizumab as an optimal first-line therapeutic treatment for patients with right-sided metastatic colon cancer: A meta-analysis of first-line clinical trials. ESMO Open (2020); 5:e000605. doi: 10.1136/esmoopen-2019-000605
40. Chatani PD, Yang JC. Mutated RAS: Targeting the "Untargetable" with T cells. Clin Cancer Res (2020) 26(3):537–44. doi: 10.1158/1078-0432.CCR-19-2138
41. Fujiyoshi K, Yamamoto G, Takahashi A, Arai Y, Yamada M, Kakuta M, et al. High concordance rate of KRAS/BRAF mutations and MSI-h between primary colorectal cancer and corresponding metastases. Oncol Rep (2017) 37(2):785–92. doi: 10.3892/or.2016.5323
42. Oliveira AF, Bretes L, Furtado I. Review of PD-1/PD-L1 inhibitors in metastatic dMMR/MSI-h colorectal cancer. Front Oncol (2019) 9:396. doi: 10.3389/fonc.2019.00396
Keywords: tumor mutation burden, colorectal cancer, KRAS mutation, prognosis, nomogram
Citation: Wang J, Song J, Liu Z, Zhang T and Liu Y (2022) High tumor mutation burden indicates better prognosis in colorectal cancer patients with KRAS mutations. Front. Oncol. 12:1015308. doi: 10.3389/fonc.2022.1015308
Received: 09 August 2022; Accepted: 10 October 2022;
Published: 14 November 2022.
Edited by:
Li Min, Affiliated Beijing Friendship Hospital, Capital Medical University, ChinaReviewed by:
Shaowei Dong, Jinan University, ChinaGianluca Russo, University of Naples Federico II, Italy
Copyright © 2022 Wang, Song, Liu, Zhang and Liu. This is an open-access article distributed under the terms of the Creative Commons Attribution License (CC BY). The use, distribution or reproduction in other forums is permitted, provided the original author(s) and the copyright owner(s) are credited and that the original publication in this journal is cited, in accordance with accepted academic practice. No use, distribution or reproduction is permitted which does not comply with these terms.
*Correspondence: Yanfeng Liu, liu19822012@163.com
†These authors have contributed equally to this work and share first authorship