- 1Institute of Oncology, Shandong Provincial Hospital, Shandong University, Jinan, Shandong, China
- 2Department of Thoracic Surgery, Shandong Provincial Hospital, Shandong University, Jinan, Shandong, China
- 3Research and Development Department, Amoy Diagnostics Co., LTD., Xiamen, Fujian, China
With the development of technologies, multiple primary lung cancer (MPLC) has been detected more frequently. Although large-scale genomics studies have made significant progress, the aberrant gene mutation in MPLC is largely unclear. In this study, 141 and 44 lesions from single and multiple primary lung adenocarcinoma (SP- and MP-LUAD) were analyzed. DNA and RNA were extracted from formalin-fixed, paraffin-embedded tumor tissue and sequenced by using the next-generation sequencing-based YuanSu450TM gene panel. We systematically analyzed the clinical features and gene mutations of these lesions, and found that there were six genes differently mutated in MP-LUAD and SP-LUAD lesions, including RBM10, CDK4, ATRX, NTRK1, PREX2, SS18. Data from the cBioPortal database indicated that mutation of these genes was related to some clinical characteristics, such as TMB, tumor type, et al. Besides, heterogeneity analysis suggested that different lesions could be tracked back to monophyletic relationships. We compared the mutation landscape of MP-LUAD and SP-LUAD and identified six differentially mutated genes (RBM10, CDK4, ATRX, NTRK1, PREX2, SS18), and certain SNV loci in TP53 and EGFR which might play key roles in lineage decomposition in multifocal samples. These findings may provide insight into personalized prognosis prediction and new therapies for MP-LUAD patients.
Introduction
Lung cancer is still the first cause of oncological death. Lung cancer can be divided into two broad categories according to histology: small-cell lung cancer and non-small-cell lung cancer (NSCLC) which accounts for approximately 85%. subdivided into adenocarcinoma (LUAD; 60%), squamous cell carcinoma (LUSC; 30–35%), large cell carcinoma, and other rare tumors, including adenosquamous carcinoma and in recent years, the ratio of LUAD among NSCLC is rising (1).
With the development of technologies, especially high-resolution computed tomography (HRCT), and the conduction of early lung cancer screening, multiple primary lung cancer (MPLC) has been detected more frequently (2–4). In particularly, adenocarcinoma was shown to be the most common pathological type in MPLC (5).
MPLC is a unique type of lung cancer, defined by presence of at least two independent primary tumors. MPLC was divided into synchronous MPLC (sMPLC) and metachronous MPLC (mMPLC) according to the diagnosis interval (6–8). According to current research, surgical resection was still recommended as the first choice for certain MPLC (9–12). Nowadays, with improved research methods such as machine learning, some studies would combine CT findings and gene sequencing technologies to diagnose and distinguish multiple primary lung cancers from pulmonary metastasis (8, 13–16). Understanding the molecular determinants of MPLC is one of the critical challenges in oncology.
Cancer is a genetic disease. Rapid advancing in next-generation sequencing technology and The Cancer Genome Atlas (TCGA) have profiled and analyzed the molecular aberrations at the DNA, RNA levels (17, 18). Genome instability and mutation is one of the hallmarks of cancer (19, 20). The accumulation of somatic mutations in the DNA affected neoplastic transformation, including driver mutations, mutations that directly affect tumor growth, such as TP53, epidermal growth factor receptor (EGFR) or RAS, and passenger mutations, which do not directly impact the growth of the cancer cell (21, 22).
In lung adenocarcinoma, several large-scale genomics studies have analyzed the genomic mutational landscape and some important targets, including EGFR, ALK, RAS, TP53 were identified to be associated with overall survival or treatments (17, 23–26). Besides, the most common therapeutic targets are EGFR and BRAF mutations and ALK and ROS1 rearrangements (17). Despite this progression, the aberrant gene mutation in MPLC compared with single primary lung cancer (SPLC) is largely unclear.
In this study, we included 141 single primary lung adenocarcinoma (SP-LUAD) patients and 44 multiple primary lung adenocarcinoma (MP-LUAD) patients to analysis the mutational landscape and there was an apparently difference between these two groups. RBM10, CDK4, ATRK, NTRK1, PREX2 and SS18 were identified as the significantly differential gene. The relationship between these genes and clinical characters was conducted using cBioPortal database. The study of associated gene mutations in MPLC will provide new insight into the mechanism, potential therapeutic targets, promote the prognosis and survival in clinic.
Material and methods
Patient cohort description
Primary lung cancers were collected between January 2018 and December 2020 in our institution. After sample collection, surgical specimens and biopsy tissues were snap-frozen in liquid nitrogen within 30 minutes of resection. Genomic DNA was extracted from all included samples. 141 SP-LUAD and 44 MP-LUAD patients were included in this study. Among these MP-LUAD specimens, 73 lesions were analyzed, including 9 lesions from 3 three-primary cases, 46 lesions from 23 dual-primary cases and 18 lesions from the other 18 MP-LUAD cases.
MP-LUAD were defined according to Warren and Gate’s criteria: 1) each tumor had to show definite features of malignancy; 2) each cancer had to be anatomically separate and distinct; 3) the possibility that one cancer was a recurrence or metastatic lesion of the first cancer had to be ruled out; and 4) the subsequent primary malignancies had to be present in either the same or different organs (27).
Tumor processing and DNA extraction
Before DNA extraction, 4 μm formalin-fixed paraffin-embedded (FFPE) specimens were taken. Histopathological examination confirmed that the area of each specimen was greater than 1 cm2 and the tumor cell density was greater than 20%.
According to the manufacturer’s instructions, 0.5-2 μg of cancer tissue DNA was extracted from 4 μm FFPE tumor samples using a DNA extraction kit (QIAamp DNA FFPE tissue kit).
Library construction
Libraries were constructed using Roche’s KAPA Hyper Prep Kit according to the manufacturer’s instructions. This custom hybrid capture panel includes more than 23,660 individually synthesized 5’-DNA biotin-labeled 120 bp oligonucleotides to target the approximately 2.6 Mb human genome, which contains 7029 extra coding nucleotides of 468 cancer-related genes exons and selected introns of 39 genes that are frequently rearranged in solid tumors.
Hybridization capture was performed according to the protocol of “IDT Company xGen LOCKDOWN probe and reagent hybridization capture DNA library”, and sequenced on Illumina Nextseq500 with an average coverage of 1000 times. According to this method, paired paired-end sequencing (2 × 150 bp) was obtained by OrigiMed (OrigiMed, College of American Pathologists (CAP) and Clinical Laboratory Improvement Amendments (CLIA) accredited laboratory, Shanghai, China) Comprehensive genomic profiling, including single nucleotide variation (SNV), short and long insertion/deletion (INDELS), copy number variation (CNV), gene rearrangements and gene fusions.
Next-generation sequencing
DNAs of both FFPE tumor tissues and matched blood were obtained by using QIAamp DNA FFPE Tissue Kit and QIAamp DNA Blood Midi Kit (Qiagen, Hilden, Germany), respectively, and sequenced by using the next-generation sequencing-based YuanSu450TM gene panel of OrigiMed (Shanghai, China), from where the laboratory was certified by CAP and CLIA. The genes were captured and sequenced with a mean depth of 800× by using Illumina Nova (Illumina, Inc., CA).
Mutation analysis
Genomic alterations were identified as following: SNVs were identified by MuTect (v1.7). Insertion-deletions (Indels) were identified by using PINDEL (V0.2.5). The functional impact of genomic alterations was annotated by SnpEff3.0. CNV regions were identified by Control FREEC (v9.7) with the following parameters: window = 50 000 and step = 10 000. Gene fusions were detected through an in house developed pipeline. Gene rearrangements were assessed by Integrative Genomics Viewer (IGV). Known somatic mutations in the Catalog of Somatic Mutations in Cancer (COSMIC) and known germline polymorphisms in the U.S. National Center for Biotechnology Information’s Single Nucleotide Polymorphism Database (dbSNP) were not counted. Tumor mutation burden (TMB) was calculated by counting the coding somatic mutations, including SNVs and Indels, per megabase of the sequence examined in each patient. The waterfall chart was drawn using the R report “ComplexHeatmap (version=2.2.0)”, and the difference in gene mutation frequency between single foci and multiple foci was compared using the fisher test.
Phylogenetic analysis
The phylogenetic analysis was conducted using LICHeE as reported before (28). Briefly, the branch evolution of the tumor within each patient was inferred by comparing the list of mutations in each tumor region. Regions containing all mutations observed in another region are indicated as their ancestors. If no such region exists, putative precursors are inferred from a set of changes common to multiple regions. Regions that did not change were considered to be parallel branches, although alternative dendrograms could be formed by assuming that these regions are ancestors of regions with mutations.
Data source
The mutated gene data was recruited from cBioPortal database (http://www.cbioportal.org), originally developed at Memorial Sloan Kettering Cancer Center, an open-access resource for the interactive exploration of multidimensional cancer genomics data sets (29). The database can provide visualization (the associations between genes and clinical characteristics), analysis and downloads of large-scale cancer genomics data sets (the different expressed genes data). The somatic mutation data was acquired from the Cancer Genome Atlas (TCGA) database (https://cancergenome.nih.gov/).
Gene set enrichment analysis
Gene Set Enrichment Analysis (GSEA) was used to analyze the correlation between gene differential expression with cellular pathway (KEGG pathways) and cellular functions (GO-molecular function). “clusterProfiler”, “org.Hs.eg.db”, “enrichplot”, “ggplot2” R packages were applied to perform GSEA and “maftools” for co-occurrence of gene mutations. Adjusted P value < 0.05 was considered as statistically significant.
Statistical analysis
All tests were performed with the R environment version 4.0.2 (Vienna, Austria), SPSS 25.0 (Chicago, US) or GraphPad Prism 7.0 (San Diego, CA). Comparisons of clinical characteristics between paired primary tumors and metastases were based on Student’ s t test, Chi-square test and Fisher’s exact test. The nonparametric Wilcoxon rank-sum test was applied for comparison of mutation counts and branch lengths. If not noted otherwise, the tests applied were two-sided. As per the convention, p<0.05 was considered statistically significant.
Results
Patient characteristics
There were 141 SP-LUAD patients and 44 MP-LUAD patients in the study. Table 1 listed all of the patients’ information. There were 86 males and 99 females in this study, with an average age of 57.7 and 59.3 years for the SP- and MP-LUAD cohorts, respectively. Weight Loss was a statistically significant factor across all these factors, indicating that weight loss may be more common in MP-LUAD. Furthermore, each lesion in these cohorts had a different histologic class. In the MP-LUAD cohort, the ratios of lepidic, adenocarcinoma in situ (AIS), and microinvasive adenocarcinoma (MIA) were higher, while acinar, solid, papillary, and micropapillary were lower.
In Table 2, the right upper lobe lesion accounted for the majority of the cases. There appeared to be no link between smoking or drinking habits and these two types of patients (Table 3), and the amount of smoking packs (daily amount of cigarettes*years of smoking/20) was barely correlated with the largest lesion diameter of MP-LUAD (R square: 0.125) (Figure 1).
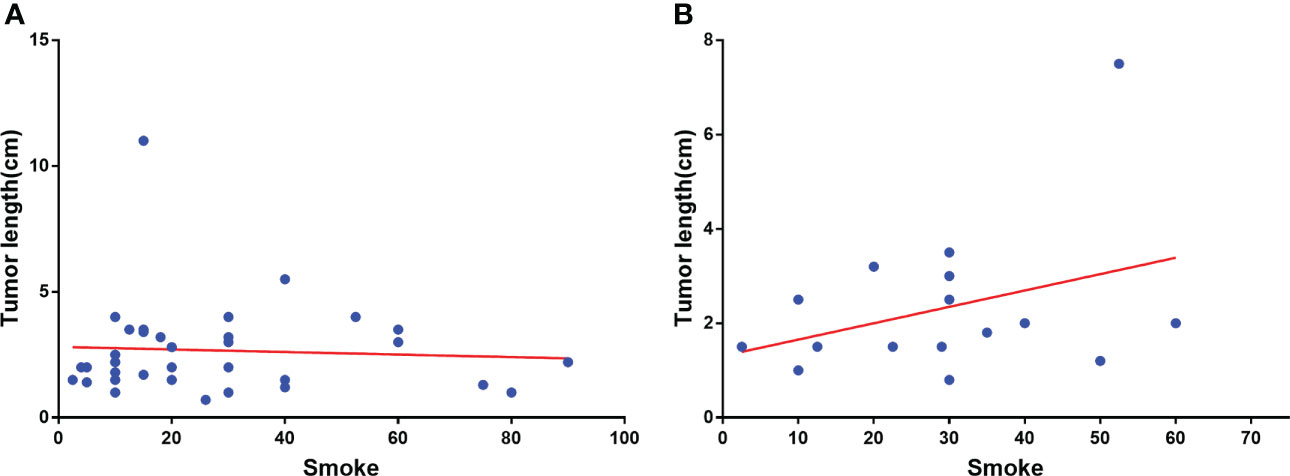
Figure 1 Correlation analysis of smoking amount with the largest lesion diameter of SP-(A) and MP-LUAD (B).
Mutation profile of the SP-LUAD and MP-LUAD cohort
We aimed to analyzed the mutational landscape in the SP-LUAD and MP-LUAD cohort and identified the top 30 most frequently mutant genes in SP-LUAD cohort, including EGFR, TP53, LRP1B, FRS2, RBM10, MDM2, PIK3CA, RB1, KRAS, SPTA1, ERBB2, GNAS, NFKBIA, NKX2-1, FAT3, ATM, FOS, HDAC9, RET, SDHA, ALK, CTNNB1, EPHA3, GLI2, KMT2D, LRP2, RICTOR, SMAD4, TERT, FAM135B (Figure 2).
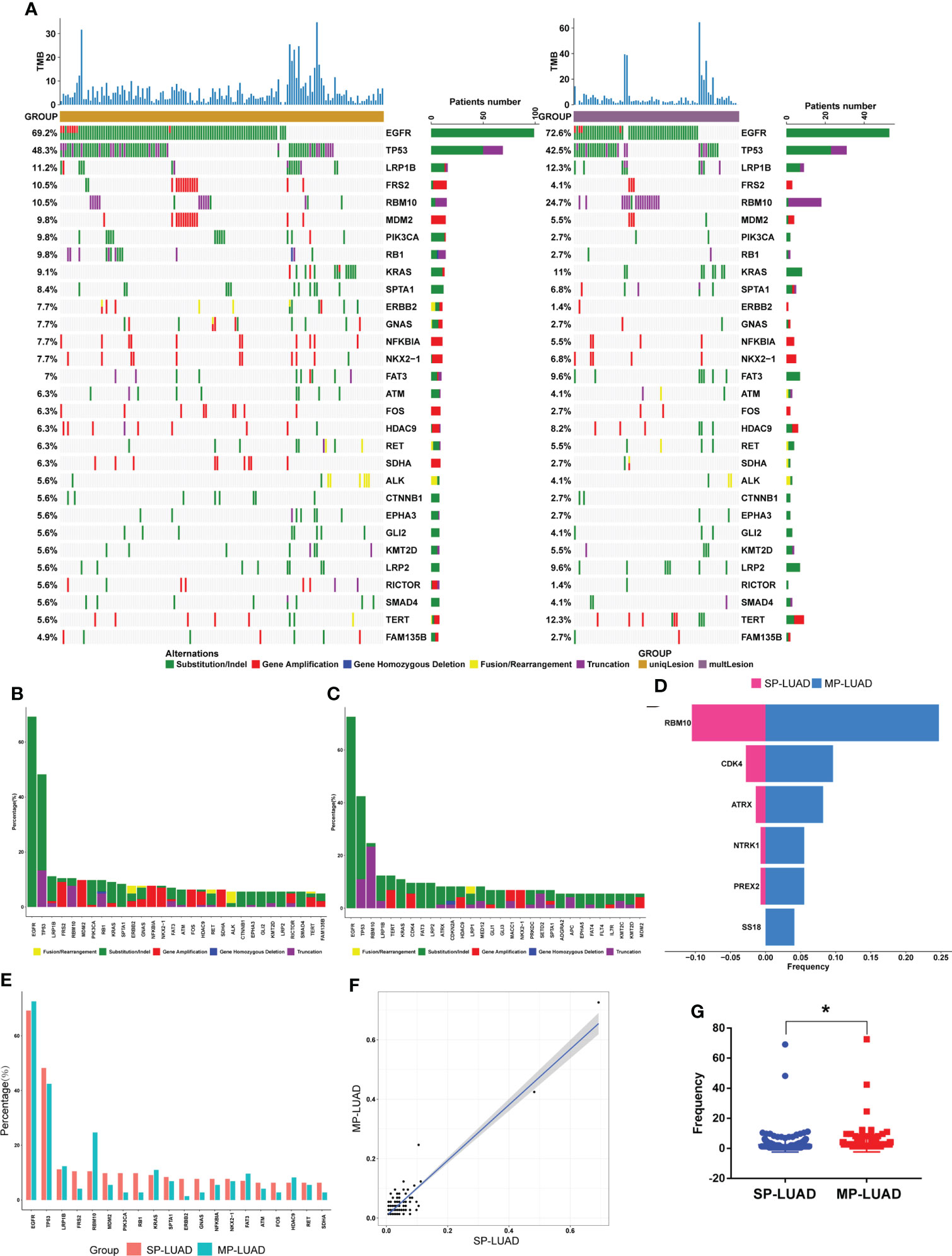
Figure 2 Comparation of SP- and MP-LUAD lesions. (A)The waterfall plot of tumor somatic mutation established based on the SP-LUAD (left) and MP-LUAD (right) cohort. (B, C, E) The difference of mutation frequency of common mutated genes between SP- and MP-LUAD lesions. (D) Six genes statistically different mutated among these two cohorts, including RBM10, CDK4, ATRX, NTRK1, PREX2, SS18. (F, G) Mutation Frequency of SP- and MP-LUAD lesions. * p<0.05.
The detail of each altered gene was shown in Figure 2A. Apparently, the ratio of each mutated gene in MP-LUAD cohort was not consistent with those in SP-LUAD cohort (Figures 2B, C). Then we compared the frequency between these two cohorts, and six genes (RBM10, CDK4, ATRX, NTRK1, PREX2, SS18) were shown to have significantly different mutation frequencies (p=0.0089, 0.0469, 0.0191, 0.0456, 0.0456, 0.0375, respectively) (Figures 2D, E). The frequency of two cohorts was shown in Figures 2F, G. The MP-LUAD cohort had a higher mutation frequency.
The gene variant type was shown in Figure 3A, B. In the MP-LUAD cohort, the Splicing Site variant was the most common while in frame deletion in the SP- LUAD cohort. Figures 3C, D shows the co-occurrence of mutant genes. The co-occurrence signature in MP-LUAD differed from that in SP-LUAD. Only three genetic changes were found to be prevalent (Figure 3E). The co-mutated genes of the SP- and MP-LUAD cohorts in EGFR-, KRAS-, and TP53-mutant patients were almost completely different, as shown in Supplementary Table 1.
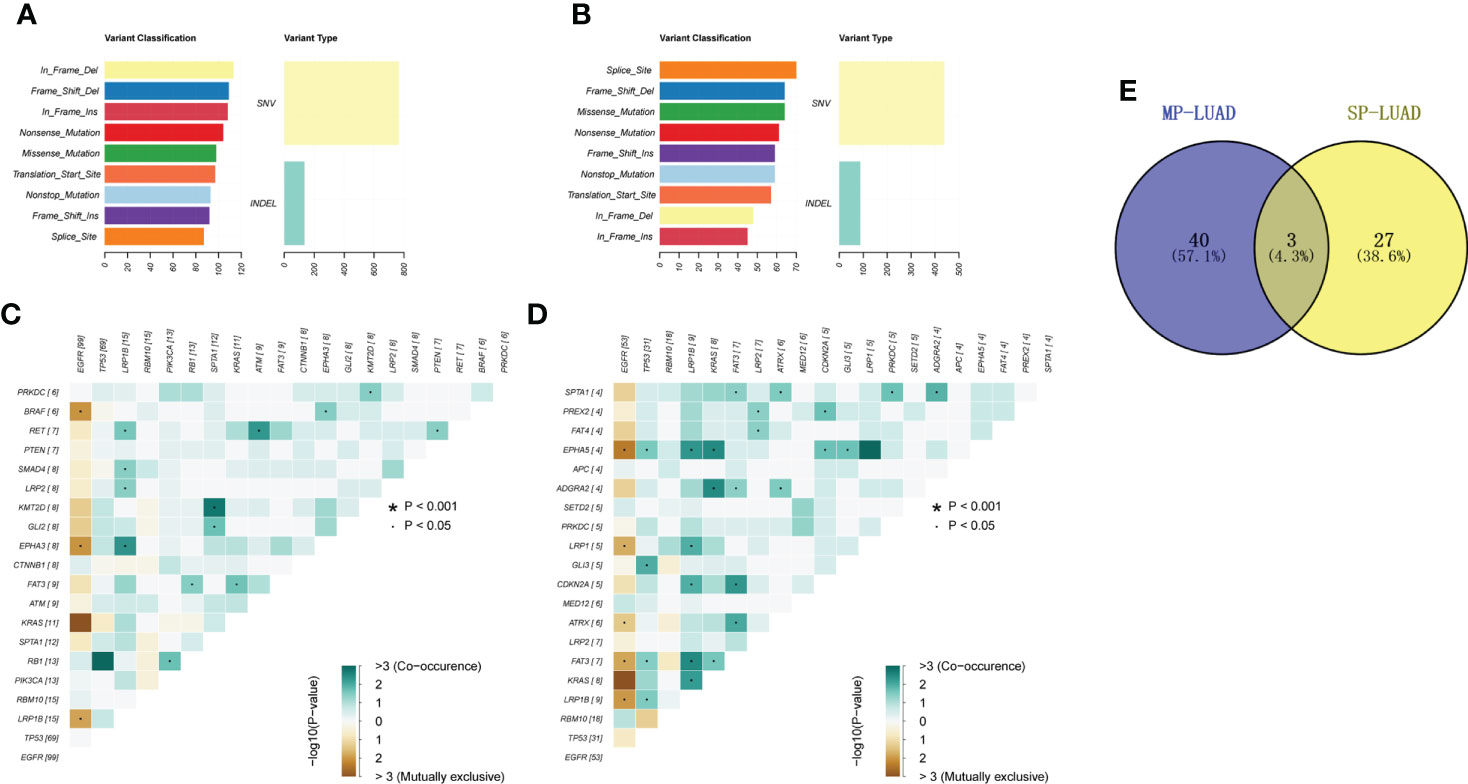
Figure 3 (A, B) Mutation types of SP-(A) and MP-LUAD (B) lesions. (C, D) Co-mutation analysis of SP-(C) and MP-LUAD (D) lesions. (E) The common co-mutated genes among SP- and MP-LUAD lesions. * p<0.05.
The associations between the six different mutated genes and patients’ clinical characteristics
To further investigate the characteristics of these six genes in the public database, we analyzed the data from the cBioPortal database and TCGA database, including the genetic alteration ratio(Figure 4A), gene location on chromosomes(Figure 4B), the CNV alteration frequency(Figure 4C) and the clinical correlations (Figure 4D).The investigation of CNV alteration frequency showed most were focused on the amplification in copy number, among which the NTRK1 and CDK4 had a higher frequency(Figure 4C). The parameters (Histology, Sex, Tumor type, TMB, Mutation Count, Age) were included (Figure 4D and Supplementary Figure 1) and the results suggested that TMB (p<0.001) and mutation count (p<0.001) in six-gene altered group was much higher. The single gene analysis of ATRX (p<0.001, p<0.001), PREX2(p<0.001, p<0.001) and SS18(p<0.001, p<0.001) got the similar results. SS18 was unique among the genes studied since it was the only one in which the mutation status was linked to Tumor Type (p=0.0137). There were differences in sex distribution between the mutated and non-mutated groups, but the differences between the two groups were not statistically significant (p=0.397, 0.628, 0.0866, 0.945, 0.602, 0.865). Apart from that, the mutations of PREX2 (p=0.0156) and SS18 (p=0.0199) were linked to Fraction Genome Alteration and Ragnum Hypoxia Score, respectively (Supplementary Figures 1C, D). However, we found that these genes were unrelated to the patients’ overall survival (Supplementary Figure 2).
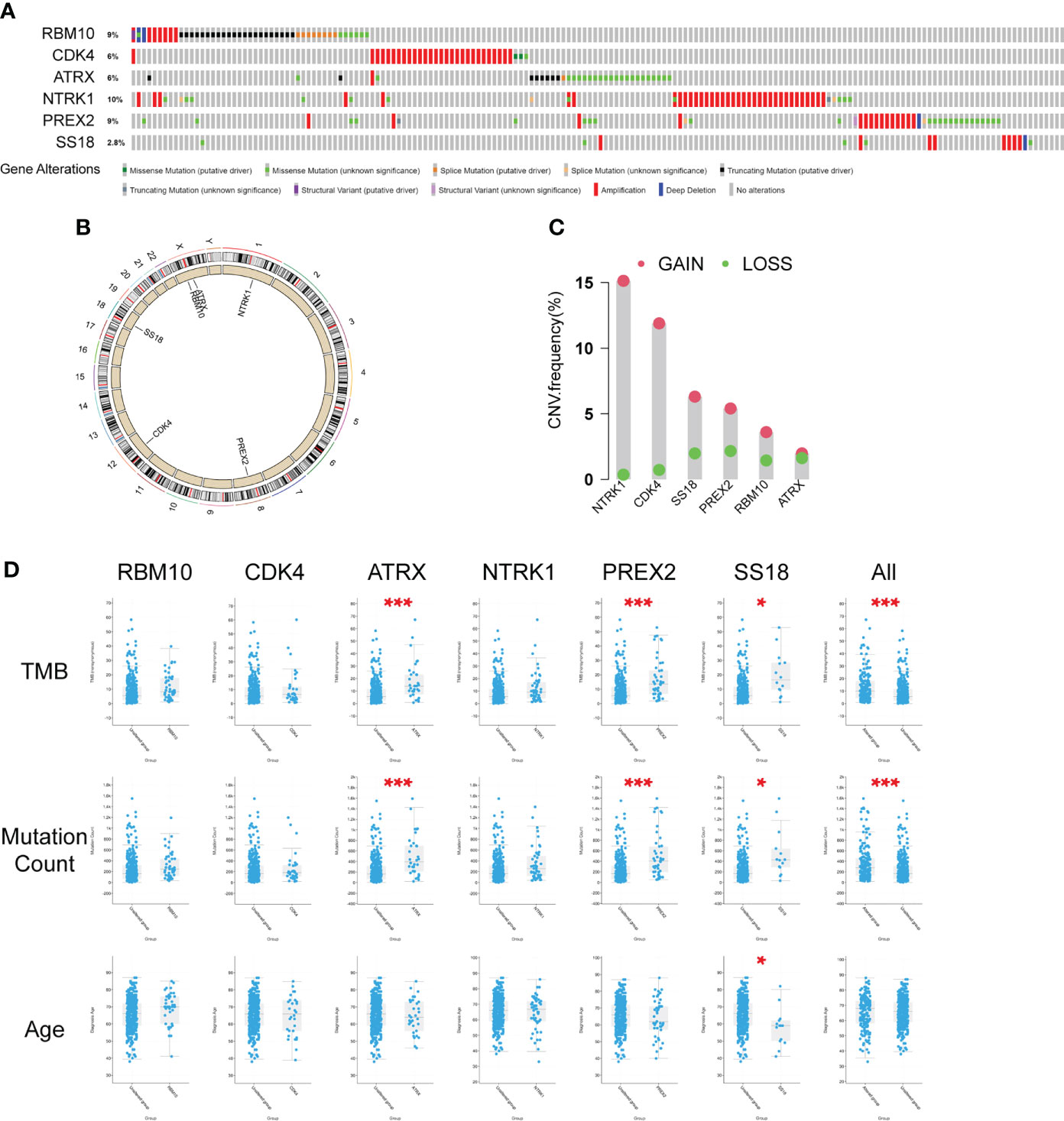
Figure 4 Characteristics of the six differently mutated genes. (A) Gene alterations obtained from cBioPortal database. (B) Gene location on chromosomes. (C) Gene CNV analysis using TCGA data. (D) Clinical correlation analysis of six genes using cBioPortal database. * p<0.05, *** p<0.001.
Gene expression differences associated with six gene mutation.
To investigate the Gene expression differences associated with six gene mutation, data from cBioPortal database was recruited, and the volcano plot of differentially expressed genes was shown in Figure 5A. Besides, based on these differentially expressed genes, GSEA was performed using R tool. The most enriched KEGG pathways and GO-molecular function terms were summarized in Figures 5B–D and the analysis of single gene was shown in Supplementary Figures 3, 4.
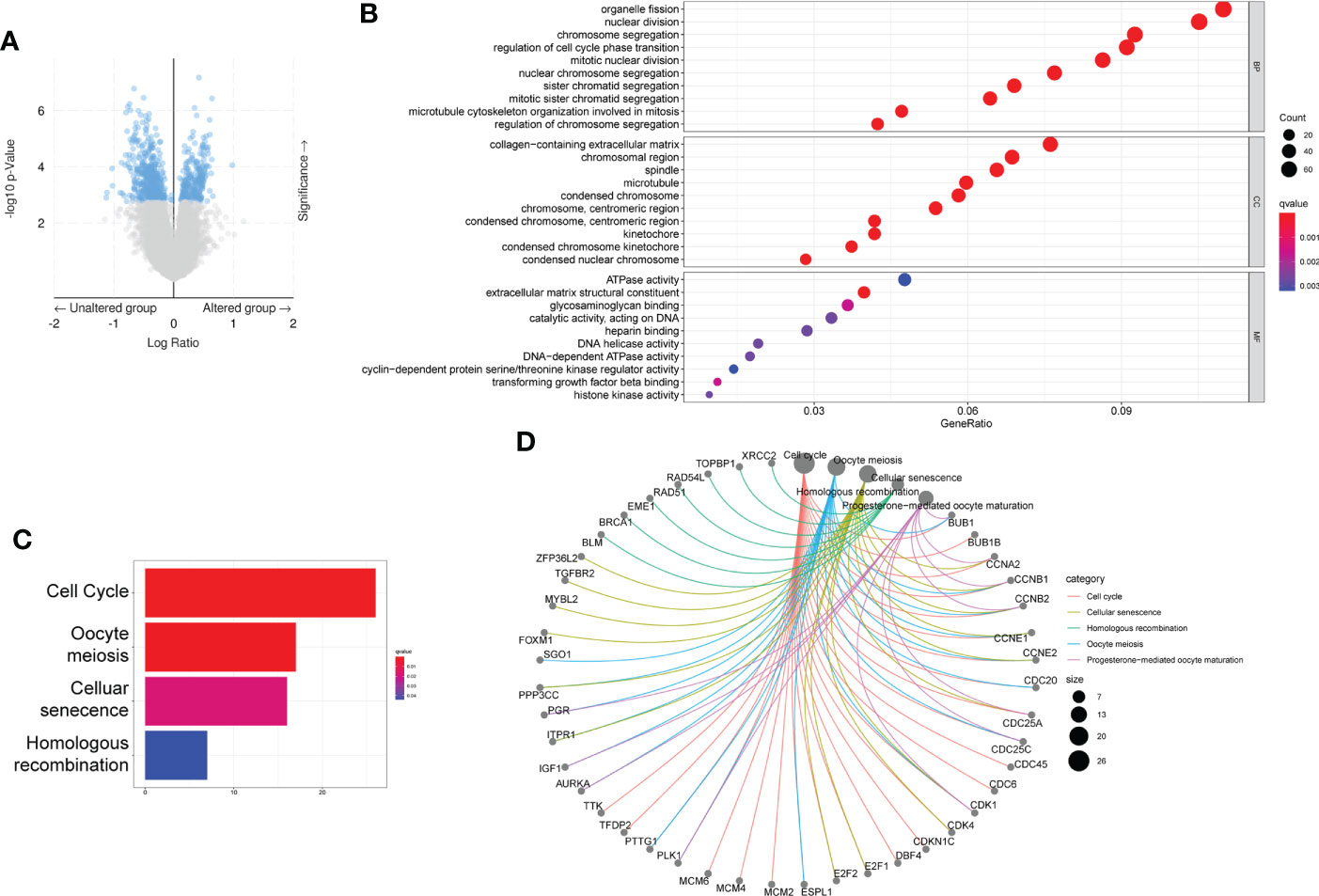
Figure 5 Differential expressed gene based on the mutation status of the six genes. (A) Volcano figure of differently expressed genes from cBioPortal database. (B–D) GO (B) and KEGG (C, D) analysis based on the differently expressed genes.
Heterogeneity of MP-LUAD and phylogenetic reconstruction of MP-LUAD
To investigate the heterogeneity of different lesions of MP-LUAD, we performed heterogeneity analysis. The percentage of mutated genes in each lesion was summarized in Figures 6, 7.
As we could see that distinct lesions from one patient were virtually entirely different. Only one case, who carried three lesions, shared one SNV, EGFR L858R, as shown in Figure 6C, while the others were completely distinct (Figures 6A, B). The phylogenetic trees of all two-lesion-cases listed in Figure 7 indicated that there were still some relationships among two lesions in most cases. The number in the circle denoted the number of SNVs that had been adjusted, while the number near the arrow signified the SNVs’ contributions. Monophyletic connections might be traced back to several lesions. We evaluated the contribution value and amino acid changes associated with these genes and discovered that five TP53 and EGFR mutations were the most significant (Supplementary Table 2).
Discussion
MPLC has become increasingly common as medical technology has advanced, but despite recent advances in large-scale genomics investigations, little is known about its gene signature, which remains a major problem of therapy failure and poor long-term survival.
In the present study, we conducted a mutation analysis and compared the difference between MP-LUAD and SP-LUAD. Obviously, the frequency of each mutated gene in MPL-LUAD cohort was not consistent with these in SP-LUAD cohort The related clinical characteristics was shown in Table 1. It seemed that while there were no significantly difference of age, chest discomfort and family history between these two groups, MP-LUAD patients were related to a higher ratio of weight loss (Table 1). One of the clinical characteristics called Time to the First Discovery (TFD) attracted us, which reflected the length of medical history. Although there was no statistical difference of TFD, probably limited by sample size, MP-LUAD patients showed little symptoms (cough, expectoration, and hemoptysis) which might reduce their willingness to further therapy and showed a longer TFD (Table 1), and this result might suggest that we had to pay more attention to TFD.
The amount of smoking was barely correlated with the diameter in MP-LUAD patients (Figure 1B). The TMB in the MP-LUAD cohort (3.381 ± 0.3586) was greater than the SP-LUAD cohort (2.434 ± 0.2615), according to our findings (Figure 2G).
Importantly, we found there were 6 genes with significantly different mutation frequency among these patients, including RBM10, CDK4, ATRX, NTRK1, PREX2, SS18. It was obviously that the mutation frequency of RBM10 in MP-LUAD cases was the highest and followed by CDK4, ATRX, NTRK1, PREX2, SS18.
RBM10 (RNA-binding motif protein 10), a member of the RNA-binding protein (RBP) family, is located at p11.3 on the X chromosome and also an alternative RNA splicing factor that participates in the regulation of gene expression (30). Recently, RBM10 was reported to function as an oncogene in LUAD by activating EGFR, mitogen-activated protein kinase (MAPK) and phosphoinositide 3-kinase (PI3K)-AKT pathways and inhibition of apoptotic pathways (31), consist with the result in this study shown in Figure 5C and Supplementary Figure 3. Apart from that, Zhao, Jiawei et al. found that RBM10 mutations contributed to lung adenocarcinoma pathogenesis by deregulating splicing (32). Vinayanuwattikun, Chanida et al. revealed that the number of RBM10 mutations was higher in invasive lung adenocarcinoma (33), which suggested that RBM10 contributed to the LUAD progression and might also explain the higher mutation frequency of RBM10 in MP-LUAD.
CDK4 (Cyclin-dependent kinase 4) is a well-recognized cyclin-dependent kinase that specifically regulate cellular transition from the G1 phase to S phase of cell cycle together with CDK6 (34–36). As Figure 4A shows, the most frequent alteration of gene CDK4 is Amplification. CDK4 Amplification was seen in several tumors, such as head and neck mucosal melanoma (37), urinary bladder cancer (38), liposarcomas (39), melanoma (40) and lung cancer (41–43). Dysregulation of the cyclin D–CDK4/6–INK4–Rb pathway results in increased proliferation and due to the importance of CDK4/6 activity in cancer cells, CDK4/6 inhibitors seem as promising treatment (44). However, CDK4 amplification may reduce sensitivity to CDK4/6 inhibition in some cases (45). As Supplementary Figure 3 showed, mutation of gene CDK4 was related to “regulation of response to drug”, and it was reported that in lung cancer, amplification of CDK4 was significant in de novo EGFR TKI resistance (43).
Gene ATRX (Alpha thalassemia/mental retardation syndrome X-linked chromatin remodeler) was first discovered in the X-linked mental retardation syndrome (ATRX syndrome) patients (46). And nowadays, its role in cancer is emerging. Araujo-Castro Marta et al. reported that ATRX mutation was linked to a shorter disease-specific survival (47). ATRX protein is one of the SWI/SNF2 (SWItch/Sucrose Non-Fermentable) family of chromatin remodeling proteins, and maintains genomic stability through its deposition of the replication-independent histone variant H3.3 at telomeres and pericentromeric heterochromatin (48). As shown in Figure 4A, Nonsense Mutation of ATRX was the most frequent, and ATRX loss has been shown to promote ALT, DNA damage and replicative stress (49–51). The GSEA analysis indicated that mutation of gene ATRX was related to “humoral immune response” (Supplementary Figure 3), and now it has been reported in many tumors including pleomorphic Sarcomas (52), glioma (53, 54), gastric cancer patients (55), while the relationship between mutation of ATRX with cytochrome P450 was not reported.
NTRK1 (Neurotrophic receptor tyrosine kinase 1) was originally identified as a fusion oncogene, trkA (tropomyosin receptor kinase) (56). The NTRK1 gene belongs to nerve growth factor receptor genes family, which mainly expressed in neuronal system (57). Several fusion partner genes of NTRK1 were reported in the past few years in thyroid cancer, glioblastoma and lung cancer (58). Many drugs have been developed for the treatment of NTRK1-rearanged cancers. However, it is worth noting that fusions of the NTRK1 genes with CD74 and MPRIP genes were identified in only 3% of some American patients and none of others (59). In this study, we identified two NTRK1 gene alterations including Gene Amplification (1.37% in MP-LUAD) and Substitution (0.7% and 4.11% in SP- and MP-LUAD, respectively) (Figures 2B, C) and it showed a highest gene alteration ratio and CNV frequency in the public database (Figures 4A, C). However, in the clinical correlation analysis, NTRK1 alteration was shown to be statistically unrelated to any clinical factor (Figure 4D). Mutations of gene NTRK1 were also reported in lung cancer (60, 61), while the probable mechanism how these mutations excluding Rearrangement mutation contributed to lung cancer progression was still unknown.
PREX2 (Phosphatidylinositol 3,4,5-trisphosphate-dependent Rac exchanger 2) is considered to be an oncogene for the PREX2 protein’ inhibition of phosphatase and tensin homolog (PTEN) and thus activation of PI3K signaling pathway (62–64). The somatic mutation of PREX2 has been reported in several cancers including hepatocellular cancer (65), breast cancer (66), Melanoma (67, 68) and lung cancer (69). In the study of hepatocellular cancer, most mutant forms of PREX2, had an extended half-life compared with wild-type PREX2, and mutated PREX2 also promoted migration and activated the AKT pathway (65). In lung cancer, PREX2 played an important role in mediating the activation of PI3K/Akt signaling pathway (64), which might provide the evidence for the higher mutation frequency in MP-LUAD cases.
SS18 is the only gene that alternated in MP-LUAD (2 and 1 cases for Rearrangement and Amplification, respectively) rather than SP-LUAD. Fusion of SS18 was frequently detected in synovial sarcoma (70–73). In the GSEA analysis, SS18 was shown to be associated with nuclear division and cell adhesion molecules (Supplementary Figure 3) indicating that SS18 might promote proliferation and metastasis of cancer cells. Knowledge about the actual oncogenic signals effected by SS18-fusion protein in lung cancer is still limited. In the study of synovial sarcoma, the SS18-SSX fusion protein would induce aberrant YAP/TAZ signals (71) and associated with SWI/SNF and Polycomb chromatin complexes to dysregulate gene expression (74–76), which might provide ideas for the aberrant alternation of SS18 in MP-LUAD.
Co-occurrence of genetic abnormalities was found to impact the response of lung cancer to several anticancer therapy (77). There were 40 co-occurring genomic changes in MP-LUAD patients and only three in common, as shown in Figure 3E and Supplementary Table 1. In the MP-LUAD cohort, the Splicing Site variation was the most common. The co-mutated genes in EGFR-, KRAS-, and TP53-mutant LUAD were virtually entirely different, as indicated in Supplementary Table 1. KRAS and LRP1B were all mutational exclusivity in EGFR-mutated LUAD patients, whereas genes like ATRX, EPHA5, and LRP1 were enriched in MP-LUAD patients. LRP1B was shown to be co-mutated in TP53- and KRAS-mutated MP-LUAD patients, which is comparable to a previous finding (78). Besides, LRP1B was reported to be associated with outcomes to immune checkpoint inhibitors, especially co-mutation of FAT3 and LRP1B in LUAD (79, 80).
Finally, we performed an analysis of the multiple lesions from MP-LUAD patients. According the results in Figures 6, 7, through comparing the contribution value and amino acid variations, we discovered that TP53 and EGFR were the most significantly different mutated genes that might contribute to the oncogenesis of MP-LUAD, and that certain SNV loci in TP53 (F143S, H193R) and EGFR (L747 P753 delinsS, S768 D770dup, T790M) might play key roles in lineage decomposition in multifocal samples (Supplementary Table 2).
The present study has several limitations. First, the sample size is too small, which may lead us to neglect some factors; Second, not all the samples were fresh-frozen and we could not guarantee the purity of these samples even though with a strict pathologic evaluation; Third, some MP-LUAD patients who did not undergo surgical treatment were not included, which might lead to selection and information biases.
Conclusion
In conclusion, we conducted a mutation analysis of SP- and MP-LUAD patients and identified genomic alterations and evolutionary trajectories underlying MP-LUAD. These findings will provide new insights into the oncogenesis of MP-LUAD and useful information for development novel approach to target MP-LUAD. Nevertheless, further research is required to elucidate the functions of these genes and their pathways.
Data availability statement
The original contributions presented in the study are included in the article/Supplementary Material. Further inquiries can be directed to the corresponding author.
Ethics statement
The studies involving human participants were reviewed and approved by The ethics committees of Shandong Provincial Hospital(No. 2022-159). The patients/participants provided their written informed consent to participate in this study.
Author contributions
JD contributed to the designing and supervising the study, and correspondence. Co-authors YW and GW analyzed the data and completed the manuscript. HZ, JL, GM, GH, QS helped to collect specimens, search for references and offered guidelines of statistical methods. All authors contributed to the article and approved the submitted version.
Funding
This work is supported by Shandong Provincial Natural Science Foundation (Grant No. ZR2021MH192 and ZR2020QH215).
Acknowledgments
Thanks for everyone who helped to complete this study.
Conflict of interest
Authors GH and QS are employed by Amoy Diagnostics Co., LTD.
The remaining authors declare that the research was conducted in the absence of any commercial or financial relationships that could be construed as a potential conflict of interest.
Publisher’s note
All claims expressed in this article are solely those of the authors and do not necessarily represent those of their affiliated organizations, or those of the publisher, the editors and the reviewers. Any product that may be evaluated in this article, or claim that may be made by its manufacturer, is not guaranteed or endorsed by the publisher.
Supplementary material
The Supplementary Material for this article can be found online at: https://www.frontiersin.org/articles/10.3389/fonc.2022.1014997/full#supplementary-material
Abbreviations
NSCLC, non-small-cell lung cancer; LUAD, adenocarcinoma; LUSC, squamous cell carcinoma; HRCT, high-resolution computed tomography; MPLC, multiple primary lung cancer; SPLC, single primary lung cancer; TCGA, The Cancer Genome Atlas; EGFR, epidermal growth factor receptor; MP-LUAD, multiple primary lung adenocarcinoma; SP-LUAD, single primary lung adenocarcinoma; FFPE, formalin-fixed paraffin-embedded; CAP, College of American Pathologists; CLIA, Clinical Laboratory Improvement Amendments; SNV, single nucleotide variation; INDELS, short and long insertion/deletion; CNV, copy number variation; IGV, Integrative Genomics Viewer; TMB, Tumor mutation burden; GSEA, Gene Set Enrichment Analysis; TFD, time to the first discovery; AIS, adenocarcinoma in situ; MIA, microinvasive adenocarcinoma; RBP, RNA-binding protein; MAPK, mitogen-activated protein kinase; PI3K, phosphoinositide 3-kinase; RBM10, RNA-binding motif protein 10; CDK4, Cyclin-dependent kinase 4; ATRX, Alpha thalassemia/mental retardation syndrome X-linked chromatin remodeler; NTRK1, Neurotrophic receptor tyrosine kinase 1; PREX2, Phosphatidylinositol 3,4,5-trisphosphate-dependent Rac exchanger 2; PTEN, phosphatase and tensin homolog.
References
1. Huang C, Qu X, Du J. Proportion of lung adenocarcinoma in female never-smokers has increased dramatically over the past 28 years. J Thorac Dis (2019) 11(7):2685–8. doi: 10.21037/jtd.2019.07.08
2. Li M, Wan Y, Zhang L, Zhou L-N, Shi Z, Zhang R, et al. Synchronous multiple lung cancers presenting as multifocal pure ground glass nodules: are whole-body positron emission tomography/computed tomography and brain enhanced magnetic resonance imaging necessary? Transl Lung Cancer Res (2019) 8(5):649–57. doi: 10.21037/tlcr.2019.09.10
3. Tie H, Luo J, Shi R, Li Z, Chen D, Wu Q. Characteristics and prognosis of synchronous multiple primary lung cancer after surgical treatment: A systematic review and meta-analysis of current evidence. Cancer Med (2021) 10(2):507–20. doi: 10.1002/cam4.3614
4. Zhou LN, Wu N, Zhao SJ, Yang L, Wang YL, Wang RB, et al. [HRCT features differentiate synchronous multiple primary lung adenocarcinomas from intrapulmonary metastases]. Zhonghua Zhong Liu Za Zhi (2020) 42(6):449–55. doi: 10.3760/cma.j.cn112152-20200227-00126
5. Chen K, Chen W, Cai J, Yang F, Lou F, Wang X, et al. Favorable prognosis and high discrepancy of genetic features in surgical patients with multiple primary lung cancers. J Thorac Cardiovasc Surg (2018) 155(1):371–379. doi: 10.1016/j.jtcvs.2017.08.141
6. Pfannschmidt J, Dienemann H. Surgical treatment of oligometastatic non-small cell lung cancer. Lung Cancer (2010) 69(3):251–8. doi: 10.1016/j.lungcan.2010.05.003
7. Martini N, Melamed MR. Multiple primary lung cancers. J Thorac Cardiovasc Surg (1975) 70(4):606–12. doi: 10.1016/S0022-5223(19)40289-4
8. Wang J, Zhang T, Ding H, Dong G, Xu L, Jiang F. [Research progress in distinguishing methods of simultaneous multiple primary lung cancer and intrapulmonary metastasis]. Zhongguo Fei Ai Za Zhi (2021) 24(5):365–71. doi: 10.3779/j.issn.1009-3419.2021.101.12
9. Zuin A, Andriolo LG, Marulli G, Schiavon M, Nicotra S, Calabrese F, et al. Is lobectomy really more effective than sublobar resection in the surgical treatment of second primary lung cancer? Eur J Cardiothorac Surg (2013) 44(2):e120–5. doi: 10.1093/ejcts/ezt219
10. Xiao F, Liu D, Guo Y, Shi B, Song Z, Tian Y, et al. Survival rate and prognostic factors of surgically resected clinically synchronous multiple primary non-small cell lung cancer and further differentiation from intrapulmonary metastasis. J Thorac Dis (2017) 9(4):990–1001. doi: 10.21037/jtd.2017.03.59
11. Wang Y, Wang L, Zhang D. [Diagnosis and surgical treatment of double primary lung cancer]. Zhonghua Zhong Liu Za Zhi (2001) 23(5):428–30.
12. Nie Y, Wang X, Yang F, Zhou Z, Wang J, Chen K. Surgical prognosis of synchronous multiple primary lung cancer: Systematic review and meta-analysis. Clin Lung Cancer (2021) 22(4):341–350. doi: 10.1016/j.cllc.2020.10.022
13. Mansuet-Lupo A, Barritault M, Alifano M, Janet-Vendroux A, Zarmaev M, Biton J, et al. Proposal for a combined histomolecular algorithm to distinguish multiple primary adenocarcinomas from intrapulmonary metastasis in patients with multiple lung tumors. J Thorac Oncol (2019) 14(5):844–56. doi: 10.1016/j.jtho.2019.01.017
14. Ma J-W, Fu Y-L, Miao L, Li M. Imaging of synchronous multiple primary lung adenocarcinoma with concomitant and mutations: a case report and review of the literature. Transl Cancer Res (2021) 10(5):2509–15. doi: 10.21037/tcr-20-3258
15. Goodwin D, Rathi V, Conron M, Wright GM. Genomic and clinical significance of multiple primary lung cancers as determined by next-generation sequencing. J Thorac Oncol (2021) 16(7):1166–75. doi: 10.1016/j.jtho.2021.03.018
16. Belardinilli F, Pernazza A, Mahdavian Y, Cerbelli B, Bassi M, Gradilone A, et al. A multidisciplinary approach for the differential diagnosis between multiple primary lung adenocarcinomas and intrapulmonary metastases. Pathol Res Pract (2021) 220:153387. doi: 10.1016/j.prp.2021.153387
17. The Cancer Genome Atlas Research Network. Comprehensive molecular profiling of lung adenocarcinoma. Nature (2014) 511(7511):543–50. doi: 10.1038/nature13385
18. The Cancer Genome Atlas Research Network. Comprehensive genomic characterization of squamous cell lung cancers. Nature (2012) 489(7417):519–25. doi: 10.1038/nature11404
19. Hanahan D. Hallmarks of cancer: New dimensions. Cancer Discovery (2022) 12(1):31–46. doi: 10.1158/2159-8290.CD-21-1059
20. Hanahan D, Weinberg RA. Hallmarks of cancer: the next generation. Cell. (2011) 144(5):646–74. doi: 10.1016/j.cell.2011.02.013
21. Kinzler KW, Vogelstein B. Landscaping the cancer terrain. Science. (1998) 280(5366):1036–7. doi: 10.1126/science.280.5366.1036
22. Wood LD, Parsons DW, Jones S, Lin J, Sjöblom T, Leary RJ, et al. The genomic landscapes of human breast and colorectal cancers. Science. (2007) 318(5853):1108–13. doi: 10.1126/science.1145720
23. Chen J, Yang H, Teo ASM, Amer LB, Sherbaf FG, Tan CQ, et al. Genomic landscape of lung adenocarcinoma in East asians. Nat Genet (2020) 52(2):177–86. doi: 10.1038/s41588-019-0569-6
24. Imielinski M, Berger AH, Hammerman PS, Hernandez B, Pugh TJ, Hodis E, et al. Mapping the hallmarks of lung adenocarcinoma with massively parallel sequencing. Cell. (2012) 150(6):1107–20. doi: 10.1016/j.cell.2012.08.029
25. Campbell JD, Alexandrov A, Kim J, Wala J, Berger AH, Pedamallu CS, et al. Distinct patterns of somatic genome alterations in lung adenocarcinomas and squamous cell carcinomas. Nat Genet (2016) 48(6):607–16. doi: 10.1038/ng.3564
26. Kadara H, Choi M, Zhang J, Parra ER, Rodriguez-Canales J, Gaffney SG, et al. Whole-exome sequencing and immune profiling of early-stage lung adenocarcinoma with fully annotated clinical follow-up. Ann Oncol (2017) 28(1):75–82. doi: 10.1093/annonc/mdw436
27. Li F, Zhong W-Z, Niu F-Y, Zhao N, Yang J-J, Yan H-H, et al. Multiple primary malignancies involving lung cancer. BMC Cancer (2015) 15:696. doi: 10.1186/s12885-015-1733-8
28. Popic V, Salari R, Hajirasouliha I, Kashef-Haghighi D, West RB, Batzoglou S. Fast and scalable inference of multi-sample cancer lineages. Genome Biol (2015) 16:91. doi: 10.1186/s13059-015-0647-8
29. Cerami E, Gao J, Dogrusoz U, Gross BE, Sumer SO, Aksoy BA, et al. The cBio cancer genomics portal: an open platform for exploring multidimensional cancer genomics data. Cancer Discovery (2012) 2(5):401–4. doi: 10.1158/2159-8290.CD-12-0095
30. Sun Y, Bao Y, Han W, Song F, Shen X, Zhao J, et al. Autoregulation of RBM10 and cross-regulation of RBM10/RBM5 via alternative splicing-coupled nonsense-mediated decay. Nucleic Acids Res (2017) 45(14):8524–40. doi: 10.1093/nar/gkx508
31. Sun X, Jia M, Sun W, Feng L, Gu C, Wu T. Functional role of RBM10 in lung adenocarcinoma proliferation. Int J Oncol (2019) 54(2):467–78. doi: 10.3892/ijo.2018.4643
32. Zhao J, Sun Y, Huang Y, Song F, Huang Z, Bao Y, et al. Functional analysis reveals that RBM10 mutations contribute to lung adenocarcinoma pathogenesis by deregulating splicing. Sci Rep (2017) 7:40488. doi: 10.1038/srep40488
33. Vinayanuwattikun C, Le Calvez-Kelm F, Abedi-Ardekani B, Zaridze D, Mukeria A, Voegele C, et al. Elucidating genomic characteristics of lung cancer progression from In situ to invasive adenocarcinoma. Sci Rep (2016) 6:31628. doi: 10.1038/srep31628
34. Fassl A, Geng Y, Sicinski P. CDK4 and CDK6 kinases: From basic science to cancer therapy. Science (2022) 375(6577):eabc1495. doi: 10.1126/science.abc1495
35. Fry DW, Harvey PJ, Keller PR, Elliott WL, Meade M, Trachet E, et al. Specific inhibition of cyclin-dependent kinase 4/6 by PD 0332991 and associated antitumor activity in human tumor xenografts. Mol Cancer Ther (2004) 3(11):1427–38. doi: 10.1158/1535-7163.1427.3.11
36. Goel S, DeCristo MJ, McAllister SS, Zhao JJ. CDK4/6 inhibition in cancer: Beyond cell cycle arrest. Trends Cell Biol (2018) 28(11):911–25. doi: 10.1016/j.tcb.2018.07.002
37. Lyu J, Miao Y, Yu F, Chang C, Guo W, Zhu H. CDK4 and TERT amplification in head and neck mucosal melanoma. J Oral Pathol Med (2021) 50(10):971–8. doi: 10.1111/jop.13180
38. Simon R, Struckmann K, Schraml P, Wagner U, Forster T, Moch H, et al. Amplification pattern of 12q13-q15 genes (MDM2, CDK4, GLI) in urinary bladder cancer. Oncogene. (2002) 21(16):2476–83. doi: 10.1038/sj.onc.1205304
39. Italiano A, Bianchini L, Gjernes E, Keslair F, Ranchere-Vince D, Dumollard J-M, et al. Clinical and biological significance of CDK4 amplification in well-differentiated and dedifferentiated liposarcomas. Clin Cancer Res (2009) 15(18):5696–703. doi: 10.1158/1078-0432.CCR-08-3185
40. Muthusamy V, Hobbs C, Nogueira C, Cordon-Cardo C, McKee PH, Chin L, et al. Amplification of CDK4 and MDM2 in malignant melanoma. Genes Chromosomes Cancer (2006) 45(5):447–54. doi: 10.1002/gcc.20310
41. Schrock AB, Frampton GM, Suh J, Chalmers ZR, Rosenzweig M, Erlich RL, et al. Characterization of 298 patients with lung cancer harboring MET exon 14 skipping alterations. J Thorac Oncol (2016) 11(9):1493–502. doi: 10.1016/j.jtho.2016.06.004
42. Yu HA, Suzawa K, Jordan E, Zehir A, Ni A, Kim R, et al. Concurrent alterations in EGFR-mutant lung cancers associated with resistance to EGFR kinase inhibitors and characterization of MTOR as a mediator of resistance. Clin Cancer Res (2018) 24(13):3108–18. doi: 10.1158/1078-0432.CCR-17-2961
43. Sitthideatphaiboon P, Teerapakpinyo C, Korphaisarn K, Leelayuwatanakul N, Pornpatrananrak N, Poungvarin N, et al. Co-Occurrence CDK4/6 amplification serves as biomarkers of de novo EGFR TKI resistance in sensitizing EGFR mutation non-small cell lung cancer. Sci Rep (2022) 12(1):2167. doi: 10.1038/s41598-022-06239-y
44. Hamilton E, Infante JR. Targeting CDK4/6 in patients with cancer. Cancer Treat Rev (2016) 45:129–38. doi: 10.1016/j.ctrv.2016.03.002
45. Olanich ME, Sun W, Hewitt SM, Abdullaev Z, Pack SD, Barr FG. CDK4 amplification reduces sensitivity to CDK4/6 inhibition in fusion-positive rhabdomyosarcoma. Clin Cancer Res (2015) 21(21):4947–59. doi: 10.1158/1078-0432.CCR-14-2955
46. Gibbons RJ, Picketts DJ, Villard L, Higgs DR. Mutations in a putative global transcriptional regulator cause X-linked mental retardation with alpha-thalassemia (ATR-X syndrome). Cell. (1995) 80(6):837–45. doi: 10.1016/0092-8674(95)90287-2
47. Araujo-Castro M, Pascual-Corrales E, Molina-Cerrillo J, Moreno Mata N, Alonso-Gordoa T. Bronchial carcinoids: From molecular background to treatment approach. Cancers (Basel) (2022) 14(3):520. doi: 10.3390/cancers14030520
48. Pekmezci M, Rice T, Molinaro AM, Walsh KM, Decker PA, Hansen H, et al. Adult infiltrating gliomas with WHO 2016 integrated diagnosis: additional prognostic roles of ATRX and TERT. Acta Neuropathol (2017) 133(6):1001–16. doi: 10.1007/s00401-017-1690-1
49. Ramamoorthy M, Smith S. Loss of ATRX suppresses resolution of telomere cohesion to control recombination in ALT cancer cells. Cancer Cell (2015) 28(3):357–69. doi: 10.1016/j.ccell.2015.08.003
50. Cai J, Chen J, Zhang W, Yang P, Zhang C, Li M, et al. Loss of ATRX, associated with DNA methylation pattern of chromosome end, impacted biological behaviors of astrocytic tumors. Oncotarget. (2015) 6(20):18105–15. doi: 10.18632/oncotarget.3906
51. Haase S, Garcia-Fabiani MB, Carney S, Altshuler D, Núñez FJ, Méndez FM, et al. Mutant ATRX: Uncovering a new therapeutic target for glioma. Expert Opin Ther Targets (2018) 22(7):599–613. doi: 10.1080/14728222.2018.1487953
52. Darmusey L, Pérot G, Thébault N, Le Guellec S, Desplat N, Gaston L, et al. ATRX alteration contributes to tumor growth and immune escape in pleomorphic sarcomas. Cancers (Basel) (2021) 13(9):2151. doi: 10.3390/cancers13092151
53. Hu C, Wang K, Damon C, Fu Y, Ma T, Kratz L, et al. ATRX loss promotes immunosuppressive mechanisms in IDH1 mutant glioma. Neuro Oncol (2021) 24(6):888–900. doi: 10.1093/neuonc/noab292
54. Babikir H, Wang L, Shamardani K, Catalan F, Sudhir S, Aghi MK, et al. ATRX regulates glial identity and the tumor microenvironment in IDH-mutant glioma. Genome Biol (2021) 22(1):311. doi: 10.1186/s13059-021-02535-4
55. Ge Y, Wei F, Du G, Fei G, Li W, Li X, et al. The association of sex-biased ATRX mutation in female gastric cancer patients with enhanced immunotherapy-related anticancer immunity. BMC Cancer (2021) 21(1):240. doi: 10.1186/s12885-021-07978-3
56. Martin-Zanca D, Hughes SH, Barbacid M. A human oncogene formed by the fusion of truncated tropomyosin and protein tyrosine kinase sequences. Nature. (1986) 319(6056):743–8. doi: 10.1038/319743a0
57. Snider WD. Functions of the neurotrophins during nervous system development: what the knockouts are teaching us. Cell. (1994) 77(5):627–38. doi: 10.1016/0092-8674(94)90048-5
58. Amatu A, Sartore-Bianchi A, Siena S. Gene fusions as novel targets of cancer therapy across multiple tumour types. ESMO Open (2016) 1(2):e000023. doi: 10.1136/esmoopen-2015-000023
59. Vaishnavi A, Capelletti M, Le AT, Kako S, Butaney M, Ercan D, et al. Oncogenic and drug-sensitive NTRK1 rearrangements in lung cancer. Nat Med (2013) 19(11):1469–72. doi: 10.1038/nm.3352
60. Pu X, Zhu L, Li F, Zheng J, Wu H, Fu Y, et al. Target molecular treatment markers in intrahepatic cholangiocarcinoma based on Chinese population. Pathol Res Pract (2020) 216(9):153116. doi: 10.1016/j.prp.2020.153116
61. Li H, Yan S, Liu Y, Ma L, Liu X, Liu Y, et al. Analysis of NTRK mutation and clinicopathologic factors in lung cancer patients in northeast China. Int J Biol Markers (2020) 35(3):36–40. doi: 10.1177/1724600820949883
62. Rosenfeldt H, Vázquez-Prado J, Gutkind JS. P-REX2, a novel PI-3-kinase sensitive rac exchange factor. FEBS Lett (2004) 572(1-3):167–71. doi: 10.1016/j.febslet.2004.06.097
63. Pandiella A, Montero JC. Molecular pathways: P-Rex in cancer. Clin Cancer Res (2013) 19(17):4564–9. doi: 10.1158/1078-0432.CCR-12-1662
64. Yeung YT, Fan S, Lu B, Yin S, Yang S, Nie W, et al. CELF2 suppresses non-small cell lung carcinoma growth by inhibiting the PREX2-PTEN interaction. Carcinogenesis. (2020) 41(3):377–89. doi: 10.1093/carcin/bgz113
65. Yang M-H, Yen C-H, Chen Y-F, Fang C-C, Li C-H, Lee K-J, et al. Somatic mutations of PREX2 gene in patients with hepatocellular carcinoma. Sci Rep (2019) 9(1):2552. doi: 10.1038/s41598-018-36810-5
66. Srijakotre N, Man J, Ooms LM, Lucato CM, Ellisdon AM, Mitchell CA. P-Rex1 and p-Rex2 RacGEFs and cancer. Biochem Soc Trans (2017) 45(4):963–77. doi: 10.1042/BST20160269
67. Berger MF, Hodis E, Heffernan TP, Deribe YL, Lawrence MS, Protopopov A, et al. Melanoma genome sequencing reveals frequent PREX2 mutations. Nature. (2012) 485(7399):502–6. doi: 10.1038/nature11071
68. Horrigan SK, Courville P, Sampey D, Zhou F, Cai S. Replication study: Melanoma genome sequencing reveals frequent mutations. Elife. (2017) 6:e21634. doi: 10.7554/eLife.21634
69. Wang H, Shan Q, Guo J, Han X, Zhao C, Li H, et al. PDL1 high expression without TP53, KEAP1 and EPHA5 mutations could better predict survival for patients with NSCLC receiving atezolizumab. Lung Cancer (2021) 151:76–83. doi: 10.1016/j.lungcan.2020.11.006
70. Jerby-Arnon L, Neftel C, Shore ME, Weisman HR, Mathewson ND, McBride MJ, et al. Opposing immune and genetic mechanisms shape oncogenic programs in synovial sarcoma. Nat Med (2021) 27(2):289–300. doi: 10.1038/s41591-020-01212-6
71. Isfort I, Cyra M, Elges S, Kailayangiri S, Altvater B, Rossig C, et al. SS18-SSX-Dependent YAP/TAZ signaling in synovial sarcoma. Clin Cancer Res (2019) 25(12):3718–31. doi: 10.1158/1078-0432.CCR-17-3553
72. Stacchiotti S, Van Tine BA. Synovial sarcoma: Current concepts and future perspectives. J Clin Oncol (2018) 36(2):180–7. doi: 10.1200/JCO.2017.75.1941
73. Li J, Mulvihill TS, Li L, Barrott JJ, Nelson ML, Wagner L, et al. A role for SMARCB1 in synovial sarcomagenesis reveals that SS18-SSX induces canonical BAF destruction. Cancer Discovery (2021) 11(10):2620–37. doi: 10.1158/2159-8290.CD-20-1219
74. Kadoch C, Crabtree GR. Reversible disruption of mSWI/SNF (BAF) complexes by the SS18-SSX oncogenic fusion in synovial sarcoma. Cell. (2013) 153(1):71–85. doi: 10.1016/j.cell.2013.02.036
75. Banito A, Li X, Laporte AN, Roe J-S, Sanchez-Vega F, Huang C-H, et al. The SS18-SSX oncoprotein hijacks KDM2B-PRC1.1 to drive synovial sarcoma. Cancer Cell (2018) 34(2):346–8. doi: 10.1016/j.ccell.2018.07.006
76. McBride MJ, Pulice JL, Beird HC, Ingram DR, D'Avino AR, Shern JF, et al. The SS18-SSX fusion oncoprotein hijacks BAF complex targeting and function to drive synovial sarcoma. Cancer Cell (2018) 33(6):1128–1141. doi: 10.1016/j.ccell.2018.05.002
77. Skoulidis F, Heymach JV. Co-Occurring genomic alterations in non-small-cell lung cancer biology and therapy. Nat Rev Cancer (2019) 19(9):495–509. doi: 10.1038/s41568-019-0179-8
78. Hu C, Zhao L, Liu W, Fan S, Liu J, Liu Y, et al. Genomic profiles and their associations with TMB, PD-L1 expression, and immune cell infiltration landscapes in synchronous multiple primary lung cancers. J Immunother Cancer (2021) 9(12)::e003773. doi: 10.1136/jitc-2021-003773
79. Brown LC, Tucker MD, Sedhom R, Schwartz EB, Zhu J, Kao C, et al. LRP1B mutations are associated with favorable outcomes to immune checkpoint inhibitors across multiple cancer types. J Immunother Cancer (2021) 9(3):e001792. doi: 10.1136/jitc-2020-001792
Keywords: lung adenocarcinoma, multiple primary lung cancer, multifocal lung cancer, mutation, next generation sequencing
Citation: Wang Y, Wang G, Zheng H, Liu J, Ma G, Huang G, Song Q and Du J (2022) Distinct gene mutation profiles among multiple and single primary lung adenocarcinoma. Front. Oncol. 12:1014997. doi: 10.3389/fonc.2022.1014997
Received: 09 August 2022; Accepted: 14 November 2022;
Published: 02 December 2022.
Edited by:
Ye Wang, The Second Affiliated Hospital of Medical College of Qingdao University, ChinaReviewed by:
Jun Wang, Fourth Hospital of Hebei Medical University, ChinaMasayuki Chida, Dokkyo Medical University, Japan
Copyright © 2022 Wang, Wang, Zheng, Liu, Ma, Huang, Song and Du. This is an open-access article distributed under the terms of the Creative Commons Attribution License (CC BY). The use, distribution or reproduction in other forums is permitted, provided the original author(s) and the copyright owner(s) are credited and that the original publication in this journal is cited, in accordance with accepted academic practice. No use, distribution or reproduction is permitted which does not comply with these terms.
*Correspondence: Jiajun Du, ZHVqaWFqdW5Ac2R1LmVkdS5jbg==
†These authors have contributed equally to this work