- 1Shanxi Province Cancer Hospital/Shanxi Hospital Affiliated to Cancer Hospital, Chinese Academy of Medical Sciences/Cancer Hospital Affiliated to Shanxi Medical University, Taiyuan, China
- 2Department of Pathology, Shanxi Cancer Hospital, Affiliated Cancer Hospital of Shanxi Medical University, Taiyuan, China
- 3Department of Medicine, Shanghai Yuanqi Biomedical Technology Co., Ltd., Shanghai, China
- 4Department of Technology, Shanghai Yuanqi Biomedical Technology Co., Ltd., Shanghai, China
- 5Department of Biochemistry and Molecular Biology, School of Basic Medical Sciences, Shanxi Medical University, Taiyuan, China
- 6Department of Microbial and Biochemical Pharmacy, School of Pharmaceutical Sciences, Sun Yat-sen University, Guangzhou, China
- 7Department of Hematology, The Second Affiliated Hospital, College of Medicine, Zhejiang University, Hangzhou, China
Background: Characterization of gene mutation profiles can provide new treatment options for patients with diffuse large B-cell lymphoma (DLBCL). However, this method is challenged by the limited source of tissue specimens, especially those of DLBCL patients at advanced stages. Therefore, in the current study, we aimed to describe the gene mutation landscape of DLBCL using circulating tumor DNA (ctDNA) samples obtained from patients’ blood samples, as well as to explore the relationship between ctDNA mutations and the prognosis and treatment response of patients with newly diagnosed DLBCL.
Methods: A total of 169 newly diagnosed Chinese DLBCL patients were included in this study, among which 85 patients were divided into a training set and 84 were assigned into a validation set. The mutation profile of a 59-gene panel was analyzed by targeted next generation sequencing (NGS) of the patients’ ctDNA samples. Differences in clinical factors between patients with and without ctDNA mutations were analyzed. In addition, we also explored gene mutation frequencies between GCB and non-GCB subtypes, and the relationship between gene mutation status, clinical factors, mean VAF (variant allele frequencies) and the patients’ overall survival (OS) and progression-free survival (PFS).
Results: ctDNA mutations were detected in 64 (75.3%) patients of the training set and 67 (79.8%) patients of the validation set. The most commonly mutated genes in both sets were PCLO, PIM1, MYD88, TP53, KMT2D, CD79B, HIST1H1E and LRP1B, with mutation frequencies of >10%. Patients with detectable ctDNA mutations trended to present advanced Ann Arbor stages (III-IV), elevated LDH (lactate dehydrogenase) levels, shorter OS and PFS, and a lower complete response (CR) rate to the R-CHOP regimen compared with DLBCL patients without ctDNA mutations. In addition, mean VAF (≥4.94%) and PCLO mutations were associated with poor OS and PFS.
Conclusion: We investigated the ctDNA mutation landscape in Chinese patients with newly diagnosed DLBCL and found that ctDNA could reflect tumor burden and patients with detectable ctDNA mutations trended to have shorter OS and PFS and a lower CR rate.
Background
Diffuse large B-cell lymphoma (DLBCL) is the most common type of non-Hodgkin’s lymphoma (NHL) worldwide with high clinical and genetic heterogeneity and worse outcomes (1). Gene expression profiling (GEP) divides DLBCL into two main subtypes, namely the germinal center B-cell (GCB) and activated B-cell (ABC) subtypes, with different responses to chemotherapy and targeted agents (2, 3). Recently, Schmitz et al. (4) and Wright et al. (5) classified DLBCL into five and seven genetic subtypes based on gene mutation and translocation profiles. Although these current genotyping techniques are widely accepted, they are challenged by the limited source of tissue specimens, especially for the detection of minimal residual disease (MRD). Thus, it is vital to develop alternative genotyping methods based on patients’ body fluids.
Liquid biopsy is a non-invasive method reflecting intra-tumor heterogeneity with no need for fresh tissues (6) and has potential values in diagnosis, MRD monitoring and treatment choice of lymphomas (7, 8). Circulating tumor DNA (ctDNA) is the DNA fragment derived from tumor cells, which accounts for about 0.1% of cell-free DNA (cfDNA) and emerges as one of the most powerful tools for the early diagnosis of cancers (9). Evidence has demonstrated that the allele frequencies (AFs) of individual mutations detected in tumor samples are highly correlated with those observed in paired plasma cfDNA samples (8, 10). Thus, an analysis of ctDNA in cancer patients can reveal both genetic alterations, including single nucleotide variants (SNVs), insertions/deletions (Indels), chromosomal rearrangements, and copy number variations (CNVs), which can be used for genotyping, and ctDNA content, which can reflect tumor burden (11). Kurtz et al. (11) explored the prognostic value of ctDNA level before and during immunochemotherapy for patients with DLBCL from North America and Europe; they found that pretreatment ctDNA level was an independent prognostic factor in DLBCL. Liu et al. (10) explored the mutation profiles in Chinese patients with newly diagnosed and relapsed/refractory (R/R) DLBCL and observed highly consistent ctDNA and tissue mutation profiles in these patients (sensitivity: 87.50%).
Considering that different races can have varied gene mutation profiles and that the clinical value of ctDNA in Chinese patients remains largely unknown, in this study, we explored the clinical significance of ctDNA in 169 newly diagnosed Chinese DLBCL patients. These patients were first divided into a training set and a validation set. Then we assessed the relationship between ctDNA mutations and clinicopathological features, as well as the roles of ctDNA mutations, including the detected mutation site/gene number, the mean VAF (variant allele frequency) and the mutation status of genes, in the overall survival (OS) and progression-free survival (PFS) in these patients.
Patients and methods
Patients
A total of 169 newly diagnosed Chinese DLBCL patients were enrolled at Shanxi Cancer Hospital from June 2018 to December 2019. Patients were considered eligible for inclusion if they aged ≥ 18 years and had histologically confirmed DLBCL according to the 2016 World Health Organization (WHO) Classification of Tumors of Haematopoietic and Lymphoid Tissues (12). The patients were classified into GCB and non-GCB subgroups according to the Hans algorithm (13). The disease was staged based on the 2014 Lugano Classification and the international prognositic index (IPI) was applied for risk stratification. Bone marrow involvement was assessed by flow cytometry, combined with immunoglobulin (Ig) gene rearrangement and positron emission tomography-computed tomography (PET-CT). A tumor lesion was judged as a bulky disease if the product of length and width of the tumor was ≥ 7.5 cm. All the patients were treated with the R-CHOP regimen (rituximab, cyclophosphamide, doxorubicin, vindesine, prednisone) and followed up until March, 2022. The treatment response, including CR (complete response), PR (partial response), SD (stable disease) and PD (progression disease), was assessed by CT/magnetic resonance imaging (MRI) and PET/CT according to the 2022 Guidelines of Chinese Society of Clinical Oncology (CSCO) after two to four cycles of the R-CHOP regimen. The data described in this manuscript were approved by the Ethics Committee of Shanxi Cancer Hospital (Ethical approval No.2021013) and conducted in accordance with the Helsinki declaration.
All study activities were approved by the Ethics Committee of Shanxi Cancer Hospital (Ethical approval No.2021013), and informed consent was obtained in accordance with the Declaration of Helsinki.
DNA extraction and targeted sequencing
Ten milimeter of peripheral blood samples were collected using EDTA-containing tubes within 1 week of receiving anticancer treatment and centrifuged at 820 g for 10 min to obtain plasma samples, which were centrifuged at 20,000 g for 10 min. Next, cfDNA was extracted using the QIAamp Circulating Nucleic Acid Kit (QIAGEN, Gemany) according to the manufacturer’s instructions. Subsequently, the mutation profile of a 59-gene panel based on literatures (8, 14) was analyzed by targeted next generation sequencing (NGS) of the cfDNA samples (Shanghai Rightongene Bio-tech Co. Ltd, Shanghai, China; Supplementary Table 1) with Illumina NovaSeq 5000 (2×150-bp paired-end sequencing). In this study, VAF was defined as the ratio of the number of mutated alleles to the total number of alleles detected by NGS at a specific genome locus. Mutations with a VAF value ranging from 45% to 55% and ≥ 95% were identified and considered as heterozygous and homozygous germline mutations, respectively. Mean VAF was calculated as follows: Mean VAF = The sum of VAF values of all mutations/the total number of mutations.
Statistical analysis
The maftools (“clinical Enrichment”) package of R was used to analyze the differences in clinical factors and gene mutation frequencies between the GCB and non-GCB subgroups using Chi-square test or Fisher’s exact test. The tableone package of R was applied to analyze the differences in mean VAF between the two groups. Survival probabilities were estimated using the Kaplan-Meier method. We considered two survival endpoints: PFS, the time intervals from diagnosis to progression, relapse, or death from any cause; and OS, the time intervals from diagnosis to death resulting from any cause. Factors with a P value <0.1 were included in the multivariate Cox regression models. P values < 0.05 were considered as statistically significant.
Results
Relationship between clinicopathological features and ctDNA mutation status in patients with newly diagnosed DLBCL
A total of 169 newly diagnosed DLBCL cases with valid targeted NGS data were included in this study, with 85 patients in the training set and 84 in the validation set. Detailed clinical information of the 169 patients is provided in Supplementary Table 2. Sixty-four (75.3%) patients of the training set carried ctDNA mutations. These patients were significantly enriched in Ann Arbor stages III-IV (69.8% vs. 38.1% in Ann Arbor stages I-II, P=0.002) and tended to have elevated LDH (lactic dehydrogenase) levels (53.1% vs. 14.3%, P=0.004) as compared with the patients without detectable ctDNA mutations (Table 1). Similar results were observed in the validation set, in which 67 (79.8%) patients having detectable ctDNA mutations were significantly enriched in advanced Ann Arbor stages (76.1% vs. 47.1%, P=0.041) and exhibited elevated LDH levels (56.7% vs. 18.8%, P=0.014) as compared with those without detectable ctDNA mutations (Table 2). In addition, the presence of ctDNA mutations was also associated with a higher incidence of bulky disease (41.8% vs. 0.0%, P=0.003) only in the validation group (Table 2). These results indicated that ctDNA mutation status was closely associated with the staging and LDH level of newly diagnosed DLBCL patients.
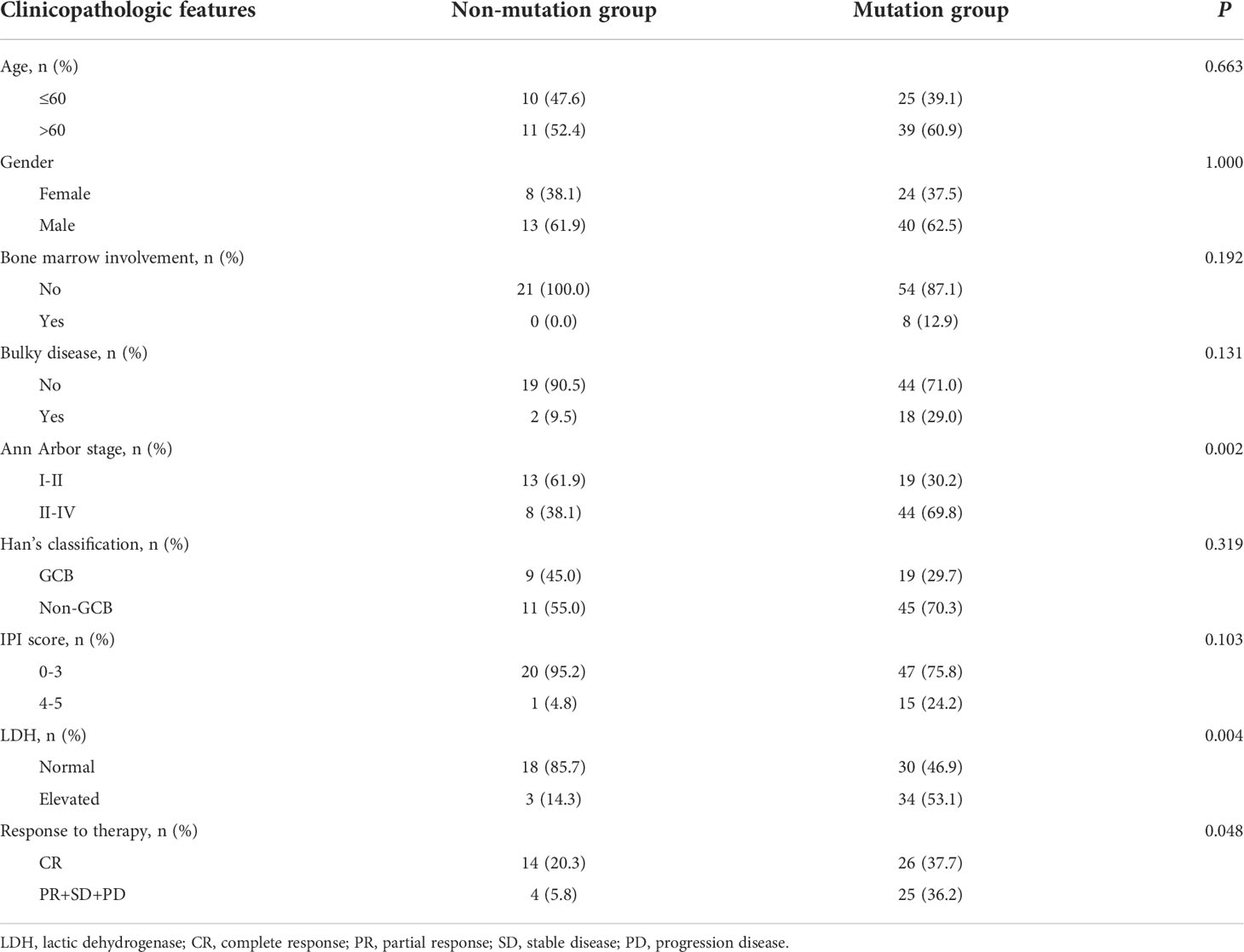
Table 1 The clinicopathologic features of DLBCL patients with mutation or without in training group.
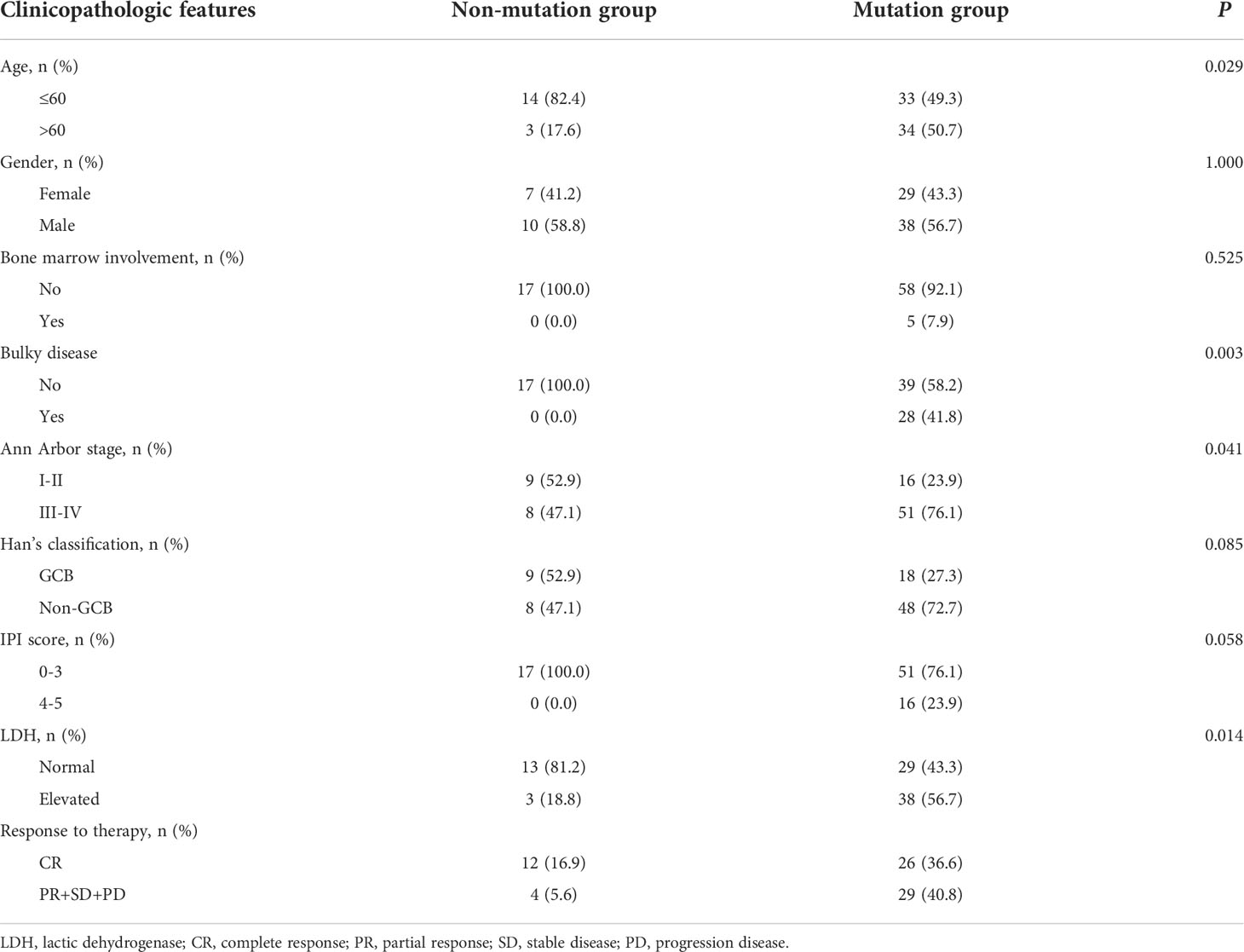
Table 2 The clinicopathologic features of DLBCL patients with mutation or without in validation group.
ctDNA mutation profiles of patients with newly diagnosed DLBCL
Next, we explored the ctDNA mutation profiles of DLBCL patients of the training and validation sets. Detailed information on mutation sites is provided in Supplementary Table 3 and Supplementary Table 4. On average, we detected 6.1 ± 7.1 genetic mutations in patients with confirmed ctDNA mutation. Mutations in PCLO (26%), PIM1 (25%), MYD88 (21%), TP53 (20%), KMT2D (16%), CD79B (12%), HIST1H1E (12%) and LRP1B (11%) genes were the most frequently detected variations, with mutations in each of the genes being found in no less than 9 patients (10%) of the training set (Figure 1A). Consistently, PCLO (26%), PIM1 (24%), MYD88 (20%), TP53 (24%), KMT2D (17%), CD79B (17%), HIST1H1E (17%) and LRP1B (20%) genes also showed high mutation frequencies in the validation set (Figure 1B). In addition, we assessed the difference in ctDNA mutation profile between the GCB and non-GCB subtypes in the 169 DLBCL patients. The results demonstrated that the mutation frequencies of PIM1 (30.4% vs. 12.7%) and CD79B (18.8% vs. 5.5%) were significantly higher in patients of the non-GCB subtype than in those of the GCB subtype (Figure 1C). These results depicted the ctDNA mutation landscape of patients with newly diagnosed DLBCL.
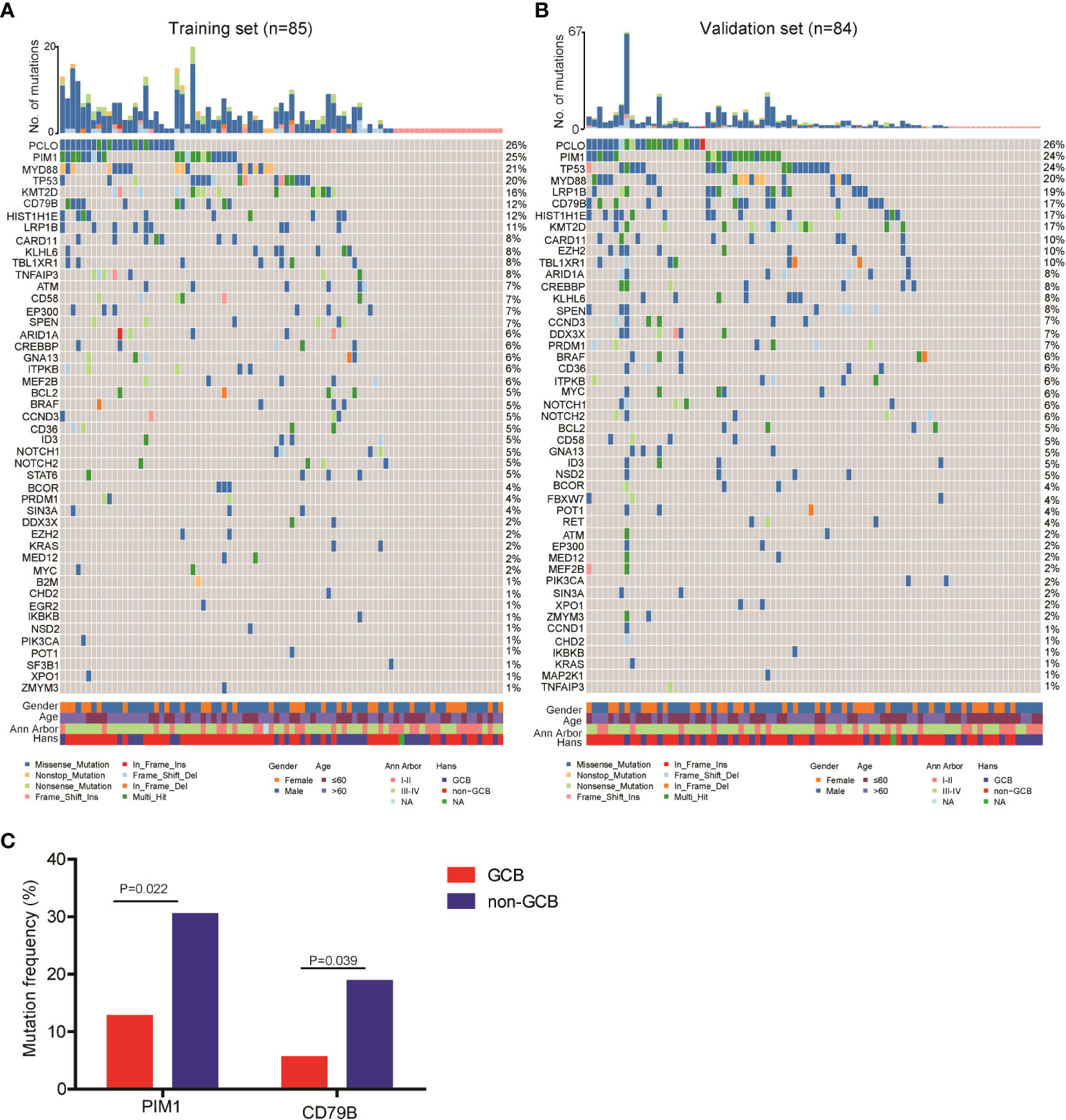
Figure 1 Mutation landscape of ctDNA samples from newly diagnosed DLBCL patients. ctDNA mutation profiles of newly diagnosed DLBCL patients in the (A) training and (B) validation sets. (C) The mutation frequencies of PIM1 and CD79B were significantly increased in the ctDNA samples of non-GCB DLBCL patients as compared with GCB DLBCL patients.
ctDNA mutation status was associated with the response to R-CHOP and clinical manifestation in newly diagnosed DLBCL patients
Next, we assessed the relationship between ctDNA mutation status and the response to R-CHOP regimen in newly diagnosed DLBCL patients. The CR rate in DLBCL patients without ctDNA mutations was obviously higher than that in those carrying ctDNA mutations in both the training (P=0.048) and validation sets (P=0.050) (Tables 1, 2). However, there were no valid differences in the rates of PR, SD and PD between DLBCL patients with different mutation numbers, which is mean VAF values and mutation profiles because the training and validation sets exhibited inconsistent findings. In addition, we compared the mean VAF value in patients with different ages (≤60 vs. >60 years), genders (male vs. female), bone marrow involvement statuses (positive vs. negative), Hans classifications (GCB vs. non-GCB), bulky disease statuses (positive vs. negative), IPIs (1-3 vs. 4-5), Ann Arbor stages (I-II vs. III-IV) and LDH levels (high vs. low). The results showed that the mean VAF value was significantly increased in patients with bone marrow involvement, higher IPI scores (4, 5) and elevated LDH levels in both of the training (Figures 2A–C) and validation sets (Figures 2D–F). Collectively, these results demonstrated that ctDNA mutations were associated with a lower CR rate and aggressive clinical manifestation in patients with newly diagnosed DLBCL.
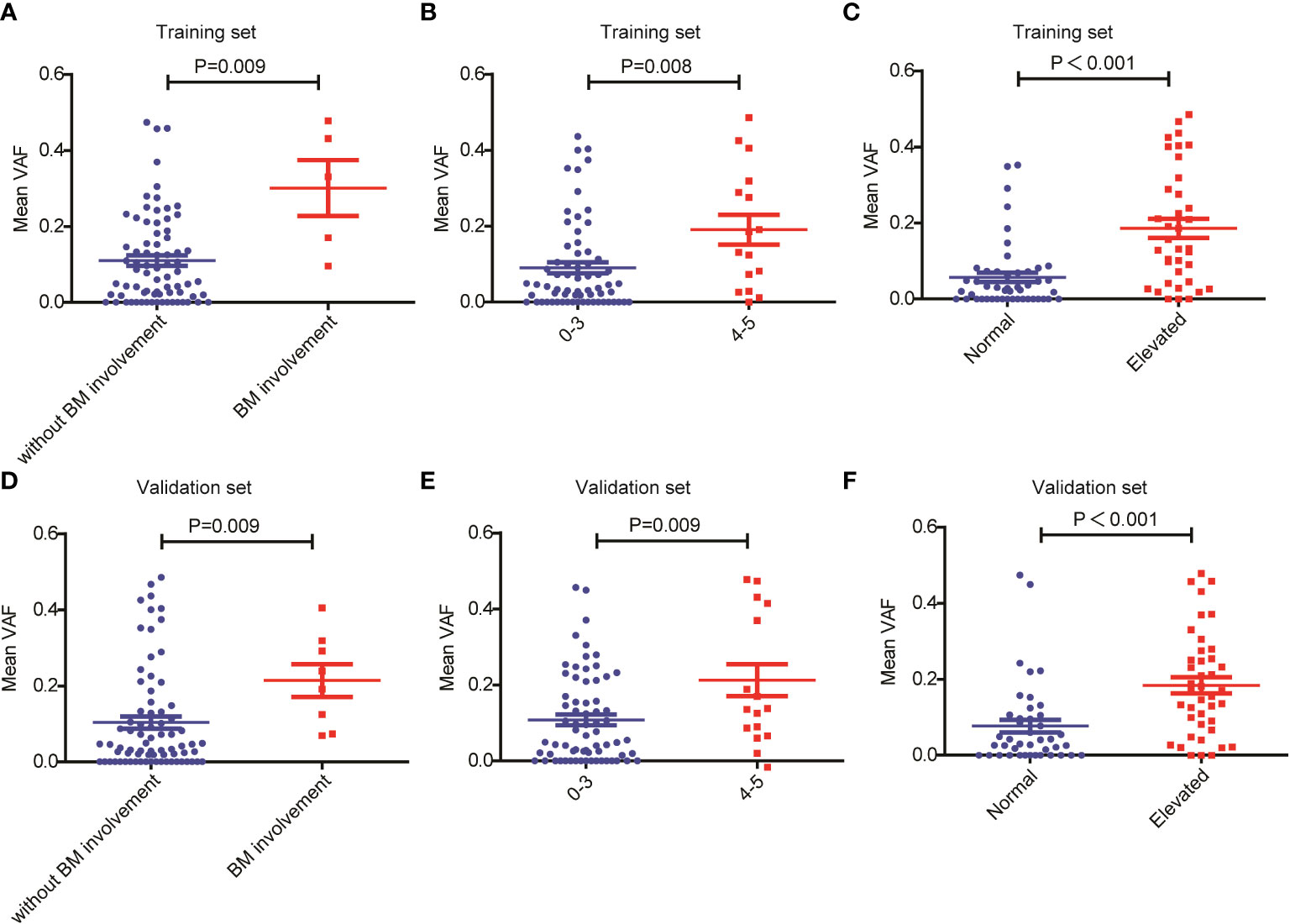
Figure 2 Relationship between mean VAF and clinical features in patients with newly diagnosed DLBCL. Mean VAF was increased in patients with (A, D) bone marrow involvement, (B, E) higher IPI scores and (C, F) elevated LDH levels in the training and validation sets.
DLBCL patients carrying ctDNA mutations demonstrated poor prognosis
We also compared survival outcomes between patients with and without ctDNA mutations. In the training set, the 64 patients with ctDNA mutations exhibited significantly shorter OS than the 19 patients without ctDNA mutations (P=0.03) (Figure 3A). PFS was also shorter in patients with ctDNA mutations, albeit the difference was not statistically significant (P=0.095) (Figure 3B). Next, we validated these results in the validation set, in which 67 patients had detectable ctDNA mutations and 17 patients did not. Compared with patients without ctDNA mutations, both OS (P=0.011) (Figure 3C) and PFS (P=0.0032) (Figure 3D) were significantly shorter in patients with ctDNA mutations. These results indicated that ctDNA mutations were associated with poor prognosis in patients with newly diagnosed DLBCL.
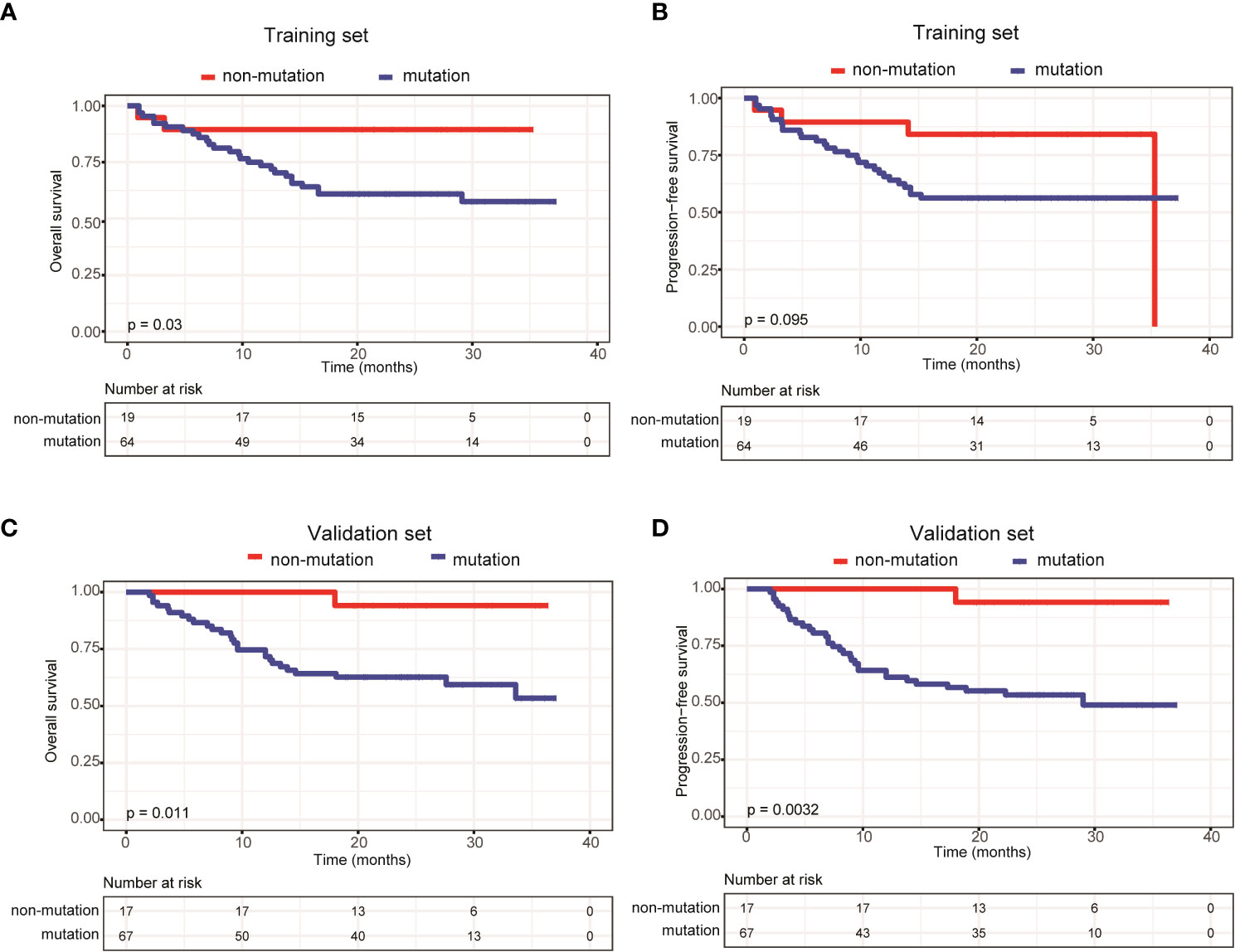
Figure 3 Patients carrying ctDNA mutations trended to have poor prognosis. Kaplan-Meier curves were applied to compare the (A) OS and (B) PFS between patients with and without ctDNA mutations in the training set. Kaplan-Meier curves were applied to compare the (C) OS and (D) PFS between patients with and without ctDNA mutations in the validation set.
Mean VAF and PCLO mutations were associated with poor prognosis in patients with newly diagnosed DLBCL
To further explore the relationship between ctDNA mutation status and the prognosis of patients with newly diagnosed DLBCL, we assessed the effects of mutation number, mutated gene number and mean VAF on OS and PFS using Kaplan-Meier curves generated based the parameters’ average/median values. The results demonstrated that only mean VAF (the median value of which was 4.94%) was closely associated with patients’ prognosis in the training set. Specifically, the OS (P=0.024) and PFS (P=0.043) of patients with a mean VAF ≥ 4.94% were significantly shorter than those of patients with a mean VAF < 4.94% in the training set (Figures 4A, B). We next verified these findings in the validation set. Compared with those in patients with a mean VAF < 4.94%, the OS (P=0.093) and PFS (P=0.014) were shorter in patients with a mean VAF ≥ 4.94%, albeit the difference in OS was not statistically significant (Figures 4C, D).
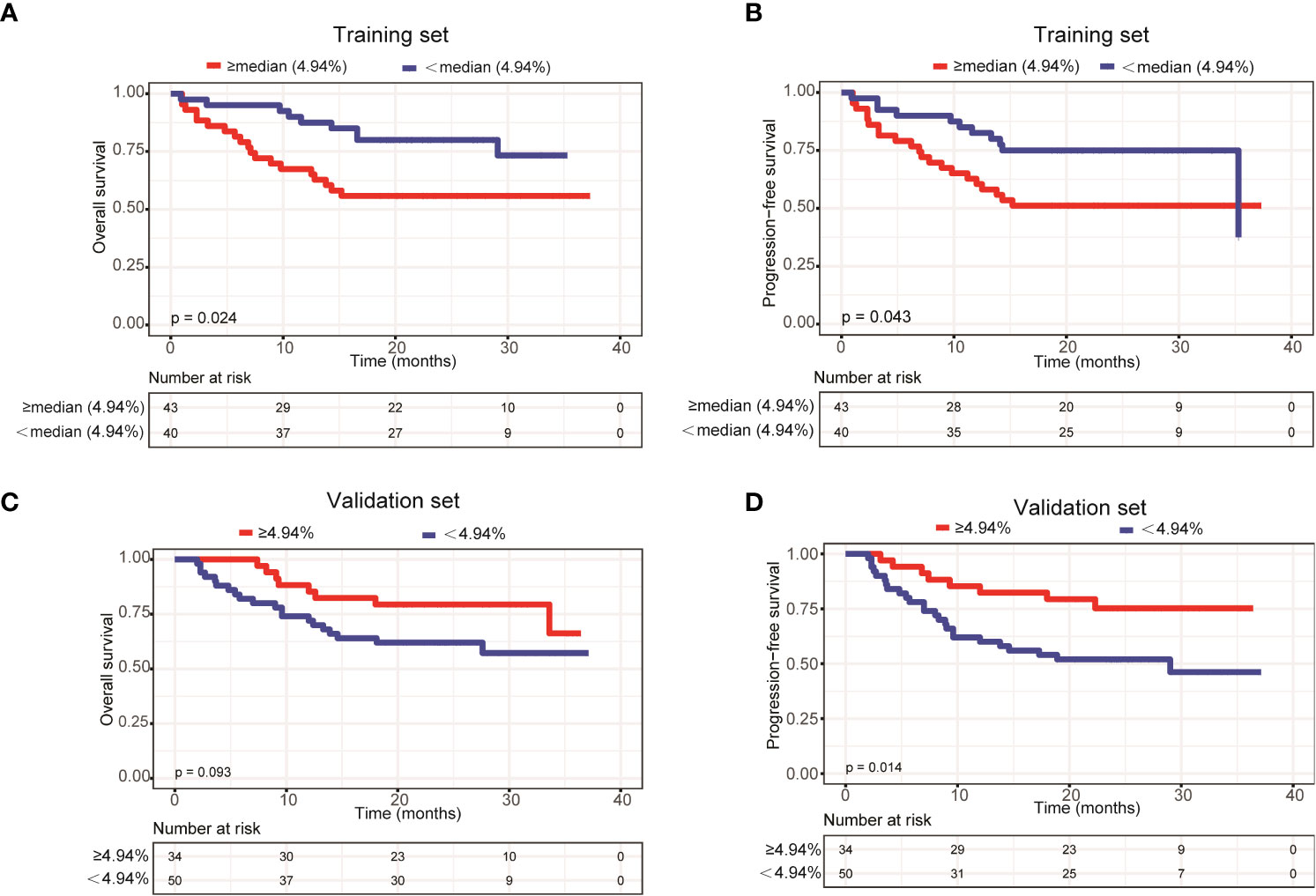
Figure 4 Patients with a mean VAF value ≥ 4.94% trended to have poor prognosis. Kaplan-Meier curves were applied to compare the (A) OS and (B) PFS between patients with mean VAF values ≥ 4.94% and < 4.94% in the training set. Kaplan-Meier curves were applied to compare the (C) OS and (D) PFS between patients with mean VAF values ≥ 4.94% and < 4.94% in the validation set.
In addition, we assessed the effects of gene mutation status on the OS and PFS of patients with newly diagnosed DLBCL. Due to the relatively small sample size, we only assessed genes with a mutation frequency ≥ 10%. In the training set, LRP1B (Supplementary Figures 1A, B) and PCLO mutations (Figures 5A, B) were significantly associated with shorter OS and PFS; whereas in the validation set, only PCLO mutations were significantly associated with shorter OS and PFS (Figures 5C, D). These results demonstrated that a high mean VAF value and PCLO mutations predicted poor prognosis in patients with newly diagnosed DLBCL.
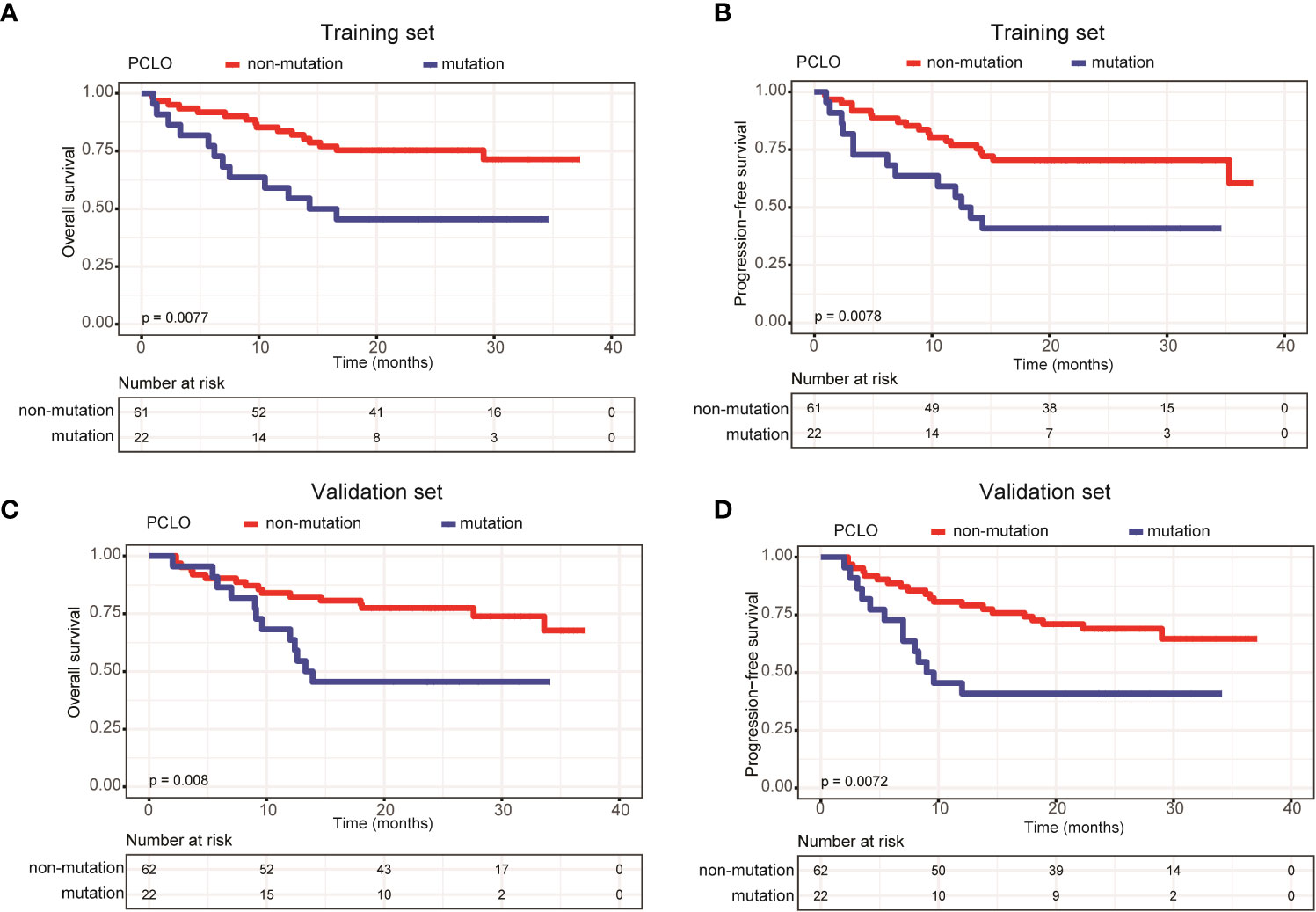
Figure 5 Patients carrying PCLO mutations trended to have poor prognosis. Kaplan-Meier curves were applied to compare the (A) OS and (B) PFS between patients with and without PCLO mutations in the training set. Kaplan-Meier curves were applied to compare the (C) OS and (D) PFS between patients with and without PCLO mutations in the validation set.
Multivariate analysis of prognostic factors in patients with newly diagnosed DLBCL
Finally, multivariate Cox analysis was performed to further explore prognostic factors in patients with newly diagnosed DLBCL. The univariate Cox analysis showed that age > 60 years was an influencing factor on both OS (P=0.038) and PFS (P=0.083) in the training set; meanwhile, bulky disease status (P=0.099) was an influencing factor on PFS in the training set (Table 3). Afterwards, factors with a P value < 0.1, namely the clinical factors (age and/or bulky disease status), mean VAF, and PCLO mutation status, were included in the multivariate analysis. The results showed that age (> 60 years) and mean VAF (≥ 4.94%) were independent influencing factors on both OS and PFS in the training set (Table 4). In the validation set, age (> 60 years) and PCLO mutation status were influencing factors on OS, while age (> 60 years) and bulky disease status were influencing factors on PFS (Table 4) . These results further verified the close relationship between ctDNA mutation and the prognosis of patients with newly diagnosed DLBCL.
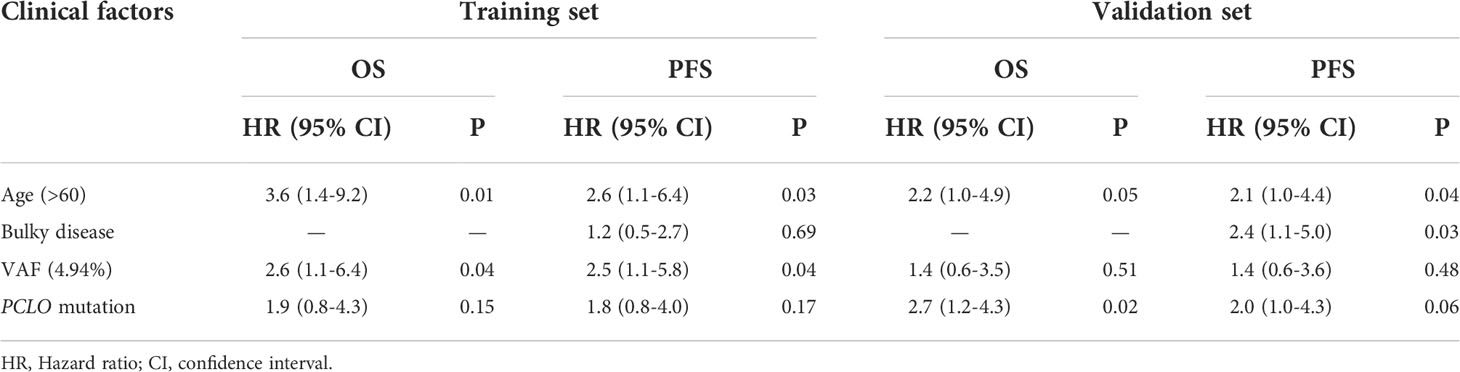
Table 4 Multivariate Cox analysis of the influencing factors of PFS and OS in the training set and validation set.
Discussion
Genetic heterogeneity is a major cause of increased risk and treatment failure in DLBCL. Several studies (8, 10, 14–16) have proved that the mutations detected in blood samples were similar to those identified in tumor tissue, with a concordance rate over 80%.In the present study, we performed targeted sequencing of 59 lymphoma-related genes, the same panel as Liu et al. (10) to analyze the clinical value of ctDNA mutation in 169 Chinese patients with newly diagnosed DLBCL. To increase the reliability of our findings, the 169 patients were randomly divided into a training set (n=85) and a validation set (n=84). Our results demonstrated that detectable ctDNA mutations, a mean VAF value ≥ 4.94%, and PCLO mutations were strongly associated with shorter OS and PFS in the newly diagnosed DLBCL patients.
We found that PCLO (piccolo presynaptic cytomatrix protein), PIM1, CD79B and MYD88 (genes involved in the NF-κB signaling pathway), LRP1B and TP53 (tumor suppressive genes), as well as KMT2D and HIST1H1E (histone modifying genes) were the most commonly mutated genes in the 169 newly diagnosed DLBCL patients. According to the genetic landscape of DLBCL in western countries, the most frequently mutated genes are sequentially KMT2D, MYD88, CREBBP, TP53 and PIM1 (17, 18). In contract, the most frequently mutated genes in Chinese DLBCL patients are sequentially PIM1, BTG2, TP53, HIST1H1E and KMT2D (19). The higher proportion of non-GCB DLBCL cases in Chinese patients may be a reason for this difference. According to literature, genes related to histone methylation or acetylation (EZH2, EP300, CREBBP and KMT2D) and the PI3K/AKT and JAK/STAT pathways are commonly mutated in the GCB subtype of DLBCL patients, while genes related to the B-cell receptor and NF-κB signaling pathways, such as MYD88, CD79A/B, CARD11, PIM1 and TNFAIP3, are commonly mutated in the ABC subtype (20). Consistently, we found that the mutation frequencies of PIM1 and CD79B were significantly higher in DLBCL patients with the non-GCB subtype than in those with the GCB subtype.
In addition, we were able to detect ctDNA mutations in 64 (75.3%) out of 85 patients in the training set and 67 (79.8%) out of 84 patients in the validation set. Rivas-Delgado et al. (21) were able to detect at least one ctDNA mutation in 69 of 79 patients (87%) with DLBCL. This slight difference in ctDNA mutation detection rate may be caused by different panels of genes sequenced: Rivas-Delgado et al. (21) performed targeted sequencing on 112 genes, while we analyzed 59 genes. In addition, we found that patients with detectable ctDNA mutations had shorter OS and PFS in both the training and validation sets. Furthermore, patients carrying ctDNA mutations were significantly enriched in more advanced Ann Arbor stages (stages III-IV) and generally exhibited elevated LDH levels. These findings establish a link between ctDNA mutation status and the prognosis of patients with DLBCL. Recently, Kurtz et al. (22) indicated that 25% of ctDNA-negative patients demonstrated by cancer personalized profiling by deep sequencing (CAPP-Seq) were found to be ctDNA-positive, as revealed by phased variant enrichment and detection sequencing (PhasED-Seq), after two cycles of therapy and presented with poor outcomes.
ctDNA VAF has been closely associated with the clinical features and prognosis of various cancers, and is considered as a new biomarker for tumor burden (23, 24). For example, Fu et al. (25) found that the VAF values of TP53 p.Y88C and LATS2 p.F972L were decreased in B-cell lymphoma patients with CR. Desch et al. (26) reported that ctDNA VAF values were strongly associated with total metabolic tumor volume (TMTV) and the incidence of bulky disease in pediatric Hodgkin’s lymphoma. In addition. the median VAF of non-DNMT3A clones increased from 1% at the time of autologous stem cell transplantation (ASCT) to 37% at the diagnosis of therapy-related myeloid neoplasms (tMNs) (27). In the present study, we found that the mean VAF values were significantly increased in patients with bone marrow involvement, higher IPI scores and elevated LDH levels in both the training and validation sets. Additionally, we observed that in the training set, patients with a mean VAF ≥ 4.94% showed inferior OS and PFS as compared with patients with a mean VAF < 4.94%. This finding was verified in the validation set.
Moreover, we assessed the relationship between ctDNA mutation status and the prognosis of patients with newly diagnosed DLBCL. Notably, we found that patients with PCLO mutations had shorter OS and PFS. PCLO encodes a protein that functions as a part of the presynaptic cytoskeletal matrix, which is thought to be involved in neurotransmitter release regulation. It has been suggested that PCLO might play a role in calcium sensing. PCLO mutations have been detected by whole-exom sequencing in a variety of tumors, including DLBCL (28–31). In the mesenchymal subtype of glioblastomas, PCLO mutations have been shown to be associated with poor prognosis (31), but its association with the prognosis of DLBCL has not been reported. Mutations in PCLO are usually considered as passenger mutations with no functional consequences in DLBCL (28). In this study, PIM1 (34.1%), MYD88 (31.8%) and TP53 (20.5%) were the most common co-mutated genes with PCLO mutations detected in the ctDNA samples of DLBCL patients. Furthermore, we found that the mutation frequency of TNFAIP3 in PCLO mutated DLBCL patients was significantly higher than that of DLBCL patients without PCLO mutations [1.6% (2/125) vs. 13.6% (6/44)]. These four genes (PIM1, MYD88, TP53, TNFAIP3) has been identified to be the mutational drivers in DLBCL, which might partly explain the poor prognosis of patients carrying PCLO mutations (32–35). Additional work is needed to resolve the mechanism of action and role of PCLO mutations in DLBCL.
Evidence has demonstrated that ctDNA mutations are correlated with treatment response in DLBCL patients (36). According to the current gold standard for evaluating treatment response in lymphoma, the sensitivity and specificity of ctDNA profiling were 94.7% and 83.3% in refractory or relapse (r/r) DLBCL patients after CAR-T treatment; the median numbers of baseline ctDNA mutations in patients who remained long-term CR and in patients who relapsed or became refractory to CAR-T therapy were 3.0 and 14.3, respectively (36). Herein, we explored the relationship between ctDNA mutation status, the number of ctDNA mutations and mean VAF and the curative effect of R-CHOP regimen in DLBCL patients. Our results showed that patients without detectable ctDNA mutations had a higher CR rate to R-CHOP treatment as compared with patients with detectable ctDNA mutations, while the ctDNA mutation number and mean VAF showed no significant impacts on the CR rate.
Our study showed that age (> 60 years) and mean VAF (≥ 4.94%) were independent influencing factors on prognosis in the training set, while age (> 60 years), PCLO mutations and bulky disease status were independent influencing factors on prognosis in the validation set. The high heterogeneity of DLBCL may have caused these differences between the training and validation sets. Of course, the small sample size of our study may be another reason for the differences. In fact, the relatively small sample size is the main limitation of the present study, although we have recruited the largest cohort of Chinese DLBCL patients to date. To this end, we intend to include more Chinese DLBCL patients for analysis in the future.
Taken together, we herein have described the ctDNA mutation landscape of a largest cohort of Chinese patients with newly diagnosed DLBCL to date. Our results suggested that patients with detectable ctDNA mutations, a higher mean VAF value or PCLO mutations trended to have shorter OS and PFS and a lower CR rate. Our study provides evidence to support the feasibility of using ctDNA samples obtained from patients’ blood in prognosis prediction of newly diagnosed DLBCL.
Data availability statement
The data presented in the study are deposited in the National Genomics Data Center repository (https://ngdc.cncb.ac.cn), accession number PRJCA012539.
Ethics statement
The studies involving human participants were reviewed and approved by The Ethics Committee of Shanxi Cancer Hospital. Written informed consent for participation was not required for this study in accordance with the national legislation and the institutional requirements.
Author contributions
Concept and design: LS, WQ, JD. Acquisition, analysis, or interpretation of data: TG, MeZ, JL, XL, YR, YY, HW, XG, YH. Patients’ follow-up: MiZ, YY, MeZ. Statistical analysis: BX, TG, MJZ. Drafting of the manuscript: TG, MiZ, BX. Supervision: LS. All authors contributed to the article and approved the submitted version.
Funding
This study was supported by the foundation of Key Laboratory Construction Project Supported by Health Commission of Shanxi Province (Grant No. 2020SYS11), Shanxi Province Health Research Project Supported by Health Commission of Shanxi Province (Grant No. 2022121), and Research Project Supported by Shanxi Scholarship Council of China (Grant No. 2020-194).
Acknowledgments
We thank Shanghai Rightongene Biotechnology Co. Ltd. (Shanghai, China) for the assistance in targeted sequencing and mutation analysis. In the meanwhile, we sincerely thank Hongsheng Wang, Department of Tumor Pharmacology, SUN YAT-SEN UNIVERSITY, for his linguistic and logic advise.
Conflict of interest
Author BX and YR are employed by Shanghai Yuanqi Biomedical Technology Co., Ltd.
The remaining authors declare that the research was conducted in the absence of any commercial or financial relationships that could be construed as a potential conflict of interest.
Publisher’s note
All claims expressed in this article are solely those of the authors and do not necessarily represent those of their affiliated organizations, or those of the publisher, the editors and the reviewers. Any product that may be evaluated in this article, or claim that may be made by its manufacturer, is not guaranteed or endorsed by the publisher.
Supplementary material
The Supplementary Material for this article can be found online at: https://www.frontiersin.org/articles/10.3389/fonc.2022.1003957/full#supplementary-material
References
1. Coiffier B, Thieblemont C, Van Den Neste E, Lepeu G, Plantier I, Castaigne S, et al. Long-term outcome of patients in the lnh-98.5 trial, the first randomized study comparing rituximab-chop to standard chop chemotherapy in dlbcl patients: A study by the groupe d'etudes des lymphomes de l'adulte. Blood (2018) 2010(12):2040–5.
2. Alizadeh AA, Eisen MB, Davis RE, Ma C, Lossos IS, Rosenwald A, et al. Distinct types of diffuse Large b-cell lymphoma identified by gene expression profiling. Nature (2000) 403(6769):503–11. doi: 10.1038/35000501
3. Rosenwald A, Wright G, Chan WC, Connors JM, Campo E, Fisher RI, et al. The use of molecular profiling to predict survival after chemotherapy for diffuse Large-B-Cell lymphoma. N Engl J Med (2002) 346(25):1937–47. doi: 10.1056/NEJMoa012914
4. Schmitz R, Wright GW, Huang DW, Johnson CA, Phelan JD, Wang JQ, et al. Genetics and pathogenesis of diffuse Large b-cell lymphoma. N Engl J Med (2018) 378(15):1396–407. doi: 10.1056/NEJMoa1801445
5. Wright GW, Huang DW, Phelan JD, Coulibaly ZA, Roulland S, Young RM, et al. A probabilistic classification tool for genetic subtypes of diffuse Large b cell lymphoma with therapeutic implications. Cancer Cell (2020) 37(4):551–68.e14. doi: 10.1016/j.ccell.2020.03.015
6. Decruyenaere P, Offner F, Vandesompele J. Circulating rna biomarkers in diffuse Large b-cell lymphoma: A systematic review. Exp Hematol Oncol (2021) 10(1):13. doi: 10.1186/s40164-021-00208-3
7. Cirillo M, Craig AFM, Borchmann S, Kurtz DM. Liquid biopsy in lymphoma: Molecular methods and clinical applications. Cancer Treat Rev (2020) 91:102106. doi: 10.1016/j.ctrv.2020.102106
8. Rossi D, Diop F, Spaccarotella E, Monti S, Zanni M, Rasi S, et al. Diffuse Large b-cell lymphoma genotyping on the liquid biopsy. Blood (2017) 129(14):1947–57. doi: 10.1182/blood-2016-05-719641
9. Cescon DW, Bratman SV, Chan SM, Siu LL. Circulating tumor DNA and liquid biopsy in oncology. Nat Cancer (2020) 1(3):276–90. doi: 10.1038/s43018-020-0043-5
10. Liu H, Yang C, Zhao X, Le J, Wu G, Wei J, et al. Genotyping on ctdna identifies shifts in mutation spectrum between newly diagnosed and Relapse/Refractory dlbcl. Onco Targets Ther (2020) 13:10797–806. doi: 10.2147/OTT.S275334
11. Kurtz DM, Scherer F, Jin MC, Soo J, Craig AFM, Esfahani MS, et al. Circulating tumor DNA measurements as early outcome predictors in diffuse Large b-cell lymphoma. J Clin Oncol (2018) 36(28):2845–53. doi: 10.1200/JCO.2018.78.5246
12. Swerdlow SH, Campo E, Pileri SA, Harris NL, Stein H, Siebert R, et al. The 2016 revision of the world health organization classification of lymphoid neoplasms. Blood (2016) 127(20):2375–90. doi: 10.1182/blood-2016-01-643569
13. Hans CP, Weisenburger DD, Greiner TC, Gascoyne RD, Delabie J, Ott G, et al. Confirmation of the molecular classification of diffuse Large b-cell lymphoma by immunohistochemistry using a tissue microarray. Blood (2004) 103(1):275–82. doi: 10.1182/blood-2003-05-1545
14. Scherer F, Kurtz DM, Newman AM, Stehr H, Craig AF, Esfahani MS, et al. Distinct biological subtypes and patterns of genome evolution in lymphoma revealed by circulating tumor DNA. Sci Transl Med (2016) 8(364):364ra155. doi: 10.1126/scitranslmed.aai8545
15. Bohers E, Viailly PJ, Dubois S, Bertrand P, Maingonnat C, Mareschal S, et al. Somatic mutations of cell-free circulating DNA detected by next-generation sequencing reflect the genetic changes in both germinal center b-Cell-Like and activated b-Cell-Like diffuse Large b-cell lymphomas at the time of diagnosis. Haematologica (2015) 100(7):e280–4. doi: 10.3324/haematol.2015.123612
16. Camus V, Sarafan-Vasseur N, Bohers E, Dubois S, Mareschal S, Bertrand P, et al. Digital pcr for quantification of recurrent and potentially actionable somatic mutations in circulating free DNA from patients with diffuse Large b-cell lymphoma. Leuk Lymphoma (2016) 57(9):2171–9. doi: 10.3109/10428194.2016.1139703
17. Karube K, Enjuanes A, Dlouhy I, Jares P, Martin-Garcia D, Nadeu F, et al. Integrating genomic alterations in diffuse Large b-cell lymphoma identifies new relevant pathways and potential therapeutic targets. Leukemia (2018) 32(3):675–84. doi: 10.1038/leu.2017.251
18. Reddy A, Zhang J, Davis NS, Moffitt AB, Love CL, Waldrop A, et al. Genetic and functional drivers of diffuse Large b cell lymphoma. Cell (2017) 171(2):481–94.e15. doi: 10.1016/j.cell.2017.09.027
19. Ren W, Ye X, Su H, Li W, Liu D, Pirmoradian M, et al. Genetic landscape of hepatitis b virus-associated diffuse Large b-cell lymphoma. Blood (2018) 131(24):2670–81. doi: 10.1182/blood-2017-11-817601
20. Pasqualucci L, Dalla-Favera R. Genetics of diffuse Large b-cell lymphoma. Blood (2018) 131(21):2307–19. doi: 10.1182/blood-2017-11-764332
21. Rivas-Delgado A, Nadeu F, Enjuanes A, Casanueva-Eliceiry S, Mozas P, Magnano L, et al. Mutational landscape and tumor burden assessed by cell-free DNA in diffuse Large b-cell lymphoma in a population-based study. Clin Cancer Res (2021) 27(2):513–21. doi: 10.1158/1078-0432.CCR-20-2558
22. Kurtz DM, Soo J, Co Ting Keh L, Alig S, Chabon JJ, Sworder BJ, et al. Enhanced detection of minimal residual disease by targeted sequencing of phased variants in circulating tumor DNA. Nat Biotechnol (2021) 39(12):1537–47. doi: 10.1038/s41587-021-00981-w
23. van Velzen MJM, Creemers A, van den Ende T, Schokker S, Krausz S, Reinten RJ, et al. Circulating tumor DNA predicts outcome in metastatic gastroesophageal cancer. Gastric Cancer (2022) 25(5):906–15. doi: 10.1007/s10120-022-01313-w
24. Arisi MF, Dotan E, Fernandez SV. Circulating tumor DNA in precision oncology and its applications in colorectal cancer. Int J Mol Sci (2022) 23(8):4441. doi: 10.3390/ijms23084441
25. Fu H, Zhou H, Qiu Y, Wang J, Ma Z, Li H, et al. Sept6_Trim33 gene fusion and mutated Tp53 pathway associate with unfavorable prognosis in patients with b-cell lymphomas. Front Oncol (2021) 11:765544. doi: 10.3389/fonc.2021.765544
26. Desch AK, Hartung K, Botzen A, Brobeil A, Rummel M, Kurch L, et al. Genotyping circulating tumor DNA of pediatric Hodgkin lymphoma. Leukemia (2020) 34(1):151–66. doi: 10.1038/s41375-019-0541-6
27. Soerensen JF, Aggerholm A, Rosenberg CA, Bill M, Kerndrup GB, Ebbesen LH, et al. Clonal evolution in patients developing therapy-related myeloid neoplasms following autologous stem cell transplantation. Bone marrow Transplant (2022) 57(3):460–5. doi: 10.1038/s41409-022-01567-z
28. Lohr JG, Stojanov P, Lawrence MS, Auclair D, Chapuy B, Sougnez C, et al. Discovery and prioritization of somatic mutations in diffuse Large b-cell lymphoma (Dlbcl) by whole-exome sequencing. Proc Natl Acad Sci U S A (2012) 109(10):3879–84. doi: 10.1073/pnas.1121343109
29. Wang H, Shen L, Li Y, Lv J. Integrated characterisation of cancer genes identifies key molecular biomarkers in stomach adenocarcinoma. J Clin Pathol (2020) 73(9):579–86. doi: 10.1136/jclinpath-2019-206400
30. Qiu Z, Lin A, Li K, Lin W, Wang Q, Wei T, et al. A novel mutation panel for predicting etoposide resistance in small-cell lung cancer. Drug Des Devel Ther (2019) 13:2021–41. doi: 10.2147/DDDT.S205633
31. Park AK, Kim P, Ballester LY, Esquenazi Y, Zhao Z. Subtype-specific signaling pathways and genomic aberrations associated with prognosis of glioblastoma. Neuro Oncol (2019) 21(1):59–70. doi: 10.1093/neuonc/noy120
32. Juskevicius D, Lorber T, Gsponer J, Perrina V, Ruiz C, Stenner-Liewen F, et al. Distinct genetic evolution patterns of relapsing diffuse Large b-cell lymphoma revealed by genome-wide copy number aberration and targeted sequencing analysis. Leukemia (2016) 30(12):2385–95. doi: 10.1038/leu.2016.135
33. Ngo VN, Young RM, Schmitz R, Jhavar S, Xiao W, Lim KH, et al. Oncogenically active Myd88 mutations in human lymphoma. Nature (2011) 470(7332):115–9. doi: 10.1038/nature09671
34. Lu TX, Young KH, Xu W, Li JY. Tp53 dysfunction in diffuse Large b-cell lymphoma. Crit Rev Oncol Hematol (2016) 97:47–55. doi: 10.1016/j.critrevonc.2015.08.006
35. Chapuy B, Stewart C, Dunford AJ, Kim J, Kamburov A, Redd RA, et al. Molecular subtypes of diffuse Large b cell lymphoma are associated with distinct pathogenic mechanisms and outcomes. Nat Med (2018) 24(5):679–90. doi: 10.1038/s41591-018-0016-8
Keywords: diffuse large B cell lymphoma, circulating tumor DNA, targeted next-generation sequencing, mutation, prognosis
Citation: Guan T, Zhang M, Liu X, Li J, Xin B, Ren Y, Yang Y, Wang H, Zhao M, Huang Y, Guo X, Du J, Qian W and Su L (2022) Circulating tumor DNA mutation profile is associated with the prognosis and treatment response of Chinese patients with newly diagnosed diffuse large B-cell lymphoma. Front. Oncol. 12:1003957. doi: 10.3389/fonc.2022.1003957
Received: 26 July 2022; Accepted: 17 October 2022;
Published: 17 November 2022.
Edited by:
Aliyah Sohani, Department of Pathology, Massachusetts General Hospital and Harvard Medical School, United StatesReviewed by:
Youngwoo Jeon, Catholic University of Korea, South KoreaMir Basharath Alikhan, NorthShore University HealthSystem, United States
Copyright © 2022 Guan, Zhang, Liu, Li, Xin, Ren, Yang, Wang, Zhao, Huang, Guo, Du, Qian and Su. This is an open-access article distributed under the terms of the Creative Commons Attribution License (CC BY). The use, distribution or reproduction in other forums is permitted, provided the original author(s) and the copyright owner(s) are credited and that the original publication in this journal is cited, in accordance with accepted academic practice. No use, distribution or reproduction is permitted which does not comply with these terms.
*Correspondence: Liping Su, c3VscDIwMDVAMTYzLmNvbQ==; Wenbin Qian, cWlhbndiQHpqdS5lZHUuY24=; Jun Du, ZHVqdW5AbWFpbC5zeXN1LmVkdS5jbg==
†These authors have contributed equally to this work and share first authorship