- 1Department of Radiology, Chengdu Second People’s Hospital, Chengdu, China
- 2Department of Radiology, Huadong Hospital Affiliated With Fudan University, Shanghai, China
Purpose: To compare conventional diffusion weighted imaging (DWI), intravoxel incoherent motion imaging (IVIM) and diffusion kurtosis imaging (DKI) in differentiating malignant and benign lung lesions.
Method: Fifty-five consecutive patients with lung lesions underwent multiple b-value DWI. The apparent diffusion coefficient (ADC), IVIM and DKI parameters were calculated using postprocessing software and compared between the malignant and benign groups. Receiver operating characteristic (ROC) analysis was performed for all parameters.
Results: ADC and D were lower in malignant lesions than in benign lesions, while Kapp was higher (P < 0.05). The differences in D*, f, and Dapp between the two groups were not significant (P > 0.05). The areas under the curves (AUCs) of ADC, D, and Kapp were 0.816, 0.864, and 0.822. The combination of all the significant parameters yielded an AUC of 0.880. There were no significant differences in diagnostic efficacy among ADC, D, Kapp and the predictor factor (PRE).
Conclusions: In this study, traditional DWI (ADC), IVIM (D), and DKI (Kapp) all had good diagnostic performance in differentiating malignant lung lesions from benign lesions, but the combination of ADC, D, and Kapp value had better diagnostic efficacy than these parameters alone.
Introduction
Lung cancer is the leading malignant tumour in the world in terms of morbidity and mortality (1). The accurate diagnosis and differential diagnosis of patients with lung lesions are beneficial to the selection of treatment options. Because of the overlap of the morphological characteristics of benign and malignant lesions, it is challenging to distinguish them on computed tomography (CT) based on morphology. Positron emission tomography (PET)-CT is not only expensive but also has a high false-positive rate. Some inflammatory lesions also have high fluorodeoxyglucose (FDG) uptake (2).
In recent years, with the development of magnetic resonance (MR) technology, traditional diffusion-weighted imaging (DWI) has become a means to distinguish benign and malignant lung lesions (3). However, the apparent diffusion coefficient (ADC), which reflects the degree of diffusion limitation, not only reflects the diffusion of water molecules in tissues but is also affected by microcirculation (4). Compared with conventional DWI, intravoxel incoherent motion (IVIM) can separate the diffusion of water molecules and the microcirculation of tissues (5). Theoretically, IVIM can more accurately reflect the diffusion of water molecules in tissues. IVIM has been proven useful for the identification of tumours (6). Currently, the diagnostic performance outcomes of IVIM parameters in the differentiation of lung lesions are inconsistent (7).
In addition, diffusion kurtosis imaging (DKI) can reflect the diffusion of water molecules in a non-Gaussian distribution and the complexity of tissues (8). At present, DKI is mainly used in the study of the central nervous system, abdomen, pelvis, breast and other body parts, while it is less commonly used in the study of pulmonary lesions (4, 9).
Therefore, the purpose of this study was to compare traditional DWI, IVIM and DKI in differentiating benign and malignant lung lesions, providing more references for clinical practice.
Materials and Methods
Patients
This prospective study was approved by the local ethics committee. Written informed consent was obtained from all patients. Sixty-five patients with lung lesions from October 2019 to November 2020 were enrolled.
The inclusion criteria were as follows (1): All patients had pulmonary lesions found by CT, the diameter of the lesions was ≥ 10 mm (3), and the solid component was more than 50% (2). Pathological results were obtained, and some inflammatory lesions were confirmed by follow-up after anti-inflammatory treatment (3). All patients underwent an MRI scan within 1 week after lung lesions were found on CT and did not undergo any treatment before scanning.
The exclusion criteria were as follows (1): contraindications of MRI scanning; and (2) unsatisfactory imaging quality.
Image Acquisition
All MRI examinations were performed with a 3.0T MR scanner (MAGNETOM Prisma, Siemens Healthcare, Erlangen, Germany) using a 32-channel body coil and an integrated spine coil. Routine scanning included T1-volumetric interpolated breath-hold examination (VIBE) and coronal and transverse half-Fourier-acquisition single-shot turbo spin-echo (HASTE) T2-weighted imaging (T2WI). The following parameters were employed for conventional axial T2WI: TR/TE, 1400/91 ms; slice thickness, 5 mm; intersection gap, 0.3 mm; matrix, 320×320; and FOV, 400 mm×400 mm.
A multiple b-value DWI with a single-shot echo-planar imaging pulse sequence in the axial orientation during free breathing was performed. The parameters were as follows: twelve b values from 0 to 2000 s/mm2 (b=0, 20, 60, 80, 150, 200, 400, 600, 800, 1200, 1600, and 2000 s/mm2); TR/TE, 4000/54 ms; slice thickness, 5 mm; gap, 0.2 mm; matrix, 128×128; FOV, 380 mm×380 mm; directions, 3; and scanning time, 8 min 24 s (Table 1).
Image Data Analysis
The MRI data were processed using Whole Body Diffusion Toolbox software (Siemens Medical Systems). Multiple b-value DWI data were postprocessed with different models. ADC was calculated using the monoexponential model from DWI with b values of 0 and 800 s/mm² using a monoexponential fit of signal intensity with the following equation (4):
where Sb represents the signal intensity at a specified b value, and S0 is the signal intensity at b = 0 s/mm2.
Meanwhile, the IVIM parameters (D, D*, and f) were obtained with biexponential fit models using 9 b values (0, 20, 60, 80, 150, 200, 400, 600, and 800 s/mm2). The DWI signal intensity and b factors were fitted to the following equation (5):
where D is the true diffusion coefficient representing pure molecular diffusion, D* is the pseudo-diffusion coefficient representing incoherent microcirculation, and f is the fraction of perfusion.
In addition, DKI parameters (Kapp and Dapp) were derived using six b value signal intensities (b = 0, 600, 800, 1200, 1600, and 2000 s/mm²). The following equation was used for the DKI parameter calculation (10):
where Kapp is a unitless parameter, representing the deviation of water motion from Gaussian diffusion, and Dapp is the kurtosis-corrected diffusion coefficient.
Two radiologists (with 5 and 8 years of experience in thoracic MRI) who were blinded to the pathologic results drew regions of interest (ROIs) on the ADC maps and recorded the values of each parameter independently. Each lesion was outlined twice, and then the average value was taken for analysis. Tumour ROIs were drawn by outlining tumour borders on ADC maps showing the largest tumour cross-sections and avoiding necrotic areas and adjacent large vessels by referring to T2WI and DWI images. The minimum size of ROIs is 121mm2. The ROIs were automatically copied from the ADC maps to the corresponding IVIM and DKI parametric maps to obtain the values of D, D*, f, Dapp, and Kapp.
Statistical Analysis
The data are presented as median and interquartile range. The intraclass correlation coefficient (ICC) with a 95% confidence interval (CI) was used to evaluate interobserver agreement for parameter measurements (0.00-0.20, poor agreement; 0.21-0.40, fair agreement; 0.41-0.60, moderate agreement; 0.61-0.80, good agreement; and 0.81-1.00, excellent agreement) (11).
ADC, IVIM and DKI parameters were compared between the malignant and benign groups by Student’s t-test or the Mann-Whitney U test. A P < 0.05 was considered statistically significant. The significant parameters were fitted by logistic regression, and a predictive factor (PRE) was generated. Receiver operating characteristic (ROC) curve analyses were further performed to evaluate the diagnostic efficacy and determine the optimal cut-off value of each parameter in predicting malignancy. The areas under the curve (AUCs), sensitivity, specificity, and Youden index were calculated. AUCs were compared using the DeLong method (12). Statistical analyses were performed with SPSS 22.0 (IBM SPSS Statistics, USA), GraphPad Prism 5.0 (Prism, USA) and MedCalc 19.0.4 (MedCalc, Ostend, Belgium).
Results
Patients’ Characteristics
A total of sixty-five patients were consecutively included in our study. Four patients were excluded due to MRI contraindications, and six patients were excluded due to inferior imaging quality. The remaining fifty-five patients were enrolled in the final cohort, including twenty-nine males (52.73%) and twenty-six females (47.27%) with an average age of 54 years (range of 32–86 years). Among the fifty-five patients included, thirty-two patients had malignant tumours and twenty-three had benign lesions. Malignant lesions were adenocarcinoma (n=19), squamous cell carcinoma (n=6), large cell carcinoma (n=2), small cell lung cancer (SCLC) (n=4) and solitary metastatic tumour (n=1). Benign lesions were pulmonary tuberculosis (n=10), organizing pneumonia (n=7), inflammatory pseudotumour (n=5), and sclerosing pneumocytoma (n=1).
Comparison of ADC, IVIM and DKI Parameters Between Malignant and Benign Lesions
The interobserver reproducibility ranged from good to excellent for the ADC, IVIM and DKI parameters (ADC: ICC = 0.848, 95% CI = 0.753–0.909; D: ICC = 0.881, 95% CI = 0.804–0.929; D*: ICC = 0.810, 95% CI = 0.695–0.885; f: ICC = 0.706, 95% CI = 0.544–0.817; Kapp: ICC = 0.845, 95% CI = 0.748–0.907; Dapp: ICC = 0.811, 95% CI = 0.697–0.885).
The differences in ADC, IVIM and DKI parameters between benign and malignant lesions are shown in Table 2 and Figure 1. The ADC and D values of malignant tumours were lower than those of benign lesions, while the Kapp value of malignant tumours was higher than that of benign lesions, and the differences were statistically significant (P < 0.05). The differences in D*, f, and Dapp between these two groups were not significantly different (P> 0.05). Typical cases are shown in Figures 2 and 3.
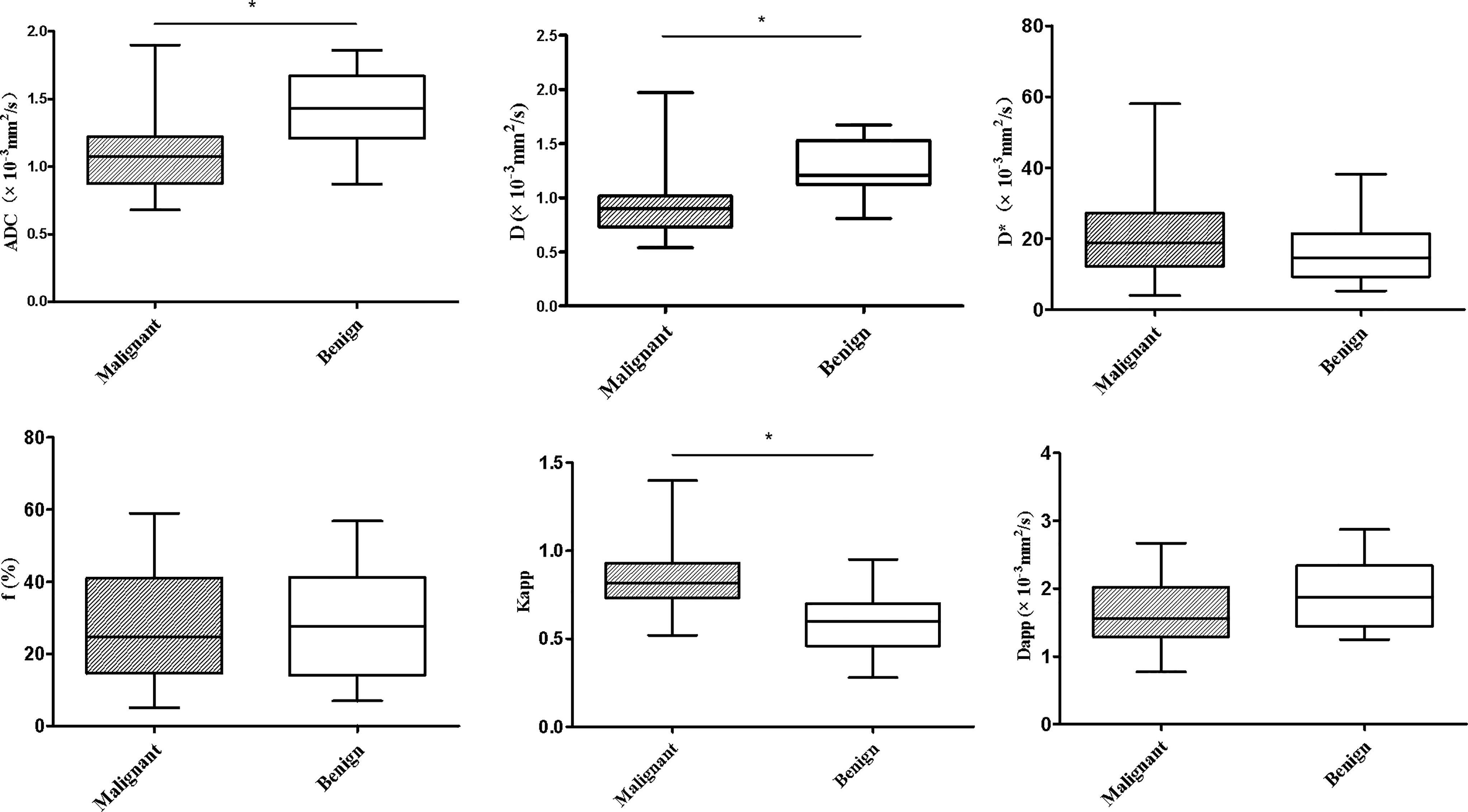
Figure 1 Whiskers boxplots of ADC, D, D*, f, Kapp, Dapp values of malignant and benign lung lesions. *p < 0.05.
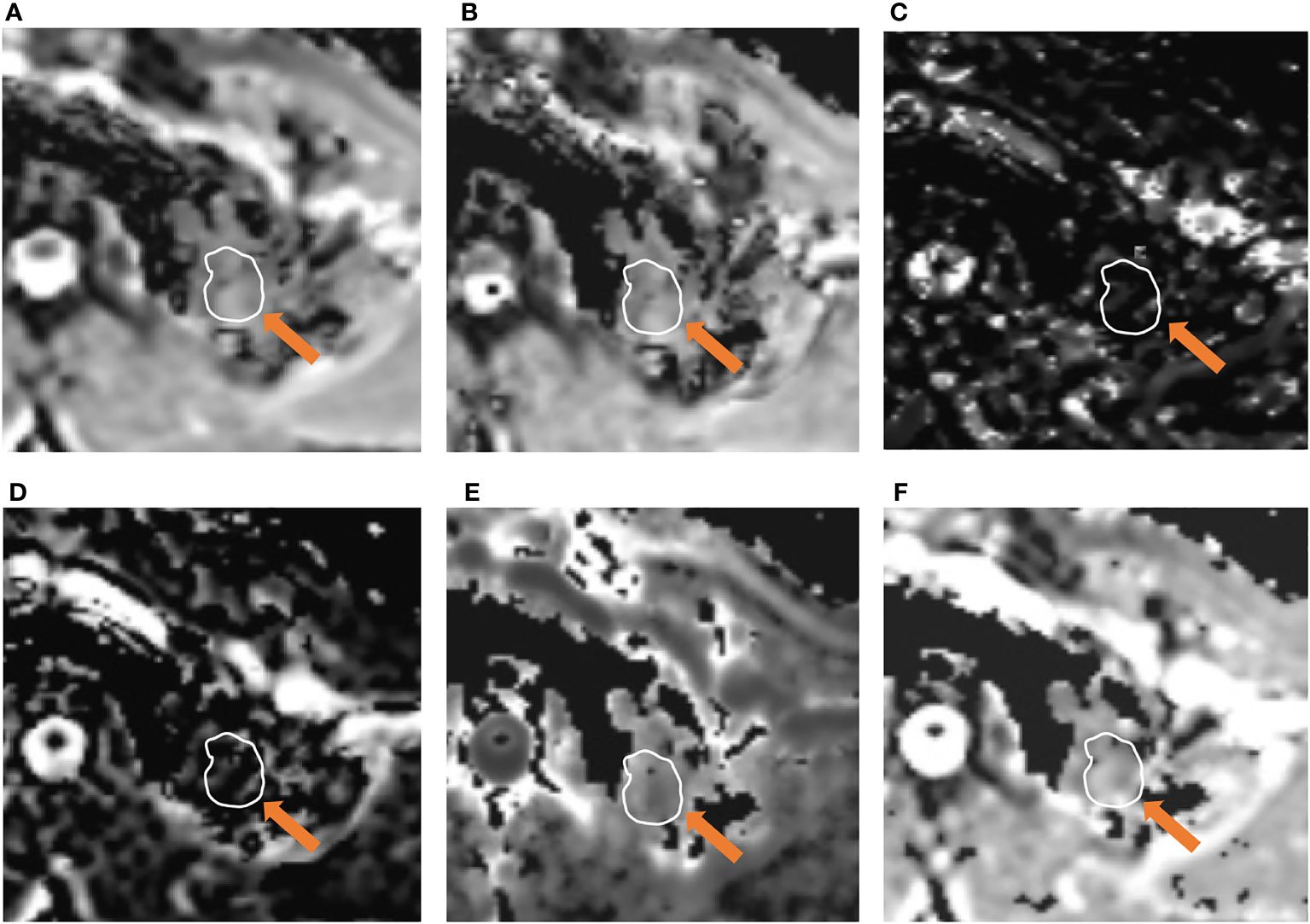
Figure 2 A 51-year-old male was diagnosed with pulmonary tuberculosis in the left upper lobe. Parameter map of ADC, D, D*, f, Kapp, and Dapp calculated from multiple b-value DWI data (A–F). The calculated mean values of ADC, D, D*, f, Kapp, and Dapp were 1.21×10-3 mm2/s, 1.13×10-3mm2/s, 8.74×10-3 mm2/s, 12.62%, 0.65, and 2.34×10-3 mm2/s, respectively.
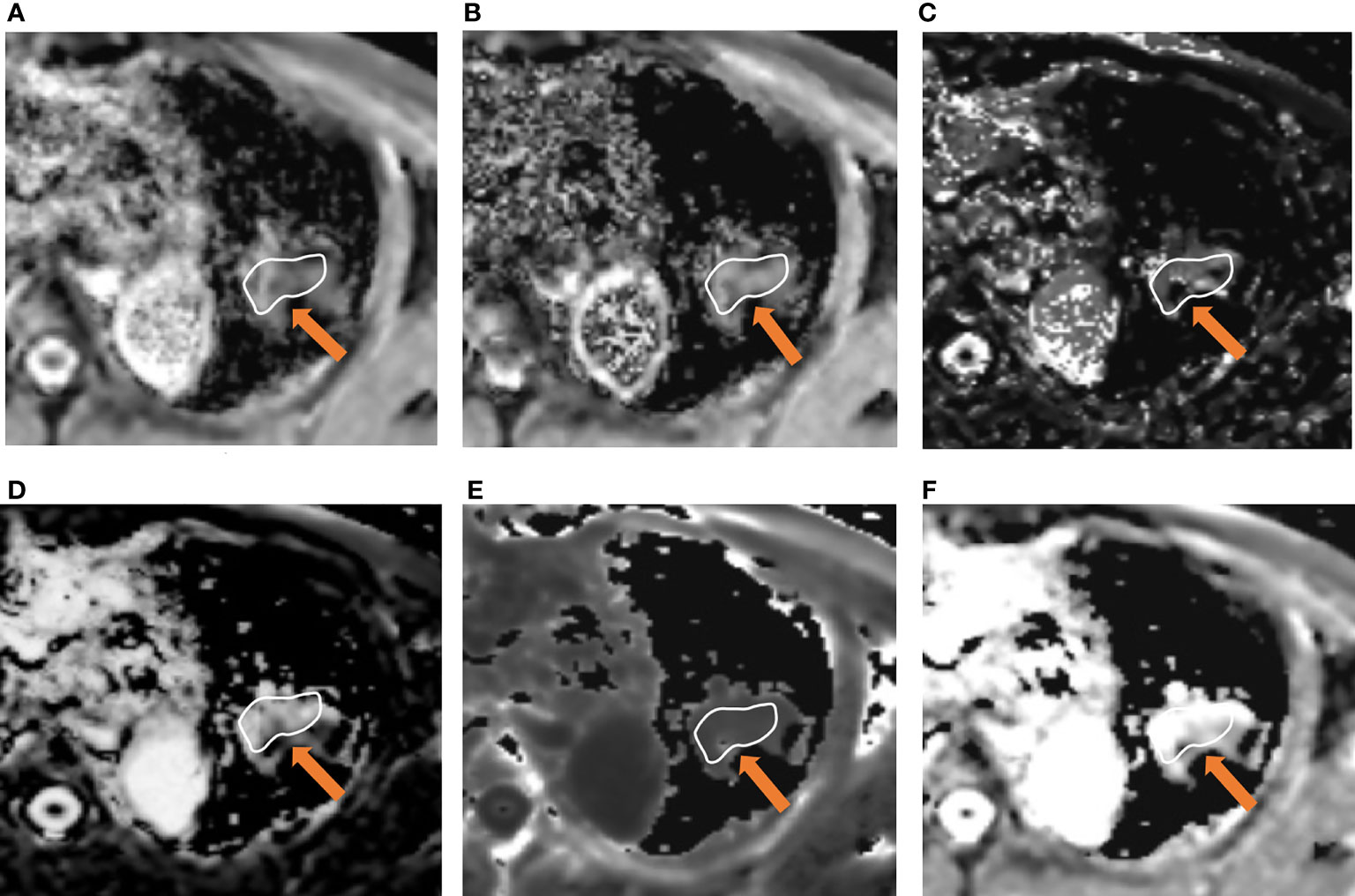
Figure 3 A 66-year-old male was diagnosed with lung adenocarcinoma in the left upper lobe. Parameter map of ADC, D, D*, f, Kapp, and Dapp calculated from multiple b-value DWI data (A–F). The calculated mean values of ADC, D, D*, f, Kapp, and Dapp were 0.84×10-3 mm2/s, 0.65×10-3mm2/s, 35.23×10-3 mm2/s, 32.31%, 1.01, and 2.21×10-3 mm2/s, respectively.
Diagnostic Efficiency of Quantitative Parameters
The ROC curves of quantitative parameters for distinguishing malignant lung lesions from benign lesions are shown in Figure 4, and the corresponding diagnostic performance are plotted in Table 3. The AUCs of ADC, D, and Kapp were 0.816, 0.864, and 0.822, respectively. Among the significant parameters, the best predictive parameter was D (AUC = 0.845, cut-off value ≤ 1.07 × 10-3 mm2/s, sensitivity = 84.37%, and specificity = 82.61%). The above factors were then fitted by logistic regression, and a PRE was generated, the results of logistic regression are shown in Table 4. The PRE achieved a high AUC of 0.887, with 91.3% sensitivity and 78.12% specificity. There were no statistically significant differences in diagnostic efficacy among ADC, D, Kapp and PRE when pairwise comparisons of ROC curves were performed.
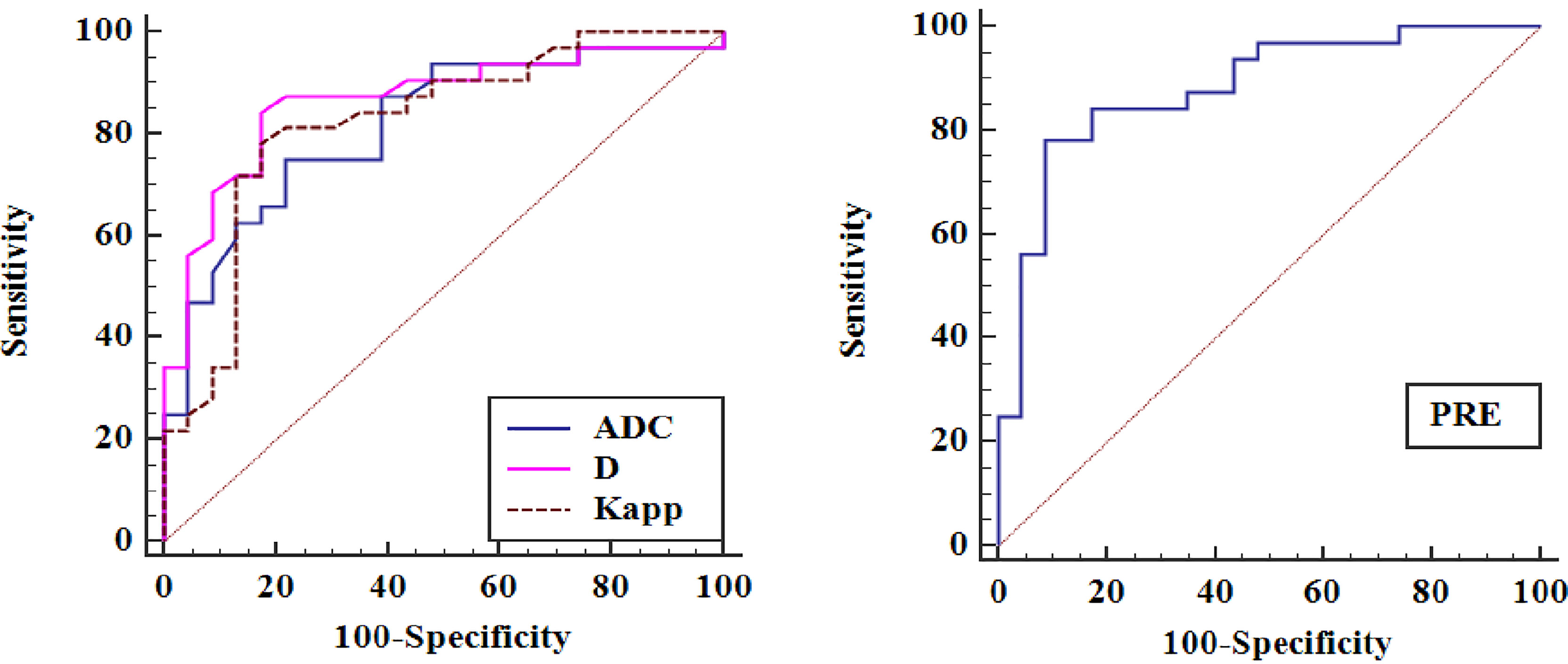
Figure 4 Receiver operating characteristic curves (ROCs) for ADC, D, Kapp and PRE in distinguishing pulmonary malignant tumours from benign lesions.
Discussion
We found that the DWI-derived parameter ADC, the IVIM-derived parameter diffusion coefficient D and the DKI-derived parameter diffusion kurtosis Kapp are useful for the diagnosis of lung lesions. Compared with benign lesions, malignant lesions had significantly lower D and ADC values and significantly higher Kapp values. Among these significant parameters, the D value had a higher AUC than the ADC and Kapp values. Finally, the combination of all the significant parameters fitted by logistic regression yielded an AUC of 0.880, which had the strongest predictive value. Therefore, the combination of ADC, D, and Kapp value had better diagnostic efficacy than these parameters alone
DWI, characterizing the restriction of random thermal motion of water molecules, has been applied for the detection and characterization of lung lesions. Several studies (13–16) have shown that the ADC value of malignant lung tumours is significantly lower than that of benign lesions. Our data showed that the average ADC values were higher than their D values. The reason may be that ADC not only reflects the diffusion of water molecules but is also affected by microcirculation perfusion. In this study, DWI with multiple b values (b values from 0 to 2000 s/mm2) were used, and it was found that the ADC and D values of malignant lung lesions were significantly lower than those of benign lung lesions. The reason may be that malignant tumour cells proliferate rapidly, resulting in high cell density. In addition, malignant tumour cells have larger nuclei and less cytoplasm, which reduces the extracellular space and aggravates the restriction of water molecules.
However, the diffusion parameter Dapp derived from DKI showed a lower trend in malignant tumours, but there was no significant difference between the two groups, which was inconsistent with the findings of some previous studies (9, 17, 18), which found that the Dapp of malignant lesions was significantly lower than that of benign lesions. This discrepancy may be due to the heterogeneity of the tumour and the size and number of b values selected in DKI. In addition, the Kapp of malignant lung tumours was significantly higher than that of benign lung lesions. Theoretically, the cell structure and tissue environment of malignant tumours are more complex than those of benign lesions, resulting in a more obvious non-Gaussian distribution of tumour tissues and a higher diffusion kurtosis value. Previous studies have also shown that the diffusion kurtosis of malignant tumours is higher than that of benign lesions (17–19).
Our study showed that the D value has better diagnostic efficiency than the ADC value, which may be related to its imaging principles because it only reflects the pure diffusion of water molecules without the influence of microcirculation perfusion and can more accurately reflect the restriction of tissue water molecules. In addition, the combination of all the significant parameters fitted by logistic regression yielded an AUC of 0.887, which shows that compared with a single indicator, the combined indicators can more accurately distinguish malignant and benign lung lesions in clinical practice.
Some previous studies have shown that f is significantly meaningful in the diagnosis of lung lesions (20, 21) and other lesions (22, 23). In this study, the perfusion-related parameters D* and f were not significantly different between malignant lung tumours and benign lesions, which is in line with the findings of previous studies (4, 24, 25). The reason may be that the repeatability of D* and f values in pulmonary diffusion imaging is poor and has great variability, which is affected by the shape, size and location of the lesion (26). Similarly, in our study, the ICCs for f were lower than those of the other parameters. Moreover, the f value is affected by the T2 contributions of both perfusion and pure molecular diffusion compartments (11).
This study has some limitations. First, the sample size was not large, and the types of lung lesions were limited; therefore, it is necessary to expand the sample size and increase the types of pulmonary lesions in further research. Second, there is no unified standard for the selection of b values in IVIM and DKI imaging. Although more b values can improve the accuracy, the corresponding scanning time will be prolonged. Third, the mean value of the ROI was taken to define the measured parameter, and perhaps a histogram and volumetric analysis of these heterogeneous lesions may have been more useful to diagnose these lesions.
In conclusion, our results indicate that mean ADC, D, and Kapp values are useful for the discrimination between pulmonary malignant and benign lesions, which indicates that multiple b-value DWI provides additional information for clinical diagnosis. Larger, prospective studies are needed to confirm our findings.
Data Availability Statement
The original contributions presented in the study are included in the article/supplementary material. Further inquiries can be directed to the corresponding authors.
Ethics Statement
The studies involving human participants were reviewed and approved by the Ethic Committee of the Huadong Hospital Affiliated with Fudan University. The patients/participants provided their written informed consent to participate in this study.
Author Contributions
NL and SHL conceived and presented idea. JL and YZ collected the data. KC, XCZ, HS, SJL, XZ and ZD analyzed the data. YZ drafted the manuscript. All authors reviewed the manuscript, and NL made corrections to the manuscript. All authors contributed to the article and approved the submitted version.
Funding
This study was supported by Medical Scientific Research Project of Sichuan Province (S17007), and the General Project from Shanghai Municipal Health Bureau (CN) (201940174).
Conflict of Interest
The authors declare that the research was conducted in the absence of any commercial or financial relationships that could be construed as a potential conflict of interest.
Publisher’s Note
All claims expressed in this article are solely those of the authors and do not necessarily represent those of their affiliated organizations, or those of the publisher, the editors and the reviewers. Any product that may be evaluated in this article, or claim that may be made by its manufacturer, is not guaranteed or endorsed by the publisher.
Abbreviations
ROIs, regions of interest; ICC, intraclass correlation coefficient; CI, confidence interval; PRE, predictive factor; AUCs, areas under the curve.
References
1. Torre LA, Bray F, Siegel RL, Ferlay J, Lortet-Tieulent J, Jemal A. Global Cancer Statistics, 2012. CA Cancer J Clin (2015) 65:87–108. doi: 10.3322/caac.21262
2. Nomori H, Cong Y, Sugimura H, Kato Y. Diffusion-Weighted Imaging can Correctly Identify False-Positive Lymph Nodes on Positron Emission Tomography in non-Small Cell Lung Cancer. Surg Today (2016) 46:1146–51. doi: 10.1007/s00595-015-1285-1
3. Shen G, Jia Z, Deng H. Apparent Diffusion Coefficient Values of Diffusion-Weighted Imaging for Distinguishing Focal Pulmonary Lesions and Characterizing the Subtype of Lung Cancer: A Meta-Analysis. Eur Radiol (2016) 26:556–66. doi: 10.1007/s00330-015-3840-y
4. Wan Q, Deng YS, Lei Q, Bao YY, Wang YZ, Zhou JX, et al. Differentiating Between Malignant and Benign Solid Solitary Pulmonary Lesions: Are Intravoxel Incoherent Motion and Diffusion Kurtosis Imaging Superior to Conventional Diffusion-Weighted Imaging? Eur Radiol (2019) 29:1607–15. doi: 10.1007/s00330-018-5714-6
5. Le Bihan D, Breton E, Lallemand D, Aubin ML, Vignaud J, Laval-Jeantet M. Separation of Diffusion and Perfusion in Intravoxel Incoherent Motion MR Imaging. Radiology (1988) 168:497–505. doi: 10.1148/radiology.168.2.3393671
6. Minh DN. The Diagnostic Function of Intravoxel Incoherent Motion for Distinguishing Between Pilocytic Astrocytoma and Ependymoma. PloS One (2021) 16:e0247899. doi: 10.1371/journal.pone.0247899
7. Liang J, Li J, Li Z, Meng T, Chen J, Ma W, et al. Differentiating the Lung Lesions Using Intravoxel Incoherent Motion Diffusion-Weighted Imaging: A Meta-Analysis. BMC Cancer (2020) 20:799. doi: 10.1186/s12885-020-07308-z
8. Rosenkrantz AB, Padhani AR, Chenevert TL, Koh DM, De Keyzer F, Taouli B, et al. Body Diffusion Kurtosis Imaging: Basic Principles, Applications, and Considerations for Clinical Practice. J Magn Reson Imaging (2015) 42:1190–202. doi: 10.1002/jmri.24985
9. Das SK, Yang DJ, Wang JL, Zhang C, Yang HF. Non-Gaussian Diffusion Imaging for Malignant and Benign Pulmonary Nodule Differentiation: A Preliminary Study. Acta Radiol (2017) 58:19–26. doi: 10.1177/0284185116639763
10. Jensen JH, Helpern JA, Ramani A, Lu H, Kaczynski K. Diffusional Kurtosis Imaging: The Quantification of non-Gaussian Water Diffusion by Means of Magnetic Resonance Imaging. Magn Reson Med (2005) 53:1432–40. doi: 10.1002/mrm.20508
11. Nougaret S, Vargas HA, Lakhman Y, Sudre R, Do RKG, Bibeau F, et al. Intravoxel Incoherent Motion-Derived Histogram Metrics for Assessment of Response After Combined Chemotherapy and Radiation Therapy in Rectal Cancer: Initial Experience and Comparison Between Single-Section and Volumetric Analyses. Radiology (2016) 280:446–54. doi: 10.1148/radiol.2016150702
12. DeLong ER, DeLong DM, Clarke-Pearson DL. Comparing the Areas Under Two or More Correlated Receiver Operating Characteristic Curves: A Nonparametric Approach. Biometrics (1988) 44:837–45. doi: 10.2307/2531595
13. Çakmak V, Ufuk F, Karabulut N. Diffusion-Weighted MRI of Pulmonary Lesions: Comparison of Apparent Diffusion Coefficient and Lesion-to-Spinal Cord Signal Intensity Ratio in Lesion Characterization. J Magn Reson Imaging (2017) 45:845–54. doi: 10.1002/jmri.25426
14. Çakır Ç, Gençhellaç H, Temizöz O, Polat A, Şengül E, Duygulu G. Diffusion Weighted Magnetic Resonance Imaging for the Characterization of Solitary Pulmonary Lesions. Balkan Med J (2015) 32:403–9. doi: 10.5152/balkanmedj.2015.15663
15. Usuda K, Sagawa M, Maeda S, Motono N, Tanaka M, Machida Y, et al. Diagnostic Performance of Whole-Body Diffusion-Weighted Imaging Compared to PET-CT Plus Brain MRI in Staging Clinically Resectable Lung Cancer. Asian Pac J Cancer Prev (2016) 17:2775–80. doi: 10.1016/S0959-8049(17)30652-4
16. Tondo F, Saponaro A, Stecco A, Lombardi M, Casadio C, Carriero A. Role of Diffusion-Weighted Imaging in the Differential Diagnosis of Benign and Malignant Lesions of the Chest-Mediastinum. Radiol Med (2011) 116:720–33. doi: 10.1007/s11547-011-0629-1
17. Nogueira L, Brandão S, Matos E, Nunes RG, Loureiro J, Ramos I, et al. Application of the Diffusion Kurtosis Model for the Study of Breast Lesions. Eur Radiol (2014) 24:1197–203. doi: 10.1007/s00330-014-3146-5
18. Jiang JX, Tang ZH, Zhong YF, Qiang JW. Diffusion Kurtosis Imaging for Differentiating Between the Benign and Malignant Sinonasal Lesions. J Magn Reson Imaging (2016) 45:1446–54. doi: 10.1002/jmri.25500
19. Ogawa M, Kan H, Arai N, Murai T, Manabe Y, Sawada Y, et al. Differentiation Between Malignant and Benign Musculoskeletal Tumors Using Diffusion Kurtosis Imaging. Skeletal Radiol (2018) 48:285–92. doi: 10.1007/s00256-018-2946-0
20. Wang LL, Lin J, Liu K, Chen CZ, Liu H, Lv P, et al. Intravoxel Incoherent Motion Diffusion-Weighted MR Imaging in Differentiation of Lung Cancer From Obstructive Lung Consolidation: Comparison and Correlation With Pharmacokinetic Analysis From Dynamic Contrast-Enhanced MR Imaging. Eur Radiol (2014) 24:1914–22. doi: 10.1007/s00330-014-3176-z
21. Liu H, Zheng L, Shi G, Xu Q, Wang Q, Zhu H, et al. Pulmonary Functional Imaging for Lung Adenocarcinoma: Combined MRI Assessment Based on IVIM-DWI and OE-UTE-MRI. Front Oncol (2021) 11:677942. doi: 10.3389/fonc.2021.677942
22. Togao O, Hiwatashi A, Yamashita K, Kikuchi K, Mizoguchi M, Yoshimoto K, et al. Differentiation of High-Grade and Low-Grade Diffuse Gliomas by Intravoxel Incoherent Motion MR Imaging. Neuro Oncol (2016) 18:132–41. doi: 10.1093/neuonc/nov147
23. Liu C, Liang C, Liu Z, Zhang S, Huang B. Intravoxel Incoherent Motion (IVIM) in Evaluation of Breast Lesions: Comparison With Conventional DWI. Eur J Radiol (2013) 82:e782–9. doi: 10.1016/j.ejrad.2013.08.006
24. Wan Q, Deng YS, Zhou JX, Yu YD, Bao YY, Lei Q, et al. Intravoxel Incoherent Motion Diffusion-Weighted MR Imaging in Assessing and Characterizing Solitary Pulmonary Lesions. Sci Rep (2017) 7:43257. doi: 10.1038/srep43257
25. Yuan M, Zhang YD, Zhu C, Yu TF, Shi HB, Shi ZF, et al. Comparison of Intravoxel Incoherent Motion Diffusion-Weighted MR Imaging With Dynamic Contrast-Enhanced MRI for Differentiating Lung Cancer From Benign Solitary Pulmonary Lesions. J Magn Reson Imaging (2015) 43:669–79. doi: 10.1002/jmri.25018
Keywords: apparent diffusion coefficient, intravoxel incoherent motion, diffusion kurtosis imaging, magnetic resonance imaging, lung lesions
Citation: Zheng Y, Li J, Chen K, Zhang X, Sun H, Li S, Zhang X, Deng Z, Liang N and Li S (2022) Comparison of Conventional DWI, Intravoxel Incoherent Motion Imaging, and Diffusion Kurtosis Imaging in Differentiating Lung Lesions. Front. Oncol. 11:815967. doi: 10.3389/fonc.2021.815967
Received: 16 November 2021; Accepted: 22 December 2021;
Published: 20 January 2022.
Edited by:
Leonard Wee, Maastro Clinic, NetherlandsReviewed by:
Nguyen Minh Duc, Pham Ngoc Thach University of Medicine, VietnamGuolin Ma, China-Japan Friendship Hospital, China
Copyright © 2022 Zheng, Li, Chen, Zhang, Sun, Li, Zhang, Deng, Liang and Li. This is an open-access article distributed under the terms of the Creative Commons Attribution License (CC BY). The use, distribution or reproduction in other forums is permitted, provided the original author(s) and the copyright owner(s) are credited and that the original publication in this journal is cited, in accordance with accepted academic practice. No use, distribution or reproduction is permitted which does not comply with these terms.
*Correspondence: Na Liang, liangn2022@163.com; Shihong Li, summer_329@163.com