- 1Imaging Group, European Organisation of Research and Treatment in Cancer (EORTC), Brussels, Belgium
- 2European Society of Oncologic Imaging (ESOI), European Society of Radiology, Vienna, Austria
- 3Université de Paris, Assistance Publique–Hôpitaux de Paris (AP-HP), Hopital europeen Georges Pompidou, Department of Radiology, Paris Cardiovascular Research Center (PARCC) Unité Mixte de Recherche (UMRS) 970, Institut national de la santé et de la recherche médicale (INSERM), Paris, France
- 4Department of Radiology, Leiden University Medical Center, Leiden, Netherlands
- 5Biomedical Photonic Imaging Group, University of Twente, Enschede, Netherlands
- 6Department of Surgical Sciences, University of Turin, Turin, Italy
- 7Radiology Unit, Candiolo Cancer Institute, Fondazione del Piemonte per l’Oncologia-Istituto Di Ricovero e Cura a Carattere Scientifico (FPO-IRCCS), Turin, Italy
- 8Department of Radiology & Nuclear Medicine, Cancer Centre Amsterdam, Amsterdam University Medical Centers [Vrije Universiteit (VU) University], Amsterdam, Netherlands
- 9Medical Imaging Department, Mater Dei Hospital, University of Malta, Msida, Malta
- 10College of Science, University of Lincoln, Lincoln, United Kingdom
- 11Institute of Radiology, University Hospital Erlangen, Friedrich-Alexander-Universität Erlangen-Nürnberg (FAU), Erlangen, Germany
- 12Nuclear Medicine Unit, Istituto Di Ricovero e Cura a Carattere Scientifico (IRCCS) – Humanitas Research Hospital, Milan, Italy
- 13Department of Radiology, Institut de Recherche Expérimentale et Clinique (IREC), Cliniques Universitaires Saint Luc, Université Catholique de Louvain (UCLouvain), Brussels, Belgium
- 14Department of Radiology, Memorial Sloan Kettering Cancer Center, New York, NY, United States
- 15Department of Biomedical Imaging and Image-guided Therapy, Medical University of Vienna, Vienna, Austria
- 16Department of Radiology, University Hospital, Ludwig Maximilian University (LMU) Munich, Munich, Germany
- 17Department of Radiotherapy, University Medical Center Utrecht, Utrecht University, Utrecht, Netherlands
- 18Department of Medical-Surgical Sciences and Translational Medicine, Sapienza University of Rome, Rome, Italy
- 19Department of Radiology & Nuclear Medicine, Erasmus MC, University Medical Centre Rotterdam, Rotterdam, Netherlands
- 20Brain Tumour Centre, Erasmus Medical Centre (MC) Cancer Institute, Rotterdam, Netherlands
- 21Institute and Policlinic for Diagnostic and Interventional Radiology, University Hospital, Carl-Gustav-Carus Technical University Dresden, Dresden, Germany
- 22Department of Radiology, School of Medicine, National and Kapodistrian University of Athens, Areteion Hospital, Athens, Greece
- 23Department of Radiology, The Netherlands Cancer Institute, Amsterdam, Netherlands
- 24School For Oncology and Developmental Biology (GROW) School for Oncology and Developmental Biology, Maastricht University, Maastricht, Netherlands
- 25Diagnostic and Interventional Radiology, Department of Translational Research and of New Surgical and Medical Technologies, University of Pisa, Pisa, Italy
- 26Division of Radiotherapy and Imaging, The Institute of Cancer Research and Royal Marsden National Health Service (NHS) Foundation Trust, London, United Kingdom
- 27European Imaging Biomarkers Alliance (EIBALL), European Society of Radiology, Vienna, Austria
- 28Quantitative Imaging Biomarkers Alliance, Radiological Society of North America, Oak Brook, IL, United States
- 29Nuclear Medicine, University Hospitals Leuven, Leuven, Belgium
- 30Nuclear Medicine & Molecular Imaging, Department of Imaging and Pathology, Katholieke Universiteit (KU) Leuven, Leuven, Belgium
- 31Radiology Department, Hôpital Marie Lannelongue, Groupe Hospitalier Paris Saint Joseph Centre International des Cancers Thoraciques, Université Paris-Saclay, Le Plessis-Robinson, France
Response evaluation criteria in solid tumours (RECIST) v1.1 are currently the reference standard for evaluating efficacy of therapies in patients with solid tumours who are included in clinical trials, and they are widely used and accepted by regulatory agencies. This expert statement discusses the principles underlying RECIST, as well as their reproducibility and limitations. While the RECIST framework may not be perfect, the scientific bases for the anticancer drugs that have been approved using a RECIST-based surrogate endpoint remain valid. Importantly, changes in measurement have to meet thresholds defined by RECIST for response classification within thus partly circumventing the problems of measurement variability. The RECIST framework also applies to clinical patients in individual settings even though the relationship between tumour size changes and outcome from cohort studies is not necessarily translatable to individual cases. As reproducibility of RECIST measurements is impacted by reader experience, choice of target lesions and detection/interpretation of new lesions, it can result in patients changing response categories when measurements are near threshold values or if new lesions are missed or incorrectly interpreted. There are several situations where RECIST will fail to evaluate treatment-induced changes correctly; knowledge and understanding of these is crucial for correct interpretation. Also, some patterns of response/progression cannot be correctly documented by RECIST, particularly in relation to organ-site (e.g. bone without associated soft-tissue lesion) and treatment type (e.g. focal therapies). These require specialist reader experience and communication with oncologists to determine the actual impact of the therapy and best evaluation strategy. In such situations, alternative imaging markers for tumour response may be used but the sources of variability of individual imaging techniques need to be known and accounted for. Communication between imaging experts and oncologists regarding the level of confidence in a biomarker is essential for the correct interpretation of a biomarker and its application to clinical decision-making. Though measurement automation is desirable and potentially reduces the variability of results, associated technical difficulties must be overcome, and human adjudications may be required.
Introduction
Imaging plays a major role in the evaluation of tumour response to cancer treatments. It provides an objective in-vivo measurement of tumour burden, and helps oncologists determine whether a treatment should be pursued, interrupted or adapted.
Response evaluation criteria in solid tumours (RECIST) v1.1 currently is the reference standard for evaluating efficacy of therapies in patients with solid tumours who are included in clinical trials, and it is widely used and accepted by regulatory agencies (1). However, many publications question both the reproducibility and the clinical relevance of RECIST. This paper is an expert statement aiming to answer some of the questions regarding the principles underlying RECIST and its reproducibility compared to other biomarkers, as well as the limitations to its application and continued role in an era where other biomarkers exist that are more explicitly geared towards tumour-specific properties.
How Were RECIST Thresholds Established?
RECIST has instituted several overarching principles underpinning its approach to tumour response evaluation. Primarily, RECIST defines which lesions are measurable in a reliable manner. Among these, it defines a maximal number of lesions (‘target lesions’) to be measured to yield a quantitative value representative of tumour burden. The remainder are considered ‘non-target lesions’ and are evaluated qualitatively. On follow-up scans, new lesions indicate progression (Table 1). The threshold for response is defined as a decrease of at least 30% of sum of diameters (SOD) of target lesions compared to baseline, AND no progression of non-target lesions AND no new lesions. The threshold for progressive disease (PD) is defined as an increase of at least 20% of SOD of target lesions compared to nadir AND/OR unequivocal progression of non-target lesions AND/OR appearance of new lesions.
The first publication addressing thresholds for determining treatment efficacy was published by Moertel and Hanley in 1976 (2). In this study, 16 observers were asked to measure by clinical examination using a calliper the diameters of solid spheres of variable sizes arranged randomly underneath a mattress. Authors suggested the product of two diameters should be used, as this would be more reliable if lesions were not spherical. For this ‘clinical’ estimate, a 50% reduction in the product of two diameters was shown to have an acceptable measurement error estimated between 7-8%. Interestingly, the authors specifically stated that “the purpose was not to predict long-term efficacy but to determine what change in bidimensional size could be confidently considered a change”. Progression, on the other hand, was defined as an increase in the product of diameters ≥ 25%, but the authors could not justify this cut-off, other than by specifying it “should not necessarily be regarded as influencing the management of the patient”.
In 1979, the World Health Organization (WHO) provided recommendations for the evaluation of cancer treatments in clinical trials on imaging. Criteria were based not only on the bidimensional measurement of lesions on clinical examination, but also CT or standard radiography (3), transposing results of Moertel and Hanley’s study and setting cut-offs for definition of response to -50% and of progression to +25%. However, many technical aspects were not detailed, such as the number of lesions to be measured or what constituted a measurable lesion.
In 2000, a working group of European, American and Canadian cancer research organizations (EORTC, NCI, NCIC) defined the Response Evaluation Criteria In Solid Tumours – RECIST (4). They used data from over 4,600 patients enrolled in 14 clinical trials to formulate criteria based on imaging. RECIST used unidimensional measurement of lesions, justified by an extensive comparison of methods of measurement (1D vs. 2D) (5). Moreover, this working group specified conditions of measurement, number of lesions, and detailed how to document progression. Regarding cut-off values for response and progression, the -50% value for response for bidimensional measurement was altered to -30% for unidimensional measurements, and the +25% value for progression for bidimensional measurement was altered to +20% for unidimensional measurements (Table 2).
RECIST was then revised in 2009 (version 1.1) (1), introducing specific rules for measurement of small axis of lymph nodes and reducing the number of target lesions to five per patient. This new version was also based on data analysis, including a literature review and a simulation using a database of over 6,500 patients and 18,000 lesions. The number of target lesions for example, was chosen by determining the minimum number for which response rates and time to progression were not altered from RECIST 1.0 results (6, 7).
Statement #1
RECIST thresholds were chosen to produce a comparable classification of patients in a given category of response when comparing trials or even when comparing patients, taking into account tumour measurement variability.
Do RECIST Categories Predict Outcome?
RECIST criteria were originally tested and validated to provide an objective and reproducible assessment of treatment effect in cancer patients, without any references to patient outcome (8). Yet it seems intuitive that when a tumour decreases in size, a patient will have a better outcome, and vice versa. There is evidence to support this, including some large studies, which pool data from various trials. In over 500 patients with metastatic colorectal cancer treated with combination chemotherapy, a decrease in size resulted in a decreased hazard ratio for overall survival (OS) (9). In a meta-analysis of 24 phase I trials, a linear relationship was shown between change in tumour size and survival (10). In a pooled analysis of over 2,700 patients with metastatic renal cell carcinoma treated with anti-angiogenic agents, tumour shrinkage of ≥ 30% resulted in improved OS and progression-free survival (PFS) (11). In addition, the authors demonstrated that tumour shrinkage between 60% and 100% at 6-month follow-up represented an independent prognostic factor for OS. Litière et al. also demonstrated in an even larger pooled analysis of over 23,000 patients treated with targeted agents, chemotherapy or a combination thereof (12), that a decrease in tumour size was consistently associated with a lower hazard ratio, while an increase in size was associated with a higher hazard ratio.
Tumour response according to RECIST can only be quantified by a decrease in size or number of target lesions, as non-target lesions are not taken into consideration for partial response (PR). Regarding progression however, it is important to consider non-target lesions, as unequivocal progression of non-target lesions or emergence of new lesions defines tumour progression. In over 3,700 patients from 13 trials in the RECIST trial database, the presence of new lesions and progression of non-target lesions were most strongly associated with worse OS (hazard ratios range 1.5–2.3) regardless of tumour type, whereas percentage tumour growth in target lesions contributed less in a multivariate model of OS (13).
Finally, in two separate studies (14, 15), An et al. compared the predictive ability of RECIST categories vs. longitudinal tumour measurement–based continuous metrics and alternative categorical response metrics such as slope (absolute change in tumour size) and percent change (relative change in tumour size) to predict OS. The databases consisted respectively of almost 2,100 patients from 13 trials and over 1,500 patients from 3 trials with breast cancer, non–small cell lung cancer (NSCLC) or colorectal cancer. Although there seemed to be a slightly better performance for continuous variables, it was not statistically significant, which led the authors to conclude there was no evidence that growth rate or a continuous evaluation of percent change would improve prediction of outcome. However, it may be noted that timing of evaluations, particularly when considering non-continuous variables, may have an impact on their performance and results.
Statement #2
Tumour size changes correlate to outcome at a statistical (cohort) level.
How Reproducible Is RECIST?
When considering whether RECIST evaluates tumour response correctly, metrology principles guide us to consider two aspects (16): is the measurement “true” (when compared to a “real” value, which defines its accuracy), and is the measurement “precise” (i.e. repeatable and reproducible)?
Assessing accuracy of change in size measurements would require obtaining “true” values of change in size. As it is not possible to surgically excise all tumours for comparison with imaging, and often inaccurate to compare ex vivo with in vivo measurements, the true value of an imaging biomarker must be derived from data obtained through a combination of primary tumour excision and phantom studies.
Precision refers to the variability of the measurement process and can be evaluated by repeatability (when measurement conditions do not change) and reproducibility (when measurement conditions vary). The precision of RECIST and of response categories has been studied extensively. Table 3 lists the documented reproducibility of RECIST and factors that may impact it. Overall, SOD reproducibility is in the order of +/-20% in multi-observer studies, and +/-10% in single observer studies (17). Important factors associated with RECIST measurement reproducibility are the choice and number of target lesions (Figure 1) and the experience of the reader(s). Where multiple target lesions are used, their selection affects variability: agreement ranges from 0.58 when different targets are chosen to 0.97 when the same targets are used (23). Variability also increases with the number of target lesions selected. For this reason, it has been recommended that a central review in clinical trials should include two readers and one adjudicator (29). Finally, reader experience has major impact on variability, from the selection of the correct reference examination (baseline vs. previous CT) to the detection and proper interpretation of new lesions (20, 21, 25, 26). Measurements of well-demarcated lesions and bigger lesions are also more reproducible (17–19), which vindicates RECIST recommendations for the choice of target lesions.
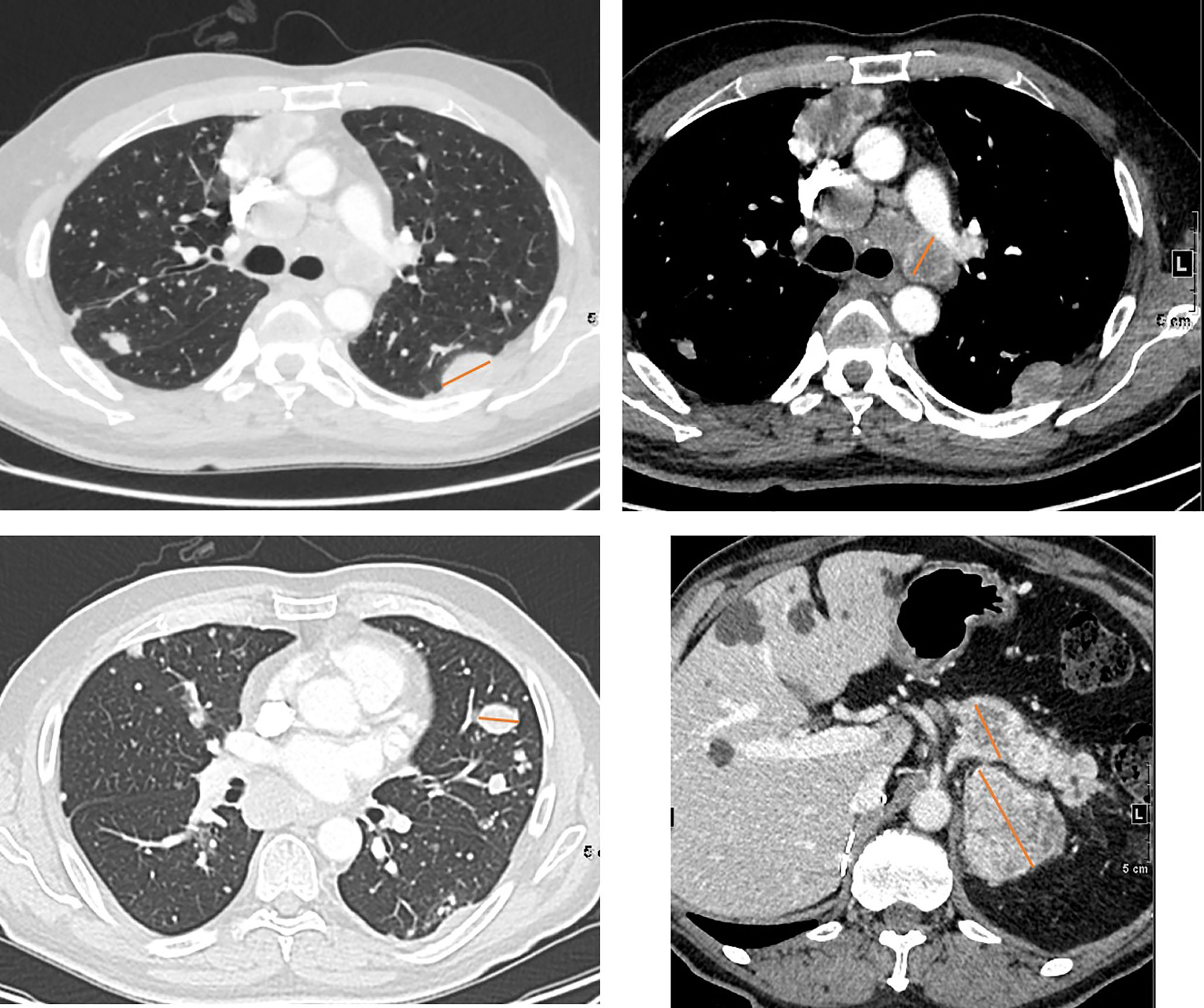
Figure 1 Selecting target lesions in a 58 yo patient with metastatic renal cell carcinoma. Multiple lung, lymph node, pancreatic and adrenal metastases are present. Lymph nodes should be sampled from different locations where possible. Selection of target lesions at baseline from multiple organ sites is important for response evaluation at a patient level.
Statement #3
RECIST reproducibility is impacted by reader experience, choice of target lesions, lesion characteristics, and detection/interpretation of new lesions. At an individual level, this can result in patients being categorised incorrectly when values of SOD are near thresholds or when new lesions are either missed or incorrectly interpreted.
How Reproducible Are Other Biomarkers?
Table 4 summarises repeatability and reproducibility of some of the other biomarkers suggested or used as alternatives to RECIST for evaluating response. With the abundance of suggested candidate biomarkers in the published literature, the purpose here is not to be comprehensive, but to give a general overview of some of the most frequently explored options for providing a level of comparison with RECIST.
A first alternative to measuring a single size dimension as a response biomarker, would be to measure volume of a single or several lesions as an indicator of tumour bulk. This seems particularly important when lesions are irregular in shape, or when they change orientation and are therefore not identically represented on standard axial follow-up scans. Volumetric response on first follow-up CT has been shown to better predict OS than RECIST response (65). Tumour volume response has been utilised in lung (66), cervical (67), and other solid malignancies (68). Despite a trend towards better intra- and inter-observer reproducibility, the routine use of volume has been hampered by the need for manual segmentation, which is user-dependent and time-consuming and does not improve the discrepancies linked to the choice of target lesions (24, 28). Aside from tumour bulk, metabolic activity of tumours through functional imaging (e.g. positron emission tomography - PET)) is highly predictive of response in lymphoma (69), lung cancer (70), and metastatic melanoma (71). Other radioligands are utilised for response or recurrence detection, e.g. 18F-fluoroestradiol (FES) in hormone-dependent breast cancer (72) and 18F- or 68Ga Prostate-Specific Membrane Antigen (PSMA) ligands in prostate cancer (73). Additionally, radiolabelled ligands of various metabolites and biologically active molecules can assess proliferation, hypoxia, angiogenesis, apoptosis and gene transfection (74).While parameters used for the quantification and measurement of tumour metabolism by PET are generally based on semi-quantitative assessments, these can be made relatively reproducible and harmonised throughout the world through standardised imaging protocols and dedicated initiatives promoted by the international scientific societies (75, 76), such as the accreditation program developed by the EANM Research Ltd. (EARL) (34, 77).
Other alternate imaging biomarkers include perfusion and diffusion imaging. As tumours are commonly characterised by neo-angiogenesis, perfusion and permeability derived from dynamic-contrast enhanced studies (e.g. with MR or CT) have been contenders for measuring early response (78), and vascularity can be quantified using most imaging techniques, such as MRI, CT, ultrasound and PET. The utility of biomarkers of vascularity has been demonstrated particularly where anti-angiogenic agents such as bevacizumab have been part of the therapeutic strategy (79). However, their quantitation, which depends on measuring or estimating an arterial input function, is susceptible to large potential variations (80), and the reproducibility of such data is often low, thus limiting their clinical utility (81). Another biomarker reflecting tissue cellularity, the apparent diffusion coefficient (ADC) from DW-MRI, has proven a robust quantitative measure with good repeatability and reproducibility across vendor platforms (82), and has the potential to detect therapeutic response earlier than size measurements. It is increasingly being introduced routinely into scanning protocols, as it does not require injection of an extrinsic contrast agent and is simple and fast to acquire and analyse. Increasing automation with artificial intelligence (AI) systems may aid the translation of biomarkers indicative of tumour characteristics other than bulk into routine clinical workflows. Unfortunately, tightened legal rules are slowing down the process of their adoption (83).
Although historically dependent on imaging, response assessment for malignancies may now also include liquid biopsies [quantification of circulating tumour cells or DNA (CTC, ctDNA)], as well as histological sampling. ctDNA shedding is influenced by the overall tumour burden (cells) and may thus inform the use of imaging in relation to likely tumour size (84), because ctDNA estimations require less workflow and infrastructure than repeated monitoring with imaging. Initial clinical evaluations showed that ctDNA detected response earlier than imaging-based assessment (85). The simplest clinical implementation of ctDNA may be in postoperative monitoring of disease recurrence (86) but even here reproducibility and standardisation issues remain limiting. In one study, ctDNA quantities based on measurement of some target genes (e.g. TERT) were, on average, more than two-fold higher than those of other assays (e.g. ERV3) (87). In another, quantities of cell-free DNA for the different isolation methods for detection of EGFR variants in NSCLC varied between medians of 1.6 ng/mL and 28.1 ng/mL (88). Moreover, concordance between tissue and plasma variant detection for leading platforms has been shown to range from 70% to 90% (89). Thus, ctDNA extraction/isolation methods (87, 88) may need to be standardised before routine clinical use.
Finally, histopathology may also be a method for tumour response evaluation. However, serial histological sampling is not routinely used for response assessment and has thus far shown agreement with imaging-based responses only in a few studies (90). Histopathological evaluation of response is performed usually after neoadjuvant therapy, when the organ is surgically resected. Qualitative or semi-quantitative histopathological evaluation also presents variable reproducibility according to organs, methods and published studies (91–94). Agreement between pathologists yielded kappa values ranging from 0.21 for extent in prostate cancer (92), to 0.49 for multiple well-trained observers in cervical cancer (93), 0.64 for a 5-point tumour regression grade in rectal cancer (90) and 0.83 for a central review in bladder cancer (91). As with macroscopic imaging, reader experience (94), and central review (92) improve reproducibility.
Statement #4:
Alternative biomarkers for tumour response yield reproducibility generally comparable to RECIST. Each technique has its sources of variability, and it is important to understand inherent variability and limitations of individual biomarkers. It is critical that imaging experts communicate their level of confidence in any chosen biomarker.
What Are Common RECIST Limitations?
Challenging Organs: Bone
Bone metastases were considered unmeasurable in the initial RECIST initiative, because of the lack of sensitivity of existing techniques to bone marrow infiltration (4). On CT it is the bone’s osteolytic or osteosclerotic reaction to the presence of tumour, or its response to therapy (flare lesions) that is visualised rather than the tumour itself (95, 96). With the updated RECIST 1.1. version, bone metastases with soft tissue masses ≥10 mm are recognized as measurable target lesions (1). Nevertheless, bone lesions without soft tissue involvement, whether lytic, mixed or sclerotic, remain unmeasurable by RECIST. Since the early 1990s, bone marrow MRI has been shown to be superior to bone scintigraphy and CT for the assessment of bone metastatic disease. Bone marrow replacement by neoplastic foci is detected and quantified on T1-weighted and fat-suppressed T2-weighted MRI sequences (97, 98), more recently complemented with diffusion-weighted imaging (DWI) sequences (99, 100). However, to date, RECIST 1.1 has not validated quantitative bone MRI for tumour response assessment. Positron Emission Tomography Response Criteria in Solid Tumours (PERCIST), introduced in 2009 (30, 101), enables response to be measured in 18F-fluorodeoxyglucose (18F-FDG) avid bone metastatic lesions based on their metabolic activity in the absence of any obvious anatomic changes. Finally, PSMA-PET appears promising for identifying bone marrow invasion due to prostate cancer, regardless of the impact on the bone mineral content (102, 103).
Challenging Diseases: GIST and mCRC
As RECIST is not organ-specific, it might not capture the key parameters that are associated with survival outcomes in certain cancer types, and under certain types of treatment. In gastrointestinal malignancies, the hepatic tumour burden and its response commonly outperform other sites of metastatic disease for survival prediction. A study in metastatic colorectal cancer (mCRC) showed that the depth and uniformity of response in liver metastases represented a highly useful and clinically relevant indicator for therapy monitoring (104). Organ-specific response patterns may also occur under immunotherapy possibly due to varying immune microenvironments in organs or the lymphatic system (105–107). Thus, choice of target lesions would largely impact the response observed according to the organ, as well as the predictive ability of RECIST. In this case also, reader experience and knowledge of the disease is crucial for proper target lesion selection.
Response to therapy in patients with advanced GIST was drastically improved by the introduction of imatinib, a tyrosine-kinase inhibitor. Imatinib treatment has been shown to induce necrosis with a marked decrease in vascularity of GIST lesions, resulting in a decrease in CT density often before any significant decrease in size is seen, thus leading to underestimation of the initial tumour response (108, 109) (Figure 2). A paradoxical increase in volume is occasionally observed, simulating progression (110). Choi et al. therefore proposed adapted criteria for GIST, combining changes in tumour density on contrast-enhanced CT expressed in Hounsfield units (HU) and/or size to determine tumour response (109): PR is defined as a decrease of ≥10% in the SOD or a decrease of ≥ 15% in tumour density of target lesions, whereas PD is defined as a ≥ 10% increase in size and not meeting the PR criteria by tumour density. PD may also occur if new intra-tumoural nodules are present or existing intra-tumoural nodules show an increase in size, factors which are not catered for in RECIST. In patients treated with imatinib, Choi criteria showed a significantly better correlation with survival rates than RECIST (111).
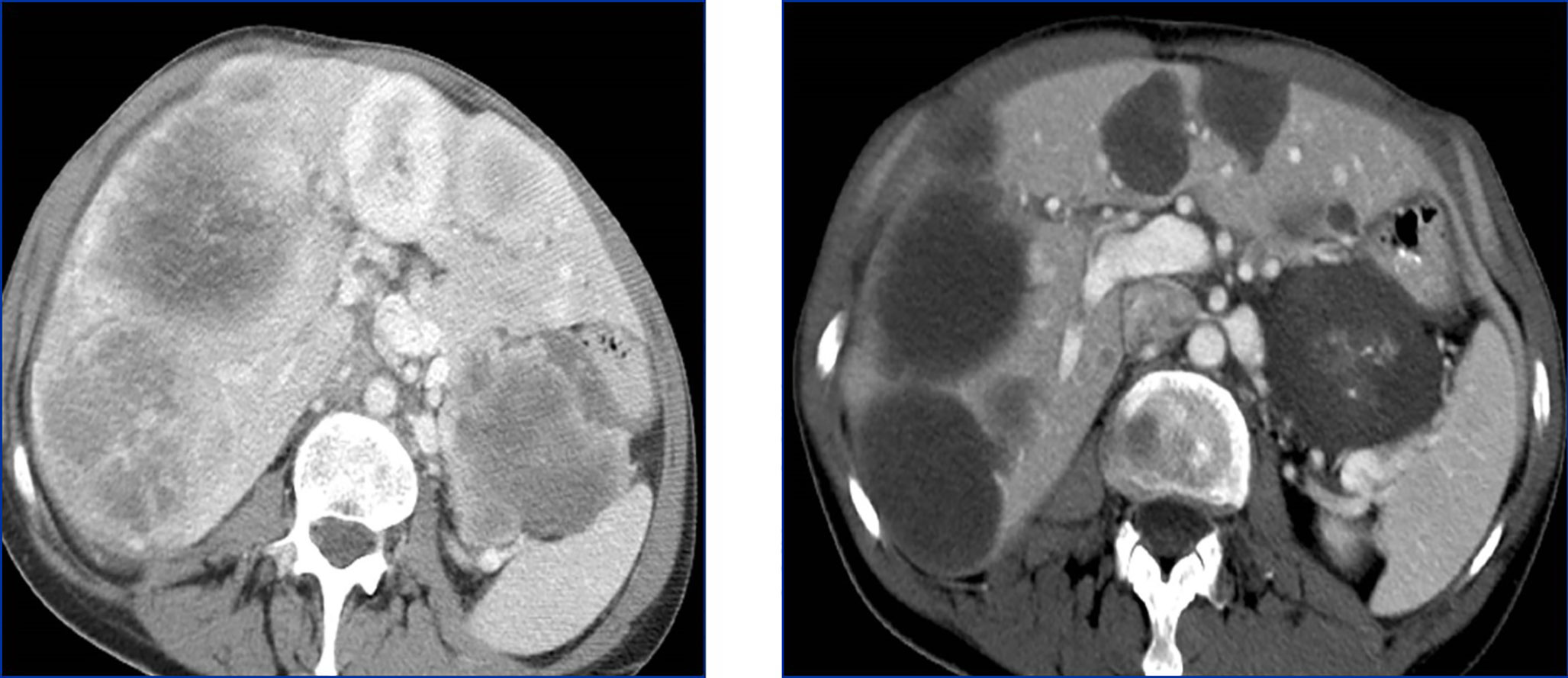
Figure 2 Response unrelated to tumour size in a 66 yo patient treated with imatinib for a gastrointestinal stromal tumour (GIST). Compared to the baseline image (left), after treatment (right) the tumour shows a dramatic decrease in density rather than in size.
Challenging Treatments: Focal Therapies
Treatment of tumour lesions with ablative therapies, such as radiofrequency ablation, microwave ablation or cryoablation, results in a larger defect than the original lesion and such treated lesions are not considered measurable unless there is progression at this site (1), such as the development of a new measurable nodule within the ablation defect. Distinguishing normal post-ablation changes from residual disease and recurrence can be challenging (112).
Intravascular therapies are also a challenge for the use of RECIST. Trans-arterial radioembolization (TARE) induces inflammatory changes with a generally delayed morphologic response (112). A reduction of 18F-FDG uptake on early PET-CT has been found to be helpful in predicting further outcome of these patients (113). As a consequence, both TARE and intra-arterial therapies such as trans-arterial chemoembolization (TACE) in hepatocarcinoma require modified RECIST (mRECIST) criteria derived from arterial and portal venous enhancement phases of CT or MRI (114), and which take into account both lesion size and vascularity.
High-intensity focused ultrasound (HIFU), under the guidance of ultrasound or MRI, has also been used as a non-invasive technique for tissue ablation in prostate cancer and more recently in recurrent gynecological malignancy (115). The use of HIFU for hepatic tumour lesions is still in the exploratory stage. As for other ablative therapies and for similar reasons (116), RECIST 1.1 appears to be unsuitable for local response evaluation following HIFU applied to liver lesions.
Finally, tumour lesions in a previously irradiated area (via CyberKnife, stereotactic radiotherapy or traditional fractionated radiation therapy) are not considered measurable (1) and must be excluded from RECIST evaluation due to the inflammatory or fibrotic changes that may be observed, thus making evaluation of size unreliable.
Statement #5
There are several scenarios in which RECIST criteria fail to evaluate treatment-induced changes correctly. Informed appreciation that RECIST criteria are not applicable to all tumour sites and situations is thus crucial for proper interpretation and again dependent on reader experience.
When Is RECIST Response Assessment Misleading?
Pseudo-Progression
During immunotherapy, RECIST may describe progression that can be misleading and is thus classified as “pseudo-progression”. In fact, in around 5 to 10% of patients with metastatic disease treated with check-point inhibitors, an initial increase of tumour burden has been observed, followed by actual response or long-term stabilisation of disease (117–119). This phenomenon relates to the mechanism of action of immunotherapy, which stimulates the immune response and initially induces inflammation and tumour swelling, thus delaying visible tumour shrinkage. For this reason, adaptations of RECIST criteria for assessing treatment response to immunotherapy (iRECIST) have been developed. The first ascertainment of progression by iRECIST is considered as “immune unconfirmed progressive disease”(or iUPD), and requires, if possible, a subsequent evaluation 4 to 8 weeks later in order to confirm true progression (120) (Figure 3).
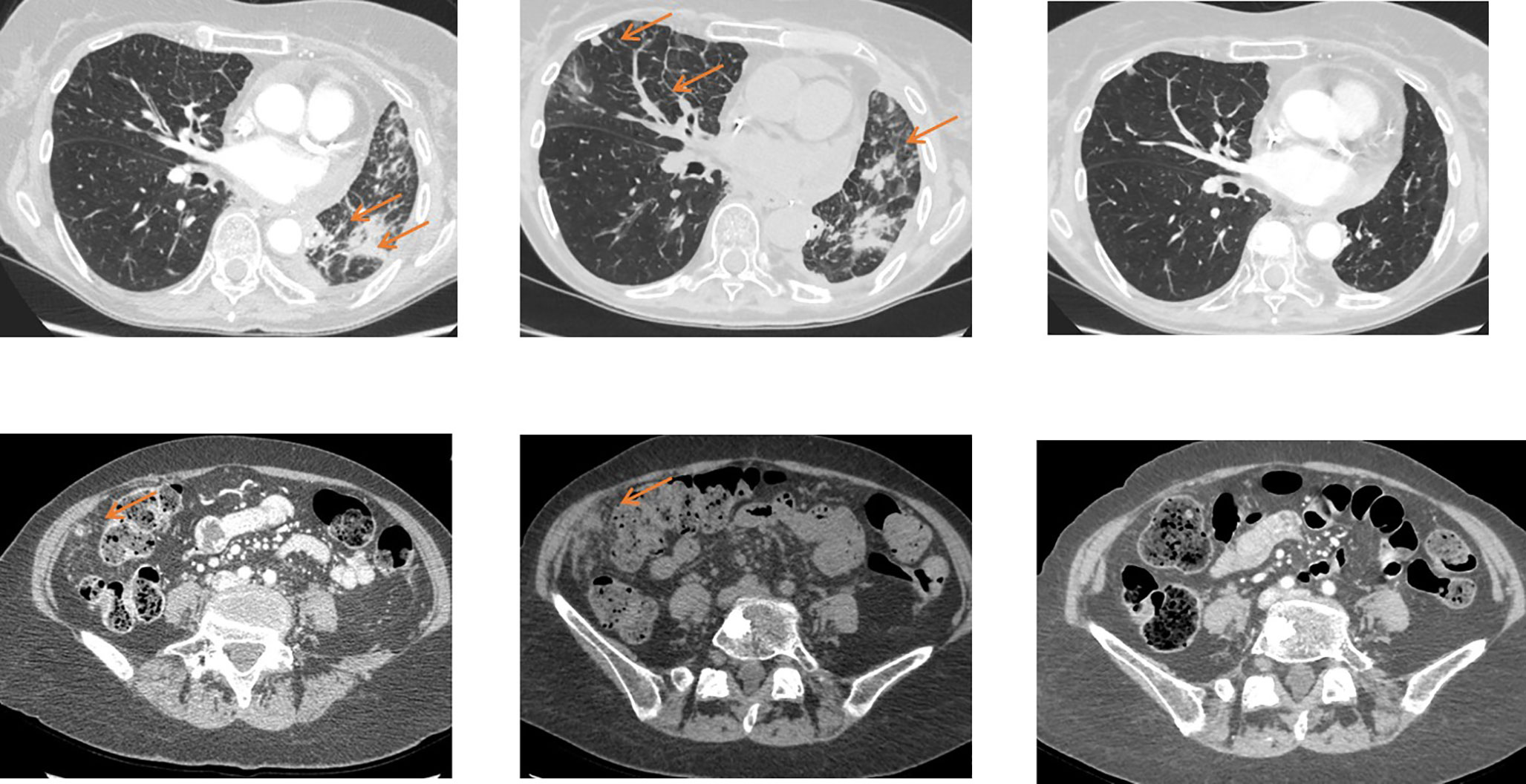
Figure 3 Pseudoprogression on immunotherapy in a 56 yo patient with metastatic non-small cell lung cancer. The baseline image (left) shows lung and peritoneal nodules (arrows). After 4 wks of antiPDL1 therapy (middle), CT shows an increase in previous lesions and the appearance of new lung nodules. Disease was considered immune unconfirmed progressive disease. Six weeks later (right) a dramatic response in all previous lesions was seen classifying the patients as a complete responder and endorsing an earlier diagnosis of pseudoprogression.
Mixed Response/Progression
In some patients, the tumour bulk does not respond homogeneously, with some lesions increasing and others decreasing. Mixed or heterogeneous response is defined as an increase in size of some tumour lesions and decrease of others in the same patient during treatment. This lesion-specific response has been attributed to the emergence of drug-resistant clones and indicates that tumour heterogeneity is likely causing treatment failure (121, 122). Mixed response has the same incidence in patients treated with targeted cancer agents and those undergoing chemotherapy alone or even combined with targeted agents (12, 28).
Since RECIST records overall patient response rather than individual lesion response, the choice of target lesions critically affects the objective assessment of overall patient response in patients with mixed response in individual lesions (Figure 4) (12). As lesions escaping treatment control will weigh negatively on patient prognosis (123), their presence should be annotated in order to offer the best alternative treatment for the patient.
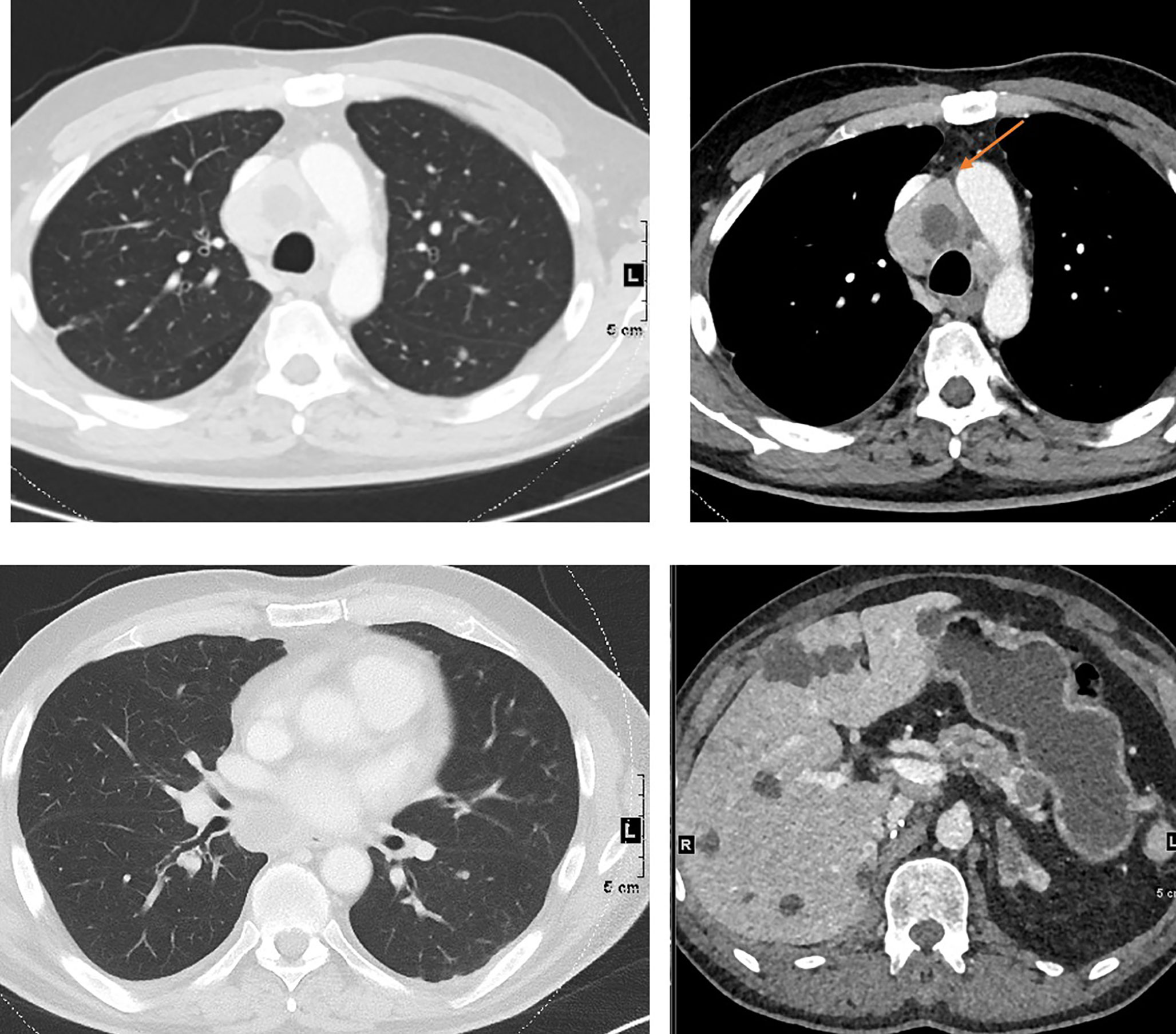
Figure 4 Mixed response to treatment in the same patient illustrated in Figure 1. Eight weeks after targeted therapy lung, adrenal and pancreatic metastases decreased, whereas one mediastinal lymph node (top right, arrow) increased.
Lesion cavitation, necrosis and residual non-viable masses represent other forms of response than decrease in size and may complicate RECIST assessment (124). Tumour necrosis with cavitation is present in approximately 14-24% of NSCLC patients undergoing anti-angiogenic drug therapy (125–127). When cavitation is present, lesion size may not change significantly and RECIST may therefore under-estimate the effect of therapy. Conversely, cavitation also risks missing progression if there is tumour regrowth inside the cavity. While alternative criteria have been proposed in such cases, e.g. subtracting the longest cavitation diameter from the largest lesion diameter (such as Crabb criteria) (126), these are not commonly used.
When residual tissue is present after therapy, evaluation with RECIST criteria is subject to pitfalls. First, an asymmetric shrinkage of the tumour may result in a similar longest diameter and consequent stable disease (SD) rating not reflecting the real response to treatment (Figure 2). Second, it may be difficult to distinguish between viable tumour and fibrosis. In such cases, best response assessment, an important endpoint in phase 2 studies (partial vs. complete response; PR vs. CR) may be affected (126). According to RECIST guidelines, in equivocal cases, residual lesions should be evaluated by either biopsy or PET(-CT) (Figure 2). This may well then allow upgrading PR to CR. However, false positive PET findings are not uncommon (128). Alternatively, other advanced imaging tests, such as DWI-MRI or perfusion imaging (e.g. from MR or CT) could be used.
Statement #6
Some patterns of response/progression cannot be correctly documented by RECIST. These require specialist reader experience and communication with oncologists to determine appropriate evaluation approaches and/or therapeutic options.
Should (Could) RECIST be Automated?
The core assumption of RECIST is that a single diameter on the cross-sectional imaging slice presenting the largest cross-section of a given lesion (or sum thereof) is a surrogate for tumour burden. This assumes that lesions are grossly spherical and that their size represents their overall activity. To streamline the determination of this single diameter and make it less subject to possible human-induced variability, semi- or fully-automated 2D or even 3D segmentation techniques can be applied to target lesions, which can also be semi- or fully-automatically tracked between scans acquired at different time points (129–134). The 2D or 3D mask resulting from the segmentation process then readily permits the automated and accurate extraction of the largest diameter from the segmented lesion. With 3D segmentation, the full volume of a target lesion can be provided alongside an automatically extracted largest diameter, which may not be oriented in the 2D plane of the source images in a broader RECIST interpretation, together with any other geometric metric of relevance. Using the largest 3D diameter would allow RECIST to be used beyond 2D constraints, and can account for non-orthogonal motion of target lesions between scans at different time-points. While segmentation and tracking can now plausibly be fully automated, especially with newer approaches using machine learning, and such capabilities are already implemented in several commercially available clinical systems, some challenges remain with key RECIST operations, such as the proper selection of target lesions and dealing with new or disappeared lesions. These are currently still best addressed or verified with a human (e.g. a radiologist) in the loop (20, 135).
Statement #7
Though automation is desirable to streamline the process and potentially reduce the variability of results within the RECIST paradigm, remaining technical challenges must be overcome to ensure proper repeatability, and human adjudication is still required.
RECIST in Novel Drug Development
RECIST measurements play a pivotal role in the development of novel oncological drugs (136). In most registered randomised controlled trials (RCTs), studies are powered to meet primary endpoints such as OS/PFS, which determines the number of patients recruited. A study of RCTs between 2006 and 2015 looking for evidence of clinical efficacy of novel oncology drugs in order to gain US Food and Drug Administration (FDA) approvals had PFS as primary endpoint in 28 out of 42 RCTs (66%), and OS in 14 (33%). In 2012, 12 novel anticancer drugs were approved by the FDA; only three drugs showed improvement of overall survival (137). Similarly, a study of drugs approved by the European Medicine Agency (EMA) between 2009 and 2013 also showed that only 18 of 68 (26%) novel drug uses were supported by OS data, whereas PFS was used in 31 (46%) (138). In the vast majority of trials, PFS is determined using the RECIST1.1 framework, or iRECIST for immune-oncology trials. It is acknowledged however, that in some disease types other criteria are used: e.g. Lugano criteria for 18F-FDG PET/CT or RECIL in lymphoma (139, 140) and RANO criteria for brain tumours (141, 142). The fact that PFS can predict OS outcome in large patients cohorts with commonly occurring cancers, reinforces the use of RECIST criteria in clinical trials (143). Moreover, rapid progress in drug development will make the reliance on OS as endpoint for novel drugs in oncology increasingly challenging because treatment options on progression on trial, including in-trial cross-over, are increasing.
Statement #8
Although the RECIST framework might not be perfect, the scientific basis for the anticancer drugs that have been approved using a RECIST-based surrogate endpoint remains valid.
RECIST: Only as Good as its Users?
RECIST criteria were developed for clinical trials and thresholds chosen to produce a comparable classification of patients, taking into account tumour measurement variability. These criteria are widely used in clinical trials and accepted by regulatory agencies. Despite some limitations, the scientific basis for the anticancer drugs that have been approved using a RECIST-based surrogate endpoint remains valid. Reader experience, choice of target lesions and detection of new lesions impact RECIST reproducibility, which necessitates adequate training of radiologists using these criteria. Automation is not currently sufficiently reliable to replace human experience. Unfortunately, some organ-, disease- or drug-specific patterns of response/progression cannot be correctly documented by RECIST.
This expert statement includes that RECIST remains a tool for radiologists that needs to be used with discrimination and good understanding of its purpose and limitations. Training of radiologists is essential to improve its application and reproducibility. RECIST conclusions should not go against common (or informed) sense. Furthermore, RECIST criteria have the advantage of simplicity, availability, cost-effectiveness, and intuitiveness. Overall, therefore, RECIST provides a common language between oncologists and imaging experts (e.g. radiologists), provided there is full understanding of how measurements are made, what they represent, and their inherent limitations.
Data Availability Statement
The original contributions presented in the study are included in the article/supplementary material, further inquiries can be directed to the corresponding author.
Author Contributions
All authors contributed to conception and design. CC and LF wrote the first draft of the manuscript. All authors contributed to manuscript revision, read, and approved the submitted version.
Conflict of Interest
The authors declare that the research was conducted in the absence of any commercial or financial relationships that could be construed as a potential conflict of interest.
Publisher’s Note
All claims expressed in this article are solely those of the authors and do not necessarily represent those of their affiliated organizations, or those of the publisher, the editors and the reviewers. Any product that may be evaluated in this article, or claim that may be made by its manufacturer, is not guaranteed or endorsed by the publisher.
Acknowledgments
We would like to thank the numerous colleagues for their insightful discussions and comments that have facilitated the work in this manuscript.
References
1. Eisenhauer EA, Therasse P, Bogaerts J, Schwartz LH, Sargent D, Ford R, et al. New Response Evaluation Criteria in Solid Tumours: Revised RECIST Guideline (Version 1.1). Eur J Cancer (2009) 45(2):228–47. doi: 10.1016/j.ejca.2008.10.026
2. Moertel CG, Hanley JA. The Effect of Measuring Error on the Results of Therapeutic Trials in Advanced Cancer. Cancer (1976) 38(1):388–94. doi: 10.1002/1097-0142(197607)38:1<388::AID-CNCR2820380156>3.0.CO;2-A
3. Miller AB, Hoogstraten B, Staquet M, Winkler A. Reporting Results of Cancer Treatment. Cancer (1981) 47(1):207–14. doi: 10.1002/1097-0142(19810101)47:1<207::AID-CNCR2820470134>3.0.CO;2-6
4. Therasse P, Arbuck SG, Eisenhauer EA, Wanders J, Kaplan RS, Rubinstein L, et al. New Guidelines to Evaluate the Response to Treatment in Solid Tumors. JNCI: J Natl Cancer Inst (2000) 92(3):205–16. doi: 10.1093/jnci/92.3.205
5. James K, Eisenhauer E, Christian M, Terenziani M, Vena D, Muldal A, et al. Measuring Response in Solid Tumors: Unidimensional Versus Bidimensional Measurement. JNCI: J Natl Cancer Inst (1999) 91(6):523–8. doi: 10.1093/jnci/91.6.523
6. Bogaerts J, Ford R, Sargent D, Schwartz LH, Rubinstein L, Lacombe D, et al. Individual Patient Data Analysis to Assess Modifications to the RECIST Criteria. Eur J Cancer (2009) 45(2):248–60. doi: 10.1016/j.ejca.2008.10.027
7. Moskowitz CS, Jia X, Schwartz LH, Gönen M. A Simulation Study to Evaluate the Impact of the Number of Lesions Measured on Response Assessment. Eur J Cancer (2009) 45(2):300–10. doi: 10.1016/j.ejca.2008.11.010
8. Booth CM, Eisenhauer EA. Progression-Free Survival: Meaningful or Simply Measurable? JCO (2012) 30(10):1030–3. doi: 10.1200/JCO.2011.38.7571
9. Suzuki C, Blomqvist L, Sundin A, Jacobsson H, Byström P, Berglund Å, et al. The Initial Change in Tumor Size Predicts Response and Survival in Patients With Metastatic Colorectal Cancer Treated With Combination Chemotherapy. Ann Oncol (2012) 23(4):948–54. doi: 10.1093/annonc/mdr350
10. Jain RK, Lee JJ, Ng C, Hong D, Gong J, Naing A, et al. Change in Tumor Size by RECIST Correlates Linearly With Overall Survival in Phase I Oncology Studies. J Clin Oncol (2012) 30(21):2684–90. doi: 10.1200/JCO.2011.36.4752
11. Grünwald V, McKay RR, Krajewski KM, Kalanovic D, Lin X, Perkins JJ, et al. Depth of Remission is a Prognostic Factor for Survival in Patients With Metastatic Renal Cell Carcinoma. Eur Urol (2015) 67(5):952–8. doi: 10.1016/j.eururo.2014.12.036
12. Litière S, Isaac G, De Vries EGE, Bogaerts J, Chen A, Dancey J, et al. RECIST 1.1 for Response Evaluation Apply Not Only to Chemotherapy-Treated Patients But Also to Targeted Cancer Agents: A Pooled Database Analysis. J Clin Oncol (2019) 37(13):1102–10. doi: 10.1200/JCO.18.01100
13. Litière S, de Vries EGE, Seymour L, Sargent D, Shankar L, Bogaerts J. The Components of Progression as Explanatory Variables for Overall Survival in the Response Evaluation Criteria in Solid Tumours 1.1 Database. Eur J Cancer (2014) 50(10):1847–53. doi: 10.1016/j.ejca.2014.03.014
14. An M-W, Mandrekar SJ, Branda ME, Hillman SL, Adjei AA, Pitot HC, et al. Comparison of Continuous Versus Categorical Tumor Measurement-Based Metrics to Predict Overall Survival in Cancer Treatment Trials. Clin Cancer Res (2011) 17(20):6592–9. doi: 10.1158/1078-0432.CCR-11-0822
15. An M-W, Dong X, Meyers J, Han Y, Grothey A, Bogaerts J, et al. Evaluating Continuous Tumor Measurement-Based Metrics as Phase II Endpoints for Predicting Overall Survival. J Natl Cancer Inst (2015) 107(11):djv239. doi: 10.1093/jnci/djv239
16. Sullivan DC, Obuchowski NA, Kessler LG, Raunig DL, Gatsonis C, Huang EP, et al. Metrology Standards for Quantitative Imaging Biomarkers. Radiol (2015) 277(3):813–25. doi: 10.1148/radiol.2015142202
17. Yoon SH, Kim KW, Goo JM, Kim D-W, Hahn S. Observer Variability in RECIST-Based Tumour Burden Measurements: A Meta-Analysis. Eur J Cancer (2016) 53:5–15. doi: 10.1016/j.ejca.2015.10.014
18. Oxnard GR, Zhao B, Sima CS, Ginsberg MS, James LP, Lefkowitz RA, et al. Variability of Lung Tumor Measurements on Repeat Computed Tomography Scans Taken Within 15 Minutes. J Clin Oncol (2011) 29(23):3114–9. doi: 10.1200/JCO.2010.33.7071
19. McErlean A, Panicek DM, Zabor EC, Moskowitz CS, Bitar R, Motzer RJ, et al. Intra- and Interobserver Variability in CT Measurements in Oncology. Radiol (2013) 269(2):451–9. doi: 10.1148/radiol.13122665
20. Beaumont H, Evans TL, Klifa C, Guermazi A, Hong SR, Chadjaa M, et al. Discrepancies of Assessments in a RECIST 1.1 Phase II Clinical Trial - Association Between Adjudication Rate and Variability in Images and Tumors Selection. Cancer Imaging (2018) 18(1):50. doi: 10.1186/s40644-018-0186-0
21. Bellomi M, De Piano F, Ancona E, Lodigiani AF, Curigliano G, Raimondi S, et al. Evaluation of Inter-Observer Variability According to RECIST 1.1 and Its Influence on Response Classification in CT Measurement of Liver Metastases. Eur J Radiol (2017) 95:96–101. doi: 10.1016/j.ejrad.2017.08.001
22. Jacene HA, Leboulleux S, Baba S, Chatzifotiadis D, Goudarzi B, Teytelbaum O, et al. Assessment of Interobserver Reproducibility in Quantitative 18F-FDG PET and CT Measurements of Tumor Response to Therapy. J Nucl Med (2009) 50(11):1760–9. doi: 10.2967/jnumed.109.063321
23. Kuhl CK, Alparslan Y, Schmoee J, Sequeira B, Keulers A, Brümmendorf TH, et al. Validity of RECIST Version 1.1 for Response Assessment in Metastatic Cancer: A Prospective, Multireader Study. Radiol (2019) 290(2):349–56. doi: 10.1148/radiol.2018180648
24. Keil S, Barabasch A, Dirrichs T, Bruners P, Hansen NL, Bieling HB, et al. Target Lesion Selection: An Important Factor Causing Variability of Response Classification in the Response Evaluation Criteria for Solid Tumors 1.1. Invest Radiol (2014) 49(8):509–17. doi: 10.1097/RLI.0000000000000048
25. Karmakar A, Kumtakar A, Sehgal H, Kumar S, Kalyanpur A. Interobserver Variation in Response Evaluation Criteria in Solid Tumors 1.1. Acad Radiol (2019) 26(4):489–501. doi: 10.1016/j.acra.2018.05.017
26. Suzuki C, Torkzad MR, Jacobsson H, Aström G, Sundin A, Hatschek T, et al. Interobserver and Intraobserver Variability in the Response Evaluation of Cancer Therapy According to RECIST and WHO-Criteria. Acta Oncol (2010) 49(4):509–14. doi: 10.3109/02841861003705794
27. Rothe JH, Grieser C, Lehmkuhl L, Schnapauff D, Fernandez CP, Maurer MH, et al. Size Determination and Response Assessment of Liver Metastases With Computed Tomography–Comparison of RECIST and Volumetric Algorithms. Eur J Radiol (2013) 82(11):1831–9. doi: 10.1016/j.ejrad.2012.05.018
28. Zimmermann M, Kuhl CK, Engelke H, Bettermann G, Keil S. Factors That Drive Heterogeneity of Response-To-Treatment of Different Metastatic Deposits Within the Same Patients as Measured by RECIST 1.1 Analyses. Acad Radiol (2020) 28(8):e235–9. doi: 10.1016/j.acra.2020.05.029
29. Ford R, Schwartz L, Dancey J, Dodd LE, Eisenhauer EA, Gwyther S, et al. Lessons Learned From Independent Central Review. Eur J Cancer (2009) 45(2):268–74. doi: 10.1016/j.ejca.2008.10.031
30. Wahl RL, Jacene H, Kasamon Y, Lodge MA. From RECIST to PERCIST: Evolving Considerations for PET Response Criteria in Solid Tumors. J Nucl Med (2009) 50 Suppl 1:122S–50S. doi: 10.2967/jnumed.108.057307
31. Young H, Baum R, Cremerius U, Herholz K, Hoekstra O, Lammertsma AA, et al. Measurement of Clinical and Subclinical Tumour Response Using [18F]-Fluorodeoxyglucose and Positron Emission Tomography: Review and 1999 EORTC Recommendations. European Organization for Research and Treatment of Cancer (EORTC) PET Study Group. Eur J Cancer (1999) 35(13):1773–82. doi: 10.1016/S0959-8049(99)00229-4
32. de Langen AJ, Vincent A, Velasquez LM, van Tinteren H, Boellaard R, Shankar LK, et al. Repeatability of 18F-FDG Uptake Measurements in Tumors: A Metaanalysis. J Nucl Med (2012) 53(5):701–8. doi: 10.2967/jnumed.111.095299
33. Burger IA, Huser DM, Burger C, von Schulthess GK, Buck A. Repeatability of FDG Quantification in Tumor Imaging: Averaged SUVs Are Superior to SUVmax. Nucl Med Biol (2012) 39(5):666–70. doi: 10.1016/j.nucmedbio.2011.11.002
34. Aide N, Lasnon C, Veit-Haibach P, Sera T, Sattler B, Boellaard R. EANM/EARL Harmonization Strategies in PET Quantification: From Daily Practice to Multicentre Oncological Studies. Eur J Nucl Med Mol Imaging (2017) 44(Suppl 1):17–31. doi: 10.1007/s00259-017-3740-2
35. Kurland BF, Peterson LM, Shields AT, Lee JH, Byrd DW, Novakova-Jiresova A, et al. Test-Retest Reproducibility of 18F-FDG PET/CT Uptake in Cancer Patients Within a Qualified and Calibrated Local Network. J Nucl Med (2019) 60(5):608–14. doi: 10.2967/jnumed.118.209544
36. Brendle C, Kupferschläger J, Nikolaou K, la Fougère C, Gatidis S, Pfannenberg C. Is the Standard Uptake Value (SUV) Appropriate for Quantification in Clinical PET Imaging? - Variability Induced by Different SUV Measurements and Varying Reconstruction Methods. Eur J Radiol (2015) 84(1):158–62. doi: 10.1016/j.ejrad.2014.10.018
37. Miyashita K, Takahashi N, Oka T, Asakawa S, Lee J, Shizukuishi K, et al. SUV Correction for Injection Errors in FDG-PET Examination. Ann Nucl Med (2007) D21(10):607–13. doi: 10.1007/s12149-007-0068-1
38. Boellaard R, Oyen WJG, Hoekstra CJ, Hoekstra OS, Visser EP, Willemsen AT, et al. The Netherlands Protocol for Standardisation and Quantification of FDG Whole Body PET Studies in Multi-Centre Trials. Eur J Nucl Med Mol Imaging (2008) 35(12):2320–33. doi: 10.1007/s00259-008-0874-2
39. Zhuang M, García DV, Kramer GM, Frings V, Smit EF, Dierckx R, et al. Variability and Repeatability of Quantitative Uptake Metrics in 18F-FDG PET/CT of Non-Small Cell Lung Cancer: Impact of Segmentation Method, Uptake Interval, and Reconstruction Protocol. J Nucl Med (2019) 60(5):600–7. doi: 10.2967/jnumed.118.216028
40. Laffon E, de Clermont H, Marthan R. A Method of Adjusting SUV for Injection-Acquisition Time Differences in (18)F-FDG PET Imaging. Eur Radiol (2011) 21(11):2417–24. doi: 10.1007/s00330-011-2204-5
41. Kamimura K, Nagamachi S, Wakamatsu H, Higashi R, Ogita M, Ueno S, et al. Associations Between Liver (18)F Fluoro-2-Deoxy-D-Glucose Accumulation and Various Clinical Parameters in a Japanese Population: Influence of the Metabolic Syndrome. Ann Nucl Med (2010) 24(3):157–61. doi: 10.1007/s12149-009-0338-1
42. Krak NC, Boellaard R, Hoekstra OS, Twisk JWR, Hoekstra CJ, Lammertsma AA. Effects of ROI Definition and Reconstruction Method on Quantitative Outcome and Applicability in a Response Monitoring Trial. Eur J Nucl Med Mol Imaging (2005) 32(3):294–301. doi: 10.1007/s00259-004-1566-1
43. Lasnon C, Quak E, Le Roux P-Y, Robin P, Hofman MS, Bourhis D, et al. EORTC PET Response Criteria Are More Influenced by Reconstruction Inconsistencies Than PERCIST But Both Benefit From the EARL Harmonization Program. EJNMMI Phys (2017) 4(1):17. doi: 10.1186/s40658-017-0185-4
44. Akamatsu G, Ikari Y, Nishida H, Nishio T, Ohnishi A, Maebatake A, et al. Influence of Statistical Fluctuation on Reproducibility and Accuracy of SUVmax and SUVpeak: A Phantom Study. J Nucl Med Technol (2015) 43(3):222–6. doi: 10.2967/jnmt.115.161745
45. Paquet N, Albert A, Foidart J, Hustinx R. Within-Patient Variability of (18)F-FDG: Standardized Uptake Values in Normal Tissues. J Nucl Med (2004) 45(5):784–8.
46. JH O, Lim SJ, Wang H, Leal JP, Shu H-KG, Wahl RL, et al. Quantitation of Cancer Treatment Response by 2-[18F]FDG PET/CT: Multi-Center Assessment of Measurement Variability Using AUTO-PERCISTTM. EJNMMI Res (2021) 11(1):15. doi: 10.1186/s13550-021-00754-1
47. Apostolova I, Wiemker R, Paulus T, Kabus S, Dreilich T, van den Hoff J, et al. Combined Correction of Recovery Effect and Motion Blur for SUV Quantification of Solitary Pulmonary Nodules in FDG PET/Ct. Eur Radiol (2010) 20(8):1868–77. doi: 10.1007/s00330-010-1747-1
48. Lodge MA, Chaudhry MA, Wahl RL. Noise Considerations for PET Quantification Using Maximum and Peak Standardized Uptake Value. J Nucl Med (2012) 53(7):1041–7. doi: 10.2967/jnumed.111.101733
49. Adams MC, Turkington TG, Wilson JM, Wong TZ. A Systematic Review of the Factors Affecting Accuracy of SUV Measurements. AJR Am J Roentgenol (2010) 195(2):310–20. doi: 10.2214/AJR.10.4923
50. Quak E, Le Roux P-Y, Hofman MS, Robin P, Bourhis D, Callahan J, et al. Harmonizing FDG PET Quantification While Maintaining Optimal Lesion Detection: Prospective Multicentre Validation in 517 Oncology Patients. Eur J Nucl Med Mol Imaging (2015) 42(13):2072–82. doi: 10.1007/s00259-015-3128-0
51. Gürses B, Altınmakas E, Böge M, Aygün MS, Bayram O, Balık E. Multiparametric MRI of Rectal Cancer-Repeatability of Quantitative Data: A Feasibility Study. Diagn Interv Radiol (2020) 26(2):87–94. doi: 10.5152/dir.2019.19127
52. Lundsgaard Hansen M, Fallentin E, Axelsen T, Lauridsen C, Norling R, Svendsen LB, et al. Interobserver and Intraobserver Reproducibility With Volume Dynamic Contrast Enhanced Computed Tomography (DCE-CT) in Gastroesophageal Junction Cancer. Diagn (Basel) (2016) 6(1):E8. doi: 10.3390/diagnostics6010008
53. Conte GM, Castellano A, Altabella L, Iadanza A, Cadioli M, Falini A, et al. Reproducibility of Dynamic Contrast-Enhanced MRI and Dynamic Susceptibility Contrast MRI in the Study of Brain Gliomas: A Comparison of Data Obtained Using Different Commercial Software. Radiol Med (2017) 122(4):294–302. doi: 10.1007/s11547-016-0720-8
54. Aronhime S, Calcagno C, Jajamovich GH, Dyvorne HA, Robson P, Dieterich D, et al. DCE-MRI of the Liver: Effect of Linear and Nonlinear Conversions on Hepatic Perfusion Quantification and Reproducibility. J Magn Reson Imaging (2014) 40(1):90–8. doi: 10.1002/jmri.24341
55. Smits M, Bendszus M, Collette S, Postma LA, Dhermain F, Hagenbeek RE, et al. Repeatability and Reproducibility of Relative Cerebral Blood Volume Measurement of Recurrent Glioma in a Multicentre Trial Setting. Eur J Cancer (2019) 114:89–96. doi: 10.1016/j.ejca.2019.03.007
56. Ng CS, Waterton JC, Kundra V, Brammer D, Ravoori M, Han L, et al. Reproducibility and Comparison of DCE-MRI and DCE-CT Perfusion Parameters in a Rat Tumor Model. Technol Cancer Res Treat (2012) 11(3):279–88. doi: 10.7785/tcrt.2012.500296
57. Newitt DC, Zhang Z, Gibbs JE, Partridge SC, Chenevert TL, Rosen MA, et al. Test-Retest Repeatability and Reproducibility of ADC Measures by Breast DWI: Results From the ACRIN 6698 Trial. J Magn Reson Imaging (2019) 49(6):1617–28. doi: 10.1002/jmri.26539
58. Kwee RM, Dik AK, Sosef MN, Berendsen RCM, Sassen S, Lammering G, et al. Interobserver Reproducibility of Diffusion-Weighted MRI in Monitoring Tumor Response to Neoadjuvant Therapy in Esophageal Cancer. PLoS One (2014) 9(4):e92211. doi: 10.1371/journal.pone.0092211
59. Weller A, Papoutsaki MV, Waterton JC, Chiti A, Stroobants S, Kuijer J, et al. Diffusion-Weighted (DW) MRI in Lung Cancers: ADC Test-Retest Repeatability. Eur Radiol (2017) 27(11):4552–62. doi: 10.1007/s00330-017-4828-6
60. Koh D-M, Blackledge M, Collins DJ, Padhani AR, Wallace T, Wilton B, et al. Reproducibility and Changes in the Apparent Diffusion Coefficients of Solid Tumours Treated With Combretastatin A4 Phosphate and Bevacizumab in a Two-Centre Phase I Clinical Trial. Eur Radiol (2009) 19(11):2728–38. doi: 10.1007/s00330-009-1469-4
61. Sorace AG, Elkassem AA, Galgano SJ, Lapi SE, Larimer BM, Partridge SC, et al. Imaging for Response Assessment in Cancer Clinical Trials. Semin Nucl Med (2020) 50(6):488–504. doi: 10.1053/j.semnuclmed.2020.05.001
62. Galbán CJ, Ma B, Malyarenko D, Pickles MD, Heist K, Henry NL, et al. Multi-Site Clinical Evaluation of DW-MRI as a Treatment Response Metric for Breast Cancer Patients Undergoing Neoadjuvant Chemotherapy. PLoS One (2015) 10(3):e0122151. doi: 10.1371/journal.pone.0122151
63. Newitt DC, Tan ET, Wilmes LJ, Chenevert TL, Kornak J, Marinelli L, et al. Gradient Nonlinearity Correction to Improve Apparent Diffusion Coefficient Accuracy and Standardization in the American College of Radiology Imaging Network 6698 Breast Cancer Trial. J Magn Reson Imaging (2015) 42(4):908–19. doi: 10.1002/jmri.24883
64. Newitt DC, Amouzandeh G, Partridge SC, Marques HS, Herman BA, Ross BD, et al. Repeatability and Reproducibility of ADC Histogram Metrics From the ACRIN 6698 Breast Cancer Therapy Response Trial. Tomogr (2020) 6(2):177–85. doi: 10.18383/j.tom.2020.00008
65. Hayes SA, Pietanza MC, O’Driscoll D, Zheng J, Moskowitz CS, Kris MG, et al. Comparison of CT Volumetric Measurement With RECIST Response in Patients With Lung Cancer. Eur J Radiol (2016) 85(3):524–33. doi: 10.1016/j.ejrad.2015.12.019
66. Lee JH, Lee HY, Ahn M-J, Park K, Ahn JS, Sun J-M, et al. Volume-Based Growth Tumor Kinetics as a Prognostic Biomarker for Patients With EGFR Mutant Lung Adenocarcinoma Undergoing EGFR Tyrosine Kinase Inhibitor Therapy: A Case Control Study. Cancer Imaging (2016) 1616:5. doi: 10.1186/s40644-016-0063-7
67. deSouza NM, Soutter WP, Rustin G, Mahon MM, Jones B, Dina R, et al. Use of Neoadjuvant Chemotherapy Prior to Radical Hysterectomy in Cervical Cancer: Monitoring Tumour Shrinkage and Molecular Profile on Magnetic Resonance and Assessment of 3-Year Outcome. Br J Cancer (2004) 90(12):2326–31. doi: 10.1038/sj.bjc.6601870
68. Fenerty KE, Folio LR, Patronas NJ, Marté JL, Gulley JL, Heery CR. Predicting Clinical Outcomes in Chordoma Patients Receiving Immunotherapy: A Comparison Between Volumetric Segmentation and RECIST. BMC Cancer (2016) 16(1):672. doi: 10.1186/s12885-016-2699-x
69. Schmitz C, Hüttmann A, Müller SP, Hanoun M, Boellaard R, Brinkmann M, et al. Dynamic Risk Assessment Based on Positron Emission Tomography Scanning in Diffuse Large B-Cell Lymphoma: Post-Hoc Analysis From the PETAL Trial. Eur J Cancer (2020) 124:25–36. doi: 10.1016/j.ejca.2019.09.027
70. Mac Manus MP, Hicks RJ, Matthews JP, Wirth A, Rischin D, Ball DL. Metabolic (FDG-PET) Response After Radical Radiotherapy/Chemoradiotherapy for Non-Small Cell Lung Cancer Correlates With Patterns of Failure. Lung Cancer (2005) 49(1):95–108. doi: 10.1016/j.lungcan.2004.11.024
71. Sachpekidis C, Anwar H, Winkler J, Kopp-Schneider A, Larribere L, Haberkorn U, et al. The Role of Interim 18F-FDG PET/CT in Prediction of Response to Ipilimumab Treatment in Metastatic Melanoma. Eur J Nucl Med Mol Imaging (2018) 45(8):1289–96. doi: 10.1007/s00259-018-3972-9
72. Evangelista L, Guarneri V, Conte PF. 18f-Fluoroestradiol Positron Emission Tomography in Breast Cancer Patients: Systematic Review of the Literature & Meta-Analysis. Curr Radiopharm (2016) 9(3):244–57. doi: 10.2174/1874471009666161019144950
73. Tan N, Oyoyo U, Bavadian N, Ferguson N, Mukkamala A, Calais J, et al. PSMA-Targeted Radiotracers Versus 18F Fluciclovine for the Detection of Prostate Cancer Biochemical Recurrence After Definitive Therapy: A Systematic Review and Meta-Analysis. Radiol (2020) 296(1):44–55. doi: 10.1148/radiol.2020191689
74. Wester H-J. Nuclear Imaging Probes: From Bench to Bedside. Clin Cancer Res (2007) 13(12):3470–81. doi: 10.1158/1078-0432.CCR-07-0264
75. Boellaard R, O’Doherty MJ, Weber WA, Mottaghy FM, Lonsdale MN, Stroobants SG, et al. FDG PET and PET/CT: EANM Procedure Guidelines for Tumour PET Imaging: Version 1.0. Eur J Nucl Med Mol Imaging (2010) 37(1):181–200. doi: 10.1007/s00259-009-1297-4
76. Graham MM, Wahl RL, Hoffman JM, Yap JT, Sunderland JJ, Boellaard R, et al. Summary of the UPICT Protocol for 18F-FDG PET/CT Imaging in Oncology Clinical Trials. J Nucl Med (2015) 56(6):955–61. doi: 10.2967/jnumed.115.158402
77. Makris NE, Huisman MC, Kinahan PE, Lammertsma AA, Boellaard R. Evaluation of Strategies Towards Harmonization of FDG PET/CT Studies in Multicentre Trials: Comparison of Scanner Validation Phantoms and Data Analysis Procedures. Eur J Nucl Med Mol Imaging (2013) 40(10):1507–15. doi: 10.1007/s00259-013-2465-0
78. Jun W, Cong W, Xianxin X, Daqing J. Meta-Analysis of Quantitative Dynamic Contrast-Enhanced MRI for the Assessment of Neoadjuvant Chemotherapy in Breast Cancer. Am Surg (2019) 85(6):645–53. doi: 10.1177/000313481908500630
79. Hirashima Y, Yamada Y, Tateishi U, Kato K, Miyake M, Horita Y, et al. Pharmacokinetic Parameters From 3-Tesla DCE-MRI as Surrogate Biomarkers of Antitumor Effects of Bevacizumab Plus FOLFIRI in Colorectal Cancer With Liver Metastasis. Int J Cancer (2012) 130(10):2359–65. doi: 10.1002/ijc.26282
80. Rata M, Collins DJ, Darcy J, Messiou C, Tunariu N, Desouza N, et al. Assessment of Repeatability and Treatment Response in Early Phase Clinical Trials Using DCE-MRI: Comparison of Parametric Analysis Using MR- and CT-Derived Arterial Input Functions. Eur Radiol (2016) 26(7):1991–8. doi: 10.1007/s00330-015-4012-9
81. Shukla-Dave A, Obuchowski NA, Chenevert TL, Jambawalikar S, Schwartz LH, Malyarenko D, et al. Quantitative Imaging Biomarkers Alliance (QIBA) Recommendations for Improved Precision of DWI and DCE-MRI Derived Biomarkers in Multicenter Oncology Trials. J Magn Reson Imaging (2019) 49(7):e101–21. doi: 10.1002/jmri.26518
82. Winfield JM, Tunariu N, Rata M, Miyazaki K, Jerome NP, Germuska M, et al. Extracranial Soft-Tissue Tumors: Repeatability of Apparent Diffusion Coefficient Estimates From Diffusion-Weighted MR Imaging. Radiol (2017) 284(1):88–99. doi: 10.1148/radiol.2017161965
83. FDA: Food and Drug Agency. Health C for D and R. Artificial Intelligence and Machine Learning in Software as a Medical Device. FDA (2021). Available at: https://www.fda.gov/medical-devices/software-medical-device-samd/artificial-intelligence-and-machine-learning-software-medical-device.
84. Avanzini S, Kurtz DM, Chabon JJ, Moding EJ, Hori SS, Gambhir SS, et al. A Mathematical Model of ctDNA Shedding Predicts Tumor Detection Size. Sci Adv (2020) 6(50):eabc4308. doi: 10.1126/sciadv.abc4308
85. Nabet BY, Esfahani MS, Moding EJ, Hamilton EG, Chabon JJ, Rizvi H, et al. Noninvasive Early Identification of Therapeutic Benefit From Immune Checkpoint Inhibition. Cell (2020) 183(2):363–76. doi: 10.1016/j.cell.2020.09.001
86. Dasari A, Morris VK, Allegra CJ, Atreya C, Benson AB, Boland P, et al. ctDNA Applications and Integration in Colorectal Cancer: An NCI Colon and Rectal-Anal Task Forces Whitepaper. Nat Rev Clin Oncol (2020) 17(12):757–70. doi: 10.1038/s41571-020-0392-0
87. Devonshire AS, Whale AS, Gutteridge A, Jones G, Cowen S, Foy CA, et al. Towards Standardisation of Cell-Free DNA Measurement in Plasma: Controls for Extraction Efficiency, Fragment Size Bias and Quantification. Anal Bioanal Chem (2010) 406(26):6499–512. doi: 10.1007/s00216-014-7835-3
88. Fleischhacker M, Schmidt B, Weickmann S, Fersching DM, Leszinski GS, Siegele B, et al. Methods for Isolation of Cell-Free Plasma DNA Strongly Affect DNA Yield. Clin Chim Acta (2011) 412(23‐24):2085–8. doi: 10.1016/j.cca.2011.07.011
89. Merker JD, Oxnard GR, Compton C, Diehn M, Hurley P, Lazar AJ, et al. Circulating Tumor DNA Analysis in Patients With Cancer: American Society of Clinical Oncology and College of American Pathologists Joint Review. J Clin Oncol (2018) 36(16):1631–41. doi: 10.1200/JCO.2017.76.8671
90. Tapia C, Aung PP, Roy-Chowdhuri S, Xu M, Ouyang F, Alshawa A, et al. Decrease in Tumor Content Assessed in Biopsies is Associated With Improved Treatment Outcome Response to Pembrolizumab in Patients With Rare Tumors. J Immunother Cancer (2020) 8(1). doi: 10.1136/jitc-2020-000665
91. Ryan R, Gibbons D, Hyland JMP, Treanor D, White A, Mulcahy HE, et al. Pathological Response Following Long-Course Neoadjuvant Chemoradiotherapy for Locally Advanced Rectal Cancer. Histopathol (2005) 47(2):141–6. doi: 10.1111/j.1365-2559.2005.02176.x
92. Voskuilen CS, Oo HZ, Genitsch V, Smit LA, Vidal A, Meneses M, et al. Multicenter Validation of Histopathologic Tumor Regression Grade After Neoadjuvant Chemotherapy in Muscle-Invasive Bladder Carcinoma. Am J Surg Pathol (2019) 43(12):1600–10. doi: 10.1097/PAS.0000000000001371
93. Jaraj SJ, Camparo P, Boyle H, Germain F, Nilsson B, Petersson F, et al. Intra- and Interobserver Reproducibility of Interpretation of Immunohistochemical Stains of Prostate Cancer. Virchows Arch (2009) 455(4):375–81. doi: 10.1007/s00428-009-0833-8
94. Stoler MH, Schiffman M. Atypical Squamous Cells of Undetermined Significance-Low-Grade Squamous Intraepithelial Lesion Triage Study (ALTS) Group. Interobserver Reproducibility of Cervical Cytologic and Histologic Interpretations: Realistic Estimates From the ASCUS-LSIL Triage Study. JAMA (2001) 285(11):1500–5. doi: 10.1001/jama.285.11.1500
95. Stattaus J, Hahn S, Gauler T, Eberhardt W, Mueller SP, Forsting M, et al. Osteoblastic Response as a Healing Reaction to Chemotherapy Mimicking Progressive Disease in Patients With Small Cell Lung Cancer. Eur Radiol (2009) 19(1):193–200. doi: 10.1007/s00330-008-1115-6
96. Messiou C, Cook G, Reid AHM, Attard G, Dearnaley D, de Bono JS, et al. The CT Flare Response of Metastatic Bone Disease in Prostate Cancer. Acta Radiol (2011) 52(5):557–61. doi: 10.1258/ar.2011.100342
97. Ciray I, Lindman H, Aström KG, Bergh J, Ahlström KH. Early Response of Breast Cancer Bone Metastases to Chemotherapy Evaluated With MR Imaging. Acta Radiol (2001) 42(2):198–206. doi: 10.1080/028418501127346503
98. Tombal B, Rezazadeh A, Therasse P, Van Cangh PJ, Vande Berg B, Lecouvet FE. Magnetic Resonance Imaging of the Axial Skeleton Enables Objective Measurement of Tumor Response on Prostate Cancer Bone Metastases. Prostate (2005) 65(2):178–87. doi: 10.1002/pros.20280
99. Padhani AR, Lecouvet FE, Tunariu N, Koh D-M, De Keyzer F, Collins DJ, et al. METastasis Reporting and Data System for Prostate Cancer: Practical Guidelines for Acquisition, Interpretation, and Reporting of Whole-Body Magnetic Resonance Imaging-Based Evaluations of Multiorgan Involvement in Advanced Prostate Cancer. Eur Urol (2017) 71(1):81–92. doi: 10.1016/j.eururo.2016.05.033
100. Van Nieuwenhove S, Van Damme J, Padhani AR, Vandecaveye V, Tombal B, Wuts J, et al. Whole-Body Magnetic Resonance Imaging for Prostate Cancer Assessment: Current Status and Future Directions. J Magn Reson Imaging (2020). doi: 10.1002/jmri.27485
101. JH O, Lodge MA, Wahl RL. Practical PERCIST: A Simplified Guide to PET Response Criteria in Solid Tumors 1.0. Radiol (2016) 280(2):576–84. doi: 10.1148/radiol.2016142043
102. Schmidkonz C, Cordes M, Schmidt D, Bäuerle T, Goetz TI, Beck M, et al. 68Ga-PSMA-11 PET/CT-Derived Metabolic Parameters for Determination of Whole-Body Tumor Burden and Treatment Response in Prostate Cancer. Eur J Nucl Med Mol Imaging (2018) 45(11):1862–72. doi: 10.1007/s00259-018-4042-z
103. Schmidkonz C, Cordes M, Goetz TI, Prante O, Kuwert T, Ritt P, et al. 68Ga-PSMA-11 PET/CT Derived Quantitative Volumetric Tumor Parameters for Classification and Evaluation of Therapeutic Response of Bone Metastases in Prostate Cancer Patients. Ann Nucl Med (2019) 33(10):766–75. doi: 10.1007/s12149-019-01387-0
104. Zhou J, Li Q, Cao Y. Spatiotemporal Heterogeneity Across Metastases and Organ-Specific Response Informs Drug Efficacy and Patient Survival in Colorectal Cancer. Cancer Res (2021) 81(9):2522–33. doi: 10.1158/0008-5472.CAN-20-3665
105. Pires da Silva I, Lo S, Quek C, Gonzalez M, Carlino MS, Long GV, et al. Site-Specific Response Patterns, Pseudoprogression, and Acquired Resistance in Patients With Melanoma Treated With Ipilimumab Combined With Anti-PD-1 Therapy. Cancer (2020) 126(1):86–97. doi: 10.1002/cncr.32522
106. Osorio JC, Arbour KC, Le DT, Durham JN, Plodkowski AJ, Halpenny DF, et al. Lesion-Level Response Dynamics to Programmed Cell Death Protein (PD-1) Blockade. J Clin Oncol (2019) 37(36):3546–55. doi: 10.1200/JCO.19.00709
107. Schmid S, Diem S, Li Q, Krapf M, Flatz L, Leschka S, et al. Organ-Specific Response to Nivolumab in Patients With Non-Small Cell Lung Cancer (NSCLC). Cancer Immunol Immunother (2018) 67(12):1825–32. doi: 10.1007/s00262-018-2239-4
108. Choi H, Charnsangavej C, de Castro Faria S, Tamm EP, Benjamin RS, Johnson MM, et al. CT Evaluation of the Response of Gastrointestinal Stromal Tumors After Imatinib Mesylate Treatment: A Quantitative Analysis Correlated With FDG PET Findings. AJR Am J Roentgenol (2004) 183(6):1619–28. doi: 10.2214/ajr.183.6.01831619
109. Choi H, Charnsangavej C, Faria SC, Macapinlac HA, Burgess MA, Patel SR, et al. Correlation of Computed Tomography and Positron Emission Tomography in Patients With Metastatic Gastrointestinal Stromal Tumor Treated at a Single Institution With Imatinib Mesylate: Proposal of New Computed Tomography Response Criteria. J Clin Oncol (2007) 25(13):1753–9. doi: 10.1200/JCO.2006.07.3049
110. Fournier L, Ammari S, Thiam R, Cuénod C-A. Imaging Criteria for Assessing Tumour Response: RECIST, mRECIST, Cheson. Diagn Interv Imaging (2014) 95(7–8):689–703. doi: 10.1016/j.diii.2014.05.002
111. Benjamin RS, Choi H, Macapinlac HA, Burgess MA, Patel SR, Chen LL, et al. We Should Desist Using RECIST, at Least in GIST. J Clin Oncol (2007) 25(13):1760–4. doi: 10.1200/JCO.2006.07.3411
112. Maas M, Beets-Tan R, Gaubert J-Y, Gomez Munoz F, Habert P, Klompenhouwer LG, et al. Follow-Up After Radiological Intervention in Oncology: ECIO-ESOI Evidence and Consensus-Based Recommendations for Clinical Practice. Insights Imaging (2020) 11(1):83. doi: 10.1186/s13244-020-00884-5
113. Sabet A, Meyer C, Aouf A, Sabet A, Ghamari S, Pieper CC, et al. Early Post-Treatment FDG PET Predicts Survival After 90Y Microsphere Radioembolization in Liver-Dominant Metastatic Colorectal Cancer. Eur J Nucl Med Mol Imaging (2015) 42(3):370–6. doi: 10.1007/s00259-014-2935-z
114. Lencioni R, Llovet JM. Modified RECIST (mRECIST) Assessment for Hepatocellular Carcinoma. Semin Liver Dis (2010) 30(1):52–60. doi: 10.1055/s-0030-1247132
115. Imseeh G, Giles SL, Taylor A, Brown MRD, Rivens I, Gordon-Williams R, et al. Feasibility of Palliating Recurrent Gynecological Tumors With MRGHIFU: Comparison of Symptom, Quality-of-Life, and Imaging Response in Intra and Extra-Pelvic Disease. Int J Hyperthermia (2021) 38(1):623–32. doi: 10.1080/02656736.2021.1904154
116. Ji Y, Zhu J, Zhu L, Zhu Y, Zhao H. High-Intensity Focused Ultrasound Ablation for Unresectable Primary and Metastatic Liver Cancer: Real-World Research in a Chinese Tertiary Center With 275 Cases. Front Oncol (2020) 10:519164. doi: 10.3389/fonc.2020.519164
117. Wolchok JD, Hoos A, O’Day S, Weber JS, Hamid O, Lebbe C, et al. Guidelines for the Evaluation of Immune Therapy Activity in Solid Tumors: Immune-Related Response Criteria. Clin Cancer Res (2009) 15(23):7412–20. doi: 10.1158/1078-0432.CCR-09-1624
118. Tazdait M, Mezquita L, Lahmar J, Ferrara R, Bidault F, Ammari S, et al. Patterns of Responses in Metastatic NSCLC During PD-1 or PDL-1 Inhibitor Therapy: Comparison of RECIST 1.1, irRECIST and iRECIST Criteria. Eur J Cancer (2018) 88:38–47. doi: 10.1016/j.ejca.2017.10.017
119. Park HJ, Kim KW, Pyo J, Suh CH, Yoon S, Hatabu H, et al. Incidence of Pseudoprogression During Immune Checkpoint Inhibitor Therapy for Solid Tumors: A Systematic Review and Meta-Analysis. Radiol (2020) 297(1):87–96. doi: 10.1148/radiol.2020200443
120. Seymour L, Bogaerts J, Perrone A, Ford R, Schwartz LH, Mandrekar S, et al. iRECIST: Guidelines for Response Criteria for Use in Trials Testing Immunotherapeutics. Lancet Oncol (2017) 18(3):e143–52. doi: 10.1016/S1470-2045(17)30074-8
121. Russo M, Crisafulli G, Sogari A, Reilly NM, Arena S, Lamba S, et al. Adaptive Mutability of Colorectal Cancers in Response to Targeted Therapies. Sci (2019) 366(6472):1473–80. doi: 10.1126/science.aav4474
122. Siravegna G, Lazzari L, Crisafulli G, Sartore-Bianchi A, Mussolin B, Cassingena A, et al. Radiologic and Genomic Evolution of Individual Metastases During HER2 Blockade in Colorectal Cancer. Cancer Cell (2018) 34(1):148–62.e7. doi: 10.1016/j.ccell.2018.06.004
123. Dong Z-Y, Zhai H-R, Hou Q-Y, Su J, Liu S-Y, Yan H-H, et al. Mixed Responses to Systemic Therapy Revealed Potential Genetic Heterogeneity and Poor Survival in Patients With Non-Small Cell Lung Cancer. Oncologist (2017) 22(1):61–9. doi: 10.1634/theoncologist.2016-0150
124. de Castro J, Cobo M, Isla D, Puente J, Reguart N, Cabeza B, et al. Recommendations for Radiological Diagnosis and Assessment of Treatment Response in Lung Cancer: A National Consensus Statement by the Spanish Society of Medical Radiology and the Spanish Society of Medical Oncology. Clin Transl Oncol (2015) 17(1):11–23. doi: 10.1007/s12094-014-1231-5
125. Ferretti GR, Reymond E, Delouche A, Sakhri L, Jankowski A, Moro-Sibilot D, et al. Personalized Chemotherapy of Lung Cancer: What the Radiologist Should Know. Diagn Interv Imaging (2016) 97(3):287–96. doi: 10.1016/j.diii.2015.11.013
126. Crabb SJ, Patsios D, Sauerbrei E, Ellis PM, Arnold A, Goss G, et al. Tumor Cavitation: Impact on Objective Response Evaluation in Trials of Angiogenesis Inhibitors in Non-Small-Cell Lung Cancer. J Clin Oncol (2009) 27(3):404–10. doi: 10.1200/JCO.2008.16.2545
127. Marom EM, Martinez CH, Truong MT, Lei X, Sabloff BS, Munden RF, et al. Tumor Cavitation During Therapy With Antiangiogenesis Agents in Patients With Lung Cancer. J Thorac Oncol (2008) 3(4):351–7. doi: 10.1097/JTO.0b013e318168c7e9
128. Kong BY, Menzies AM, Saunders CAB, Liniker E, Ramanujam S, Guminski A, et al. Residual FDG-PET Metabolic Activity in Metastatic Melanoma Patients With Prolonged Response to Anti-PD-1 Therapy. Pigment Cell Melanoma Res (2016) 29(5):572–7. doi: 10.1111/pcmr.12503
129. Rubin DL, Willrett D, O’Connor MJ, Hage C, Kurtz C, Moreira DA. Automated Tracking of Quantitative Assessments of Tumor Burden in Clinical Trials. Transl Oncol (2014) 7(1):23–35. doi: 10.1593/tlo.13796
130. Barash Y, Klang E. Automated Quantitative Assessment of Oncological Disease Progression Using Deep Learning. Ann Transl Med (2019) 7(Suppl 8):S379. doi: 10.21037/atm.2019.12.101
131. Kickingereder P, Isensee F, Tursunova I, Petersen J, Neuberger U, Bonekamp D, et al. Automated Quantitative Tumour Response Assessment of MRI in Neuro-Oncology With Artificial Neural Networks: A Multicentre, Retrospective Study. Lancet Oncol (2019) 20(5):728–40. doi: 10.1016/S1470-2045(19)30098-1
132. Baidya Kayal E, Kandasamy D, Yadav R, Bakhshi S, Sharma R, Mehndiratta A. Automatic Segmentation and RECIST Score Evaluation in Osteosarcoma Using Diffusion MRI: A Computer Aided System Process. Eur J Radiol (2020) 133:109359. doi: 10.1016/j.ejrad.2020.109359
133. Tang Y, Yan K, Xiao J, Summers RM. “One Click Lesion RECIST Measurement and Segmentation on CT Scans”. In: Martel AL, Abolmaesumi P, Stoyanov D, Mateus D, Zuluaga MA, Zhou SK, et al. editors. Medical Image Computing and Computer Assisted Intervention – MICCAI 2020, vol. p . Cham: Springer International Publishing (2020). p. 573–83. (Lecture Notes in Computer Science).
134. Moawad AW, Fuentes D, Khalaf AM, Blair KJ, Szklaruk J, Qayyum A, et al. Feasibility of Automated Volumetric Assessment of Large Hepatocellular Carcinomas’ Responses to Transarterial Chemoembolization. Front Oncol (2020) 10:572. doi: 10.3389/fonc.2020.00572
135. Iannessi A, Beaumont H, Liu Y, Bertrand A-S. RECIST 1.1 and Lesion Selection: How to Deal With Ambiguity at Baseline? Insights Imaging (2021) 12(1):36. doi: 10.1186/s13244-021-00976-w
136. Ruchalski K, Braschi-Amirfarzan M, Douek M, Sai V, Gutierrez A, Dewan R, et al. A Primer on RECIST 1.1 for Oncologic Imaging in Clinical Drug Trials. Radiol Imaging Cancer (2021) 3(3):e210008. doi: 10.1148/rycan.2021210008
137. Kantarjian HM, Fojo T, Mathisen M, Zwelling LA. Cancer Drugs in the United States: Justum Pretium–the Just Price. J Clin Oncol (2013) 31(28):3600–4. doi: 10.1200/JCO.2013.49.1845
138. Davis C, Naci H, Gurpinar E, Poplavska E, Pinto A, Aggarwal A. Availability of Evidence of Benefits on Overall Survival and Quality of Life of Cancer Drugs Approved by European Medicines Agency: Retrospective Cohort Study of Drug Approvals 2009-13. BMJ (2017) 359:j4530. doi: 10.1136/bmj.j4530
139. Cheson BD, Fisher RI, Barrington SF, Cavalli F, Schwartz LH, Zucca E, et al. Recommendations for Initial Evaluation, Staging, and Response Assessment of Hodgkin and Non-Hodgkin Lymphoma: The Lugano Classification. J Clin Oncol (2014) 32(27):3059–68. doi: 10.1200/JCO.2013.54.8800
140. Younes A, Hilden P, Coiffier B, Hagenbeek A, Salles G, Wilson W, et al. International Working Group Consensus Response Evaluation Criteria in Lymphoma (RECIL 2017). Ann Oncol (2017) 28(7):1436–47. doi: 10.1093/annonc/mdx097
141. van den Bent MJ, Wefel JS, Schiff D, Taphoorn MJB, Jaeckle K, Junck L, et al. Response Assessment in Neuro-Oncology (a Report of the RANO Group): Assessment of Outcome in Trials of Diffuse Low-Grade Gliomas. Lancet Oncol (2011) 12(6):583–93. doi: 10.1016/S1470-2045(11)70057-2
142. Lin NU, Lee EQ, Aoyama H, Barani IJ, Barboriak DP, Baumert BG, et al. Response Assessment Criteria for Brain Metastases: Proposal From the RANO Group. Lancet Oncol (2015) 16(6):e270–278. doi: 10.1016/S1470-2045(15)70057-4
Keywords: tumour, biomarker, imaging, response, RECIST
Citation: Fournier L, de Geus-Oei L-F, Regge D, Oprea-Lager D-E, D’Anastasi M, Bidaut L, Bäuerle T, Lopci E, Cappello G, Lecouvet F, Mayerhoefer M, Kunz WG, Verhoeff JC, Caruso D, Smits M, Hoffmann R-T, Gourtsoyianni S, Beets-Tan R, Neri E, deSouza NM, Deroose CM and Caramella C (2022) Twenty Years On: RECIST as a Biomarker of Response in Solid Tumours an EORTC Imaging Group – ESOI Joint Paper. Front. Oncol. 11:800547. doi: 10.3389/fonc.2021.800547
Received: 23 October 2021; Accepted: 30 November 2021;
Published: 10 January 2022.
Edited by:
Zaver Bhujwalla, Johns Hopkins University, United StatesReviewed by:
Cyril Jaudet, Centre François Baclesse, FranceScott Heitaka Okuno, Mayo Clinic, United States
Shiteng Suo, Shanghai Jiao Tong University, China
Copyright © 2022 Fournier, de Geus-Oei, Regge, Oprea-Lager, D’Anastasi, Bidaut, Bäuerle, Lopci, Cappello, Lecouvet, Mayerhoefer, Kunz, Verhoeff, Caruso, Smits, Hoffmann, Gourtsoyianni, Beets-Tan, Neri, deSouza, Deroose and Caramella. This is an open-access article distributed under the terms of the Creative Commons Attribution License (CC BY). The use, distribution or reproduction in other forums is permitted, provided the original author(s) and the copyright owner(s) are credited and that the original publication in this journal is cited, in accordance with accepted academic practice. No use, distribution or reproduction is permitted which does not comply with these terms.
*Correspondence: Laure Fournier, laure.fournier@u-paris.fr