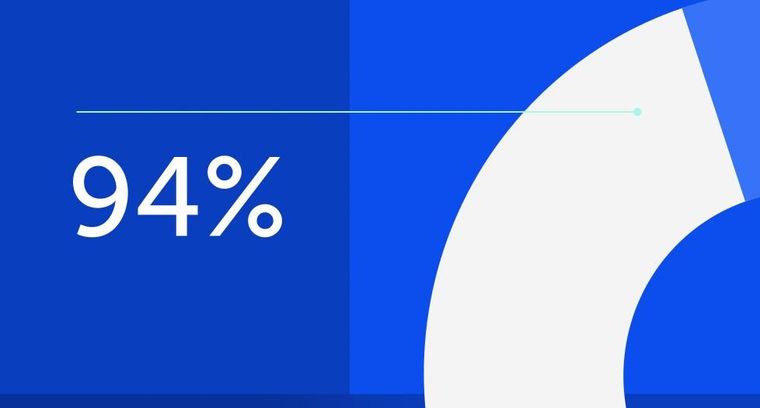
94% of researchers rate our articles as excellent or good
Learn more about the work of our research integrity team to safeguard the quality of each article we publish.
Find out more
SYSTEMATIC REVIEW article
Front. Oncol., 18 November 2021
Sec. Radiation Oncology
Volume 11 - 2021 | https://doi.org/10.3389/fonc.2021.772663
This article is part of the Research TopicWomen in Radiation Oncology: 2021View all 22 articles
Background and Purpose: Machine learning (ML) is emerging as a feasible approach to optimize patients’ care path in Radiation Oncology. Applications include autosegmentation, treatment planning optimization, and prediction of oncological and toxicity outcomes. The purpose of this clinically oriented systematic review is to illustrate the potential and limitations of the most commonly used ML models in solving everyday clinical issues in head and neck cancer (HNC) radiotherapy (RT).
Materials and Methods: Electronic databases were screened up to May 2021. Studies dealing with ML and radiomics were considered eligible. The quality of the included studies was rated by an adapted version of the qualitative checklist originally developed by Luo et al. All statistical analyses were performed using R version 3.6.1.
Results: Forty-eight studies (21 on autosegmentation, four on treatment planning, 12 on oncological outcome prediction, 10 on toxicity prediction, and one on determinants of postoperative RT) were included in the analysis. The most common imaging modality was computed tomography (CT) (40%) followed by magnetic resonance (MR) (10%). Quantitative image features were considered in nine studies (19%). No significant differences were identified in global and methodological scores when works were stratified per their task (i.e., autosegmentation).
Discussion and Conclusion: The range of possible applications of ML in the field of HN Radiation Oncology is wide, albeit this area of research is relatively young. Overall, if not safe yet, ML is most probably a bet worth making.
Cancers of the head and neck (HN) region involve anatomically complex and functionally essential structures, whose damage may severely compromise quality of life, especially in long-surviving patients (1). If the management of HN cancers (HNCs) has always been challenging in Radiation Oncology, in the last years, the clinical scenario has rapidly evolved, due to changes in the epidemiology of the disease (2–4), to the introduction of novel systemic therapies and surgical procedures (5–8) and to the availability of more sophisticated irradiation techniques (9–11). Additionally, as for other cancer sites, understanding on HN neoplasms is taking advantage from progresses in the fields of radiogenomics and quantitative imaging analysis (12–15). Such “big data”-based approaches are progressively being integrated into a more traditional body of knowledge on tumor biology and inter-patient variability which, arguably, may represent a concrete step toward a personalized medicine approach (16).
Nevertheless, this increasing amount of information is hardly manageable by single practitioners, and there is an unprecedented demand of novel, informatics-based tools to structure and solve complex clinical questions. To this aim, machine learning (ML)—a branch of artificial intelligence (AI) relying on patterns and inference to execute a specific task—could provide Radiation Oncologists (ROs) with accurate models to optimize patients’ care paths (17).
As compared with statistical methods, ML focuses on the identification of predictive patterns rather than on drawing inferences from a sample. Starting from sampling and power calculations, statistical models aim to assess whether a relationship between two or more variables describes a true effect and to interpret the extent of the above-mentioned relationship. A quantitative measure of confidence can therefore be provided to test hypothesis and/or verify assumptions (18). By contrast, ML makes use of general-purpose algorithms with no or minimal assumptions. While this may produce hardly interpretable and generalizable results, ML can be useful in case of poorly understood and complex phenomena, when the number of input variable exceeds the number of subjects and complicated nonlinear interactions are present (19). However, statistics- and ML-based models should not be regarded as antagonistic and mutually exclusive. As an example, some methods (i.e., bootstrapping) can be used for both the purpose of statistical inference and for the development of ML models, and a distinct boundary between the two is not always easily traceable.
The choice of the most suitable ML algorithm to solve a given problem starts with the characterization of available data, which can be either labeled (e.g., implemented with additional information, such as: “this computed tomography (CT) slice contains the contour of the tumor”) or unlabeled (e.g., data do not contain any supplementary tag, such as a collection of CT slices). In the first case, the learning problem is of supervised nature, meaning that the algorithm uses labeled data (training set) to assign a class label to unseen, unlabeled instances (test set). Conversely, unsupervised learning uses unlabeled data to identify previously undetected patterns in the data set and reacts to the existence or absence of such patterns in new instances, without the need of human supervision. However, the aim of the model is the same: to assign similar, contiguous pixels with the correct label (PG vs. non-PG) by a computationally efficient and generalizable algorithm. Other than by input data type, models can be categorized according to their output. Broadly, if the output is a number (i.e., grade of acute toxicity per the Common Terminology Criteria of Adverse Events (CTCAE) system), the task is defined as a regression problem, if it is a class (i.e., tumor vs. nontumor), the task is called a classification problem, and if it is a set of input groups (i.e., clinical and dosimetric variables), it is a clustering problem.
Following the idea of a “big-data” approach for cancer care, several publications in the field of Radiation Oncology have come to life, with algorithms encompassing segmentation accuracy, treatment planning optimization, and prediction of both oncological and toxicity outcomes (17, 20–22). A visual representation of the ML workflow applied in this clinical setting is provided in Figure 1. Given the lack of comparable efforts in current literature and the hotness of the topic, we decided to perform a clinically oriented systematic review of the available evidence for ML applications in HNCs. In doing so, we also chose to focus on the methodology of published works and to rate their quality according to a ML-dedicated checklist by Luo et al. (23), generated in 2016 by a multidisciplinary panel of experts in compliance with the Delphi method (24). Ultimately, our goal is to propagate awareness of ROs on ML applications in HNCs. Expectantly, this would contribute to fostering further research and collaboration among different professionals, and to define a novel, data-driven approach to clinical Radiation Oncology for this subset of patients.
Segmentation of target volumes and organs at risk (OARs) is a critical component in the Radiation Oncology workflow. Following the recognition of intensity-modulated radiotherapy (IMRT) as a standard of care for HNC (25), accurate delineation has been associated with improved oncological and toxicity outcomes (26–28). Consequently, minimizing inter- and intraoperator variability in segmentation is crucial, and several guidelines have been published and updated to foster standardization in HNC contouring. Another relevant issue in the current clinical management is the time needed for completing the segmentation of an HNC case, which approximates 3.0 h (29): other than representing a significant commitment to the RO, time represents a limitation toward a more systematic use of adaptive radiotherapy (ART), which requires rapid recontouring and replanning (30). In this context, ML-based autosegmentation holds the promise of optimizing the clinical management for HNC patients and to increase consistency and reproducibility of delineated structures. ML can be implemented to either single or multiple autosegmentation atlases in order to improve registration and segmentation performance. Specifically, such model-based approaches can compare patient’s images with a reference gold standard (ground truth) and overcome acquired imaging limitations including low soft tissue contrast and presence of dental metal artifacts. However, inter- and intrapatient variability and large computational time for registration represent two significant pitfalls of the atlas-based approach (31). Deep learning has the potential to overcome these limitations and has already found several applications in the field of computer vision tasks which, as a whole, can be defined as the automatic extraction, analysis, and understanding of any relevant information from either a single image or a series of images through the construction of dedicated datasets (21, 32).
Treatment planning for HNC is challenging: expertise in both the medical (i.e., knowledge of complex HN anatomy and patterns of disease recurrence, awareness of tolerance of healthy tissues to irradiation) and in the physical field (i.e., coverage of irregularly shaped target volumes, multiple dose prescription levels) is required, and timely delivery of radiotherapy (RT) is mandatory not to compromise oncological outcomes (33). In recent years, an increasing body of evidence has demonstrated that geometrical and anatomical variations can occur during the course of curative-intent treatments for HNC, thus leading to potentially meaningful modifications in dose distribution. Several variables have been investigated, and include, but are not limited to, patients’ weight loss, tumor response, and PG shrinkage (34, 35). The use of ART can quantify and overcome the dosimetric impact of these modifications and restore the desirable therapeutic ratio in this subset of patients (36). Yet, routine implementation of ART in clinical practice is limited by temporal and logistic issues: CT rescanning, recontouring, and replanning require efficient scheduling and execution and involve the whole staff of a Radiation Oncology Department, from radiation therapists to medical physicists.
Outcome prediction is crucial in the field of Radiation Oncology, especially in the era of personalized treatments. As deintensification strategies are being tested in clinical trials (37), and biological and quantitative imaging parameters are gaining the spotlight as promising prognosticators (38, 39), there is an increasing need for effective models integrating this growing body of information (13). A typical problem in outcomes prediction with ML is the management of time-dependent endpoints (i.e., overall survival (OS), local control, progression-free survival). These outcomes, often referred to as “right censored”, may not have yet occurred at the time of the last follow-up, but still require to be considered, as they could present at a later time. Although the pre-processing method for such variables is often influenced by the ML algorithm of choice, it has been recognized that inappropriate recognition of right-censored events may lead to poorly calibrated models (40–43).
Other than achieving disease control by the irradiation of the gross and clinical tumor volumes (GTV and CTV, respectively), the optimal radiation treatment plan aims at the preservation of healthy surrounding structures. Although the introduction of modern RT techniques has ameliorated the therapeutic ratio, acute and chronic RT-related toxicities still represent a significant burden for patients’ quality of life and may compromise timely treatment delivery (25). In recent years, refined anatomical knowledge of normal tissues (i.e., the coexistence of serial and parallel components in architecturally complex patterns in salivary glands) and the recognition of a stem cell compartment in healthy organs have shed light on the need of further improving dose distribution, especially when curative-intent treatments are delivered (44).
To this aim, the use of spatial dose metrics, such as gradient and direction, may provide more comprehensive information than the sole absolute mean and maximum doses (45, 46). Additionally, genetic determinants are thought to impact on individual radiosensitivity/radioresistance of healthy tissues as much as for the 80% (47). ML may combine these emerging factors with more established determinants of toxicity, such as patient factors, administration of systemic therapies and absolute dosimetric parameters (48, 49). Adequate consideration of these covariables in dedicated algorithms could discriminate the probability for a given patient to experience a specific toxicity, and therefore contribute to refine clinical decisions (i.e., prophylactic feeding tube positioning in patients at high risk for severe weight loss) (47, 50).
Study methodology complied with the outlines of the Preferred Reporting Items for Systematic Reviews and Meta-Analyses (PRISMA) (51). Original manuscripts on ML applications for HNC were considered eligible for the analysis; publications encompassing any other cancers were excluded. Interventions included investigations on (auto)segmentation, treatment planning, and outcome prediction (either oncological or toxicity); works whose focus was exclusively diagnostic were considered beyond the scope of the current review. Full papers of any study design except systematic reviews and case reports were considered; only works written in English were included.
Electronic databases (namely, National Center for Biotechnology Information PubMed, Elsevier EMBASE and Elsevier Scopus) were screened up to May 2021 without date restrictions by an author experienced in bibliographic search (SV). Free text, Boolean operators, truncation, and proximity operators were tested. No filters were applied, in order not to exclude potentially relevant publications. The full-search strategy is provided in Supplementary Materials S1.
Findings from the above-reported search were independently screened and selected based on titles by two Authors (SV, RS); disagreements were subsequently discussed in presence of three other authors (FB, MP, MZ). All types of ML algorithms were considered eligible for the analysis, as well as studies encompassing the use of extracted quantitative imaging features. The selection process is shown in Figure 2, while Figure 3 provides an overview of the algorithms considered for the analysis. A more detailed insight of ML models/algorithms included is provided in Table 1.
Figure 2 Study selection process per the Preferred Reporting Items for Systematic Reviews and Meta-Analysis (PRISMA) guidelines.
Figure 3 Classification of the machine-learning algorithms included in the analysis. *Comprehend: ANN, CNN and FCNN. ANN, Artificial Neural Network; CNN, Convolutional Neural Network; FCNN, Fully CNN; HMM, Hidden Markov Model; k-NN, k-Nearest Neighbour; MARS, Multiadaptive Regression Splines; PCA, principal component analysis; PCR, principal component regression; SVC, support vector classifier; SVM, support vector machine.
The quality of the studies included in the analysis was rated by an adapted version of the qualitative checklist originally developed by Luo et al. for the reporting of predictive modeling in biomedical research (23). This checklist, compared with others present in the literature, provides a multidisciplinary overview of ML models, as it was developed taking into account inputs from different professional figures usually involved in medical research, such as clinicians, statisticians, and ML experts. The organization of the checklist was maintained, and the following subsections were rated for each study: “Title and abstract”, “Introduction”, “Methods”, “Results”, and “Discussion”. Each of the 55 items required a dichotomous answer (yes or no, coded as 1 and 0, respectively); two items were divided into three subsections, thus allowing for a maximum achievable score of 58. The complete adapted Luo scoring system can be reviewed in detail in Supplementary Materials S2.
Descriptive statistics (median, mean, interquartile range (IQR), min, max, standard deviation) were provided for global score and methodological score from the modified Luo classification (23). Score differences across study groups (per task and use of quantitative imaging analysis) were assessed with Wilcoxon sum-rank test (when groups = 2) or Kruskal-Wallis test (when groups >2) and graphically evaluated with boxplots. p-values corrected for false-discovery rate (FDR) were also provided to account for multiple testing, considering a threshold of 0.05. All statistical analyses were carried out using R version 3.6.1.
Forty-eight studies were included in the analysis: publication years ranged between 1998 and 2021; with more than a half having been published after 2018 (56%). Twenty-one (44%) focused on ML algorithms for autosegmentation, four (8%) were dedicated to treatment planning, 12 (25%) to oncological outcomes prediction, 10 (21%) to RT-related toxicity, and one (2%) to the determinants of postoperative RT delays following surgery for HNC.
Twenty-one works (44%) considered more than one HNC subsite, while the most common single primary site was the nasopharynx, which was the focus of seven studies (15%). Of note, this information was missing in six cases (12%). The most common imaging modality was CT (40%), followed by magnetic resonance (MR) (10%). Quantitative image features were considered in nine studies (19%) and were mainly CT based (75%). Dosimetric parameters were used in six of the analyzed works, five on toxicity outcomes prediction, and one on the identification of candidates to replanning.
Here follows a detailed description of the studies sorted by main topic, with each topic representing a critical step in the modern workflow for HNC patients in Radiation Oncology.
The majority of the included studies (21/48) focused on the design of ML algorithms for autosegmentation: seven were for the segmentation of treating volumes (either CTV or GTV) and 13 for OARs. Considering the former, tumor GTV (GTV-T) was the target of prediction for six studies; in one of these, the algorithm was used for the delineation of the nodal GTV (GTV-N) and the CTV as well. Additionally, one study aimed at the sole segmentation of the left and right II–IV nodal levels. A fully automated approach was used in all but one study (52). Overall, all models included in the analysis compared favorably with either competing, previously published algorithms, or with the ground truth represented by manual segmentation (52–55). Specifically, the latter showed an overlap with the manual contours measured by the Dice Similarity Coefficient (DSC) ranging from 0.766 to 0.809 for GTV-T and from 0.623 to 0.698 for GTV-N (54, 55). The only study in which the CTV was autosegmented showed a good agreement with manual delineation, achieving a DSC of 0.826, and outperforming the results of the previously published convolutional neural network (CNN), visual geometry group-16 (VGG-16) (55). Notably, the use of a semiautomated method for GTV-T segmentation proved to be less time consuming and correlated with an increase in the intra- and interoperator agreement when compared with fully manual segmentation (52).
Among algorithms for OAR delineation, studies were heterogeneous in the choice of the target(s) of segmentation. The majority of studies (12/13) considered PG segmentation as a primary endpoint (56–68), with the PG being the only considered region of interest (ROI) in four of the selected works (63, 65–67). The segmentation performance assessed by the DSC for all OARs investigated in the included studies is provided in Table 2.
Overall, autosegmentation studies were mainly CT based (13/21); in decreasing order of frequency were MR (three of 21), CT + MR (two of 21), positron emission tomography (PET, two of 21), and CT + PET (one of 21). Sample size varied considerably, ranging from 5 to 486 (median: 46, IQR: 15–166).
A complete description of individual studies characteristics is provided in Table 3.
Of the included studies, two focused on the identification of predictive factors for replanning (74, 75). Guidi et al. (74) used support vector machine (SVM) on a retrospectively collected cohort of 40 HNC patients and 1,200 megavoltage CTs to recognize those who could benefit from ART based on weekly anatomical and dosimetric divergences in CTV and OARs (namely, spinal cord, mandible, and PGs) during the course of treatment. Specifically, the authors could demonstrate that from the fourth week, 77% of patients underwent significant morphological and dosimetric changes, advocating the need for replanning. Of note, PGs were the most prone to modifications, with significant variations from the original plan occurring as early as from the third week of treatment. In the second study, Yu et al. (75) used radiomic features from contrast-enhanced T1-weighted and T2-weighted pre-RT MR images and Least Absolute Shrinkage and Selection Operator (LASSO) logistic regression to build models predicting the need of treatment replanning in a retrospective cohort of 70 patients with nasopharyngeal carcinoma (NPC). The combined T1–T2 model outperformed the ones based on either single MR sequence, with average areas under the curve (AUCs) in the training and testing sets of 0.984 (95% confidence interval (CI): 0.983–0.984) and 0.930 (95% CI: 0.928–0.933), respectively, and six radiomic features selected as significant.
A third study on ML for RT planning was published by Nguyen et al. (76) and focused on the use of a hierarchically densely connected U-net architecture (HD U-net) to predict three-dimensional dose distribution for the planning target volume and 22 OARs in a retrospectively retrieved population of 120 HNC patients. When compared with two variant net architectures (namely, Standard U-net and DenseNet), the proposed algorithm showed better performance in the prediction of the maximum and mean dose to the OARs, better dose homogeneity, conformity, and coverage on the test data. Additionally, the HD U-net requires fewer trainable parameters and a reduced computational time when compared with the Standard U-net and with the DenseNet, respectively.
Finally, Thummerer et al. (77) in their study compared synthetic CT images (sCTs) derived from cone-beam CTs (CBCTs) and MRs for HN patients in terms of both image quality and accuracy in proton dose calculation, considering planning CTs as the ground truth. Image quality was quantified through mean absolute error (MAE) and DSC. The sCTs from CBCTs provided higher image quality with an average MAE of 40 ± 4 HU and a DSC of 0.95, while for MR-based sCTs a MAE of 65 ± 4 HU and a DSC of 0.89 were observed. Overall, the study reports that CBCT- and MR-based sCTs have the potential to be reliably implemented into the ART workflow for proton therapy application, thus overcoming the need of performing multiple planning CTs.
Overall, 12 of the included studies considered oncological outcomes following curative-intent treatment as their target of prediction. In details, six studies (40, 42, 78–81) aimed at predicting OS, while five (40, 82–85) considered loco-regional control (LRC) and one (86) distant metastasis-free survival (DMFS). Only two works focused on more than one oncological outcomes (40, 87). Feature selection methods were applied in two cases (40, 42), both studies used radiomic features extracted from the GTV as input parameters for outcome prediction. Other than these works, four additional publications included texture analysis; overall, features were derived from CT images in two works (40, 86), from MR images in one (84) and from multiple diagnostic modalities in the remaining three cases (42, 82, 83).
A single disease subsite was considered by two studies, with Zdilar et al. (40) including only patients with oropharyngeal cancer (OPC), and Jiang et al. focusing on patients diagnosed with neoplasms of the nasopharynx. Conversely, Bryce et al. (79) and Parmar et al. (42) applied ML to mixed HNC populations; information on subsite distribution could be retrieved in only one case (79). Despite relevant heterogeneity in the choice of ML algorithms and populations, the best performing models in each study reached an AUC between 0.72 and 0.78; the best performance was reached by the only study using Artificial Neural Networks (ANNs) (79).
LRC was the target of prediction in four cases (40, 82–84); population size varied considerably, from the 32 NPC patients included in the study by Tran et al. (82) to the 529 patients diagnosed with OPC in the study published by Zdilar et al. (40). All studies considered the radiomic features extracted from the pretreatment GTV as input parameters for model construction. Three studies evaluated ML models through AUC values (40, 82, 83), with the best performing models being k-nearest neighbors and ANNs; Fujima et al. (84) assessed the performance of their nonlinear SVM models by sensibility, specificity, and positive and negative predictive values (for further details, please refer to Table 4).
Lastly, the prediction of DMFS was the objective of one study (86). Wu et al. proved that the incorporation of pre- and mid-treatment radiomic features extracted from both the primary and nodal GTVs improved the performance of random survival forest models trained and validated on a cohort of 140 locally advanced OPC patients (86).
A total of 11 studies focused on RT-induced toxicities; in each publication algorithms were developed for addressing the prediction task on a single outcome (i.e., xerostomia, dysphagia).
Four studies (), predominantly encompassing multiple HN subsites, focused on xerostomia prediction; all but one included dosimetric parameters in the data set (88). The PGs were the only considered ROI except for the work by Guo et al. (89), where the submandibular glands were included. Despite the common clinical focus, different endpoints for the task of xerostomia prediction were considered. Acute xerostomia was the focus of one study, which aimed to predict parotid shrinkage (88), late xerostomia was investigated in one publication (45), while the development of xerostomia at any time following RT was considered by Soares et al. (90). Gabrys et al. built distinct algorithms for the prediction of early, late, and long-term xerostomia; longitudinal models were developed as well (91). Notably, ML-based classifiers outperformed classic Normal Tissue Complication Probability (NTCP) models based on the sole mean dose to the parotids, thus underlying the need of incorporating multiple parameters for accurate outcome prediction (i.e., gland volume and dose gradients in the right-left and anterior-posterior direction for long-term xerostomia). Overall, sample size was comparable across studies focusing on xerostomia prediction (138–153), except for the one by Pota et al., which analyzed 21 patients (88).
The remaining studies presented different toxicity outcomes (namely, acute dysphagia, weight loss at 3 months following the end of RT, osteoradionecrosis, sensorineural loss, and brain injury) (46, 92–95). A full list of the developed algorithms and statistical findings for all studies included in this subsection is provided in Table 5.
Considering a maximum achievable score of 58 in the adapted Luo rating system for ML applications in biomedical research, median score of the included studies was 39 (IQR: 36–44), with minimum and maximum values being 27 and 53, respectively. When analyzing the Methods items only, median rank was 22 (IQR: 20–25), with the worst and best scores being 15 and 32, respectively. As it can be noted in Figure 4, the groups achieved comparable scores and no statistically significant difference was noted in studies global and methodological ranking (p = 0.48 and 0.67, respectively; FDR-corrected p = 0.62 and 0.67, respectively). Yet, studies dedicated to outcome modeling and treatment planning achieved numerically lower scores in both the global and methodological assessment.
Figure 4 Boxplots for global and methodological scores (modified Luo classification) for the studies included in the analysis, categorized according to the task of the proposed algorithm(s). Tr, treatment.
The scores for studies implementing imaging data (n = 37) categorized according to the use of texture analysis vs. other imaging-derived metrics or deep learning (n = 10 and 27, respectively) were evaluated. Since the analysis of quantitative extracted features usually requires an intensive work of statistical preprocessing, frequently lacking in deep learning studies, we tested the hypothesis that studies extracting features are associated with higher methodological scores. Even though no significant difference was found, a trend favoring texture analysis publications was noted especially for methodological study quality (p = 0.45 [FDR-corrected p = 0.67] vs. p = 0.62 [FDR-corrected p = 0.62] when the global score was considered, as shown in Figure 5).
Figure 5 Boxplots for global and methodological scores (modified Luo classification) for the studies included in the analysis, categorized according to imaging data used as input parameters (texture analysis vs. no texture analysis).
The complete evaluation of each study is provided in Figure 6.
Figure 6 Boxplots representing global and methodological scores (modified Luo classification) for the studies included in the analysis, categorized per the presence of texture analysis.
Results from our systematic review show a wide range of possible applications of ML in the field of HN Radiation Oncology, although this area of research is relatively young, with the majority of studies having been published in the last 3 years. The implementation of quantitative imaging features and the use of a longitudinally collected data as input parameters are both promising in refining model performance and open doors to further investigations.
The present analysis indicates a prevalence of algorithms dedicated to autocontouring, which mirrors the still unmet need for computationally affordable and user-friendly tools for clinical practice implementation. Even if only some authors have attempted to provide a full set of ROIs (56–61, 64, 67, 68), they could demonstrate a general improvement over existing models, with average times for task completion ranging between 0.12 and 30 s. However, the segmentation of small and/or low-contrasted areas, which are common in HN anatomy (e.g., optic chiasm, lenses, brainstem) remains challenging, and more efforts are warranted to equal, or at least to approximate, the performance of semiautomated or fully manual segmentation.
Currently available works on ML for treatment planning are scarce and show significant heterogeneity both in the choice of algorithms and in the characteristics of patients’ populations. Nevertheless, results are promising, as they pave the way to the possibility of effectively reconstructing three-dimensional dose distribution of integrating MR in ART and of predicting the need for replanning based on geometrical and dosimetric modifications during treatment. It is straightforward to understand how the fulfillment of these objective may be relevant in everyday clinical practice, especially in the era of image-guided IMRT for HNC (25). Additionally, reliable ML-based predicting tools may be beneficial also for proton treatment planning, as dose deposition is heavily influenced by patient’s set-up and anatomical variations of both target volumes and OARs (77, 97, 98).
Intriguing findings were reported for outcome prediction, as well. Considering oncological outcomes, supervised and unsupervised models were used with an overall satisfactory performance in small- to medium-sized datasets. Notably, the use of combined models incorporating radiomics (40) and longitudinal characteristics (86) yielded the best results. Moreover, neural networks outperformed competing algorithms in the prediction of recurrence patterns in NPC and survival in a population of locally advanced HNCs, respectively (79, 83). Conversely, only two studies incorporated ANNs for the prediction of RT-related toxicities (90, 95), and a prevalence of binary classifiers using labelled data was noticed, as expected. Gabrys et al. (91) were the only ones who compared ML univariate and multivariate logistic regression models to classical NTCP models based on the mean dose to the PGs. In their study, the authors could demonstrate that clinical characteristics and organ- and dose-shape features can improve xerostomia prediction, thus emphasizing the need of multidimensional input parameters to model complex outcomes.
Only one study focused on the use of ML for the analysis of organizational features of RT. In detail, Shew et al. (99) used a supervised classifier to discriminate risk factors correlating with delays in adjuvant treatment delivery. Despite several methodological limitations, the work is based on a large cohort from the National Cancer Database (NCDB), and includes a total of 76,573 patients. Another worth of this study relies in the use of ML for optimizing treatment scheduling: while prediction accuracy needs improving, the proposed model still provides a valuable example on how ML could be used in Radiation Oncology departments to facilitate executional tasks and, ultimately, to improve the quality of care.
Despite desirable, it is not currently possible to perform a reliable comparison among models, even for algorithms designed for the same task (i.e., autosegmentation). Not only was the choice of algorithms, features and variables widely heterogeneous, but most studies considered small- to medium-sized datasets and mixed disease subsites. In particular, sample size could strongly affect the quality of ML models as the training sets size is widely recognized as one of the main issues in pattern recognition studies. In fact, as the number of considered features increases, larger training sets become mandatory to avoid the so-called curse of dimensionality (100). To partially overcome this issue, we have performed a qualitative comparison based on a modified version of a reporting guideline validated by Luo et al. (23), which was previously introduced by Jethanandani et al. (12) in their systematic review on MR-based radiomic studies in HNCs. As pointed out by the authors, the checklist is not without limitations, including difficult and/or subjective interpretability of some items, as noted by our group as well.
Considering these pitfalls, and the fact that the checklist was not designed to provide a quantitative assessment, relevant findings still emerged. Firstly, studies aiming at toxicity prediction resulted to have the highest quality in both global and methodological scores as compared with those classified in the other categories. Secondly, works incorporating quantitative image features as input parameters had better median methodological scores, which could be at least partially explained by adequate reporting on the preprocessing on imaging data. Finally, works having a nonclinician as first author achieved a higher ranking, with a strong statistical significance. This finding could derive from the scarcity of dedicated educational training on ML and statistics in most medical schools and residency programs.
The DSC was the performance evaluation metric used in all works dedicated to autosegmentation, while the AUC was implemented in one study only (63). Considering the remaining publications, the AUC was the metric of choice in 17/27 (63%) cases. Despite its popularity for model assessment, limitations of the AUC have been extensively discussed (101). While a dissertation on the matter is beyond the scope of this work, those approaching ML should consider that AUC weights false positive and false negative predictions equally, which can be extremely relevant in the clinical setting (i.e., when the aim is to predict if a patient will develop mild vs. severe xerostomia).
Admittedly, our work presents some limitations. As for all systematic reviews, eligible publications of the last months may be missing, albeit the search was repeated regularly while the manuscript was being written. Moreover, despite our attempt to perform a comprehensive search, the lack of a common ontology in ML may have led to the exclusion of some works: to overcome this potential bias, cross-references from the included works were screened for eligibility. To conclude, we provided the full search strategy for future reference, as we are aware that several additional works will be published in the upcoming months, given the fast-growing nature of this field.
Acknowledging these issues, we do believe that, other than being a full overview of existing literature, the value of our work is to provide a systematic quality assessment of published works, which could be informative for both general and advanced readers. Large-scale datasets, common ontology, study design, and performance reporting will most probably be needed to concretely implement ML in clinical practice, and discussion on this regard is both expected and encouraged. To this aim, the inclusion of dedicated AI courses in the educational track of future ROs would arguably foster the quality of scientific outputs in the field.
Finally, ML-based modeling for HNC is a promising and rapidly expanding field, even though more solidly constructed and validated algorithms are warranted to overcome the boundaries of speculative investigation and to open doors to better tailored Radiation Oncology for this subset of patients. Overall, if not safe yet, ML is most probably a bet worth making.
The individual scores assigned to all the studies included in the manuscript are available upon request to the corresponding author.
SV, MP, MZ, FB, RS, GM, and BJF were responsible for conception and design of the study and wrote the first draft of the manuscript. SV, MP, MZ, and FB were responsible for data acquisition and wrote sections of the manuscript. LJI, DA, SG, AS, ML, and RO wrote sections of the manuscript. All authors contributed to manuscript revision and read and approved the submitted version.
The study was fully funded by the University of Milan with APC funds. The Institution of some authors (IEO) was partially supported by the Italian Ministry of Health with Ricerca Corrente and 5 × 1,000 funds. SV was supported by the Department of Oncology and Hemato-Oncology (DIPO) of the University of Milan with “Progetto di Eccellenza”. MZ received a research grant from the European Institute of Oncology-Cardiologic Center Monzino Foundation (FIEO-CCM), with a project entitled “Proton therapy vs. photon-based IMRT for parotid gland tumors: a model based approach with Normal Tissue Complication Probability (NTCP)” outside the current study. SV, FB, and LJI are PhD students within the European School of Molecular Medicine (SEMM), Milan. The sponsors did not play any role in the study design, collection, analysis, and interpretation of data, nor in the writing of the manuscript, nor in the decision to submit the manuscript for publication.
The authors declare that the research was conducted in the absence of any commercial or financial relationships that could be construed as a potential conflict of interest.
All claims expressed in this article are solely those of the authors and do not necessarily represent those of their affiliated organizations, or those of the publisher, the editors and the reviewers. Any product that may be evaluated in this article, or claim that may be made by its manufacturer, is not guaranteed or endorsed by the publisher.
The Supplementary Material for this article can be found online at: https://www.frontiersin.org/articles/10.3389/fonc.2021.772663/full#supplementary-material
AI, artificial intelligence; ANN, Artificial Neural Network; ART, adaptive RT; AUC, area under the curve; CBCT, cone-beam CT; CI, confidence interval; CNN, Convolutional Neural Network; CT, computed tomography; CTCAE, common terminology criteria of adverse events; CTV, clinical target volume; DMFS, distant metastasis-free survival; DSC, Dice Similarity Coefficient; FDR, false-discovery rate; GTV, gross tumor volume; GTV-N, GTV-nodal; GTV-T, GTV-tumor; HD U-net, Hierarchically Densely connected U-net; HN, head and neck; HNC, HN cancer; IMRT, intensity-modulated RT; IQR, interquartile range; LASSO, Least Absolute Shrinkage and Selection Operator; LRC, loco-regional control; MAE, mean absolute error; ML, machine learning; MR, magnetic resonance; NCDB, National Cancer Database; NPC, nasopharyngeal carcinoma; NTCP, normal tissue complication probability; OAR, organ at risk; OPC, oropharyngeal cancer; OS, overall survival; PET, positron emission tomography; PG, parotid gland; PRISMA, Preferred Reporting Items for Systematic Reviews and Meta-Analysis; RO, radiation oncologist; ROI, region of interest; RT, radiotherapy; sCT, synthetic CT; SVM, support vector machine; VGG-16, Visual Geometry Group-16.
1. Chow LQM. Head and Neck Cancer. Longo DL, Ed. N Engl J Med (2020) 382(1):60–72. doi: 10.1056/NEJMra1715715
2. IARC- International Agency for Research on Cancer. GLOBOCAN Cancer Fact Sheet 2018. Available at: https://gco.iarc.fr/today/data/factsheets/cancers/39-All-cancers-fact-sheet.pdf.
3. D’Souza G, Westra WH, Wang SJ, van Zante A, Wentz A, Kluz N, et al. Differences in the Prevalence of Human Papillomavirus (HPV) in Head and Neck Squamous Cell Cancers by Sex, Race, Anatomic Tumor Site, and HPV Detection Method. JAMA Oncol (2017) 3(2):169. doi: 10.1001/jamaoncol.2016.3067
4. Maxwell JH, Grandis JR, Ferris RL. HPV-Associated Head and Neck Cancer: Unique Features of Epidemiology and Clinical Management. Annu Rev Med (2016) 67(1):91–101. doi: 10.1146/annurev-med-051914-021907
5. Scholfield DW, Gujral DM, Awad Z. Transoral Robotic Surgery for Oropharyngeal Squamous Cell Carcinoma: Improving Function While Maintaining Oncologic Outcome. Otolaryngol Neck Surg (2020) 162(3):267–8. doi: 10.1177/0194599820902043
6. Alsahafi E, Begg K, Amelio I, Raulf N, Lucarelli P, Sauter T, et al. Clinical Update on Head and Neck Cancer: Molecular Biology and Ongoing Challenges. Cell Death Dis (2019) 10(8):540. doi: 10.1038/s41419-019-1769-9
7. Golusiński W. Functional Organ Preservation Surgery in Head and Neck Cancer: Transoral Robotic Surgery and Beyond. Front Oncol (2019) 9:293. doi: 10.3389/fonc.2019.00293
8. Moskovitz J, Moy J, Ferris RL. Immunotherapy for Head and Neck Squamous Cell Carcinoma. Curr Oncol Rep (2018) 20(2):22. doi: 10.1007/s11912-018-0654-5
9. Meijer TWH, Scandurra D, Langendijk JA. Reduced Radiation-Induced Toxicity by Using Proton Therapy for the Treatment of Oropharyngeal Cancer. Br J Radiol (2020) 93(1107):20190955. doi: 10.1259/bjr.20190955
10. Gupta T, Kannan S, Ghosh-Laskar S, Agarwal JP. Systematic Review and Meta-Analyses of Intensity-Modulated Radiation Therapy Versus Conventional Two-Dimensional and/or or Three-Dimensional Radiotherapy in Curative-Intent Management of Head and Neck Squamous Cell Carcinoma. Woloschak GE, Ed. PLoS One (2018) 13(7):e0200137. doi: 10.1371/journal.pone.0200137
11. Jakobi A, Bandurska-Luque A, Stützer K, Haase R, Löck S, Wack LJ, et al. Identification of Patient Benefit From Proton Therapy for Advanced Head and Neck Cancer Patients Based on Individual and Subgroup Normal Tissue Complication Probability Analysis. Int J Radiat Oncol (2015) 92(5):1165–74. doi: 10.1016/j.ijrobp.2015.04.031
12. Jethanandani A, Lin TA, Volpe S, Elhalawani H, Mohamed ASR, Yang P, et al. Exploring Applications of Radiomics in Magnetic Resonance Imaging of Head and Neck Cancer: A Systematic Review. Front Oncol (2018) 8:131. doi: 10.3389/fonc.2018.00131
13. Wong AJ, Kanwar A, Mohamed AS, Fuller CD. Radiomics in Head and Neck Cancer: From Exploration to Application. Transl Cancer Res (2016) 5(4):371–82. doi: 10.21037/tcr.2016.07.18
14. Tinhofer I, Stenzinger A, Eder T, Konschak R, Niehr F, Endris V, et al. Targeted Next-Generation Sequencing Identifies Molecular Subgroups in Squamous Cell Carcinoma of the Head and Neck With Distinct Outcome After Concurrent Chemoradiation. Ann Oncol (2016) 27(12):2262–8. doi: 10.1093/annonc/mdw426
15. The Cancer Genome Atlas Network. Comprehensive Genomic Characterization of Head and Neck Squamous Cell Carcinomas. Nature (2015) 517(7536):576–82. doi: 10.1038/nature14129
16. Malone E, Siu LL. Precision Medicine in Head and Neck Cancer: Myth or Reality? Clin Med Insights Oncol (2018) 12:117955491877958. doi: 10.1177/1179554918779581
17. Feng M, Valdes G, Dixit N, Solberg TD. Machine Learning in Radiation Oncology: Opportunities, Requirements, and Needs. Front Oncol (2018) 8:110. doi: 10.3389/fonc.2018.00110
18. Bzdok D, Altman N, Krzywinski M. Statistics Versus Machine Learning. Nat Methods (2018) 15(4):233–4. doi: 10.1038/nmeth.4642
19. Bzdok D, Krzywinski M, Altman N. Points of Significance: Machine Learning: A Primer. Nat Methods (2017) 14(12):1119–20. doi: 10.1038/nmeth.4526
20. Isaksson LJ, Pepa M, Zaffaroni M, Marvaso G, Alterio D, Volpe S, et al. Machine Learning-Based Models for Prediction of Toxicity Outcomes in Radiotherapy. Front Oncol (2020) 10:790. doi: 10.3389/fonc.2020.00790
21. Boldrini L, Bibault J-E, Masciocchi C, Shen Y, Bittner M-I. Deep Learning: A Review for the Radiation Oncologist. Front Oncol (2019) 9:977. doi: 10.3389/fonc.2019.00977
22. Jarrett D, Stride E, Vallis K, Gooding MJ. Applications and Limitations of Machine Learning in Radiation Oncology. Br J Radiol (2019) 92(1100):20190001. doi: 10.1259/bjr.20190001
23. Luo W, Phung D, Tran T, Gupta S, Rana S, Karmakar C, et al. Guidelines for Developing and Reporting Machine Learning Predictive Models in Biomedical Research: A Multidisciplinary View. J Med Internet Res (2016) 18(12):e323. doi: 10.2196/jmir.5870
24. Banno M, Tsujimoto Y, Kataoka Y. Reporting Quality of the Delphi Technique in Reporting Guidelines: A Protocol for a Systematic Analysis of the EQUATOR Network Library. BMJ Open (2019) 9(4):e024942. doi: 10.1136/bmjopen-2018-024942
25. National Comprehensive Cancer Network. NCCN Clinical Practice Guidelines in Oncology (NCCN Guidelines). Head and Neck Cancers. Version 1.2020 - February 12 (2020). Available at: https://www.nccn.org/professionals/physician_gls/pdf/head-and-neck.pdf.
26. Walker GV, Awan M, Tao R, Koay EJ, Boehling NS, Grant JD, et al. Prospective Randomized Double-Blind Study of Atlas-Based Organ-at-Risk Autosegmentation-Assisted Radiation Planning in Head and Neck Cancer. Radiother Oncol (2014) 112(3):321–5. doi: 10.1016/j.radonc.2014.08.028
27. Mukesh M, Benson R, Jena R, Hoole A, Roques T, Scrase C, et al. Interobserver Variation in Clinical Target Volume and Organs at Risk Segmentation in Post-Parotidectomy Radiotherapy: Can Segmentation Protocols Help? Br J Radiol (2012) 85(1016):e530–6. doi: 10.1259/bjr/66693547
28. Feng MU, Demiroz C, Vineberg KA, Balter JM, Eisbruch A. Intra-Observer Variability of Organs at Risk for Head and Neck Cancer: Geometric and Dosimetric Consequences. Int J Radiat Oncol (2010) 78(3):S444–5. doi: 10.1016/j.ijrobp.2010.07.1044
29. Kosmin M, Ledsam J, Romera-Paredes B, Mendes R, Moinuddin S, de Souza D, et al. Rapid Advances in Auto-Segmentation of Organs at Risk and Target Volumes in Head and Neck Cancer. Radiother Oncol (2019) 135:130–40. doi: 10.1016/j.radonc.2019.03.004
30. Levendag PC, Hoogeman M, Teguh D, Wolf T, Hibbard L, Wijers O, et al. Atlas Based Auto-Segmentation of CT Images: Clinical Evaluation of Using Auto-Contouring in High-Dose, High-Precision Radiotherapy of Cancer in the Head and Neck. Int J Radiat Oncol (2008) 72(1):S401. doi: 10.1016/j.ijrobp.2008.06.1285
31. Langerak TR, Berendsen FF, van der Heide UA, Kotte ANTJ, Pluim JPW. Multiatlas-Based Segmentation With Preregistration Atlas Selection: Multiatlas-Based Segmentation With Preregistration Atlas Selection. Med Phys (2013) 40(9):091701. doi: 10.1118/1.4816654
32. Sandeep Kumar E, Satya Jayadev P. “Deep Learning for Clinical Decision Support Systems: A Review From the Panorama of Smart Healthcare”. In: Dash S, Acharya BR, Mittal M, Abraham A, Kelemen A, editors. Deep Learning Techniques for Biomedical and Health Informatics, Studies in Big Data, vol 68. Cham: Springer (2020). p. 79–99. doi: 10.1007/978-3-030-33966-1_5
33. Inal A, Duman E, Ozkan E. Evaluating Different Radiotherapy Treatment Plans, in Terms of Critical Organ Scoring Index, Conformity Index, Tumor Control Probability, and Normal Tissue Complication Probability Calculations in Early Glottic Larynx Carcinoma. J Cancer Res Ther (2020) 16(3):485. doi: 10.4103/jcrt.JCRT_888_18
34. Stauch Z, Zoller W, Tedrick K, Walston S, Christ D, Hunzeker A, et al. An Evaluation of Adaptive Planning by Assessing the Dosimetric Impact of Weight Loss Throughout the Course of Radiotherapy in Bilateral Treatment of Head and Neck Cancer Patients. Med Dosim (2020) 45(1):52–9. doi: 10.1016/j.meddos.2019.05.003
35. Svecic A, Roberge D, Kadoury S. Prediction of Inter-Fractional Radiotherapy Dose Plans With Domain Translation in Spatiotemporal Embeddings. Med Image Anal (2020) 64:101728. doi: 10.1016/j.media.2020.101728
36. Liu Q, Liang J, Zhou D, Krauss DJ, Chen PY, Yan D. Dosimetric Evaluation of Incorporating Patient Geometric Variations Into Adaptive Plan Optimization Through Probabilistic Treatment Planning in Head and Neck Cancers. Int J Radiat Oncol (2018) 101(4):985–97. doi: 10.1016/j.ijrobp.2018.03.062
37. Patel RR, Ludmir EB, Augustyn A, Zaorsky NG, Lehrer EJ, Ryali R, et al. De-Intensification of Therapy in Human Papillomavirus Associated Oropharyngeal Cancer: A Systematic Review of Prospective Trials. Oral Oncol (2020) 103:104608. doi: 10.1016/j.oraloncology.2020.104608
38. Tanadini-Lang S, Balermpas P, Guckenberger M, Pavic M, Riesterer O, Vuong D, et al. Radiomic Biomarkers for Head and Neck Squamous Cell Carcinoma. Strahlenther Onkol (2020) 196(10):868–78. doi: 10.1007/s00066-020-01638-4
39. Konings H, Stappers S, Geens M, De Winter BY, Lamote K, van Meerbeeck JP, et al. A Literature Review of the Potential Diagnostic Biomarkers of Head and Neck Neoplasms. Front Oncol (2020) 10:1020. doi: 10.3389/fonc.2020.01020
40. Zdilar L, Vock DM, Marai GE, Fuller CD, Mohamed ASR, Elhalawani H, et al. Evaluating the Effect of Right-Censored End Point Transformation for Radiomic Feature Selection of Data From Patients With Oropharyngeal Cancer. JCO Clin Cancer Inform (2018) 2):1–19. doi: 10.1200/CCI.18.00052
41. Leger S, Zwanenburg A, Pilz K, Lohaus F, Linge A, Zöphel K, et al. A Comparative Study of Machine Learning Methods for Time-to-Event Survival Data for Radiomics Risk Modelling. Sci Rep (2017) 7(1):13206. doi: 10.1038/s41598-017-13448-3
42. Parmar C, Grossmann P, Rietveld D, Rietbergen MM, Lambin P, Aerts HJWL. Radiomic Machine-Learning Classifiers for Prognostic Biomarkers of Head and Neck Cancer. Front Oncol (2015) 5:272. doi: 10.3389/fonc.2015.00272
43. Vock DM, Wolfson J, Bandyopadhyay S, Adomavicius G, Johnson PE, Vazquez-Benitez G, et al. Adapting Machine Learning Techniques to Censored Time-to-Event Health Record Data: A General-Purpose Approach Using Inverse Probability of Censoring Weighting. J BioMed Inform (2016) 61:119–31. doi: 10.1016/j.jbi.2016.03.009
44. Jeong J, Baek H, Kim Y-J, Choi Y, Lee H, Lee E, et al. Human Salivary Gland Stem Cells Ameliorate Hyposalivation of Radiation-Damaged Rat Salivary Glands. Exp Mol Med (2013) 45(11):e58–8. doi: 10.1038/emm.2013.121
45. Guo Y, Jiang W, Lakshminarayanan P, Han P, Cheng Z, Bowers M, et al. Spatial Radiation Dose Influence on Xerostomia Recovery and Its Comparison to Acute Incidence in Patients With Head and Neck Cancer. Adv Radiat Oncol (2019) 5(2):221–20. doi: 10.1016/j.adro.2019.08.009
46. Dean J, Wong K, Gay H, Welsh L, Jones AB, Schick U, et al. Incorporating Spatial Dose Metrics in Machine Learning-Based Normal Tissue Complication Probability (NTCP) Models of Severe Acute Dysphagia Resulting From Head and Neck Radiotherapy. Clin Transl Radiat Oncol (2018) 8:27–39. doi: 10.1016/j.ctro.2017.11.009
47. Scaife JE, Barnett GC, Noble DJ, Jena R, Thomas SJ, West CM, et al. Exploiting Biological and Physical Determinants of Radiotherapy Toxicity to Individualize Treatment. Br J Radiol (2015) 88(1051):20150172. doi: 10.1259/bjr.20150172
48. West CML, Dunning AM, Rosenstein BS. Genome-Wide Association Studies and Prediction of Normal Tissue Toxicity. Semin Radiat Oncol (2012) 22(2):91–9. doi: 10.1016/j.semradonc.2011.12.007
49. Christopherson KM, Ghosh A, Mohamed ASR, Kamal M, Gunn GB, Dale T, et al. Chronic Radiation-Associated Dysphagia in Oropharyngeal Cancer Survivors: Towards Age-Adjusted Dose Constraints for Deglutitive Muscles. Clin Transl Radiat Oncol (2019) 18:16–22. doi: 10.1016/j.ctro.2019.06.005
50. Yang DW, Wang TM, Zhang JB, Li XZ, He YQ, Xiao R, et al. Genome-Wide Association Study Identifies Genetic Susceptibility Loci and Pathways of Radiation-Induced Acute Oral Mucositis. J Transl Med (2020) 18(1):224. doi: 10.1186/s12967-020-02390-0
51. Liberati A, Altman DG, Tetzlaff J, Mulrow C, Gøtzsche PC, Ioannidis JP, et al. The PRISMA Statement for Reporting Systematic Reviews and Meta-Analyses of Studies That Evaluate Healthcare Interventions: Explanation and Elaboration. BMJ (2009) 339(jul21 1):b2700–0. doi: 10.1136/bmj.b2700
52. Beichel RR, Van Tol M, Ulrich EJ, Bauer C, Chang T, Plichta KA, et al. Semiautomated Segmentation of Head and Neck Cancers in 18F-FDG PET Scans: A Just-Enough-Interaction Approach: Semiautomated Segmentation of Head and Neck Cancers. Med Phys (2016) 43(6Part1):2948–64. doi: 10.1118/1.4948679
53. Doshi T, Soraghan J, Petropoulakis L, Di Caterina G, Grose D, MacKenzie K, et al. Automatic Pharynx and Larynx Cancer Segmentation Framework (PLCSF) on Contrast Enhanced MR Images. BioMed Signal Process Control (2017) 33:178–88. doi: 10.1016/j.bspc.2016.12.001
54. Chen A, Deeley MA, Niermann KJ, Moretti L, Dawant BM. Combining Registration and Active Shape Models for the Automatic Segmentation of the Lymph Node Regions in Head and Neck CT Images: Registration and ASM Segmentation of Lymph Node Regions. Med Phys (2010) 37(12):6338–46. doi: 10.1118/1.3515459
55. Men K, Chen X, Zhang Y, Zhang T, Dai J, Yi J, et al. Deep Deconvolutional Neural Network for Target Segmentation of Nasopharyngeal Cancer in Planning Computed Tomography Images. Front Oncol (2017) 7:315. doi: 10.3389/fonc.2017.00315
56. Wang Y, Zhao L, Wang M, Song Z. Organ at Risk Segmentation in Head and Neck CT Images Using a Two-Stage Segmentation Framework Based on 3D U-Net. IEEE Access (2019) 7:144591–602. doi: 10.1109/ACCESS.2019.2944958
57. Liang S, Tang F, Huang X, Yang K, Zhong T, Hu R, et al. Deep-Learning-Based Detection and Segmentation of Organs at Risk in Nasopharyngeal Carcinoma Computed Tomographic Images for Radiotherapy Planning. Eur Radiol (2019) 29(4):1961–7. doi: 10.1007/s00330-018-5748-9
58. Vandewinckele L, Willems S, Robben D, Van Der Veen J, Crijns W, Nuyts S, et al. Segmentation of Head-and-Neck Organs-at-Risk in Longitudinal CT Scans Combining Deformable Registrations and Convolutional Neural Networks. Comput Methods Biomech BioMed Eng Imaging Vis (2019) 11045:1–10. doi: 10.1080/21681163.2019.1673824
59. Zhu W, Huang Y, Zeng L, Chen X, Liu Y, Qian Z, et al. AnatomyNet: Deep Learning for Fast and Fully Automated Whole-Volume Segmentation of Head and Neck Anatomy. Med Phys (2019) 46(2):576–89. doi: 10.1002/mp.13300
60. Nikolov S, Blackwell S, Mendes R, et al. Deep Learning to Achieve Clinically Applicable Segmentation of Head and Neck Anatomy for Radiotherapy. ArXiv180904430 Phys Stat (2018). Available at: http://arxiv.org/abs/1809.04430 (Accessed July 10, 2020).
61. Tong N, Gou S, Yang S, Ruan D, Sheng K. Fully Automatic Multi-Organ Segmentation for Head and Neck Cancer Radiotherapy Using Shape Representation Model Constrained Fully Convolutional Neural Networks. Med Phys (2018) 45(10):4558–67. doi: 10.1002/mp.13147
62. Močnik D, Ibragimov B, Xing L, Strojan P, Likar B, Pernuš F, et al. Segmentation of Parotid Glands From Registered CT and MR Images. Phys Med (2018) 52:33–41. doi: 10.1016/j.ejmp.2018.06.012
63. Hänsch A, Schwier M, Gass T, Morgas T. Evaluation of Deep Learning Methods for Parotid Gland Segmentation From CT Images. J Med Imaging (2018) 6(01):1. doi: 10.1117/1.JMI.6.1.011005
64. Ibragimov B, Xing L. Segmentation of Organs-at-Risks in Head and Neck CT Images Using Convolutional Neural Networks. Med Phys (2017) 44(2):547–57. doi: 10.1002/mp.12045
65. Yang X, Wu N, Cheng G, Zhou Z, Yu DS, Beitler JJ, et al. Automated Segmentation of the Parotid Gland Based on Atlas Registration and Machine Learning: A Longitudinal MRI Study in Head-and-Neck Radiation Therapy. Int J Radiat Oncol Biol Phys (2014) 90(5):1225–33. doi: 10.1016/j.ijrobp.2014.08.350
66. Cheng G, Yang X, Wu N, Xu Z, Zhao H, Wang Y. Multi-Atlas-Based Segmentation of the Parotid Glands of MR Images in Patients Following Head-and-Neck Cancer Radiotherapy. Medical Imaging (2013) 8670:86702Q. doi: 10.1117/12.2007783
67. Qazi AA, Pekar V, Kim J, Xie J, Breen SL, Jaffray DA. Auto-Segmentation of Normal and Target Structures in Head and Neck CT Images: A Feature-Driven Model-Based Approach: Feature-Driven Model-Based Segmentation. Med Phys (2011) 38(11):6160–70. doi: 10.1118/1.3654160
68. Brunenberg EJL, Steinseifer IK, van den Bosch S, Kaanders JHAM, Brouwer CL, Gooding MJ, et al. External Validation of Deep Learning-Based Contouring of Head and Neck Organs at Risk. Phys Imaging Radiat Oncol (2020) 15:8–15. doi: 10.1016/j.phro.2020.06.006
69. Ma Z, Zhou S, Wu X, Zhang H, Yan W, Sun S, et al. Nasopharyngeal Carcinoma Segmentation Based on Enhanced Convolutional Neural Networks Using Multi-Modal Metric Learning. Phys Med Biol (2019) 64(2):025005. doi: 10.1088/1361-6560/aaf5da
70. Ren X, Xiang L, Nie D, Shao Y, Zhang H, Shen D, et al. Interleaved 3d-CNNs for Joint Segmentation of Small-Volume Structures in Head and Neck CT Images. Med Phys (2018) 45(5):2063–75. doi: 10.1002/mp.12837
71. Liang S, Tang F, Huang X, Yang K, Zhong T, Hu R, et al. Deep-Learning-Based Detection and Segmentation of Organs at Risk in Nasopharyngeal Carcinoma Computed Tomographic Images for Radiotherapy Planning. Eur Radiol (2019) 29(4):1961–7. doi: 10.1007/s00330-018-5748-9
72. Stefano A, Vitabile S, Russo G, Ippolito M, Sabini MG, Sardina D, et al. An Enhanced Random Walk Algorithm for Delineation of Head and Neck Cancers in PET Studies. Med Biol Eng Comput (2017) 55(6):897–908. doi: 10.1007/s11517-016-1571-0
73. Yu H, Caldwell C, Mah K, Poon I, Balogh J, MacKenzie R, et al. Automated Radiation Targeting in Head-And-Neck Cancer Using Region-Based Texture Analysis of PET and CT Images. Int J Radiat Oncol (2009) 75(2):618–25. doi: 10.1016/j.ijrobp.2009.04.043
74. Guidi G, Maffei N, Vecchi C, Ciarmatori A, Mistretta GM, Gottardi G, et al. A Support Vector Machine Tool for Adaptive Tomotherapy Treatments: Prediction of Head and Neck Patients Criticalities. Phys Med (2015) 31(5):442–51. doi: 10.1016/j.ejmp.2015.04.009
75. Yu HT, Lam SK, To LT, Tse KY, Cheng NY, Fan YN, et al. Pretreatment Prediction of Adaptive Radiation Therapy Eligibility Using MRI-Based Radiomics for Advanced Nasopharyngeal Carcinoma Patients. Front Oncol (2019) 9:1050. doi: 10.3389/fonc.2019.01050
76. Nguyen D, Jia X, Sher D, Lin MH, Iqbal Z, Liu H, et al. 3D Radiotherapy Dose Prediction on Head and Neck Cancer Patients With a Hierarchically Densely Connected U-Net Deep Learning Architecture. Phys Med Biol (2019) 64(6):65020. doi: 10.1088/1361-6560/ab039b
77. Thummerer A, de Jong BA, Zaffino P, Meijers A, Marmitt GG, Seco J, et al. Comparison of the Suitability of CBCT- and MR-Based Synthetic CTs for Daily Adaptive Proton Therapy in Head and Neck Patients. Phys Med Biol (2020) 65(23):235036. doi: 10.1088/1361-6560/abb1d6
78. Jiang R, You R, Pei XQ, Zou X, Zhang MX, Wang TM, et al. Development of a Ten-Signature Classifier Using a Support Vector Machine Integrated Approach to Subdivide the M1 Stage Into M1a and M1b Stages of Nasopharyngeal Carcinoma With Synchronous Metastases to Better Predict Patients’ Survival. Oncotarget (2016) 7(3):3645–57. doi: 10.18632/oncotarget.6436
79. Bryce TJ, Dewhirst MW, Floyd CE, Hars V, Brizel DM. Artificial Neural Network Model of Survival in Patients Treated With Irradiation With and Without Concurrent Chemotherapy for Advanced Carcinoma of the Head and Neck. Int J Radiat Oncol (1998) 41(2):339–45. doi: 10.1016/S0360-3016(98)00016-9
80. De Felice F, Humbert-Vidan L, Lei M, King A, Guerrero Urbano T. Analyzing Oropharyngeal Cancer Survival Outcomes: A Decision Tree Approach. Br J Radiol (2020) 93(1111):20190464. doi: 10.1259/bjr.20190464
81. Howard FM, Kochanny S, Koshy M, Spiotto M, Pearson AT. Machine Learning–Guided Adjuvant Treatment of Head and Neck Cancer. JAMA Netw Open (2020) 3(11):e2025881. doi: 10.1001/jamanetworkopen.2020.25881
82. Tran WT, Suraweera H, Quaioit K, Cardenas D, Leong KX, Karam I, et al. Predictive Quantitative Ultrasound Radiomic Markers Associated With Treatment Response in Head and Neck Cancer. Future Sci OA (2020) 6(1):FSO433. doi: 10.2144/fsoa-2019-0048
83. Li S, Wang K, Hou Z, Yang J, Ren W, Gao Z, et al. Use of Radiomics Combined With Machine Learning Method in the Recurrence Patterns After Intensity-Modulated Radiotherapy for Nasopharyngeal Carcinoma: A Preliminary Study. Front Oncol (2018) 8:648. doi: 10.3389/fonc.2018.00648
84. Fujima N, Shimizu Y, Yoshida D, Kano S, Mizumachi T, Homma A, et al. Machine-Learning-Based Prediction of Treatment Outcomes Using MR Imaging-Derived Quantitative Tumor Information in Patients With Sinonasal Squamous Cell Carcinomas: A Preliminary Study. Cancers (2019) 11(6):800. doi: 10.3390/cancers11060800
85. Starke S, Leger S, Zwanenburg A, Leger K, Lohaus F, Linge A, et al. 2D and 3D Convolutional Neural Networks for Outcome Modelling of Locally Advanced Head and Neck Squamous Cell Carcinoma. Sci Rep (2020) 10(1):15625. doi: 10.1038/s41598-020-70542-9
86. Wu J, Gensheimer MF, Zhang N, Han F, Liang R, Qian Y, et al. Integrating Tumor and Nodal Imaging Characteristics at Baseline and Mid-Treatment Computed Tomography Scans to Predict Distant Metastasis in Oropharyngeal Cancer Treated With Concurrent Chemoradiotherapy. Int J Radiat Oncol (2019) 104(4):942–52. doi: 10.1016/j.ijrobp.2019.03.036
87. Tseng Y-J, Wang H-Y, Lin T-W, Lu J-J, Hsieh C-H, Liao C-T. Development of a Machine Learning Model for Survival Risk Stratification of Patients With Advanced Oral Cancer. JAMA Netw Open (2020) 3(8):e2011768. doi: 10.1001/jamanetworkopen.2020.11768
88. Pota M, Scalco E, Sanguineti G, Cattaneo GM, Esposito M, Rizzo G. Early Classification of Parotid Glands Shrinkage in Radiotherapy Patients: A Comparative Study. Biosyst Eng (2015) 138:77–89. doi: 10.1016/j.biosystemseng.2015.06.007
89. Guo C, Shi X, Ding X, Zhou Z. Analysis of Radiation Effects in Digital Subtraction Angiography of Intracranial Artery Stenosis. World Neurosurg (2018) 115:e472–5. doi: 10.1016/j.wneu.2018.04.072
90. Soares I, Dias J, Rocha H, Khouri L, do Carmo Lopes M, Ferreira B. Predicting Xerostomia After IMRT Treatments: A Data Mining Approach. Health Technol (2018) 8(1-2):159–68. doi: 10.1007/s12553-017-0204-4
91. Gabrys HS, Buettner F, Sterzing F, Hauswald H, Bangert M. Design and Selection of Machine Learning Methods Using Radiomics and Dosiomics for Normal Tissue Complication Probability Modeling of Xerostomia. Front Oncol (2018) 8:35. doi: 10.3389/fonc.2018.00035
92. Abdollahi H, Mostafaei S, Cheraghi S, Shiri I, Rabi Mahdavi S, Kazemnejad A. Cochlea CT Radiomics Predicts Chemoradiotherapy Induced Sensorineural Hearing Loss in Head and Neck Cancer Patients: A Machine Learning and Multi-Variable Modelling Study. Phys Med PM Int J Devoted Appl Phys Med Biol Off J Ital Assoc BioMed Phys AIFB (2018) 45:192–7. doi: 10.1016/j.ejmp.2017.10.008
93. Leng X, Fang P, Lin H, Qin C, Tan X, Liang Y, et al. Application of a Machine Learning Method to Whole Brain White Matter Injury After Radiotherapy for Nasopharyngeal Carcinoma. Cancer Imaging Off Publ Int Cancer Imaging Soc (2019) 19(1):19. doi: 10.1186/s40644-019-0203-y
94. Zhang B, Lian Z, Zhong L, Zhang X, Dong Y, Chen Q, et al. Machine-Learning Based MRI Radiomics Models for Early Detection of Radiation-Induced Brain Injury in Nasopharyngeal Carcinoma. BMC Cancer (2020) 20(1):502. doi: 10.1186/s12885-020-06957-4
95. Humbert-Vidan L, Patel V, Oksuz I, King AP, Guerrero Urbano T. Comparison of Machine Learning Methods for Prediction of Osteoradionecrosis Incidence in Patients With Head and Neck Cancer. Br J Radiol (2021) 94(1120):20200026. doi: 10.1259/bjr.20200026
96. Cheng Z, Nakatsugawa M, Hu C, Robertson SP, Hui X, Moore JA, et al. Evaluation of Classification and Regression Tree (CART) Model in Weight Loss Prediction Following Head and Neck Cancer Radiation Therapy. Adv Radiat Oncol (2018) 3(3):346–55. doi: 10.1016/j.adro.2017.11.006
97. Deiter N, Chu F, Lenards N, Hunzeker A, Lang K, Mundy D. Evaluation of Replanning in Intensity-Modulated Proton Therapy for Oropharyngeal Cancer: Factors Influencing Plan Robustness. Med Dosim (2020) 45:S095839472030100X. doi: 10.1016/j.meddos.2020.06.002
98. Moreno AC, Frank SJ, Garden AS, Rosenthal DI, Fuller CD, Gunn GB, et al. Intensity Modulated Proton Therapy (IMPT) – The Future of IMRT for Head and Neck Cancer. Oral Oncol (2019) 88:66–74. doi: 10.1016/j.oraloncology.2018.11.015
99. Shew M, New J, Bur AM. Machine Learning to Predict Delays in Adjuvant Radiation Following Surgery for Head and Neck Cancer. Otolaryngol Head Neck Surg (2019) 160(6):1058–64. doi: 10.1177/0194599818823200
100. Balki I, Amirabadi A, Levman J, Martel AL, Emersic Z, Meden B, et al. Sample-Size Determination Methodologies for Machine Learning in Medical Imaging Research: A Systematic Review. Can Assoc Radiol J J Assoc Can Radiol (2019) 70(4):344–53. doi: 10.1016/j.carj.2019.06.002
Keywords: systematic review, artificial intelligence, machine learning, radiotherapy, head and neck cancer
Citation: Volpe S, Pepa M, Zaffaroni M, Bellerba F, Santamaria R, Marvaso G, Isaksson LJ, Gandini S, Starzyńska A, Leonardi MC, Orecchia R, Alterio D and Jereczek-Fossa BA (2021) Machine Learning for Head and Neck Cancer: A Safe Bet?—A Clinically Oriented Systematic Review for the Radiation Oncologist . Front. Oncol. 11:772663. doi: 10.3389/fonc.2021.772663
Received: 08 September 2021; Accepted: 25 October 2021;
Published: 18 November 2021.
Edited by:
Henry Soo-Min Park, Yale University, United StatesReviewed by:
Sanjay Aneja, Yale University, United StatesCopyright © 2021 Volpe, Pepa, Zaffaroni, Bellerba, Santamaria, Marvaso, Isaksson, Gandini, Starzyńska, Leonardi, Orecchia, Alterio and Jereczek-Fossa. This is an open-access article distributed under the terms of the Creative Commons Attribution License (CC BY). The use, distribution or reproduction in other forums is permitted, provided the original author(s) and the copyright owner(s) are credited and that the original publication in this journal is cited, in accordance with accepted academic practice. No use, distribution or reproduction is permitted which does not comply with these terms.
*Correspondence: Federica Bellerba, ZmVkZXJpY2EuYmVsbGVyYmFAaWVvLml0
†These authors share first authorship
‡These authors share last authorship
Disclaimer: All claims expressed in this article are solely those of the authors and do not necessarily represent those of their affiliated organizations, or those of the publisher, the editors and the reviewers. Any product that may be evaluated in this article or claim that may be made by its manufacturer is not guaranteed or endorsed by the publisher.
Research integrity at Frontiers
Learn more about the work of our research integrity team to safeguard the quality of each article we publish.