- 1Department of Colorectal Surgery, Shaoxing People’s Hospital (Shaoxing Hospital, Zhejiang University School of Medicine), Shaoxing, China
- 2Department of Breast and Thyroid Surgery, Affiliated Jinhua Hospital, Zhejiang University School of Medicine, Jinhua, China
- 3Department of Colorectal Surgery, Affiliated Jinhua Hospital, Zhejiang University School of Medicine, Jinhua, China
- 4Department of Hepatobiliary Surgery, Affiliated Jinhua Hospital, Zhejiang University School of Medicine, Jinhua, China
Colorectal cancer (CRC) is the second leading cause of cancer death in the world. Immunotherapy using monoclonal antibodies, immune-checkpoint inhibitors, adoptive cell therapy, and cancer vaccines has raised great hopes for treating poor prognosis metastatic CRCs that are resistant to the conventional therapies. However, high inter-tumor and intra-tumor heterogeneity hinder the success of immunotherapy in CRC. Patients with a similar tumor phenotype respond differently to the same immunotherapy regimen. Mutation-based classification, molecular subtyping, and immunoscoring of CRCs facilitated the multi-aspect grouping of CRC patients and improved immunotherapy. Personalized immunotherapy using tumor-specific neoantigens provides the opportunity to consider each patient as an independent group deserving of individualized immunotherapy. In the recent decade, the development of sequencing and multi-omics techniques has helped us classify patients more precisely. The expansion of such advanced techniques along with the neoantigen-based immunotherapy could herald a new era in treating heterogeneous tumors such as CRC. In this review article, we provided the latest findings in immunotherapy of CRC. We elaborated on the heterogeneity of CRC patients as a bottleneck of CRC immunotherapy and reviewed the latest advances in personalized immunotherapy to overcome CRC heterogeneity.
1 Introduction
Colorectal cancer (CRC) had 1.93 million new patients and caused 935,000 deaths worldwide in 2020, making it the third most common cancer and the second leading cause of cancer death in the world (1). The 5-year survival of non-metastatic CRCs is between 70-90%, which is reduced to 12-14% for patients with metastatic CRC (mCRC) (2, 3). In developed countries, CRC accounts for more than 65% of cancers. Although early diagnosis in these countries has improved patient survival, a quarter of patients are still in the metastatic stage at the time of referral, and about half of low-stages patients progress to mCRC (2, 3). Familial adenomatous polyposis (FAP) and hereditary non-polyposis colon cancer (HNPCC) are among the predisposing factors for CRC, accounting for about 5% of CRC cases (4). The primary treatment for those CRCs that are limited to the colon wall is a combination of surgery, radiotherapy, and chemotherapy based on fluoropyrimidine, irinotecan, and oxaliplatin such as FOLFOX and FOLFIRI (5). The metastatic cases are usually not resectable. The combination of radio/chemotherapy and new targeted therapies against epidermal growth factor receptor (EGFR) and vascular endothelial growth factor (VEGF) are the main treatments in this group (5–7).
CRC is among the cancers with tremendous somatic mutations causing high heterogeneity (8, 9). In 15% of CRCs, the high mutational burden (>12 mutations per megabase) is due to a defect in the genes of mismatch repair (MMR) systems such as MLH1, MSH2, MSH6, and PMS2, which causes transcriptional problems, especially in regions with repetitive nucleotides such as microsatellites. This group has microsatellite instability and is called deficient MMR/microsatellite instability-high (dMMR/MSI-H) CRCs. The second category, which comprises 85% of CRCs, has a much lower mutational burden (<8.24 mutations per megabase) and is known as proficient (p)MMR/MSI-low(L) (10, 11). dMMR/MSI-H CRCs have a better prognosis and survival (15% higher survival rate) than the pMMR/MSI-L group (12). Another cause of high mutational load in CRC is mutations in the catalytic subunit of DNA polymerase epsilon (POLE), which is observed in 3% of CRC patients and causes an ultramutated subtype with more than 100 mutations per megabase (10, 13–15). Approximately 40% of CRC cases have been reported to have mutations in KRAS, which induces cell proliferation and angiogenesis and inhibits apoptosis (5). Over 5,000 different KRAS mutations have been identified in CRC (5). Mutations in MMR, POLE, and KRAS, along with mutations in BRAF, NRAS, and other genes, cause high heterogeneity in the treatment response hindering CRC treatment (16, 17). Therefore, the development of new effective treatment strategies for CRC seems necessary (18).
In recent decades, the remarkable success of immunotherapy in achieving long-lasting responses in solid tumors such as melanoma and lung cancer has led to a strong tendency towards the immunotherapy of other tumors (2, 19). Despite the relative responses of CRC patients to immunotherapy, clinical trials have shown that patients do not respond equally to these treatments, and special attention should be paid to the tumor microenvironment (TME) and patient-related factors in each individual (5). The advances in CRC classification based on the mutational signatures have facilitated the decision-making for appropriate immunotherapy. However, the poor prognosis of mCRC patients indicates the need for more personalization of immunotherapy in CRC patients.
In this article, we provided the latest findings in immunotherapy of CRC. We elaborated on the heterogeneity of CRC patients as a bottleneck of CRC immunotherapy and reviewed the latest advances in personalized immunotherapy to overcome CRC heterogeneity.
2 CRC Immunotherapy: Common Approaches
2.1 CRC Microenvironment
CRC microenvironment (CRCME) is a heterogeneous microenvironment containing a variety of immune cells. Numerous studies have linked high infiltration of CD8+ T cells, CD4+ type-1 helper T cells (Th1), follicular helper T cells (Tfh), M1 macrophages, natural killer (NK) cells, and dendritic cells (DCs) with good prognosis in CRC. Contrarily, high infiltration of myeloid-derived suppressor cells (MDSCs), B cells, M2 macrophages, and Th17 cells are associated with poor prognosis (20–23). MDSCs induce pre-metastatic niches, increase angiogenesis, migration, and invasion of tumor cells, and make tumor chemoresistant (24–26). MDSCs also suppress the antitumor activity of NK and T cells and recruit other immunosuppressive cells such as regulatory T (Treg) cells into the tumor site (27, 28). The frequency of MDSCs and Treg cells in the blood and tumor of CRC patients is higher than healthy individuals and healthy tissues around the tumor, respectively (21, 22, 29, 30). However, the relationship between the amount of Treg cells and disease prognosis is still controversial, with some studies associating it with a bad prognosis and some with a good prognosis (31–34).
CRCME is immunologically active in which a variety of immune cells are infiltrated, and different tumor-associated antigens (TAA) and tumor-specific antigens (TSA) are expressed. Besides, numerous molecular markers and receptors such as VEGF, EGFR, insulin-like growth factor-1 receptor (IGF-1R), human epidermal growth factor receptor-2 (HER2), integrins, mucin 5AC (MUC5AC), death receptor-5 (DR5), cytotoxic T-lymphocyte-associated protein-4 (CTLA-4), programmed cell death protein-1 (PD1) are overexpressed in the CRCME (35–43). Hence, immunotherapy targeting these molecules could be a promising therapeutic candidate for CRC treatment (18). Various immunotherapy methods, including monoclonal antibodies (mAbs), immune-checkpoint inhibitors (ICIs), adoptive T cell therapy (ACT), and cancer vaccines, are currently used in CRC (44).
2.2 Antibodies and Derivatives
Approval of mAbs such as Bevacizumab, Cetuximab, Panitumumab, and Ramucirumab as the first and second line of treatment with chemotherapy in mCRC led to greater interest in CRC immunotherapy (5, 45). In 2004, two mAbs, Bevacizumab and Cetuximab, were approved for the treatment of mCRC (5, 18). Bevacizumab is a humanized antibody against VEGF that inhibits angiogenesis, and combined with chemotherapy, increases overall survival (OS) and progression-free survival (PFS) in mCRC patients (46). Other anti-angiogenic mAbs include Ramucirumab and Tanibirumab, which are anti-VEGF receptors (VEGFR). The former has been approved as the second line of mCRC therapy combined with chemotherapy, and the latter has not been approved thus far but has shown positive results in reducing tumor growth (47, 48). Vanucizumab is a bispecific antibody against VEGF and angiopoietin-2 that suppresses angiogenesis and metastasis (49). Aflibercept is a fusion protein containing the extracellular domains of VEGFR1,2 fused to Fc of human IgG, which inhibits VEGF and, in combination with chemotherapy, improves the OS of mCRC patients (50).
Another group of mAbs approved for mCRC is anti-EGFR mAbs, including Cetuximab, Panitumumab, and Necitumumab (18). The first two mAbs are confirmed as the first and second line of treatment in mCRC patients with wild-type Ras, respectively (51, 52). Necitumumab has a higher affinity for EGFR than the other two mAbs. It has shown positive results in reducing tumor growth in mCRC patients, which should be approved in further trials (53). Various studies have reported that patients with mutations in KRAS and other RAS genes are resistant to anti-EGFR mAbs, suggesting the need to evaluate RAS mutations before selecting appropriate treatment (5, 54–56). Other mAbs used in mCRC, including anti-DR5 and anti-IGF-1R mAbs, showed transient effects as monotherapy or in combination with chemotherapy (57).
2.3 Immune-Checkpoint Inhibitors
In recent decades, ICIs have achieved astonishing success in treating solid tumors that had not previously responded well to treatment (2). ICs physiologically suppress the overreaction of immune cells to prevent autoimmunity. By increasing the expression of ICs, tumor cells misuse their inhibitory signals to escape the antitumor immune responses (58, 59). ICIs block ICs or their ligands on the surface of immune cells or tumor cells to restore the antitumor immune responses (2).
The use of ICIs in unclassified CRC patients did not provide an acceptable overall response. It was observed that some patients responded appropriately to the ICIs while the others were ICI-resistant (60, 61). Further studies revealed that hypermutant tumors express a high level of ICs, leading to appropriate responses to ICIs (62). In fact, in tumors with a high mutational burden, neoantigens are more likely to be produced and presented, and immune cells are more likely to infiltrate hypermutant tumors. That is probably why such tumors have a good prognosis and responses to various immunotherapies (63). CRCME in dMMR/MSI-H tumors is highly infiltrated with CD8+ T cells, Th1 cells, macrophages, and enriched with IFN-I (63, 64). Interestingly, the amount of somatic mutations in CRC is associated with treatment response (65). Given the promising results of ICIs in increasing OS and PFS of dMMR/MSI-H patients, the Chinese Society of Clinical Oncology (CSCO) guideline recommends anti-PD1 (Nivolumab and Pembrolizumab) as the first line of treatment for dMMR/MSI-H mCRC patients (66). Besides dMMR/MSI-H patients, POLE-mutated patients also have a high immune cell infiltration and IC expression, resulting in a favorable prognosis and response to ICIs (67).
Unlike hypermutant patients, ICI in pMMR-MSI-L patients did not show promising results, possibly due to the lack of tumor infiltration with immune cells (2, 68). The combination of ICI with radio/chemotherapy and anti-angiogenic agents is currently being investigated in these patients (NCT02563002, NCT03122509). Radio/chemotherapy causes the release of neoantigens through direct damages to the tumor cells (69, 70). The released neoantigens and damage-associated molecular patterns (DAMPs) recruit immune cells into the tumor site, leading to patients’ better response to immunotherapy (69–72). Inhibition of the RAS-AMP activated protein kinase (RAS-AMPK) pathway also increases the tumor infiltration of immune cells and improves the response of pMMR/MSI-L patients to ICI (73–75). Though, it did not show significant changes in the OS of pMMR/MSI-L patients compared to other treatments (73–75).
Bispecific antibodies against TAA and CD3 are another way of increasing the infiltration of immune cells into the CRCME to increase the responses to ICI. Since carcinoembryonic antigen (CEA) is a highly expressed TAA in CRC, CEA-TCB (RG7802) binds to the CEA on the surface of tumor cells and CD3 on the surface of T cells, recruiting more T cells into the tumor site. The combination of CEA-TCB with Atezolizumab (anti-PDL1) was encouraging in pMMR/MSI-L patients, and further investigations are ongoing (NCT02324257, NCT02650713) (76). Targeting other ICs such as T-cell immunoglobulin and mucin domain-containing protein-3 (TIM3), Lymphocyte activation gene-3 protein (LAG3), T cell immunoreceptor with immunoglobulin and ITIM domains (TIGIT), and V-domain immunoglobulin suppressor of T-cell activation (VISTA), as well as the use of agonist for costimulatory molecules such as CD27, OX40, 4-1BB, glucocorticoid-induced tumor necrosis factor receptor (GITR), and CD40 are under investigation in both pMMR and dMMR patients with positive results (2, 64, 77–84).
Besides pMMR/MSI-L patients, the use of ICIs in a large proportion of dMMR/MSI-H patients was not clinically significant (85, 86). For example, tumors with mutations in β2M have defects in the expression of β2-microglobulin with major histocompatibility complex (MHC)-I, leading to antigen presentation deficiency and immune escape of tumors (87). β2M mutation causes resistance to ICI even in dMMR tumors (65, 87). Mutations in genes involved in the antigen processing and presentation pathways as well as in IFN-γ signaling pathways also cause resistance to ICI (64, 88, 89). To sum up, the above cases show that the use of immunotherapy regardless of the tumor and patient conditions leads to treatment inefficiency, which indicates the need to examine each individual’s tumor to select the appropriate treatment option.
3 CRC Heterogeneity: A Challenge of Immunotherapy
CRC is one of the cancers with high heterogeneity among patients and even within a tumor, which has challenged the treatment of this disease (6). Genetic and environmental differences, diverse mutations, differences in the infiltration of immune cells into the CRCME, and even differences in the nutrition and microbiome of patients cause extensive heterogeneity in CRC (16, 17, 90–92).
Hypermutation is a hallmark of CRC, although 20% of these mutations cause cancer and far fewer mutations are common in two or more tumors (6). Patients within a mutational group are even heterogeneous (93). For example, in patients with the KRAS mutation, those with the mutation in codon 13 (G13D) respond to the combination of chemotherapy and targeted therapy, while patients with the mutation in codon 12 (G12R) do not respond well (94). Heterogeneity is even associated with the location of the tumor. It has been observed that BRAF mutations are more common in tumors originating from the right side of the body, and these tumors are hypermutant/MSI-H (6, 95). On the other hand, left tumors usually show chromosomal instability and gene expression profiles associated with EGFR pathway activation (13, 96, 97). These differences also influence the choice of treatment options. Generally, anti-EGFR and chemotherapy are prescribed for left-sided tumors, while a combination of Bevacizumab and chemotherapy is recommended for right-sided tumors (5).
In addition to interpersonal heterogeneity, a tumor has heterogeneity within it (98). This heterogeneity can be manifested between cells in a tumor, between metastatic lesions from a tumor, and even between cells in a metastatic lesion (16). Surprisingly, tumors with the same genetic lineage can exhibit different behaviors, growth rates, and treatment responses (99). It has been revealed that increased intra-tumor heterogeneity is directly associated with poor prognosis and decreased OS/PFS in patients (100). Studies of multiple biopsies from various CRC sites have shown that over 65% of tumors have intra-tumor heterogeneity, and there was 10-30% heterogeneity in KRAS and BRAF mutations within tumors (101–104). Heterogeneity of tumors also changes during cancer progression. In the early stages of CRC, intra-tumor heterogeneity is high and decreases during the disease progression (105). Such vast heterogeneity undermines the value of single-biopsy in determining the phenotype and mutational profile of the tumor to select the appropriate treatment. It accentuates the need for further examination of the tumor with biopsy from different areas and the use of advanced instruments to evaluate CRC heterogeneity (101, 106).
3.1 Efforts to Overcome CRC Heterogeneity
3.1.1 CRC Classification
One way to overcome CRC heterogeneity, especially in choosing the appropriate treatment strategy, is to classify CRC patients from different molecular and immunological aspects. A successful step in this field was the Consensus Molecular Subgroups (CMS) presented by CRC Subtyping Consortium (13, 107). CMS1 subtype includes MSI tumors with high mutational burden and active anti-tumor responses thanks to high infiltration of anti-tumor cells such as CD8+ T cells, Th1 cells, DCs, NK cells, and M1 macrophages as well as a minimum amount of Treg cells (13, 64, 108). CMS1 is known as the MSI-immune subtype and has the best prognosis and well response to immunotherapy (109). The CMS2 subtype is called “canonical”, highlighting its epithelial features and activation of the WNT and MYC pathways. Tumors with the lowest MSI (less than 2%) are in this group (64). Due to the low mutation of these tumors, the infiltration of immune cells is very low, making them known as the immune-desert subtype (6, 13, 108). Given the dysregulated metabolic pathways, CMS3 tumors are called metabolic types. These tumors have mutations in KRAS, and some of them are MSI (13, 107). Immune cell infiltration in CMS3 is slightly higher than CMS2 but still low and has an immunologically-inactive CRCME, referred to as immune-excluded subtype (13, 64, 107). Eventually, CMS4 tumors are called mesenchymal type because they have mesenchymal properties such as strong endothelial-mesenchymal transition activity and high stromal content (13, 107). They are highly infiltrated with immunosuppressive cells such as Treg cells, M2 macrophages, and myeloid cells, and the presence of anti-tumor immune cells such as DCs, activated NK cells, Th1, and CD8+ T cells in their CRCME is very low. Besides, CMS4 tumors have activated VEGF, TGF-β, and CXCL12 signaling pathways, all of which cause the worst prognosis of CMS4 among the four categories (64, 108).
3.1.2 Immunoscore
Besides molecular classification, CRCs can also be classified based on immunological properties. As mentioned, the mutational burden is a hallmark for the response to immunotherapy. However, the resistance of some dMMR tumors and the appropriate response of some pMMR tumors suggest that a high mutational burden alone is not responsible for the response to immunotherapy (2). It seems that the mutations indirectly increase the response to immunotherapy by increasing the probability of immune cell infiltration into the CRCME (2). The amount of mutations in dMMR tumors is roughly 20 times more than that of pMMR tumors, which produce more (20-fold) neoantigens, increase immune cell infiltration, and induce antitumor responses (110). As a result, it makes more sense to evaluate the immune status of CRCME to predict the response to immunotherapy. Accordingly, recent studies suggest using immunoscore in predicting the response to immunotherapy (2, 64, 111). Immunoscore is evaluated based on the rate of T cells, especially CD8+ T cells in the tumor center and invasive margins of the tumor. Therefore, the high amount of CD3+ cells and CD8+ cells in both center and margin of the tumor are determined with a score of 4, and the low number of these cells in both areas is determined with a score of zero (111–113). CD45RO has also been used as a marker in some immunoscore assessments (112, 114).
Interestingly enough, stage I patients with low immunoscore have been shown to have a poor prognosis and low rate of disease-free survival (DFS) similar to those in stage IV (114). On the other hand, high immunoscore was observed in some pMMR-MSI-L patients, and the rate of DFS, OS, and recurrence was similar in MSI-H and MSI-L patients with high immunoscore (115). Also, in patients with low immunoscore, microsatellite status had no beneficial effect on survival (115). These observations indicate that the prognostic value of immunoscore in clinical outcome and response to immunotherapy is higher than the conventional classification system (UICC-TNM) and even classification based on MMR and MSI (64, 115). Noteworthy, classifying patients into only four groups based on the presence or absence of a few subtypes of immune cells does not seem sufficient. More advanced immunophenotyping methods are needed by considering other immune cells, the infiltration site of each immune cell subset, immune activation/exhaustion markers, and other immunological parameters.
3.1.3 Heterogeneity Assessment Methods
Despite the efforts to study CRC heterogeneity, our current knowledge in this field is just the tip of the iceberg. Expanding next-generation sequencing (NGS), single-cell sequencing, and whole-exome sequencing techniques along with the application of omics data at various levels, including genomics, epigenomics, transcriptomics, peptidomics, proteinomics, and metabolomics, could give us valuable information on the heterogeneity of CRCs (116–123).
Advances in computational biology tools and integrating information obtained from different omics methods could develop accurate and rapid models to predict tumor behavior and response to treatment (124–126). Examples of attempts to create virtual tumor models include virtual patient-ModCell and genome-scale metabolic models used in various cancers, including CRC (127–129). The purpose of these models is to use literature to obtain predictive and prognostic biomarkers, including molecular, metabolic, and immunological signatures, to predict disease progression and treatment response (124, 130, 131). Therefore, identifying reliable biomarkers is an urgent need to increase the accuracy of these models. This modeling is in its infancy and requires to address challenges such as a large amount of information obtained from different omics for each individual, the precise classification of each patient uniquely into a category, the inclusion of immunological variables in models, and prediction of tumor response to treatment (124).
Due to the tumor’s dynamic behavior, the study of tumor behavior requires the evaluation of accessible biomarkers that can reflect tumor changes during treatment without the need for biopsy. Therefore, identifying biomarkers in body fluids that can accurately, quickly, and cost-effectively reflect the stage and characteristics of the tumor is desired. Circulating exosomes, microRNAs, tumor cells (CTC), tumor DNA (ctDNA) can be ideal indicators for tumor heterogeneity changes in the course of treatment (6). The similarity of the genetic profile of CTCs with tumors has been reported 50-77% (132, 133). Interestingly, the genetic profile similarity between cell-free DNAs (cfDNA) or ctDNAs and tumors has been reported to be more than 90% (134–136). Examination of the genetic contents of exosomes is an available method to study tumor mutations with acceptable accuracy. Surprisingly, KRAS mutant genes are more likely to be loaded in exosomes than normal KRAS genes (137, 138).
An emerging method for modeling patient tumors to evaluate the treatment response is establishing patient-derived organoids (PDOs) (139). PDOs are three-dimensional structures of the extracellular matrix containing patient-derived tumor cells fed with culture medium and growth factors. The close similarity (70-90%) of PDOs with the primary tumor in terms of structure, function, and even heterogeneity has made PDOs more reliable models than cell lines that have only 10% of the characteristics of primary tumors (139–144). PDOs have been shown to predict treatment responses with an accuracy of 88-100% (139–144). However, the absence of immune cells in PDOs limits this model in predicting the response to immunotherapy (64). Recently, air-liquid interface culture systems enable PDOs to infiltrate with different immune cells and fibroblasts, making PDOs very similar to the tumor microenvironment (TME). These models are reliable tools for assessing the response of patients to immunotherapies (145). Further studies are required to develop these models and confirm their ability to predict the response to various immunotherapies in CRC.
4 Personalized Immunotherapy in CRC
4.1 Cancer Vaccines
Cancer vaccines are immune system boosters that invigorate patients’ immune responses against cancer by exposing tumor antigens to immune cells (44, 146). The immunotherapies discussed so far is targeting TAAs that are overexpressed in the CRCME. Most TAAs targeted in CRC include CEA, EGFR, VEGFR1/2, survivin, mucin-1 (MUC-1), melanoma antigen gene (MAGE), Wilms tumor antigen-1 (WT1), transmembrane-4 superfamily member-5 protein (TM4SF5), mitotic centromere-associated kinesin (MCAK), ring-finger protein-43 (RNF43), translocase of the outer mitochondrial membrane-34 (TOMM34), squamous cell carcinoma antigen recognized by T cells-3 (SART3), insulin-like growth factor II m-RNA-binding protein-3 (IMP3), kinase of the outer chloroplast membrane-1 (KOC1), 5T4, guanylyl cyclase C (GUCY2C), and human telomerase reverse transcriptase (hTERT) (44, 147–160).
Another type of cancer antigen is TSAs or neoantigens, which are nucleotide or polypeptide sequences that are mutated and might be identified as non-self antigens (44). Neoantigens are expressed only on the surface of tumor cells and significantly reduce the risk of adverse effects of vaccination (15, 161). Hypermutant tumors have a higher chance of producing neoantigens, which is one reason for the increase in immune cell infiltration and good prognosis of these tumors (110, 162). The specificity of neoantigens and their association with the prognosis have made neoantigens ideal targets for cancer immunotherapy, especially for personalized cancer vaccines (64). Neoantigens are generated from frameshift and framework mutations, single nucleotide or structural variations, insertions or deletion, and alternative splicing (146). Most of the neoantigens produced in CRC are derived from TTN gene mutations. Mutations in other genes such as TGFBR2, TAF1B, HT001, MARCKS1/2, CDX2, AIM2, TCF7L2, KRAS, PCNXL2, BAX-1, MUC16, SOX9, RNF43, KMT2D, ARID1A, APC, ZFP5N2 could also produce neoantigens (146). Mutations that occur in tumors are divided into driver and passenger types. The products of driver mutations are critical for the survival of tumor cells, and therefore targeting these mutations reduces the likelihood of immune escape by the tumor (15).
Vaccination with short peptides induces a limited immune response. Therefore, the cocktail of several long peptides is usually used in vaccines to induce both class-I and class-II MHC-mediated responses (150, 163, 164). Frameshift mutations generate long neoantigenic peptides with predictable sequences that can be used in vaccines. Interestingly, the number of vaccine peptides that induce the CD8+ T cell responses is directly related to patient survival (151). It has been demonstrated that vaccines with more than two neoantigens were able to control the tumor for a long time in preclinical and clinical models (165). In a trial, vaccination with three frameshift-derived neoantigens was performed on advanced-stage CRC patients, which induced a response in all patients and stopped the disease in one patient (166). Due to the high heterogeneity of CRC patients, some studies are attempting to use cocktail neoantigens or establish a library of DNA, RNA, and peptide vaccines as off-the-shelf vaccines (150, 151, 167–169). The list of clinical trials in neoantigen-based off-the-shelf vaccines and ACT is provided in Table 1.
Neoantigen vaccines are extensively investigated in melanoma and glioblastoma with promising results in increasing patients’ survival (173–175). Given that glioblastoma has a low mutational and neoantigenic burden (8, 176, 177), these results suggest that neoantigen-based vaccines are effective even in tumors with low mutation rates such as pMMR/MSI-L (64, 175). Neoantigen vaccines may improve the infiltration of immune cells into CRCME and increase the immunoscore of tumors, leading to increased tumor responses to ICIs and other immunotherapies (64). The combination of neoantigen vaccines with ICI is currently being investigated in clinical trials (NCT02600949, NCT03289962).
In neoantigen vaccines, prioritizing the selection of neoantigens is a critical step influencing the effectiveness of vaccination. Some studies prioritize selecting those with a high prevalence in CRC patients (146, 167, 168). However, in personalized immunotherapy, the goal is to identify the neoantigens expressed in the patient’s tumor and select the most immunogenic and appropriate ones for vaccination. The application of the NGS technique to perform whole-exome sequencing, whole-genome sequencing, and RNA sequencing, together with the use of omics data, helps us identify mutated regions and neoantigens created in the patient tumor to be used in the personalized vaccine (146).
Due to the presentation of all antigens in the body by MHCs, determining patient MHC profile and selecting appropriate peptides with high binding affinity to the patient MHCs should also be considered to select immunogenic neoantigens (146). Many personalized vaccine studies consider the presence of targeted neoantigens in the patient’s tumor as a criterion for vaccine design. The next step in achieving an effective vaccine is to assess the patient’s immune status in terms of the presence or absence of previous immune response to the selected neoantigen (178, 179). Tumor-derived neoantigens that have not been able to stimulate the immune responses cannot induce strong immune responses in the vaccine formulation (180, 181). Therefore, after selecting the ideal neoantigens and matching them with the patient MHC, it is necessary to evaluate the pre-existing immune responses against those neoantigens by determining the frequency of antigen-specific cytotoxic T cells (CTLs) as well as the antigen-specific IgG titer in the serum (15, 182). Considering the pre-existing immunity in neoantigens selection could enhance the vaccination efficacy and reduce the adverse events (15). Clinical trials using this type of personalized vaccination have shown encouraging results in inducing an antitumor immune response and increasing OS even in chemoresistant CRC patients (178, 179, 182). The process of personalized immunotherapy in CRC is illustrated in Figure 1.
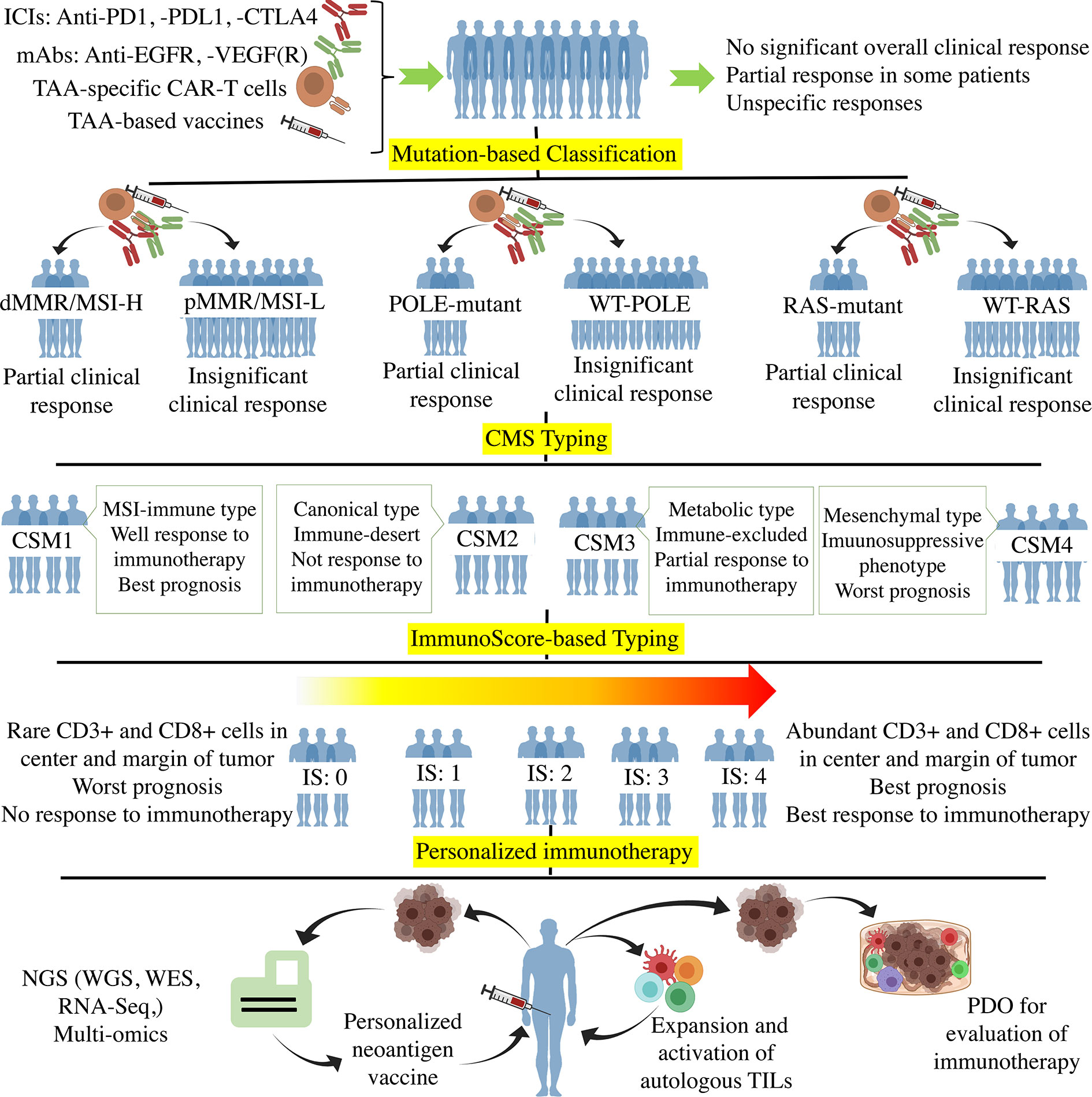
Figure 1 The Process of personalized immunotherapy in CRC. Off-the-shelf immunotherapy such as ICIs, mAbs (anti-EGFR, -VEGF(R)), TAA-specific CAR-T cells, and TAA-base cancer vaccines resulted in tumor regression and stable disease in some patients. However, such immunotherapy did not achieve significant overall clinical responses when used in unclassified CRC patients. The mutation-based classifications according to MMR, POLE, and RAS genes showed that the mutant subtypes are more responsive to immunotherapy. CMS typing more clarified the CRC classification using molecular differences. Regarding the fact that immunotherapy is the method of choice for those tumors with high infiltration of immune cells, the immune-based classification such as immunoscore (Ranged between 0-4) grouped the patients based on the tumor infiltration of immune cells. Ultimately, Personalized immunotherapy tried to classify each patient as an independent group in which the individualized neoantigens is determined through the hi-tech NGS and multi-omics to optimize and personalize the cancer vaccines. Besides, autologous ACT and PDO-based prediction of the immunotherapy responses are the other approaches towards personalized immunotherapy. ICI, Immune-checkpoint inhibitor; PD1, Programmed cell death protein 1; PDL1, PD ligand 1; CTLA4, cytotoxic T-lymphocyte-associated protein 4; TAA, Tumor-associated antigen; EGFR, Epidermal growth factor receptor; VEGFR, Vascular-endothelial growth factor receptor; CAR-T cell, Chimeric antigen receptor T cell; d/pMMR, Deficient/proficient miss match repair; MSI-H/L, Microsatellite instability high/low; POLE, DNA polymerase epsilon; CMS, Consensus molecular subgroup; NGS, Next-generation sequencing; WES, Whole-exome sequencing; RNA-Seq, RNA sequencing; TIL, Tumor-infiltrating lymphocyte; PDO, Patient-derived organoid.
Neoantigen vaccines can be designed and produced in various forms. Peptide/protein vaccines are safe and have a convenient and cost-effective production process but usually do not have sufficient immunogenicity and require adjuvant (178, 183, 184). In addition, the antigenic escape necessitates the need to use antigen cocktails (151, 178, 184). The AutoSynVax vaccine is a personalized vaccine using neoantigens and QS-21 adjuvant that is undergoing a phase 1 clinical trial in advanced cancers (NCT02992977).
DNA and mRNA vaccines are highly immunogenic due to the presentation of produced antigens by both MHC-I and -II pathways. They also stimulate the innate immune sensors in the cytosol (185–187). The mRNA vaccines are safer than DNA vaccines due to the lack of concerns about unwilling integration to the host genome (44, 188). Also, the convenience, speed, and inexpensiveness of mRNA manipulation have led to the widespread acceptance of mRNA vaccines in personalized immunotherapies, which has yielded promising results in CRC (188–190). DCs are ideal candidates to carry the neoantigens into the tumor because they can present neoantigens via both MHC-I/-II pathways and provide costimulatory molecules required for optimum immune responses. They can be pulsed ex vivo with neoantigens or mRNA, matured with cytokines, and then returned to the patient as autologous DC vaccines (191). These vaccines are being tested in clinical trials (Table 2).
Viral, bacterial, and yeast vectors can also be loaded with genes encoding neoantigens and induce robust immune responses against the tumor (44). These vectors release pathogen-associated molecular patterns (PAMPs) that stimulate the innate and adaptive immune response and increase the infiltration of immune cells into the tumor site (206–210). Adenoviruses, lentiviruses, retroviruses, and Poxviruses are important viral vectors in vaccine design (211). Clinical trials with viral vectors loaded with neoantigens and TAAs have been performed on mCRC patients with promising results in inducing the immune responses and improving OS (212, 213). However, viral vector safety concerns such as the possibility of mutation and becoming a pathogen still remain and need further studies (214, 215). The use of engineered bacteria as vectors of neoantigens in clinical trials has also yielded positive results. ADXS-NEO is a personalized neoantigen vaccine with Listeria monocytogenes vector that increased CD8+ T cell infiltration in CRCME in 40% of patients and induced specific responses against 90% of neoantigens (NCT03265080). Yeast vectors are a promising future option for cancer vaccines due to their high safety, ease of large-scale production, no need for adjuvants, and induction of effective responses against neoantigens (207, 216). Several clinical trials are investigating the effectiveness of yeast vectors in mCRC vaccines (44).
Another strategy for personalized vaccination is the use of autologous tumor cells or tumor lysates. Vaccination with whole tumor lysate reduces the possibility of immune escape due to the presence of all tumor antigens (44). However, many of these antigens are normal self-antigens that do not stimulate the immune response and reduce the effectiveness of whole lysate vaccines. Strategies such as using oncolytic viruses in vivo and in vitro or tumor cells infected with oncolytic viruses release PAMPs, which increases immune responses (217, 218). The results of phase I and II trials showed that the use of Newcastle virus-infected tumor cells reduced recurrence and increased OS in CRC patients (44, 219). However, the high levels of self-antigens present in tumor lysates cause the lack of specificity of immune responses and increase the possibility of promoting autoimmune responses, limiting the use of this method in susceptible individuals (220, 221).
4.2 Adoptive T Cell Therapy
ACT is a cancer immunotherapy method in which T cells are collected from the tumor, lymph nodes, or peripheral blood of a patient and returned to the patient’s body after proliferation and selection of tumor-specific T cells. ACT can be performed with unmanipulated cells or engineered cells that express chimeric antigen receptors (CAR-T cells). CAR-T cells are independent of MHCs, and due to carrying costimulatory domains, they could induce strong antitumor responses (2). CAR-T cells are mainly against TAAs that overexpress in CRC, including CEA, EGFR, mesothelin, MUC-1, NKG2D ligand, HER2, c-met, CD133, GUCY2C, epithelial cell adhesion molecule (EpCAM), and Tumor-associated glycoprotein (TAG)-72 (18, 157). These CAR-T cells contain immune activating domains of CD28 and CD137. In the context of mCRC, CAR-T cells as monotherapy or in combination with cytokines such as IL-12 had encouraging effects such as tumor reduction and long-term disease stability in some patients (222–225). However, challenges such as on-target/off-tumor toxicity and damage to other organs due to the lack of specificity of target antigens are seen. Identification of TSAs is one of the current challenges in CAR-T cell therapy (18). A second concern is cytokine release syndrome due to the CAR-T cells activation following binding to antigens in both tumor cells and normal cells (226).
The use of tumor-specific unmanipulated cells has also yielded positive results in CRC. In one study, tumor-infiltrating lymphocytes (TILs) were collected from metastatic lesions of a patient carrying KRAS-G12D mutation. The mutation-specific CD8+ T cells were selected and returned to the patient, resulting in the elimination of 85% of metastatic lesions (227). In another study, sentinel lymph node T was used instead of TILs, which resulted in complete response and disease stability in some patients and partial response in others (228, 229). The combination of ACT with chemotherapy and bevacizumab caused 80% overall response, 26.7% complete response, and stopped tumor progression in stage IV CRC patients (230). Various trials are investigating the effects of ACT alone or combined with other immunotherapies such as ICI in CRC patients (NCT03935893, NCT02757391, NCT01174121, NCT03904537). In these trials, various omics data are used to identify personalized antigens (146). These primary successes suggest that ACT, along with neoantigen vaccines, could be promising candidates for personalized immunotherapy in CRC.
4.3 Role of the Microbiome in Personalized Immunotherapy
Human microbiome is defined as all the microbiota in and on the human body plus their genomes, structural elements, and productions (231). The microbiota composition depends on genetic and environmental factors such as diet, exposure to chemicals, drugs, especially antibiotics, and is unique to each individual (232). Gut microbiota plays an essential role in the formation and regulation of gastrointestinal immune responses by producing various metabolites (233). Thus, dysbiosis in gut microbiota composition alters their metabolites such as short-chain fatty acids SCFAs, polyphenols, tryptophan catabolites, and some vitamins, and is associated with the pathogenesis of many diseases, including CRC (234). The researchers found several pathogen-derived proteins in the gastrointestinal tract of CRC patients (234, 235). They also showed that the high levels of antibodies against these proteins might have a diagnostic value for CRC patients (235, 236).
Besides, microbiota composition plays a critical role in patients’ treatment responses (237, 238). Presumably, the microbiota diversity among patients is one of the reasons for interpatient variety in response to cancer treatment (238). Microbiota can affect the response to chemotherapy. It has been observed that some bacteria metabolize and reduce the effects of chemotherapy at the tumor site (239). Besides, microbiota are chief players in regulating inflammatory responses in GI, through which they can influence the response of CRC patients to immunotherapy (240–242). Evidence suggests that exposure to antibiotics during anti-PD1 treatment alters the patients’ responses to anti-PD1 (241). Comparing the microbiota of patients who were well-responsive to anti-PD1 with those of patients who were poor-responsive to anti-PD1 showed that the presence of some bacteria (such as Akkermansia, Faecalibacterium, and Bifidobacterium) was associated with a better response to immunotherapy (238, 241). Contrarily, high levels of bacteria such as Bacteroidale are associated with a low response to anti-PD1 therapy (238, 241). This finding indicates that disruption of the microbiota network and reduction of beneficial bacteria reduce the patients’ responses to immunotherapy.
Interestingly, by manipulating the microbiota composition, responses to immunotherapy can be improved. Fecal microbiota transplant (FMT) is a well-known way to manipulate the network of GI microbiota. In FMT, the stool of patients who have responded well to treatment is transferred to the GI tract of non-responsive patients. Chen 2019 Matson 2021 Zhou 2021. It was observed that FMT of mice from anti-PD1-sensitive patients induced a favorable response to anti-PD1 in transplanted mice (241, 243). On the other hand, mice that received FMT from anti-PD1-resistant patients were still resistant to anti-PD1 after transplantation (241, 243). The use of microbiota in improving treatment response has entered clinical studies, and several clinical trials are investigating the combination of FMT with chemotherapy and immunotherapy (NCT04130763, NCT03782428). These studies, which are still in early phases, are investigating the role of FMT or probiotic supplements along with ICIs in CRC patients who have not previously responded well to immunotherapy.
In a nutshell, the evidence suggests that the microbiome is a determinant variable in immunotherapy and is involved in all aspects of CRC, including the diagnosis, prognosis, monitoring, and response to immunotherapy. Therefore, microbiomics should be used along with other omics data in optimizing personalized immunotherapy (238). In this era, molecular pathological epidemiology (MPE) has been recently developed to investigate the relationship between gut microbiota, host, environmental factors, diet, disease etiology and pathogenesis to investigate the role of microbiota and its factors in disease development and treatment response (244–246).
4.4 Combination Therapy
Although personalized immunotherapy approaches promise a new horizon in the treatment of CRC, longtime challenges of researchers with cancer treatment show that cancer is the most complex disease that is not supposed to cure with monotherapy (247). Regarding the high heterogeneity of CRC, it seems that it could escape even from personalized immunotherapy (44). Therefore, multi-aspect combinations of immunotherapy, chemotherapy, radiotherapy and other targeted therapies are required to fight cancers. It has been revealed that the combination of immunotherapies such as ICIs and mAbs with chemotherapy and radiotherapy could reinvigorate the antitumor responses and increase the patients’ survival (247). Personalized immunotherapy could also be combined with other therapeutic approaches to maximize the antitumor effects. In this regard, combination therapy using cancer vaccines (peptide, DNA, RNA, DC, and viral vector vaccines), adoptive cell therapy, ICIs, cytokines, chemotherapy, and radiotherapy increased antitumor effects leading to improved overall survival (151, 213, 227, 247–255). A phase I clinical trial of a neoantigen-encoding mRNA cancer vaccine combined with PD-1 blocking showed acceptable safety and well induction of neoantigen-specific immunity leading to partial and complete response in MSI-H CRC patients (189).
Interestingly, combination therapy is also better to be personalized to achieve the optimum response. The systems biology and simulation models can help find the best combination therapy in each patient. More recently, a quantitative systems pharmacology model predicted the efficacy of monotherapy with a bispecific T cell engager, a PD-L1 blocker, and combination therapy in CRC patients based on their individual characteristics (256). The development of these models could offer the best combination therapy for each patient to improve clinical efficacy.
5 Conclusion
The high heterogeneity in cancer patients has led to a paradigm shift that considers cancer as an individual rather than a general disease. Significant advances in immunotherapy have raised great hopes for the treatment of poor prognosis cancers. However, different responses of patients to immunotherapy necessitate the classification of patients into smaller groups so that eventually, each patient is in a separate classification and deserving personalized treatment. Personalized immunotherapy has various aspects generally based on specific neoantigens created in each individual’s tumor. Neoantigen-based personalized immunotherapy is now a time-consuming and costly method due to the need for up-to-date tools to identify and prioritize neoantigens. With the advancement of technologies such as sequencing and multi-omics, this type of treatment will be available quickly and affordably soon and will replace the current treatments.
Author Contributions
Both L-FH and H-RL equally contributed to writing the manuscript and sourcing references for the review. DH contributed to discussions and editing of the manuscript. X-ML and K-TJ conceived the outline of this paper and participated in critical review and further revision of the manuscript. All authors contributed to critical discussions and finalizing the manuscript before submission. They have all given approval to the final form of the manuscript.
Funding
This work was supported by Zhejiang Provincial Science and Technology Projects (grant no. LGD19H160001 to K-TJ).
Conflict of Interest
The authors declare that the research was conducted in the absence of any commercial or financial relationships that could be construed as a potential conflict of interest.
Publisher’s Note
All claims expressed in this article are solely those of the authors and do not necessarily represent those of their affiliated organizations, or those of the publisher, the editors and the reviewers. Any product that may be evaluated in this article, or claim that may be made by its manufacturer, is not guaranteed or endorsed by the publisher.
References
1. World Health Organization Cancer Statistics. (2021). Available at: https://www.who.int/news-room/fact-sheets/detail/cancer.
2. Ganesh K, Stadler ZK, Cercek A, Mendelsohn RB, Shia J, Segal NH, et al. Immunotherapy in Colorectal Cancer: Rationale, Challenges and Potential. Nat Rev Gastroenterol Hepatol (2019) 16(6):361–75. doi: 10.1038/s41575-019-0126-x
3. Siegel RL, Miller KD, Goding Sauer A, Fedewa SA, Butterly LF, Anderson JC, et al. Colorectal Cancer Statistics, 2020. CA: Cancer J Clin (2020) 70(3):145–64. doi: 10.3322/caac.21601
4. Mantovani G, De Angelis M, Asteria C, Di Lecce F, Mazza P, Vicenzi L, et al. Hereditary Nonpolyposis Colorectal Cancer (HNPCC): From Diagnosis to Surgical Management of the Main Hereditary Form of Colorectal Cancer: A Review. J Surg Res (2020) 3(1):20–30. doi: 10.26502/jsr.10020051
5. Yu IS, Cheung WY. Metastatic Colorectal Cancer in the Era of Personalized Medicine: A More Tailored Approach to Systemic Therapy. Can J Gastroenterol Hepatol (2018) 2018(9450754):11. doi: 10.1155/2018/9450754
6. Molinari C, Marisi G, Passardi A, Matteucci L, De Maio G, Ulivi P. Heterogeneity in Colorectal Cancer: A Challenge for Personalized Medicine? Int J Mol Sci (2018) 19(12):3733. doi: 10.3390/ijms19123733
7. Hansen TF, Qvortrup C, Pfeiffer P. Angiogenesis Inhibitors for Colorectal Cancer. A Review of the Clinical Data. Cancers (2021) 13(5):1031. doi: 10.3390/cancers13051031
8. Schumacher TN, Schreiber RD. Neoantigens in Cancer Immunotherapy. Science (2015) 348(6230):69–74. doi: 10.1126/science.aaa4971
9. Ibáñez-Sanz G, Sanz-Pamplona R, Garcia M, Group M-SR. Future Prospects of Colorectal Cancer Screening: Characterizing Interval Cancers. Cancers (2021) 13(6):1328. doi: 10.3390/cancers13061328
10. Network CGA. Comprehensive Molecular Characterization of Human Colon and Rectal Cancer. Nature (2012) 487(7407):330. doi: 10.1038/nature11252
11. Ahtiainen M, Elomaa H, Väyrynen JP, Wirta E-V, Kuopio T, Helminen O, et al. Immune Contexture of MMR-Proficient Primary Colorectal Cancer and Matched Liver and Lung Metastases. Cancers (2021) 13(7):1530. doi: 10.3390/cancers13071530
12. Kang S, Na Y, Joung SY, Lee SI, Oh SC, Min BW. The Significance of Microsatellite Instability in Colorectal Cancer After Controlling for Clinicopathological Factors. Medicine (2018) 97(9) 145–51. doi: 10.1097/MD.0000000000010019
13. Guinney J, Dienstmann R, Wang X, De Reynies A, Schlicker A, Soneson C, et al. The Consensus Molecular Subtypes of Colorectal Cancer. Nat Med (2015) 21(11):1350. doi: 10.1038/nm.3967
14. Palles C, Cazier J-B, Howarth KM, Domingo E, Jones AM, Broderick P, et al. Germline Mutations Affecting the Proofreading Domains of POLE and POLD1 Predispose to Colorectal Adenomas and Carcinomas. Nat Genet (2013) 45(2):136–44. doi: 10.1038/ng.2503
15. Wagner S, Mullins CS, Linnebacher M. Colorectal Cancer Vaccines: Tumor-Associated Antigens vs Neoantigens. World J Gastroenterol (2018) 24(48):5418. doi: 10.3748/wjg.v24.i48.5418
16. Vogelstein B, Papadopoulos N, Velculescu VE, Zhou S, Diaz LA, Kinzler KW. Cancer Genome Landscapes. Science (2013) 339(6127):1546–58. doi: 10.1126/science.1235122
17. Grady WM, Carethers JM. Genomic and Epigenetic Instability in Colorectal Cancer Pathogenesis. Gastroenterology (2008) 135(4):1079–99. doi: 10.1053/j.gastro.2008.07.076
18. Jin K-T, Chen B, Liu Y-Y, Yan J-P. Monoclonal Antibodies and Chimeric Antigen Receptor (CAR) T Cells in the Treatment of Colorectal Cancer. Cancer Cell Int (2021) 21(1):1–15. doi: 10.1186/s12935-021-01763-9
19. Barzaman K, Moradi-Kalbolandi S, Hosseinzadeh A, Kazemi MH, Khorramdelazad H, Safari E, et al. Breast Cancer Immunotherapy: Current and Novel Approaches. Int Immunopharmacol (2021) 98:107886. doi: 10.1016/j.intimp.2021.107886
20. Tosolini M, Kirilovsky A, Mlecnik B, Fredriksen T, Mauger S, Bindea G, et al. Clinical Impact of Different Classes of Infiltrating T Cytotoxic and Helper Cells (Th1, Th2, Treg, Th17) in Patients With Colorectal Cancer. Cancer Res (2011) 71(4):1263–71. doi: 10.1158/0008-5472.CAN-10-2907
21. Ge P, Wang W, Li L, Zhang G, Gao Z, Tang Z, et al. Profiles of Immune Cell Infiltration and Immune-Related Genes in the Tumor Microenvironment of Colorectal Cancer. Biomed Pharmacother (2019) 118:109228. doi: 10.1016/j.biopha.2019.109228
22. Ye L, Zhang T, Kang Z, Guo G, Sun Y, Lin K, et al. Tumor-Infiltrating Immune Cells Act as a Marker for Prognosis in Colorectal Cancer. Front Immunol (2019) 10:2368. doi: 10.3389/fimmu.2019.02368
23. Roelands J, Kuppen PJ, Vermeulen L, Maccalli C, Decock J, Wang E, et al. Immunogenomic Classification of Colorectal Cancer and Therapeutic Implications. Int J Mol Sci (2017) 18(10):2229. doi: 10.3390/ijms18102229
24. Marech I, Ammendola M, Sacco R, Sammarco G, Zuccalà V, Zizzo N, et al. Tumour-Associated Macrophages Correlate With Microvascular Bed Extension in Colorectal Cancer Patients. J Cell Mol Med (2016) 20(7):1373–80. doi: 10.1111/jcmm.12826
25. Lan J, Sun L, Xu F, Liu L, Hu F, Song D, et al. M2 Macrophage-Derived Exosomes Promote Cell Migration and Invasion in Colon Cancer. Cancer Res (2019) 79(1):146–58. doi: 10.1158/0008-5472.CAN-18-0014
26. Wei C, Yang C, Wang S, Shi D, Zhang C, Lin X, et al. M2 Macrophages Confer Resistance to 5-Fluorouracil in Colorectal Cancer Through the Activation of CCL22/PI3K/AKT Signaling. OncoTargets Ther (2019) 12:3051. doi: 10.2147/OTT.S198126
27. Fleming V, Hu X, Weber R, Nagibin V, Groth C, Altevogt P, et al. Targeting Myeloid-Derived Suppressor Cells to Bypass Tumor-Induced Immunosuppression. Front Immunol (2018) 9:398. doi: 10.3389/fimmu.2018.00398
28. Zhang Y, Rajput A, Jin N, Wang J. Mechanisms of Immunosuppression in Colorectal Cancer. Cancers (2020) 12(12):3850. doi: 10.3390/cancers12123850
29. Limagne E, Euvrard R, Thibaudin M, Rébé C, Derangère V, Chevriaux A, et al. Accumulation of MDSC and Th17 Cells in Patients With Metastatic Colorectal Cancer Predicts the Efficacy of a FOLFOX–bevacizumab Drug Treatment Regimen. Cancer Res (2016) 76(18):5241–52. doi: 10.1158/0008-5472.CAN-15-3164
30. Strasser K, Birnleitner H, Beer A, Pils D, Gerner MC, Schmetterer KG, et al. Immunological Differences Between Colorectal Cancer and Normal Mucosa Uncover a Prognostically Relevant Immune Cell Profile. Oncoimmunology (2019) 8(2):e1537693. doi: 10.1080/2162402X.2018.1537693
31. Wilczynski J, Duechler M, Czyz M. Targeting NF-κb and HIF-1 Pathways for the Treatment of Cancer: Part I. Archivum Immunol Ther Exp (2011) 59(4):289–99. doi: 10.1007/s00005-011-0131-4
32. De Simone M, Arrigoni A, Rossetti G, Gruarin P, Ranzani V, Politano C, et al. Transcriptional Landscape of Human Tissue Lymphocytes Unveils Uniqueness of Tumor-Infiltrating T Regulatory Cells. Immunity (2016) 45(5):1135–47. doi: 10.1016/j.immuni.2016.10.021
33. Parker JS, Mullins M, Cheang MC, Leung S, Voduc D, Vickery T, et al. Supervised Risk Predictor of Breast Cancer Based on Intrinsic Subtypes. J Clin Oncol (2009) 27(8):1160. doi: 10.1200/JCO.2008.18.1370
34. Vlad C, Kubelac P, Fetica B, Vlad D, Irimie A, Achimas-Cadariu P. The Prognostic Value of FOXP3+ T Regulatory Cells in Colorectal Cancer. J BUON (2015) 20(1):114–9.
35. Del Carmen S, Corchete LA, Gervas R, Rodriguez A, Garcia M, Álcazar JA, et al. Prognostic Implications of EGFR Protein Expression in Sporadic Colorectal Tumors: Correlation With Copy Number Status, mRNA Levels and miRNA Regulation. Sci Rep (2020) 10(1):1–16. doi: 10.1038/s41598-020-61688-7
36. Alagaratnam S, Loizidou M, Yang S-Y, Fuller B, Ramesh B. Increased Expression of IGF-1Ec With Increasing Colonic Polyp Dysplasia and Colorectal Cancer. J Cancer Res Clin Oncol (2020) 146(11):2861–70. doi: 10.1007/s00432-020-03345-0
37. Moradi Marjaneh R, Hassanian SM, Ghobadi N, Ferns GA, Karimi A, Jazayeri MH, et al. Targeting the Death Receptor Signaling Pathway as a Potential Therapeutic Target in the Treatment of Colorectal Cancer. J Cell Physiol (2018) 233(10):6538–49. doi: 10.1002/jcp.26640
38. Pothuraju R, Rachagani S, Krishn SR, Chaudhary S, Nimmakayala RK, Siddiqui JA, et al. Molecular Implications of MUC5AC-CD44 Axis in Colorectal Cancer Progression and Chemoresistance. Mol Cancer (2020) 19(1):1–14. doi: 10.1186/s12943-020-01156-y
39. Beaulieu J-F. Integrin α6β4 in Colorectal Cancer: Expression, Regulation, Functional Alterations and Use as a Biomarker. Cancers (2020) 12(1):41. doi: 10.3390/cancers12010041
40. Boudjadi S, Bernatchez G, Sénicourt B, Beauséjour M, Vachon PH, Carrier JC, et al. Involvement of the Integrin α1β1 in the Progression of Colorectal Cancer. Cancers (2017) 9(8):96. doi: 10.3390/cancers9080096
41. Wang X-Y, Zheng Z-X, Sun Y, Bai Y-H, Shi Y-F, Zhou L-X, et al. Significance of HER2 Protein Expression and HER2 Gene Amplification in Colorectal Adenocarcinomas. World J Gastrointest Oncol (2019) 11(4):335. doi: 10.4251/wjgo.v11.i4.335
42. Goulart A, Ferreira C, Rodrigues A, Coimbra B, Sousa N, Leão P. The Correlation Between Serum Vascular Endothelial Growth Factor (VEGF) and Tumor VEGF Receptor 3 in Colorectal Cancer. Ann Surg Treat Res (2019) 97(1):15. doi: 10.4174/astr.2019.97.1.15
43. Yonesaka K. HER2-/HER3-Targeting Antibody—Drug Conjugates for Treating Lung and Colorectal Cancers Resistant to EGFR Inhibitors. Cancers (2021) 13(5):1047. doi: 10.3390/cancers13051047
44. Shahnazari M, Samadi P, Pourjafar M, Jalali A. Therapeutic Vaccines for Colorectal Cancer: The Progress and Future Prospect. Int Immunopharmacol (2020) 88:106944. doi: 10.1016/j.intimp.2020.106944
45. Van Cutsem E, Cervantes A, Adam R, Sobrero A, Van Krieken J, Aderka D, et al. ESMO Consensus Guidelines for the Management of Patients With Metastatic Colorectal Cancer. Ann Oncol (2016) 27(8):1386–422. doi: 10.1093/annonc/mdw235
46. Baraniskin A, Buchberger B, Pox C, Graeven U, Holch JW, Schmiegel W, et al. Efficacy of Bevacizumab in First-Line Treatment of Metastatic Colorectal Cancer: A Systematic Review and Meta-Analysis. Eur J Cancer (2019) 106:37–44. doi: 10.1016/j.ejca.2018.10.009
47. Verdaguer H, Tabernero J, Macarulla T. Ramucirumab in Metastatic Colorectal Cancer: Evidence to Date and Place in Therapy. Ther Adv Med Oncol (2016) 8(3):230–42. doi: 10.1177/1758834016635888
48. Lee SH. Tanibirumab (TTAC-0001): A Fully Human Monoclonal Antibody Targets Vascular Endothelial Growth Factor Receptor 2 (VEGFR-2). Arch Pharm Res (2011) 34(8):1223–6. doi: 10.1007/s12272-011-0821-9
49. Kienast Y, Klein C, Scheuer W, Raemsch R, Lorenzon E, Bernicke D, et al. Ang-2-VEGF-A CrossMab, a Novel Bispecific Human IgG1 Antibody Blocking VEGF-A and Ang-2 Functions Simultaneously, Mediates Potent Antitumor, Antiangiogenic, and Antimetastatic Efficacy. Clin Cancer Res (2013) 19(24):6730–40. doi: 10.1158/1078-0432.CCR-13-0081
50. Van Cutsem E, Tabernero J, Lakomy R, Prenen H, Prausová J, Macarulla T, et al. Addition of Aflibercept to Fluorouracil, Leucovorin, and Irinotecan Improves Survival in a Phase III Randomized Trial in Patients With Metastatic Colorectal Cancer Previously Treated With an Oxaliplatin-Based Regimen. J Clin Oncol (2012) 30(28):3499–506. doi: 10.1200/JCO.2012.42.8201
51. Bokemeyer C, Bondarenko I, Hartmann J, De Braud F, Schuch G, Zubel A, et al. Efficacy According to Biomarker Status of Cetuximab Plus FOLFOX-4 as First-Line Treatment for Metastatic Colorectal Cancer: The OPUS Study. Ann Oncol (2011) 22(7):1535–46. doi: 10.1093/annonc/mdq632
52. Battaglin F, Puccini A, Djaballah SA, Lenz H-J. The Impact of Panitumumab Treatment on Survival and Quality of Life in Patients With RAS Wild-Type Metastatic Colorectal Cancer. Cancer Manage Res (2019) 11:5911. doi: 10.2147/CMAR.S186042
53. Elez E, Hendlisz A, Delaunoit T, Sastre J, Cervantes A, Varea R, et al. Phase II Study of Necitumumab Plus Modified FOLFOX6 as First-Line Treatment in Patients With Locally Advanced or Metastatic Colorectal Cancer. Br J Cancer (2016) 114(4):372–80. doi: 10.1038/bjc.2015.480
54. Allegra CJ, Rumble RB, Hamilton SR, Mangu PB, Roach N, Hantel A, et al. Extended RAS Gene Mutation Testing in Metastatic Colorectal Carcinoma to Predict Response to Anti–Epidermal Growth Factor Receptor Monoclonal Antibody Therapy: American Society of Clinical Oncology Provisional Clinical Opinion Update 2015. J Clin Oncol (2016) 34(2):179–85. doi: 10.1200/JCO.2015.63.9674
55. Peeters M, Oliner KS, Price TJ, Cervantes A, Sobrero AF, Ducreux M, et al. Analysis of KRAS/NRAS Mutations in a Phase III Study of Panitumumab With FOLFIRI Compared With FOLFIRI Alone as Second-Line Treatment for Metastatic Colorectal Cancer. Clin Cancer Res (2015) 21(24):5469–79. doi: 10.1158/1078-0432.CCR-15-0526
56. Sorich M, Wiese M, Rowland A, Kichenadasse G, McKinnon R, Karapetis C. Extended RAS Mutations and Anti-EGFR Monoclonal Antibody Survival Benefit in Metastatic Colorectal Cancer: A Meta-Analysis of Randomized, Controlled Trials. Ann Oncol (2015) 26(1):13–21. doi: 10.1093/annonc/mdu378
57. Karp DD, Paz-Ares LG, Novello S, Haluska P, Garland L, Cardenal F, et al. Phase II Study of the Anti–Insulin-Like Growth Factor Type 1 Receptor Antibody CP-751,871 in Combination With Paclitaxel and Carboplatin in Previously Untreated, Locally Advanced, or Metastatic Non–Small-Cell Lung Cancer. J Clin Oncol (2009) 27(15):2516–22. doi: 10.1200/JCO.2008.19.9331
58. Hajifathali A, Parkhideh S, Kazemi MH, Chegeni R, Roshandel E, Gholizadeh M. Immune Checkpoints in Hematologic Malignancies: What Made the Immune Cells and Clinicians Exhausted! J Cell Physiol (2020) 235(12):9080–97. doi: 10.1002/jcp.29769
59. Kazemi MH, Najafi A, Karami J, Ghazizadeh F, Yousefi H, Falak R, et al. Immune and Metabolic Checkpoints Blockade: Dual Wielding Against Tumors. Int Immunopharmacol (2021) 94:107461. doi: 10.1016/j.intimp.2021.107461
60. Chung KY, Gore I, Fong L, Venook A, Beck SB, Dorazio P, et al. Phase II Study of the Anti-Cytotoxic T-Lymphocyte–Associated Antigen 4 Monoclonal Antibody, Tremelimumab, in Patients With Refractory Metastatic Colorectal Cancer. J Clin Oncol (2010) 28(21):3485–90. doi: 10.1200/JCO.2010.28.3994
61. Morse MA, Hochster H, Benson A. Perspectives on Treatment of Metastatic Colorectal Cancer With Immune Checkpoint Inhibitor Therapy. Oncol (2020) 25(1):33. doi: 10.1634/theoncologist.2019-0176
62. Cohen R, Colle R, Pudlarz T, Heran M, Duval A, Svrcek M, et al. Immune Checkpoint Inhibition in Metastatic Colorectal Cancer Harboring Microsatellite Instability or Mismatch Repair Deficiency. Cancers (2021) 13(5):1149. doi: 10.3390/cancers1305114
63. Lin A, Zhang J, Luo P. Crosstalk Between the MSI Status and Tumor Microenvironment in Colorectal Cancer. Front Immunol (2020) 11:2039. doi: 10.3389/fimmu.2020.02039
64. Picard E, Verschoor CP, Ma GW, Pawelec G. Relationships Between Immune Landscapes, Genetic Subtypes and Responses to Immunotherapy in Colorectal Cancer. Front Immunol (2020) 11:369. doi: 10.3389/fimmu.2020.00369
65. Le DT, Durham JN, Smith KN, Wang H, Bartlett BR, Aulakh LK, et al. Mismatch Repair Deficiency Predicts Response of Solid Tumors to PD-1 Blockade. Science (2017) 357(6349):409–13. doi: 10.1126/science.aan6733
66. André T, Shiu K-K, Kim TW, Jensen BV, Jensen LH, Punt C, et al. Pembrolizumab in Microsatellite-Instability–High Advanced Colorectal Cancer. N Engl J Med (2020) 383(23):2207–18. doi: 10.1056/NEJMoa2017699
67. Domingo E, Freeman-Mills L, Rayner E, Glaire M, Briggs S, Vermeulen L, et al. Somatic POLE Proofreading Domain Mutation, Immune Response, and Prognosis in Colorectal Cancer: A Retrospective, Pooled Biomarker Study. Lancet Gastroenterol Hepatol (2016) 1(3):207–16. doi: 10.1016/S2468-1253(16)30014-0
68. Overman MJ, Lonardi S, Wong KYM, Lenz H-J, Gelsomino F, Aglietta M, et al. Durable Clinical Benefit With Nivolumab Plus Ipilimumab in DNA Mismatch Repair-Deficient/Microsatellite Instability-High Metastatic Colorectal Cancer. J Clin Onco (2018) 36:773–9. doi: 10.1200/JCO.2017.76.9901
69. Martin-Romano P, Ammari S, El-Dakdoukti Y, Baldini C, Varga A, Vuagnat P, et al. Chemotherapy Beyond Immune Checkpoint Inhibitors in Patients With Metastatic Colorectal Cancer. Eur J Cancer (2020) 137:117–26. doi: 10.1016/j.ejca.2020.06.030
70. Pfirschke C, Engblom C, Rickelt S, Cortez-Retamozo V, Garris C, Pucci F, et al. Immunogenic Chemotherapy Sensitizes Tumors to Checkpoint Blockade Therapy. Immunity (2016) 44(2):343–54. doi: 10.1016/j.immuni.2015.11.024
71. Wallin J, Pishvaian MJ, Hernandez G, Yadav M, Jhunjhunwala S, Delamarre L, et al. Clinical Activity and Immune Correlates From a Phase Ib Study Evaluating Atezolizumab (Anti-PDL1) in Combination With FOLFOX and Bevacizumab (Anti-VEGF) in Metastatic Colorectal Carcinoma. AACR (2016) 76:215–21. doi: 10.1158/1538-7445.AM2016-2651
72. Park SS, Dong H, Liu X, Harrington SM, Krco CJ, Grams MP, et al. PD-1 Restrains Radiotherapy-Induced Abscopal Effect. Cancer Immunol Res (2015) 3(6):610–9. doi: 10.1158/2326-6066.CIR-14-0138
73. Bendell JC, Bang Y-J, Chee CE, Ryan DP, McRee AJ, Chow LQ, et al. A Phase Ib Study of Safety and Clinical Activity of Atezolizumab (A) and Cobimetinib (C) in Patients (Pts) With Metastatic Colorectal Cancer (mCRC). Am Soc Clin Oncol (2018) 36, 132–141. doi: 10.1200/JCO.2018.36.4_suppl.560
74. Shin MH, Kim J, Lim SA, Kim J, Lee K-M. Current Insights Into Combination Therapies With MAPK Inhibitors and Immune Checkpoint Blockade. Int J Mol Sci (2020) 21(7):2531. doi: 10.3390/ijms21072531
75. Ebert PJ, Cheung J, Yang Y, McNamara E, Hong R, Moskalenko M, et al. MAP Kinase Inhibition Promotes T Cell and Anti-Tumor Activity in Combination With PD-L1 Checkpoint Blockade. Immunity (2016) 44(3):609–21. doi: 10.1016/j.immuni.2016.01.024
76. Segal N, Saro J, Melero I, Ros W, Argiles G, Marabelle A, et al. Phase I Studies of the Novel Carcinoembryonic Antigen T-Cell Bispecific (CEA-CD3 TCB) Antibody as a Single Agent and in Combination With Atezolizumab: Preliminary Efficacy and Safety in Patients (Pts) With Metastatic Colorectal Cancer (mCRC). Ann Oncol (2017) 28:v134. doi: 10.1093/annonc/mdx367.036
77. Sanborn RE, Pishvaian MJ, Callahan MK, Weise AM, Sikic BI, Rahma OE, et al. Anti-CD27 Agonist Antibody Varlilumab (Varli) With Nivolumab (Nivo) for Colorectal (CRC) and Ovarian (OVA) Cancer: Phase (Ph) 1/2 Clinical Trial Results. Am Soc Clin Oncol (2018) 36:525–31. doi: 10.1200/JCO.2018.36.15_suppl.3001
78. Sawada R, Arai Y, Sagawa Y, Nagata Y, Nishimura T, Noguchi M, et al. High Blood Levels of Soluble OX40 (CD134), an Immune Costimulatory Molecule, Indicate Reduced Survival in Patients With Advanced Colorectal Cancer. Oncol Rep (2019) 42(5):2057–64. doi: 10.3892/or.2019.7304
79. Piha-Paul S, Bendell J, Tolcher A, Hurvitz S, Patnaik A, Shroff R, et al. O82 A Phase 1 Dose Escalation Study of PRS-343, a HER2/4–1BB Bispecific Molecule, in Patients With HER2-Positive Malignancies. BMJ Spec J (2020) 8:354–6. doi: 10.1136/LBA2019.2
80. Rakké Y, Carrascosa LC, van Beek A, de Ruiter V, Doukas M, ter Borg S, et al. 588 Targeting GITR Enhances Human Tumour-Infiltrating T Cell Functionality in Mismatch Repair Proficient Primary Colorectal Carcinoma and Liver Metastases. BMJ Spec J (2020) 8:559. doi: 10.1136/jitc-2020-SITC2020.0588
81. Manji G, Bahary N, Chung V, Dalenc F, Ducreux M, Gomez-Roca C, et al. 259 Phase Ib/II Open-Label, Randomized Evaluation of Atezolizumab (Atezo)+ Selicrelumab (Seli)+ Gemcitabine+ Nab-Paclitaxel (Gem+ Nabp) or Bevacizumab (Bev) vs Control in MORPHEUS-PDAC,-TNBC and-CRC. BMJ Spec J (2020) 8:157–8. doi: 10.1136/jitc-2020-SITC2020.0259
82. Xie S, Huang J, Qiao Q, Zang W, Hong S, Tan H, et al. Expression of the Inhibitory B7 Family Molecule VISTA in Human Colorectal Carcinoma Tumors. Cancer Immunol Immunother (2018) 67(11):1685–94. doi: 10.1007/s00262-018-2227-8
83. Blessin NC, Simon R, Kluth M, Fischer K, Hube-Magg C, Li W, et al. Patterns of TIGIT Expression in Lymphatic Tissue, Inflammation, and Cancer. Dis Markers (2019) 2019(5160565):13. doi: 10.1155/2019/5160565
84. Kang C-W, Dutta A, Chang L-Y, Mahalingam J, Lin Y-C, Chiang J-M, et al. Apoptosis of Tumor Infiltrating Effector TIM-3+ CD8+ T Cells in Colon Cancer. Sci Rep (2015) 5(1):1–12. doi: 10.1038/srep15659
85. Gurjao C, Liu D, Hofree M, AlDubayan SH, Wakiro I, Su M-J, et al. Intrinsic Resistance to Immune Checkpoint Blockade in a Mismatch Repair–Deficient Colorectal Cancer. Cancer Immunol Res (2019) 7(8):1230–6. doi: 10.1158/2326-6066.CIR-18-0683
86. Eng C, Kim TW, Bendell J, Argilés G, Tebbutt NC, Di Bartolomeo M, et al. Atezolizumab With or Without Cobimetinib Versus Regorafenib in Previously Treated Metastatic Colorectal Cancer (IMblaze370): A Multicentre, Open-Label, Phase 3, Randomised, Controlled Trial. Lancet Oncol (2019) 20(6):849–61. doi: 10.1016/S1470-2045(19)30027-0
87. Yeon SY, Jung S-H, Jo YS, Choi EJ, Kim MS, Chung Y-J, et al. Immune Checkpoint Blockade Resistance-Related B2M Hotspot Mutations in Microsatellite-Unstable Colorectal Carcinoma. Pathol Res Pract (2019) 215(1):209–14. doi: 10.1016/j.prp.2018.11.014
88. Sveen A, Johannessen B, Tengs T, Danielsen SA, Eilertsen IA, Lind GE, et al. Multilevel Genomics of Colorectal Cancers With Microsatellite Instability—Clinical Impact of JAK1 Mutations and Consensus Molecular Subtype 1. Genome Med (2017) 9(1):1–16. doi: 10.1186/s13073-017-0434-0
89. Garcia-Diaz A, Shin DS, Moreno BH, Saco J, Escuin-Ordinas H, Rodriguez GA, et al. Interferon Receptor Signaling Pathways Regulating PD-L1 and PD-L2 Expression. Cell Rep (2017) 19(6):1189–201. doi: 10.1016/j.celrep.2017.04.031
90. Ogino S, Nowak JA, Hamada T, Phipps AI, Peters U, Milner DA Jr., et al. Integrative Analysis of Exogenous, Endogenous, Tumour and Immune Factors for Precision Medicine. Gut (2018) 67(6):1168–80. doi: 10.1136/gutjnl-2017-315537
91. Bramsen JB, Rasmussen MH, Ongen H, Mattesen TB, Ørntoft M-BW, Arnadottir SS, et al. Molecular-Subtype-Specific Biomarkers Improve Prediction of Prognosis in Colorectal Cancer. Cell Rep (2017) 19(6):1268–80. doi: 10.1016/j.celrep.2017.04.045
92. Wierdak M, Surmiak M, Milian-Ciesielska K, Rubinkiewicz M, Rzepa A, Wysocki M, et al. Immunonutrition Changes Inflammatory Response in Colorectal Cancer: Results From a Pilot Randomized Clinical Trial. Cancers (2021) 13(6):1444. doi: 10.3390/cancers13061444
93. Perna C, Navarro A, Ruz-Caracuel I, Caniego-Casas T, Cristóbal E, Leskelä S, et al. Molecular Heterogeneity of High Grade Colorectal Adenocarcinoma. Cancers (2021) 13(2):233. doi: 10.3390/cancers13020233
94. Yokota T. Are KRAS/BRAF Mutations Potent Prognostic and/or Predictive Biomarkers in Colorectal Cancers? Anti Cancer Agents Med Chem (Formerly Curr Med Chem Anti Cancer Agents) (2012) 12(2):163–71. doi: 10.2174/187152012799014968
95. Mogensen M, Rossing M, Østrup O, Larsen P, Engel PH, Jørgensen LN, et al. Genomic Alterations Accompanying Tumour Evolution in Colorectal Cancer: Tracking the Differences Between Primary Tumours and Synchronous Liver Metastases by Whole-Exome Sequencing. BMC Cancer (2018) 18(1):1–13. doi: 10.1186/s12885-018-4639-4
96. Ulivi P, Scarpi E, Chiadini E, Marisi G, Valgiusti M, Capelli L, et al. Right-Vs. Left-Sided Metastatic Colorectal Cancer: Differences in Tumor Biology and Bevacizumab Efficacy. Int J Mol Sci (2017) 18(6):1240. doi: 10.3390/ijms18061240
97. Tejpar S, Stintzing S, Ciardiello F, Tabernero J, Van Cutsem E, Beier F, et al. Prognostic and Predictive Relevance of Primary Tumor Location in Patients With RAS Wild-Type Metastatic Colorectal Cancer: Retrospective Analyses of the CRYSTAL and FIRE-3 Trials. JAMA Oncol (2017) 3(2):194–201. doi: 10.1001/jamaoncol.2016.3797
98. Walter RJ, Sonnentag SJ, Orian-Rousseau V, Munoz-Sagredo L. Plasticity in Colorectal Cancer: Why Cancer Cells Differentiate. Cancers (2021) 13(4):918. doi: 10.3390/cancers13040918
99. Kreso A, O'Brien CA, Van Galen P, Gan OI, Notta F, Brown AM, et al. Variable Clonal Repopulation Dynamics Influence Chemotherapy Response in Colorectal Cancer. Science (2013) 339(6119):543–8. doi: 10.1126/science.1227670
100. Sveen A, Løes IM, Alagaratnam S, Nilsen G, Høland M, Lingjærde OC, et al. Intra-Patient Inter-Metastatic Genetic Heterogeneity in Colorectal Cancer as a Key Determinant of Survival After Curative Liver Resection. PLoS Genet (2016) 12(7):e1006225. doi: 10.1371/journal.pgen.1006225
101. Jones HG, Jenkins G, Williams N, Griffiths P, Chambers P, Beynon J, et al. Genetic and Epigenetic Intra-Tumour Heterogeneity in Colorectal Cancer. World J Surg (2017) 41(5):1375–83. doi: 10.1007/s00268-016-3860-z
102. Jeantet M, Tougeron D, Tachon G, Cortes U, Archambaut C, Fromont G, et al. High Intra-and Inter-Tumoral Heterogeneity of RAS Mutations in Colorectal Cancer. Int J Mol Sci (2016) 17(12):2015. doi: 10.3390/ijms17122015
103. Farber L, Efrati E, Elkin H, Peerless Y, Sabo E, Ben-Izhak O, et al. Molecular Morphometric Analysis Shows Relative Intra-Tumoural Homogeneity for KRAS Mutations in Colorectal Cancer. Virchows Arch (2011) 459(5):487–93. doi: 10.1007/s00428-011-1158-y
104. Baisse B, Bouzourene H, Saraga EP, Bosman FT, Benhattar J. Intratumor Genetic Heterogeneity in Advanced Human Colorectal Adenocarcinoma. Int J Cancer (2001) 93(3):346–52. doi: 10.1002/ijc.1343
105. Losi L, Baisse B, Bouzourene H, Benhattar J. Evolution of Intratumoral Genetic Heterogeneity During Colorectal Cancer Progression. Carcinogenesis (2005) 26(5):916–22. doi: 10.1093/carcin/bgi044
106. Fisher R, Pusztai L, Swanton C. Cancer Heterogeneity: Implications for Targeted Therapeutics. Br J Cancer (2013) 108(3):479–85. doi: 10.1038/bjc.2012.581
107. Komor MA, Bosch LJ, Bounova G, Bolijn AS, Delis-van Diemen PM, Rausch C, et al. Consensus Molecular Subtype Classification of Colorectal Adenomas. J Pathol (2018) 246(3):266–76. doi: 10.1002/path.5129
108. Karpinski P, Rossowska J, Sasiadek MM. Immunological Landscape of Consensus Clusters in Colorectal Cancer. Oncotarget (2017) 8(62):105299. doi: 10.18632/oncotarget.22169
109. Linnekamp JF, van Hooff SR, Prasetyanti PR, Kandimalla R, Buikhuisen JY, Fessler E, et al. Consensus Molecular Subtypes of Colorectal Cancer are Recapitulated in In Vitro and In Vivo Models. Cell Death Differ (2018) 25(3):616–33. doi: 10.1038/s41418-017-0011-5
110. Giannakis M, Mu XJ, Shukla SA, Qian ZR, Cohen O, Nishihara R, et al. Genomic Correlates of Immune-Cell Infiltrates in Colorectal Carcinoma. Cell Rep (2016) 15(4):857–65. doi: 10.1016/j.celrep.2016.03.075
111. Angell HK, Bruni D, Barrett JC, Herbst R, Galon J. The Immunoscore: Colon Cancer and Beyond. Clin Cancer Res (2020) 26(2):332–9. doi: 10.1158/1078-0432.CCR-18-1851
112. Galon J, Mlecnik B, Bindea G, Angell HK, Berger A, Lagorce C, et al. Towards the Introduction of the ‘Immunoscore’ in the Classification of Malignant Tumours. J Pathol (2014) 232(2):199–209. doi: 10.1002/path.4287
113. El Sissy C, Kirilovsky A, Zeitoun G, Marliot F, Haicheur N, Lagorce-Pagès C, et al. Therapeutic Implications of the Immunoscore in Patients With Colorectal Cancer. Cancers (2021) 13(6):1281. doi: 10.3390/cancers13061281
114. Galon J, Costes A, Sanchez-Cabo F, Kirilovsky A, Mlecnik B, Lagorce-Pagès C, et al. Type, Density, and Location of Immune Cells Within Human Colorectal Tumors Predict Clinical Outcome. Science (2006) 313(5795):1960–4. doi: 10.1126/science.1129139
115. Pagès F, Mlecnik B, Marliot F, Bindea G, Ou F-S, Bifulco C, et al. International Validation of the Consensus Immunoscore for the Classification of Colon Cancer: A Prognostic and Accuracy Study. Lancet (2018) 391(10135):2128–39. doi: 10.1016/S0140-6736(18)30789-X
116. Middha S, Zhang L, Nafa K, Jayakumaran G, Wong D, Kim HR, et al. Reliable Pan-Cancer Microsatellite Instability Assessment by Using Targeted Next-Generation Sequencing Data. JCO Precis Oncol (2017) 1:1–17. doi: 10.1200/PO.17.00084
117. Li C, Sun Y-D, Yu G-Y, Cui J-R, Lou Z, Zhang H, et al. Integrated Omics of Metastatic Colorectal Cancer. Cancer Cell (2020) 38(5):734–47.e9. doi: 10.1016/j.ccell.2020.08.002
118. Xu X, Gong C, Wang Y, Hu Y, Liu H, Fang Z. Multi-Omics Analysis to Identify Driving Factors in Colorectal Cancer. Epigenomics (2020) 12(18):1633–50. doi: 10.2217/epi-2020-0073
119. Wang W, Zhong Y, Zhuang Z, Xie J, Lu Y, Huang C, et al. Multiregion Single-Cell Sequencing Reveals the Transcriptional Landscape of the Immune Microenvironment of Colorectal Cancer. Clin Trans Med (2021) 11(1):e253. doi: 10.1002/ctm2.253
120. Skopelitou D, Miao B, Srivastava A, Kumar A, Kuswick M, Dymerska D, et al. Whole Exome Sequencing Identifies APCDD1 and HDAC5 Genes as Potentially Cancer Predisposing in Familial Colorectal Cancer. Int J Mol Sci (2021) 22(4):1837. doi: 10.3390/ijms22041837
121. Wang W, Kandimalla R, Huang H, Zhu L, Li Y, Gao F, et al. Molecular Subtyping of Colorectal Cancer: Recent Progress, New Challenges and Emerging Opportunities. Semin Cancer Biol (2019) 55:37–52. doi: 10.1016/j.semcancer.2018.05.002
122. Soares de Lima Y, Arnau-Collell C, Díaz-Gay M, Bonjoch L, Franch-Expósito S, Muñoz J, et al. Germline and Somatic Whole-Exome Sequencing Identifies New Candidate Genes Involved in Familial Predisposition to Serrated Polyposis Syndrome. Cancers (2021) 13(4):929. doi: 10.3390/cancers13040929
123. Ali SR, Orang A, Marri S, McKinnon RA, Meech R, Michael MZ. Integrative Transcriptomic Network Analysis of Butyrate Treated Colorectal Cancer Cells. Cancers (2021) 13(4):636. doi: 10.3390/cancers13040636
124. Aziz MA, Yousef Z, Saleh AM, Mohammad S, Al Knawy B. Towards Personalized Medicine of Colorectal Cancer. Crit Rev Oncol Hematol (2017) 118:70–8. doi: 10.1016/j.critrevonc.2017.08.007
125. Anaya DA, Dogra P, Wang Z, Haider M, Ehab J, Jeong DK, et al. A Mathematical Model to Estimate Chemotherapy Concentration at the Tumor-Site and Predict Therapy Response in Colorectal Cancer Patients With Liver Metastases. Cancers (2021) 13(3):444. doi: 10.3390/cancers13030444
126. Hildebrand LA, Pierce CJ, Dennis M, Paracha M, Maoz A. Artificial Intelligence for Histology-Based Detection of Microsatellite Instability and Prediction of Response to Immunotherapy in Colorectal Cancer. Cancers (2021) 13(3):391. doi: 10.3390/cancers13030391
127. Agren R, Mardinoglu A, Asplund A, Kampf C, Uhlen M, Nielsen J. Identification of Anticancer Drugs for Hepatocellular Carcinoma Through Personalized Genome-Scale Metabolic Modeling. Mol Syst Biol (2014) 10(3):721. doi: 10.1002/msb.145122
128. Mardinoglu A, Nielsen J. Systems Medicine and Metabolic Modelling. J Internal Med (2012) 271(2):142–54. doi: 10.1111/j.1365-2796.2011.02493.x
129. Henderson D, Ogilvie LA, Hoyle N, Keilholz U, Lange B, Lehrach H, et al. Personalized Medicine Approaches for Colon Cancer Driven by Genomics and Systems Biology: OncoTrack. Biotechnol J (2014) 9(9):1104–14. doi: 10.1002/biot.201400109
130. Baart GJ, Martens DE. Genome-Scale Metabolic Models: Reconstruction and Analysis. In: Neisseria Meningitidis. Springer (2012). p. 107–26. doi: 10.1007/978-1-61779-346-2_7
131. Reidy E, Leonard NA, Treacy O, Ryan AE. A 3d View of Colorectal Cancer Models in Predicting Therapeutic Responses and Resistance. Cancers (2021) 13(2):227. doi: 10.3390/cancers13020227
132. Kondo Y, Hayashi K, Kawakami K, Miwa Y, Hayashi H, Yamamoto M. KRAS Mutation Analysis of Single Circulating Tumor Cells From Patients With Metastatic Colorectal Cancer. BMC Cancer (2017) 17(1):1–10. doi: 10.1186/s12885-017-3305-6
133. Denis JA, Patroni A, Guillerm E, Pépin D, Benali-Furet N, Wechsler J, et al. Droplet Digital PCR of Circulating Tumor Cells From Colorectal Cancer Patients Can Predict KRAS Mutations Before Surgery. Mol Oncol (2016) 10(8):1221–31. doi: 10.1016/j.molonc.2016.05.009
134. Vidal J, Muinelo L, Dalmases A, Jones F, Edelstein D, Iglesias M, et al. Plasma ctDNA RAS Mutation Analysis for the Diagnosis and Treatment Monitoring of Metastatic Colorectal Cancer Patients. Ann Oncol (2017) 28(6):1325–32. doi: 10.1093/annonc/mdx125
135. Grasselli J, Elez E, Caratù G, Matito J, Santos C, Macarulla T, et al. Concordance of Blood-and Tumor-Based Detection of RAS Mutations to Guide Anti-EGFR Therapy in Metastatic Colorectal Cancer. Ann Oncol (2017) 28(6):1294–301. doi: 10.1093/annonc/mdx112
136. Schmiegel W, Scott RJ, Dooley S, Lewis W, Meldrum CJ, Pockney P, et al. Blood-Based Detection of RAS Mutations to Guide Anti-EGFR Therapy in Colorectal Cancer Patients: Concordance of Results From Circulating Tumor DNA and Tissue-Based RAS Testing. Mol Oncol (2017) 11(2):208–19. doi: 10.1002/1878-0261.12023
137. Ruiz-López L, Blancas I, Garrido JM, Mut-Salud N, Moya-Jódar M, Osuna A, et al. The Role of Exosomes on Colorectal Cancer: A Review. J Gastroenterol Hepatol (2018) 33(4):792–9. doi: 10.1111/jgh.14049
138. Jia S, Zhang R, Li Z, Li J. Clinical and Biological Significance of Circulating Tumor Cells, Circulating Tumor DNA, and Exosomes as Biomarkers in Colorectal Cancer. Oncotarget (2017) 8(33):55632. doi: 10.18632/oncotarget.17184
139. Weeber F, Ooft SN, Dijkstra KK, Voest EE. Tumor Organoids as a Pre-Clinical Cancer Model for Drug Discovery. Cell Chem Biol (2017) 24(9):1092–100. doi: 10.1016/j.chembiol.2017.06.012
140. Vlachogiannis G, Hedayat S, Vatsiou A, Jamin Y, Fernández-Mateos J, Khan K, et al. Patient-Derived Organoids Model Treatment Response of Metastatic Gastrointestinal Cancers. Science (2018) 359(6378):920–6. doi: 10.1126/science.aao2774
141. Pasch CA, Favreau PF, Yueh AE, Babiarz CP, Gillette AA, Sharick JT, et al. Patient-Derived Cancer Organoid Cultures to Predict Sensitivity to Chemotherapy and Radiation. Clin Cancer Res (2019) 25(17):5376–87. doi: 10.1158/1078-0432.CCR-18-3590
142. Ooft SN, Weeber F, Dijkstra KK, McLean CM, Kaing S, van Werkhoven E, et al. Patient-Derived Organoids Can Predict Response to Chemotherapy in Metastatic Colorectal Cancer Patients. Sci Trans Med (2019) 11(513): 256–65. doi: 10.1126/scitranslmed.aay2574
143. Weeber F, van de Wetering M, Hoogstraat M, Dijkstra KK, Krijgsman O, Kuilman T, et al. Preserved Genetic Diversity in Organoids Cultured From Biopsies of Human Colorectal Cancer Metastases. Proc Natl Acad Sci (2015) 112(43):13308–11. doi: 10.1073/pnas.1516689112
144. van de Wetering M, Francies HE, Francis JM, Bounova G, Iorio F, Pronk A, et al. Prospective Derivation of a Living Organoid Biobank of Colorectal Cancer Patients. Cell (2015) 161(4):933–45. doi: 10.1016/j.cell.2015.03.053
145. Neal JT, Li X, Zhu J, Giangarra V, Grzeskowiak CL, Ju J, et al. Organoid Modeling of the Tumor Immune Microenvironment. Cell (2018) 175(7):1972–88. e16. doi: 10.1016/j.cell.2018.11.021
146. Rus Bakarurraini NAA, Ab Mutalib NS, Jamal R, Abu N. The Landscape of Tumor-Specific Antigens in Colorectal Cancer. Vaccines (2020) 8(3):371. doi: 10.3390/vaccines8030371
147. Guo M, Luo B, Pan M, Li M, Zhao F, Dou J. MUC1 Plays an Essential Role in Tumor Immunity of Colorectal Cancer Stem Cell Vaccine. Int Immunopharmacol (2020) 85:106631. doi: 10.1016/j.intimp.2020.106631
148. Sabeerabi B, Tatireddygari VRA, Vadde R. Therapeutic Vaccines for Gastrointestinal Malignancies. Immunother Gastrointest Malig: Springer (2020) p:113–58. doi: 10.1007/978-981-15-6487-1_8
149. Sprooten J, Ceusters J, Coosemans A, Agostinis P, De Vleeschouwer S, Zitvogel L, et al. Trial Watch: Dendritic Cell Vaccination for Cancer Immunotherapy. Oncoimmunology (2019) 8(11):1638212. doi: 10.1080/2162402X.2019.1638212
150. Hazama S, Nakamura Y, Takenouchi H, Suzuki N, Tsunedomi R, Inoue Y, et al. A Phase I Study of Combination Vaccine Treatment of Five Therapeutic Epitope-Peptides for Metastatic Colorectal Cancer; Safety, Immunological Response, and Clinical Outcome. J Trans Med (2014) 12(1):1–11. doi: 10.1186/1479-5876-12-63
151. Okuno K, Sugiura F, Inoue K, Sukegawa Y. Clinical Trial of a 7-Peptide Cocktail Vaccine With Oral Chemotherapy for Patients With Metastatic Colorectal Cancer. Anticancer Res (2014) 34(6):3045–52.
152. Taniguchi H, Iwasa S, Yamazaki K, Yoshino T, Kiryu C, Naka Y, et al. Phase 1 Study of OCV-C02, a Peptide Vaccine Consisting of Two Peptide Epitopes for Refractory Metastatic Colorectal Cancer. Cancer Sci (2017) 108(5):1013–21. doi: 10.1111/cas.13227
153. Kwon S, Kim Y-E, Park J-A, Kim D-S, Kwon H-J, Lee Y. Therapeutic Effect of a TM4SF5-Specific Peptide Vaccine Against Colon Cancer in a Mouse Model. BMB Rep (2014) 47(4):215. doi: 10.5483/BMBRep.2014.47.4.157
154. Ahsan A, Ramanand SG, Bergin IL, Zhao L, Whitehead CE, Rehemtulla A, et al. Efficacy of an EGFR-Specific Peptide Against EGFR-Dependent Cancer Cell Lines and Tumor Xenografts. Neoplasia (2014) 16(2):105–W2. doi: 10.1593/neo.14182
155. Shimodaira S, Sano K, Hirabayashi K, Koya T, Higuchi Y, Mizuno Y, et al. Dendritic Cell-Based Adjuvant Vaccination Targeting Wilms’ Tumor 1 in Patients With Advanced Colorectal Cancer. Vaccines (2015) 3(4):1004–18. doi: 10.3390/vaccines3041004
156. Kimura T, McKolanis JR, Dzubinski LA, Islam K, Potter DM, Salazar AM, et al. MUC1 Vaccine for Individuals With Advanced Adenoma of the Colon: A Cancer Immunoprevention Feasibility Study. Cancer Prev Res (2013) 6(1):18–26. doi: 10.1158/1940-6207.CAPR-12-0275
157. Lisby AN, Flickinger JC Jr., Bashir B, Weindorfer M, Shelukar S, Crutcher M, et al. GUCY2C as a Biomarker to Target Precision Therapies for Patients With Colorectal Cancer. Expert Rev Precis Med Drug Dev (2021) 6:1–13. doi: 10.1080/23808993.2021.187651
158. Miyagi Y, Imai N, Sasatomi T, Yamada A, Mine T, Katagiri K, et al. Induction of Cellular Immune Responses to Tumor Cells and Peptides in Colorectal Cancer Patients by Vaccination With SART3 Peptides. Clin Cancer Res (2001) 7(12):3950–62.
159. Besneux M, Greenshields-Watson A, Scurr MJ, MacLachlan BJ, Christian A, Davies MM, et al. The Nature of the Human T Cell Response to the Cancer Antigen 5T4 Is Determined by the Balance of Regulatory and Inflammatory T Cells of the Same Antigen-Specificity: Implications for Vaccine Design. Cancer Immunol Immunother (2019) 68(2):247–56. doi: 10.1007/s00262-018-2266-1
160. Kameshima H, Tsuruma T, Torigoe T, Takahashi A, Hirohashi Y, Tamura Y, et al. Immunogenic Enhancement and Clinical Effect by Type-I Interferon of Anti-Apoptotic Protein, Survivin-Derived Peptide Vaccine, in Advanced Colorectal Cancer Patients. Cancer Sci (2011) 102(6):1181–7. doi: 10.1111/j.1349-7006.2011.01918.x
161. Wirth TC, Kühnel F. Neoantigen Targeting—Dawn of a New Era in Cancer Immunotherapy? Front Immunol (2017) 8:1848. doi: 10.3389/fimmu.2017.01848
162. Brown SD, Warren RL, Gibb EA, Martin SD, Spinelli JJ, Nelson BH, et al. Neo-Antigens Predicted by Tumor Genome Meta-Analysis Correlate With Increased Patient Survival. Genome Res (2014) 24(5):743–50. doi: 10.1101/gr.165985.113
163. Melief CJ, van der Burg SH. Immunotherapy of Established (Pre) Malignant Disease by Synthetic Long Peptide Vaccines. Nat Rev Cancer (2008) 8(5):351–60. doi: 10.1038/nrc2373
164. Takahashi N, Ohkuri T, Homma S, Ohtake J, Wakita D, Togashi Y, et al. First Clinical Trial of Cancer Vaccine Therapy With Artificially Synthesized Helper/Killer-Hybrid Epitope Long Peptide of MAGE-A4 Cancer Antigen. Cancer Sci (2012) 103(1):150–3. doi: 10.1111/j.1349-7006.2011.02106.x
165. Kreiter S, Vormehr M, Van de Roemer N, Diken M, Löwer M, Diekmann J, et al. Mutant MHC Class II Epitopes Drive Therapeutic Immune Responses to Cancer. Nature (2015) 520(7549):692–6. doi: 10.1038/nature14426
166. Kloor M, Reuschenbach M, Pauligk C, Karbach J, Rafiyan M-R, Al-Batran S-E, et al. A Frameshift Peptide Neoantigen-Based Vaccine for Mismatch Repair-Deficient Cancers: A Phase I/IIa Clinical Trial. Clin Cancer Res (2020) 26(17):4503–10. doi: 10.1158/1078-0432.CCR-19-3517
167. Qin LL, Li YJ, Liang ZR, Dai L, Li WH, Chen C, et al. A Method of Screening Highly Common Neoantigens With Immunogenicity in Colorectal Cancer Based on Public Somatic Mutation Library. Yi Chuan Hereditas (2020) 42(6):599–612. doi: 10.16288/j.yczz.20-032
169. Roshandel E, Noorazar L, Farhadihosseinabadi B, Mehdizadeh M, Kazemi MH, Parkhideh S. PI3 Kinase Signaling Pathway in Hematopoietic Cancers: A Glance in miRNA's Role. J Clin Lab Anal (2021) 35:e23725. doi: 10.1002/jcla.23725
170. Rahma OE, Hamilton JM, Wojtowicz M, Dakheel O, Bernstein S, Liewehr DJ, et al. The Immunological and Clinical Effects of Mutated Ras Peptide Vaccine in Combination With IL-2, GM-CSF, or Both in Patients With Solid Tumors. J Trans Med (2014) 12(1):1–12. doi: 10.1186/1479-5876-12-55
171. Toubaji A, Achtar M, Provenzano M, Herrin VE, Behrens R, Hamilton M, et al. Pilot Study of Mutant Ras Peptide-Based Vaccine as an Adjuvant Treatment in Pancreatic and Colorectal Cancers. Cancer Immunol Immunother (2008) 57(9):1413–20. doi: 10.1007/s00262-008-0477-6
172. Kloor M, Reuschenbach M, Karbach J, Rafiyan M, Al-Batran S-E, Pauligk C, et al. Vaccination of MSI-H Colorectal Cancer Patients With Frameshift Peptide Antigens: A Phase I/IIa Clinical Trial. Am Soc Clin Oncol (2015) 33:3020. doi: 10.1200/jco.2015.33.15_suppl.3020
173. Ott PA, Hu Z, Keskin DB, Shukla SA, Sun J, Bozym DJ, et al. An Immunogenic Personal Neoantigen Vaccine for Patients With Melanoma. Nature (2017) 547(7662):217–21. doi: 10.1038/nature22991
174. Hilf N, Kuttruff-Coqui S, Frenzel K, Bukur V, Stevanović S, Gouttefangeas C, et al. Actively Personalized Vaccination Trial for Newly Diagnosed Glioblastoma. Nature (2019) 565(7738):240–5. doi: 10.1038/s41586-018-0810-y
175. Keskin DB, Anandappa AJ, Sun J, Tirosh I, Mathewson ND, Li S, et al. Neoantigen Vaccine Generates Intratumoral T Cell Responses in Phase Ib Glioblastoma Trial. Nature (2019) 565(7738):234–9. doi: 10.1038/s41586-018-0792-9
176. Alexandrov LB, Nik-Zainal S, Wedge DC, Aparicio SA, Behjati S, Biankin AV, et al. Signatures of Mutational Processes in Human Cancer. Nature (2013) 500(7463):415–21. doi: 10.1038/nature12477
177. Jin K, Mao C, Chen L, Wang L, Liu Y, Yuan J. Adenosinergic Pathway: A Hope in the Immunotherapy of Glioblastoma. Cancers (2021) 13:229. doi: 10.3390/cancers13020229
178. Nelde A, Rammensee H-G, Walz JS. The Peptide Vaccine of the Future. Mol Cell Proteomics (2021) 20:100022. doi: 10.1074/mcp.R120.002309
179. Parizadeh SM, Jafarzadeh-Esfehani R, Ghandehari M, Rezaei-Kalat A, Parizadeh SM, Javanbakht A, et al. Personalized Peptide-Based Vaccination for Treatment of Colorectal Cancer: Rational and Progress. Curr Drug Targets (2019) 20(14):1486–95. doi: 10.2174/1389450120666190619121658
180. Sakamoto S, Yutani S, Shichijo S, Morita M, Yamada A, Itoh K, et al. Immunological Evaluation of Personalized Peptide Vaccination for Patients With Histologically Unfavorable Carcinoma of Unknown Primary Site. Cancer Immunol Immunother (2016) 65(10):1223–31. doi: 10.1007/s00262-016-1887-5
181. Mochizuki K, Sato Y, Tsuda N, Shomura H, Sakamoto M, Matsuura K, et al. Immunological Evaluation of Vaccination With Pre-Designated Peptides Frequently Selected as Vaccine Candidates in an Individualized Peptide Vaccination Regimen. Int J Oncol (2004) 25(1):121–31. doi: 10.3892/ijo.25.1.121
182. Ohtake J, Wada S, Yada E, Fujimoto Y, Uchiyama H, Yoshida S, et al. Personalized Immunotherapy in Colorectal Cancer. Expert Rev Precis Med Drug Dev (2016) 1(3):267–77. doi: 10.1080/23808993.2016.1174060
183. Miyamoto S, Kochin V, Kanaseki T, Hongo A, Tokita S, Kikuchi Y, et al. The Antigen ASB4 on Cancer Stem Cells Serves as a Target for CTL Immunotherapy of Colorectal Cancer. Cancer Immunol Res (2018) 6(3):358–69. doi: 10.1158/2326-6066.CIR-17-0518
184. Hazama S, Nakamura Y, Tanaka H, Hirakawa K, Tahara K, Shimizu R, et al. A Phase II Study of Five Peptides Combination With Oxaliplatin-Based Chemotherapy as a First-Line Therapy for Advanced Colorectal Cancer (FXV Study). J Trans Med (2014) 12(1):1–10. doi: 10.1186/1479-5876-12-108
185. Weinberger EE, Isakovic A, Scheiblhofer S, Ramsauer C, Reiter K, Hauser-Kronberger C, et al. The Influence of Antigen Targeting to Sub-Cellular Compartments on the Anti-Allergic Potential of a DNA Vaccine. Vaccine (2013) 31(51):6113–21. doi: 10.1016/j.vaccine.2013.08.005
186. Haabeth OA, Blake TR, McKinlay CJ, Waymouth RM, Wender PA, Levy R. mRNA Vaccination With Charge-Altering Releasable Transporters Elicits Human T Cell Responses and Cures Established Tumors in Mice. Proc Natl Acad Sci (2018) 115(39):E9153–E61. doi: 10.1073/pnas.1810002115
187. Manickan E, Karem KL, Rouse BT. DNA Vaccines–A Modern Gimmick or a Boon to Vaccinology? Crit Rev Immunol (2017) 37(2-6):139–54. doi: 10.1615/CritRevImmunol.v37.i2-6.140
188. Pardi N, Hogan MJ, Weissman D. Recent Advances in mRNA Vaccine Technology. Curr Opin Immunol (2020) 65:14–20. doi: 10.1016/j.coi.2020.01.008
189. Burris HA, Patel MR, Cho DC, Clarke JM, Gutierrez M, Zaks TZ, et al. A Phase I Multicenter Study to Assess the Safety, Tolerability, and Immunogenicity of mRNA-4157 Alone in Patients With Resected Solid Tumors and in Combination With Pembrolizumab in Patients With Unresectable Solid Tumors. Am Soc Clin Oncol (2019) 37:2523. doi: 10.1200/JCO.2019.37.15_suppl.2523
190. Cafri G, Gartner JJ, Hopson K, Meehan RS, Zaks TZ, Robbins P, et al. Immunogenicity and Tolerability of Personalized mRNA Vaccine mRNA-4650 Encoding Defined Neoantigens Expressed by the Autologous Cancer. Am Soc Clin Oncol (2019) 37:2643. doi: 10.1200/JCO.2019.37.15_suppl.2643
191. Wooster AL, Girgis LH, Brazeale H, Anderson TS, Wood LM, Lowe DB. Dendritic Cell Vaccine Therapy for Colorectal Cancer. Pharmacol Res (2021) 164:105374. doi: 10.1016/j.phrs.2020.105374
192. Sato Y, Fujiwara T, Mine T, Shomura H, Homma S, Maeda Y, et al. Immunological Evaluation of Personalized Peptide Vaccination in Combination With a 5-Fluorouracil Derivative (TS-1) for Advanced Gastric or Colorectal Carcinoma Patients. Cancer Sci (2007) 98(7):1113–9. doi: 10.1111/j.1349-7006.2007.00498.x
193. Hattori T, Mine T, Komatsu N, Yamada A, Itoh K, Shiozaki H, et al. Immunological Evaluation of Personalized Peptide Vaccination in Combination With UFT and UZEL for Metastatic Colorectal Carcinoma Patients. Cancer Immunol Immunother (2009) 58(11):1843–52. doi: 10.1007/s00262-009-0695-6
194. Kibe S, Yutani S, Motoyama S, Nomura T, Tanaka N, Kawahara A, et al. Phase II Study of Personalized Peptide Vaccination for Previously Treated Advanced Colorectal Cancer. Cancer Immunol Res (2014) 2(12):1154–62. doi: 10.1158/2326-6066.CIR-14-0035
195. Gubin MM, Artyomov MN, Mardis ER, Schreiber RD. Tumor Neoantigens: Building a Framework for Personalized Cancer Immunotherapy. J Clin Invest (2015) 125(9):3413–21. doi: 10.1172/JCI80008
196. Khleif SN, Abrams SI, Hamilton JM, Bergmann-Leitner E, Chen A, Bastian A, et al. A Phase I Vaccine Trial With Peptides Reflecting Ras Oncogene Mutations of Solid Tumors. J Immunother (Hagerstown Md: 1997) (1999) 22(2):155–65. doi: 10.1097/00002371-199903000-00007
197. Cafri G, Gartner JJ, Zaks T, Hopson K, Levin N, Paria BC, et al. mRNA Vaccine–Induced Neoantigen-Specific T Cell Immunity in Patients With Gastrointestinal Cancer. J Clin Invest (2020) 130(11):5976–88. doi: 10.1172/JCI134915
198. Gao D, Li C, Xie X, Zhao P, Wei X, Sun W, et al. Autologous Tumor Lysate-Pulsed Dendritic Cell Immunotherapy With Cytokine-Induced Killer Cells Improves Survival in Gastric and Colorectal Cancer Patients. PLoS One (2014) 9(4):e93886. doi: 10.1371/journal.pone.0093886
199. Harris JE, Ryan L, Hoover HC Jr., Stuart RK, Oken MM, Benson AB III, et al. Adjuvant Active Specific Immunotherapy for Stage II and III Colon Cancer With an Autologous Tumor Cell Vaccine: Eastern Cooperative Oncology Group Study E5283. J Clin Oncol (2000) 18(1):148–. doi: 10.1200/JCO.2000.18.1.148
200. Vermorken J, Claessen A, van Tinteren H, Gall H, Ezinga R, Meijer S, et al. Active Specific Immunotherapy for Human Colon Cancer: A Prospectively Randomized Trial in Stage II and II Disease. Lancet (1999) 353:345–50. doi: 10.1016/S0140-6736(98)07186-4
201. Schulze T, Kemmner W, Weitz J, Wernecke K-D, Schirrmacher V, Schlag P. Efficiency of Adjuvant Active Specific Immunization With Newcastle Disease Virus Modified Tumor Cells in Colorectal Cancer Patients Following Resection of Liver Metastases: Results of a Prospective Randomized Trial. Cancer Immunol Immunother (2009) 58(1):61–9. doi: 10.1007/s00262-008-0526-1
202. Li W, Zhang C, Chen C, Zhuang G. Correlation Between Expression of DcR3 on Tumor Cells and Sensitivity to FasL. Cell Mol Immunol (2007) 4(6):455–60. doi: 10.1007/s00262-007-0299-y
203. Liu Y, Zhang W, Zhang B, Yin X, Pang Y. DC Vaccine Therapy Combined Concurrently With Oral Capecitabine in Metastatic Colorectal Cancer Patients. Hepato-Gastroenterology (2013) 60(121):23–7. doi: 10.5754/hge12522
204. Barth RJ, Fisher DA, Wallace PK, Channon JY, Noelle RJ, Gui J, et al. A Randomized Trial of Ex Vivo CD40L Activation of a Dendritic Cell Vaccine in Colorectal Cancer Patients: Tumor-Specific Immune Responses Are Associated With Improved Survival. Clin Cancer Res (2010) 16(22):5548–56. doi: 10.1158/1078-0432.CCR-10-2138
205. Nair SK, Morse M, Boczkowski D, Cumming RI, Vasovic L, Gilboa E, et al. Induction of Tumor-Specific Cytotoxic T Lymphocytes in Cancer Patients by Autologous Tumor RNA-Transfected Dendritic Cells. Ann Surg (2002) 235(4):540. doi: 10.1097/00000658-200204000-00013
206. Wang J, Stenzel D, Liu A, Liu D, Brown D, Ambrogelly A. Quantification of a Recombinant Antigen in an Immuno-Stimulatory Whole Yeast Cell-Based Therapeutic Vaccine. Anal Biochem (2018) 545:65–71. doi: 10.1016/j.ab.2018.01.006
207. Kumar R. Investigating the Long-Term Stability of Protein Immunogen (s) for Whole Recombinant Yeast-Based Vaccines. FEMS Yeast Res (2018) 18(7):foy071. doi: 10.1093/femsyr/foy071
208. Kumar S. Live-Attenuated Bacterial Vectors for Delivery of Mucosal Vaccines, DNA Vaccines, and Cancer Immunotherapy. Pharm Microbes: Springer (2019) 39–73. doi: 10.1007/978-3-030-01881-8_2
209. Zhou S, Gravekamp C, Bermudes D, Liu K. Tumour-Targeting Bacteria Engineered to Fight Cancer. Nat Rev Cancer (2018) 18(12):727–43. doi: 10.1038/s41568-018-0070-z
210. Bakhshaei P, Kazemi MH, Golara M, Abdolmaleki S, Khosravi-Eghbal R, Khoshnoodi J, et al. Investigation of the Cellular Immune Response to Recombinant Fragments of Filamentous Hemagglutinin and Pertactin of Bordetella Pertussis in BALB/c Mice. J Interferon Cytokine Res (2018) 38(4):161–70. doi: 10.1089/jir.2017.0060
211. Zhang Q, Wu W, Zhang J, Xia X. Merits of the “Good” Viruses: The Potential of Virus-Based Therapeutics. Expert Opin Biol Ther (2021) 21:731–40. doi: 10.1080/14712598.2021.1865304
212. Rowe J, Cen P. TroVax in Colorectal Cancer. Hum Vaccines Immunother (2014) 10(11):3196–200. doi: 10.4161/21645515.2014.973323
213. Kaufman HL, Lenz H-J, Marshall J, Singh D, Garett C, Cripps C, et al. Combination Chemotherapy and ALVAC-CEA/B7. 1 Vaccine in Patients With Metastatic Colorectal Cancer. Clin Cancer Res (2008) 14(15):4843–9. doi: 10.1158/1078-0432.CCR-08-0276
214. Kalyan A, Kircher S, Shah H, Mulcahy M, Benson A. Updates on Immunotherapy for Colorectal Cancer. J Gastrointest Oncol (2018) 9(1):160. doi: 10.21037/jgo.2018.01.17
215. Kazemi MH, Dehaghi BK, Roshandel E, Parkhideh S, Mehdizadeh M, Salimi M, et al. Oncolytic Virotherapy in Hematopoietic Stem Cell Transplantation. Hum Immunol (2021) 82:640–8. doi: 10.1016/j.humimm.2021.05.007
216. Kumar R, Kumar P. Yeast-Based Vaccines: New Perspective in Vaccine Development and Application. FEMS Yeast Res (2019) 19(2):foz007. doi: 10.1093/femsyr/foz007
217. Jin K-T, Du W-L, Liu Y-Y, Lan H-R, Si J-X, Mou X-Z. Oncolytic Virotherapy in Solid Tumors: The Challenges and Achievements. Cancers (2021) 13(4):588. doi: 10.3390/cancers13040588
218. Angelova A, Ferreira T, Bretscher C, Rommelaere J, Marchini A. Parvovirus-Based Combinatorial Immunotherapy: A Reinforced Therapeutic Strategy Against Poor-Prognosis Solid Cancers. Cancers (2021) 13(2):342. doi: 10.3390/cancers13020342
219. Song H, Zhong L-P, He J, Huang Y, Zhao Y-X. Application of Newcastle Disease Virus in the Treatment of Colorectal Cancer. World J Clin cases (2019) 7(16):2143. doi: 10.12998/wjcc.v7.i16.2143
220. Mosolits S, Nilsson B, Mellstedt H. Towards Therapeutic Vaccines for Colorectal Carcinoma: A Review of Clinical Trials. Expert Rev Vaccines (2005) 4(3):329–50. doi: 10.1586/14760584.4.3.329
221. Klebanoff CA, Acquavella N, Yu Z, Restifo NP. Therapeutic Cancer Vaccines: Are We There Yet? Immunol Rev (2011) 239(1):27–44. doi: 10.1111/j.1600-065X.2010.00979.x
222. Sur D, Havasi A, Cainap C, Samasca G, Burz C, Balacescu O, et al. Chimeric Antigen Receptor T-Cell Therapy for Colorectal Cancer. J Clin Med (2020) 9(1):182. doi: 10.3390/jcm9010182
223. Deng X, Gao F, Li N, Li Q, Zhou Y, Yang T, et al. Antitumor Activity of NKG2D CAR-T Cells Against Human Colorectal Cancer Cells In Vitro and In Vivo. Am J Cancer Res (2019) 9(5):945.
224. Hege KM, Bergsland EK, Fisher GA, Nemunaitis JJ, Warren RS, McArthur JG, et al. Safety, Tumor Trafficking and Immunogenicity of Chimeric Antigen Receptor (CAR)-T Cells Specific for TAG-72 in Colorectal Cancer. J Immunother Cancer (2017) 5(1):1–14. doi: 10.1186/s40425-017-0222-9
225. Chi X, Yang P, Zhang E, Gu J, Xu H, Li M, et al. Significantly Increased Anti-Tumor Activity of Carcinoembryonic Antigen-Specific Chimeric Antigen Receptor T Cells in Combination With Recombinant Human IL-12. Cancer Med (2019) 8(10):4753–65. doi: 10.1002/cam4.2361
226. Parkhurst MR, Yang JC, Langan RC, Dudley ME, Nathan D-AN, Feldman SA, et al. T Cells Targeting Carcinoembryonic Antigen Can Mediate Regression of Metastatic Colorectal Cancer But Induce Severe Transient Colitis. Mol Ther (2011) 19(3):620–6. doi: 10.1038/mt.2010.272
227. Tran E, Robbins PF, Lu Y-C, Prickett TD, Gartner JJ, Jia L, et al. T-Cell Transfer Therapy Targeting Mutant KRAS in Cancer. N Engl J Med (2016) 375(23):2255–62. doi: 10.1056/NEJMoa1609279
228. Karlsson M, Marits P, Dahl K, Dagöö T, Enerbäck S, Thörn M, et al. Pilot Study of Sentinel-Node-Based Adoptive Immunotherapy in Advanced Colorectal Cancer. Ann Surg Oncol (2010) 17(7):1747–57. doi: 10.1245/s10434-010-0920-8
229. Zhen Y-H, Liu X-H, Yang Y, Li B, Tang J-L, Zeng Q-X, et al. Phase I/II Study of Adjuvant Immunotherapy With Sentinel Lymph Node T Lymphocytes in Patients With Colorectal Cancer. Cancer Immunol Immunother (2015) 64(9):1083–93. doi: 10.1007/s00262-015-1715-3
230. Yoshida Y, Naito M, Yamada T, Aisu N, Daibo K, Mera T, et al. Adoptive Chemoimmunotherapy Using Activated αβ T Cells for Stage IV Colorectal Cancer. Anticancer Res (2016) 36(7):3741–6.
231. Bomberg M, Ahonen L. Geomicrobes: Life in Terrestrial Deep Subsurface. Front Microbiol (2017) 8:103. doi: 10.3389/fmicb.2017.00103
232. Sánchez-Alcoholado L, Ramos-Molina B, Otero A, Laborda-Illanes A, Ordóñez R, Medina JA, et al. The Role of the Gut Microbiome in Colorectal Cancer Development and Therapy Response. Cancers (2020) 12(6):1406. doi: 10.3390/cancers12061406
233. Rooks MG, Garrett WS. Gut Microbiota, Metabolites and Host Immunity. Nat Rev Immunol (2016) 16(6):341–52. doi: 10.1038/nri.2016.42
234. Genua F, Raghunathan V, Jenab M, Gallagher WM, Hughes DJ. The Role of Gut Barrier Dysfunction and Microbiome Dysbiosis in Colorectal Cancer Development. Front Oncol (2021) 11:1016. doi: 10.3389/fonc.2021.626349
235. Abed J, Emgård JE, Zamir G, Faroja M, Almogy G, Grenov A, et al. Fap2 Mediates Fusobacterium Nucleatum Colorectal Adenocarcinoma Enrichment by Binding to Tumor-Expressed Gal-GalNAc. Cell Host Microbe (2016) 20(2):215–25. doi: 10.1016/j.chom.2016.07.006
236. Wang H-F, Li L-F, Guo S-H, Zeng Q-Y, Ning F, Liu W-L, et al. Evaluation of Antibody Level Against Fusobacterium Nucleatum in the Serological Diagnosis of Colorectal Cancer. Sci Rep (2016) 6(1):1–10. doi: 10.1038/srep33440
237. Yuan C, Zhao X, Wangmo D, Alshareef D, Gates TJ, Subramanian S. Tumor Models to Assess Immune Response and Tumor-Microbiome Interactions in Colorectal Cancer. Pharmacol Ther (2021) 107981. doi: 10.1016/j.pharmthera.2021.107981
238. Behrouzi A, Nafari AH, Siadat SD. The Significance of Microbiome in Personalized Medicine. Clin Trans Med (2019) 8(1):1–9. doi: 10.1186/s40169-019-0232-y
239. Kalasabail S, Engelman J, Zhang LY, El-Omar E, Yim HCH. A Perspective on the Role of Microbiome for Colorectal Cancer Treatment. Cancers (2021) 13(18):4623. doi: 10.3390/cancers13184623
240. Sun S, Luo L, Liang W, Yin Q, Guo J, Rush AM, et al. Bifidobacterium Alters the Gut Microbiota and Modulates the Functional Metabolism of T Regulatory Cells in the Context of Immune Checkpoint Blockade. Proc Natl Acad Sci (2020) 117(44):27509–15. doi: 10.1073/pnas.1921223117
241. Routy B, Le Chatelier E, Derosa L, Duong CP, Alou MT, Daillère R, et al. Gut Microbiome Influences Efficacy of PD-1–Based Immunotherapy Against Epithelial Tumors. Science (2018) 359(6371):91–7. doi: 10.1126/science.aan3706
242. Sivan A, Corrales L, Hubert N, Williams JB, Aquino-Michaels K, Earley ZM, et al. Commensal Bifidobacterium Promotes Antitumor Immunity and Facilitates Anti–PD-L1 Efficacy. Science (2015) 350(6264):1084–9. doi: 10.1126/science.aac4255
243. Park R, Umar S, Kasi A. Immunotherapy in Colorectal Cancer: Potential of Fecal Transplant and Microbiota-Augmented Clinical Trials. Curr Colorectal Cancer Rep (2020) 16:81–8. doi: 10.1007/s11888-020-00456-1
244. Hamada T, Keum N, Nishihara R, Ogino S. Molecular Pathological Epidemiology: New Developing Frontiers of Big Data Science to Study Etiologies and Pathogenesis. J Gastroenterol (2017) 52(3):265–75. doi: 10.1007/s00535-016-1272-3
245. Ogino S, Nowak JA, Hamada T, Milner DA Jr, Nishihara R. Insights Into Pathogenic Interactions Among Environment, Host, and Tumor at the Crossroads of Molecular Pathology and Epidemiology. Annu Rev Pathol: Mech Dis (2019) 14:83–103. doi: 10.1146/annurev-pathmechdis-012418-012818
246. Hughes LA, Simons CC, van den Brandt PA, van Engeland M, Weijenberg MP. Lifestyle, Diet, and Colorectal Cancer Risk According to (Epi) Genetic Instability: Current Evidence and Future Directions of Molecular Pathological Epidemiology. Curr Colorectal Cancer Rep (2017) 13(6):455–69. doi: 10.1007/s11888-017-0395-0
247. Patel SA, Minn AJ. Combination Cancer Therapy With Immune Checkpoint Blockade: Mechanisms and Strategies. Immunity (2018) 48(3):417–33. doi: 10.1016/j.immuni.2018.03.007
248. Zhao J, Chen Y, Ding Z-Y, Liu J-Y. Safety and Efficacy of Therapeutic Cancer Vaccines Alone or in Combination With Immune Checkpoint Inhibitors in Cancer Treatment. Front Pharmacol (2019) 10:1184. doi: 10.3389/fphar.2019.01184
249. Rocha-Lima CM, Junior E, Bayraktar S, Broome P, Weissman C, Nowacki M, et al. A Multicenter Phase II Study of G17DT Immunogen Plus Irinotecan in Pretreated Metastatic Colorectal Cancer Progressing on Irinotecan. Cancer Chemother Pharmacol (2014) 74(3):479–86. doi: 10.1007/s00280-014-2520-y
250. Danishmalik SN, Sin J-I. Therapeutic Tumor Control of HER2 DNA Vaccines is Achieved by an Alteration of Tumor Cells and Tumor Microenvironment by Gemcitabine and Anti-Gr-1 Ab Treatment in a HER2-Expressing Tumor Model. DNA Cell Biol (2017) 36(9):801–11. doi: 10.1089/dna.2017.3810
251. Liu C, Xie Y, Sun B, Geng F, Zhang F, Guo Q, et al. MUC1-And Survivin-Based DNA Vaccine Combining Immunoadjuvants CpG and Interleukin-2 in a Bicistronic Expression Plasmid Generates Specific Immune Responses and Antitumour Effects in a Murine Colorectal Carcinoma Model. Scand J Immunol (2018) 87(2):63–72. doi: 10.1111/sji.12633
252. Yarchoan M, Huang CY, Zhu Q, Ferguson AK, Durham JN, Anders RA, et al. A Phase 2 Study of GVAX Colon Vaccine With Cyclophosphamide and Pembrolizumab in Patients With Mismatch Repair Proficient Advanced Colorectal Cancer. Cancer Med (2020) 9(4):1485–94. doi: 10.1002/cam4.2763
253. Sakakibara M, Kanto T, Hayakawa M, Kuroda S, Miyatake H, Itose I, et al. Comprehensive Immunological Analyses of Colorectal Cancer Patients in the Phase I/II Study of Quickly Matured Dendritic Cell Vaccine Pulsed With Carcinoembryonic Antigen Peptide. Cancer Immunol Immunother (2011) 60(11):1565–75. doi: 10.1007/s00262-011-1051-1
254. Ni Q, Zhang F, Liu Y, Wang Z, Yu G, Liang B, et al. A Bi-Adjuvant Nanovaccine That Potentiates Immunogenicity of Neoantigen for Combination Immunotherapy of Colorectal Cancer. Sci Adv (2020) 6(12):eaaw6071. doi: 10.1126/sciadv.aaw6071
255. Zhou X, Mo X, Qiu J, Zhao J, Wang S, Zhou C, et al. Chemotherapy Combined With Dendritic Cell Vaccine and Cytokine-Induced Killer Cells in the Treatment of Colorectal Carcinoma: A Meta-Analysis. Cancer Manage Res (2018) 10:5363. doi: 10.2147/CMAR.S173201
Keywords: colorectal cancer, immunotherapy, personalized medicine, neoantigen, heterogeneity
Citation: Hu L-F, Lan H-R, Huang D, Li X-M and Jin K-T (2021) Personalized Immunotherapy in Colorectal Cancers: Where Do We Stand? Front. Oncol. 11:769305. doi: 10.3389/fonc.2021.769305
Received: 15 September 2021; Accepted: 26 October 2021;
Published: 23 November 2021.
Edited by:
Gianluca Tomasello, IRCCS Ca ‘Granda Foundation Maggiore Policlinico Hospital, ItalyReviewed by:
Feng Wei, Tianjin Medical University Cancer Institute and Hospital, ChinaLouise Catherine Connell, Cornell University, United States
Copyright © 2021 Hu, Lan, Huang, Li and Jin. This is an open-access article distributed under the terms of the Creative Commons Attribution License (CC BY). The use, distribution or reproduction in other forums is permitted, provided the original author(s) and the copyright owner(s) are credited and that the original publication in this journal is cited, in accordance with accepted academic practice. No use, distribution or reproduction is permitted which does not comply with these terms.
*Correspondence: Ke-Tao Jin, amlua2V0YW8yMDAxQHpqdS5lZHUuY24=; Xue-Min Li, bHhtMjEwMDIzNkAxNjMuY29t
†These authors have contributed equally to this work