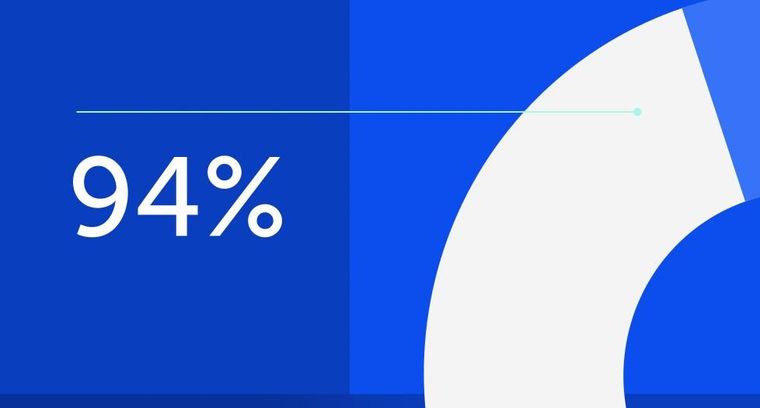
94% of researchers rate our articles as excellent or good
Learn more about the work of our research integrity team to safeguard the quality of each article we publish.
Find out more
ORIGINAL RESEARCH article
Front. Oncol., 21 September 2021
Sec. Neuro-Oncology and Neurosurgical Oncology
Volume 11 - 2021 | https://doi.org/10.3389/fonc.2021.748229
This article is part of the Research TopicBrain Tumor Segmentation, Grading and Patient Survival PredictionView all 20 articles
Background: Glioma is the most common intra-axial tumor, and its location relative to critical areas of the brain is important for treatment decision-making. Studies often report tumor location based on anatomical taxonomy alone since the estimation of eloquent regions requires considerable knowledge of functional neuroanatomy and is, to some degree, a subjective measure. An unbiased and reproducible method to determine tumor location and eloquence is desirable, both for clinical use and for research purposes.
Objective: To report on a voxel-based method for assessing anatomical distribution and proximity to eloquent regions in diffuse lower-grade gliomas (World Health Organization grades 2 and 3).
Methods: A multi-institutional population-based dataset of adult patients (≥18 years) histologically diagnosed with lower-grade glioma was analyzed. Tumor segmentations were registered to a standardized space where two anatomical atlases were used to perform a voxel-based comparison of the proximity of segmentations to brain regions of traditional clinical interest.
Results: Exploring the differences between patients with oligodendrogliomas, isocitrate dehydrogenase (IDH) mutated astrocytomas, and patients with IDH wild-type astrocytomas, we found that the latter were older, more often had lower Karnofsky performance status, and that these tumors were more often found in the proximity of eloquent regions. Eloquent regions are found slightly more frequently in the proximity of IDH-mutated astrocytomas compared to oligodendrogliomas. The regions included in our voxel-based definition of eloquence showed a high degree of association with performing biopsy compared to resection.
Conclusion: We present a simple, robust, unbiased, and clinically relevant method for assessing tumor location and eloquence in lower-grade gliomas.
Glioma is the most common intra-axial tumor, and its location relative to eloquent areas of the brain is important for treatment decisions (1–3). Diffuse lower-grade gliomas (dLGGs) are preferentially located in functional areas near primary eloquent areas of the human brain (4, 5). Besides playing an important role in clinical management of adult patients with glioma, tumor location is also linked to the underlying tumor biology (6, 7).
Epidemiological studies often report tumor location crudely based on anatomical taxonomy, while eloquence often is classified using the University of California San Francisco (UCSF) classification (8) or the classification by Sawaya (9). Such methods require considerable knowledge of neuroanatomy by the rater and add a degree of subjectivity to the evaluation. Yet, identification of eloquent areas (8, 10) is key for dLGG treatment management, and an unbiased and reproduceable method to determine eloquence is desirable.
A robust classification of eloquence could be of high value to surgeons prior to surgery, facilitate risk assessment, and be useful for assessing the need for functional diagnostic work-ups (e.g., functional magnetic resonance imaging (fMRI), diffusion tensor imaging (DTI), or transcranial magnetic stimulation (TMS)), and for assessing the need for intraoperative mapping or monitoring. A robust estimation of proximity to areas of assumed eloquence that is measurable in all patients would also be of importance for research purposes. More overreaching, the strength of this epidemiological approach is to gain awareness of commonly eloquent areas under dLGG influence. Areas often encountered should be emphasized in teaching and surgical training. Neurorehabilitation may design programs based upon regions most often involved and potentially injured by tumor growth or treatment. Also, neuroscientists may find this epidemiological approach useful to understand which regions that frequently can be studied in patients with slow-growing brain tumors in studies on, for instance, region-specific plasticity and specific functional networks. Recently, a tool using normative data in glioblastomas was published where surgeons can upload patient images and get information of involved areas and expected surgical results based upon historical data (1). The use of larger dLGG datasets with richer clinical variables may open the way to develop a similar tool for patients with dLGG.
In this study, we aim to report a voxel-based method where we assess overall dLGG distribution and proximity to critical eloquent regions of interest to neurosurgeons. This will provide an epidemiological background on dLGG predilection sites and proximity to eloquent areas of well-established relevance. To demonstrate the relevance of the model, we will present preliminary data on the association of location with surgical treatment and tumor biology. The chosen software and parameter settings are fully disclosed.
This study was produced in the frame of a collaboration between three neurosurgical departments in Norway and Sweden. Patients screened for inclusion were 18 years or older and underwent primary surgery (either biopsy or resection) in the time period 2010–2018. Included patients had a histopathological verified supratentorial diffuse glioma World Health Organization (WHO) grade 2 or 3. We chose to include WHO grades 2 and 3 tumors as they usually have no significant edema causing additional mass effect and distortion of anatomy.
Clinical and radiological data were retrieved from the electronic health records (EHR) at each institution or collected from research projects conducted locally. Pseudonymized data from each institution were gathered for analysis. Clinical data included patient demographics, the Karnofsky performance score (KPS) (11), symptoms at presentation, histopathological tumor grade, isocitrate dehydrogenase (IDH) and chromosomal arms 1p and 19q status (1p19q codeletion or intact), main tumor location, tumor largest diameter, and presumed eloquence based on UCSF criteria. A cohort consisting of 343 patients was curated for analysis.
Histopathological analyses and molecular evaluations were conducted locally, either as clinical practice or reclassified for research purposes following the 2016 World Health Organization classification of tumors of the central nervous system (WHO 2016) (12). Mutational status of IDH was assessed with immunohistochemistry staining and next-generation sequencing; 1p19q codeletion was evaluated with fluorescence in situ hybridization or methylation array (13, 14). In a minority of cases, reclassification according to the WHO 2016 was not possible due to lack of tissue.
The main tumor location and the largest diameter were registered from anatomical magnetic resonance imaging (MRI) using T2 weighted image (T2) or fluid-attenuated inversion-recovery (FLAIR) sequences. Location taxonomy followed the anatomical lobe mainly involved by the lesion. Multifocal lesions were classified according to the largest tumor. Presumed eloquent brain areas were identified preoperatively following the areas listed in the USCF LGG score (8).
Radiological data included preoperative MRI acquired at different hospitals (1.5T and 3.0T scanners). Scanners were routinely maintained by the vendors; sequences were originally optimized at the respective hospitals as part of the clinical preoperative work for clinical evaluation of brain lesions. Technical data such as scan vendors, software releases, and image acquisition parameters were not acquired for this study. Sequences gathered for this study included T1 weighted image (T1), T1 with gadolinium postcontrast (T1c), T2, and FLAIR. Since all sequences were not available for all patients, only patients with key pair sequences of either T2 or FLAIR, and either T1 or T1c, were included in the analysis. T2 and FLAIR sequences had a mean voxel size of 0.7 mm (0.4–1.2 min–max) for both axes in the axial plane and a mean slice thickness of 3.5 mm (0.5–7.0 min–max), while 43% of these sequences had a voxel size equal or inferior to 1 mm3, 27% had voxel size between 1 and 2 mm3, and 30% had a voxel size over 2 mm.
Digital Imaging and Communications in Medicine (DICOM) data of all sequences were converted to Neuroimaging Informatics Technology Initiative file format (NIfTI) with the software 3D Slicer (15). Several trained raters segmented the tumors based upon T2 or FLAIR images. All segmentations were produced in a semi-automatic manner on a case-by-case basis using the tools “Paint,” “Draw,” and “Level tracing” from the Module “Segment Editor” and exported as binary label maps in the 3D Slicer.
All segmentations were further validated by a neurosurgeon (AJ) with long experience in LGG management and research, including volumetric assessment. Raters performing segmentations were blinded to the clinical status of the subjects at the time of the tumor segmentation. Since diffuse glioma WHO grades 2 or 3 infrequently has significant surrounding edema, hyperintense areas on the T2 or FLAIR sequence were considered as tumor invaded. In exceptional cases, attributable edema areas without convincing signs of tumor invasion were excluded from the segmentation.
Standard preprocessing was done with Functional Magnetic Resonance Imaging of the Brain Software Library (FLS) (16) as follows: all sequences for a given patient were registered to the T2 or FLAIR image (matching the modality selected for segmentation).
The registered T1 or T1c images were then individually registered to the Montreal Neurological Institute (MNI) space, for which the T1 symmetric MNI 09a was used as the registration target (17). Tumor segmentations and T2 or FLAIR images were then transformed to the MNI space by applying the transformation matrix generated during T1 or T1c registration to the MNI space. All tumor segmentations and T2 or FLAIR images transformed to the MNI space were individually controlled for errors or unexpected deformations by a single rater with experience in glioma image analysis (TG).
Image registration was performed using 12 parameter affine transformations in FSL’s Functional Magnetic Resonance Imaging of the Brain Linear Image Registration Tool (FLIRT). All registration parameters are provided in the Supplementary Material.
In order to assess the tumor proximity to the regions of interest (ROIs), two anatomical atlases were used. A well-known probability atlas of three-dimensional reconstructed white matter tracs (18) was included together with the recently released Cerebrum Atlas (CerebrA)—a cortical and subcortical parcellation atlas (19).
Grounded on traditional clinical interest in the neurosurgical community, we focused on regions based on a priori anatomical identification and on relevant regions identified previously in documented intraoperative mappings. Traditionally, eloquent parcellated areas include the basal ganglia, visual cortex, and Broca’s and Wernicke’s areas (8, 20). These anatomical regions were mapped to the following parcellations: precentral, pars opercularis, pars triangularis, postcentral, supramarginal and inferior parietal, and pericalcarine area. Due to the frequent involvement of the medial temporal lobe and the importance of this area in memory and learning, we also included the hippocampus and the parahippocampal area. The corresponding subcortical white matter tract anatomy previously reported in the literature (21–23) were included: corticospinal (CS); perisylvian anterior, posterior and long components of the superior longitudinal fasciculus (SLF) with a separate report for arcuate fasciculus (AF); inferior fronto-occipital fasciculus (IFOF); and the optic radiations (OR). Inferior parietal, supramarginal, pars triangularis, pars opercularis, SLF, AF, and IFOF were only considered eloquent and analyzed when involvement was on the left side due to their involvement in language most often being left lateralized.
For each registered spatial segmentation of the patient’s tumor, we computed the extent of the segmentation overlapping the ROIs (accounting for both white matter tracts and parcellated areas). To assess the presence of a white matter tract in a given voxel of the probability atlas, we chose a likelihood above 50% (24–27). A minimum overlapping volume of 1 mm3 between the ROI and tumor segmentations was considered as proximity to the ROI in this study. To check the robustness of results, a sensitivity analysis applying a threshold of 10 mm3 was used in one application.
To assess the tumors in the proximity of the ROIs, an automated calculation of individual tumor segmentation, anatomical parcellations, and white matter tract overlap was produced. It included a binary identification of the overlapping ROIs, the volume of the overlapped area, and the volumes of the tumor and ROIs in the MNI space. Calculations were performed in Python using NumPy (28) and SimpleITK (29) libraries. In the Supplementary Material, the code structure for calculations is presented.
Heatmap visualizations were generated in the 3D Slicer (15). All tumor location maps are three-dimensional. However, illustrations for the tumor location heatmaps are represented showing axial and coronal slices. For visualization purposes, the heatmaps were normalized by computing the cumulative number of observed segmentations for each voxel and divided by the total amount of cases in that group (N). Circular bar plots were generated in Python using NumPy, Pandas (30), and Matplotlib (31).
Analysis of the result of Python calculations and patient data was conducted in IBM SPSS version 28 (IBM Corp., Armonk, NY, USA). Central tendencies are presented either with percentages, means with 95% confidence interval (CI), or medians with quartiles 1 and 3 (Q1, Q3). All tests were two-sided; the statistical significance level was set to P < 0.002 due to multiple testing. Comparisons between groups were conducted with unpaired t-test, Mann–Whitney U test, and Fisher’s exact test or one-way ANOVA, Kruskal–Wallis test, and Fisher–Freeman–Halton exact test when appropriate. Interrater reliability analysis was performed using Cohen’s Kappa statistic. Univariable logistic regression was used in consecutive test with choice of primary surgical strategy and binarized KPS as response. Age at surgery, preoperative KPS, tumor volume, tumor classification, preoperative eloquence, and voxel-based eloquence were used as dependent variables.
A total of 343 patients were eligible for inclusion in this study. Subsequently, 61 cases were excluded due to administrative or technical problems together with 5 cases with infratentorial tumors; see Figure 1. Thus, 277 patients were included in the analyses. The mean age at surgery was 45.1 ± 14.7 years, and 160 (57.8%) patients were males.
We had complete molecular data according to WHO 2016 in 222 cases (80.1% of total). There were 73/277 (26.3%) patients with oligodendroglioma, 67/277 (24.2%) were IDH-mutated astrocytoma, and 82/277 (29.6%) were IDH wild-type astrocytoma. The remaining 55 cases (19.9%) were not characterized molecularly. In total, 182/277 (65.7%) were WHO grade 2, and 95/277 (34.3%) were WHO grade 3. Demographic distribution, histomolecular data, and clinical variables of the study cohort are shown in Table 1.
A location heatmap showing the spatial distribution for all 277 tumors is shown in Figure 2. Circular bar plots showing the frequencies of tumors in their proximity to the predefined critical regions are shown in Figure 3A. For a description of associations between voxel-based and clinician reported eloquence, see Supplementary Table 1.
Figure 2 Tumor heatmap of all included cases. (A) N=277, axial slices number 33, 15, 0, -15, -33. (B) N=277, coronal slices number 40, 15, 0, -15, -40. Axial-coronal coordinates in MNI space. The color intensity represents the voxel-based percentual distribution of the selected tumor segmentations.
Figure 3 Circular bar plots—proximity to predefined eloquent regions. (A) All included cases N=277, (B) presumed eloquent N=182, and (C) non-eloquent N=95. PreC, Precentral; PostC, Postcentral; PeriCal, Pericalcarine; Hipp, Hippocampus; ParaHipp, Para hippocampus; SupMarg, Supramarginal; ParsTri, Pars Triangularis; ParsOp, Pars Opercularis; InfPar, Inferior parietal; CS, Corticospinal; OR, Optic radiations; IFOF, Inferior fronto-occipital fasciculus; SLF, Perisylvian anterior, posterior, and long components of the superior longitudinal fasciculus; AF, Arcuate fasciculus. “L” and “R” indicate left and right sides, respectively. Size and color intensity represent the percentage of tumors in the proximity to predefined eloquent regions by group. Fisher exact test was used for two-group comparison between Presumed eloquent and Non-eloquent groups. *P value equal or inferior to 0.002.
Choice of primary surgical strategy in all cases (N=277) was used as the target to evaluate the relevance of the areas included in our voxel-based eloquence. Age, eloquence according to UCSF criteria, and tumor volume were found independent predictors of choice of biopsy as primary surgical strategy (95% CI [0.028 to 0.072], p <0.001; 95% CI [1.313 to 3.701], p <0.001; and 95% CI [2.485E-6 to 9.626E-6], p <0.001, respectively). When applying the regression analysis only in cases characterized molecularly according to WHO 2016 (N=222), IDH wild-type astrocytoma was found to be an independent predictor of choice of biopsy as primary surgical strategy (95% CI [1.175 to 3.061], p <0.001). Out of the 21 predefined areas, 10 were significantly associated with performing biopsy instead of resection. Location heatmaps showing the spatial distribution by choice of primary surgical strategy are shown in Figure 4. Circular bar plots showing the frequencies of tumors in their proximity to the predefined critical regions are shown in Figure 5. A description of frequencies on tumor proximity is shown in Table 2.
Figure 4 Tumor heatmap by choice of primary surgical strategy. (A) Biopsy heatmap N=55—axial slices number 33, 15, 0, -15, -33. (B) Resection heatmap N=222—axial slices number 33, 15, 0, -15, -33. Axial coordinates in MNI space. The color intensity represents the voxel-based percentual distribution of each group.
Figure 5 Circular bar plots by choice of primary surgical strategy. (A) Only biopsy N=55. (B) Tumor resection N=222. PreC, Precentral; PostC, Postcentral; PeriCal, Pericalcarine; Hipp, Hippocampus; ParaHipp, Para hippocampus; SupMarg, Supramarginal; ParsTri, Pars Triangularis; ParsOp, Pars Opercularis; InfPar, Inferior parietal; CS, Corticospinal; OR, Optic radiations; IFOF, Inferior fronto-occipital fasciculus; SLF, Perisylvian anterior, posterior, and long components of the superior longitudinal fasciculus; AF, Arcuate fasciculus. “L” and “R” indicate left and right sides. Size and color intensity represent the percentage of tumors in the proximity to predefined eloquent regions by group. Fisher’s exact test was used for two-group comparison between only biopsied and resected tumors. *P value equal or inferior to 0.002.
Table 2 Tumor proximity to selected ROI—Comparison by choice of surgical strategy//Sensitivity analysis with overlap threshold 10 mm3.
Binarized preoperative KPS in all cases (N=277) was used as the target to explore associations of the areas included in our voxel-based eloquence and functional status. The KPS cutoff was set to 90 identifying patients with normal performance status or with minor symptoms. In 12 of 21 areas, an association with the KPS score was observed as can be seen in Supplementary Table 1.
To rule out that the results were affected by inaccuracies in the registration method, a sensitivity analysis was conducted adjusting the parameters of the computational analysis. Results for this, showing the overlap of tumor location and ROIs, are displayed together with the other results in Table 2. In this sensitivity analysis, results were consistent using an overlap threshold of 10 mm3; hence, for the rest of the analyses, we used an overlap threshold of 1 mm3.
To evaluate the concordance of the voxel-based method with traditional measure of eloquence using the UCSF criteria, an interrater reliability analysis was performed. Voxel-based eloquence was considered positive when any of the predefined eloquent regions were overlapping with the tumor segmentation. When comparing the whole cohort (N=277), the analysis showed fair agreement between preoperative UCSF eloquence and voxel-based eloquence. Interrater reliability analysis is displayed in Table 3. Since not all regions of our definition of voxel-based based criteria are included in the UCSF criteria (and vice versa), we explored all our predefined regions according to UCSF definition of eloquence in Figures 3B, C. A description of frequencies according to UCSF criteria can be found in Supplementary Table 1. The pars triangularis, parahippocampal areas, the calcarine area on the right side, and the hippocampus on the right side were not associated with the clinician-reported UCSF criteria of eloquence.
Only tumors classified molecularly according to WHO 2016 were included in the analysis of proximity to eloquent regions in molecular subgroups (N=222). Heatmaps showing anatomical tumor location in patients with IDH wild-type astrocytoma either grade 2 or grade 3 (N=82), IDH-mutated astrocytoma either grade 2 or grade 3 (N=67), and oligodendroglioma either grade 2 or grade 3 (N=73) are presented in Figure 6. Frequencies of proximity to each of the predefined eloquent areas are represented in circular bar plots in Figure 7. Details on tumor location and comparative results according to molecular subgroups can be found in Table 4. Overall, IDH wild-type astrocytomas were more often found in the proximity of the hippocampus, parahippocampal area, optic radiations, and arcuate fasciculus. Involvement of critical eloquent regions was found in a biological gradient.
Figure 6 Tumor heatmaps of molecular subgroups according to WHO 2016. (A) IDH wild-type astrocytomas heatmap N=82—axial slices number 33, 15, 0, -15, -33. (B) IDH-mutated astrocytomas heatmap N=67—axial slices number 33, 15, 0, -15, -33. (C) Oligodendrogliomas N=73—axial slices number 33, 15, 0, -15, -33. Axial coordinates in MNI space. The color intensity represents the voxel-based percentual distribution of each group.
Figure 7 Circular bar plots—proximity to predefined eloquent regions by molecular subgroups according to WHO 2016. (A) IDH wild-type astrocytomas N=82, (B) IDH-mutated astrocytomas N=67, and (C) Oligodendrogliomas N=73. PreC, Precentral; PostC, Postcentral; PeriCal, Pericalcarine; Hipp, Hippocampus; ParaHipp, Para hippocampus; SupMarg, Supramarginal; ParsTri, Pars Triangularis; ParsOp, Pars Opercularis; InfPar, Inferior parietal; CS, Corticospinal; OR, Optic radiations; IFOF, Inferior fronto-occipital fasciculus; SLF, Perisylvian anterior, posterior, and long components of the superior longitudinal fasciculus; AF, Arcuate fasciculus. “L” and “R” indicate left and right sides. Size and color intensity represent the percentage of tumors in the proximity to predefined eloquent regions by tumor groups. Fisher–Freeman–Halton exact tests were used when appropriate for three-group comparison between IDH wild-type astrocytoma, IDH-mutated astrocytoma, and oligodendroglioma. *P value equal or inferior to 0.002.
Table 4 Details on voxel-based eloquence and comparative results by molecular subgroups according to WHO 2016.
We present a voxel-based method depicting the overall anatomical distribution of dLGG tumors in a population-based sample and their proximity to eloquent regions. As expected, tumor distribution is linked to molecular status. Our voxel-based definition of eloquence had only a fair agreement with clinical reported presumed eloquence using the UCSF criteria. Almost half of the predefined eloquent regions were associated with undergoing biopsy instead of resection, suggesting that our captured regions hold clinical relevance.
Our atlas-based approach to both location and eloquence is based on normative data and not patient-specific functional data. An epidemiological and unselected approach of dLGG relations to subcortical and cortical anatomy mapped to functional data would require DTI and fMRI or TMS to be performed in an unselected manner, which is not a clinical routine in most European specialized centers (32). Such examinations are most often performed when deemed clinically useful on an individual level. Although there exist several methods in use to localize certain functions, there is, however, no perfect match between preoperative functional mapping (fMRI, DTI, TMS), intraoperative mapping/stimulation, and ultimately the final operative result (33–37). There are also discrepancies between dissection-based anatomical studies and DTI studies (38). Our voxel-based mapping of the spatial proximity of the tumor to eloquent cortical regions and related connectivity may provide realistic estimates in an unselected population, although we acknowledge that there are weaknesses at an individual level. Voxel-based mapping, as presented in this study, does not represent an advantage compared to abovementioned methods; however, it allows us to access a larger cohort in an unbiased manner.
With the aim to report a voxel-based method highlighting the overall dLGG anatomical distribution and their proximity to critical regions of interest to neurosurgeons, we showed that eloquent regions are found in a biological gradient that is independent of tumor volume, with most eloquent regions involved in IDH wild-type astrocytomas and least eloquent regions involved in oligodendrogliomas. Earlier publications prior to the inclusion of molecular markers in WHO 2016 classification have demonstrated that the frontal, temporal, and insular lobes near eloquent regions are the preferential location of dLGG (39–42). After the inclusion of molecular differentiation for dLGG classification, studies describing the anatomical predilection of IDH-mutated subgroups have drawn more detailed conclusions when addressing main lobe location or location in relation to eloquent areas. For instance, studies comparing three molecular subgroups of diffuse gliomas WHO grade 2 (43) reported location in relation to areas of gliogenesis, showing that frontal location was common for IDH-mutated tumors without particular differential predilection sites depending on 1p19q status. Others found that 1p19q defined oligodendrogliomas WHO grade 2 were mainly located in the frontal lobes (44), specifically within the deep white matter (45). Studies including patients across all diffuse glioma grades also demonstrated that IDH-mutated gliomas are primarily situated in the frontal lobes (46, 47). In clinical practice, IDH-mutated tumors had been demonstrated to be more amenable to resection, therefore consistent with the preference of IDH wild-type tumors in more critical locations (48, 49). As expected, these studies on dLGG general locations within the brain, together with studies describing more critical location in IDH wild-type tumors (50, 51), are in line with our findings. These observations, for instance the more aggressive clinical course in patients with IDH wild-type dLGG, make it necessary to reevaluate the extensive literature on residual tumor volume (or extent of resection) and outcome as the historical results may suffer from confounding by tumor biology (12, 52–56).
dLGGs are frequently located in eloquent areas. However, controversies regarding the definition of eloquence have not been settled (57). While the USCF criteria were developed and validated to predict overall survival and progression-free survival (58), application of Sawaya’s grading was found ambiguous due to its definition of the near-eloquent brain (59). Still, the UCSF score and Sawaya’s grading are used as good general predictors of the clinical outcome in patients with dLGG. Our method complements such approaches by including a more detailed account of the involved eloquent regions. Of note, our method is not only reproducible and unbiased but also flexible if the research question makes it relevant to study another area or more specific area of interest (e.g., motor system with supplementary motor area, motor strip and corticospinal tract; language areas for language studies; or hippocampus for memory studies). The strength of our methodological approach is that it is adaptive, where involvement of certain regions can be looked for specifically or in an unselected manner depending on the output of interest. This can be easily done by replacing our definition of eloquence with an a priori selection of the tracts and cortical areas of interest to the research topic. With more and richer data available, unsupervised analyses may also be of interest to study associations between symptoms or findings with specific areas in more explorative studies.
Preoperative methods to assess eloquence in patients with dLGG are limited and bond to a traditional view of eloquence (60). Compared to other methods, our approach relies on a detailed description of the involvement of the different chosen structures that encode function within the brain. In this study, we proposed a reproducible and objective method, yet dynamic with respect to the chosen area of interest. Further work on this method in relation to tumor remnants and/or neurological and neurocognitive postoperative problems will be explored in future work.
A major strength of the present technique is that it is available for all routinely MRI scanned patients. Its simplicity makes it even accessible in context where DTI is not available or when exposure to DTI scanning times is not achievable. The main limitations of the present technique concern the approximation inherent to the atlas approach together with other limitations intrinsic to DTI-tractography techniques (33).
In this report, we aimed to present a general comparison between groups of patients with dLGG. Ideally, and especially in light of 2021 fifth edition of the WHO Classification of the Tumors of the Central Nervous System, a further subtyping of the IDH wild-type tumors into glioblastoma or pediatric-type gliomas would be preferable (56). Similarly, the astrocytoma IDH-mutant grade 4, as identified through CDKN2A/B homozygous deletions, could preferably have been excluded from our cohort. But since the vast majority of non-enhancing gliomas IDH wild type in adults are molecular glioblastomas, and that CDKN2A/B homozygous deletion is rare in the WHO grade 2 group (61), we believe that the results at the group level would not differ from what we have presented.
We chose to include tumor maps of molecular markers as corroboration of findings in previous studies to strengthen the external validity of our model. Despite its simplicity, the linear registration method has been previously benchmarked showing comparably accurate results as nonlinear registration for glioma localization (21, 62). For other aims, a different selection of ROI and/or a more restrictive overlapping threshold could also be applied. In this sense, a voxel-based approach is dynamic but without compromising reproducibility and objectiveness.
A consistent difference can be observed when comparing tumor location heatmaps with tables and plots over the proximity of a tumor to the eloquent areas. While percentages on heatmaps represent voxel-wise overlap, percentages on tables and plots are based on a binary identification of this overlap. Thus, precise quantification of the severity of the presumable lesion caused by the tumor is a remaining challenge. In this study, as a surrogate measure of the lesion, we used a binary identification for when a given ROI was presumably intersected by a tumor. However, this only provides an approximation of the overall involvement of the ROI, and in many instances, this will only mean proximity as tracts may be dislocated rather than infiltrated.
We have reported overall diffuse lower-grade gliomas (WHO grades 2 and 3) distributions with special emphasis on eloquent areas with a simple and robust method, which may facilitate the reporting of neurosurgical eloquence in an unbiased and comparable, yet dynamic manner.
A biological gradient was observed with most eloquent regions involved in IDH wild-type astrocytomas and least critical regions involved in oligodendrogliomas. The regions included in our voxel-based definition of eloquence showed a high degree of association with performing biopsy compared to resection, demonstrating its relevance in clinical practice.
The raw data supporting the conclusions of this article will be made available by the authors upon reasonable request, without undue reservation.
The studies involving human participants were reviewed and approved by the Regional Committee for Medical and Health Research Ethics in Central Norway (REC reference 2017/1780) and by the Regional Committee of Western Sweden (EPN reference 705/17). All data were pseudo-anonymized; biological material was not shared across institutions. The need for informed consent was waived by both committees. Written informed consent for participation was not required for this study in accordance with the national legislation and the institutional requirements.
All authors participated equally in the initial design and final reviewing of the manuscript. TG contributed with planning of the study, data curation, data analysis, and drafting of the manuscript, including design of figures and tables, revising, and submission of the manuscript. AN contributed with data curation, computational scripting and design of figures, reviewing the manuscript, and approval of the final manuscript. AC contributed by recruiting patients, tumor segmentations, reviewing manuscript, and approval of the final manuscript. JB and MJ contributed by recruiting patients, tumor segmentations, reviewing the manuscript, and approval of the final manuscript. OS contributed by planning of the study, recruiting patients, reviewing the manuscript, and approval of the final manuscript. IR contributed with methodological input, reviewing the manuscript, and approval of the final manuscript. AJ contributed with planning of the study, establishing partnerships across institutions, recruiting patients, evaluating the initial tumor segmentations, reviewing the manuscript, and approval of the final manuscript. All authors contributed to the article and approved the submitted version.
AJ holds research grants from the Swedish Research Council (2017–00944) and the agreement between the Swedish government and the county councils (the ALF agreement, ALFGBG-716671).
The authors declare that the research was conducted in the absence of any commercial or financial relationships that could be construed as a potential conflict of interest.
All claims expressed in this article are solely those of the authors and do not necessarily represent those of their affiliated organizations, or those of the publisher, the editors and the reviewers. Any product that may be evaluated in this article, or claim that may be made by its manufacturer, is not guaranteed or endorsed by the publisher.
The Supplementary Material for this article can be found online at: https://www.frontiersin.org/articles/10.3389/fonc.2021.748229/full#supplementary-material
1. Kommers I, Bouget D, Pedersen A, Eijgelaar RS, Ardon H, Barkhof F, et al. Glioblastoma Surgery Imaging—Reporting and Data System: Standardized Reporting of Tumor Volume, Location, and Resectability Based on Automated Segmentations. Cancers (2021) 13(12):2854. doi: 10.3390/cancers13122854
2. Ostrom QT, Cioffi G, Gittleman H, Patil N, Waite K, Kruchko C, et al. CBTRUS Statistical Report: Primary Brain and Other Central Nervous System Tumors Diagnosed in the United States in 2012-2016. Neuro Oncol (2019) 21(Suppl 5):v1–v100. doi: 10.1093/neuonc/noz150
3. Weller M, van den Bent M, Preusser M, Le Rhun E, Tonn JC, Minniti G, et al. EANO Guidelines on the Diagnosis and Treatment of Diffuse Gliomas of Adulthood. Nat Rev Clin Oncol (2021) 18(3):170–86. doi: 10.1038/s41571-020-00447-z
4. Duffau H, Capelle L. Preferential Brain Locations of Low-Grade Gliomas. Cancer (2004) 100(12):2622–6. doi: 10.1002/cncr.20297
5. Antonsson M, Jakola A, Longoni F, Carstam L, Hartelius L, Thordstein M, et al. Post-Surgical Effects on Language in Patients With Presumed Low-Grade Glioma. Acta Neurol Scand (2018) 137(5):469. doi: 10.1111/ane.12887
6. Suh CH, Kim HS, Jung SC, Choi CG, Kim SJ. Imaging Prediction of Isocitrate Dehydrogenase (IDH) Mutation in Patients With Glioma: A Systemic Review and Meta-Analysis. Eur Radiol (2019) 29(2):745–58. doi: 10.1007/s00330-018-5608-7
7. Enomoto T, Aoki M, Hamasaki M, Abe H, Nonaka M, Inoue T, et al. Midline Glioma in Adults: Clinicopathological, Genetic, and Epigenetic Analysis. Neurol Medico-chirurgica (2020) 60(3):136–46. doi: 10.2176/nmc.oa.2019-0168
8. Chang EF, Smith JS, Chang SM, Lamborn KR, Prados MD, Butowski N, et al. Preoperative Prognostic Classification System for Hemispheric Low-Grade Gliomas in Adults. J Neurosurg (2008) 109(5):817–24. doi: 10.3171/JNS/2008/109/11/0817
9. Sawaya R, Hammoud M, Schoppa D, Hess KR, Wu SZ, Shi WM, et al. Neurosurgical Outcomes in a Modern Series of 400 Craniotomies for Treatment of Parenchymal Tumors. Neurosurgery (1998) 42(5):1044–55; discussion 55-6. doi: 10.1097/00006123-199805000-00054
10. Jakola AS, Unsgard G, Myrmel KS, Kloster R, Torp SH, Lindal S, et al. Low Grade Gliomas in Eloquent Locations - Implications for Surgical Strategy, Survival and Long Term Quality of Life.(Research Article). PloS One (2012) 7(12):e51450. doi: 10.1371/journal.pone.0051450
11. Karnofsky D JHB. The Clinical Evaluation of Chemotherapeutic Agents in Cancer. In: MacLeod CM, editor. Evaluation of Chemotherapeutic Agents. New York, USA: Columbia University Press (1949). p. 191–205.
12. Louis DN, Perry A, Reifenberger G, von Deimling A, Figarella-Branger D, Cavenee WK, et al. The 2016 World Health Organization Classification of Tumors of the Central Nervous System: A Summary. Acta Neuropathol (2016) 131(6):803–20. doi: 10.1007/s00401-016-1545-1
13. Young JS, Gogos AJ, Morshed RA, Hervey-Jumper SL, Berger MS. Molecular Characteristics of Diffuse Lower Grade Gliomas: What Neurosurgeons Need to Know. Acta Neurochir (Wien) (2020) 162(8):1929–39. doi: 10.1007/s00701-020-04426-2
14. Ferreyra Vega S, Bontell TO, Corell A, Smits A, Jakola AS, Carén H. DNA Methylation Profiling for Molecular Classification of Adult Diffuse Lower-Grade Gliomas. Clin Epigenet (2021) 13(1):102. doi: 10.1186/s13148-021-01085-7
15. Fedorov A, Beichel R, Kalpathy-Cramer J, Finet J, Fillion-Robin JC, Pujol S, et al. 3d Slicer as an Image Computing Platform for the Quantitative Imaging Network. Magn Reson Imaging (2012) 30(9):1323–41. doi: 10.1016/j.mri.2012.05.001
16. Jenkinson M, Beckmann CF, Behrens TE, Woolrich MW, Smith SM. FSL. Neuroimage (2012) 62(2):782–90. doi: 10.1016/j.neuroimage.2011.09.015
17. Fonov V, Evans A, McKinstry RC, Almli CR, Collins DL. Unbiased Nonlinear Average Age-Appropriate Brain Templates From Birth to Adulthood. NeuroImage (Orlando Fla) (2009) 47:S102–S. doi: 10.1016/S1053-8119(09)70884-5
18. Catani M, Thiebaut de Schotten M. A Diffusion Tensor Imaging Tractography Atlas for Virtual In Vivo Dissections. Cortex (2008) 44(8):1105–32. doi: 10.1016/j.cortex.2008.05.004
19. Manera AL, Dadar M, Fonov V, Collins DL. CerebrA, Registration and Manual Label Correction of Mindboggle-101 Atlas for MNI-ICBM152 Template. Sci Data (2020) 7(1):237. doi: 10.1038/s41597-020-0557-9
20. Chang EF, Clark A, Smith JS, Polley MY, Chang SM, Barbaro NM, et al. Functional Mapping-Guided Resection of Low-Grade Gliomas in Eloquent Areas of the Brain: Improvement of Long-Term Survival. Clinical Article. J Neurosurg (2011) 114(3):566–73. doi: 10.3171/2010.6.JNS091246
21. Sarubbo S, Tate M, De Benedictis A, Merler S, Moritz-Gasser S, Herbet G, et al. Mapping Critical Cortical Hubs and White Matter Pathways by Direct Electrical Stimulation: An Original Functional Atlas of the Human Brain. Neuroimage (2020) 205:116237. doi: 10.1016/j.neuroimage.2019.116237
22. Duffau H. Updated Perspectives on Awake Neurosurgery With Cognitive and Emotional Assessment for Patients With Low-Grade Gliomas. Expert Rev Neurother (2021) 21(4):463–73. doi: 10.1080/14737175.2021.1901583
23. Martino J, De Witt Hamer PC, Berger MS, Lawton MT, Arnold CM, de Lucas EM, et al. Analysis of the Subcomponents and Cortical Terminations of the Perisylvian Superior Longitudinal Fasciculus: A Fiber Dissection and DTI Tractography Study. Brain Struct Funct (2013) 218(1):105–21. doi: 10.1007/s00429-012-0386-5
24. Foulon C, Cerliani L, Kinkingnehun S, Levy R, Rosso C, Urbanski M, et al. Advanced Lesion Symptom Mapping Analyses and Implementation as BCBtoolkit. Gigascience (2018) 7(3):1–17. doi: 10.1093/gigascience/giy004
25. Urbanski M, Brechemier ML, Garcin B, Bendetowicz D, Thiebaut de Schotten M, Foulon C, et al. Reasoning by Analogy Requires the Left Frontal Pole: Lesion-Deficit Mapping and Clinical Implications. Brain (2016) 139(Pt 6):1783–99. doi: 10.1093/brain/aww072
26. Thiebaut de Schotten M, Tomaiuolo F, Aiello M, Merola S, Silvetti M, Lecce F, et al. Damage to White Matter Pathways in Subacute and Chronic Spatial Neglect: A Group Study and 2 Single-Case Studies With Complete Virtual “In Vivo” Tractography Dissection. Cereb Cortex (2014) 24(3):691–706. doi: 10.1093/cercor/bhs351
27. Thiebaut de Schotten M, Ffytche DH, Bizzi A, Dell'Acqua F, Allin M, Walshe M, et al. Atlasing Location, Asymmetry and Inter-Subject Variability of White Matter Tracts in the Human Brain With MR Diffusion Tractography. Neuroimage (2011) 54(1):49–59. doi: 10.1016/j.neuroimage.2010.07.055
28. Harris CR, Millman KJ, van der Walt SJ, Gommers R, Virtanen P, Cournapeau D, et al. Array Programming With NumPy. Nature (2020) 585(7825):357–62. doi: 10.1038/s41586-020-2649-2
29. Lowekamp B, Chen D, Ibanez L, Blezek D. The Design of SimpleITK. Front Neuroinform (2013) 7(45):1–14. doi: 10.3389/fninf.2013.00045
30. McKinney W. Data Structures for Statistical Computing in Python. In: Proceedings of the 9th Python in Science Conference (2010). p. 56–61.
31. Hunter JD. Matplotlib: A 2d Graphics Environment. Comput Sci Eng (2007) 9(3):90–5. doi: 10.1109/MCSE.2007.55
32. Freyschlag CF, Krieg SM, Kerschbaumer J, Pinggera D, Forster MT, Cordier D, et al. Imaging Practice in Low-Grade Gliomas Among European Specialized Centers and Proposal for a Minimum Core of Imaging. J Neurooncol (2018) 139(3):699–711. doi: 10.1007/s11060-018-2916-3
33. Azad TD, Duffau H. Limitations of Functional Neuroimaging for Patient Selection and Surgical Planning in Glioma Surgery. Neurosurg Focus (2020) 48(2):E12. doi: 10.3171/2019.11.FOCUS19769
34. Wakana S, Caprihan A, Panzenboeck MM, Fallon JH, Perry M, Gollub RL, et al. Reproducibility of Quantitative Tractography Methods Applied to Cerebral White Matter. Neuroimage (2007) 36(3):630–44. doi: 10.1016/j.neuroimage.2007.02.049
35. Weng HH, Noll KR, Johnson JM, Prabhu SS, Tsai YH, Chang SW, et al. Accuracy of Presurgical Functional MR Imaging for Language Mapping of Brain Tumors: A Systematic Review and Meta-Analysis. Radiology (2018) 286(2):512–23. doi: 10.1148/radiol.2017162971
36. Conti Nibali M, Rossi M, Sciortino T, Riva M, Gay LG, Pessina F, et al. Preoperative Surgical Planning of Glioma: Limitations and Reliability of fMRI and DTI Tractography. J Neurosurg Sci (2019) 63(2):127–34. doi: 10.23736/s0390-5616.18.04597-6
37. Motomura K, Takeuchi H, Nojima I, Aoki K, Chalise L, Iijima K, et al. Navigated Repetitive Transcranial Magnetic Stimulation as Preoperative Assessment in Patients With Brain Tumors. Sci Rep-Uk (2020) 10(1):9044. doi: 10.1038/s41598-020-65944-8
38. Maier-Hein KH, Neher PF, Houde JC, Cote MA, Garyfallidis E, Zhong J, et al. The Challenge of Mapping the Human Connectome Based on Diffusion Tractography. Nat Commun (2017) 8(1):1349. doi: 10.1038/s41467-017-01285-x
39. Parisot S, Darlix A, Baumann C, Zouaoui S, Yordanova Y, Blonski M, et al. A Probabilistic Atlas of Diffuse WHO Grade II Glioma Locations in the Brain. PloS One (2016) 11(1):e0144200. doi: 10.1371/journal.pone.0144200
40. Wang Y, Zhang T, Li S, Fan X, Ma J, Wang L, et al. Anatomical Localization of Isocitrate Dehydrogenase 1 Mutation: A Voxel-Based Radiographic Study of 146 Low-Grade Gliomas. Eur J Neurol (2015) 22(2):348–54. doi: 10.1111/ene.12578
41. Ius T, Angelini E, Thiebaut de Schotten M, Mandonnet E, Duffau H. Evidence for Potentials and Limitations of Brain Plasticity Using an Atlas of Functional Resectability of WHO Grade II Gliomas: Towards a “Minimal Common Brain”. Neuroimage (2011) 56(3):992–1000. doi: 10.1016/j.neuroimage.2011.03.022
42. Larjavaara S, Mantyla R, Salminen T, Haapasalo H, Raitanen J, Jaaskelainen J, et al. Incidence of Gliomas by Anatomic Location. Neuro Oncol (2007) 9(3):319–25. doi: 10.1215/15228517-2007-016
43. Skjulsvik AJ, Bo HK, Jakola AS, Berntsen EM, Bo LE, Reinertsen I, et al. Is the Anatomical Distribution of Low-Grade Gliomas Linked to Regions of Gliogenesis? J Neurooncol (2020) 147(1):147–57. doi: 10.1007/s11060-020-03409-8
44. Darlix A, Deverdun J, Menjot de Champfleur N, Castan F, Zouaoui S, Rigau V, et al. IDH Mutation and 1p19q Codeletion Distinguish Two Radiological Patterns of Diffuse Low-Grade Gliomas. J Neuro-Oncol (2017) 133(1):37–45. doi: 10.1007/s11060-017-2421-0
45. Latini F, Fahlstrom M, Hesselager G, Zetterling M, Ryttlefors M. Differences in the Preferential Location and Invasiveness of Diffuse Low-Grade Gliomas and Their Impact on Outcome. Cancer Med (2020) 9(15):5446–58. doi: 10.1002/cam4.3216
46. Tejada Neyra MA, Neuberger U, Reinhardt A, Brugnara G, Bonekamp D, Sill M, et al. Voxel-Wise Radiogenomic Mapping of Tumor Location With Key Molecular Alterations in Patients With Glioma. Neuro-Oncology (2018) 20(11):1517–24. doi: 10.1093/neuonc/noy134
47. Tang Q, Lian Y, Yu J, Wang Y, Shi Z, Chen L. Anatomic Mapping of Molecular Subtypes in Diffuse Glioma. BMC Neurol (2017) 17(1):183. doi: 10.1186/s12883-017-0961-8
48. Beiko J, Suki D, Hess KR, Fox BD, Cheung V, Cabral M, et al. IDH1 Mutant Malignant Astrocytomas are More Amenable to Surgical Resection and Have a Survival Benefit Associated With Maximal Surgical Resection. Neuro Oncol (2014) 16(1):81–91. doi: 10.1093/neuonc/not159
49. Qi S, Yu L, Li H, Ou Y, Qiu X, Ding Y, et al. Isocitrate Dehydrogenase Mutation is Associated With Tumor Location and Magnetic Resonance Imaging Characteristics in Astrocytic Neoplasms. Oncol Lett (2014) 7(6):1895–902. doi: 10.3892/ol.2014.2013
50. Wijnenga MMJ, van der Voort SR, French PJ, Klein S, Dubbink HJ, Dinjens WNM, et al. Differences in Spatial Distribution Between WHO 2016 Low-Grade Glioma Molecular Subgroups. Neuro-Oncol Adv (2019) 1(1):1–9. doi: 10.1093/noajnl/vdz001
51. Wijnenga MMJ, French PJ, Dubbink HJ, Dinjens WNM, Atmodimedjo PN, Kros JM, et al. The Impact of Surgery in Molecularly Defined Low-Grade Glioma: An Integrated Clinical, Radiological, and Molecular Analysis. Neuro Oncol (2018) 20(1):103–12. doi: 10.1093/neuonc/nox176
52. Smith JS, Chang EF, Lamborn KR, Chang SM, Prados MD, Cha S, et al. Role of Extent of Resection in the Long-Term Outcome of Low-Grade Hemispheric Gliomas. J Clin Oncol (2008) 26(8):1338–45. doi: 10.1200/jco.2007.13.9337
53. Capelle L, Fontaine D, Mandonnet E, Taillandier L, Golmard JL, Bauchet L, et al. Spontaneous and Therapeutic Prognostic Factors in Adult Hemispheric World Health Organization Grade II Gliomas: A Series of 1097 Cases: Clinical Article. J Neurosurg (2013) 118(6):1157–68. doi: 10.3171/2013.1.JNS121
54. Kim Y-H, Nobusawa S, Mittelbronn M, Paulus W, Brokinkel B, Keyvani K, et al. Molecular Classification of Low-Grade Diffuse Gliomas. Am J Pathol (2010) 177(6):2708–14. doi: 10.2353/ajpath.2010.100680
55. Brat DJ, Verhaak RG, Aldape KD, Yung WK, Salama SR, Cooper LA, et al. Comprehensive, Integrative Genomic Analysis of Diffuse Lower-Grade Gliomas. N Engl J Med (2015) 372(26):2481–98. doi: 10.1056/NEJMoa1402121
56. Louis DN, Perry A, Wesseling P, Brat DJ, Cree IA, Figarella-Branger D, et al. The 2021 WHO Classification of Tumors of the Central Nervous System: A Summary. Neuro Oncol (2021) 23(8):1231–51. doi: 10.1093/neuonc/noab106
57. Kahn E, Lane M, Sagher O. Eloquent: History of a Word’s Adoption Into the Neurosurgical Lexicon. J Neurosurg JNS (2017) 127(6):1461–6. doi: 10.3171/2017.3.JNS17659
58. Chang EF, Clark A, Jensen RL, Bernstein M, Guha A, Carrabba G, et al. Multiinstitutional Validation of the University of California at San Francisco Low-Grade Glioma Prognostic Scoring System. Clinical Article. J Neurosurg (2009) 111(2):203–10. doi: 10.3171/2009.2.Jns081101
59. Friedlein K, Bozhkov Y, Hore N, Merkel A, Sommer B, Brandner S, et al. A New Functional Classification System (FGA/B) With Prognostic Value for Glioma Patients. Sci Rep (2015) 5:12373. doi: 10.1038/srep12373
60. Sagberg LM, Iversen DH, Fyllingen EH, Jakola AS, Reinertsen I, Solheim O. Brain Atlas for Assessing the Impact of Tumor Location on Perioperative Quality of Life in Patients With High-Grade Glioma: A Prospective Population-Based Cohort Study. NeuroImage Clin (2019) 21:101658. doi: 10.1016/j.nicl.2019.101658
61. Shirahata M, Ono T, Stichel D, Schrimpf D, Reuss DE, Sahm F, et al. Novel, Improved Grading System(s) for IDH-Mutant Astrocytic Gliomas. Acta Neuropathol (2018) 136(1):153–66. doi: 10.1007/s00401-018-1849-4
Keywords: glioma grade 2, glioma grade 3, surgical oncology (Mesh), diagnostic imaging—methods, magnetic resonance imaging—methods, neurologic deficit
Citation: Gómez Vecchio T, Neimantaite A, Corell A, Bartek J Jr, Jensdottir M, Reinertsen I, Solheim O and Jakola AS (2021) Lower-Grade Gliomas: An Epidemiological Voxel-Based Analysis of Location and Proximity to Eloquent Regions. Front. Oncol. 11:748229. doi: 10.3389/fonc.2021.748229
Received: 27 July 2021; Accepted: 27 August 2021;
Published: 21 September 2021.
Edited by:
Metin Gurcan, Wake Forest University, United StatesReviewed by:
Karsten Wrede, University of Duisburg-Essen, GermanyCopyright © 2021 Gómez Vecchio, Neimantaite, Corell, Bartek, Jensdottir, Reinertsen, Solheim and Jakola. This is an open-access article distributed under the terms of the Creative Commons Attribution License (CC BY). The use, distribution or reproduction in other forums is permitted, provided the original author(s) and the copyright owner(s) are credited and that the original publication in this journal is cited, in accordance with accepted academic practice. No use, distribution or reproduction is permitted which does not comply with these terms.
*Correspondence: Tomás Gómez Vecchio, dG9tYXMuZ29tZXoudmVjY2hpb0BndS5zZQ==
Disclaimer: All claims expressed in this article are solely those of the authors and do not necessarily represent those of their affiliated organizations, or those of the publisher, the editors and the reviewers. Any product that may be evaluated in this article or claim that may be made by its manufacturer is not guaranteed or endorsed by the publisher.
Research integrity at Frontiers
Learn more about the work of our research integrity team to safeguard the quality of each article we publish.