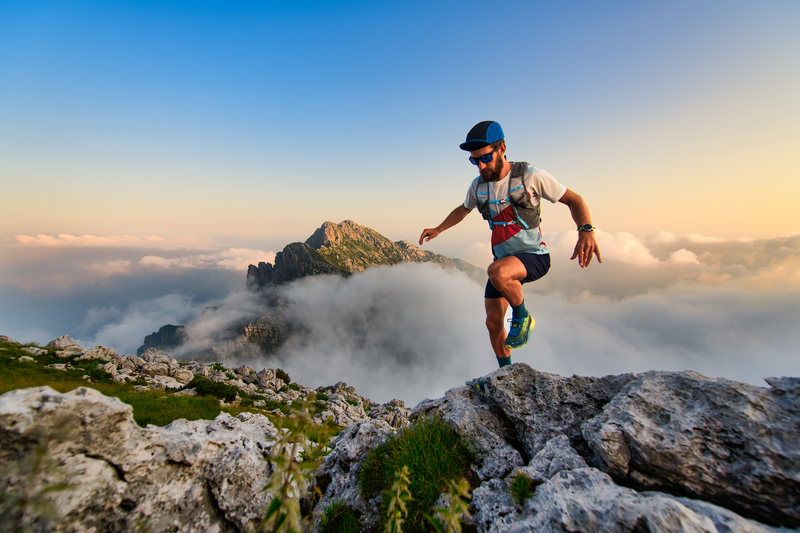
94% of researchers rate our articles as excellent or good
Learn more about the work of our research integrity team to safeguard the quality of each article we publish.
Find out more
ORIGINAL RESEARCH article
Front. Oncol. , 30 August 2021
Sec. Cancer Epidemiology and Prevention
Volume 11 - 2021 | https://doi.org/10.3389/fonc.2021.729471
Background: There are rare prediction models for esophageal squamous cell carcinoma (ESCC) for rural Chinese population. We aimed to develop and validate a prediction model for ESCC based on a cohort study for the population.
Methods: Data of 115,686 participants were collected from esophageal cancer (EC) early diagnosis and treatment of cancer program as derivation cohort while data of 54,750 participants were collected as validation cohort. Risk factors considered included age, sex, smoking status, alcohol drinking status, body mass index (BMI), tea drinking status, marital status, annual household income, source of drinking water, education level, and diet habit. Cox proportional hazards model was used to develop ESCC prediction model at 5 years. Calibration ability, discrimination ability, and decision curve analysis were analyzed in both derivation and validation cohort. A score model was developed based on prediction model.
Results: One hundred eighty-six cases were diagnosed during 556,949.40 person-years follow-up in the derivation cohort while 120 cases from 277,302.70 in the validation cohort. Prediction model included the following variables: age, sex, alcohol drinking status, BMI, tea drinking status, and fresh fruit. The model had good discrimination and calibration performance: R2, D statistic, and Harrell’s C statistic of prediction model were 43.56%, 1.70, and 0.798 in derivation cohort and 45.19%, 1.62, and 0.787 in validation cohort. The calibration analysis showed good coherence between predicted probabilities and observed probabilities while decision curve analysis showed clinical usefulness. The score model was as follows: age (3 for 45–49 years old; 4 for 50–54 years old; 7 for 55–59 years old; 9 for 60–64 years; 10 for 65–69 years), sex (5 for men), BMI (1 for ≤25), alcohol drinking status (2 for alcohol drinkers), tea drinking status (2 for tea drinkers), and fresh fruit (2 for never) and showed good discrimination ability with area under the curve and its 95% confidence interval of 0.792 (0.761,0.822) in the deviation cohort and 0.773 (0.736,0.811) in the validation cohort. The calibration analysis showed great coherence between predicted probabilities and observed probabilities.
Conclusions: We developed and validated an ESCC prediction model using cohort study with good discrimination and calibration capability which can be used for EC screening for rural Chinese population.
Cancer is a particularly important health problem among noncommunicable diseases and is also the first or second cause of premature mortality in more than 90 countries across the world (1). Esophageal cancer (EC) remains a major cause of cancer mortality and burden across the world despite reductions in age-standardized incidence and mortality rates and is of great difference between incidence rate of EC among different races and regions (2, 3). As the most populous country across the world, more than 23% of new cancer cases, about 30% of cancer deaths, and about half of the new cases of EC worldwide occur in China (4). Also, ESCC accounts for 90% of EC cases and is the main type of EC in China (5–7).
Endoscopy examination is one of the main inspection modality for ESCC or precursor lesion and endoscopic screening programs have been performed for high-risk populations across the world (8, 9). Several screening programs for EC have been carried out in China, as follows: a community-based endoscopic screening for esophageal cancer for residents aged 25–65 years from six villages in Hua County, Henan Province, China which was carried out from 2007 (10); regional organized esophageal cancer screening programs since 2005 in endemic areas in China for 40–69-year-old adults (11, 12); endoscopic screening for esophageal cancer in China (ESECC), a cluster RCT, carried out from January 2012 to September 2016 for people aged 45–69 years (13, 14); “Taihang Anti-cancer Campaign” including three stations in Linxian in Henan Province, Cixian in Hebei Province and Yangchen in Shanxi Province for people aged ≥40 years old (15); a population-based case-control study from October 2010 to September 2013 in Taixing for participants aged 40–84 years (16); and the Esophageal, Stomach, Liver Cancer Screening Program (ESLCSP) carried out in four provinces: Jiangsu, Anhui, Shandong, and Henan in China for people aged 40 to 69 years (17). For people with special situations, such as, a history of head-and-neck cancers (18, 19), high-risk population-tylosis, disease onset for Achalasia, injury history of caustic esophageal injury (11), and Barrett’s esophagus may be advised to have regular endoscopic examinations (8, 20).
In 2006, a national early diagnosis and treatment guideline for EC was introduced and stated that cancer screening was to be performed by endoscopy accompanied with iodine staining and indicative biopsy in high-risk population in China (21, 22). Since then, rural residents aged 40 to 69 years participated in the cancer screening at the national esophageal cancer early diagnosis and treatment base. The prevalence of ESCC and precancerous lesions, risk factors associated with ESCC and precancerous lesions, the survival of patients with precancerous lesion, early cancer, and advanced cancer after treatment had been reported basically based on the cancer screening data (23–27).
To the best of our knowledge, there are few risk prediction models for ESCC. Previous prediction models were developed in China, Japan, Sweden, and Iran population based on case-control studies (28–31) and were also developed based on the cohort study in China and from the Nord-Trondelag Health Study (17, 32). These models show good discriminative performance as area under the receiver operating curve (AUC) ranged from 0.76 to 0.81 and were mainly based on risk factors such as age, sex, smoking, alcohol drinking, body mass index (BMI), diet habits like consumption of fresh fruits, salted food, alarming symptoms of retrosternal pain, alarming symptoms of back pain, alarming symptoms of neck pain, family history of upper gastrointestinal cancer, and disease history of esophagitis or peptic ulcer. The previous models have the following limitations: (1) the factors used in previous models are difficult to obtain or quantified such as alarming symptoms; (2) lack of external validation; and (3) most of them were based on case-control studies.
The aim of this paper was to develop a risk prediction model of ESCC for rural Chinese population and tested its performance in both derivation cohort and validation cohort.
This study was based on EC cancer screening data based on early diagnosis and treatment program from centers of the cancer prevention and treatment in Shandong province. Supplementary Figure S1 shows the screening procedure, and it had been described in detail elsewhere (22). Briefly, before cancer screening, an informed consent form would be signed and a questionnaire including personal basic information, risk factors of esophageal cancer, family history, and simple physical examination should be filled. The participants were then assessed whether they were suitable for endoscopy and endoscopy examination was performed.
The derivation cohort came from cancer prevention and treatment centers except in Feicheng city in Shandong province, and the data were collected from 2012 to 2018. The validation cohort came from the centers in Feicheng city, and data were collected from 2012 to 2019.
Inclusion criteria of the study were as follows: (a) participants enrolled in the early diagnosis and treatment of cancer programs from 2012 in Shandong province; (b) participants aged older than 39 and younger than 70 years; (c) participants did not have a history of cancer recorded by the cancer registry and were not diagnosed of cancer at baseline; (d) participants were not diagnosed with dysplasia or worse at baseline by pathologic diagnosis; (e) participants who had filled the baseline questionnaire survey correctly; and (f) participants who had signed informed consent.
The data that included questionnaire information, endoscopic examination results, and pathologic diagnosis of participants were collected in the derivation cohort and validation cohort. The questionnaire information included age, sex, height, weight, marital status, education level, source of drinking water, smoking, drinking, diet habits, history of gastrointestinal diseases and age diagnosed, family history of cancer, etc. The results of endoscopic examination included location, size and shape of lesions, and endoscopic diagnosis. Biopsies were taken at the site of the lesion, and the pathological diagnosis was made by biopsy. Pathological diagnosis was recorded as each participant’s highest-level diagnosis. Endoscopy, specimen treatment, and pathologic diagnosis are referred to Chinese cancer screening, early diagnosis, and early treatment technology published by the People’s Health Publishing House.
The risk factors to be studied were selected according to previous research results, review articles, etc. (17, 28, 31, 33–42). The candidate variables were age, sex, BMI, smoking status, alcohol drinking status, tea drinking status, annual household income, marital status, education level, source of drinking water and diet habit of fresh fruit, high-temperature food, fried food, and pickled food. Age was divided into six categories: 40–44, 45–49, 50–54, 55–59, 60–64, or 65–69 years old. BMI was divided into two categories: 0 (>25 kg/m2) and 1 (≤25 kg/m2). Smoking status was divided into two categories: 0 (nonsmokers) and 1 (smokers). Alcohol drinking status was also divided into two categories: 0 (nonalcohol drinkers) and 1 (alcohol drinkers). Tea drinking status was also divided into two categories: 0 (nontea drinkers) and 1 (tea drinkers). Annual household income was divided into four categories (low, medium-low, medium-high, and high) according to the quantile. Marital status was divided into two categories: 0 (single, divorced, or widowed participants) and 1 (married participants). Education level was divided into two categories: 0 (junior high school or lower level) and 1 (senior high school or higher level). Source of drinking water was divided into two categories: 0 (untreated water: water obtained from spring, well, or river) and 1 (treated water: running water or purified water). Diet habit of fresh fruit and high-temperature food was divided into two categories: 0 (yes, had eaten the food) and 1 (never). Diet habit of pickled food and fried food was divided into two categories: 0 (less than twice a week on average) and 1 (two times or more per week on average).
New ESCC were diagnosed according to the International Classification of Diseases, 10th version as well as histology (ICD-O morphology codes). The follow-up time of the derivation cohort and validation cohort was up to December 31, 2020. The outcome was confirmed by cancer registration data.
The risk predictors in the prediction model were determined using three steps. The first step was fitting full model with all candidate variables using a multivariable Cox regression model. The variable was eliminated from the full model if its coefficient was greater than 0.90 and less than 1.10 and was not statistically significant at level 0.01 in the second step (43, 44). In the third step, the candidate variables eliminated in the second step was added into the multivariable model one by one and if performance statistics of the model were improved significantly, they would be added to the model again. We examined interactions between variables and age in the final model and included significant interactions at level 0.05 into the final model. We computed a 5-year risk prediction model of ESCC in the derivation cohort and used complete case for all analyses.
R2 value, D statistic and its 95% confidence interval (95% CI), Harrell’s C statistic and its 95% CI, calibration curve and decision curve analysis were used to test the model performance (43, 44). R2 value means the variation the model explained, and higher value of R2 is better. D statistic and Harrell’s C statistic are measures of discriminative ability, and higher values mean better discrimination performance. Harrell’s C is similar to AUC but considers the censored nature of the survival data. We also calculated AUC and its 95% CI as well as Somers’ D statistic for comparison with other models previously established. Calibration curves were used to assessed the agreement of predicted probability and observed probability (45). Calibration-in-the-large (A) and calibration slope (B) was calculated (46). The closer A is to 0 and the closer B is to 1, the better the model calibration performance is. Decision curve analysis was performed for clinical use and higher net-benefit indicated better clinical usefulness.
We tested the performance of the 5-year ESCC prediction model in validation cohort. R2 value, D statistic and 95% CI, Harrell’s C statistic and 95% CI, calibration curve, and decision curve were used to do the external validation.
The score model was derived based on the prediction model. The risk scores of each risk predictor were calculated by its corresponding coefficient dividing the minimum coefficient from the prediction model. The risk scores were finally derived by rounding to the nearest 1 (47). The total scores of participants were obtained by calculating the scores of each risk factor. AUC (95% CI), Somers’ D statistics, and calibration curve were used to test the performance of this score model both in the derivation cohort and validation cohort.
We comprehensively estimated the performance of each cutoff value in the score model. We calculate proportion of high-risk population whose total score was more than the cutoff value. The validity was evaluated by sensitivity, specificity, Youden’s index, and likelihood ratio including positive likelihood ratio and negative likelihood ratio. We also calculate accuracy rate and predictive value including positive predictive value and negative predictive value. The number needed to be screened and the predicted risk of developing ESCC within 5 years were also calculated. The candidate cutoff value should have a higher Youden’s index and a similar proportion of high-risk population to that reported before.
Participants diagnosed with dysplasia were reentered into the derivation cohort and validation cohort to perform sensitivity analyses. The R2 value, D statistic and 95% CI, and Harrell’s C statistic and 95% CI were also used for sensitivity analyses. We also did the predictor selection progress using forward stepwise method, and the AIC optimization criterion was used.
The analyses were performed using R software version 4.0.4 (https://www.r-project.org/). Package survival was used for Cox regression analysis. Package cmprsk and package ggDCA were used for decision curve analysis.
One hundred fifteen thousand six hundred eighty-six individuals were included in the derivation cohort after excluding 14,047 participants with any cancer diagnosis or dysplasia, 3,794 participants aged older than 69 or younger than 40 years, 2,166 participants with missing value of risk factors in Table 3, and 199 participants with incorrect investigation data as indicated in Figure 1. Supplementary Figures S2–S6 show the cumulative incidence of ESCC by age, sex, BMI, alcohol drinking status, and tea drinking status in the derivation cohort. For the validation cohort, 54,750 individuals were included after excluding 6,423 participants with any cancer diagnosis or dysplasia, 2,080 participants aged older than 69 or younger than 40 years, 4,692 participants with missing value of risk factors in Table 3, and 340 participants with incorrect investigation data as indicated in Figure 1.
Figure 1 Flow chart of the study population data collation including derivation cohort and validation cohort.
Table 1 shows the characteristics in the derivation cohort and validation cohort. Women accounted for 57.70% of the derivation cohort and 60.42% of the validation cohort, participants younger than 50 years old accounted for 27.32% and 31.90%, respectively, BMI greater than 25 accounted for 35.21% and 45.68%, respectively, smokers accounted for 21.37% and 39.19%, respectively, alcohol drinkers accounted for 21.34% and 41.33%, respectively, tea drinkers accounted for 22.24% and 71.95%, respectively, low level of annual household income accounted for 17.13% and 33.51%, respectively, participants married accounted for 95.85% and 95.61%, respectively, participants drinking treated water accounted for 70.63% and 28.88%, respectively, participants with senior high school or higher education level accounted for 49.61% and 60.66%, respectively, participants never has fresh fruit accounted for 13.29% and 7.49%, respectively, participants had high-temperature food accounted for 82.90% and 43.92%, respectively, participants with low level of fried food accounted for 34.53% and 91.41%, respectively, and participants with high level of pickled food intake accounted for 53.81% and 28.64%, respectively.
Table 2 shows the incidence rate in the derivation cohort and validation cohort. A total of 186 new cases of ESCC were diagnosed during the 556,949.40 person-years follow-up in the derivation cohort. The incidence rate of ESCC was 33.40 per 100,000 person-years. The incidence rate of ESCC was 13.52 per 100,000 person-years for women and 61.35 for men. The average follow-up time was 4.81 years. The incidence rate of ESCC increased with age.
Table 2 Incidence rates of ESCC per 100,000 person-years in the derivation cohort and validation cohort.
In the validation cohort, a total of 120 new cases of ESCC were diagnosed during the 277,302.70 person-years follow-up. The incidence rate of ESCC was 43.27 per 100,000 person-years in the validation cohort. The incidence rate of ESCC was 19.57 per 100,000 person-years for women and 80.03 for man. The average follow-up time was 5.06 years. The incidence rate of ESCC also increased with age.
Table 3 shows the adjusted hazard ratios of risk factors in multivariable regression model. The final prediction model included risk factors, such as, age, sex, BMI, alcohol drinking status, tea drinking status, and fresh fruit after selecting procedure. There was no statistically significant interaction between age and other variables in the prediction model. In the prediction model, men were associated with 236% increased risk of ESCC, BMI lower than 25 was associated with 30% increased risk of ESCC, alcohol drinkers were associated with 59% increased risk of ESCC, tea drinkers were associated with 51% increased risk of ESCC, and never had fresh fruit were associated with 52% increased risk of ESCC in derivation cohort.
Table 4 shows the discrimination performance of the prediction model in the derivation cohort and validation cohort. The prediction model explained 43.56% of the variation, the D statistic was 1.70 and Harrell’s C was 0.798 in the derivation cohort, and the corresponding values were 45.19%, 1.62 and 0.787 in the validation cohort.
Figure 2 shows observed proportion of ESCC and predicted risk of ESCC within 5 years for the prediction model in both the derivation cohort and validation cohort. The horizontal axis in the figure was the predicted probability of ESCC for 10 groups divided according to the quantile of predicted probability. The ordinate axis represented the observed proportion of ESCC corresponding to 10 groups. There was agreement between predicted risk and observed risk in the derivation cohort as the points in Figure 2 was close to the line with slope of 1 and intercept of 0 and A was 0.0000533 and B was 1.159. There was an agreement between the predicted risk and observed risk in the validation cohort as the points in Figure 2 was close to the line with slope of 1 and intercept of 0 and A was −0.0000122 and B was 1.066.
Figure 2 Observed proportion of ESCC and predicted risk of ESCC within 5 years in the derivation cohort and validation cohort. (A) The calibration plot of prediction model of derivation cohort; (B) the calibration plot of prediction model of validation cohort; (C) the calibration plot of score model of derivation cohort; (D) the calibration plot of score model of validation cohort. The A,B,C in the figures: (A) The intercept. (B) The slope. (C) The AUC value.
Figure 3 shows decision curves in the derivation cohort and validation cohort. The horizontal axis of this picture was threshold probability. When one’s risk of ESCC reached a certain threshold, it was defined as positive and some intervention measures were taken. The ordinate axis was the net benefit (NB) after the advantages were subtracted by the disadvantages. Prediction model had a higher NB than treating all the participants as high- or low-risk population. It indicated that the model developed in this study was clinical usefulness.
Figure 3 Decision curves in the derivation cohort and validation cohort. (A) The decision curve in the derivation cohort, (B) the decision curve in the validation cohort. The horizontal axis of this picture was threshold probability. The ordinate axis was the net benefit after the advantages were subtracted by the disadvantages.
Table 5 shows the risk scores of each risk factor in the score model: age (3 for 45–49 years old; 4 for 50–54 years old; 7 for 55–59 years old; 9 for 60–64 years; 10 for 65–69 years), sex (5 for men), BMI (1 for ≤25), alcohol drinking status (2 for alcohol drinkers), tea drinking status (2 for tea drinkers), and fresh fruit (2 for never). Supplementary Table S1 shows the score model has good discrimination ability with AUC (95%CI) of 0.792 (0.761, 0.822) in the deviation cohort and 0.773 (0.736, 0.811) in the validation cohort. There was an agreement between predicted risk and observed risk in the derivation cohort as the points in Figure 2 was close to the line with slope of 1 and intercept of 0 and A was −0.000132 and B was 1.490. There was an agreement between the predicted risk and observed risk in the validation cohort as the points in Figure 2 was close to the line with slope of 1 and intercept of 0 and A was −0.000112 and B was 1.070.
Supplementary Table S2 shows the performances of each cutoff value. As the cutoff value increased, the predicted risk of ESCC, specificity, and accuracy rate increased while the proportion of high-risk individuals and the sensitivity in detecting ESCC decreased. The Youden’s index first increased and then decreased as the cutoff value increased and reached its maximum when the cutoff value was 13. The total risk scores ranged from 0 to 22. Considering high-risk individuals, sensitivity, and Youden’s index, the candidate cutoff values of 12 and 13 could be selected as the criteria for identifying high-risk individuals for cancer screening.
The score of participants was used as a predictor to predict the incidence of ESCC. The regression coefficient was 0.245 as the score was a continuous variable. We then divided the participants into five categories using quantiles. Compared with the lowest quintile, the HR (95% CI) of the highest quintile was 46.47 (11.48, 188.07), the HR (95% CI) of the fourth quintile was 16.87 (4.07, 70.01), the HR (95% CI) of the third quintile was 7.30 (1.73, 30.89) and the HR (95% CI) of the second quintile was 1.92 (0.52, 10.47).
Supplementary Table S3 shows the result of sensitivity analysis. Sensitivity analysis showed that the model was stable without deletion of dysplasia at baseline with R2 of 43.82%, D statistic of 1.69, and Harrell’s C of 0.799 (0.769, 0.828) in the derivation cohort and R2 of 35.38%, D statistic of 1.33, and Harrell’s C of 0.729 (0.700, 0.758) in the validation cohort. Supplementary Table S4 shows the forward stepwise selection and the best combination of risk factors was age, sex, BMI, alcohol drinking status, tea drinking status, and fresh fruit as shown in Supplementary Table S4.
We developed and validated an individual 5-year risk prediction model of ESCC using cohort study for population aged 40 to 69 years and provided an efficient tool for rural Chinese in this study. The prediction model included six variables, such as, age, sex, BMI, alcohol drinking status, tea drinking status, and diet habit of fresh fruit and showed good discrimination and calibration ability in both the derivation cohort and validation cohort. Decision curve analysis showed that the prediction model had clinical usefulness. A score model was derived from the prediction model and also showed good discrimination and calibration ability in both the internal validation and external validation.
Since 2006, as the first batch of pilot, Feicheng city, a high incidence area of ESCC which the validation cohort came from, began the EC cancer screen program, and evidence had shown that the screen is associated with a reduction of the incidence of ESCC (48). It was reported that, from 2006 to 2016, the detection rate of precancerous lesions of ESCC was 6.81% in the Feicheng screening program (26). The research based on the screening data of Feicheng showed that alcohol drinking, men, tea drinking, etc. were the risk factors for the occurrence and development of precancerous lesions (24, 49, 50). After that, EC screening programs were carried out in other centers in rural China which the derivation cohort came from. The baseline characteristics and incidence rate of derivation cohort and validation cohort were different; however, the model established in this study performs well in both cohorts. The model was still stable after sensitivity analysis. Therefore, the model may be suitable for popularization and application. During the clinical practice in the EC screening program, the candidates for the screening were selected mainly based on age and whether they had contraindications of endoscopic screening due to being inconvenient of the evaluation tool. In this study, besides age, the risk factors in this study were divided into two groups, which was easy to obtain, can be accurately measured, and easy to apply in practical work.
There were rare prediction models for ESCC based on the cohort study. Chen et al. used logistic regression model and developed an ESCC prediction model based on multicenter cohort study in rural China without validation (17). The model included risk factors such as pain of back was difficult to obtain and quantify accurately. Wang et al. developed an ESCC prediction model based on Nord-Trondelag Health Study in Norway and validated the model in a cohort based on UK Biobank (32). The prediction model included five risk factors: age, sex, BMI, smoking status, and drinking status. The AUC of the 5-year ESCC prediction model was 0.76 in derivation cohort and was 0.70 in validation cohort. The classification criteria of the following risk factors such as drinking status are different. The etiology of ESCC is multifaceted, and there are great differences in various populations. According to the results of this study, tea drinking was a risk factor in the prediction model and that is meaningful because it is a very traditional habit among Chinese population.
This study focused attention on questionnaire-related risk factors other than genetic or other risk factors. Previous studies had shown that the risk of ESCC in men was several times higher than that in women, and the ratio varied greatly among different populations (51). In this study, incidence rate of ESCC in men was about three times of the incidence rate in women. Alcohol drinking was an important risk factor of ESCC and a dose-response relationship has been found (52–55). People with lower weight have a higher risk of ESCC (32). In this study, tea drinking is an important risk factor for ESCC, and the reason may be the high temperature of the tea (56, 57). Chinese traditional health-keeping philosophy believes that drinking hot liquids in the heat will increase sweating and benefit health. Drinking tea is a very common and popular habit in China and is also a traditional way of leisure and entertainment. The relationship between tea drinking and cancer is still unclear while green tea is generally considered to be cancer resistant because it contains catechin or polyphenols (58, 59). According to our study, preventive measures should be carried out for risk factors such as reducing alcohol drinking, reducing hot tea drinking, and keep proper weight.
This paper also has some limitations. (1) The data were collected from one province of China, which could not represent the 40- to 69-year population of China. On the other hand, Shandong is a big province in China and accounting for nearly one-tenth of the population of China. To some extent, it is also representative. (2) Although our cohort was large, the number of outcomes with esophageal cancer is small, which was likely to cause residual bias. This problem could be solved by longer follow-up. (3) There might have a recall bias for the risk factors. (4) We did not obtain the data of the following risk factors: PAH and trace element. We will add related questions to the questionnaire in the near future.
In summary, this paper provides a prediction model for ESCC with good discrimination and calibration in internal verification and external verification. Our prediction model is discriminative as previous established models with AUC of 0.796 in the derivation cohort and higher discriminative with AUC of 0.779 in the validation cohort as shown in the Supplementary Table S5. The prediction model offered a reliable and accurate prediction and can be used for screening program for ESCC. In the future, we will conduct more extensive external validation and adjust the model by adding other characteristics of participants.
In this study, we developed and validated an ESCC prediction model for rural Chinese population based on a multicenter cohort study. The prediction model included five risk factors: age, sex, BMI, alcohol drinking status, tea drinking status, and diet habit of fresh fruit. The prediction model showed good discrimination and calibration ability in both the derivation cohort and validation cohort. Decision curve analysis showed its clinical usefulness. We transferred the prediction model into a score model for clinical use, and the score model also showed good discrimination and calibration ability in the derivation cohort and validation cohort. We provided a simple and useful prediction tool for ESCC and may be used for ESCC cancer screen.
Data used in this research and related data could be offered upon reasonable request for the corresponding author.
The studies involving human participants were reviewed and approved by Ethics Committee of Public Health, Shandong University. The patients/participants provided their written informed consent to participate in this study.
JH and FX designed the study. JH carried out the analysis and wrote the first version of the manuscript. LW provided help in the process of data analysis. HZ, SM, ZW, GZ, YL, DZ, and JW helped with data collection and collation. All the authors participated in the discussion and agreed to publish the model in its current format. All authors contributed to the article and approved the submitted version.
This study is funded by the National Key R&D Program of China (2020YFC2003500) from the Ministry of Science and Technology of the People’s Republic of China.
The authors all declare that the research was conducted in the absence of any commercial or financial relationships that could be construed as a potential conflict of interest.
All claims expressed in this article are solely those of the authors and do not necessarily represent those of their affiliated organizations, or those of the publisher, the editors and the reviewers. Any product that may be evaluated in this article, or claim that may be made by its manufacturer, is not guaranteed or endorsed by the publisher.
The authors thank all individuals who provided help in the whole process of writing.
The Supplementary Material for this article can be found online at: https://www.frontiersin.org/articles/10.3389/fonc.2021.729471/full#supplementary-material
A, calibration in large; AUC, area under the receiver operating curve; B, calibration slope; BMI, body mass index; EC, esophageal cancer; ESCC, esophageal squamous cell carcinoma; ESECC, endoscopic screening for esophageal cancer in China; ESLCSP, the Esophageal, Stomach, Liver Cancer Screening Program; NB, net benefit; 95% CI, 95% confidence interval.
1. Wild CP, Weiderpass E, Stewart BW. eds. World Cancer Report: Cancer Research for Cancer Prevention. Lyon, France: International Agency for Research on Cancer (2020). Available at: http://publications.iarc.fr/586. Licence: CC BY-NC-ND3.0 IGO.
2. Kamangar F, Nasrollahzadeh D, Safiri S, Sepanlou SG, Fitzmaurice C, Ikuta KS, et al. The Global, Regional, and National Burden of Oesophageal Cancer and its Attributable Risk Factors in 195 Countries and Territories, 1990-2017: A Systematic Analysis for the Global Burden of Disease Study 2017. Lancet Gastroenterol Hepatol (2020) 5:582–97. doi: 10.1016/S2468-1253(20)30007-8
3. Smyth EC, Lagergren J, Fitzgerald RC, Lordick F, Shah MA, Lagergren P, et al. Oesophageal Cancer. Nat Rev Dis Primers (2017) 3:17048. doi: 10.1038/nrdp.2017.48
4. The Global Cancer Observatory Cancer Today. (2020). Available at: https://gco.iarc.fr/today/data/factsheets/cancers/6-Oesophagus-fact-sheet.pdf (Accessed December, 2020).
5. Rustgi A, El-Serag HB. Esophageal Carcinoma. N Engl J Med (2015) 372:1472–3. doi: 10.1056/NEJMc1500692
6. Abnet CC, Arnold M, Wei WQ. Epidemiology of Esophageal Squamous Cell Carcinoma. Gastroenterology (2018) 154:360–73. doi: 10.1053/j.gastro.2017.08.023
7. Lagergren J, Smyth E, Cunningham D, Lagergren P. Oesophageal Cancer. Lancet (2017) 390:2383–96. doi: 10.1016/S0140-6736(17)31462-9
8. Codipilly DC, Qin Y, Dawsey SM, Kisiel J, Topazian M, Ahlquist D, et al. Screening for Esophageal Squamous Cell Carcinoma: Recent Advances. Gastrointest Endosc (2018) 88:413–26. doi: 10.1016/j.gie.2018.04.2352
9. He Z, Ke Y. Precision Screening for Esophageal Squamous Cell Carcinoma in China. Chin J Cancer Res (2020) 32:673–82. doi: 10.21147/j.issn.1000-9604.2020.06.01
10. Liu M, He Z, Guo C, Xu R, Li F, Ning T, et al. Effectiveness of Intensive Endoscopic Screening for Esophageal Cancer in China: A Community-Based Study. Am J Epidemiol (2019) 188:776–84. doi: 10.1093/aje/kwy291
11. Chen W, Li H, Zheng R, Ren J, Shi J, Cao M, et al. An Initial Screening Strategy Based on Epidemiologic Information in Esophageal Cancer Screening: A Prospective Evaluation in a Community-Based Cancer Screening Cohort in Rural China. Gastrointest Endosc (2021) 93:110–8.e2. doi: 10.1016/j.gie.2020.05.052
12. Wei WQ, Hao CQ, Guan CT, Song GH, Wang M, Zhao DL, et al. Esophageal Histological Precursor Lesions and Subsequent 8.5-Year Cancer Risk in a Population-Based Prospective Study in China. Am J Gastroenterol (2020) 115:1036–44. doi: 10.14309/ajg.0000000000000640
13. Li F, Li X, Guo C, Xu R, Li F, Pan Y, et al. Estimation of Cost for Endoscopic Screening for Esophageal Cancer in a High-Risk Population in Rural China: Results From a Population-Level Randomized Controlled Trial. Pharmacoeconomics (2019) 37:819–27. doi: 10.1007/s40273-019-00766-9
14. He Z, Liu Z, Liu M, Guo C, Xu R, Li F, et al. Efficacy of Endoscopic Screening for Esophageal Cancer in China (ESECC): Design and Preliminary Results of a Population-Based Randomised Controlled Trial. Gut (2019) 68:198–206. doi: 10.1136/gutjnl-2017-315520
15. Dong Z, Tang P, Li L, Wang G. The Strategy for Esophageal Cancer Control in High-Risk Areas of China. Jpn J Clin Oncol (2002) 32 Suppl:S10–2. doi: 10.1093/jjco/hye122
16. Yang X, Suo C, Zhang T, Yin X, Man J, Yuan Z, et al. A Nomogram for Screening Esophageal Squamous Cell Carcinoma Based on Environmental Risk Factors in a High-Incidence Area of China: A Population-Based Case-Control Study. BMC Cancer (2021) 21:343. doi: 10.1186/s12885-021-08053-7
17. Chen W, Li H, Ren J, Zheng R, Shi J, Li J, et al. Selection of High-Risk Individuals for Esophageal Cancer Screening: A Prediction Model of Esophageal Squamous Cell Carcinoma Based on a Multicenter Screening Cohort in Rural China. Int J Cancer (2021) 148:329–39. doi: 10.1002/ijc.33208
18. Su YY, Chen WC, Chuang HC, Guo CS, Lin YT, Luo SD, et al. Effect of Routine Esophageal Screening in Patients With Head and Neck Cancer. JAMA Otolaryngol Head Neck Surg (2013) 139:350–4. doi: 10.1001/jamaoto.2013.46
19. Chung CS, Lo WC, Chen KC, Lin CL, Wen MH, Hsieh CH, et al. Clinical Benefits From Endoscopy Screening of Esophageal Second Primary Tumor for Head and Neck Cancer Patients: Analysis of a Hospital-Based Registry. Oral Oncol (2019) 96:27–33. doi: 10.1016/j.oraloncology.2019.06.038
20. Kim GH, Liang PS, Bang SJ, Hwang JH. Screening and Surveillance for Gastric Cancer in the United States: Is It Needed? Gastrointest Endosc (2016) 84:18–28. doi: 10.1016/j.gie.2016.02.028
21. Davydov Capital EM C, Delektorskaya VV, Kuvshinov YP, Lisovsky M, Pirogov SS, Udagawa H, et al. Superficial and Early Cancers of the Esophagus. Ann N Y Acad Sci (2014) 1325:159–69. doi: 10.1111/nyas.12527
22. Li YY, Du LB, Hu XQ, Jaiswal S, Gu SY, Gu YX, et al. A Suggested Framework for Conducting Esophageal Cancer Screening in China. J Digest Dis (2018) 19:722–9. doi: 10.1111/1751-2980.12675
23. Sun Y, Zhang T, Wu W, Zhao D, Zhang N, Cui Y, et al. Risk Factors Associated With Precancerous Lesions of Esophageal Squamous Cell Carcinoma: A Screening Study in a High Risk Chinese Population. J Cancer (2019) 10(14):3284–90. doi: 10.7150/jca.29979
24. Li X, Zhao L, Shi S, Yang J, Zhang N, Wang J. Analysis of the Risk Factors of Esophageal Cancer and Precancerous Lesions in Rural Areas of Feicheng City by Multivariate Ordinal Logistic Regression Analysis. Chin J Cancer Prev Treat (2020) 27:499–504. doi: 10.16073/j.cnki.cjcpt.2020.07.01
25. Li Y, Lu P, Zheng C, Wang G, Wei W, Zhao D, et al. Long Term Survival of Esophageal Squamous Cell Carcinoma and Precancerous Lesions in Feicheng City. Chin J Cancer Prev Treat (2020) 27:1183–8. doi: 10.16073/j.cnki.cjcpt.2020.15.01
26. Gao D, Li D, Li Y, Zhao D, Zhang N, Wang J. Analysis of Distribution and Outcome of Precancerous Lesions of Esophageal Squamous Cell Carcinoma in Rural Residents Aged 40-69 Years in Feicheng City. Chin J Cancer Prev Treat (2020) 27:583–9. doi: 10.16073/j.cnki.cjcpt.2020.08.01
27. Zhao T, Li Y, Chen R, Zhang N, Wang J, Wei W, et al. Long-Term Effects of Endoscopic Screening for Upper Gastrointestinal Cancer in Feicheng. Chin J Cancer Prev Treat (2020) 27:1470–5. doi: 10.16073/j.cnki.cjcpt.2020.18.06
28. Wang QL, Lagergren J, Xie SH. Prediction of Individuals at High Absolute Risk of Esophageal Squamous Cell Carcinoma. Gastrointest Endosc (2019) 89:726–32.e2. doi: 10.1016/j.gie.2018.10.025
29. Chang J, Huang Y, Wei L, Ma B, Miao X, Li Y, et al. Risk Prediction of Esophageal Squamous-Cell Carcinoma With Common Genetic Variants and Lifestyle Factors in Chinese Population. Carcinogenesis (2013) 34:1782–6. doi: 10.1093/carcin/bgt106
30. Etemadi A, Abnet CC, Golozar A, Malekzadeh R, Dawsey SM. Modeling the Risk of Esophageal Squamous Cell Carcinoma and Squamous Dysplasia in a High Risk Area in Iran. Arch Iran Med (2012) 15:18–21. doi: 012151/AIM.007
31. Yokoyama A, Oda J, Iriguchi Y, Kumagai Y, Okamura Y, Matsuoka M, et al. A Health-Risk Appraisal Model and Endoscopic Mass Screening for Esophageal Cancer in Japanese Men. Dis Esophagus (2013) 26:148–53. doi: 10.1111/j.1442-2050.2012.01343.x
32. Wang QL, Ness-Jensen E, Santoni G, Xie SH, Lagergren J. Development and Validation of a Risk Prediction Model for Esophageal Squamous Cell Carcinoma Using Cohort Studies. Am J Gastroenterol (2020) 116(4):683–91. doi: 10.14309/ajg.0000000000001094
33. Castro C, Peleteiro B, Lunet N. Modifiable Factors and Esophageal Cancer: A Systematic Review of Published Meta-Analyses. J Gastroenterol (2018) 53:37–51. doi: 10.1007/s00535-017-1375-5
34. Yokoyama A, Kumagai Y, Yokoyama T, Omori T, Kato H, Igaki H, et al. Health Risk Appraisal Models for Mass Screening for Esophageal and Pharyngeal Cancer: An Endoscopic Follow-Up Study of Cancer-Free Japanese Men. Cancer Epidemiol Biomarkers Prev (2009) 18:651–5. doi: 10.1158/1055-9965.EPI-08-0758
35. Yokoyama T, Yokoyama A, Kumagai Y, Omori T, Kato H, Igaki H, et al. Health Risk Appraisal Models for Mass Screening of Esophageal Cancer in Japanese Men. Cancer Epidemiol Biomarkers Prev (2008) 17:2846–54. doi: 10.1158/1055-9965.EPI-08-0397
36. Morita M, Kumashiro R, Kubo N, Nakashima Y, Yoshida R, Yoshinaga K, et al. Alcohol Drinking, Cigarette Smoking, and the Development of Squamous Cell Carcinoma of the Esophagus: Epidemiology, Clinical Findings, and Prevention. Int J Clin Oncol (2010) 15:126–34. doi: 10.1007/s10147-010-0056-7
37. Moaven O, Raziee HR, Sima HR, Ganji A, Malekzadeh R, A’Rabi A, et al. Interactions Between Glutathione-S-Transferase M1, T1 and P1 Polymorphisms and Smoking, and Increased Susceptibility to Esophageal Squamous Cell Carcinoma. Cancer Epidemiol (2010) 34:285–90. doi: 10.1016/j.canep.2010.03.009
38. Yokoyama A, Yokoyama T, Omori T, Maesato H, Takimura T, Iwahara C, et al. Endoscopic Screening Using Esophageal Iodine Staining and Genotypes of ADH1B and ALDH2 in Japanese Alcohol-Dependent Women. PloS One (2019) 14:e0210546. doi: 10.1371/journal.pone.0210546
39. Yokoyama A, Hirota T, Omori T, Yokoyama T, Kawakubo H, Matsui T, et al. Development of Squamous Neoplasia in Esophageal Iodine-Unstained Lesions and the Alcohol and Aldehyde Dehydrogenase Genotypes of Japanese Alcoholic Men. Int J Cancer (2012) 130:2949–60. doi: 10.1002/ijc.26296
40. Sheikh M, Poustchi H, Pourshams A, Etemadi A, Islami F, Khoshnia M, et al. Individual and Combined Effects of Environmental Risk Factors for Esophageal Cancer Based on Results From the Golestan Cohort Study. Gastroenterology (2019) 156:1416–27. doi: 10.1053/j.gastro.2018.12.024
41. Islami F, Pourshams A, Nasrollahzadeh D, Kamangar F, Fahimi S, Shakeri R, et al. Tea Drinking Habits and Oesophageal Cancer in a High Risk Area in Northern Iran: Population Based Case-Control Study. BMJ (2009) 338:b929. doi: 10.1136/bmj.b929
42. Golozar A, Etemadi A, Kamangar F, Fazeltabar MA, Islami F, Nasrollahzadeh D, et al. Food Preparation Methods, Drinking Water Source, and Esophageal Squamous Cell Carcinoma in the High-Risk Area of Golestan, Northeast Iran. Eur J Cancer Prev (2016) 25:123–9. doi: 10.1097/CEJ.0000000000000156
43. Hippisley-Cox J, Coupland C, Brindle P. Development and Validation of QRISK3 Risk Prediction Algorithms to Estimate Future Risk of Cardiovascular Disease: Prospective Cohort Study. BMJ (2017) 357:j2099. doi: 10.1136/bmj.j2099
44. Hippisley-Cox J, Coupland C. Development and Validation of QDiabetes-2018 Risk Prediction Algorithm to Estimate Future Risk of Type 2 Diabetes: Cohort Study. BMJ (2017) 359:j5019. doi: 10.1136/bmj.j5019
45. Xie SH, Lagergren J. A Model for Predicting Individuals’ Absolute Risk of Esophageal Adenocarcinoma: Moving Toward Tailored Screening and Prevention. Int J Cancer (2016) 138:2813–9. doi: 10.1002/ijc.29988
46. Steyerberg EW, Vergouwe Y. Towards Better Clinical Prediction Models: Seven Steps for Development and an ABCD for Validation. Eur Heart J (2014) 35:1925–31. doi: 10.1093/eurheartj/ehu207
47. Kunzmann AT, Thrift AP, Cardwell CR, Lagergren J, Xie S, Johnston BT, et al. Model for Identifying Individuals at Risk for Esophageal Adenocarcinoma. Clin Gastroenterol Hepatol (2018) 16:1229–36.e4. doi: 10.1016/j.cgh.2018.03.014
48. Zhang N, Li Y, Chang X, Lei F, Ma H, Liu J, et al. Long-Term Effectiveness of One-Time Endoscopic Screening for Esophageal Cancer: A Community-Based Study in Rural China. Cancer-Am Cancer Soc (2020) 126:4511–20. doi: 10.1002/cncr.33119
49. Gao D, Zhang N, Wang l. Analysis of Endoscopic Screening Results of 676 Cases of Esophageal Precancerous Lesion From 2006 to 2012 in Feicheng County,Shandong Province. China Cancer (2020) 29:334–8. doi: 10.11735/j.issn.1004-0242.2020.05.A003
50. Lu P, Gu J, Zhang N, Sun Y, Wang J. Risk Factors for Precancerous Lesions of Esophageal Squamous Cell Carcinoma in High-Risk Areas of Rural China: A Population-Based Screening Study. Med (Baltimore) (2020) 99:e21426. doi: 10.1097/MD.0000000000021426
51. Abnet CC, Arnold M, Wei WQ. Epidemiology of Esophageal Squamous Cell Carcinoma. Gastroenterology (2018) 154:360–73. doi: 10.1053/j.gastro.2017.08.023
52. Castellsague X, Munoz N, De Stefani E, Victora CG, Castelletto R, Rolon PA, et al. Independent and Joint Effects of Tobacco Smoking and Alcohol Drinking on the Risk of Esophageal Cancer in Men and Women. Int J Cancer (1999) 82:657–64. doi: 10.1002/(sici)1097-0215(19990827)82:5<657::aid-ijc7>3.0.co;2-c
53. Bagnardi V, Rota M, Botteri E, Tramacere I, Islami F, Fedirko V, et al. Alcohol Consumption and Site-Specific Cancer Risk: A Comprehensive Dose-Response Meta-Analysis. Br J Cancer (2015) 112:580–93. doi: 10.1038/bjc.2014.579
54. Freedman ND, Murray LJ, Kamangar F, Abnet CC, Cook MB, Nyren O, et al. Alcohol Intake and Risk of Oesophageal Adenocarcinoma: A Pooled Analysis From the BEACON Consortium. Gut (2011) 60:1029–37. doi: 10.1136/gut.2010.233866
55. Tran GD, Sun XD, Abnet CC, Fan JH, Dawsey SM, Dong ZW, et al. Prospective Study of Risk Factors for Esophageal and Gastric Cancers in the Linxian General Population Trial Cohort in China. Int J Cancer (2005) 113:456–63. doi: 10.1002/ijc.20616
56. Islami F, Poustchi H, Pourshams A, Khoshnia M, Gharavi A, Kamangar F, et al. A Prospective Study of Tea Drinking Temperature and Risk of Esophageal Squamous Cell Carcinoma. Int J Cancer (2020) 146:18–25. doi: 10.1002/ijc.32220
57. Yang X, Ni Y, Yuan Z, Chen H, Plymoth A, Jin L, et al. Very Hot Tea Drinking Increases Esophageal Squamous Cell Carcinoma Risk in a High-Risk Area of China: A Population-Based Case-Control Study. Clin Epidemiol (2018) 10:1307–20. doi: 10.2147/CLEP.S171615
58. Kim TL, Jeong GH, Yang JW, Lee KH, Kronbichler A, van der Vliet HJ, et al. Tea Consumption and Risk of Cancer: An Umbrella Review and Meta-Analysis of Observational Studies. Adv Nutr (2020) 11:1437–52. doi: 10.1093/advances/nmaa077
Keywords: esophageal squamous cell carcinoma, risk prediction, individualized assessment, China, Cox proportional hazards model
Citation: Han J, Wang L, Zhang H, Ma S, Li Y, Wang Z, Zhu G, Zhao D, Wang J and Xue F (2021) Development and Validation of an Esophageal Squamous Cell Carcinoma Risk Prediction Model for Rural Chinese: Multicenter Cohort Study. Front. Oncol. 11:729471. doi: 10.3389/fonc.2021.729471
Received: 23 June 2021; Accepted: 06 August 2021;
Published: 30 August 2021.
Edited by:
Imtiaz Ahmad Siddiqui, University of Colorado Anschutz Medical Campus, United StatesReviewed by:
Neetika Khurana, Northern Illinois University, United StatesCopyright © 2021 Han, Wang, Zhang, Ma, Li, Wang, Zhu, Zhao, Wang and Xue. This is an open-access article distributed under the terms of the Creative Commons Attribution License (CC BY). The use, distribution or reproduction in other forums is permitted, provided the original author(s) and the copyright owner(s) are credited and that the original publication in this journal is cited, in accordance with accepted academic practice. No use, distribution or reproduction is permitted which does not comply with these terms.
*Correspondence: Fuzhong Xue, eHVlZnpoQHNkdS5lZHUuY24=; orcid.org/0000-0003-0378-7956
Disclaimer: All claims expressed in this article are solely those of the authors and do not necessarily represent those of their affiliated organizations, or those of the publisher, the editors and the reviewers. Any product that may be evaluated in this article or claim that may be made by its manufacturer is not guaranteed or endorsed by the publisher.
Research integrity at Frontiers
Learn more about the work of our research integrity team to safeguard the quality of each article we publish.