- Department of Respiratory Medicine, The Affiliated Xuzhou City Hospital of Xuzhou Medical University, Xuzhou, China
Aberrant expression of microRNAs may affect tumorigenesis and progression by regulating their target genes. This study aimed to construct a risk model for predicting the prognosis of patients with lung adenocarcinoma (LUAD) based on differentially expressed microRNA-regulated target genes. The miRNA sequencing data, RNA sequencing data, and patients’ LUAD clinical data were downloaded from the The Cancer Genome Atlas (TCGA) database. Differentially expressed miRNAs and genes were screened out by combining differential analysis with LASSO regression analysis to further screen out miRNAs associated with patients’ prognosis, and target gene prediction was performed for these miRNAs using a target gene database. Overlapping gene screening was performed for target genes and differentially expressed genes. LASSO regression analysis and survival analysis were then used to identify key genes. Risk score equations for prognostic models were established using multifactorial COX regression analysis to construct survival prognostic models, and the accuracy of the models was evaluated using subject working characteristic curves. The groups were divided into high- and low-risk groups according to the median risk score, and the correlation with the clinicopathological characteristics of the patients was observed. A total of 123 up-regulated miRNAs and 22 down-regulated miRNAs were obtained in this study. Five prognosis-related miRNAs were screened using LASSO regression analysis and Kaplan-Meier method validation, and their target genes were screened with the overlap of differentially expressed genes before multifactorial COX analysis finally resulted in an 11-gene risk model for predicting patient prognosis. The area under the ROC curve proved that the model has high accuracy. The 11-gene risk-prediction model constructed in this study may be an effective predictor of prognosis.
Introduction
Despite advances in lung cancer treatment, in 2020, lung cancer remained the deadliest type of cancer worldwide according to the World Health Organization (WHO) (1). The 5-year survival rate for lung cancer patients is only 19 percent (2). Part of the reason for the short survival rate in lung cancer mainly contributes to the lung cancer-associated pulmonary hypertension caused by blockage of pulmonary blood vessels due to cancer cell proliferation, which eventually leads to death (3, 4). Lung adenocarcinoma (LUAD) belongs to a subtype of non-small cell lung cancer (NSCLC), and NSCLC accounts for another 85 percent of all lung cancers (5). Most patients with LUAD have an advanced or metastatic disease at the time of diagnosis (6); therefore, early diagnosis is crucial, prolonging patient survival and significantly improving survival rates. LUAD is highly heterogeneous at multiple clinical, behavioural, cellular, and molecular levels (7, 8). The cellular and molecular mechanisms regarding the biological behaviour of tumours remain largely unknown.
Aberrant expression of miRNAs in several cancers, including lung cancer, is associated with tumorigenesis and progression (9, 10). With the application of gene sequencing in tumours, miRNAs can be considered as new biomarkers in patient prognosis prediction and drug resistance (11–13). In addition, integration of multiple miRNAs may be more efficient than a single miRNA for prognosis prediction (14, 15). A large number of studies have identified a series of miRNA signatures that can serve as potential biomarkers for LUAD patient prognosis prediction (15, 16). It is well known that miRNAs have important roles in the regulation of gene expression, either through a single miRNA that regulates the expression of multiple genes or through the combination of several miRNAs that finely regulate the expression of a gene, which in turn regulates various physiological processes, cellular functions, and signalling pathways (17, 18). Studies have been conducted to identify key miRNAs and hub genes in LUAD by bioinformatics and functional analysis (19), Li X et al. identified prognostic biomarkers in lung adenocarcinoma based on aberrant lncRNA–miRNA–mRNA networks and Cox regression models. Gu et al. (20),constructed a DElncRNA-DEmiRNA-DEmRNA ceRNA network for deeper understanding the underlying molecular mechanism of lung adenocarcinoma and for evaluating prognosis. But not much research has been reported on the construction of patient prognostic predictive risk models based on differentially expressed miRNA-target gene-differentially expressed gene networks in LUAD.
In this study, we first analysed the expression profiles of miRNAs in the TCGA database for LUAD to obtain differentially expressed miRNAs. Those miRNAs associated with patient prognosis were then screened by LASSO regression analysis and KM validation, and their target genes were combined with differentially expressed genes in LUAD to construct a prognostic risk-prediction model and verify the validity of the risk model. The patients were then divided into two groups according to risk scores – high risk and low risk – and the correlation between different risk groups and clinicopathological characteristics of LUAD patients was observed to assess the prognostic significance of this risk-prediction model in LUAD.
Methods
Data Collection
The miRNA-seq, RNA-seq, and clinical information of lung cancer (LUAD) were obtained by downloading from the TCGA database. If there were multiple probes to detect the same miRNA expression during the analysis, the average of the miRNA expression was taken as an expression value of the miRNA. For the analysis of patient clinical information, clinical information of patients with unknown survival time and survival time of 0 was deleted. The independent validation cohort GSE50081 (21) were obtained from the Gene Expression Omnibus (https://www.ncbi.nlm.nih.gov/geo) by the GEO query R package.
Differential Analysis
Differentially expressed miRNAs in LUAD were screened using the edgeR package in R software with |logFC|≧1, adjust P value < 0.05.Due to the large amount of differentially expressed genes, we changed the cut-off values with |logFC|≧2, adjust P value < 0.05 for the screen of differentially expressed genes. Volcano plots of differentially expressed miRNAs and genes were plotted using ggplot2.
Target Gene Prediction
The miRTarBase (http://miRTarBase.cuhk.edu.cn/), TargetScan (http://www.targetscan.org/vert_72/) and RNA22 (https://cm.jefferson.edu/rna22/) target gene prediction databases were used for target gene prediction of miRNAs.
Kaplan-Meier Survival Analysis
Survival analysis was performed using Survival in the R package. p-values and hazard ratios (HR) with 95% confidence intervals (CI) in Kaplan-Meier curves were derived by log rank test and univariate Cox proportional hazards regression.
LASSO/Cox Regression Analysis
The LASSO regression algorithm was used for feature selection, and a 10-fold cross-validation was used to determine the parameters and obtain a suitable model. The genes obtained from LASSO regression were then subjected to multifactorial Cox regression analysis, and the multifactorial regression coefficients were calculated for each gene to construct the risk score equation.
Gene Ontology Enrichment Analyses
GO (is a recognized bioinformatics tool for annotating genes and the analysis of the biological process of target genes.7 To explore the function of 11 genes, biological analysis was performed using DAVID online database. P<0.05 was considered statistically significant.
Establishment and Analysis of Risk Prognostic Model
Based on the results of the above multi-factor Cox regression analysis, the risk score equation based on gene expression was constructed. Based on the median value of the risk score values, LUAD patients were divided into high-risk score groups and low-risk score groups. Column plots of the model predicting prognosis were drawn using R software, and ROC curves and calibration curves of the model were plotted to evaluate the sensitivity and specificity of the model.
Statistical Analysis
R3.6.3 was used for all statistical analyses. Values of p<0.05 were defined as statistically significant. In the survival analysis, the survival outcome was defined as overall survival based on clinical record. Univariate and multivariate cox regression analyses were used to assess the influences of the genes on patients ‘survival.
Results
Differential Expression miRNA Screening
The edgeR package was applied to analyse differential miRNA expression in LUAD, and the results were demonstrated by the volcano plot (Figure 1A). A total of 123 up-regulated miRNAs and 22 down-regulated miRNAs were obtained. Further screening of these miRNAs using LASSO regression (Figure 1B) and modelling using cross-validation (Figure 1C) was conducted. A total of 23 miRNAs associated with prognosis (hsa-miR-450a-5p, hsa-miR-548v, hsa-miR-490-3p, hsa-miR-142-3p, hsa-miR-20a-5p, hsa-miR-323a-3p, hsa-miR-301b-5p, hsa-miR-940, hsa-miR-550a-5p, hsa-miR-106a-5p, hsa-miR-3653-5p, hsa-let-7c-5p, hsa-miR-31-5p, hsa-miR-137, hsa-miR-192-5p, hsa-miR-642a-5p, hsa-miR-148a-3p, hsa-miR-5698, hsa-miR-196b-5p, hsa-miR-3607-3p, hsa-miR-9-3, hsa-let-7g-3p, hsa-miR-31-3p) were obtained by constituting a multivariate linear model, which was divided into high and low risk according to the prognostic index of each sample, and KM curves showed that high- and low-risk patients survived with significant differences (Figure 1D). To verify the accuracy of this prognostic model, it was further corroborated by the ROC curves, and the results showed that the prognostic model performed well (Figure 1E).
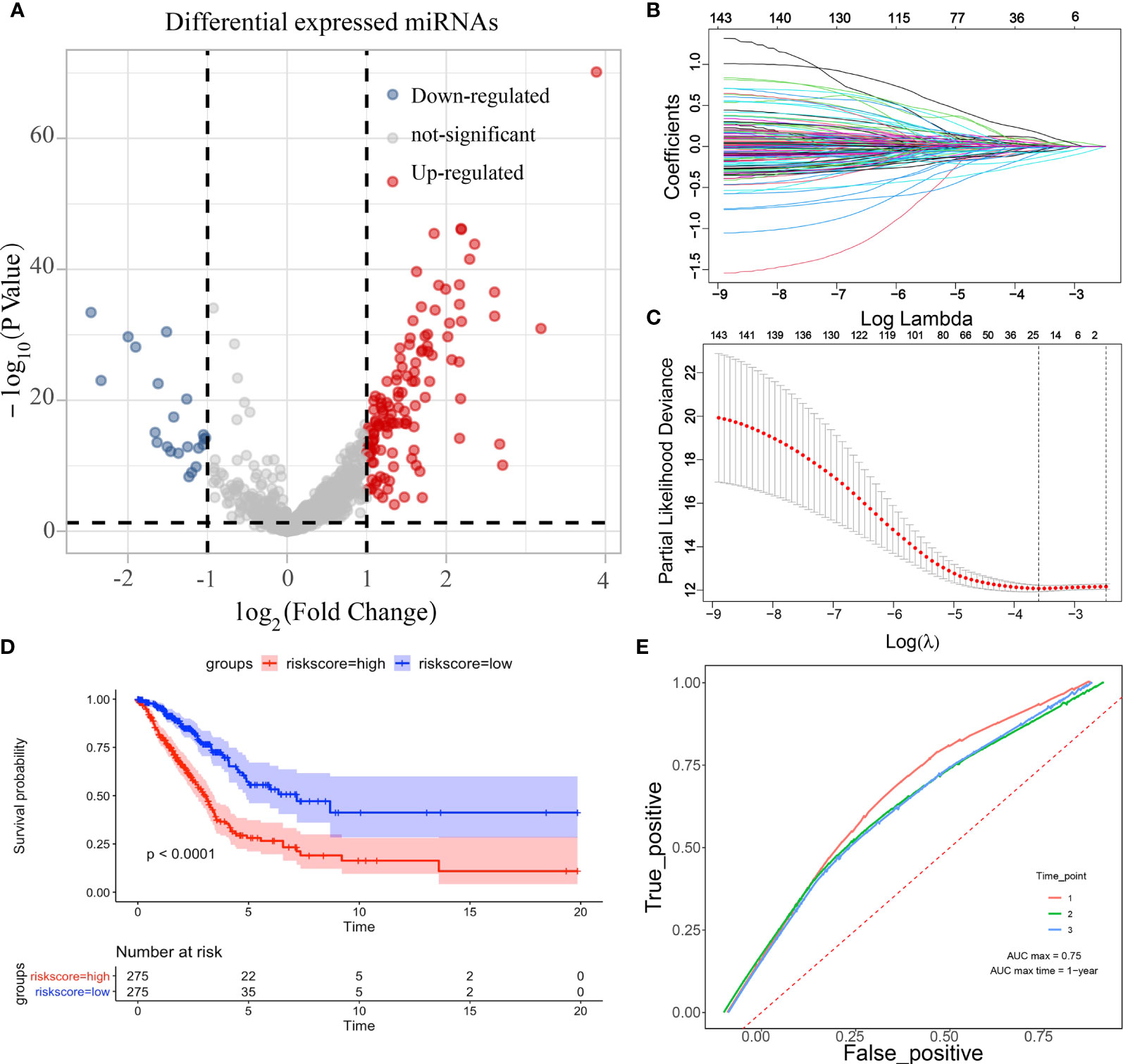
Figure 1 Differential expression miRNA screening. (A: volcano plot showing differentially expressed miRNAs; B: coefficients of selected features shown by lambda parameters; C: partial likelihood deviation versus log(λ); D: survival curves of high-and low-risk samples under LASSO regression; E; ROC curves under LASSO regression).
Kaplan-Meier Method to Validate 23 miRNAs
The relationship between the expression of 23 miRNAs and the survival prognosis of LUAD patients was analysed using the UALCAN database (Figures 2A–V). The results showed that the expression of hsa-miR-490-3p, hsa-miR-940, hsa-miR-31-3p, hsa-miR-31-5p and hsa-let-7c-5p were significantly associated with the survival prognosis of LUAD patients, and high expression of these miRNAs was associated with poor prognosis of LUAD patients.
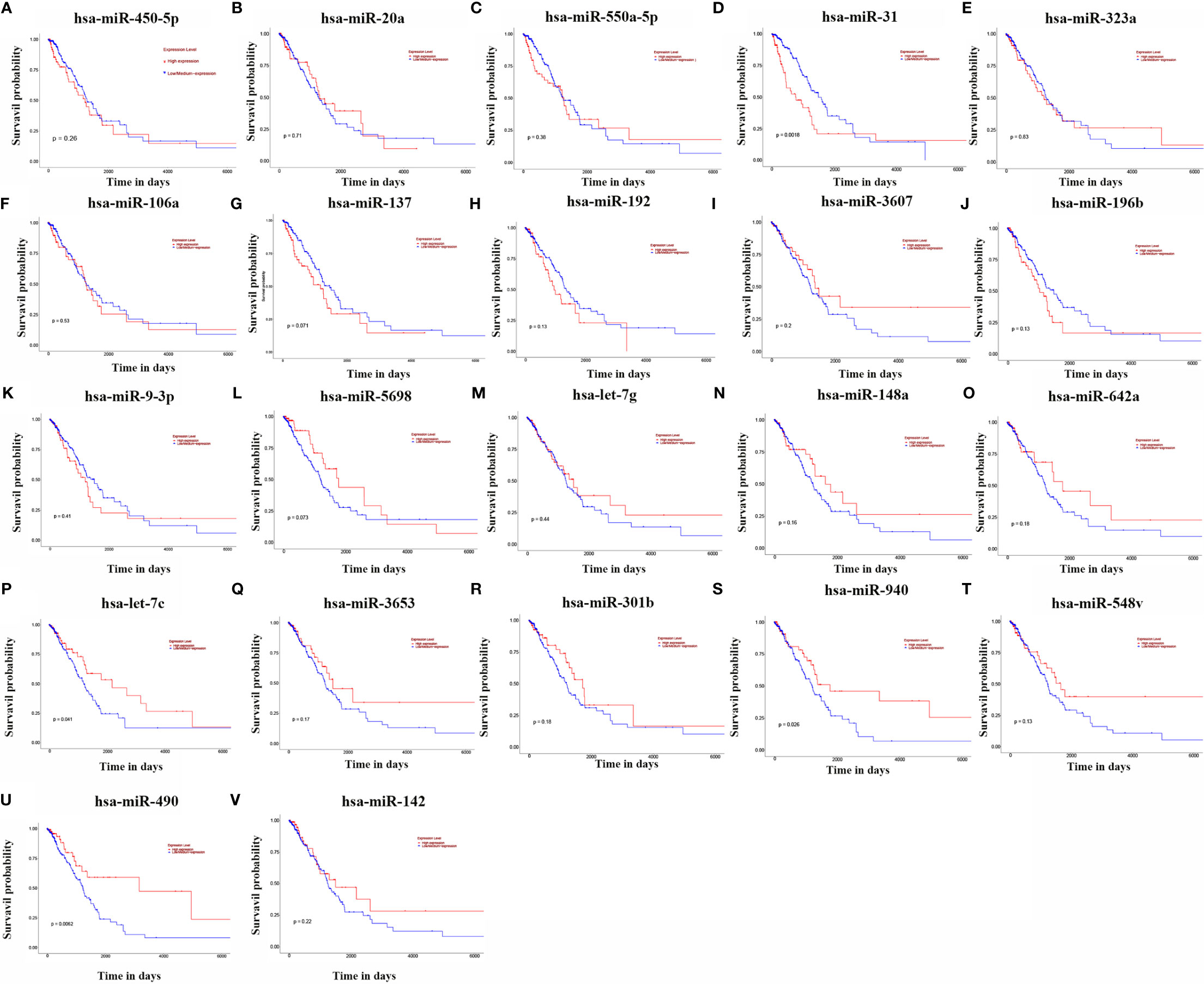
Figure 2 KM curves of 23 miRNAs. (A: hsa-miR-450a-5p; B: hsa-miR-20a; C: hsa-miR-550a-5p; D: hsa-miR-31; E: hsa-miR-323a; F: hsa-miR-106a; G: hsa-miR-137; H: hsa-miR-192; I: hsa- miR-3607; J: hsa-miR-196b; K: hsa-miR-9-3p; L: hsa-miR-5698; M: hsa-let-7g; N: hsa-miR-148a; O: hsa-miR-642a; P: hsa-let-7c; Q: hsa-miR-3653; R: hsa -miR-301b; S: hsa-miR-940; T: hsa-miR-548v; U: hsa-miR-490; V: hsa-miR-142).
Screening of miRNAs Target Genes and Differentially Expressed Genes
The miRTarBase, TargetScan, and RNA22 databases were used to predict the target genes of hsa-miR-490-3p, hsa-miR-940, hsa-miR-31-3p, hsa-miR-31-5p, and hsa-let-7c-5p, and then the target genes of the five miRNAs were screened, and a total of 2002 intersecting target genes was obtained (Figure 3A). Differential analysis of RNA-seq was performed on 513 LUAD tumour samples and 59 normal samples in the TCGA database, and the 256 up-regulated genes and 608 down-regulated genes obtained from the screening were presented using volcano maps (Figure 3B). A total of 84 key genes were obtained by overlapping screening of intersecting target genes and differentially expressed genes (Figure 3C).
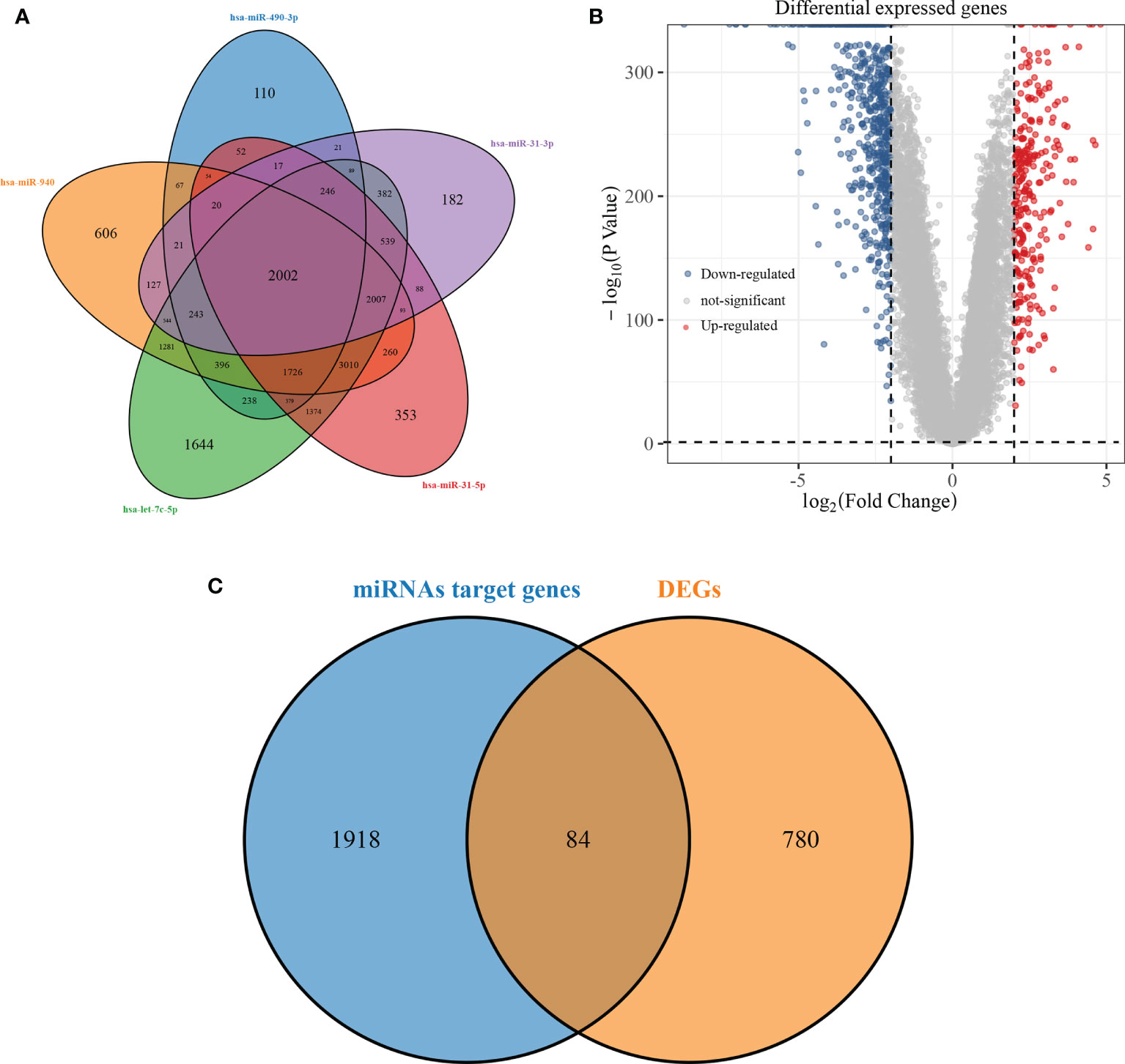
Figure 3 Screening of miRNAs target genes and differentially expressed genes. (A: overlapping Venn diagram of predicted target genes for 5 miRNAs; B: volcano diagram of differentially expressed genes in LUAD; C: overlapping Venn diagram of predicted target genes and differentially expressed genes for miRNAs).
LASSO Regression Analysis
A 24-gene prediction model was obtained by screening 84 genes using LASSO regression analysis (Figures 4A, B), and LUAD patients were divided into a high-risk score group and a low-risk score group based on the median value of the risk score (Figure 4C). The LASSO regression survival curves (Figure 4D) showed that high-risk patients were worse than low-risk patients. The subjects’ working curves (Figure 4E) showed that the AUC areas of the 1-, 3-, and 5-year survival time curves were 0.752, 0.736, and 0.743, respectively, indicating that the model has a strong predictive accuracy.
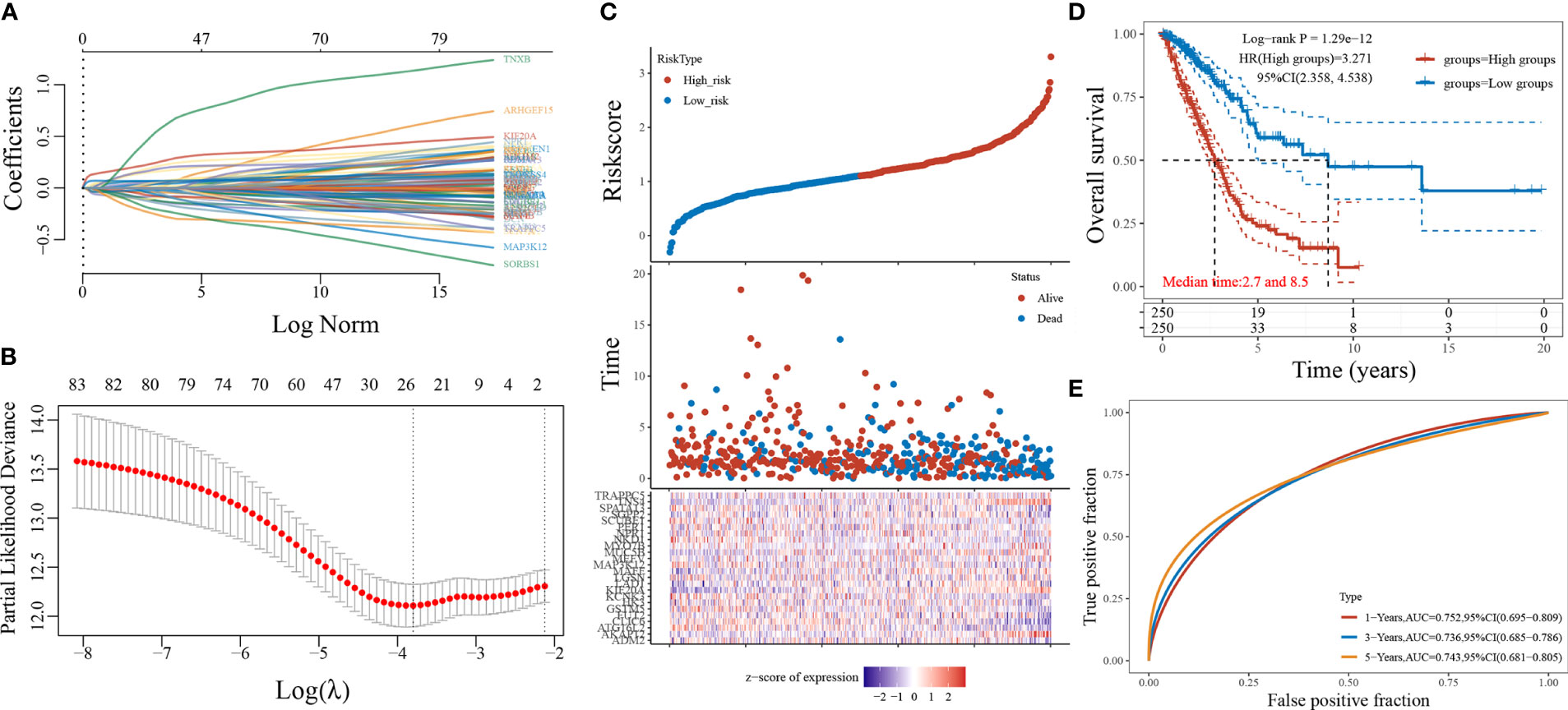
Figure 4 LASSO regression model screening 84 genes. (A: different characteristics of genes and their corresponding coefficients; B: cross-validation to build the model; C: risk score with survival time and survival status cases; D: KM curve of this prediction model; E: ROC curve plotted under the prediction model).
Kaplan-Meier Method to Validate 24 Genes
The Kaplan-Meier method was used to validate the overall survival time of 24 key genes, and the hazard coefficients HR of the 95% CI of the 24 genes were obtained by log rank test and univariate Cox proportional hazards regression. Results are presented in Table 1, which shows that a total of 11 genes were significantly associated with overall survival in LUAD. Their KM curves are shown in Figures 5A–K. The expression of these 11 genes in LUAD is shown in Figure 5L. Compared with normal tissues, the expression of ADM2, CLIC6, KIF20A, LAD1, MUC5B, and TNS4 was up-regulated, and the expression of ATG16L2, KCNK3, MAFF, NKD1, and SPATA13 was down-regulated in lung cancer tissues.
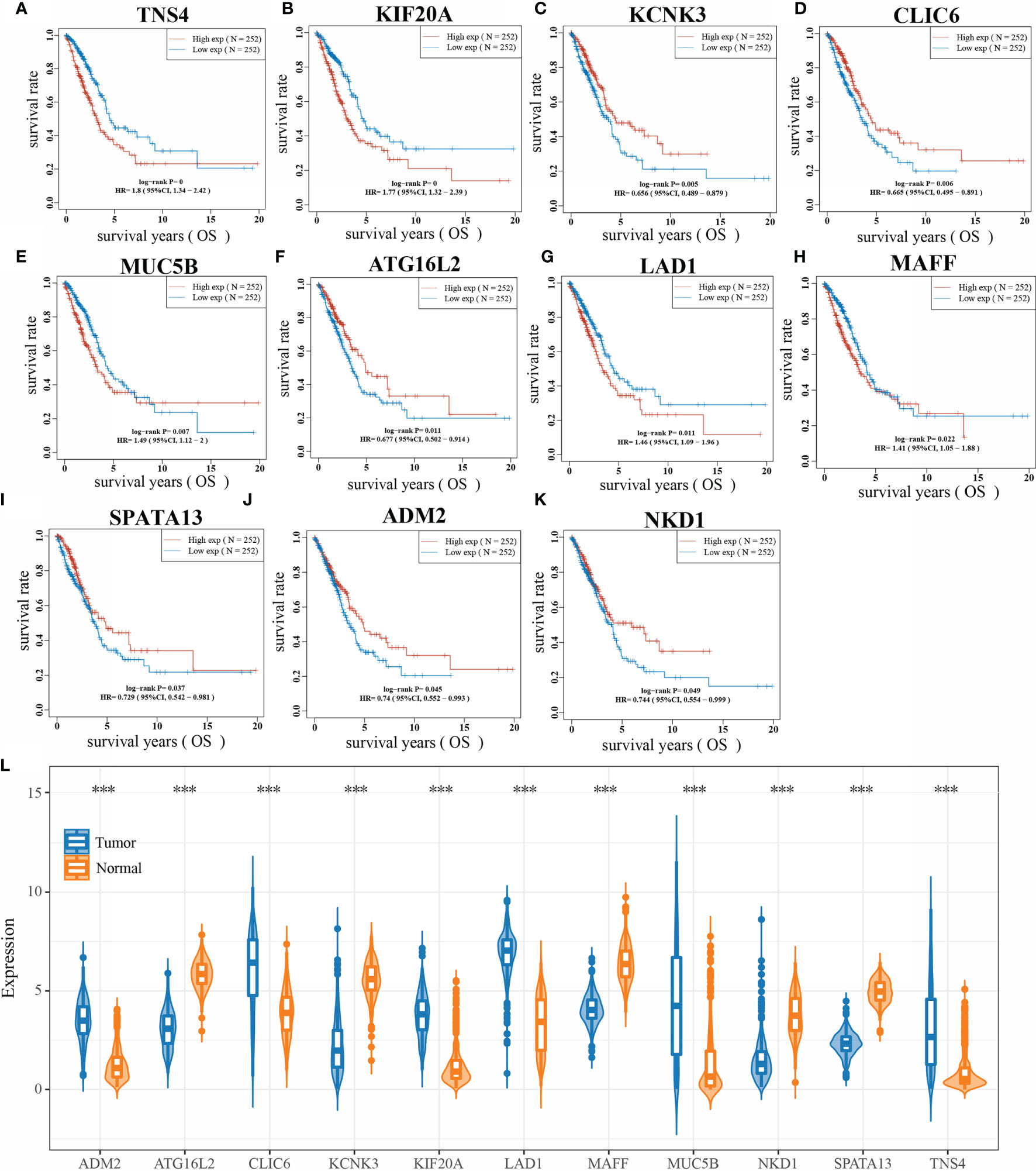
Figure 5 Kaplan-Meier method to validate 23 genes. (A: KM curve of TNS4; B: KM curve of KIF20A; C: KM curve of KCNK3; D: KM curve of CLIC6; E: KM curve of MUC5B; F: KM curve of ATG16L2; G: KM curve of LAD1; H: KM curve of MAFF; I: KM curve of SPATA13; J: KM curve of ADM2 curve; K: KM curve of NKD1; L: expression of 11 genes in LUAD; ***P < 0.0001).
Construction of a Risk-Prediction Model Based on 11 Genes
A prediction model based on LASSO regression analysis was constructed for the 11-gene signature (Figures 6A, B), and its predicted risk score consisted mainly of the following:
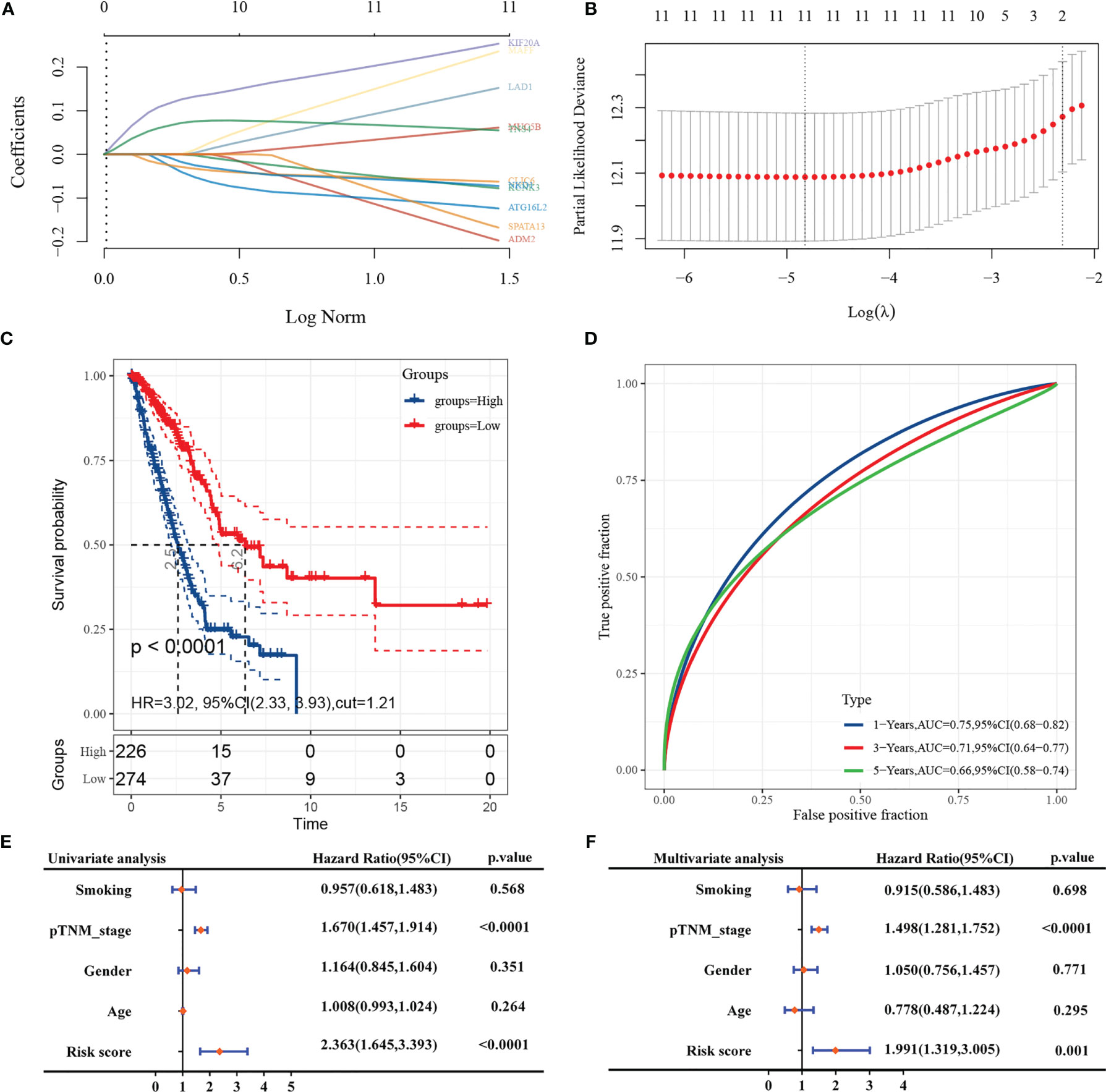
Figure 6 LASSO construction prediction model and COX analysis. (A: coefficients of selected characteristics shown by lambda parameters; B: partial likelihood deviation versus log(λ); C: KM curves for risk grouping; D: ROC curves for this risk prediction model; E: forest plot for univariate COX regression; F: forest plot for multifactor COX regression).
The sample was divided into high-risk and low-risk groups according to the risk score ranking, using the best cut-off risk score as the threshold, and the Kaplan-Meier analysis showed that the prognosis of patients in the high-risk group was significantly worse than that of low-risk patients (Figure 6C). The subject working characteristic curves indicated that the prediction model had high predictive accuracy for patients’ 1- and 3-year survival prognosis (1-Year AUC: 0.749, 3 Years AUC: 0.708) (Figure 6D).
Further GO analysis of the 11 genes showed that the gene signatures were mainly associated with voltage-gated channel activity、S100 protein binding、potassium ion leak channel activity (Table 2).
In univariate Cox regression analysis, pathological TNM stage and risk score was associated with poorer prognosis of patients (Figure 6E). In multivariate Cox regression, pathological TNM stage and risk score were identified as independent prognostic predictors (Figure 6F).
Validating the Predictive Value of Risk Model in Independent Cohort
To further verify the predictive value of risk model, GSE50081 from GEO were employed as a validation cohort. Cox regression analysis and the Kaplan-Meier curve showed that high-risk patients were worse than low-risk patients, which agreed with the results found in the TCGA-LUAD cohort (Figure 7A). The AUCs of FRRS at 1, 3 and 5 years were 0.61, 0.55, and 0.54, respectively (Figure 7B).
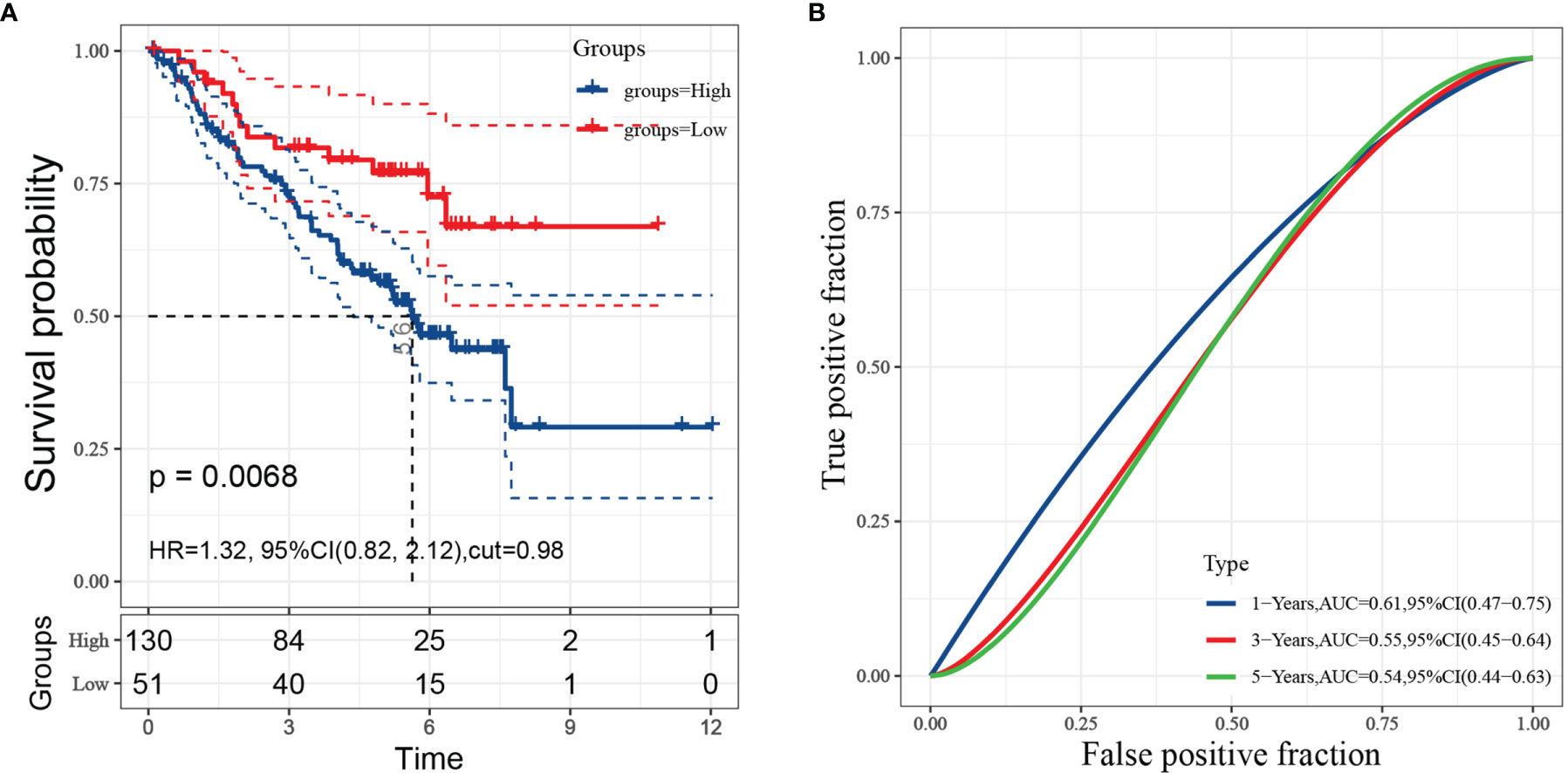
Figure 7 Evaluation of risk model performance in independent datasets. (A: Kaplan-Meier curves and univariate Cox regression of overall survival in GSE50081; B: ROC curve analyses in GSE50081).
Clinicopathological Characteristics of Different Risk Score Subgroups
The distribution of clinicopathological characteristics of different risk score subgroups (high risk and low risk) was presented as a heat map (Figure 8A); the relationship between different age, gender, smoking history, race, pathological TNM stage, pathological T stage, pathological N stage, pathological M stage, and risk score was observed, and the results showed (Figures 8B–I) that Stage I patients’ risk score was significantly different from that of Stage II and Stage III patients; the difference between risk score of Stage T1 and T2 and T3 patients was statistically significant. The risk score of Stage N0 patients was significantly different from that of Stage N1 and N2 patients.
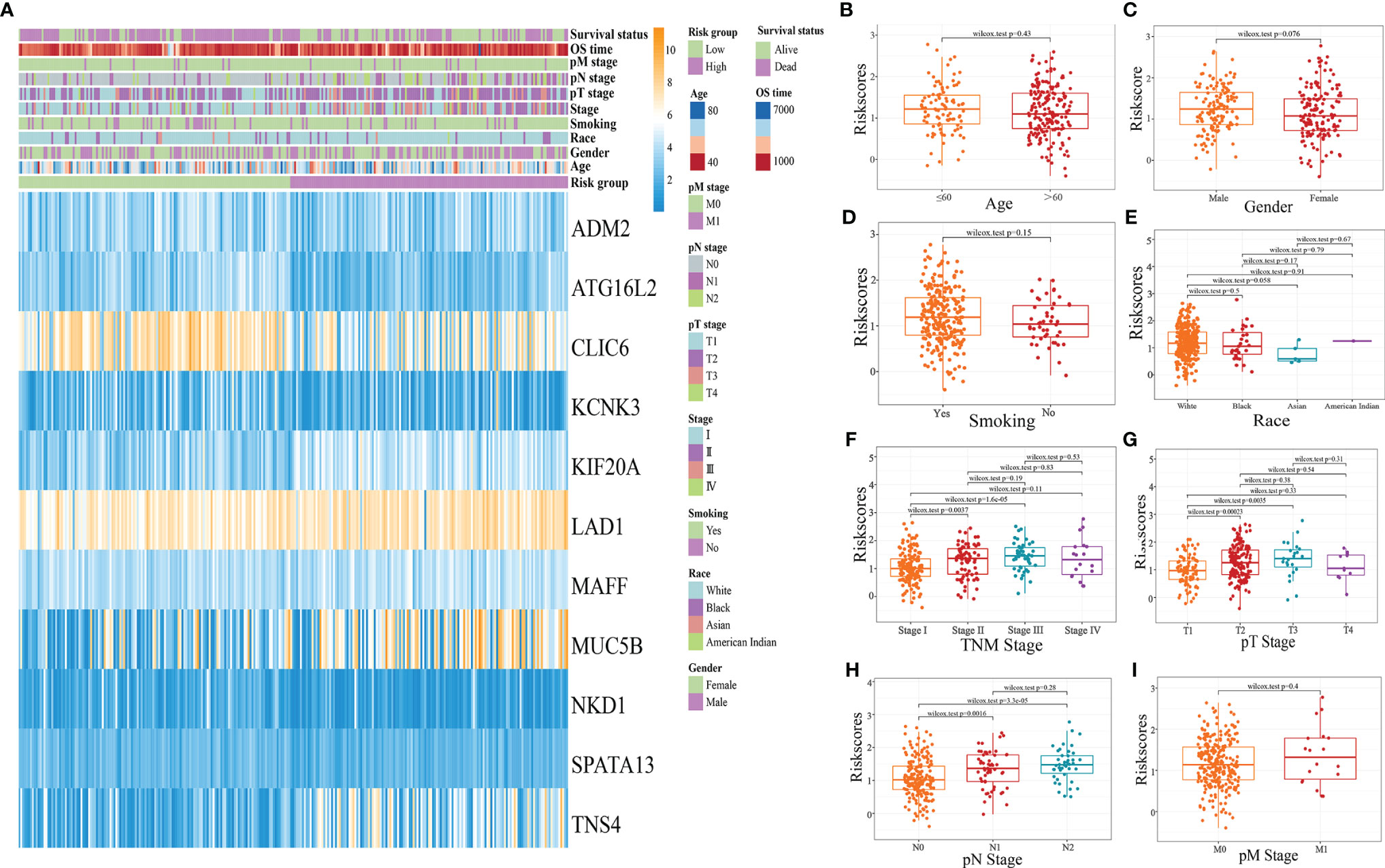
Figure 8 Clinicopathological characteristics of different risk score subgroups. (A: heat map of clinicopathological characteristics of different risk score subgroups; B: the relationship between age and risk score; C: the relationship between sex and risk score; D: the relationship between smoking history and Riskscore; E: the relationship between race and Riskscore; F: the relationship between pathological TNM stage and Riskscore; G: the relationship between pathological T stage and Riskscore; H;. Relationship between pathological N-stage and Riskscore; I: Relationship between pathological M-stage and Riskscore).
Discussion
miRNAs are not independent regulators, and their action is achieved through binding to target genes. Although the vast majority of genes in the human genome are regulated by the expression of miRNAs, not all of them play a decisive role in tumour development. Therefore, although certain miRNAs are differentially expressed in tumours, it does not mean that this has an impact on tumorigenesis and progression. In this study, we first obtained a total of 123 up-regulated miRNAs and 22 down-regulated miRNAs, 256 up-regulated genes and 608 down-regulated genes by screening differentially expressed miRNAs and genes in LUAD. We then further subjected these miRNAs to LASSO regression analysis and validated them with KM to obtain a total of five prognosis-related LUAD patients. These five miRNAs have been shown to be differentially expressed in LUAD by bioinformatics analysis in previous studies (22). Subsequently, we predicted the target genes of these five miRNAs and found that there were 2002 target genes in which the five miRNAs acted together, and a total of 84 genes were obtained by screening them with overlapping differentially expressed genes in LUAD. The vast majority of studies using training groups to develop and construct molecular markers rely on the selection of overlapping genes in multiple databases, which may lead to the recurrence of certain genes in new signatures, a phenomenon that may lead to similarity or convergence of results and may hinder the efficiency and predictive power of prediction models (23). To improve our accuracy, we performed downscaling and validation of 84 genes to finally construct a risk-prediction model with 11 gene signatures consisting of 11 genes significantly associated with LUAD prognosis. This 11-gene signature risk-prediction model classified patients into high-risk and low-risk groups, and there was a significant difference in the overall survival prognosis between the high- and low-risk groups. This model could be a valid and promising prognostic biomarker for lung adenocarcinoma patients as an independent prognostic predictor. Previously studies also constructed a series of models to predicting prognosis in LUAD via different bioinformatic analysis. Zhou C et al. (24) constructed prognosis predicting risk model based on platelet-related gene expression. Besides this, Ye GC et al. (25) identified key microRNAs and hub genes associated with poor prognosis in lung adenocarcinoma via miRNA-mRNA network, they indicated that PECAM1, in particular, may be a novel biomarker of survival that provided a novel diagnostic biomarkers and therapeutic targets for the treatment of LUAD. Except for miRNA or mRNA, lncRNA also plays an important role in the pathogenesis of cancer and has significant clinical value in prognosis and diagnosis (26). Li R et al. (27) applied an integrated ceRNA network analysis to identify a lncRNA-based signature for predicting the prognosis of LUAD patients. The established molecular signature with seven lncRNAs, derived from the ceRNA network, was demonstrated to be a robust and independent factor for the survival prediction of LUAD patients.
The 11 gene signatures are composed of ADM2, CLIC6, KIF20A, LAD1, MUC5B, TNS4, ATG16L2, KCNK3, MAFF, NKD1, and SPATA13. adrenomedullin-2 (ADM2) is a hypoxia-inducible endothelial peptide that stabilizes pulmonary microvascular.CLIC6 is a member of the intracellular chloride channel, one of the dopamine receptor-mediated signalling pathways, and is differentially expressed in breast cancer. Recent studies have reported evidence that CLIC6 expression is significantly associated with lung adenocarcinoma prognosis (28, 29). KIF20A (30), LAD1 (31), MUC5B (32), TNS4 (33), ATG16L2 (34), and NKD1 (35) have been shown to serve as biological markers for prognosis prediction in lung cancer. KCNK3 (36) has been reported to be involved in pulmonary hypertension, which may contribute to poor prognosis in lung cancer patients. SPATA13 is a discrete region in the adult brain enriched in a guanylate exchange factor (37), and deletion of SPATA13 has been shown to reduce and shrink the number and size of intestinal tumours in Apc (Min/+) mice (38, 39), but its study in lung cancer is rare. In addition to this, we investigated the risk scores in different LUAD clinicopathological features. The results suggest that the risk score of Stage I in patients with different TNM stages is significantly different from that of Stage II, III, and IV, and the same situation applies to different T and N stages. This result also demonstrates that this prediction model may serve as a biological marker for the early diagnosis of LUAD.
In conclusion, a valid and accurate prognostic model for LUAD based on 11 gene signatures was constructed in this study. The prediction model based on these 11 gene signatures has good predictive properties, and it can effectively distinguish between high-risk and low-risk patients based on risk scores. There are significant differences in its risk scores among patients with different TNM stages, making it an early diagnostic marker and prognostic predictor for LUAD patients and reducing the excessive cost of molecular diagnosis. However, there are some limitations to this study. First, since our study relied mainly on bioinformatics analysis, these results subsequently require a series of biological experiments to assist validation. The potential biological mechanisms and pathways associated with these 11 genes still need further investigation.
Data Availability Statement
The original contributions presented in the study are included in the article/supplementary material. Further inquiries can be directed to the corresponding author.
Author Contributions
All authors listed have made a substantial, direct, and intellectual contribution to the work and approved it for publication.
Conflict of Interest
The authors declare that the research was conducted in the absence of any commercial or financial relationships that could be construed as a potential conflict of interest.
Publisher’s Note
All claims expressed in this article are solely those of the authors and do not necessarily represent those of their affiliated organizations, or those of the publisher, the editors and the reviewers. Any product that may be evaluated in this article, or claim that may be made by its manufacturer, is not guaranteed or endorsed by the publisher.
References
1. Sung H, Ferlay J, Siegel RL, Laversanne M, Soerjomataram I, Jemal A, et al. Global Cancer Statistics 2020: GLOBOCAN Estimates of Incidence and Mortality Worldwide for 36 Cancers in 185 Countries. CA Cancer J Clin (2021) 71(3):209–49. doi: 10.3322/caac.21660
2. Siegel RL, Miller KD, Jemal A. Cancer Statistics, 2019. CA Cancer J Clin (2019) 69(1):7–34. doi: 10.3322/caac.21551
3. Nicoleau S, Wojciak-Stothard B. Beyond Thrombosis: The Role of Platelets in Pulmonary Hypertension. SciMed J (2020) 2(4):243–71. doi: 10.28991/SciMedJ-2020-0204-7
4. Pullamsetti SS, Kojonazarov B, Storn S, Gall H, Salazar Y, Wolf J, et al. Lung Cancer-Associated Pulmonary Hypertension: Role of Microenvironmental Inflammation Based on Tumor Cell-Immune Cell Cross-Talk. Sci Transl Med (2017) 9(416):eaai9048. doi: 10.1126/scitranslmed.aai9048
5. Herbst RS, Morgensztern D, Boshoff C. The Biology and Management of Non-Small Cell Lung Cancer. Nature (2018) 553(7689):446–54. doi: 10.1038/nature25183
6. Hao P, You K, Feng H, Xu X, Zhang F, Wu F, et al. Lung Adenocarcinoma Diagnosis in One Stage. Neurocomputing (2020) 392:245–52. doi: 10.1016/j.neucom.2018.11.110
7. Senosain MF, Massion PP. Intratumor Heterogeneity in Early Lung Adenocarcinoma. Front Oncol (2020) 10:349. doi: 10.3389/fonc.2020.00349
8. Hua X, Zhao W, Pesatori AC, Consonni D, Caporaso NE, Zhang T, et al. Genetic and Epigenetic Intratumor Heterogeneity Impacts Prognosis of Lung Adenocarcinoma. Nat Commun (2020) 11(1):2459. doi: 10.1038/s41467-020-16295-5
9. Wu SG, Chang TH, Liu YN, Shih JY. MicroRNA in Lung Cancer Metastasis. Cancers (Basel) (2019) 11(2):265. doi: 10.3390/cancers11020265.
10. Wu KL, Tsai YM, Lien CT, Kuo PL, Hung AJ. The Roles of MicroRNA in Lung Cancer. Int J Mol Sci (2019) 20(7):1611. doi: 10.3390/ijms20071611.
11. Papadaki C, Stoupis G, Tsalikis L, Monastirioti A, Papadaki M, Maliotis N, et al. Circulating miRNAs as a Marker of Metastatic Disease and Prognostic Factor in Metastatic Breast Cancer. Oncotarget (2019) 10(9):966–81. doi: 10.18632/oncotarget.26629
12. Moya L, Meijer J, Schubert S, Matin F, Batra J. Assessment of miR-98-5p, miR-152-3p, miR-326 and miR-4289 Expression as Biomarker for Prostate Cancer Diagnosis. Int J Mol Sci (2019) 20(5):1154. doi: 10.3390/ijms20051154
13. Zhang Z, Dong Y, Hua J, Xue H, Hu J, Jiang T, et al. A five-miRNA Signature Predicts Survival in Gastric Cancer Using Bioinformatics Analysis. Gene (2019) 699:125–34. doi: 10.1016/j.gene.2019.02.058
14. Bian Z, Huang X, Chen Y, Meng J, Feng X, Zhang M, et al. Fifteen-MiRNA-Based Signature Is a Reliable Prognosis-Predicting Tool for Prostate Cancer Patients. Int J Med Sci (2021) 18(1):284–94. doi: 10.7150/ijms.49412
15. Yang Z, Yin H, Shi L, Qian X. A Novel microRNA Signature for Pathological Grading in Lung Adenocarcinoma Based on TCGA and GEO Data. Int J Mol Med (2020) 45(5):1397–408. doi: 10.3892/ijmm.2020.4526
16. Peng Z, Pan L, Niu Z, Li W, Dang X, Wan L, et al. Identification of microRNAs as Potential Biomarkers for Lung Adenocarcinoma Using Integrating Genomics Analysis. Oncotarget (2017) 8(38):64143–56. doi: 10.18632/oncotarget.19358
17. Nagy A, Lanczky A, Menyhart O, Gyorffy B. Author Correction: Validation of miRNA Prognostic Power in Hepatocellular Carcinoma Using Expression Data of Independent Datasets. Sci Rep (2018) 8(1):11515. doi: 10.1038/s41598-018-29514-3
18. Gurtan AM, Sharp PA. The Role of miRNAs in Regulating Gene Expression Networks. J Mol Biol (2013) 425(19):3582–600. doi: 10.1016/j.jmb.2013.03.007
19. Yu DH, Ruan X-L, Huang J-Y, Liu X-P, Ma H-L, Chen C, et al. Analysis of the Interaction Network of Hub miRNAs-Hub Genes, Being Involved in Idiopathic Pulmonary Fibers and Its Emerging Role in Non-Small Cell Lung Cancer. Front Genet (2020) 11:302. doi: 10.3389/fgene.2020.00302
20. Gu C, Shi X, Huang Z, Chen J, Yang J, Shi J, et al. A Comprehensive Study of Construction and Analysis of Competitive Endogenous RNA Networks in Lung Adenocarcinoma. Biochim Biophys Acta (BBA)-Proteins Proteom (2020) 1868(8):140444. doi: 10.1016/j.bbapap.2020.140444
21. Der SD, Sykes J, Pintilie M, Zhu C-Q, Strumpf D, Liu N, et al. Validation of a Histology-Independent Prognostic Gene Signature for Early-Stage, Non–Small-Cell Lung Cancer Including Stage IA Patients. J Thorac Oncol (2014) 9(1):59–64. doi: 10.1097/JTO.0000000000000042
22. Yu H, Pang Z, Li G, Gu T. Bioinformatics Analysis of Differentially Expressed miRNAs in Non-Small Cell Lung Cancer. J Clin Lab Anal (2021) 35(2):e23588. doi: 10.1002/jcla.23588
23. Al-Dherasi A, Huang QT, Liao Y, Al-Mosaib S, Hua R, Wang Y, et al. A Seven-Gene Prognostic Signature Predicts Overall Survival of Patients With Lung Adenocarcinoma (LUAD). Cancer Cell Int (2021) 21(1):294.doi: 10.1186/s12935-021-01975-z
24. Zhou C, Wang Y, Wang Y, Lei L, Ji M-H, Zhou G, et al. Predicting Lung Adenocarcinoma Prognosis With a Novel Risk Scoring Based on Platelet-Related Gene Expression. Aging (Albany NY) (2021) 13(6):8706.doi: 10.18632/aging.202682
25. Ye G-C, Liu Y-F, Huang L, Zhang C-Y, Sheng Y-L, Wu B, et al. Key microRNAs and Hub Genes Associated With Poor Prognosis in Lung Adenocarcinoma. Aging (Albany NY) (2021) 13(3):3742. doi: 10.18632/aging.202337
26. Tripathi MK, Doxtater K, Keramatnia F, Zacheaus C, Yallapu MM, Jaggi M, et al. Role of lncRNAs in Ovarian Cancer: Defining New Biomarkers for Therapeutic Purposes. Drug Discov Today (2018) 23(9):1635–43. doi: 10.1016/j.drudis.2018.04.010
27. Li R, Han K, Xu D, Chen X, Lan S, Liao Y, et al. A Seven-Long Non-Coding RNA Signature Improves Prognosis Prediction of Lung Adenocarcinoma: An Integrated Competing Endogenous RNA Network Analysis. Front Genet (2021) 11:1813. doi: 10.3389/fgene.2020.625977
28. Pfeil U, Aslam M, Paddenberg R, Quanz K, Chang CL, Park JI, et al. Intermedin/adrenomedullin-2 Is a Hypoxia-Induced Endothelial Peptide That Stabilizes Pulmonary Microvascular Permeability. Am J Physiol Lung Cell Mol Physiol (2009) 297(5):L837–45. doi: 10.1152/ajplung.90608.2008
29. Zheng C, Quan R, Xia EJ, Bhandari A, Zhang X. Original Tumour Suppressor Gene Polycystic Kidney and Hepatic Disease 1-Like 1 Is Associated With Thyroid Cancer Cell Progression. Oncol Lett (2019) 18(3):3227–35. doi: 10.3892/ol.2019.10632
30. Xie F, He C, Gao S, Yang Z, Li L, Qiao L, et al. KIF20A Silence Inhibits the Migration, Invasion and Proliferation of Non-Small Cell Lung Cancer and Regulates the JNK Pathway. Clin Exp Pharmacol Physiol (2020) 47(1):135–42. doi: 10.1111/1440-1681.13183
31. Nagashio R, Ueda J, Ryuge S, Nakashima H, Jiang SX, Kobayashi M, et al. Diagnostic and Prognostic Significances of MUC5B and TTF-1 Expressions in Resected Non-Small Cell Lung Cancer. Sci Rep (2015) 5:8649. doi: 10.1038/srep08649
32. Wang X, Shi D, Zhao D, Hu D. Aberrant Methylation and Differential Expression of SLC2A1, TNS4, GAPDH, ATP8A2, and CASZ1 Are Associated With the Prognosis of Lung Adenocarcinoma. BioMed Res Int (2020) 2020:1807089. doi: 10.1155/2020/1807089
33. Bai H, He Y, Lin Y, Leng Q, Carrillo JA, Liu J, et al. Identification of a Novel Differentially Methylated Region Adjacent to ATG16L2 in Lung Cancer Cells Using Methyl-CpG Binding Domain Protein-Enriched Genome Sequencing. Genome (2021) 64(5):533–46. doi: 10.1139/gen-2020-0071
34. Zhang S, Wang Y, Dai SD, Wang EH. Down-Regulation of NKD1 Increases the Invasive Potential of Non-Small-Cell Lung Cancer and Correlates With a Poor Prognosis. BMC Cancer (2011) 11:186. doi: 10.1186/1471-2407-11-186
35. Wang Y. Circ-ANXA7 Facilitates Lung Adenocarcinoma Progression via miR-331/LAD1 Axis. Cancer Cell Int (2021) 21(1):85. doi: 10.1186/s12935-021-01791-5
36. Antigny F, Hautefort A, Meloche J, Belacel-Ouari M, Manoury B, Rucker-Martin C, et al. Potassium Channel Subfamily K Member 3 (KCNK3) Contributes to the Development of Pulmonary Arterial Hypertension. Circulation (2016) 133(14):1371–85. doi: 10.1161/CIRCULATIONAHA.115.020951
37. Kawasaki Y, Sagara M, Shibata Y, Shirouzu M, Yokoyama S, Akiyama T. Identification and Characterization of Asef2, A Guanine-Nucleotide Exchange Factor Specific for Rac1 and Cdc42. Oncogene (2007) 26(55):7620–267. doi: 10.1038/sj.onc.1210574
38. Bourbia N, Chandler P, Codner G, Banks G, Nolan PM. The Guanine Nucleotide Exchange Factor, Spata13, Influences Social Behaviour and Nocturnal Activity. Mamm Genome (2019) 30(3-4):54–62. doi: 10.1007/s00335-019-09800-9
Keywords: lung adenocarcinoma, miRNA, prognosis, LASSO, risk score
Citation: Zhou N, Zhou M, Ding N, Li Q and Ren G (2021) An 11-Gene Signature Risk-Prediction Model Based on Prognosis-Related miRNAs and Their Target Genes in Lung Adenocarcinoma. Front. Oncol. 11:726742. doi: 10.3389/fonc.2021.726742
Received: 17 June 2021; Accepted: 11 October 2021;
Published: 05 November 2021.
Edited by:
Ye Wang, The Second Affiliated Hospital of Medical College of Qingdao University, ChinaCopyright © 2021 Zhou, Zhou, Ding, Li and Ren. This is an open-access article distributed under the terms of the Creative Commons Attribution License (CC BY). The use, distribution or reproduction in other forums is permitted, provided the original author(s) and the copyright owner(s) are credited and that the original publication in this journal is cited, in accordance with accepted academic practice. No use, distribution or reproduction is permitted which does not comply with these terms.
*Correspondence: Min Zhou, eGllY2h1bjA4MDNAMTYzLmNvbQ==