- 1Nuffield Department of Surgical Sciences John Radcliffe Hospital, University of Oxford, Oxford, United Kingdom
- 2Patrick G Johnston Centre for Cancer Research, Queen’s University of Belfast, Belfast, United Kingdom
- 3Centre for Cancer Biomarkers, University of Bergen, Bergen, Norway
- 4Department of Clinical Science, University of Bergen, Bergen, Norway
Prostate cancer is a high-incidence cancer, often detected late in life. The prostate gland is an accessory gland that secretes citrate; an impaired citrate secretion reflects imbalances in the activity of enzymes in the TCA Cycle in mitochondria. Profiling studies on prostate tumours have identified significant metabolite, proteomic, and transcriptional modulations with an increased mitochondrial metabolic activity associated with localised prostate cancer. Here, we focus on the androgen receptor, c-Myc, phosphatase and tensin Homolog deleted on chromosome 10 (PTEN), and p53 as amongst the best-characterised genomic drivers of prostate cancer implicated in metabolic dysregulation and prostate cancer progression. We outline their impact on metabolic function before discussing how this may affect metabolite pools and in turn chromatin structure and the epigenome. We reflect on some recent literature indicating that mitochondrial mutations and OGlcNAcylation may also contribute to this crosstalk. Finally, we discuss the technological challenges of assessing crosstalk given the significant differences in the spatial sensitivity and throughput of genomic and metabolomic profiling approaches.
Introduction
Prostate cancer (PCa) is the most common cancer affecting men in the developed world. The incidence is the second highest after lung cancer in men worldwide (1, 2).
Deciphering the functional impact of prostate cancer genomics on disease progression has been a challenge in comparison to other cancer types for many reasons including sample accessibility and the limited availability of model systems. Multi-focal sampling of prostate cancer in patient samples with downstream DNA and RNA sequencing have revealed both inter- and intra-patient heterogeneity in primary tumours and metastatic samples (3, 4). Despite this heterogeneity, it has been possible to sub-type prostate cancers based not only on gene fusion status but also on the abundance of mutations associated with biological drivers of the disease, and in particular mutations affecting androgen receptor (AR) signalling), PI 3-Kinase/Akt, and DNA repair pathways (3).
Genomic Features of Prostate Cancer
In considering the crosstalk between genetic changes in prostate cancer and metabolic dysregulation, it is helpful to focus on some of the principal oncogenic drivers—AR activity, c-Myc amplification and overexpression and mutations in phosphatase and tensin homolog deleted on chromosome 10 (PTEN) and in TP53.
AR
Over the decades, a focus on targeting the androgen receptor (AR) signalling axis to block AR via androgen deprivation therapy and AR antagonists has been a conventional therapy in PCa. Aberration in AR ranges from point mutations such as W741R, V757A, R846G, H874Y, and T877A in ligand binding region of the AR imparting insensitivity towards AR antagonists (1). In addition, deletions in the region of G589-A628 has been identified in patients with CRPC, disrupting second zinc-finger domain of AR, developing resistance to AR antagonists or AR targeted therapies (1). By using ChIP-seq and transcriptomic profiling networks of AR, target genes have been identified in cell lines and in tumour samples (2). These datasets have provided insight into AR crosstalks with other transcription factors and regulated biological processes. Recent transcriptomic and cistromic studies have revealed AR as a modulator of autophagy and DNA repair (3–7). Massie et al. employed a combination of transcript profiling and ChIP-seq to identify androgen receptor target genes and pathways in prostate cancer cell lines (8). Through a meta-analysis of clinical transcriptomic data and subsequent validation using immunohistochemistry, the authors identified calcium/calmodulin-dependent kinase kinase 2 (CAMKK2) as a clinically relevant regulator of metabolism. They went on to knockdown CAMKK2 and also inhibit this kinase with a small molecule inhibitor, which impaired tumorigenesis in a prostate cancer xenograft model. Pairing these interventions with 13C-glucose metabolic flux analysis using mass spectroscopy, they showed that targeting CAMKK2 inhibited the incorporation of hydrocarbons into TCA cycle metabolites and amino acids. This work illustrates the use of genomics and metabolomics to identify metabolic regulators that are affected by AR activity. Other studies have shown that AR-associated gene targets include key components/enzymes of glucose homeostasis, mitochondrial respiration, and fatty acid oxidation (9–14).
Pathway enrichment analysis on AR-regulated gene networks has unearthed enrichments for metabolic processes amongst which the most prominent are lipid synthesis and degradation pathways (15). Importantly, the vast majority of AR-regulated metabolic enzymes are cytosolic or associated with organelles other than mitochondria; however, high rates of metabolic activity arising from AR-regulated pathways feed metabolites into mitochondria. Important examples of lipid-metabolising enzymes that are AR-dependent include FASN, ELOVL5, and ACACA (acetyl-CoA carboxylase alpha) (16). The precise functional effects of aberrant lipid metabolism remain to be determined but include changes in membrane fluidity and the generation of acetyl CoA to support the post-translational modification of proteins (acetylation and glycosylation), prominent amongst which are histones that are of relevance to the crosstalk between metabolism and the epigenome (see below) (17). In addition, a number of other important oncogenic drivers of prostate cancer also sustain aberrant lipid metabolism (see below); as such, this biology is arguably a convergence point for prostate cancer tumorigenesis and, consequently, may offer opportunities for the development of new treatments and repurposing of existing drugs (8, 18).
c-Myc
Myc is copy number amplified and overexpressed in poor-prognosis prostate cancer and exerts an impact on tumour metabolism. It has been shown to affect expression levels of enzymes of oxidative/glycolytic pathway including hexokinase 2, phosphofructokinase, enolase 1, and lactate dehydrogenase A and also GLUT1 levels (19–21). Interestingly, many of these effects are synergistic with the hypoxia-inducible factor 1 (HIF1) function (22). c-Myc also regulates glutamine transporter and mitochondrial glutaminase GLS1 expression through miRNA23a/b and subsequently enhances glutamine metabolism (23, 24). c-Myc may play a significant role in global metabolic reprogramming, such as fuelling citric acid cycle intermediates into anabolic pathways on similar line with AR (25). In addition, c-Myc expression is inversely correlated to AR activity, emphasizing the precise balance and regulation of oncogenic transcription factors thresholds playing a significant role in PCa cells (26). Interestingly, an integrative analysis of metabolomics based on mass spectroscopy revealed differential expression of metabolites; association of AKT1 and MYC activation correlated with accumulation of metabolites of aerobic glycolysis and dysregulated lipid metabolism in human tumours, mouse models, and also in cultured cells (RWPE-1 cells), establishing the oncogene-associated metabolic signatures in PCa (27). In a recent in vivo study, a high-fat diet (HFD) led to both metabolic dysregulation and upregulated the MYC transcriptional cascade. These changes favoured H4K20 histone hypomethylation at the promoter regions of MYC-regulated genes, supporting enhanced cell proliferation and tumour growth. This study exemplifies the link between the activity of oncogenic transcription factors and feedback effects on the epigenetic landscape of cancer genomes, a theme we explore further in this review (28).
PTEN
PTEN is a well-established tumour suppressor exhibiting both protein and lipid phosphatase activities. Loss of PTEN function is common in various cancers including bladder, brain, and prostate cancers, often through the deletion of a single gene copy of PTEN at chromosomal location 10q23 (29, 30). It is a negative regulator of oncogenic PI3K/AKT signalling network and plays a vital role in both lipid and glucose metabolism including mitochondrial functions (31, 32). In vivo studies with transgenic models overexpressing PTEN showed an overall change in the metabolic profile with increase in mitochondrial oxidative phosphorylation and coupled with reduction in glucose and glutamine uptake (33).
PTEN has been used as the basis for the transgenic modelling of prostate cancers, and this has revealed that deletion of this tumour suppressor leads to the activation of SREBP1, a transcription factor that regulates lipogenic genes (34). This transcriptional program is enhanced by co-deletion of PTEN with other factors (for example PML1), and tumorigenesis in these models, analogous to c-Myc, is enhanced through a high-fat diet. In a separate study using a prostate-specific conditional PTEN-null (PTEN−/−) transgenic mouse model of cancer, increased pyruvate dehydrogenase activity was shown to be required for tumorigenesis. Genetic ablation of pyruvate dehydrogenase A1 (Pdha1) in PTEN −/− tumors inhibited tumour growth, and this was associated with the reduced expression of lipogenic genes, which were components of a gene network regulated by sterol regulatory element-binding transcription factor (SREBF). Importantly, nuclear Pdha1 was found to sustain this transcriptional activity by supporting histone H3K9 acetylation at sites bound by SREBF1, putatively supporting its transcriptional activity. Interestingly, whereas the knockdown of Pdha1 in PTEN −/− prostate cancer cells reduced acetylation of histone H3 Lys9 (H3K9ac) at these sites, it had not impact at E2F1 binding sites associated with cell cycle progression genes (35). This specificity, and in fact the molecular basis of the crosstalk between metabolite pools and site-specific, as opposed to global, changes in chromatin modifications or DNA methylation remain largely undefined in this and other published studies identifying similar interplays. One example is the nuclear contribution of ATP-citrate lyase to the provision of acetyl-CoA for histone acetylation in lung cancer (36), and others will be highlighted in the course of this article. Overall, these examples of crosstalk suggest that metabolic reprogramming may sustain and be sustained by transcription factors reinforced by metabolically dependent chromatin modifications.
p53
p53 mutations are amongst the most common features of various cancer types including treatment-resistant prostate cancer (37–39). It is often marked by a loss of one allele and inactivity of the second allele resulting in p53 inactivity resulting in cell cycle deregulation and genomic stability (40, 41). Gain-of-function mutations in p53 can also confer oncogenic properties and resistance towards therapeutics (42). p53 regulates metabolism by inhibiting the expression of genes of pentose phosphate shunt pathway and counteracting Myc- and HIF-induced glycolytic flux (43). p53 can also impair nuclear factor kappa B-dependent glucose uptake and glycolysis by repressing the expression of glucose transporters, GLUT1/4 and GLUT3 (44).
p53 also regulates glutamine metabolism through activation of phosphate-activated mitochondrial glutaminase (GLS2) and mitochondrial glutaminase promoting ATP generation via oxidative phosphorylation (45). In addition, p53 inhibits AR activity; also, a loss of p53 function enhances Myc activity in PCa (46, 47). This may be partly explained by the enhanced amino acid metabolism and mitochondrial activity arising from p53 deletion. For example, p53 deletion results in mitochondrial biogenesis and in mitochondrial dysfunction mediated by PGC-1α mitochondrial in PC3 prostate cancer cells (48). p53 loss also results in enhanced serine/glycine biosynthesis and changes in one-carbon metabolism that support DNA methylation and nucleotide production (49).
Many of the transcriptional effects of p53 on metabolic gene expression are likely to arise from changes in an impact on the activity of chromatin regulators and hence histone methylation and acetylation. p53 gain of mutants modulates chromatin regulatory genes, including the methyltransferases mixed-lineage leukaemia family of histone methyltransferases 1 and 2 (MLL1 and MLL2) and acetyltransferase monocytic leukaemia zinc finger protein (MOZ) resulting in genome-wide upregulation of histone methylation and acetylation (50). p53 negatively modulates H2Bub1 expression independently of the role of p53 as a transcription factor, establishing it as a significant epigenetic modulator (51).
As highlighted, these oncogenic drivers have a significant impact on the balance between glycolytic and TCA cycle activity in cancer cells. It is worth reflecting on the fact that in normal prostate cells, both the TCA cycle and OXPHOS are impeded, and there is a net secretion of citrate. By contrast, in PCa, OXPHOS activity is increased in cancer cells in localised disease and a new dynamic exists between cancer cells and the tumour microenvironment. This dynamic entails increased production and turnover of citrate, a reduction in citrate secretion, and lactate exchange between tumour and stromal cells (52). Lactate exchange sustains both catabolism and anabolism and supports OXPHOS activity in cancer cells. As prostate cancer progresses to a treatment-resistant, metastatic state, TCA cycle activity is once again impaired, and cancers develop a Warburg-like metabolism otherwise termed aerobic glycolysis. So far, no single study has evaluated these metabolic states alongside the genomic landscape of tumour cells and other cell types within the tissue (Figure 1).
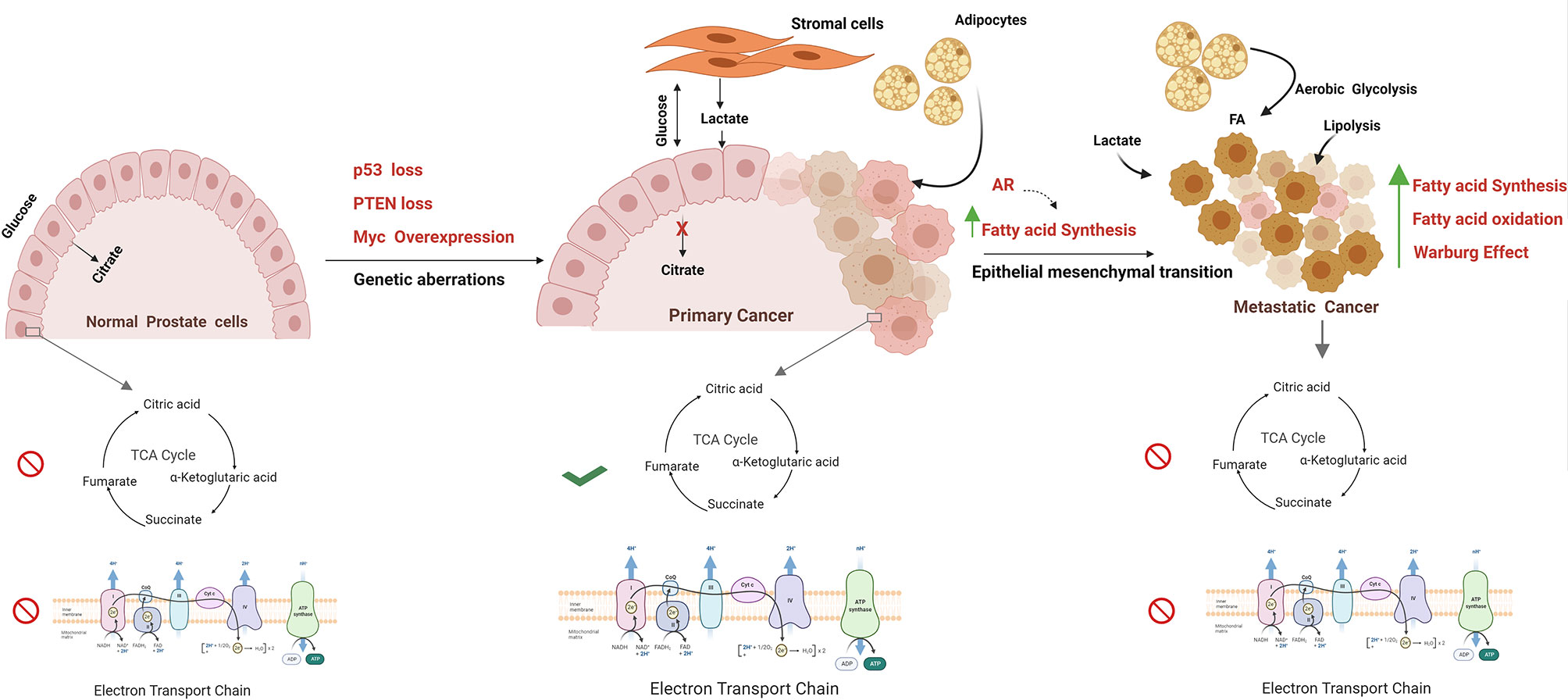
Figure 1 Metabolism alterations in prostate cancer cell. The figure represents the metabolic alterations during prostate cancer metastasis. The normal prostate epithelial cells are characterised with increased release of citrate in seminal fluid, abbreviated Krebs cycle, and reduced oxidative phosphorylation rate. Normal prostate cells undergo transformation due to genetic aberrations; for example, MYC overexpression, PTEN loss, p53 loss, mutations, and other tumor suppressors result in activation of TCA cycle and oxidize citrate and generate Acetyl CoA for lipid biosynthesis. Besides, these fatty acids are fuelled into TCA cycle through lipolysis of adipocytes. Increased Warburg effect is a common feature in metastatic PCa with high lactate secretion, provided by cancer-associated fibroblasts. Progression towards mCRPC is marked by epithelial to mesenchymal transition. In metastatic stage, energy demand is met by both fatty acid oxidation and fatty acid synthesis. The figure has been drawn using Biorender software.
The Crosstalk Between Epigenomes and Metabolism
We have previously outlined the contribution of a number of transcription factors, including p53, c-Myc, and hypoxia-inducing factor (HIF), to prostate cancer progression acting in part by regulating the expression of metabolic enzymes. Metabolism can, in turn, alter the accessibility of chromatin to these factors supplying or restricting hydrocarbon adducts required for epigenetic alterations, principally consisting of histone modifications and DNA methylation (53) (Figure 2). This dynamic relationship may allow cells to rapidly adjust their transcriptional programs in response to treatment or environmental stress and provide the basis for plasticity and the emergence of new cell lineage characteristics in resistant cells (54).
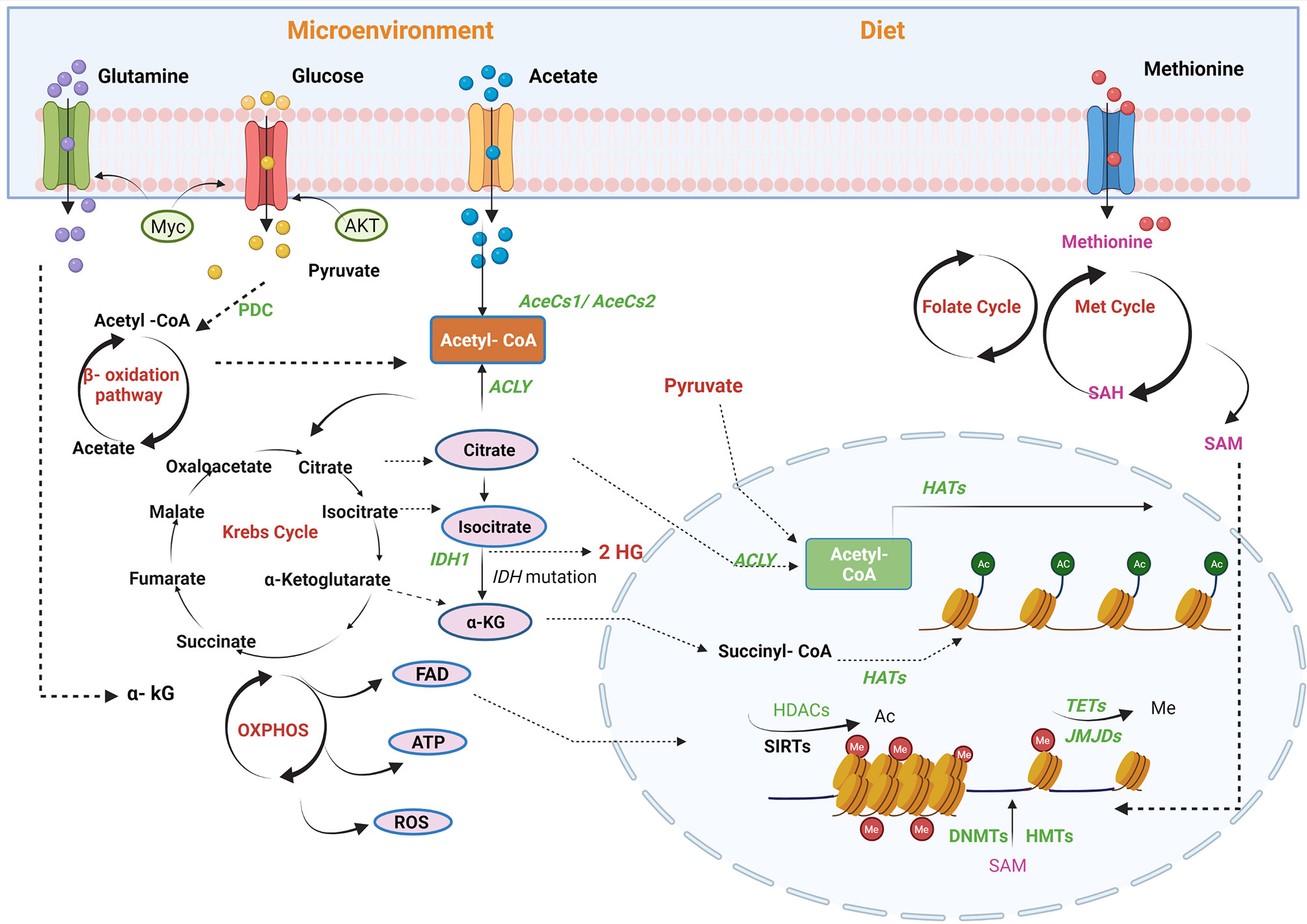
Figure 2 Regulation of PCa epigenome by metabolic pathways. Metabolites are the mediators of epigenetic regulation through mitochondrial and nucleus crosstalk. Different factors contribute to epigenetic regulation, for example, microenvironment and availability of nutrients through diet. Acetyl-CoA, a connecting link between different metabolic pathways, provides acetyl groups in the cell, catalysed by histone acetyltransferases (HATs) for histone modifications. In mitochondria, acetyl-CoA is generated via fatty acid oxidation, catalysis of pyruvate-by-pyruvate dehydrogenases complexes (PDC), and by utilising acetate by acyl-CoA synthetase short-chain family member 1/2 (AceSS1/AceSS1). Furthermore, TCA cycle metabolites, pyruvate, citrate, and acetate translocates into nucleus to generate acetyl-Co A pool by pyruvate dehydrogenase complex, ACLY and AceSS2, respectively. Another TCA cycle metabolite, α-ketoglutarate (KG), also translocates into the nucleus and is utilised by histone demethylases [Jumonji C domain-containing (JMJD)] and DNA demethylase [ten–eleven translocation (TET)]. In addition, isocitrate also diffuses into the cytosol and is converted to α-KG by isocitrate dehydrogenase enzyme. FADH2, a by-product of β-oxidation, is oxidised by electron transport chain into flavin adenine dinucleotide (FAD), which translocates to the cytosol or nucleus. The amino acid cycles, namely, methionine and folate, generate an S-adenosyl methionine (SAM), a methyl group donor utilised by DNA methyltransferase (DNMT) and histone methyltransferases (HMTs). Acetyl-CoA, acetyl-coenzyme; ACLY, ATP-citrate lyase; SIRTs, sirtuins; TETs, ten–eleven translocation family; α-KG, alpha-ketoglutarate; HDAC, histone lysine deacetylase; DNMT, DNA methyltransferase; ATP, adenosine triphosphate; FAD, flavin adenine dinucleotide; TCA, tricarboxylic acid; DNMT, DNA methyltransferase; JMJD, Jumonji C domain-containing histone demethylase. The figure has been created with Biorender.com.
DNA and Histone Methylation
DNA methylation involves the addition of a methyl group to the 5′-carbon of cytosine in CpG dinucleotide sequences catalysed by a family of DNA methyltransferases (DNMTs). CpG islands are CpG-rich regions, located proximal to promoter region of genes with high expression. The DNA methylation/demethylation can result in inhibition/activation of transcription of genes, and in prostate cancer, malignant transformation is a common feature as a result of DNA methylation (55). A study in a castration-resistant prostate metastasis exhibited a novel epigenomic subtype associated with hypermethylation and somatic mutations in TET2, DNMT3B, IDH1, and BRAF and also identified differential methylation associated with transcriptional expression of AR, ERG, and Myc oncogenic drivers (56).
In assessing early-stage drivers of prostate cancer based on their incidence, epigenetic alterations, and particularly DNA methylation change, and gene fusions are far more prevalent than somatic point mutations or indeed copy number alterations in most genomic loci (57). For example, GSTP1 promoter hypermethylation is a feature of >60% of localised prostate cancers, and by contrast, TP53 point mutations/copy number deletions are associated with approximately 10% of localised prostate cancers (58–60). Epigenetic changes are also known to be affected by perturbations in metabolic pools and, in the case of methylation, by changes in TCA cycle metabolites and metabolites associated with serine, glycine, and polyamine biosynthesis and the one-carbon cycle (61). In the TCGA prostate cancer dataset, the prostate tumours with the highest genome-wide levels of DNA hypermethylation carry IDH1 point mutations, and this suggests that perturbations in alpha-ketoglutarate and succinate levels associated with these mutations disrupting methylation status by inhibiting TET enzyme activity and the conversion of methyl- to 5-hydroxymethylcytosine marks (62). Ten–eleven translocation (TET) proteins are dioxygenases involved in the regulation of demethylation by oxidizing 5-methylcytosine to 5-hydroxymethylcytosine. Both expression and activity of TET proteins are deregulated in various ranges of cancers including prostate cancer. Mutations in TET2 and reduced TET have been associated with poor prognosis in in prostate cancer (63). The activity of the TET enzyme is regulated by metabolites from TCA cycle and oxygen pool. Dioxygenases utilise a metabolite, 2-oxoglutarate (2-OG), as an essential cofactor that is generated by isocitrate dehydrogenases (IDH).
Isocitrate dehydrogenase, an NADP+-dependent enzyme, which decarboxylates isocitrate to α-ketoglutarate in the TCA cycle, has been found to carry heterozygous mutations in the prostate including other cancers such as acute myeloid leukaemia (AML) (64). Another study by Ghiam et al., using mutational and array comparative genomic hybridization analyses, has identified IDH1 mutations (R132, R172, or R140 mutations) in localized prostate cancer (PCa) (65). IDH mutations can impair dioxygenase activity by restricting the availability of this cofactor and in turn enhancing the steady-state levels of DNA methylation genome-wide. This DNA hypermethylation phenotype, relative to the cohort as whole, has been observed in the small percentage of prostate cancer TCGA cases carrying IDH mutations (66, 67). A second impact of IDH mutations on DNA methylation has been deciphered through the use of pre-clinical models, which show that these mutations lead to the accumulation of an oncometabolite, R (−)-2-hydroxyglutarate (2HG) (68, 69). In vitro, ectopic expression of IDH1 mutants generate high levels of an oncometabolite, (R)-2HG, which perturbs DNA and histone methylation by inhibiting α-ketoglutarate-dependent enzymes including TET dioxygenases and histone demethylases Jumonji 2 (JMJD2) and JMJ C domain-containing histone demethylase-1 (JHDM1) (70–72). In addition, the perturbation in TCA cycle metabolites arising from these mutations also leads to the stabilization of HIF-1α under normoxic conditions and enhanced glycolytic activity (73).
DNA methyltransferase 1 (DNMT1), the methyltransferase enzyme that modulates gene expression by methylating cytosine residues within CpG dinucleotides, regulates DNA methylation and is found to be overexpressed in higher in localized, metastatic, and hormone-resistant PCa compared with benign prostate hyperplasia (BPH) (74–77). In PCa, high DNMT1 expression has been associated with high grade/stage cancers (78).
Overall, although methylation changes are high-incidence events in localised prostate cancer, yet there is limited evidence to suggest that genome-wide increases in methylation are prognostics. By contrast, chromatin relaxation and increased enhancer activity, associated with histone acetylation, are a feature of castrate-resistant prostate cancer (79).
Histone Acetylation
Histone acetylation occurs on lysine residues and reflects the balance of activity of histone acetyltransferases (HATs) and histone deacetylases (HDACs) (80, 81). HATs utilise acetyl-CoA derived from a number of metabolic processes, and consequently, nutrient availability and utilisation can, in principle, affect the steady-state levels of histone acetylation in tumours (82–84). For example, in PTEN-null prostate cancers, nuclear pyruvate dehydrogenase A1 (PDHA1) is a source of acetyl-CoA for histone H3 K27 acetylation and, as consequence, sustains SREBP1 transcriptional activity (85). Pyruvate dehydrogenase complex (PDH) is another example of an enzyme that links glycolysis and the TCA cycle. It converts pyruvate, a glycolytic metabolite to acetyl CoA in the mitochondria (86). The pyruvate dehydrogenase complex (PDC), however, has also been found in the nucleus in prostate cancer (87). Mitochondrial PDH regulates the availability of citrate in mitochondria for lipid biosynthesis, whereas nuclear regulates expression of sterol regulatory element-binding transcription factor (SREBF)-target genes by mediating histone acetylation. In addition, an amplified expression of PDHA1, both at protein and gene level, have been reported in prostate tumours (87). PDHA1 gene knockout in prostate cancer cells developed alterations in tumor cell metabolism with an increase in expression of glutaminase1 (GLS1) and glutamate dehydrogenase1 (GLUD1), leading to an increase in glutamine-dependent cell survival (88). All these outcomes indicate that PDH supports prostate tumorigenesis not only by regulating lipid biosynthesis but also by utilising alternate metabolic pathways for cell survival.
Altered acetyl-CoA levels significantly affect the substrate specificity of CBP and p300 acetyltransferases. For example, at a low concentration of acetyl CoA, p300 has the highest specificity for histone H4K16, for which specificity is 1018-fold higher than CBP (89). The acetyl-CoA-producing enzyme ATP-citrate lyase (ACLY) regulates histone acetylation levels in a nutrient-dependent manner in cells (36, 89). The location of ACLY in both nucleus and cytosol further suggests that it plays a role in both histone acetylation and lipid biosynthesis (90). In a limited nutrient environment (low glucose levels), cancer cells can still modulate and increase acetyl CoA pool by AKT (S473)-mediated ACLY phosphorylation and upregulates histone acetylation marks in prostate tumors (91).
Whilst a number of recent pre-clinical molecular studies have highlighted important crosstalk between acetyl-CoA production and histone acetylation, contributing tumorigenesis, nothing similar has yet been possible in the study of clinical disease. This in part is due to the dynamic changes that occur in the metabolic states of tumours, which makes it technically very challenging to generate robust high-throughput metabolomic data on a similar scale and resolution to genomic data (refer to Future Perspectives for more discussion). Given the significant progress that has been made in developing epigenetic drugs as cancer therapeutics, it is of course vital to learn more about this interplay because greater functional and clinical understanding could support ultimately the use of metabolic drugs as sensitising agents. Prostate cancers are also susceptible to inhibitors of fatty acid oxidation, a prominent source of acetyl CoA, and examples include etomoxir and perhexiline (92). These are examples of co-dependencies between metabolic and epigenetic activities that maybe amenable to combinatorial treatments if patient stratification is possible. If we consider mitochondrial activity as a primary determinant of the availability of acetyl-CoA within cancer cells, then what is the evidence that mitochondrial mutations exist within tumour cells and also have an impact on the epigenome?
Crosstalk Between Mitochondrial Activity and the Epigenome
Unlike many cancer types, OXPHOS activity is enhanced in the transition from benign/untransformed tissue to cancer in the prostate gland—as discussed above. Given the prominent role that mitochondria play in the turnover of acetyl-CoA, this change might be expected to correlate with increased acetylation. Thus far, no translational studies have attempted to assess the relationship between mitochondrial activity and histone acetylation or indeed chromatin relaxation. A number of studies have, however, shown that mitochondrial mutations accumulate during prostate cancer progression.
The human mitochondrial DNA encodes 13 polypeptides crucial for oxidative phosphorylation, 22 transfer RNA molecules, and 2 ribosomal RNA molecules essential for mitochondrial translational machinery, and the rest is encoded by nuclear genome (93–95). This coordinated expression of subunits of mitochondrial proteins and replication machinery through mitochondrial and nuclear genes is regulated by a bidirectional flow of intermediates (metabolites) and polypeptides including enzymes and is the key of co-regulated biologies of nuclear and mitochondrial processes (96). In addition to somatic mutations in nuclear genome, the mitochondrial genome shows a 55-fold higher incidence of mutation rate in comparison to nuclear genome in PCa (97). A sequencing study identified mutational hotspots in the mitochondrial genomes of 384 prostate cancer and went on to associated mitochondrial mutational burden with Myc amplification and disease recurrence in a subgroup of poor-prognosis patients (98). The functional basis for this relationship remains undefined; however, preclinically researchers have been able to deplete prostate cancer cells of mitochondrial DNA using sub-toxic doses of DNA-damaging agents, creating so-called rho-null derivatives. These depleted cell lines have reduced levels of histone acetylation (principally histone H3K9, H3K18, and H3K27), which suggests that mitochondrial content could affect the epigenetic landscape of tumours (99). In other studies, focussing on the impact of mitochondrial mutations on the tumorigenic potential of prostate cancer cells, it has been possible to use rho-null derivatives as acceptor lines in cell fusion experiments to re-complement the cells with mutated mitochondrial genomes. Since the acceptor and wild-type lines remain isogenic in their autosomal genomes, the enhanced metastatic potential of the resultant cybrids has been attributed to the mutations present in the mitochondrial genome. Using this principle, Petros et al. generated cybrids in PC3 cells with mitochondrial DNA (mtDNA) ATP6 T8993G mutations and engrafted them into immune-compromised mice, resulting in enhanced tumorigenesis compared to wild-type cells and increased production of reactive oxygene species (100). Whilst these distinct studies highlight the impact of mitochondrial activity on the epigenome and of mitochondrial mutations on tumorigenesis, no signal study has related these mechanistically using the models available.
OGlcNAcylation: A Rheostat Connecting Metabolic Dysregulation to Transcription
Histone acetylation and the OGlcNAcylation of chromatin are significant features of enhancers in the prostate cancer genome (101). Histone acetylation has been associated with increased lipid turnover under the influence of a number of the genomic drivers of prostate cancer that were discussed earlier, and particularly with PTEN-loss and Myc overexpression (28). OGlcNAcylation at enhancer sites occupied by c-Myc suggests that these metabolite-dependent modifications may sustain oncogenic activity in a feed-forward manner—transcriptionally driven metabolic dysregulation supporting oncogenic transcriptional activity (Figure 3). OGlcNAcylation, as a post-translational modification sustained by a metabolic adduct (UDP-GlcNAc), is an abundant feature of cancer cells and is, unlike other post-translational modifications, catalysed by a single enzyme, OGlcNAc transferase (OGT) (102, 103). UDP-GlcNAc is synthesised by the hexosamine biosynthesis pathway and utilised both by OGT and by enzymes in the endoplasmic reticulum for the N-linked glycosylation and proper folding of newly synthesised proteins. Consequently, whilst enzymes in the hexosamine biosynthesis pathway are AR dependent, and many are overexpressed in prostate cancer, there is not necessarily a direct relationship between their activity and the OGlcNAcylation status of OGT substrates (104). Indeed, recent studies indicate that hexosamine biosynthesis itself may restrain the emergence of castrate-resistant prostate cancer (105). OGT activity itself, however, can support cancer progression, and the challenge is in determining the nature of the substrates, and biological processes are the primary mediators of this crosstalk. There are a number of biologically compelling candidates including c-Myc, FOXM1, and HIF1a, all of which are known to be OGlcNAcylated and more active due to OGT function (106). There are also broader impacts of OGT activity; at the chromatin level, it is known to modify histones, and transcriptionally, it is known to regulate RNA polymerase II activation and processivity working in concert with cyclin-dependent kinases such as CDK7 and CDK9 (107). By implication, OGT activity reflects a nutrient-replete/”fed” state sufficient to permit glucose, glutamine, UTP, and acetyl-CoA to be used to provide adequate UDP-GlcNAc as a substrate for OGT (108). However, that alone is unlikely to provide an understanding of crosstalk; we need to establish more clearly how OGT selects protein substrates, and we also need to account for a second enzymatic activity ascribed to OGT, its proteolytic function. A recent study has indicated that in some cell types, OGT can sustain cell proliferation through a non-catalytic function that needs to be fully characterised (109). This in turn also needs to be put into a spatial context, since OGT can function as different isoforms in distinct organelles within the cell, mitochondria, and nuclei being principal examples. That final aspect is of course reminiscent of TCA cycle enzymes such as PDHA1 and ATP-citrate lyase as previously described in the context of acetylation.
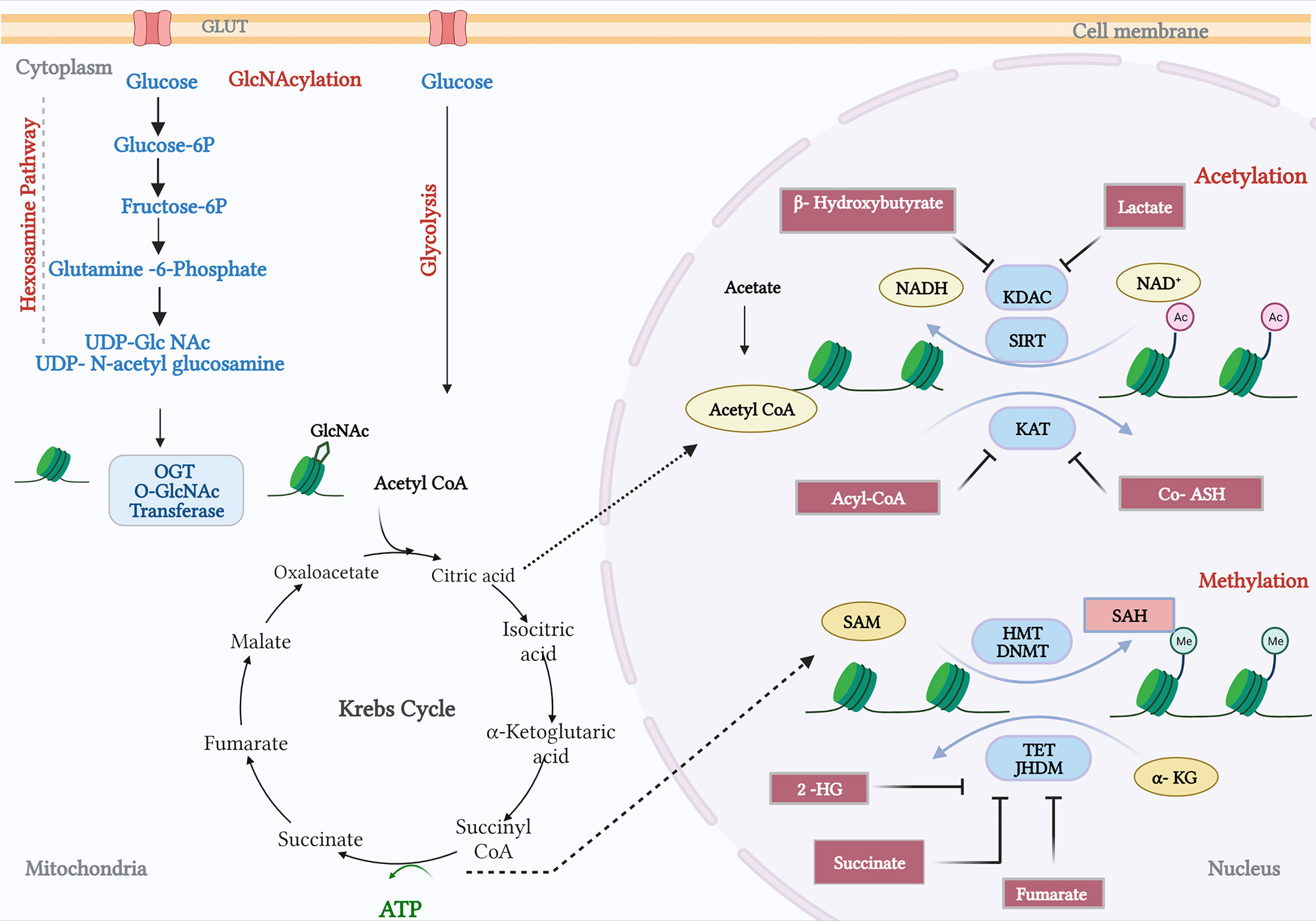
Figure 3 Crosstalk between metabolism and prostate cancer genomics. The hexosamine pathway catalyses the conversion of glucose and glutamine to provide a metabolite UDP-glucosamine (UDP-GlcNAc). The O-GlcNAc transferase (OGT) facilitates the N-acetylglucosamination (GlcNAcylation) or Glycation process. In addition, the Krebs cycle fuels metabolites private and citrate, which gets converted by acyl-COA synthetase and pyruvate dehydrogenase. Products from fatty acid oxidation, fatty Acyl CoA, inhibit the activity of lysine acetyltransferases (KAT). KAT reactions also release CoA-SH, which acts as an inhibitor. B-Hydroxybutyrate, a ketone from FA, and lactate, a by-product of glycolysis, have been shown to inhibit lysine deacetylase (KDAC). Amino acid metabolism and TCA cycle fuels in methionine and ATP, respectively, and synthesizes S-adenosylmethionine (SAM). Histone methyl transferases and DNA methyl transferases catalyse SAM to S-adenosylhomocysteine (SAH), which in turn can inhibit DNMTs and HMTs. TCA cycle intermediates, succinate, fumarate, α-ketoglutarate (α-KG), and 2-hydroxyglutarate (2-HG) acts as inhibitors of TET demethylases and Jumonji-C (JMJC) domain-containing histone demethylases (JHDMs). The figure has been adapted and modified from the review (101).
Given that a limited number of metabolic pathways may be the mediators of feedback effects of oncogenes and tumour suppressors on chromatin/the epigenome, how do we use this knowledge to benefit patients? To achieve this, we arguably need to fill a knowledge gap in our ability to identify metabolites and understand how changes in metabolic activity occur spatially within tumours.
Future Perspectives
As outlined in this article, there are significant examples of crosstalk between metabolic pathways and other cancer drivers; it remains challenging to prove that this crosstalk plays a causative role in prostate cancer progression. This is because changes in metabolic activity will inevitably impact on the redox state of the cell and the availability of metabolites for anabolic metabolism and sustain transcription and DNA replication. In addition, most metabolic processes are also contributors to the functions of untransformed cells in immune system and tumour micro-environment and are affected by the availability of nutrients and by crosstalk between ranges of cell types. In the big picture, how do we achieve cancer selectivity in modelling this interplay and in targeting this crosstalk?
First, an important factor to address is our lack of knowledge of the metabolome. As it stands, a metabolomics study can only identify maximally approximately 10% of the metabolite signals that are measurable using mass spectroscopy or other methods. This means that our understanding of the activity of mitochondria and metabolic pathways in cancer cells is constrained by our capacity to identify novel metabolites (“oncometabolites”) in a sensitive and unbiased manner. This missing information is by contrast increasingly an alien concept in the field of cancer genomics due to the capacity to sequence at high scale and decode genomic data. This has led, for example, to the discovery of highly cancer-specific non-coding transcripts and somatic DNA mutations; we have no equivalent signatures thus far in the cancer metabolome. Addressing this point is predominantly a technical challenge.
Second, clinical disease is by definition molecular heterogeneous. We know that this is true when we assess bulk sequencing data and look for high incidence mutations in multi-focal tumour samples from a single patient. However, recently, it has been possible to combine spatial information including pathology and genomic data using new platforms that permit RNA extraction, library preparation, and sequencing on a solid-phase surface/glass slide. This spatial dimension permits molecular information to be mapped onto distinct cell sub-populations and interpreted more readily in the context of associations between cell types at tumour–stromal interface and elsewhere. As a consequence, spatially resolved transcriptomics was declared to be the Method of the Year for 2020 by Nature Methods (110). Equivalent spatial resolution of those metabolic signals that we can attribute to known metabolites would provide great insights into cell–cell crosstalk in prostate cancers. This is important to test hypotheses, for example, around the compartmentalisation of metabolism in cancers, such as the idea of a lactate shuttle between cancer and stromal cells and compartmentalisation of glycolysis and oxidative phosphorylation between distinct cell types that communicate with each other in tumours (111). Significant progress is being made in developing single-cell spatial and in situ methods for the sensitive detection of well-known metabolites, and mass spectrometry imaging is showing promise in defining more complex and accurate metabolic classifiers of disease (112). However, there is a need for new devices/biomedical engineering to capture metabolic information in real time in an operating theatre, as the signals can be significantly affected by environmental factors. As matter of concern, the metabolomic signals are dynamic/unstable, and sequencing data (DNA) is stable, and technology needs to address those differences (113–117). With the vast increase in prostate cancer genomic data and other data types (clinical, pathological, imaging, metabolomics, and proteomics), there is a significant challenge in assimilating, refining, and deciphering biologically informative signals that reflect crosstalk. Machine-learning algorithms and artificial intelligence promise to alleviate this issue, since they are, in many cases, data-type agnostic. Their impact is exemplified in the sphere of medical imaging. Digital pathology, aided by artificial Intelligence (AI), has decoded large datasets to improve the reliability of diagnostic pathology and improve the prediction of treatment outcome and patient survival (118). Additionally, multiparametric MRI has significantly improved sampling of clinical significant prostate cancers at biopsy, and improvements in both the scanners and analytical approaches employed on the resultant data will further enhance and standardise this work across clinical centres (119). We can anticipate a future in which spatial genomic and metabolomic data are aligned to radiomic features and imaging to risk stratify patients and simultaneously inform treatment selection (119).
Author Contributions
RS wrote the manuscript and made the figures. IM discussed the themes and provided advice during the drafting process. All authors contributed to the article and approved the submitted version.
Conflict of Interest
The authors declare that the research was conducted in the absence of any commercial or financial relationships that could be construed as a potential conflict of interest.
Publisher’s Note
All claims expressed in this article are solely those of the authors and do not necessarily represent those of their affiliated organizations, or those of the publisher, the editors and the reviewers. Any product that may be evaluated in this article, or claim that may be made by its manufacturer, is not guaranteed or endorsed by the publisher.
Acknowledgments
IM and RS were both supported by the John Black Charitable Foundation.
References
1. Jiang Y, Palma JF, Agus DB, Wang Y, Gross ME. Detection of Androgen Receptor Mutations in Circulating Tumor Cells in Castration-Resistant Prostate Cancer. Clin Chem (2010) 56(9):1492–5. doi: 10.1373/clinchem.2010.143297
2. Sharma NL, Massie CE, Ramos-Montoya A, Zecchini V, Scott HE, Lamb AD, et al. The Androgen Receptor Induces a Distinct Transcriptional Program in Castration-Resistant Prostate Cancer in Man. Cancer Cell (2013) 23(1):35–47. doi: 10.1016/j.ccr.2012.11.010
3. Blessing AM, Rajapakshe K, Reddy Bollu L, Shi Y, White MA, Pham AH, et al. Transcriptional Regulation of Core Autophagy and Lysosomal Genes by the Androgen Receptor Promotes Prostate Cancer Progression. Autophagy (2017) 13(3):506–21. doi: 10.1080/15548627.2016.1268300
4. Shi Y, Han JJ, Tennakoon JB, Mehta FF, Merchant FA, Burns AR, et al. Androgens Promote Prostate Cancer Cell Growth Through Induction of Autophagy. Mol Endocrinol (2013) 27(2):280–95. doi: 10.1210/me.2012-1260
5. Goodwin JF, Schiewer MJ, Dean JL, Schrecengost RS, de Leeuw R, Han S, et al. A Hormone-DNA Repair Circuit Governs the Response to Genotoxic Insult. Cancer Discovery (2013) 3(11):1254–71. doi: 10.1158/2159-8290.CD-13-0108
6. Polkinghorn WR, Parker JS, Lee MX, Kass EM, Spratt DE, Iaquinta PJ, et al. Androgen Receptor Signaling Regulates DNA Repair in Prostate Cancers. Cancer Discovery (2013) 3(11):1245–53. doi: 10.1158/2159-8290.CD-13-0172
7. Jividen K, Kedzierska KZ, Yang CS, Szlachta K, Ratan A, Paschal BM, et al. Genomic Analysis of DNA Repair Genes and Androgen Signaling in Prostate Cancer. BMC Cancer (2018) 18(1):960. doi: 10.1186/s12885-018-4848-x
8. Massie CE, Lynch A, Ramos-Montoya A, Boren J, Stark R, Fazli L, et al. The Androgen Receptor Fuels Prostate Cancer by Regulating Central Metabolism and Biosynthesis. EMBO J (2011) 30(13):2719–33. doi: 10.1038/emboj.2011.158
9. Audet-Walsh E, Yee T, McGuirk S, Vernier M, Ouellet C, St-Pierre J, et al. Androgen-Dependent Repression of ERRgamma Reprograms Metabolism in Prostate Cancer. Cancer Res (2017) 77(2):378–89. doi: 10.1158/0008-5472.CAN-16-1204
10. Audet-Walsh E, Dufour CR, Yee T, Zouanat FZ, Yan M, Kalloghlian G, et al. Nuclear mTOR Acts as a Transcriptional Integrator of the Androgen Signaling Pathway in Prostate Cancer. Genes Dev (2017) 31(12):1228–42. doi: 10.1101/gad.299958.117
11. Costello LC, Franklin RB, Feng P. Mitochondrial Function, Zinc, and Intermediary Metabolism Relationships in Normal Prostate and Prostate Cancer. Mitochondrion (2005) 5(3):143–53. doi: 10.1016/j.mito.2005.02.001
12. Twum-Ampofo J, Fu DX, Passaniti A, Hussain A, Siddiqui MM. Metabolic Targets for Potential Prostate Cancer Therapeutics. Curr Opin Oncol (2016) 28(3):241–7. doi: 10.1097/CCO.0000000000000276
13. Tennakoon JB, Shi Y, Han JJ, Tsouko E, White MA, Burns AR, et al. Androgens Regulate Prostate Cancer Cell Growth via an AMPK-PGC-1alpha-Mediated Metabolic Switch. Oncogene (2014) 33(45):5251–61. doi: 10.1038/onc.2013.463
14. Bader DA, Hartig SM, Putluri V, Foley C, Hamilton MP, Smith EA, et al. Mitochondrial Pyruvate Import Is a Metabolic Vulnerability in Androgen Receptor-Driven Prostate Cancer. Nat Metab (2019) 1(1):70–85. doi: 10.1038/s42255-018-0002-y
15. Verhoeven G. Androgens and Increased Lipogenesis in Prostate Cancer. Cell Biologic and Clinical Perspectives. Verh K Acad Geneeskd Belg (2002) 64(3):189–95; discussion 195-6.
16. Butler LM, Centenera MM, Swinnen JV. Androgen Control of Lipid Metabolism in Prostate Cancer: Novel Insights and Future Applications. Endocr Relat Cancer (2016) 23(5):R219–27. doi: 10.1530/ERC-15-0556
17. Flaig TW, Salzmann-Sullivan M, Su LJ, Zhang Z, Joshi M, Gijon MA, et al. Lipid Catabolism Inhibition Sensitizes Prostate Cancer Cells to Antiandrogen Blockade. Oncotarget (2017) 8(34):56051–65. doi: 10.18632/oncotarget.17359
18. Heemers H, Vanderhoydonc F, Roskams T, Shechter I, Heyns W, Verhoeven G, et al. Androgens Stimulate Coordinated Lipogenic Gene Expression in Normal Target Tissues In Vivo. Mol Cell Endocrinol (2003) 205(1-2):21–31. doi: 10.1016/S0303-7207(03)00205-3
19. Shim H, Dolde C, Lewis BC, Wu CS, Dang G, Jungmann RA, et al. C-Myc Transactivation of LDH-A: Implications for Tumor Metabolism and Growth. Proc Natl Acad Sci USA (1997) 94(13):6658–63. doi: 10.1073/pnas.94.13.6658
20. Osthus RC, Shim H, Kim S, Li Q, Reddy R, Mukherjee M, et al. Deregulation of Glucose Transporter 1 and Glycolytic Gene Expression by C-Myc. J Biol Chem (2000) 275(29):21797–800. doi: 10.1074/jbc.C000023200
21. Kim JW, Zeller KI, Wang Y, Jegga AG, Aronow BJ, O'Donnell KA, et al. Evaluation of Myc E-Box Phylogenetic Footprints in Glycolytic Genes by Chromatin Immunoprecipitation Assays. Mol Cell Biol (2004) 24(13):5923–36. doi: 10.1128/MCB.24.13.5923-5936.2004
22. Gordan JD, Thompson CB, Simon MC. HIF and C-Myc: Sibling Rivals for Control of Cancer Cell Metabolism and Proliferation. Cancer Cell (2007) 12(2):108–13. doi: 10.1016/j.ccr.2007.07.006
23. Wise DR, DeBerardinis RJ, Mancuso A, Sayed N, Zhang XY, Pfeiffer HY, et al. Myc Regulates a Transcriptional Program That Stimulates Mitochondrial Glutaminolysis and Leads to Glutamine Addiction. Proc Natl Acad Sci USA (2008) 105(48):18782–7. doi: 10.1073/pnas.0810199105
24. Gao P, Tchernyshyov I, Chang TC, Lee YS, Kita K, Ochi T, et al. C-Myc Suppression of miR-23a/B Enhances Mitochondrial Glutaminase Expression and Glutamine Metabolism. Nature (2009) 458(7239):762–5. doi: 10.1038/nature07823
25. O’Connell BC, Cheung AF, Simkevich CP, Tam W, Ren X, Mateyak MK, et al. A Large Scale Genetic Analysis of C-Myc-Regulated Gene Expression Patterns. J Biol Chem (2003) 278(14):12563–73. doi: 10.1074/jbc.M210462200
26. Kokontis J, Takakura K, Hay N, Liao S. Increased Androgen Receptor Activity and Altered C-Myc Expression in Prostate Cancer Cells After Long-Term Androgen Deprivation. Cancer Res (1994) 54(6):1566–73.
27. Priolo C, Pyne S, Rose J, Regan ER, Zadra G, Photopoulos C, et al. AKT1 and MYC Induce Distinctive Metabolic Fingerprints in Human Prostate Cancer. Cancer Res (2014) 74(24):7198–204. doi: 10.1158/0008-5472.CAN-14-1490
28. Labbe DP, Zadra G, Yang M, Reyes JM, Lin CY, Cacciatore S, et al. High-Fat Diet Fuels Prostate Cancer Progression by Rewiring the Metabolome and Amplifying the MYC Program. Nat Commun (2019) 10(1):4358. doi: 10.1038/s41467-019-12298-z
29. Li J, Yen C, Liaw D, Podsypanina K, Bose S, Wang SI, et al. PTEN, a Putative Protein Tyrosine Phosphatase Gene Mutated in Human Brain, Breast, and Prostate Cancer. Science (1997) 275(5308):1943–7. doi: 10.1126/science.275.5308.1943
30. Alvarez-Garcia V, Tawil Y, Wise HM, Leslie NR. Mechanisms of PTEN Loss in Cancer: It’s All About Diversity. Semin Cancer Biol (2019) 59:66–79. doi: 10.1016/j.semcancer.2019.02.001
31. Stiles B, Wang Y, Stahl A, Bassilian S, Lee WP, Kim YJ, et al. Liver-Specific Deletion of Negative Regulator Pten Results in Fatty Liver and Insulin Hypersensitivity [Corrected]. Proc Natl Acad Sci USA (2004) 101(7):2082–7. doi: 10.1073/pnas.0308617100
32. Kurlawalla-Martinez C, Stiles B, Wang Y, Devaskar SU, Kahn BB, Wu H. Insulin Hypersensitivity and Resistance to Streptozotocin-Induced Diabetes in Mice Lacking PTEN in Adipose Tissue. Mol Cell Biol (2005) 25(6):2498–510. doi: 10.1128/MCB.25.6.2498-2510.2005
33. Garcia-Cao I, Song MS, Hobbs RM, Laurent G, Giorgi C, de Boer VC, et al. Systemic Elevation of PTEN Induces a Tumor-Suppressive Metabolic State. Cell (2012) 149(1):49–62. doi: 10.1016/j.cell.2012.02.030
34. Chen M, Zhang J, Sampieri K, Clohessy JG, Mendez L, Gonzalez-Billalabeitia E, et al. An Aberrant SREBP-Dependent Lipogenic Program Promotes Metastatic Prostate Cancer. Nat Genet (2018) 50(2):206–18. doi: 10.1038/s41588-017-0027-2
35. Chen J, Guccini I, Di Mitri D, Brina D, Revandkar A, Sarti M, et al. Compartmentalized Activities of the Pyruvate Dehydrogenase Complex Sustain Lipogenesis in Prostate Cancer. Nat Genet (2018) 50(2):219–28. doi: 10.1038/s41588-017-0026-3
36. Wellen KE, Hatzivassiliou G, Sachdeva UM, Bui T, Cross V, Thompson JR. ATP-Citrate Lyase Links Cellular Metabolism to Histone Acetylation. Science (2009) 324(5930):1076–80. doi: 10.1126/science.1164097
37. Hall MC, Navone NM, Troncoso P, Pollack A, Zagars G, von Eschenbach K, et al. Frequency and Characterization of P53 Mutations in Clinically Localized Prostate Cancer. Urology (1995) 45(3):470–5. doi: 10.1016/S0090-4295(99)80018-1
38. Stein Y, Rotter V, Aloni-Grinstein R. Gain-Of-Function Mutant P53: All the Roads Lead to Tumorigenesis. Int J Mol Sci (2019) 20(24). doi: 10.3390/ijms20246197
39. Ecke TH, Schlechte HH, Schiemenz K, Sachs MD, Lenk SV, Rudolph BD, et al. TP53 Gene Mutations in Prostate Cancer Progression. Anticancer Res (2010) 30(5):1579–86.
40. Kandoth C, McLellan MD, Vandin F, Ye K, Niu B, Lu C, et al. Mutational Landscape and Significance Across 12 Major Cancer Types. Nature (2013) 502(7471):333–9. doi: 10.1038/nature12634
41. Kastan MB, Berkovich E. P53: A Two-Faced Cancer Gene. Nat Cell Biol (2007) 9(5):489–91. doi: 10.1038/ncb0507-489
42. Blandino G, Levine AJ, Oren M. Mutant P53 Gain of Function: Differential Effects of Different P53 Mutants on Resistance of Cultured Cells to Chemotherapy. Oncogene (1999) 18(2):477–85. doi: 10.1038/sj.onc.1202314
43. Zawacka-Pankau J, Grinkevich VV, Hunten S, Nikulenkov F, Gluch A, Li H, et al. Inhibition of Glycolytic Enzymes Mediated by Pharmacologically Activated P53: Targeting Warburg Effect to Fight Cancer. J Biol Chem (2011) 286(48):41600–15. doi: 10.1074/jbc.M111.240812
44. Gomes AS, Ramos H, Soares J, Saraiva L. P53 and Glucose Metabolism: An Orchestra to be Directed in Cancer Therapy. Pharmacol Res (2018) 131:75–86. doi: 10.1016/j.phrs.2018.03.015
45. Vousden KH. Alternative Fuel–Another Role for P53 in the Regulation of Metabolism. Proc Natl Acad Sci USA (2010) 107(16):7117–8. doi: 10.1073/pnas.1002656107
46. Cronauer MV, Schulz WA, Burchardt T, Ackermann R, Burchardt M. Inhibition of P53 Function Diminishes Androgen Receptor-Mediated Signaling in Prostate Cancer Cell Lines. Oncogene (2004) 23(20):3541–9. doi: 10.1038/sj.onc.1207346
47. Thompson TC, Park SH, Timme TL, Ren C, Eastham J, Donehower A, et al. Loss of P53 Function Leads to Metastasis in Ras+Myc-Initiated Mouse Prostate Cancer. Oncogene (1995) 10(5):869–79.
48. Li J, Li Y, Chen L, Yu B, Xue Y, Guo R, et al. P53/PGC1alphamediated Mitochondrial Dysfunction Promotes PC3 Prostate Cancer Cell Apoptosis. Mol Med Rep (2020) 22(1):155–64. doi: 10.3892/mmr.2020.11121
49. Shuvalov O, Petukhov A, Daks A, Fedorova O, Vasileva E, Barlev NA. One-Carbon Metabolism and Nucleotide Biosynthesis as Attractive Targets for Anticancer Therapy. Oncotarget (2017) 8(14):23955–77. doi: 10.18632/oncotarget.15053
50. Zhu J, Sammons MA, Donahue G, Dou Z, Vedadi M, Getlik M, et al. Gain-Of-Function P53 Mutants Co-Opt Chromatin Pathways to Drive Cancer Growth. Nature (2015) 525(7568):206–11. doi: 10.1038/nature15251
51. Wang Y, Yang L, Zhang X, Cui W, Liu Y, Sun QR, et al. Epigenetic Regulation of Ferroptosis by H2B Monoubiquitination and P53. EMBO Rep (2019) 20(7):e47563. doi: 10.15252/embr.201847563
52. Ippolito L, Morandi A, Taddei ML, Parri M, Comito G, Iscaro A, et al. Cancer-Associated Fibroblasts Promote Prostate Cancer Malignancy via Metabolic Rewiring and Mitochondrial Transfer. Oncogene (2019) 38(27):5339–55. doi: 10.1038/s41388-019-0805-7
53. Damaschke NA, Yang B, Bhusari S, Svaren JP, Jarrard DF. Epigenetic Susceptibility Factors for Prostate Cancer With Aging. Prostate (2013) 73(16):1721–30. doi: 10.1002/pros.22716
54. Flavahan WA, Gaskell E, Bernstein BE. Epigenetic Plasticity and the Hallmarks of Cancer. Science (2017) 357(6348). doi: 10.1126/science.aal2380
55. Chin SP, Dickinson JL, Holloway AF. Epigenetic Regulation of Prostate Cancer. Clin Epigenet (2011) 2(2):151–69. doi: 10.1007/s13148-011-0041-7
56. Zhao SG, Chen WS, Li H, Foye A, Zhang M, Sjostrom M, et al. The DNA Methylation Landscape of Advanced Prostate Cancer. Nat Genet (2020) 52(8):778–89. doi: 10.1038/s41588-020-0648-8
57. Cancer Genome Atlas Research, N. The Molecular Taxonomy of Primary Prostate Cancer. Cell (2015) 163(4):1011–25. doi: 10.1016/j.cell.2015.10.025
58. Maldonado L, Brait M, Loyo M, Sullenberger L, Wang K, Peskoe SB, et al. GSTP1 Promoter Methylation Is Associated With Recurrence in Early Stage Prostate Cancer. J Urol (2014) 192(5):1542–8. doi: 10.1016/j.juro.2014.04.082
59. Nam RK, Sugar L, Wang Z, Yang W, Kitching R, Klotz LH, et al. Expression of TMPRSS2:ERG Gene Fusion in Prostate Cancer Cells Is an Important Prognostic Factor for Cancer Progression. Cancer Biol Ther (2007) 6(1):40–5. doi: 10.4161/cbt.6.1.3489
60. Robinson D, Van Allen EM, Wu YM, Schultz N, Lonigro RJ, Mosquera JM, et al. Integrative Clinical Genomics of Advanced Prostate Cancer. Cell (2015) 161(5):1215–28. doi: 10.1016/j.cell.2015.05.001
61. Sreekumar A, Poisson LM, Rajendiran TM, Khan AP, Cao Q, Yu J, et al. Metabolomic Profiles Delineate Potential Role for Sarcosine in Prostate Cancer Progression. Nature (2009) 457(7231):910–4. doi: 10.1038/nature07762
62. Smeets E, Lynch AG, Prekovic S, Van den Broeck T, Moris L, Helsen C, et al. The Role of TET-Mediated DNA Hydroxymethylation in Prostate Cancer. Mol Cell Endocrinol (2018) 462(Pt A):41–55. doi: 10.1016/j.mce.2017.08.021
63. Spans L, Van den Broeck T, Smeets E, Prekovic S, Thienpont B, Lambrechts D, et al. Genomic and Epigenomic Analysis of High-Risk Prostate Cancer Reveals Changes in Hydroxymethylation and TET1. Oncotarget (2016) 7(17):24326–38. doi: 10.18632/oncotarget.8220
64. Reitman ZJ, Yan H. Isocitrate Dehydrogenase 1 and 2 Mutations in Cancer: Alterations at a Crossroads of Cellular Metabolism. J Natl Cancer Inst (2010) 102(13):932–41. doi: 10.1093/jnci/djq187
65. Ghiam AF, Cairns RA, Thoms J, Dal Pra A, Ahmed O, Meng A, et al. IDH Mutation Status in Prostate Cancer. Oncogene (2012) 31(33):3826. doi: 10.1038/onc.2011.546
66. Sciacovelli M, Goncalves E, Johnson TI, Zecchini VR, da Costa AS, Gaude E, et al. Fumarate Is an Epigenetic Modifier That Elicits Epithelial-to-Mesenchymal Transition. Nature (2016) 537(7621):544–7. doi: 10.1038/nature19353
67. Chen MF, Chen WC, Chang YJ, Wu CF, Wu C. Role of DNA Methyltransferase 1 in Hormone-Resistant Prostate Cancer. J Mol Med (Berl) (2010) 88(9):953–62. doi: 10.1007/s00109-010-0640-3
68. Turcan S, Rohle D, Goenka A, Walsh LA, Fang F, Yilmaz C, et al. IDH1 Mutation Is Sufficient to Establish the Glioma Hypermethylator Phenotype. Nature (2012) 483(7390):479–83. doi: 10.1038/nature10866
69. Dang L, White DW, Gross S, Bennett BD, Bittinger MA, Driggers EM, et al. Cancer-Associated IDH1 Mutations Produce 2-Hydroxyglutarate. Nature (2009) 462(7274):739–44. doi: 10.1038/nature08617
70. Chowdhury R, Yeoh KK, Tian YM, Hillringhaus L, Bagg EA, Rose NR, et al. The Oncometabolite 2-Hydroxyglutarate Inhibits Histone Lysine Demethylases. EMBO Rep (2011) 12(5):463–9. doi: 10.1038/embor.2011.43
71. Tsukada Y, Fang J, Erdjument-Bromage H, Warren ME, Borchers CH, Tempst P, et al. Histone Demethylation by a Family of JmjC Domain-Containing Proteins. Nature (2006) 439(7078):811–6. doi: 10.1038/nature04433
72. Pastor WA, Aravind L, Rao A. TETonic Shift: Biological Roles of TET Proteins in DNA Demethylation and Transcription. Nat Rev Mol Cell Biol (2013) 14(6):341–56. doi: 10.1038/nrm3589
73. Yen KE, Bittinger MA, Su SM, Fantin VR. Cancer-Associated IDH Mutations: Biomarker and Therapeutic Opportunities. Oncogene (2010) 29(49):6409–17. doi: 10.1038/onc.2010.444
74. Yegnasubramanian S, Kowalski J, Gonzalgo ML, Zahurak M, Piantadosi S, Walsh PC, et al. Hypermethylation of CpG Islands in Primary and Metastatic Human Prostate Cancer. Cancer Res (2004) 64(6):1975–86. doi: 10.1158/0008-5472.CAN-03-3972
75. Maruyama R, Toyooka S, Toyooka KO, Virmani AK, Zochbauer-Muller S, Farinas AJ, et al. Aberrant Promoter Methylation Profile of Prostate Cancers and its Relationship to Clinicopathological Features. Clin Cancer Res (2002) 8(2):514–9.
76. Zhang Q, Chen L, Helfand BT, Jang TL, Sharma V, Kozlowski J, et al. TGF-Beta Regulates DNA Methyltransferase Expression in Prostate Cancer, Correlates With Aggressive Capabilities, and Predicts Disease Recurrence. PloS One (2011) 6(9):e25168. doi: 10.1371/journal.pone.0025168
77. Zhang W, Jiao H, Zhang X, Zhao R, Wang F, He W, et al. Correlation Between the Expression of DNMT1, and GSTP1 and APC, and the Methylation Status of GSTP1 and APC in Association With Their Clinical Significance in Prostate Cancer. Mol Med Rep (2015) 12(1):141–6. doi: 10.3892/mmr.2015.3402
78. Valdez CD, Kunju L, Daignault S, Wojno KJ, Day ML. The E2F1/DNMT1 Axis Is Associated With the Development of AR Negative Castration Resistant Prostate Cancer. Prostate (2013) 73(16):1776–85. doi: 10.1002/pros.22715
79. Ruggero K, Farran-Matas S, Martinez-Tebar A, Aytes A. Epigenetic Regulation in Prostate Cancer Progression. Curr Mol Biol Rep (2018) 4(2):101–15. doi: 10.1007/s40610-018-0095-9
80. Allfrey VG, Faulkner R, Mirsky AE. Acetylation and Methylation of Histones and Their Possible Role in the Regulation of Rna Synthesis. Proc Natl Acad Sci USA (1964) 51:786–94. doi: 10.1073/pnas.51.5.786
81. Bannister AJ, Kouzarides T. Regulation of Chromatin by Histone Modifications. Cell Res (2011) 21(3):381–95. doi: 10.1038/cr.2011.22
82. Pietrocola F, Galluzzi L, Bravo-San Pedro JM, Madeo F, Kroemer G. Acetyl Coenzyme A: A Central Metabolite and Second Messenger. Cell Metab (2015) 21(6):805–21. doi: 10.1016/j.cmet.2015.05.014
83. Sivanand S, Viney I, Wellen KE. Spatiotemporal Control of Acetyl-CoA Metabolism in Chromatin Regulation. Trends Biochem Sci (2018) 43(1):61–74. doi: 10.1016/j.tibs.2017.11.004
84. Choudhary C, Weinert BT, Nishida Y, Verdin E, Mann M. The Growing Landscape of Lysine Acetylation Links Metabolism and Cell Signalling. Nat Rev Mol Cell Biol (2014) 15(8):536–50. doi: 10.1038/nrm3841
85. Chen J, Guccini I, Di Mitri D, Brina D, Revandkar A, Sarti M, et al. Publisher Correction: Compartmentalized Activities of the Pyruvate Dehydrogenase Complex Sustain Lipogenesis in Prostate Cancer. Nat Genet (2018) 50(9):1343. doi: 10.1038/s41588-018-0181-1
86. Cai Z, Li CF, Han F, Liu C, Zhang A, Hsu CC, et al. Phosphorylation of PDHA by AMPK Drives TCA Cycle to Promote Cancer Metastasis. Mol Cell (2020) 80(2):263–278 e7. doi: 10.1016/j.molcel.2020.09.018
87. Atala A. Re: Compartmentalized Activities of the Pyruvate Dehydrogenase Complex Sustain Lipogenesis in Prostate Cancer. J Urol (2018) 200(4):701–2. doi: 10.1016/j.juro.2018.07.004
88. Li Y, Li X, Li X, Zhong Y, Ji Y, Yu D, et al. PDHA1 Gene Knockout in Prostate Cancer Cells Results in Metabolic Reprogramming Towards Greater Glutamine Dependence. Oncotarget (2016) 7(33):53837–52. doi: 10.18632/oncotarget.10782
89. Lafferty KJ, Ryan MA. Differences in Genetic Specificity are Observed When Spleen and Lymph Node Leucocytes are Used as the Responsive Cell Population in Mixed Leucocyte Cultures. Aust J Exp Biol Med Sci (1974) 52(Pt 3):595–8. doi: 10.1038/icb.1974.57
90. Marino G, Pietrocola F, Eisenberg T, Kong Y, Malik S, Andryushkova A, et al. Regulation of Autophagy by Cytosolic Acetyl-Coenzyme A. Mol Cell (2014) 53(5):710–25. doi: 10.1016/j.molcel.2014.01.016
91. Lee JV, Carrer A, Shah S, Snyder NW, Wei S, Venneti S, et al. Akt-Dependent Metabolic Reprogramming Regulates Tumor Cell Histone Acetylation. Cell Metab (2014) 20(2):306–19. doi: 10.1016/j.cmet.2014.06.004
92. Itkonen HM, Poulose N, Walker S, Mills IG. CDK9 Inhibition Induces a Metabolic Switch That Renders Prostate Cancer Cells Dependent on Fatty Acid Oxidation. Neoplasia (2019) 21(7):713–20. doi: 10.1016/j.neo.2019.05.001
93. Grivell LA. Mitochondrial DNA. Sci Am (1983) 248(3):78–89. doi: 10.1038/scientificamerican0383-78
94. Tabak HF, Grivell LA, Borst P. Transcription of Mitochondrial DNA. CRC Crit Rev Biochem (1983) 14(4):297–317. doi: 10.3109/10409238309102797
95. Attardi G, Schatz G. Biogenesis of Mitochondria. Annu Rev Cell Biol (1988) 4:289–333. doi: 10.1146/annurev.cb.04.110188.001445
96. Costello LC, Franklin RB. Prostate Epithelial Cells Utilize Glucose and Aspartate as the Carbon Sources for Net Citrate Production. Prostate (1989) 15(4):335–42. doi: 10.1002/pros.2990150406
97. Lindberg J, Mills IG, Klevebring D, Liu W, Neiman M, Xu J, et al. The Mitochondrial and Autosomal Mutation Landscapes of Prostate Cancer. Eur Urol (2013) 63(4):702–8. doi: 10.1016/j.eururo.2012.11.053
98. Hopkins JF, Sabelnykova VY, Weischenfeldt J, Simon R, Aguiar JA, Alkallas R, et al. Mitochondrial Mutations Drive Prostate Cancer Aggression. Nat Commun (2017) 8(1):656. doi: 10.1038/s41467-017-00377-y
99. Martinez-Reyes I, Diebold LP, Kong H, Schieber M, Huang H, Hensley CT, et al. TCA Cycle and Mitochondrial Membrane Potential Are Necessary for Diverse Biological Functions. Mol Cell (2016) 61(2):199–209. doi: 10.1016/j.molcel.2015.12.002
100. Petros JA, Baumann AK, Ruiz-Pesini E, Amin M, Sun B, Hall CQ, et al. mtDNA Mutations Increase Tumorigenicity in Prostate Cancer. Proc Natl Acad Sci USA (2005) 102(3):719–24. doi: 10.1073/pnas.0408894102
101. Kinnaird A, Zhao S, Wellen KE, Michelakis ED. Metabolic Control of Epigenetics in Cancer. Nat Rev Cancer (2016) 16(11):694–707. doi: 10.1038/nrc.2016.82
102. Hart GW, Housley MP, Slawson C. Cycling of O-Linked Beta-N-Acetylglucosamine on Nucleocytoplasmic Proteins. Nature (2007) 446(7139):1017–22. doi: 10.1038/nature05815
103. Hart GW, Slawson C, Ramirez-Correa G, Lagerlof O. Cross Talk Between O-GlcNAcylation and Phosphorylation: Roles in Signaling, Transcription, and Chronic Disease. Annu Rev Biochem (2011) 80:825–58. doi: 10.1146/annurev-biochem-060608-102511
104. Itkonen HM, Engedal N, Babaie E, Luhr M, Guldvik I, Minner J, et al. UAP1 Is Overexpressed in Prostate Cancer and Is Protective Against Inhibitors of N-Linked Glycosylation. Oncogene (2015) 34(28):3744–50. doi: 10.1038/onc.2014.307
105. Kaushik AK, Shojaie A, Panzitt K, Sonavane R, Venghatakrishnan H, Manikkam M, et al. Inhibition of the Hexosamine Biosynthetic Pathway Promotes Castration-Resistant Prostate Cancer. Nat Commun (2016) 7:11612. doi: 10.1038/ncomms11612
106. Ferrer CM, Lynch CTP, Sodi VL, Falcone JN, Schwab LP, Peacock DL, et al. O-GlcNAcylation Regulates Cancer Metabolism and Survival Stress Signaling via Regulation of the HIF-1 Pathway. Mol Cell (2014) 54(5):820–31. doi: 10.1016/j.molcel.2014.04.026
107. Itkonen HM, Poulose N, Steele RE, Martin SES, Levine ZG, Duveau DY, et al. Inhibition of O-GlcNAc Transferase Renders Prostate Cancer Cells Dependent on CDK9. Mol Cancer Res (2020) 18(10):1512–21. doi: 10.1158/1541-7786.MCR-20-0339
108. Capotosti F, Guernier S, Lammers F, Waridel P, Cai Y, Jin J, et al. O-GlcNAc Transferase Catalyzes Site-Specific Proteolysis of HCF-1. Cell (2011) 144(3):376–88. doi: 10.1016/j.cell.2010.12.030
109. Levine ZG, Potter SC, Joiner CM, Fei GQ, Nabet B, Sonnett B, et al. Mammalian Cell Proliferation Requires Noncatalytic Functions of O-GlcNAc Transferase. Proc Natl Acad Sci USA (2021) 118(4). doi: 10.1073/pnas.2016778118
110. Method of the Year 2020: Spatially Resolved Transcriptomics. Nat Methods (2021) 18(1):1. doi: 10.1038/s41592-020-01042-x
111. Pertega-Gomes N, Vizcaino JR, Attig J, Jurmeister S, Lopes C, Baltazar F. A Lactate Shuttle System Between Tumour and Stromal Cells Is Associated With Poor Prognosis in Prostate Cancer. BMC Cancer (2014) 14:352. doi: 10.1186/1471-2407-14-352
112. Rappez L, Stadler M, Triana S, Phapale P, Heikenwalder M, Alexandrov T. Spatial Single-Cell Profiling of Intracellular Metabolomes in Situ. bioRxiv (2019) p:510222. doi: 10.1101/510222
113. Danhier P, Banski P, Payen VL, Grasso D, Ippolito L, Sonveaux P, et al. Cancer Metabolism in Space and Time: Beyond the Warburg Effect. Biochim Biophys Acta Bioenerg (2017) 1858(8):556–72. doi: 10.1016/j.bbabio.2017.02.001
114. Morse N, Jamaspishvili T, Simon D, Patel PG, Ren KYM, Oleschuk J, et al. Reliable Identification of Prostate Cancer Using Mass Spectrometry Metabolomic Imaging in Needle Core Biopsies. Lab Invest (2019) 99(10):1561–71. doi: 10.1038/s41374-019-0265-2
115. Sun C, Li T, Song X, Huang L, Zang Q, Xu J, et al. Spatially Resolved Metabolomics to Discover Tumor-Associated Metabolic Alterations. Proc Natl Acad Sci USA (2019) 116(1):52–7. doi: 10.1073/pnas.1808950116
116. Koundouros N, Karali E, Tripp A, Valle A, Inglese P, Perry NJS, et al. Metabolic Fingerprinting Links Oncogenic PIK3CA With Enhanced Arachidonic Acid-Derived Eicosanoids. Cell (2020) 181(7):1596–611.e27. doi: 10.1016/j.cell.2020.05.053
117. Hanel L, Kwiatkowski M, Heikaus L, Schluter H. Mass Spectrometry-Based Intraoperative Tumor Diagnostics. Future Sci OA (2019) 5(3):FSO373. doi: 10.4155/fsoa-2018-0087
118. Tataru OS, Vartolomei MD, Rassweiler JJ, Virgil O, Lucarelli G, Porpiglia F, et al. Artificial Intelligence and Machine Learning in Prostate Cancer Patient Management-Current Trends and Future Perspectives. Diagn (Basel) (2021) 11(2). doi: 10.3390/diagnostics11020354
119. Jambor I, Falagario U, Ratnani P, Perez IM, Demir K, Merisaari H, et al. Prediction of Biochemical Recurrence in Prostate Cancer Patients Who Underwent Prostatectomy Using Routine Clinical Prostate Multiparametric MRI and Decipher Genomic Score. J Magn Reson Imaging (2020) 51(4):1075–85. doi: 10.1002/jmri.26928
Keywords: prostate cancer, metabolism, epigenetics, mitochondria, TCA cycle
Citation: Singh R and Mills IG (2021) The Interplay Between Prostate Cancer Genomics, Metabolism, and the Epigenome: Perspectives and Future Prospects. Front. Oncol. 11:704353. doi: 10.3389/fonc.2021.704353
Received: 02 May 2021; Accepted: 31 August 2021;
Published: 29 September 2021.
Edited by:
Marianna Kruithof-de Julio, University of Bern, SwitzerlandReviewed by:
Anthony Joshua, University Health Network (UHN), CanadaUgo Giovanni Falagario, University of Foggia, Italy
Copyright © 2021 Singh and Mills. This is an open-access article distributed under the terms of the Creative Commons Attribution License (CC BY). The use, distribution or reproduction in other forums is permitted, provided the original author(s) and the copyright owner(s) are credited and that the original publication in this journal is cited, in accordance with accepted academic practice. No use, distribution or reproduction is permitted which does not comply with these terms.
*Correspondence: Reema Singh, reema.singh@nds.ox.ac.uk