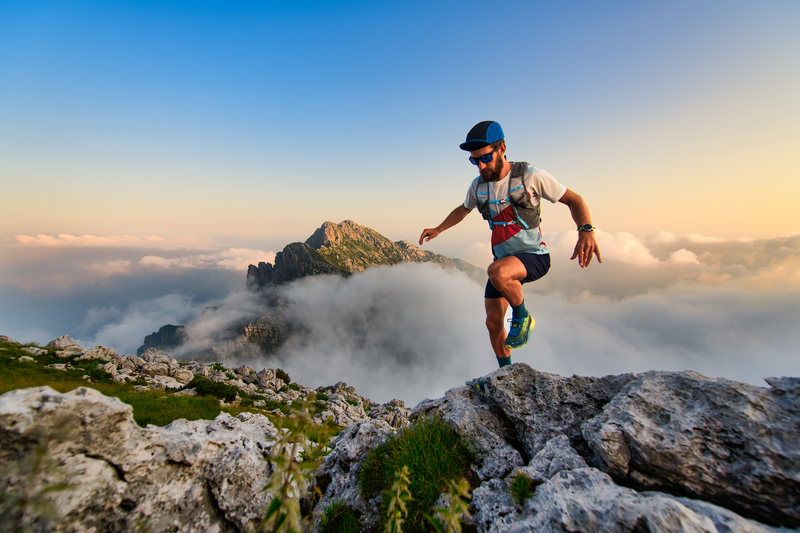
94% of researchers rate our articles as excellent or good
Learn more about the work of our research integrity team to safeguard the quality of each article we publish.
Find out more
ORIGINAL RESEARCH article
Front. Oncol. , 12 July 2021
Sec. Cancer Imaging and Image-directed Interventions
Volume 11 - 2021 | https://doi.org/10.3389/fonc.2021.698537
This article is part of the Research Topic Image-guided Particle Therapy View all 14 articles
Purpose: To integrate dose-averaged linear energy transfer (LETd) into spot-scanning proton arc therapy (SPArc) optimization and to explore its feasibility and potential clinical benefits.
Methods: An open-source proton planning platform (OpenREGGUI) has been modified to incorporate LETd into optimization for both SPArc and multi-beam intensity-modulated proton therapy (IMPT) treatment planning. SPArc and multi-beam IMPT plans with different beam configurations for a prostate patient were generated to investigate the feasibility of LETd-based optimization using SPArc in terms of spatial LETd distribution and plan delivery efficiency. One liver and one brain case were studied to further evaluate the advantages of SPArc over multi-beam IMPT.
Results: With similar dose distributions, the efficacy of spatially optimizing LETd distributions improves with increasing number of beams. Compared with multi-beam IMPT plans, SPArc plans show substantial improvement in LETd distributions while maintaining similar delivery efficiency. Specifically, for the liver case, the average LETd in the GTV was increased by 124% for the SPArc plan, and only 9.6% for the 2-beam IMPT plan compared with the 2-beam non-LETd optimized IMPT plan. In case of LET optimization for the brain case, the SPArc plan could effectively increase the average LETd in the CTV and decrease the values in the critical structures while smaller improvement was observed in 3-beam IMPT plans.
Conclusion: This work demonstrates the feasibility and significant advantages of using SPArc for LETd-based optimization, which could maximize the LETd distribution wherever is desired inside the target and averts the high LETd away from the adjacent critical organs-at-risk.
In the status quo of clinical proton therapy, most centers use a presumed constant relative biological effectiveness (RBE) value of 1.1 (1, 2) regardless of the dose, linear energy transfer (LET), physiological and biological factors, and clinical endpoint (3, 4). Historically, such RBE value was chosen conservatively for tumor control based on the in vitro and in vivo measurements at the center of spread-out Bragg Peak (SOBP) (2, 3). Recent experiments (5) and clinical studies (6) show that RBE value may increase towards the distal dose fall-off of SOBP as the LET increases, and this could be up to the magnitude of 1.7 (3). Hence, more than expected rate of toxicities may be seen if the distal end of the Bragg peaks ends up in the adjacent critical structures and such higher rate of toxicities, including necrosis have been reported in multiple studies (7–9). Therefore, being able to manipulate the location of high LET is very helpful to further improve modern proton beam therapy.
Nevertheless, in the era of traditional passive-scattering proton therapy (PSPT) delivery technique, the high LET region is inevitably located at the distal end of each beam and it is impossible to modulate its distribution. To avoid any overlapping high LET regions with the abutting critical organs, it is common to use the beam angles without aiming directly toward those organs. Fortunately, in the past few years, the pencil beam scanning (PBS) technique has emerged and quickly been adopted by new proton centers as the most advanced proton beam delivery technology (10, 11). Intensity-modulated proton therapy (IMPT), based on PBS, optimizes the intensities of individual spot from different energy layers. Compared with the PSPT, IMPT offers not only a more conformal radiation dose (12–14), but also has the potential to modify the LET distribution by incorporating the LET optimization into the planning process (4, 15–21). However, the ability of IMPT to spatially place the LET value is limited by the number of beam angles (22, 23) and thus not clinically feasible given the prolonged PBS treatment delivery time.
Such obstacles could be overcome by using spot-scanning proton arc therapy (SPArc) which is a novel spot scanning delivery technique that can deliver a treatment plan in an arc mode (24). SPArc has manifested significant advantages over the multi-beam IMPT (25–28) to improve dose distributions and delivery. A prototype of SPArc delivery has proven its feasibility on a state-of-the-art proton therapy system (29). Since the SPArc plans are delivered from hundreds of beam angles selected from a smart energy and spot selection algorithm (24), we hypothesize that with such increased degrees of freedom for optimization, SPArc can further improve the LET distribution while maintaining the delivery efficiency. Thus, we develop a novel LET-based SPArc optimization algorithm and explore its potential to improve the spatial LET distribution.
The LETd-based SPArc optimization engine was implemented based on an open-source proton planning platform (OpenREGGUI) (30). This platform uses a fast Monte Carlo dose calculation engine (MCSquare) (31–33) and an optimizer (MIROpt) (34) for dose-based multi-beam IMPT planning. Such platform was modified to incorporate the LETd-based objective functions (e.g., minimum and maximum LETd) into optimization and to iteratively generate the SPArc plans (24, 29). The details of LETd-based SPArc planning implementation is shown in Figure 1 which consists of (1) the planner defines the arc start and stop angles, (2) the engine coarsely samples the initial beams (3) the MCSquare calculates the voxel dose and LETd values from each spot, (4) the optimizer finds a solution by iteratively calculating the dose and LETd and minimizing the dose and LETd based objective functions for target volume and organs-at-risk (OARs), (5) iteratively increases the beam sampling frequency and reduces the energy layers by repeating step (3) and (4) until desired sampling frequency is reached, (6) and finally, perform deliverable optimization by specifying the minimum MU (0.02 MU) per spot. The details of the LET implementation are described as follow.
In MCSquare calculation, the ‘stopping power’ method (35) was selected, in which LETd was scored by dose-weighted average of the stopping power of particles through each voxel (36). Secondary particles were handled by specifying the options in the configuration file as: secondary photons and neutrons are not taken into account while the secondary electrons are considered as locally absorbed. Secondary protons, deuterons, and alpha’s are simulated for the dose and LETd. The calculated dose and LET values from each spot j projected to voxel i are expressed as (Dij and LETij). The dose and dose-averaged LET value for voxel i can be calculated from the intensity (monitor unit) of the spot j (ωj) as follow:
For the optimization, we used a standard quadratic objective function to control both dose and LETd distributions:
where p denotes the penalty weights, D0 and LET0 correspond to the target or constraint dose and LET value respectively. The Heaviside step function H(Di – D0) is defined as convention (i.e., equals to unity if Di > D0, otherwise equals to zero). Dose-based, dose volume based, and LET-based objective functions are implemented and used for this study.
All the SPArc and multi-beam IMPT plans (without arc sequencing part in Figure 1) were generated using this optimization engine with similar parameters (e.g., 104 particles per spot for Dij, LETij matrix calculation, 108 particles for final dose calculation to ensure the plan is within 1% statistical uncertainties for the dose in the target volume (37), and same optimization criteria). For each patient, the IMPT plan was generated first to achieve an optimized dose distribution to the target volume and OARs without taking LETd distribution into account. Based on those dose objective functions, IMPT plan was then generated by adding LETd objectives of the target and OARs in the optimization. The relative weights between dose and LETd objectives were further adjusted to maintain similar or superior dose distribution while maximizing and minimizing the average LETd values in the target volume and OARs. In terms of SPArc planning, the plans initiated from a coarse sampling frequency of 20 degree and achieved 2.5 degree final sampling frequency using the similar objective functions to the IMPT plans with LETd optimization.
To quantitatively investigate the effect of the LETd distribution with the relationship of beam number, a prostate patient was selected given its easy anatomical structures. A full arc SPArc and multi-beam IMPT plans with 2, 4, 6, and 8 equally spaced beams were generated to achieve similar clinical target volume (CTV, 134 cc) coverage (78 Gy in 39 fractions, using RBE of 1.1) and high dose sparing of rectum and bladder while maximizing the LETd value using similar objective functions inside the CTV. Urethra was not considered as an avoidance structure for the simplicity of the analysis. However any OAR can be used as an avoidance structure in optimization although this will result in a more complicated and longer calculation process. The dose, LETd, corresponding dose-volume histograms (DVHs) and LETd-volume histograms (LVHs) of all plans were analyzed and compared with the 2-beam IMPT plan without LETd optimization (2B w/o). To evaluate the treatment delivery efficiency among different plans, the delivery time was simulated based on a full gantry geometry with a rotation speed of one rotation per minute, spot switching time of 2 milliseconds, and energy-layer-switching-time of 0.6 seconds (24).
To further quantify the relationship between high LETd concentration and the size of LETd boost volume in SPArc optimization, different virtual simultaneously integrated boost (SIB) volumes (77.9, 39.9, 16.5, 4.5, and 0.3 ccs) were generated by shrinking the CTV at a step size of 0.5 cm. Multiple SPArc plans were generated to provide similar CTV coverage while maximizing the high LETd concentrating in the SIB volumes using the similar plan parameters such as arc trajectory, sampling frequency, as well as target objective functions and weights.
A liver and a brain case were then selected to compare the resulted LETd optimization between SPArc and multi-beam IMPT. For the liver case, a partial arc from 160 – 40 degree (IEC 61217) was used to prescribe 75 Gy (constant RBE of 1.1) in 25 fractions (38) to the CTV, while minimizing the mean dose to the normal liver, and maximizing the LETd value in the gross tumor volume (GTV). For the brain case, the full arc was used to optimize a uniform dose to the CTV (54 Gy in 30 fractions, constant RBE of 1.1) (39) and minimize the dose to the surrounding OARs, while maximizing the LETd value in CTV and minimizing the LETd value to the OARs. The multi-beam IMPT plans using the clinical beam angles (two beams for the liver case, and three beams for the brain cases) were also re-optimized using the same platform with and without LETd optimization. The dose, LETd, corresponding DVHs and LVHs were evaluated for all plans.
Dose and LETd distributions for a 2-beam IMPT plan without LETd optimization, and IMPT plans (2, 4, 6, 8 beam angles) and SPArc plans with LETd optimization are shown in Figure 2. This comparison shows the power of SPArc to concentrate the high LETd in the desired area of target volume. Figures 3A, B display the corresponding DVHs for CTV, rectum, and bladder for all plans. The corresponding LVHs for CTV are also shown in Figure 3C. With similar RBE 1.1 dose distributions in terms of target coverage and high dose OARs sparings, the average LETd in the target increases with the number of beams used. Compared to multi-beam IMPT plans, SPArc has a better capability of spatially centralizing the LETd distributions in the target while maintaining the delivery efficiency. Specifically, with LETd optimization, the average value of LETd in the target was 4.38, 4.65, 4.85, 4.85, and 5.06 keV/μm for IMPT plans of 2, 4, 6, 8 beams and SPArc plans, respectively. Compared with the 2-beam non-LETd optimized plan, the corresponding increase of LETd value was 21%, 29%, 34%, 34%, and 40% for the IMPT plans (2, 4, 6, 8 beams) and SPArc plans respectively. The estimated delivery time for the IMPT plans (2, 4, 6, 8 beams) and SPArc plans was 96, 150, 171, 188, and 125 seconds respectively. Hence, SPArc can enhance the LETd distribution in the target volume more efficiently.
Figure 2 The dose (upper row) and the LETd (lower row) distributions for 2 beams without LETd based optimization (2Bw/o, A, G), and with LETd based optimization for 2 (2B, B, H), 4 (4B, C, I), 6 (6B, D, J), 8 (8B, E, K) beams and SPArc (F, L) for a prostate case.
Figure 3 The dose volume histograms (DVHs) (A, B) and LETd volume histograms (LVHs) (C) for 2Bw/o and for 2, 4, 6, 8 beams and SPArc with LETd based optimization.
Moreover, the ability of SPArc to concentrate the LETd depends on the volume as shown in Figure 4. The average LETd value in the SIB volume for the prostate case could increase up to 8.5 keV/um as the size of the SIB volume decreases while maintaining similar dose distributions.
Figure 4 The relation with average achievable LETd versus volume using SPArc while keeping similar dose to the target volume for the prostate case.
To validate the capability of SPArc to optimize LET distribution, other clinical scenarios such as liver and brain cases were examined. The dose, DVHs, and LVHs for the liver case generated using two beams without LETd optimization, with LETd optimization, and SPArc are shown in Figure 5. With similar target coverage, the SPArc plans reduced the mean dose of normal liver by 1.4 Gy (RBE 1.1), and 1.5 Gy (RBE 1.1) when compared with the 2-beam IMPT plan without and with LETd optimization respectively. Moreover, the SPArc could effectively escalate the high LETd value in the GTV. Specifically, the average value of LETd in the GTV for the liver case was 4.88 and 2.39 keV/μm for the SPArc and 2-beam IMPT plans with LETd optimization respectively. Compared with the 2-beam non-LETd optimized plan, the corresponding increase in LETd was 124% for SPArc plan, and only 9.6% for the IMPT plan given the limited number of beam angles.
Figure 5 The dose (first row) and LETd (second row) distributions, the DVHs (G) and LVHs (H) of 2Bw/o (A, D), 2B (B, E), and SPArc (C, F) plans for the liver patient.
Similar results were noted in simulation of the brain case, where SPArc with LETd optimization could effectively maximize the LETd enhancement to the target volume while restricting the high LETd away from the OARs. Specifically, the average value of LETd for the SPArc plan was increased by 29% (4.03 vs. 3.13 keV/μm) for CTV, and was decreased by 22% (2.14 vs. 2.74 keV/μm), 30% (2.43 vs. 3.45 keV/μm), 28% (2.96 vs. 4.09 keV/μm), and 17% (2.66 vs. 3.22 keV/μm) for brainstem, chiasm, left, and right optical nerves, respectively, compared with the 3-beam non-LETd optimized plan. In contrast, the corresponding improvements were only 4%, 12%, 22%, 21%, and -3% for 3-beam IMPT plan compared with the 3-beam non-LETd optimized plan (Figures 6, 7).
Figure 6 The dose (upper row) and LETd (lower row) distributions of 3Bw/o (A, D), 3B (B, E), and SPArc (C, F) plans for the brain patient.
This is the first study to quantitatively evaluate the feasibility of SPArc to spatially optimize LETd distributions using the fully LETd incorporated SPArc optimization engine. Our results demonstrate that SPArc has the great capability to concentrate the high LETd inside the target volume while restricting it from the OARs. Nevertheless, as the number of beam angles increases, IMPT plans may be able to achieve similar plan quality as SPArc, however this is not feasible in clinical practice due to its prolonged treatment delivery time. Several groups (40, 41) have proposed to shorten the delivery time by filtering the energy layers for IMPT plans. Such procedure is still limited and not preferable especially for multi-room proton center due to room switching time between beams (24). Conversely, SPArc has more degrees of freedom for delivering hundreds of beamlets along the arc trajectory therefore to effectively optimize the dose and LETd distributions simultaneously without sacrificing the delivery efficiency.
More importantly, SPArc has demonstrated its capability of elevating the LETd value inside the target. The level of elevation is dependent on the target volume (Figure 4). For small target volumes (i.e., less than 5 cc), the average LETd in the target volume could reach values higher than 7 keV/um. Compared to the average mid-SOBP LETd value of 2-3 keV/um (3), the increment can be up to 250%. However, the magnitude and the conformity of LETd concentration in the target volume depends on the target size, its location and more importantly on adjacent critical normal structures and their dose and LET constraints. For instance, the maximum average LETd achieved in the GTV was compromised in the liver case (Figure 5) as only a partial arc could be used due to the location of the target. Furthermore, the irregular shape and complex geometry of the target volume and OARs can affect the distribution, conformity and the magnitude of LETd in the target volume shown in the brain case (Figure 6) as the average LETd value could only reach up to 4.03 keV/um. In order to understand the potentials and limitations of the LETd distribution and its falloff via SPArc, more studies on different anatomical sites and patient geometries are necessary and are the mainstay of our future efforts.
This study incorporated LETd optimization engine into the biological treatment planning to achieve a similar dose distribution with RBE 1.1 as used in current clinical practice. A more comprehensive biological optimization engine based on a variable RBE model could be extended from the current framework by incorporating dose, physiological and biological factors into the optimization. Thus far, none of the variable proton RBE models has been implemented into routine clinical practice due to the discrepancies between model calculations and experimental data (42, 43). In contrast to the physical parameters in the current RBE model, LET can be supported by all the variable RBE models (5), in which RBE value varies with LET values. Therefore, using this approach in this study which is maintaining similar dose (RBE 1.1) while spatially optimizing LETd distribution, could be possibly clinically adopted to improve the patient outcome.
Moreover, it is important to mention that robust optimization was not incorporated into this LETd-based SPArc treatment planning due to the slow calculation speed and extra memory allocation. We do recognize that the dose and LETd distributions could get deteriorated from the uncertainties. However, the previous study (17) have demonstrated the feasibility of improving LETd distributions by integrating LETd based optimization with robust optimization in IMPT plans. With the availability of better calculation models and computer hardware, we could integrate the robustness into LETd-based SPArc planning in our future studies.
This is the first fully LETd incorporated SPArc optimization algorithm and platform which has the capability to spatially optimize LETd. Our results demonstrate that SPArc can take advantage of arc trajectory to maximize the LETd concentration to anywhere in the target volume, and to avoid the high LETd from the OARs, while maintaining similar delivery efficiency. This technique will not only address one of the challenges in proton therapy which is the risk of toxicity associated with the LET uncertainty of the Bragg peak distal edge but also could provide the means to dose escalation via LET optimization in the target volume while sparing OARs.
The raw data supporting the conclusions of this article will be made available by the authors, without undue reservation.
The studies involving human participants were reviewed and approved by Beaumont Health Institutional Review Board. Written informed consent for participation was not required for this study in accordance with the national legislation and the institutional requirements.
XL designed the study, performed the data acquisition and analysis, wrote the manuscript. XD, WZ, and GL assisted in designing the study and revising the manuscript. GJ, KS, and AB-M provided intensive feedback to improve the algorithm. DY and CS provided physics and clinical support. PK helped to design the study, provided clinical inputs, and revised the manuscript. All authors contributed to the article and approved the submitted version.
XD and XL received the fund from Ion Beam Applications. The funder was not involved in the study design, collection, analysis, interpretation of data, the writing of this article or the decision to submit it for publication.
XD, XL, and DY have a patent related to the Particle Arc Therapy (WO2017156419). GJ was employed by Ion Beam Applications SA.
The remaining authors declare that the research was conducted in the absence of any commercial or financial relationships that could be construed as a potential conflict of interest.
LETd, dose-averaged linear energy transfer; SPArc, spot-scanning proton arc therapy; MPT, intensity-modulated proton therapy; RBE, relative biological effectiveness; SOBP, spread-out Bragg Peak; SPT, passive-scattering proton therapy; PBS, pencil beam scanning; OARs, organs-at-risk; DVH, dose-volume histogram; LVH, LETd-volume histogram; 2B w/o, 2-beam IMPT plan without LETd optimization; SIB, simultaneously integrated boost; GTV, gross tumor volume.
1. Newhauser W. International Commission on Radiation Units and Measurements Report 78: Prescribing, Recording and Reporting Proton-Beam Therapy. Radiat Prot Dosimetry (2009) 133(1):60–2. doi: 10.1093/rpd/ncp005
2. Paganetti H, Niemierko A, Ancukiewicz M, Gerweck LE, Goitein M, Loeffler JS, et al. Relative Biological Effectiveness (RBE) Values for Proton Beam Therapy. Int J Radiat Oncol Biol Phys (2002) 53(2):407–21. doi: 10.1016/S0360-3016(02)02754-2
3. Paganetti H. Relative Biological Effectiveness (RBE) Values for Proton Beam Therapy. Variations as a Function of Biological Endpoint, Dose, and Linear Energy Transfer. Phys Med Biol (2014) 59(22):R419–72. doi: 10.1088/0031-9155/59/22/R419
4. Unkelbach J, Botas P, Giantsoudi D, Gorissen and H. Paganetti BL. Reoptimization of Intensity Modulated Proton Therapy Plans Based on Linear Energy Transfer. Int J Radiat Oncol Biol Phys (2016) 96(5):1097–106. doi: 10.1016/j.ijrobp.2016.08.038
5. Guan F, Bronk L, Titt U, Lin SH, Mirkovic D, Kerr MD, et al. Spatial Mapping of the Biologic Effectiveness of Scanned Particle Beams: Towards Biologically Optimized Particle Therapy. Sci Rep (2015) 5:9850. doi: 10.1038/srep09850
6. Peeler CR, Mirkovic D, Titt U, Blanchard P, Gunther JR, Mahajan A, et al. Clinical Evidence of Variable Proton Biological Effectiveness in Pediatric Patients Treated for Ependymoma. Radiother Oncol (2016) 121(3):395–401. doi: 10.1016/j.radonc.2016.11.001
7. Harrabi S, Gudden C, Adeberg S, Bougatf N, Haberer T, Rieken S, et al. OC-0514: Radiation Necrosis After Proton Beam Therapy - When and Where Does It Happen? Radiother Oncol (2017) 123:S271. doi: 10.1016/S0167-8140(17)30954-4
8. Zhang Y, Huo W, Adams JA, Sanford NN, Lam MB, Lu Y, et al. Temporal Lobe Necrosis After Proton for Nasopharyngeal Carcinoma: Predictive Factors and Clinical RBE Estimation. Int J Radiat Oncol • Biol • Phys (2017) 99(2):E386. doi: 10.1016/j.ijrobp.2017.06.1526
9. Merchant TE, Hua CH, Sabin ND, Ezell SE, Madey MA, Wu S, et al. Necrosis, Vasculopathy, and Neurological Complications After Proton Therapy for Childhood Craniopharyngioma: Results From a Prospective Trial and a Photon Cohort Comparison. Int J Radiat Oncol • Biol • Phys (2016) 96(2):S120–1. doi: 10.1016/j.ijrobp.2016.06.294
10. Lomax A. Intensity Modulation Methods for Proton Radiotherapy. Phys Med Biol (1999) 44(1):185–205. doi: 10.1088/0031-9155/44/1/014
11. Lomax AJ, Boehringer T, Coray A, Egger E, Goitein G, Grossmann M, et al. Intensity Modulated Proton Therapy: A Clinical Example. Med Phys (2001) 28(3):317–24. doi: 10.1118/1.1350587
12. Zhang XD, Li YP, Pan XN, Li XQ, Mohan R, Komaki R, et al. Intensity-Modulated Proton Therapy Reduces the Dose to Normal Tissue Compared With Intensity-Modulated Radiation Therapy or Passive Scattering Proton Therapy and Enables Individualized Radical Radiotherapy for Extensive Stage Iiib Non-Small-Cell Lung Cancer: A Virtual Clinical Study. Int J Radiat Oncol Biol Phys (2010) 77(2):357–66. doi: 10.1016/j.ijrobp.2009.04.028
13. Liu W, Frank SJ, Li X, Li Y, Park PC, Dong L, et al. Effectiveness of Robust Optimization in Intensity-Modulated Proton Therapy Planning for Head and Neck Cancers. Med Phys (2013) 40(5):051711. doi: 10.1118/1.4801899
14. Liu W, Li YP, Li XQ, Cao WH, Zhang XD, et al. Influence of Robust Optimization in Intensity-Modulated Proton Therapy With Different Dose Delivery Techniques. Med Phys (2012) 39(6):3089. doi: 10.1118/1.4711909
15. Frese MC, Wilkens JJ, Huber PE, Jensen AD, Oelfke U, Taheri-Kadkhoda Z. Application of Constant vs. Variable Relative Biological Effectiveness in Treatment Planning of Intensity-Modulated Proton Therapy. Int J Radiat Oncol Biol Phys (2011) 79(1):80–8. doi: 10.1016/j.ijrobp.2009.10.022
16. Wilkens JJ, Oelfke U. Optimization of Radiobiological Effects in Intensity Modulated Proton Therapy. Med Phys (2005) 32(2):455–65. doi: 10.1118/1.1851925
17. An Y, Shan J, Patel SH, Wong W, Schild SE, Ding X, et al. Robust Intensity-Modulated Proton Therapy to Reduce High Linear Energy Transfer in Organs at Risk. Med Phys (2017) 44(12):6138–47. doi: 10.1002/mp.12610
18. Grassberger C, Paganetti H. Elevated LET Components in Clinical Proton Beams. Phys Med Biol (2011) 56(20):6677–91. doi: 10.1088/0031-9155/56/20/011
19. Wan Chan Tseung HS, Ma J, Kreofsky CR, Ma DJ, Beltran C. Clinically Applicable Monte Carlo-Based Biological Dose Optimization for the Treatment of Head and Neck Cancers With Spot-Scanning Proton Therapy. Int J Radiat Oncol Biol Phys (2016) 95(5):1535–43. doi: 10.1016/j.ijrobp.2016.03.041
20. Cao W, Khabazian A, Yepes PP, Lim G, Poenisch F, Grosshans DR, et al. Linear Energy Transfer Incorporated Intensity Modulated Proton Therapy Optimization. Phys Med Biol (2017) 63(1):015013. doi: 10.1088/1361-6560/aa9a2e
21. Grassberger C, Trofimov A, Lomax A, Paganetti H. Variations in Linear Energy Transfer Within Clinical Proton Therapy Fields and the Potential for Biological Treatment Planning. Int J Radiat Oncol Biol Phys (2011) 80(5):1559–66. doi: 10.1016/j.ijrobp.2010.10.027
22. Fager M, Toma-Dasu I, Kirk M, Dolney D, Diffenderfer ES, Vapiwala N, et al. Linear Energy Transfer Painting With Proton Therapy: A Means of Reducing Radiation Doses With Equivalent Clinical Effectiveness. Int J Radiat Oncol Biol Phys (2015) 91(5):1057–64. doi: 10.1016/j.ijrobp.2014.12.049
23. Toussaint L, Indelicato DJ, Holgersen KS, Petersen JBB, Stokkevag CH, Lassen- Ramshad Y, et al. Towards Proton Arc Therapy: Physical and Biologically Equivalent Doses With Increasing Number of Beams in Pediatric Brain Irradiation. Acta Oncol (2019) 58(10):1451–6. doi: 10.1080/0284186X.2019.1639823
24. Ding X, Li X, Zhang JM, Kabolizadeh P, Stevens C, Yan D. Spot-Scanning Proton Arc (SPArc) Therapy: The First Robust and Delivery-Efficient Spot-Scanning Proton Arc Therapy. Int J Radiat Oncol • Biol • Phys (2016) 96(5):1107–16. doi: 10.1016/j.ijrobp.2016.08.049
25. Ding X, Li X, Qin A, Zhou J, Yan D, Chen P, et al. Redefine the Role of Range Shifter in Treating Bilateral Head and Neck Cancer in the Era of Intensity Modulated Proton Therapy. J Appl Clin Med Phys (2018) 19(5):749–55. doi: 10.1002/acm2.12416
26. Ding X, Li X, Qin A, Zhou J, Yan D, Stevens C, et al. Have We Reached Proton Beam Therapy Dosimetric Limitations? - A Novel Robust, Delivery-Efficient and Continuous Spot-Scanning Proton Arc (SPArc) Therapy Is to Improve the Dosimetric Outcome in Treating Prostate Cancer. Acta Oncol (2018) 57(3):435–7. doi: 10.1080/0284186X.2017.1358463
27. Ding X, Zhou J, Li X, Blas K, Liu G, Wang Y, et al. Improving Dosimetric Outcome for Hippocampus and Cochlea Sparing Whole Brain Radiotherapy Using Spot-Scanning Proton Arc Therapy. Acta Oncol (2019) 1–8. doi: 10.1080/0284186X.2018.1555374
28. Li X, Kabolizadeh P, Yan D, Qin A, Zhou J, Hong Y, et al. Improve Dosimetric Outcome in Stage III Non-Small-Cell Lung Cancer Treatment Using Spot-Scanning Proton Arc (SPArc) Therapy. Radiat Oncol (2018) 13(1):35. doi: 10.1186/s13014-018-0981-6
29. Li X, Liu G, Janssens G, De Wilde O, Bossier V, Lerot X, et al. The First Prototype of Spot-Scanning Proton Arc Treatment Delivery. Radiother Oncol (2019) 137:130–6. doi: 10.1016/j.radonc.2019.04.032
30. Available at: https://openreggui.org/.
31. Huang ,S, Kang M, Souris K, Ainsley C, Solberg TD, McDonough JE, et al. Validation and Clinical Implementation of an Accurate Monte Carlo Code for Pencil Beam Scanning Proton Therapy. J Appl Clin Med Phys (2018) 19(5):558–72. doi: 10.1002/acm2.12420
32. Souris K, Lee JA, Sterpin E. Fast Multipurpose Monte Carlo Simulation for Proton Therapy Using Multi- and Many-Core CPU Architectures. Med Phys (2016) 43(4):1700. doi: 10.1118/1.4943377
33. Sorriaux J, Testa M, Paganetti H, Orban de Xivry J, Lee JA, Traneus E, et al. Experimental Assessment of Proton Dose Calculation Accuracy in Inhomogeneous Media. Phys Med (2017) 38:10–5. doi: 10.1016/j.ejmp.2017.04.020
34. Barragan Montero AM, Souris K, Sanchez-Parcerisa D, Sterpin E, Lee JA. Performance of a Hybrid Monte Carlo-Pencil Beam Dose Algorithm for Proton Therapy Inverse Planning. Med Phys (2018) 45(2):846–62. doi: 10.1002/mp.12688
35. Wagenaar D, Tran LT, Meijers A, Marmitt GG, Souris K, Bolst D, et al. Validation of Linear Energy Transfer Computed in a Monte Carlo Dose Engine of a Commercial Treatment Planning System. Phys Med Biol (2020) 65(2):025006. doi: 10.1088/1361-6560/ab5e97
36. Cortes-Giraldo MA, Carabe A. A Critical Study of Different Monte Carlo Scoring Methods of Dose Average Linear-Energy-Transfer Maps Calculated in Voxelized Geometries Irradiated With Clinical Proton Beams. Phys Med Biol (2015) 60(7):2645–69. doi: 10.1088/0031-9155/60/7/2645
37. Bueno M, Paganetti H, Duch MA, Schuemann J. An Algorithm to Assess the Need for Clinical Monte Carlo Dose Calculation for Small Proton Therapy Fields Based on Quantification of Tissue Heterogeneity. Med Phys (2013) 40(8):081704. doi: 10.1118/1.4812682
38. Crane CH, Koay EJ. Solutions That Enable Ablative Radiotherapy for Large Liver Tumors: Fractionated Dose Painting, Simultaneous Integrated Protection, Motion Management, and Computed Tomography Image Guidance. Cancer (2016) 122(13):1974–86. doi: 10.1002/cncr.29878
39. Fagundes MA, Hug EB, Liebsch NJ, Daly W, Efird J, Munzenrider JE. Radiation Therapy for Chordomas of the Base of Skull and Cervical Spine: Patterns of Failure and Outcome After Relapse. Int J Radiat Oncol Biol Phys (1995) 33(3):579–84. doi: 10.1016/0360-3016(95)02014-3
40. Cao W, Lim G, Liao L, Li Y, Jiang S, Li X, et al. Proton Energy Optimization and Reduction for Intensity-Modulated Proton Therapy. Phys Med Biol (2014) 59(21):6341–54. doi: 10.1088/0031-9155/59/21/6341
41. van de Water S, Kooy HM, Heijmen BJ, Hoogeman MS. Shortening Delivery Times of Intensity Modulated Proton Therapy by Reducing Proton Energy Layers During Treatment Plan Optimization. Int J Radiat Oncol Biol Phys (2015) 92(2):460–8. doi: 10.1016/j.ijrobp.2015.01.031
42. Polster L, Schuemann J, Rinaldi I, Burigo L, McNamara AL, Stewart RD, et al. Extension of TOPAS for the Simulation of Proton Radiation Effects Considering Molecular and Cellular Endpoints. Phys Med Biol (2015) 60(13):5053–70. doi: 10.1088/0031-9155/60/13/5053
Keywords: proton therapy, arc therapy, linear energy transfer, plan optimization, spot scanning
Citation: Li X, Ding X, Zheng W, Liu G, Janssens G, Souris K, Barragán-Montero AM, Yan D, Stevens C and Kabolizadeh P (2021) Linear Energy Transfer Incorporated Spot-Scanning Proton Arc Therapy Optimization: A Feasibility Study. Front. Oncol. 11:698537. doi: 10.3389/fonc.2021.698537
Received: 21 April 2021; Accepted: 22 June 2021;
Published: 12 July 2021.
Edited by:
Heng Li, Johns Hopkins Medicine, United StatesReviewed by:
Wenhua Cao, University of Texas MD Anderson Cancer Center, United StatesCopyright © 2021 Li, Ding, Zheng, Liu, Janssens, Souris, Barragán-Montero, Yan, Stevens and Kabolizadeh. This is an open-access article distributed under the terms of the Creative Commons Attribution License (CC BY). The use, distribution or reproduction in other forums is permitted, provided the original author(s) and the copyright owner(s) are credited and that the original publication in this journal is cited, in accordance with accepted academic practice. No use, distribution or reproduction is permitted which does not comply with these terms.
*Correspondence: Xiaoqiang Li, WGlhb3FpYW5nLkxpQGJlYXVtb250Lm9yZw==
Disclaimer: All claims expressed in this article are solely those of the authors and do not necessarily represent those of their affiliated organizations, or those of the publisher, the editors and the reviewers. Any product that may be evaluated in this article or claim that may be made by its manufacturer is not guaranteed or endorsed by the publisher.
Research integrity at Frontiers
Learn more about the work of our research integrity team to safeguard the quality of each article we publish.