- 1School of Stomatology, Qingdao University, Qingdao, China
- 2Department of Oral and Maxillofacial Surgery, Key Laboratory of Oral Clinical Medicine, The Affiliated Hospital of Qingdao University, Qingdao, China
- 3Department of Endodontics, Key Laboratory of Oral Clinical Medicine, The Affiliated Hospital of Qingdao University, Qingdao, China
Introduction: Circular RNAs (CircRNAs), an emerging non-coding RNA, have been demonstrated to be involved in tumorigenesis, metastasis, and cancer progression, and could represent novel potential biomarkers for diagnosing oral squamous cell carcinoma (OSCC). However, no meta-analysis has investigated the diagnostic role of circRNAs in OSCC. Hence, to investigate whether circRNAs could serve as specific biomarkers for OSCC, the present systematic review and meta-analysis evaluated the diagnostic efficiency of circRNAs in patients with OSCC.
Materials and Methods: A thorough search of online databases (Pubmed, Web of Science, Embase, and the Cochrane Library) was conducted to collect relevant studies up to March 30th, 2021. All eligible studies were case-control studies. The quality of each study was evaluated by the Quality Assessment of Diagnostic Accuracy Studies-2 (QUADAS-2) tool. STATA (version 15.1) and Review Manager (version 5.4) were employed to conduct the meta-analysis, and the PRISMA statement was adopted in this study.
Results: A total of 16 studies were included in the meta-analysis, with five studies on upregulated circRNAs, and 11 on downregulated circRNAs. The enrolled studies that met our eligibility criteria all derived from China. The pooled sensitivity (SEN), specificity (SPE), diagnostic odds ratio (DOR), positive likelihood ratio (PLR), negative likelihood ratio (NLR), and the area under receiver operating characteristics curve (AUC) with the 95% confidence intervals (95% CIs) were 0.74 (0.69–0.79), 0.79 (0.73–0.84), 10.74 (7.81–14.77), 3.50 (2.78–4.45), 0.33 (0.27–0.39) and 0.83 (0.79–0.86), respectively. The subgroup analysis demonstrated that serum, plasma, and saliva specimens had a better diagnostic performance than tissue samples, with a high value of sensitivity, specificity, DOR, and AUC values. The results also showed that the subgroups of upregulated circRNAs and a sample size of ≥100 manifested higher specificity, DOR, and AUC for cancer detection than downregulated circRNAs and a sample size of < 100.
Conclusions: A strong association was demonstrated between the dysregulated expression of circRNAs and the diagnosis of OSCC. Hence, circRNAs have the potential to function as promising biomarkers and therapeutic targets for OSCC.
Systematic Review Registration: PROSPERO, number CRD42021256857.
Introduction
Head-and-neck squamous cell carcinoma (HNSCC) ranks the sixth most common neoplasm by incidence globally, accounting for 650,000 new cancer cases and 350,000 deaths worldwide annually (1). HNSCCs constitute a group of epithelial malignant tumors in the oral cavity, nose, sinuses, salivary gland, larynx, and pharynx. According to previous reports, males are more likely to be affected than females, with a ratio ranging from 2:1 to 4:1 (2). Among the subtypes of HNSCC, oral squamous cell carcinoma (OSCC) is the most common malignant neoplasm and has a poor prognosis with a 5-year survival rate of <50% (3, 4). The most widely applied therapies include surgery, chemotherapy, and radiotherapy, which significantly compromise the patients’ quality of life. In etiology, the typical risk factors are mainly related to environmental carcinogens, such as tobacco and alcohol, and risky lifestyle habits, e.g., betel nut chewing (5). Recently, the human papillomavirus (HPV) has emerged as an etiologic factor contributing to the development of HNSCC (6). HPV-positive HNSCC cases, as a consequence of HPV infections, mainly occur in the oropharynx region within the lymphoid epithelium of the tongue or tonsils, primarily in patients with the HPV-16 subtype (7).
Currently, the gold standard for OSCC diagnosis is still conventional oral examination and the histological evaluation of biopsy tissue, constituting highly accurate and reliable diagnostic methods with high specificity and sensitivity. However, the clinical application is limited due to patient discomfort and sampling bias, leading to misdiagnosis (8). Some biomarkers (such as carcinoembryonic antigen [CEA] and CA199) have been developed and implemented clinically. However, they have low accuracy and have proven inefficient. Consequently, it is imperative for clinicians to search for novel biomarkers as non-invasive diagnostic tools to enhance the efficacy of OSCC diagnosis.
Circular RNAs (circRNAs), a novel class of endogenous non-coding RNAs, are derived from the back-splicing by the canonical spliceosome via exon or intron circularization (9). As the high-throughput sequencing technology has made great strides and been widely employed, several circRNAs have been captured and identified (10). Instead of the linear structure within a 5’ cap and a 3’ polyadenylated tail, this non-coding RNA is characterized by a covalently closed-loop structure (11). CircRNAs are exceedingly stable and play a pivotal role in various physiological and pathophysiological processes. Studies recently published implied that this newly found subclass of long non-coding RNA has significantly boosted research efforts in many diseases, such as heart failure, autism, diabetes mellitus, and cancer (12–15). Cumulative research has illustrated that circRNAs function as microRNA molecular sponges and regulate gene expression and other biological procedures, such as cell proliferation, invasion, and migration (16).
Considerable evidence has indicated that circRNAs could serve as a viable diagnostic option. Nonetheless, due to variations in the study design, specimen type, and sample size, no explicit clinical diagnostic significance of circRNA in OSCC has been elucidated in previous studies. Therefore, this systematic meta-analysis aimed to combine the results of previously published studies to estimate the diagnostic test accuracy of dysregulated circRNAs as biomarkers for OSCC.
Materials and Methods
The process of study selection was conducted following the Preferred Reporting Items for Systematic Reviews and Meta-Analyses guideline for diagnostic test accuracy (PRISMA-DTA) (17).
Study Design
A systematic review and meta-analysis were applied to assess the clinical diagnostic capability of circRNAs in OSCC.
Bibliography Search Strategy
All the eligible studies in this meta-analysis were selected independently by two authors (MW and LZ). A thorough electronic search was carried out in PubMed, Web of Science, Embase, and Cochrane Library online databases up to March 30th, 2021. The full and reproducible keywords used in the search are provided in Appendix 1. In addition, the two authors independently and manually screened the titles, abstracts, and full texts to identify the relevant studies. Then the authors extracted the data from the relevant articles.
Inclusion and Exclusion Criteria
All the studies included in the meta-analysis met the following inclusion criteria.
The inclusion criteria were as follows:
a. case-control study or cohort study,
b. the diagnosis of oral squamous cell carcinoma was confirmed by histological examinations,
c. the studies analyzed the relationship between circRNAs and oral cancers,
d. circRNAs expression levels were assessed with quantitative real-time polymerase chain reaction(qRT-PCR) analysis, and
e. the sample size, sensitivity, specificity, and AUC were provided to calculate true positives (TP), false positives (FP), false negatives (FN), and true negatives (TN);
The exclusion criteria were as follows:
a. duplicate data from previous studies,
b. reviews, letters, case reports, and meeting abstracts, etc.,
c. non-English and animal studies, and
d. insufficient or unqualified data.
Data Extraction and Quality Assessment
The titles and abstracts of the articles were independently screened by two authors (MW and LZ) to determine their relevance to the topic, focusing on the diagnostic application of RNA in OSCC, defined by the International Classification of Diseases 10th Revision (ICD-10) codes C00-C06. Then LG and JZ evaluated the full text of the remaining articles, independently extracted the relevant data, and cross-checked to ensure data accuracy. The following data were extracted from each study: (a) basic information including the first author’s name, publication year, country, circRNA type, circRNA expression, sample size, cancer type, specimen, and detection method; (b) clinicopathological features including gender, age, tumor size, lymph node metastasis, TNM, T-stage, differentiation, and extrathyroidal extension; and (c) diagnostic information including sample size, sensitivity, specificity, and area under the curve (AUC).
The quality of each study was assessed independently by two authors (WR and SL) using the Quality Assessment of Diagnostic Accuracy Studies-2 (QUADAS-2) tool, retaining all original domain questions in two dimensions (“Risk of Bias” and “Applicability Concerns”) (18). Each risk of bias item was graded “yes”, “no”, or “unclear”, while the applicability concerns were evaluated as “high”, “low”, or “unclear”.
Summary Measures
The diagnostic sensitivity and specificity of the upregulated or downregulated circRNAs in the OSCC patients compared to the controls were considered the primary measures.
Statistical Analysis
The meta-analysis was conducted utilizing STATA 15.1 and Review Manager 5.4 statistical softwares to analyze the diagnostic performance of circRNAs in OSCC, constructing forest plots for sensitivity (SEN), specificity (SPE), negative likelihood ratio (NLR), positive likelihood ratio (PLR), and the diagnostic odds ratio (DOR). A summary receiver operator characteristics curve (SROC) was plotted to calculate the area under the SROC curve (AUC) and 95% confidence intervals (95% CIs) for the qualitative assessment of the diagnostic value. Deek’s funnel plot and funnel chart were constructed to estimate the publication bias between the included studies (with P > 0.05 indicating no publication bias). Furthermore, the Harbord test plot was established to scrutinize the potential publication bias in the meta-analysis (with P > 0.05 indicating no publication bias). Fagan’s nomogram was constructed to calculate post-test probabilities. An LR scatter matrix plot was utilized to assess the clinical significance of individual diagnostic studies, which was divided into four quadrants. Heterogeneity was estimated using I2 statistics and the Cochrane Q-test (with I² > 50% and P < 0.05 suggesting significant heterogeneity). The analysis applied a random-effects model due to significant heterogeneity. Meta-regression and subgroup analyses were performed to identify the potential source of heterogeneity. Sensitivity analysis was conducted by omitting individual studies to test the reliability of our analyses.
Trial Sequential Analysis
Trial sequential analysis (TSA) was performed for the meta-analysis results using the TSA software V.0.9.5.5 beta (Copenhagen Trial Unit). This analysis was utilized to estimate the required information size (RIS) for the statistical significance of the present meta-analysis. When the actual sample size in the meta-analysis failed to reach the RIS, TSA was applied to combine the results and provide a cumulated sample size of the included studies with an adjusted threshold to test the statistical significance and considerably reduce type I errors (false-positive results). Theoretically, the cumulative z-curve crossing both the conventional and TSA monitoring boundaries indicated sufficient evidence for the diagnostic capability of dysregulated circRNAs for OSCC detection. The required information size, adopting an alpha risk of 5% and a beta risk of 20%, was estimated for this analysis.
Results
Search Results
Figure 1 presents the detailed search process. A total of 2105 potentially eligible articles were identified from PubMed (903 records), Web of Science (603 records), Embase (598 records), and Cochrane Library (one record). A total of 671 records remained after eliminating 1434 duplicates. Furthermore, 615 articles were excluded for the following reasons: 56 were review articles, 553 were unrelated studies, and six were articles whose full texts were not available. After evaluating the full articles, 33 records were excluded without sufficient data, and 16 were removed because no relevant results were reported (Appendix 2). Finally, 872 cases and 900 controls from 16 studies were included in the meta-analysis (19–34).
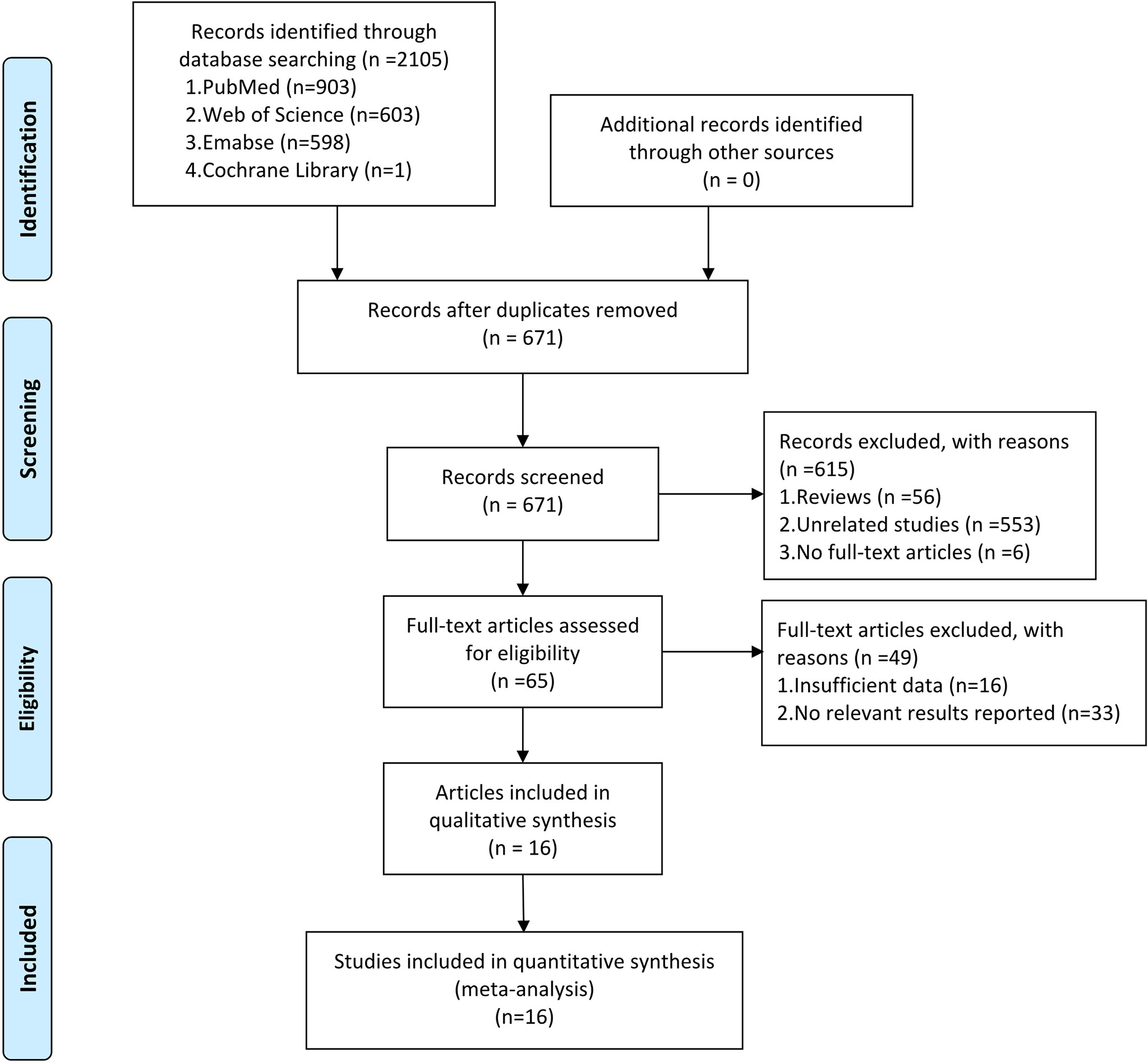
Figure 1 The PRISMA flow diagram showing the process of eligible studies search and selection. This meta-analysis identified 16 eligible studies that used circRNAs as biomarkers for tumor diagnosis.
Study Characteristics
In the meta-analysis, an evaluation was conducted on the association between circRNA expression levels and the type of OSCC to determine the accuracy of circRNA expression as an OSCC biomarker. Table 1 presents the main characteristics of each study. All the studies were released between 2018 and 2021, which were all from China. Each included study adopted the conventional case-control design. Twelve studies used paired OSCC as cases and corresponding adjacent normal tissue as controls. Four studies used saliva, plasma, or serum samples from OSCC patients as the cases and healthy volunteers as controls. The number of patients in the included studies ranged from 25 to 146. A total of 16 different circRNAs were assessed, among which five upregulated circRNAs were recognized as tumor promoters (22, 25, 29, 30, 32) and 11 were downregulated as tumor suppressors (19–21, 23, 24, 26–28, 31, 33, 34). All circRNA expression levels were detected using qRT-PCR in tissues (n = 12), plasma (n = 1), serum (n = 2), and saliva (n = 1). All the samples were collected before clinical treatment.
Risk of Bias and Applicability Concerns Within Studies
Not a single included study fulfilled all the domain criteria in the QUADAS-2 methodological quality tool. On average two out of four domains of risk of bias were fulfilled in each study. The case-control design and inappropriate exclusions(for the specific diagnosis) explained why no study was observed to have a low risk in patient selection and index test domain. Two of these studies were graded as high risk in patient selection because no exact time scope and continuity were mentioned. Items 4 and 7 were assessed as unclear because no information on blinding was reported. All the articles met the criteria of the three domains of applicability concerns (Figure 2 and Appendix 3).
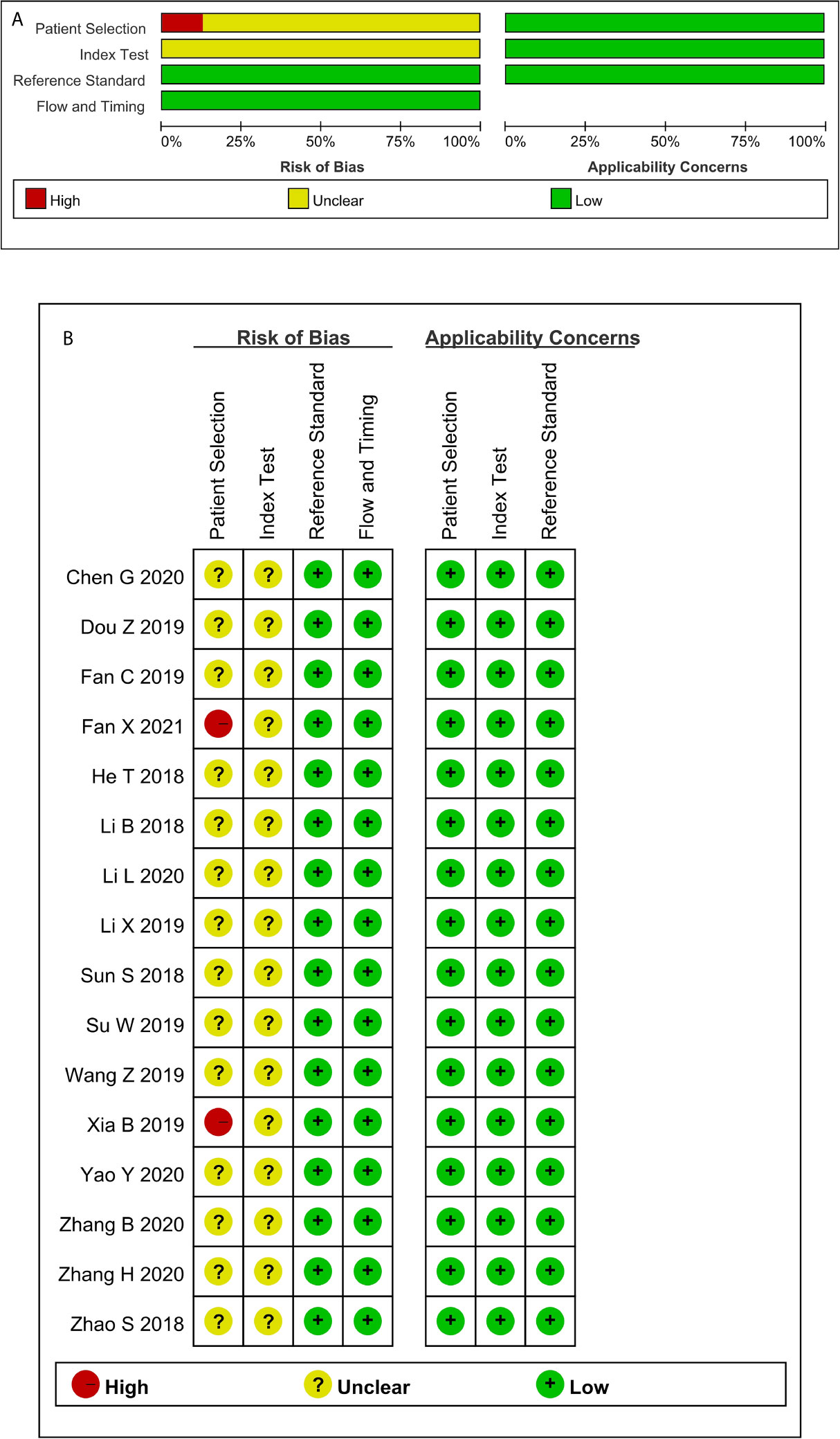
Figure 2 Quality assessment of the included studies according to QUADAS-2 (A) Methodological quality graph; (B) Methodological quality summary.
Meta-Analysis
The present meta-analysis of 16 cohorts in 872 patients and 900 controls included 16 circRNA types. A random-effects model was selected because of the significant heterogeneity (I² > 50%) between the included studies. The meta-analysis was conducted, and the pooled sensitivity, specificity, PLR, NLR, DOR, and SROC were calculated for circRNA, as illustrated in Figure 3. The pooled statistical values for sensitivity (Figure 3A), specificity (Figure 3B), PLR (Figure 3C), NLR (Figure 3D), and DOR (Figure 3E) with the 95% confidence intervals for the enrolled studies in this study were 0.74 (95% CI: 0.69 – 0.79), 0.79 (95% CI: 0.73 – 0.84), 3.50 (95% CI: 2.76 – 4.45), 0.33 (95% CI: 0.27 – 0.39), and 10.74 (95% CI: 7.81 – 14.77), respectively. The diagnostic odds ratio (DOR) represents a critical indicator that assists in a meta-analysis by focusing on diagnostic performance, and combines the advantages of both sensitivity and specificity, and describes the diagnostic value of a circRNA (35). The summary receiver operator curve (SROC, Figure 3F) plot revealed an AUC of 0.83 (95% CI: 0.79 – 0.86). The bivariate boxplot in Figure 3H presents the heterogeneity details in the included studies. Fagan’s nomogram was constructed to calculate the post-test probabilities of the circRNAs, in which the post-test possibility increased to 47% with a positive likelihood ratio (LR) of 4, with the post-test possibility decreasing to 8% with a negative LR of 0.33 (Figure 4). These findings indicated that circRNAs were a credible diagnostic biomarker with high accuracy and efficacy. Figure 5 presents an LR scattergram plotted with the combined summary points.
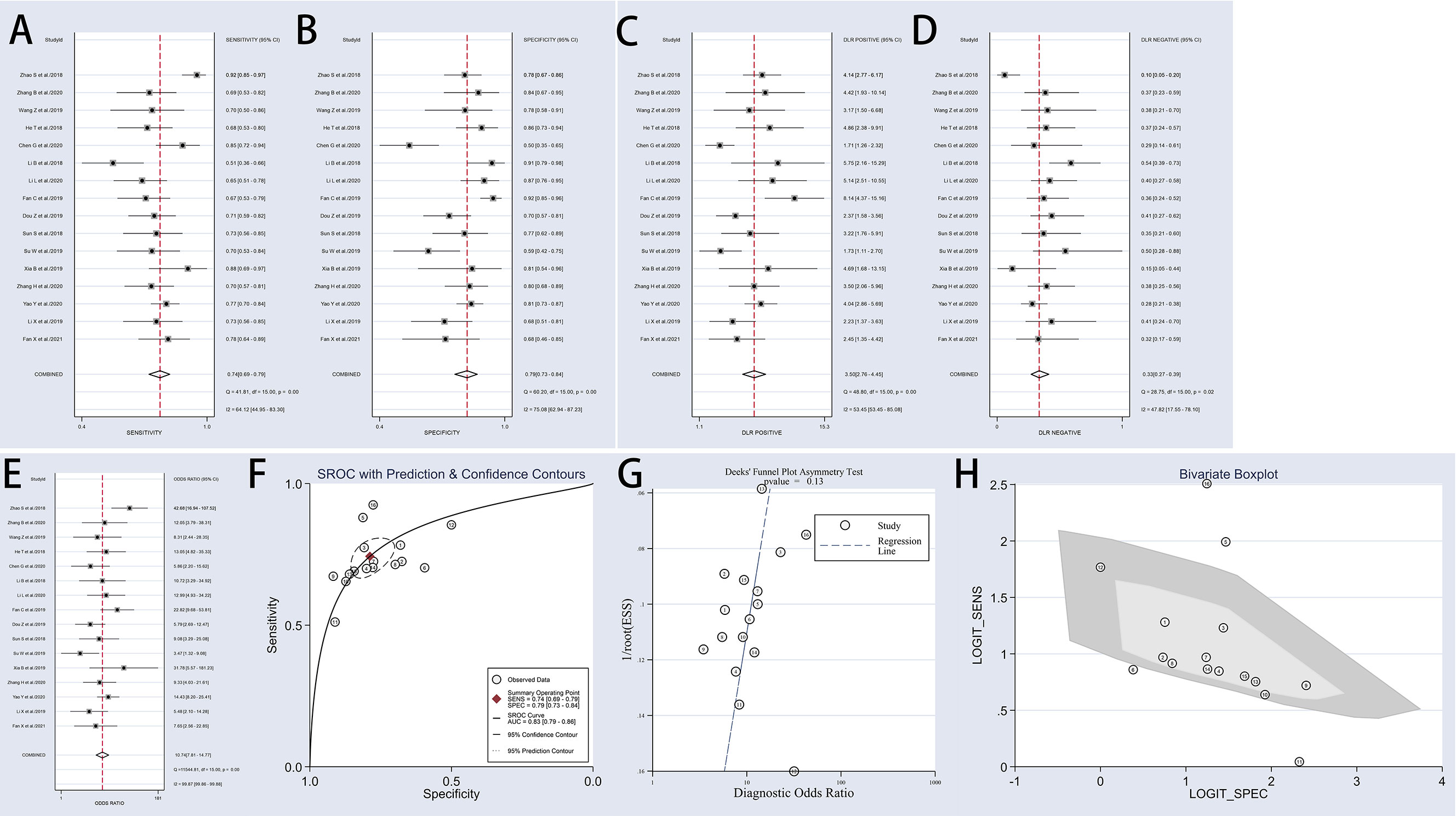
Figure 3 Forest plots of sensitivity, specificity, PLR, NLR, DOR, AUC, and funnel plot for diagnosis of circRNAs in OSCC among 16 studies. (A) Sensitivity; (B) Specificity; (C) PLR; (D) NLR; (E) DOR; (F) AUC (SROC curve); (G) Deek’s funnel plot; and (H) Bivariate boxplot. OSCC, oral squamous cell carcinoma; PLR, positive likelihood ratio;NLR, negative likelihood ratio; DOR, diagnostics odds ratio; SROC, Summary receiver operator characteristics curve; AUC, the area under SROC curve.
Therefore, taken together, the results indicated that the circRNAs had good diagnostic accuracy for OSCC and could serve as effective biomarkers of OSCC.
Meta-Regression and Subgroup Analysis
Overall, the studies exhibited relatively high heterogeneity in sensitivity and specificity (I² = 64.12 and I² = 75.08, respectively). Thus, a meta-regression analysis was first conducted to investigate the heterogeneity source (Appendix 4). The meta-regression analysis demonstrated that three covariates (specimen type, expression status, and sample size) could explain 100% of the between-study variance.
Table 2 and Figures 6–8 present further subgroup analyses to identify the source of the heterogeneity. Subgroups of studies utilizing serum (n = 2), plasma (n = 1), and saliva (n = 1) specimens exhibited better diagnostic performance with DOR (24.70 vs. 9.00) and the AUC (0.90 vs. 0.80) compared to the tissue (n = 12) subgroup. The pooled sensitivity and specificity were both greater than in the tissue subgroup. No significant heterogeneity was observed in the tissue subgroup (I² = 0.0, P = 0.450) or other specimen types (I² = 47.6%, P = 0.125). Therefore, the variance between these two subgroups of specimen types may account for the heterogeneity.
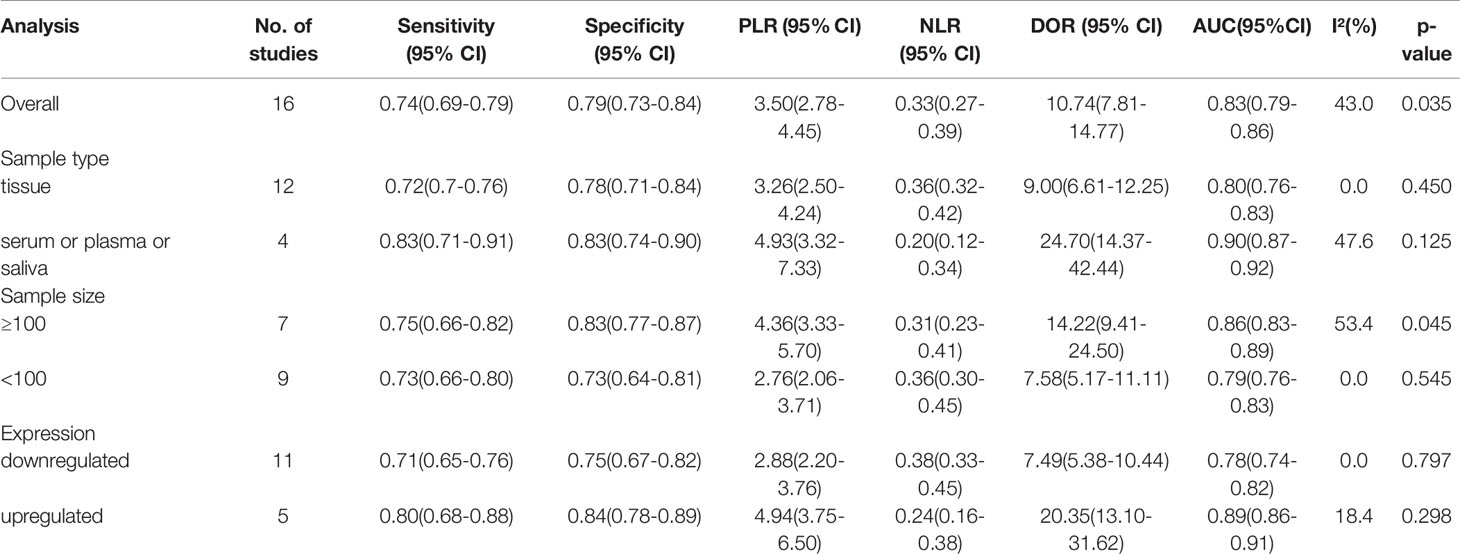
Table 2 Results of subgroup analysis of cricRNAs reported by 16 studies in diagnostic meta-analysis.
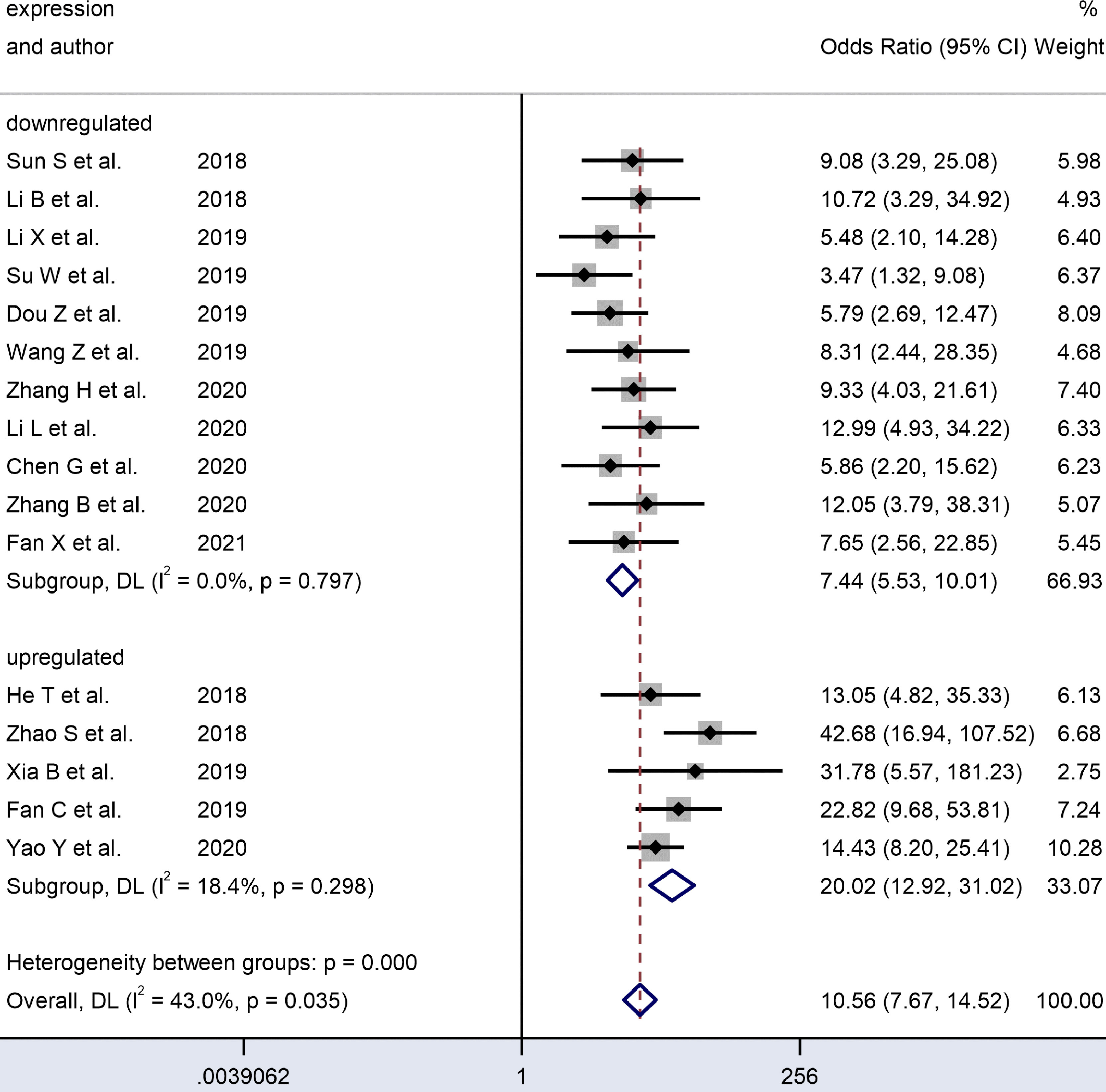
Figure 6 Forest plots of subgroup analysis of the combined ORs with 95%CIs according to expression status of circRNAs in patients with OSCC.
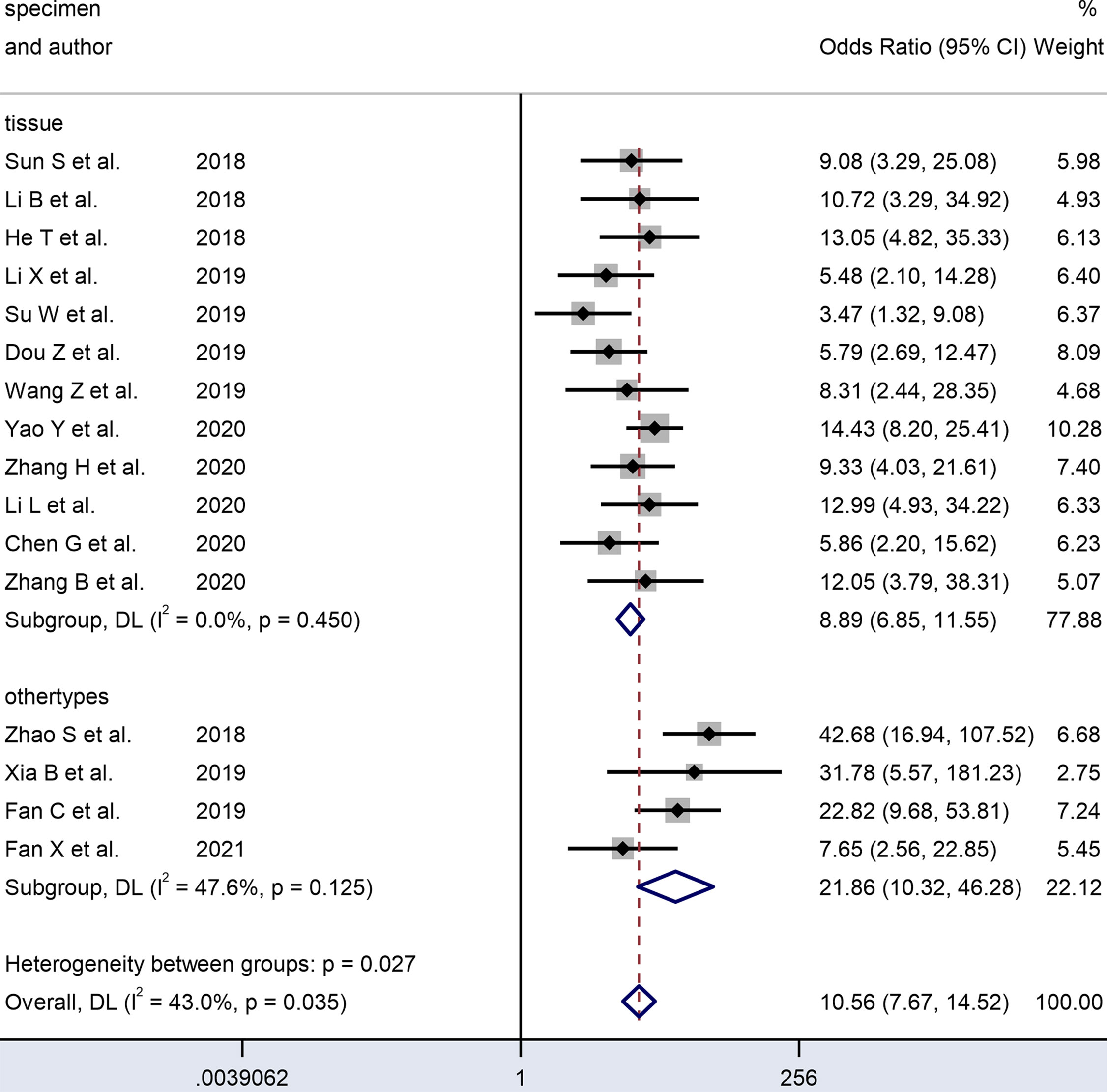
Figure 7 Forest plots of subgroup analysis of the combined ORs with 95%CIs according to specimen type of circRNAs in patients with OSCC.
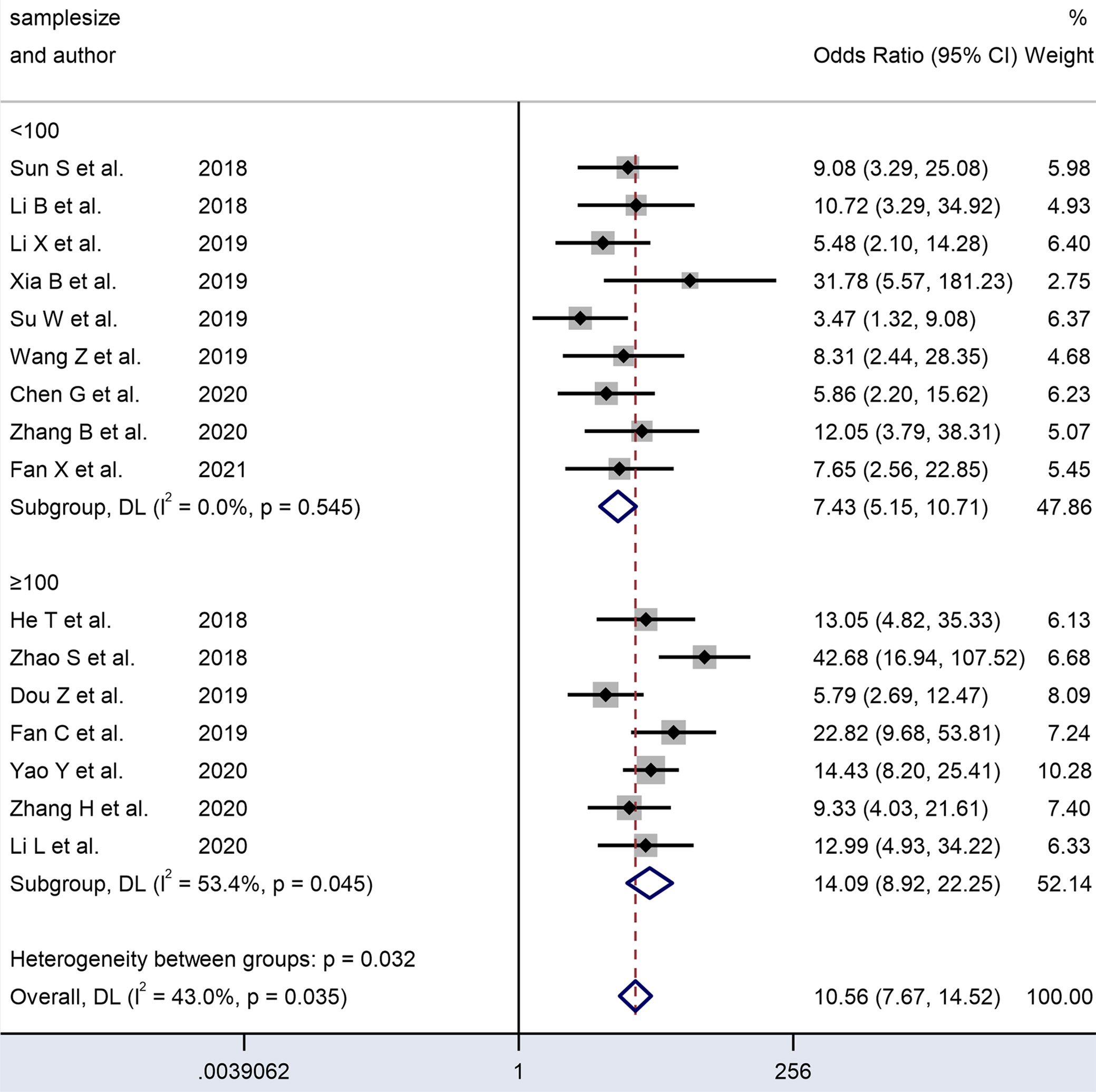
Figure 8 Forest plots of subgroup analysis of the combined ORs with 95%CIs according to sample size of circRNAs in patients with OSCC.
We analyzed the subgroups according to the expression status of dysregulated circRNAs. The studies on upregulated circRNAs (n = 5) had a significantly higher pooled DOR (20.35 vs. 7.49) and AUC (0.89 vs. 0.78) than those on downregulated circRNAs (n=11). In the forest plots, the results covered no heterogeneity in upregulated (I² = 18.4%, P = 0.298) and downregulated circRNAs (I² = 0.0, P = 0.797). Since no heterogeneity was observed in the subgroups, the difference between these two expression status subgroups may account for the heterogeneity.
An analysis of subgroups classified by the sample size of the included cohorts (≥ 100 and < 100) was also carried out. The ≥ 100 subgroup (n = 7) had a higher DOR (14.22 vs. 7.58) and AUC (0.86 vs. 0.79) than the < 100 subgroup (n = 9) (Table 2). In the former subgroup, evident heterogeneity was detected with a value of I² = 53.4 (P = 0.045). However, no heterogeneity was observed in the latter subgroup (I² = 0.0, P= 0.545). Hence, the subgroup analysis results indicated that the sample size of the enrolled cohorts might be the source of heterogeneity.
Publication Bias
Deek’s funnel plot asymmetry tests were employed to assess the publication bias, as illustrated in Figure 3G, with the results indicating no obvious publication bias (P = 0.13). The funnel plot and Harbord test shown in Figure 9 were used to track the potential publication bias in the meta-analysis. The P-value of both was > 0.05 (P = 0.13 and P = 0.175), suggesting no publication bias in the meta-analysis.
Sensitivity Analysis
A sensitivity analysis was carried out to explain the heterogeneity of each study. As shown in Figure 10, omitting any individual study had no substantial impact on the pooled statistics, indicating that the results were credible and reliable.
TSA Outcome
The overall required information size was calculated for the 1762 participants (Appendix 5). The z-curve crossed both the conventional and TSA monitoring boundaries. However, it failed to reach the RIS line, indicating statistical significance and sufficient evidence on the diagnostic performance of circRNAs as biomarkers for OSCC.
Discussion
Previous cumulative investigations have demonstrated that dysregulated circRNAs played a critical role in the cell proliferation, metastasis, and occurrence of various cancers. The closed, covalent, and continuous circular structure of circRNAs makes them more steady than their linear counterparts (36). Moreover, dysregulated circRNAs have been discovered in plasma, tissues, and serum (37). The characteristics above render circRNAs favorable as molecular biomarkers of cancer.
Overall, 16 studies were included in this meta-analysis. The final results based on all the enrolled studies showed an AUC of 0.83 for circRNAs, with a sensitivity of 0.74 and specificity of 0.79 in distinguishing OSCC patients from healthy controls. The pooled sensitivity and specificity showed moderate diagnostic test accuracy, indicating that circRNAs had sufficient statistical ability to identify or exclude suspected cases to enhance the clinical diagnosis. A DOR of 10.74 (> 1.0) was obtained in the present analysis, indicating that the dysregulated circRNAs were effective predictive biomarkers for OSCC. Furthermore, the higher AUC value showed better performance in the balance of sensitivity and specificity. Notably, the high AUC value of 0.83 in the current analysis reflects the overall relatively high diagnostic accuracy of circRNAs in OSCC detection.
To the best of our knowledge, this study was the first systematic review and meta-analysis to estimate the diagnostic ability of circRNAs as biomarkers in OSCC patients and summarize their sensitivity and specificity. Other published systematic reviews have evaluated several biomarkers for OSCC diagnosis, such as microRNAs, mRNAs, and proteins. Rapado-Gonzalez et al. performed a systematic review of microRNAs in OSCC and summarized the clinical correlation, including proliferation and progression, with a relatively high AUC of 0.91 (38). Gaba et al. assessed the diagnostic value of a specific mRNA, DUSP1, which proved insufficient with an AUC of 0.66. In addition, Gaba et al. reviewed the clinical correlation of a protein named IL1-β protein and estimated its diagnostic value, which was considered good with an AUC of 0.82 (39). However, despite the growing number of reviews and analyses based on diagnostic biomarkers for OSCC, no consensus has been reached on determining which biomarker has the best diagnostic performance for OSCC and what is the most accurate sample for testing.
Meta-regression and subgroup analyses of specimens, sample size, and the expression status of the dysregulated circRNAs were performed to explore the sources of the heterogeneity. The random-effects-based meta-regression analysis showed that these three covariates were the main sources of the heterogeneity. Studies that utilized serum, plasma, and saliva specimens performed significantly better in diagnosing OSCC patients compared to those using tissue specimens, with no heterogeneity detected. The subgroups with upregulated circRNAs and ≥ 100 samples were found to possess a much higher diagnostic accuracy than the downregulated circRNAs and those with < 100 samples and these two subgroups showed no heterogeneity. Apparent heterogeneity was found in the subgroup with ≥ 100 samples. Therefore, the heterogeneity might be related to the sample size, specimen type, and expression status.
We further considered the reasons for the differences in the diagnostic accuracy between the tissue and liquid biopsies. Concerning tissue biopsies, OSCC, as a solid tumor, exhibits tissue heterogeneity even for the same histological type. The proportion of tumor cells and mesenchymal cells vary in different patients and even in different parts of the same tumor. Therefore, the sample used for detection only accounts for part of the tumor. This cannot accurately reflect the whole tumor status, which is the potential reason for the lower accuracy in tissue samples than detection by body fluid specimens.
Currently, tissue-based diagnostic strategies require the testing of materials obtained through invasive procedures, such as biopsy or aspiration, which are usually associated with severe discomfort and medical costs (40). Compared to tissue biopsies, body fluids are a better choice for disease screening and diagnosis due to the advantages of accessibility, low invasion, low cost, and various sample types to monitor disease development (41). Several studies have reviewed the potential of mRNAs as diagnostic markers, illustrating the possible clinical application of OSCC-specific signals in body fluids (42, 43). These molecules can be prospective candidates for biomarkers due to their stable circulation in human body fluids and accessibility through non-invasive methods. Likewise, circRNA detection can be regarded as a novel method for body fluid-based biopsies, which would be helpful as significant diagnostic and monitoring tools in the clinic. At present, there is a shortage of research on the diagnostic value of circRNAs in body fluids for OSCC, which provides a new direction for researchers worldwide to utilize the saliva and serum of high-risk patients with lesions in the oral cavity suspected of oral squamous cell carcinoma.
Nevertheless, it is not yet possible to apply these molecular biomarkers in clinical diagnosis and monitoring. New techniques, such as digital PCR, make it possible to test cancer and phenotype-specific molecular changes to improve sensitivity and accuracy. After this technique is used more widely, the successful application of molecular markers in liquid biopsies of other tumors (e.g., lung carcinoma) will encourage further evaluation of this method in OSCC cases.
Several deficiencies in the present study merit consideration. (a) Based on our inclusion criteria, all samples and relevant statistics accidentally came from China. (b) Subgroup analysis of different circRNA should be further performed. (c) Conspicuous heterogeneity existed in the included studies. The sample size, specimen types, and expression status might be sources of the heterogeneity. (d) The sample size and number of the enrolled studies in this analysis were relatively small. Thus, more comprehensive high-quality studies that encompass larger scales, more regions, well-designed operations, and further exploration into the functional mechanisms are necessary.
Conclusion
In summary, this meta-analysis revealed a strong association between the altered expression of circRNAs and the diagnosis of OSCC. Hence, circRNAs can potentially serve as promising biomarkers and therapeutic targets for OSCC. Nevertheless, the clinical application of cricRNAs for the detection of OSCC requires further research.
Data Availability Statement
The original contributions presented in the study are included in the article/Supplementary Material. Further inquiries can be directed to the corresponding authors.
Author Contributions
LZ contributed to conception, analysis, and drafted manuscript. MW contributed to conception and design, acquisition and interpretation of data, and critically revised manuscript. WR contributed to design, interpretation of data, and drafted manuscript. SL contributed to design, acquisition of data, and critically revised manuscript. KZ critically revised manuscript. JZ contributed to acquisition and analysis of data. LG contributed to analysis and interpretation of data, and drafted manuscript. All authors contributed to the article and approved the submitted version.
Funding
This work was supported by the Natural Science Foundation of Shandong Province (2019GSF108273, 2017WS215, and ZR2018BH021), the Qingdao Outstanding Health Professional Development Fund, and the Qilu Health Leading Talent Project.
Supplementary Material
The Supplementary Material for this article can be found online at: https://www.frontiersin.org/articles/10.3389/fonc.2021.693284/full#supplementary-material
Conflict of Interest
The authors declare that the research was conducted in the absence of any commercial or financial relationships that could be construed as a potential conflict of interest.
References
1. Argiris A, Karamouzis MV, Raben D, Ferris RL. Head and Neck Cancer. Lancet (Lond Engl) (2008) 371(9625):1695–709. doi: 10.1016/s0140-6736(08)60728-x
2. Bray F, Ferlay J, Soerjomataram I, Siegel RL, Torre LA, Jemal A. Global Cancer Statistics 2018: GLOBOCAN Estimates of Incidence and Mortality Worldwide for 36 Cancers in 185 Countries. CA: Cancer J Clin (2018) 68(6):394–424. doi: 10.3322/caac.21492
3. Chi AC, Day TA, Neville BW. Oral Cavity and Oropharyngeal Squamous Cell Carcinoma–an Update. CA Cancer J Clin (2015) 65(5):401–21. doi: 10.3322/caac.21293
4. Leemans CR, Braakhuis BJ, Brakenhoff RH. The Molecular Biology of Head and Neck Cancer. Nat Rev Cancer (2011) 11(1):9–22. doi: 10.1038/nrc2982
5. Chai AWY, Lim KP, Cheong SC. Translational Genomics and Recent Advances in Oral Squamous Cell Carcinoma. Semin Cancer Biol (2020) 61:71–83. doi: 10.1016/j.semcancer.2019.09.011
6. Leemans CR, Snijders PJF, Brakenhoff RH. The Molecular Landscape of Head and Neck Cancer. Nat Rev Cancer (2018) 18(5):269–82. doi: 10.1038/nrc.2018.11
7. Solomon B, Young RJ, Rischin D. Head and Neck Squamous Cell Carcinoma: Genomics and Emerging Biomarkers for Immunomodulatory Cancer Treatments. Semin Cancer Biol (2018) 52(Pt 2):228–40. doi: 10.1016/j.semcancer.2018.01.008
8. Yang EC, Tan MT, Schwarz RA, Richards-Kortum RR, Gillenwater AM, Vigneswaran N. Noninvasive Diagnostic Adjuncts for the Evaluation of Potentially Premalignant Oral Epithelial Lesions: Current Limitations and Future Directions. Oral Surg Oral Med Oral Pathol Oral Radiol (2018) 125(6):670–81. doi: 10.1016/j.oooo.2018.02.020
9. Starke S, Jost I, Rossbach O, Schneider T, Schreiner S, Hung LH, et al. Exon Circularization Requires Canonical Splice Signals. Cell Rep (2015) 10(1):103–11. doi: 10.1016/j.celrep.2014.12.002
10. Guo JU, Agarwal V, Guo H, Bartel DP. Expanded Identification and Characterization of Mammalian Circular RNAs. Genome Biol (2014) 15(7):409. doi: 10.1186/s13059-014-0409-z
11. Hentze MW, Preiss T. Circular RNAs: Splicing’s Enigma Variations. EMBO J (2013) 32(7):923–5. doi: 10.1038/emboj.2013.53
12. van Heesch S, Witte F, Schneider-Lunitz V, Schulz JF, Adami E, Faber AB, et al. The Translational Landscape of the Human Heart. Cell (2019) 178(1):242–60. doi: 10.1016/j.cell.2019.05.010
13. Shan K, Liu C, Liu BH, Chen X, Dong R, Liu X, et al. Circular Noncoding RNA HIPK3 Mediates Retinal Vascular Dysfunction in Diabetes Mellitus. Circulation (2017) 136(17):1629–42. doi: 10.1161/circulationaha.117.029004
14. Gomes CPC, Schroen B, Kuster GM, Robinson EL, Ford K, Squire IB, et al. Regulatory RNAs in Heart Failure. Circulation (2020) 141(4):313–28. doi: 10.1161/circulationaha.119.042474
15. Gasparini S, Del Vecchio G, Gioiosa S, Flati T, Castrignano T, Legnini I, et al. Differential Expression of Hippocampal Circular RNAs in the BTBR Mouse Model for Autism Spectrum Disorder. Mol Neurobiol (2020) 57(5):2301–13. doi: 10.1007/s12035-020-01878-6
16. Meng X, Li X, Zhang P, Wang J, Zhou Y, Chen M. Circular RNA: An Emerging Key Player in RNA World. Brief Bioinform (2017) 18(4):547–57. doi: 10.1093/bib/bbw045
17. McInnes MDF, Moher D, Thombs BD, McGrath TA, Bossuyt PM, Clifford T, et al. Preferred Reporting Items for a Systematic Review and Meta-analysis of Diagnostic Test Accuracy Studies: The Prisma-DTA Statement. Jama (2018) 319(4):388–96. doi: 10.1001/jama.2017.19163
18. Whiting PF, Rutjes AW, Westwood ME, Mallett S, Deeks JJ, Reitsma JB, et al. Quadas-2: a Revised Tool for the Quality Assessment of Diagnostic Accuracy Studies. Ann Intern Med (2011) 155(8):529–36. doi: 10.7326/0003-4819-155-8-201110180-00009
19. Fan X, Wang Y. Circular RNA Circspata6 Inhibits the Progression of Oral Squamous Cell Carcinoma Cells by Regulating TRAF6 Via Mir-182. Cancer Manag Res (2021) 13:1817–29. doi: 10.2147/cmar.S292074
20. Zhang H, Shen Y, Zhang B, Qian M, Zhang Y, Yang H. Hsa_Circ_0003829 Serves as a Potential Diagnostic Predictor for Oral Squamous Cell Carcinoma. J Int Med Res (2020) 48(9):300060520936880. doi: 10.1177/0300060520936880
21. Zhang B, Wang Z, Shen Y, Yang H. Silencing Circular RNA Hsa_Circ_009755 Promotes Growth and Metastasis of Oral Squamous Cell Carcinoma. Genomics (2020) 112(6):5275–81. doi: 10.1016/j.ygeno.2020.09.035
22. Yao Y, Bi L, Zhang C. Circular RNA_0001742 Has Potential to Predict Advanced Tumor Stage and Poor Survival Profiles in Tongue Squamous Cell Carcinoma Management. J Clin Lab Anal (2020) 34(8):e23330. doi: 10.1002/jcla.23330
23. Li L, Zhang ZT. Hsa_Circ_0086414 Might be a Diagnostic Biomarker of Oral Squamous Cell Carcinoma. Med Sci Monit (2020) 26:e919383. doi: 10.12659/msm.919383
24. Chen G, Li Y, He Y, Zeng B, Yi C, Wang C, et al. Upregulation of Circular Rna circATRNL1 to Sensitize Oral Squamous Cell Carcinoma to Irradiation. Mol Ther Nucleic Acids (2020) 19:961–73. doi: 10.1016/j.omtn.2019.12.031
25. Xia B, Hong T, He X, Hu X, Gao Y. A Circular RNA Derived From MMP9 Facilitates Oral Squamous Cell Carcinoma Metastasis Through Regulation of MMP9 mRNA Stability. Cell Transplant (2019) 28(12):1614–23. doi: 10.1177/0963689719875409
26. Wang Z, Tang J, Wang Y, Sun S, Chen Y, Shen Y, et al. Circular RNA Hsa_Circ_009755 Downregulation Correlates With Clinicopathology in Oral Squamous Cell Carcinoma. Onco Targets Ther (2019) 12:4025–31. doi: 10.2147/ott.S196618
27. Su W, Wang Y, Wang F, Sun S, Li M, Shen Y, et al. Hsa_Circ_0005379 Regulates Malignant Behavior of Oral Squamous Cell Carcinoma Through the EGFR Pathway. BMC Cancer (2019) 19(1):400. doi: 10.1186/s12885-019-5593-5
28. Li X, Zhang H, Wang Y, Sun S, Shen Y, Yang H. Silencing Circular RNA Hsa_Circ_0004491 Promotes Metastasis of Oral Squamous Cell Carcinoma. Life Sci (2019) 239:116883. doi: 10.1016/j.lfs.2019.116883
29. He T, Li X, Xie D, Tian L. Overexpressed circPVT1 in Oral Squamous Cell Carcinoma Promotes Proliferation by Serving as a miRNA Sponge. Mol Med Rep (2019) 20(4):3509–18. doi: 10.3892/mmr.2019.10615
30. Fan CM, Wang JP, Tang YY, Zhao J, He SY, Xiong F, et al. circMAN1A2 Could Serve as a Novel Serum Biomarker for Malignant Tumors. Cancer Sci (2019) 110(7):2180–8. doi: 10.1111/cas.14034
31. Dou Z, Li S, Ren W, Wang Q, Liu J, Kong X, et al. Decreased Expression of Hsa_Circ_0072387 as a Valuable Predictor for Oral Squamous Cell Carcinoma. Oral Dis (2019) 25(5):1302–8. doi: 10.1111/odi.13094
32. Zhao SY, Wang J, Ouyang SB, Huang ZK, Liao L. Salivary Circular Rnas Hsa_Circ_0001874 and Hsa_Circ_0001971 as Novel Biomarkers for the Diagnosis of Oral Squamous Cell Carcinoma. Cell Physiol Biochem (2018) 47(6):2511–21. doi: 10.1159/000491624
33. Sun S, Li B, Wang Y, Li X, Wang P, Wang F, et al. Clinical Significance of the Decreased Expression of Hsa_Circ_001242 in Oral Squamous Cell Carcinoma. Dis Markers (2018) 2018:6514795. doi: 10.1155/2018/6514795
34. Li B, Wang F, Li X, Sun S, Shen Y, Yang H. Hsa_Circ_0008309 May be a Potential Biomarker for Oral Squamous Cell Carcinoma. Dis Markers (2018) 2018:7496890. doi: 10.1155/2018/7496890
35. Glas AS, Lijmer JG, Prins MH, Bonsel GJ, Bossuyt PM. The Diagnostic Odds Ratio: A Single Indicator of Test Performance. J Clin Epidemiol (2003) 56(11):1129–35. doi: 10.1016/s0895-4356(03)00177-x
36. Lei M, Zheng G, Ning Q, Zheng J, Dong D. Translation and Functional Roles of Circular RNAs in Human Cancer. Mol Cancer (2020) 19(1):30. doi: 10.1186/s12943-020-1135-7
37. Wang F, Nazarali AJ, Ji S. Circular RNAs as Potential Biomarkers for Cancer Diagnosis and Therapy. Am J Cancer Res (2016) 6(6):1167–76.
38. Rapado-González O, Martínez-Reglero C, Salgado-Barreira A, López-López R, Suárez-Cunqueiro MM, Muinelo-Romay L. miRNAs in Liquid Biopsy for Oral Squamous Cell Carcinoma Diagnosis: Systematic Review and Meta-Analysis. Oral Oncol (2019) 99:104465. doi: 10.1016/j.oraloncology.2019.104465
39. Gaba FI, Sheth CC, Veses V. Salivary Biomarkers and Their Efficacies as Diagnostic Tools for Oral Squamous Cell Carcinoma: Systematic Review and Meta-Analysis. J Oral Pathol Med (2021) 50(3):299–307. doi: 10.1111/jop.12791
40. Schafer CA, Schafer JJ, Yakob M, Lima P, Camargo P, Wong DT. Saliva Diagnostics: Utilizing Oral Fluids to Determine Health Status. Monogr Oral Sci (2014) 24:88–98. doi: 10.1159/000358791
41. Good DM, Thongboonkerd V, Novak J, Bascands JL, Schanstra JP, Coon JJ, et al. Body Fluid Proteomics for Biomarker Discovery: Lessons From the Past Hold the Key to Success in the Future. J Proteome Res (2007) 6(12):4549–55. doi: 10.1021/pr070529w
42. Li Y, St John MA, Zhou X, Kim Y, Sinha U, Jordan RC, et al. Salivary Transcriptome Diagnostics for Oral Cancer Detection. Clin Cancer Res (2004) 10(24):8442–50. doi: 10.1158/1078-0432.Ccr-04-1167
Keywords: circular RNA, OSCC, oral oncology, meta-analysis, biomarker, diagnosis
Citation: Wang M, Zhang L, Ren W, Li S, Zhi K, Zheng J and Gao L (2021) Diagnostic Value of CircRNAs as Potential Biomarkers in Oral Squamous Cell Carcinoma: a Meta-Analysis. Front. Oncol. 11:693284. doi: 10.3389/fonc.2021.693284
Received: 10 April 2021; Accepted: 22 June 2021;
Published: 08 July 2021.
Edited by:
Die Wang, Hudson Institute of Medical Research, AustraliaReviewed by:
Jaimanti Bakshi, Post Graduate Institute of Medical Education and Research (PGIMER), IndiaLiang Ding, Nanjing University, China
Copyright © 2021 Wang, Zhang, Ren, Li, Zhi, Zheng and Gao. This is an open-access article distributed under the terms of the Creative Commons Attribution License (CC BY). The use, distribution or reproduction in other forums is permitted, provided the original author(s) and the copyright owner(s) are credited and that the original publication in this journal is cited, in accordance with accepted academic practice. No use, distribution or reproduction is permitted which does not comply with these terms.
*Correspondence: Jingjing Zheng, zhengjingjing.1984@163.com; Ling Gao, coffee_smile@163.com
†These authors have contributed equally to this work and share first authorship