- 1Department of Radiation Oncology, Shandong Cancer Hospital and Institute, Shandong First Medical University and Shandong Academy of Medical Sciences, Jinan, China
- 2Tianjin Medical University Cancer Institute and Hospital, National Clinical Research Center for Cancer, Key Laboratory of Cancer Prevention and Therapy, Tianjin, China
- 3Department of Oncology, Tianjin Medical University, Tianjin, China
- 4Department of Computer Science and Information Technology, La Trobe University, Melbourne, VIC, Australia
- 5Department of Obstetrics and Gynecology, Qilu Hospital of Shandong University, Jinan, China
Background and Purpose: This study aims to develop a risk model to predict esophageal fistula in esophageal cancer (EC) patients by learning from both clinical data and computerized tomography (CT) radiomic features.
Materials and Methods: In this retrospective study, computerized tomography (CT) images and clinical data of 186 esophageal fistula patients and 372 controls (1:2 matched by the diagnosis time of EC, sex, marriage, and race) were collected. All patients had esophageal cancer and did not receive esophageal surgery. 70% patients were assigned into training set randomly and 30% into validation set. We firstly use a novel attentional convolutional neural network for radiographic descriptor extraction from nine views of planes of contextual CT, segmented tumor and neighboring structures. Then clinical factors including general, diagnostic, pathologic, therapeutic and hematological parameters are fed into neural network for high-level latent representation. The radiographic descriptors and latent clinical factor representations are finally associated by a fully connected layer for patient level risk prediction using SoftMax classifier.
Results: 512 deep radiographic features and 32 clinical features were extracted. The integrative deep learning model achieved C-index of 0.901, sensitivity of 0.835, and specificity of 0.918 on validation set with superior performance than non-integrative model using CT imaging alone (C-index = 0.857) or clinical data alone (C-index = 0.780).
Conclusion: The integration of radiomic descriptors from CT and clinical data significantly improved the esophageal fistula prediction. We suggest that this model has the potential to support individualized stratification and treatment planning for EC patients.
Introduction
EC is the 8th most common tumor worldwide (1), and nearly half of the cases are found in China (2). Patients with EC achieve improved prognosis with recent advance in radiotherapy, chemotherapy and immunotherapy (3). However, the treatment outcome of patients who developed esophageal fistula, a severe complication of EC, is still well below satisfaction and expectation. Perforation may lead to prolonged infection, poor nutrition, sepsis, and even massive hemorrhage, which can considerably affect survival. It is reported that the median post-fistula survival time of EC patients with esophageal fistula was approximately 3.63 months (4). Therefore, predicting esophageal fistula before treatment is highly desirable to improve prognosis in EC patients.
Previous studies on esophageal fistula mostly focused on clinical parameters, using the logistics regression analysis to establish predictive models (5–7). Such researches cannot effectively handle the complex relationship between esophageal fistula and numerous risk factors in the real world, and the predictive efficacy cannot meet the needs. Moreover, the importance of CT imaging has never been reported. The radiographic features contained in CT images, such as tumor texture features, tumor size, and other morphological information, are important potential biomarker (8). Previous research reported its application in predicting the survival (8), lymph node metastasis (9) and treatment response (10) in EC patients. Combining CT imaging and clinical features can more accurately predict esophageal fistula.
Deep learning methods can identify non-linear relationships between different types of parameters, and have been explored in large data analysis (11) and medical images diagnosis (12). However, there is no deep learning study involving esophageal fistula.
In this study, we developed a deep learning model of esophageal fistula for EC patients. Our model automatically extracted the information in the CT imaging and integrated the clinical features. In addition, the attention map was drawn to visualize the neural network based on CT images.
Materials and Methods
Patients
This retrospective study was approved by the local review board. For this type of study, formal informed consent was not required, and all collected data was kept confidential and anonymous. EC patients who developed esophageal fistula in Shandong Cancer Hospital from July 2014 to August 2019 were retrospectively enrolled as the case group. Patients who were clearly described with esophageal fistula or perforation in CT, esophagogram or endoscopy systems were collected. Because anastomotic fistula is a special type of esophageal fistula closely related to surgical methods and surgical techniques, our study did not involve anastomotic fistula after esophagus surgery. We only study the esophageal fistula caused by tumor itself and treatment. The inclusion criteria included: 1) patients diagnosed as EC pathologically with the World Health Organization (WHO) criteria; 2) availability of general, diagnostic and therapeutic data; 3) availability of contrast-enhanced CT imaging before treatment; 4) diagnosed as esophageal fistula by either endoscopy, CT or contrast radiography of the upper gastrointestinal tract. Exclusion criteria were: 1) patients treated by esophageal surgery; 2) the fistula developed due to medical injure or trauma; 3) concomitant with another carcinoma. By such, there are 186 eligible patients. At the same time, we collected a control group of 372 patients, 1:2 matched with the case group by the diagnosis time of EC, sex, marriage, and race. Patients in the control group followed the same inclusion and exclusion criteria as above but didn’t develop esophageal fistula. The included patients were divided into training set (n = 390) and validation set (n = 168) randomly. Specifically, We applied the method of simple randomization to separate the whole dataset into training and validation sets using random numbers generated by the computer.
Clinical Data Collection
We collected data from medical records using a standardized questionnaire about general, diagnostic, therapeutic and esophageal fistula data. Specifically, general parameters include gender, age at initial diagnosis, Eastern Cooperative Oncology Group performance status (ECOG PS) score, Body Mass Index (BMI), history of smoking, history of drinking, history of hypertension, history of diabetes, history of coronary heart disease and eating obstruction. Diagnostic parameters include tumor stage (T4), node stage (N2-3), stage, tumor site, longitudinal length of lesions, pathological and general type. Therapeutic parameters consist of chemotherapy, radiotherapy, target therapy and serum albumin and cholesterol. Esophageal fistula parameters include fistula type and therapy of fistula. The details are given in Table 1.
Image Acquisition
All patients underwent esophagoscopy, esophagogram and contrast-enhanced CT scan of neck, chest, and abdomen before treatment. We collected pre-treatment CT imaging and diagnostic CT of esophageal fistula. Intravenous contrast enhancement was used for all patients. The CT-scans were acquired by SOMATOM Definition AS (Siemens Healthineers) using a tube voltage of 120 kVp, a tube current of 200 mAs, a detector of 64×0.625 mm and a beam pitch of 1.5. Esophageal tumor boundaries on all 558 pre-treatment CT imaging were manually delineated with reference to esophagoscopy, barium meal or PET-CT in mediastinal window twice using 3D-Slicer by two experienced radiologists separately to reduce the deviation. For patients with satellite tumors, only the primary tumor or the tumor that caused esophageal fistula was appreciated.
Deep Learning Neural Network
To extract radiographic features from CT, we developed an attentional multi-view multi-scale CNN model (AMM-CNN). The inputs of the network were nine views of panels where there are patches of contextual CT, segmented tumor and neighboring structures in each view. To extract nine views and patches, CT images were firstly resampled to a voxel size of 1×1×1 mm3. A 200×200×200 mm3 cube was defined as located at the center of manually segmented tumor volume. We used its transverse, sagittal, coronal and six diagonal planes as nine views (Figure 1). The contextual patch was defined as a 2D slice in a view from the CT cube, which represented the contextual information of the tumor and its neighboring environment. The tumor patch was extracted from the cube of the segmented tumor volume, providing an explicit shape of tumor and boundary information. To generate anatomical surrounding patch, the pixels inside the tumor were set as zero on contextual patch.
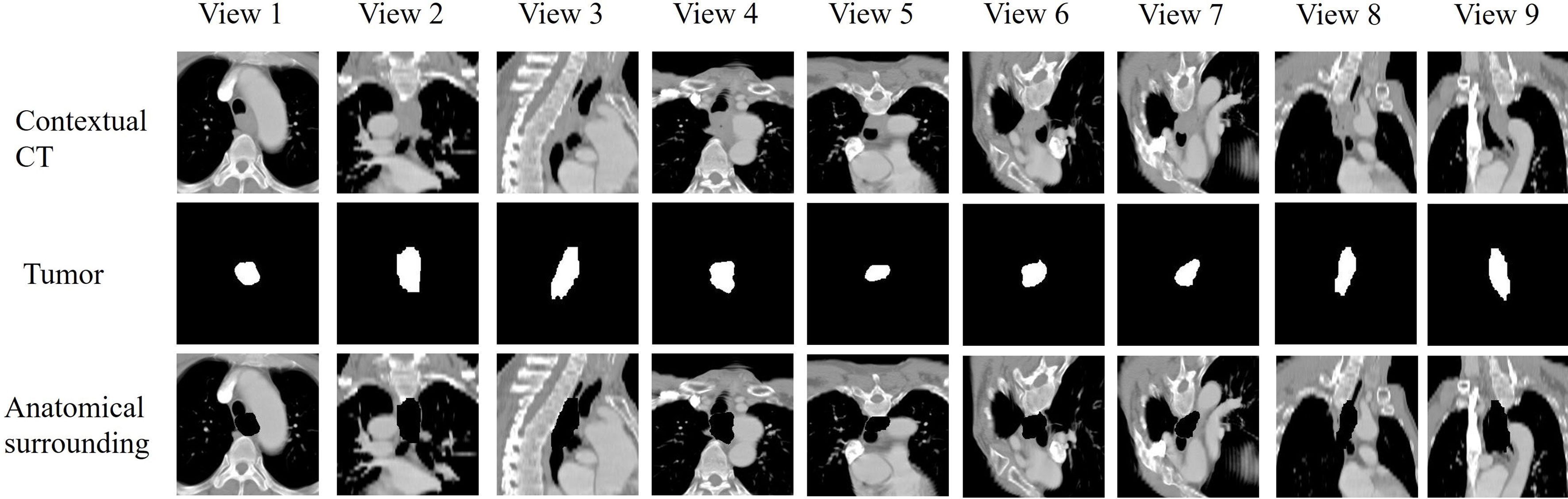
Figure 1 The nine views of planes we extracted. The nine views included transverse, sagittal, coronal and six diagonal planes.
Clinical records were fed into a neural network for high-level representation extraction. Finally, the radiographic features and clinal factor representation are associated with a fully connected layer for patient-level risk prediction using SoftMax classifier.
Performance Evaluation
The performance of the proposed risk prediction model was validated by comparing it with the risk prediction model using CT images alone and clinical records data alone.
Evaluation measures included C-index, sensitivity, and specificity. Given true positive (TP), false negative (FN), true negative (TN), and false positive (FP) numbers, sensitivity and specificity are obtained as sensitivity = TP/ (TP+FN), specificity = TN/ (TN+FP).
Results
Patient Characteristics
691 patients developed esophageal fistula during the study period. 413 had complete pre-treatment CT imaging. All perforations were developed at the location where the tumor invaded the esophagus. After excluding 227 patients with postoperative anastomotic fistulas who had surgical operations, 186 patients were finally enrolled in the case group. 372 controls never received esophageal operation matching the case cases. The detailed workflow is given in Figure 2. During the cross-validation process, in each round, all the patients were divided into a training set (randomly selected 130 pairs of positive patients and the controls) and a testing set (remaining 56 pairs of positive and controls) randomly.
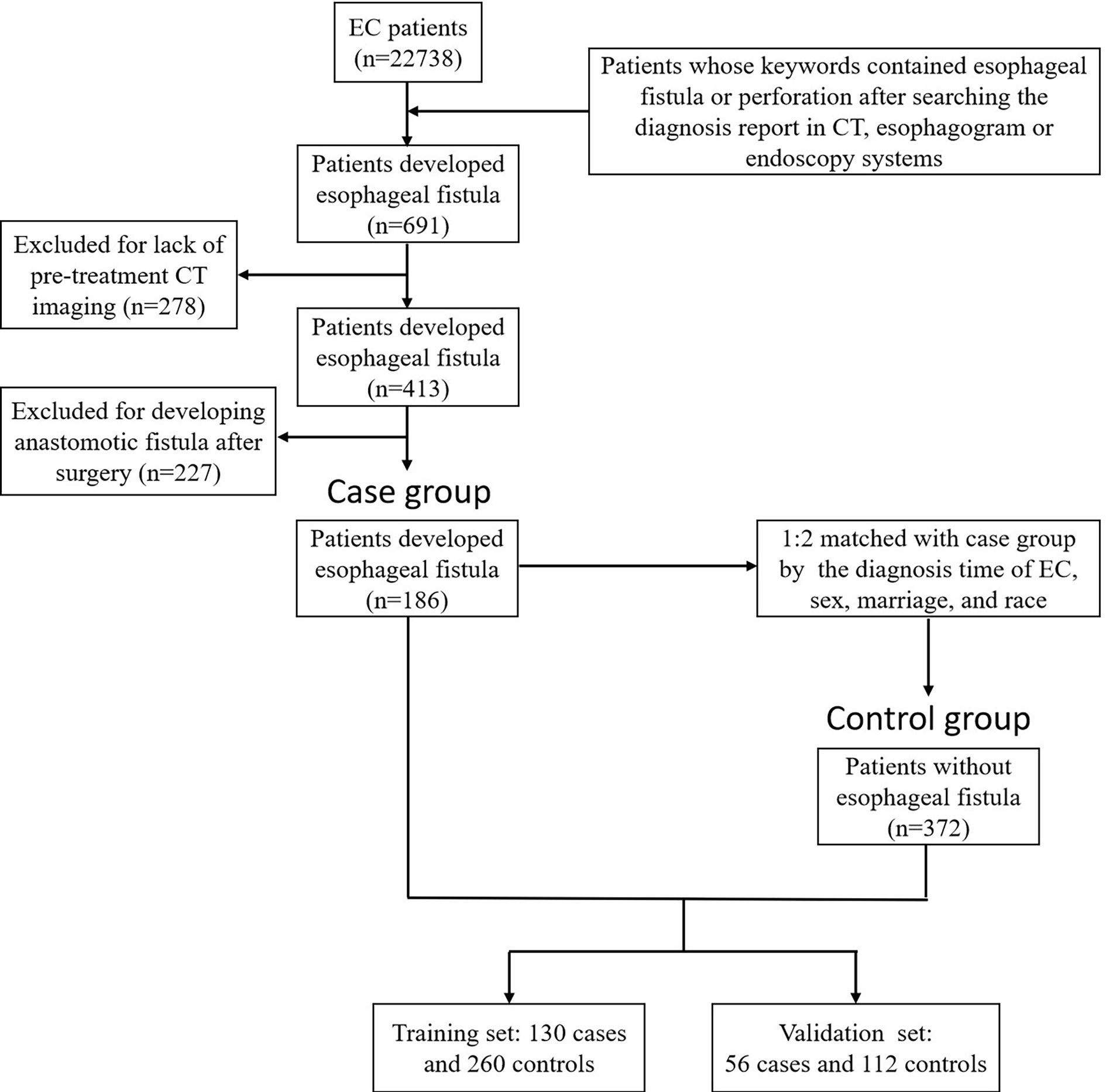
Figure 2 The overall workflow of patients. We retrospectively screened 22738 patients, and finally 186 were enrolled in the case group and 372 in the control group. All patients were randomly divided into 70% (training set) and 30% (validation set). Key words esophageal fistula or perforation, and esophageal cancer were set in the imaging system. After excluding duplicate patients, a total of 691 patients with esophageal fistula were collected. Then, patients with lack of diagnostic CT (n=278) and with postoperative anastomotic leakage (n=227) were excluded. Finally, 186 esophageal fistula patients were enrolled.
Among all 558 eligible patients, 468 (83.9%) are male and 90 (16.1%) are female. The median age is 61 (range 41-85) in the case group and 64 (range 37-89) in the control group separately. Patients with squamous carcinoma predominated account for 93.2% where most of them had stage III EC (52.2%) with T3 (63.6%) or N1 (40.1%) disease. Before developing perforation, the proportions of patients who received chemotherapy or radiotherapy were 71.5% and 54.3% respectively, while 45.7% of patients received both of them, and 12.4% received concurrent chemoradiotherapy. Besides, 37 (19.9%) patients developed esophageal fistula before treatment. The median interval time from baseline CT to the diagnosis of esophageal fistula was 5 days (3-9 days). The interval time between the development of esophageal fistula and the diagnosis of esophageal cancer ranged from 3 to 1401 days with a median value of 72 days. The median survival time after esophageal fistula is 2.9 months.
In the case group, 90 patients (48.4%) had fistula formation to the trachea or bronchus, 91 patients (48.9%) had fistula formation to the mediastinum, and two patients (1.1%) and one patient (0.5%) had fistula formation to the pleural cavity and the arteria, respectively. Two patients developed two kinds of fistula simultaneously. After the development of fistula, most patients received nutritional support. Meanwhile, some of the patients accepted nutrient canal (34.9%), esophageal stent (31.7%), gastrostomy (7.5%), and radical resection (0.5%). Conservative treatment represents only intravenous nutrition, without nutrition tubes or gastrostomy. Of all 558 patients, no patient was placed with stent before treatment or received intraluminal radiotherapy. The esophageal fistula characteristics are listed in Table 2.
Correlation Between Clinical Data and the Esophageal Fistula
In univariate logistic regression analysis, there are significant differences between patients with and without fistula in age, ECOG PS score, serum albumin, T4 stage, N stage, stage, longitudinal length of lesions, general type, and treatment-related parameters. All significant factors were further included in the multiple regression analysis. Age, ECOG PS score, serum albumin, T4 stage, N stage, general type, chemotherapy, total dose of radiotherapy, and radiotherapy range (metastatic lymph nodes) are independent risk factors for esophageal fistula. The detailed results are shown in Table 3.
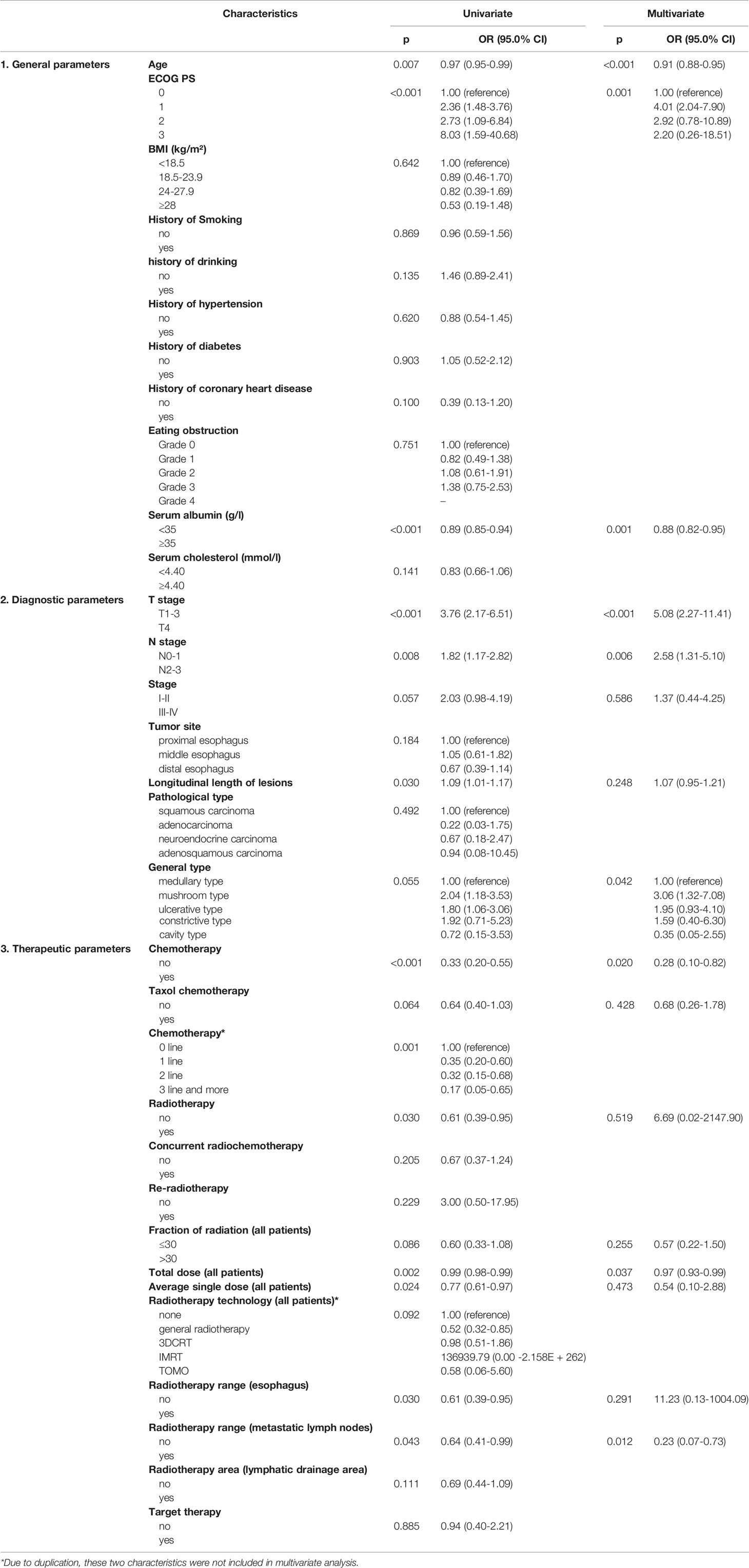
Table 3 Univariate and multivariate logistic regression analysis of clinical characteristics in the training set.
Deep Learning Prediction Model Implementation
The detailed architecture of AMM-CNN is given in Figure 3. AMM-CNN adopts the architecture of AlexNet (13) for image feature extraction and has an attentional fusion module to adaptively integrate multi-view multi-level image features. Given contextual CT, tumors, and surrounding tissues from 9 views, AMM-CNN generates 512 radiographic features. 20 clinical representations were learnt by the NN from input clinical records.
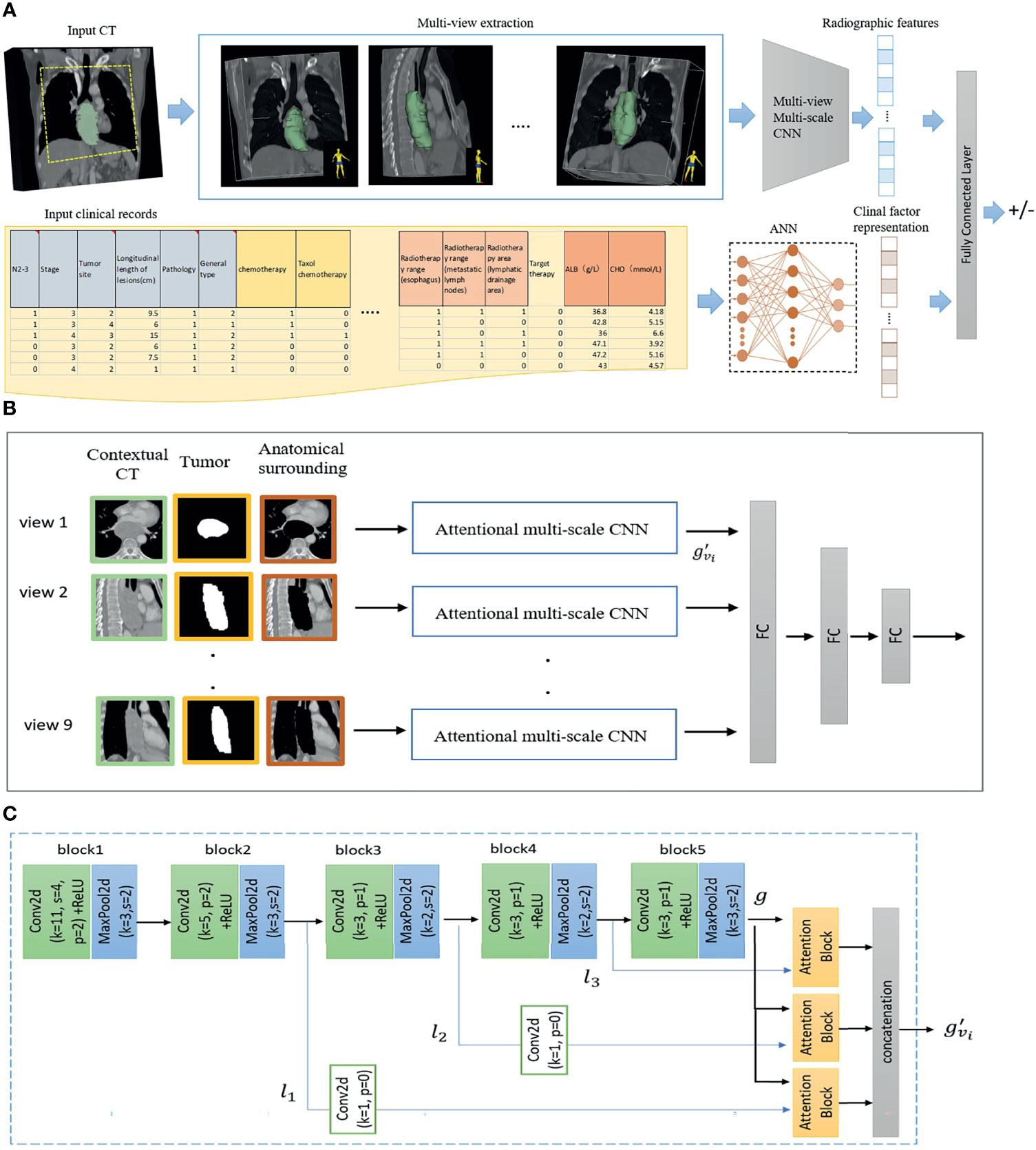
Figure 3 The proposed risk prediction model (A) Given an input CT and segmented tumor, 9 views of planes are extracted. Contextual CT, tumor and anatomical surrounding patches of each view are sent to a Multi-view Multi-scale CNN model for radiographic feature extraction. For the input clinical records, an artificial neural network (ANN) is used to extract clinical factor representations. Finally, radiographic and clinical features are fused by a fully connected layer for esophageal fistula prediction. Architecture of Multi-view Multi-scale CNN is given in (B). Contextual CT, tumor and anatomical surrounding patches extracted from each view are sent to (C) attentional multi-scale CNN. Multi-scale features are extracted from the second, third and fourth blocks in the CNN, and adaptively fused by attention blocks.
To improve the learning effectiveness, data augmentation was performed, including pixel shifting and rotation for the training set. As there were imbalanced positive and negative cases, shifting operations of -10, -5, 0, + 5, +10 pixels along the x and y-axis and rotations of -10, +10 degrees were performed for positive cases, resulting in 9750 positive samples. For negative training cases, 9360 negative samples were obtained after shifting operations of -5, 0, + 5, +10 along x and -5, 0, + 5 along y-axis, and rotations of -10, +10 degrees.
Combining clinical features and CT imaging, deep learning achieved a C-index of 0.921 in the internal validation and 0.901 in the external validation, which outperformed CT imaging alone (internal validation: 0.902; external validation: 0.857) and clinical data alone (internal validation: 0.855; external validation: 0.780). The sensitivity was 0.835, and specificity was 0.918. The integrative model produced higher predictive performance than models using single modality data. For the clinical characteristics, the C-index obtained by deep learning is 0.780, which is better than the traditional logistics regression model (internal validation: 0.823, external validation: 0.734).
Interpretability of the Model
To study the interpretability of the model, we draw the attention map to explain the focus of the neural network on CT images. As shown in Figure 4, hotter areas of the attention map represent the tissues predicted by the algorithm that has a higher impact on the formation of esophageal fistula. Our results show that there were usually two locations that receive more attention. One is the border of the tumor, and the other is the hypoechoic area inside the tumor. The visual interpretation further proved the effectiveness of the model.
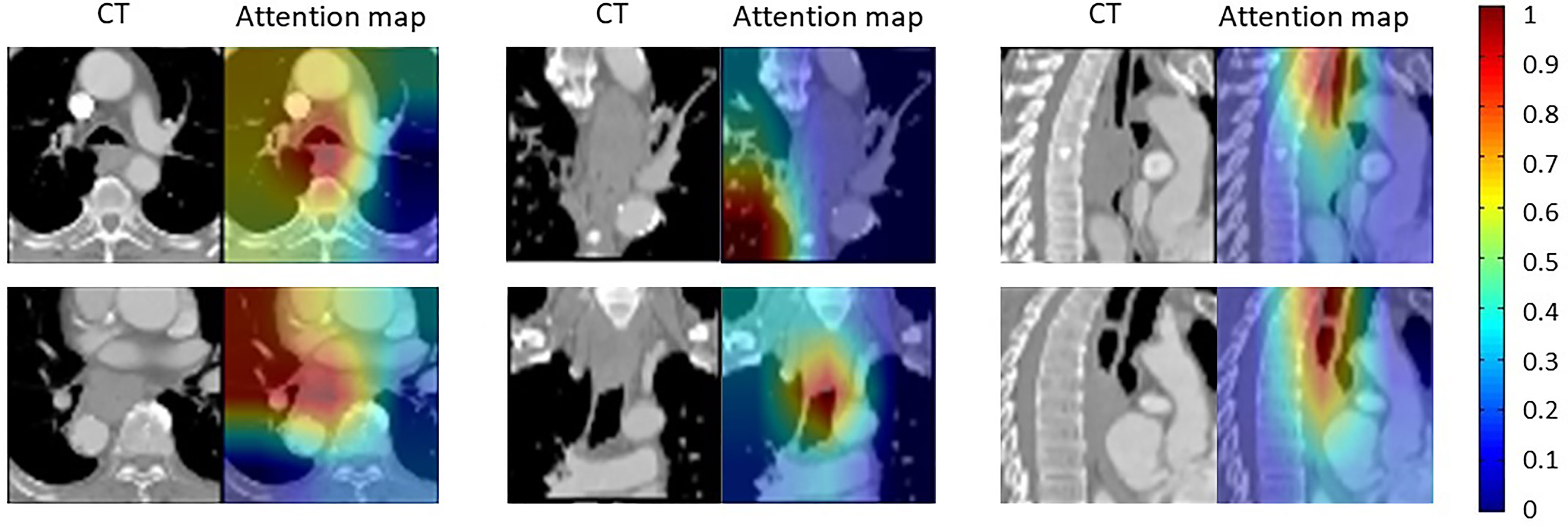
Figure 4 Visualization of attention maps of six patient examples. The attention heatmap shows the risk model’s focus on esophageal fistula prediction. The hotter areas indicate that the tissues have greater impact on esophageal fistula formation. As shown, the tumor boundaries and the hypoechoic area inside the tumor are more concerned.
Discussion
Esophageal fistula is a fatal complication of EC. Therefore, a risk prediction model integrating CT imaging and clinical features is worth investigation. In this study, we used the deep learning method to comprehensively analyze the influence of various parameters on the esophageal fistula, including clinical parameters such as stage, treatment, and CT imaging. Deep learning models can directly learn patient characteristics from raw data or imaging without feature selection or design (14). Therefore, more complete data can be included for analysis. To our knowledge, this is the first deep learning model that uses different types of parameters for esophageal fistula prediction.
The prediction performance of integrative deep learning model is better than that of a single parameter model (C-index: 0.901 vs 0.857, 0.780). Because deep learning algorithms can integrate clinical parameters and CT images well. Deep learning is very suitable for the analysis of multi-domain parameters, such as the fusion of histopathological images and genomic data (15). The integrative model contains more information than a single model and can achieve better prediction performance.
Deep learning model is also superior than traditional logistics regression. The first reason is that intuitive tumor information can be obtained from CT imaging, including the tumor size, density and invasion degree of surrounding tissues, which are all related to the esophageal fistula. The second reason is that the nomogram was established in previous studies to predict esophageal fistula (16). However, the nomogram was developed based on logistics regression analysis, which couldn’t capture the nonlinear relationship between risk factors and esophageal fistula, and the number of risk factors included was relatively small. Therefore, the performance of this nomogram is limited. Our prediction model provides an end-to-end data-driven trainable approach to learn the mapping from input images to output risk grades. The mapping serves as a feature extractor, which is automatically learned during the training process. As a result, the extractor is more general and adjustable when compared with explicitly defined hand-crafted features in previous research (17). In addition, a large volume of training data and deep learning technique equips our model the ability to extract more in-depth features and underlying image information. Therefore, deep learning models are expected to replace logistics regression analysis.
Deep learning has a certain interpretability for the image analysis (18). This study shows that the tumor boundaries and the hypoechoic area inside the tumor have the greatest predictive significance for esophageal fistula. The tumor boundaries are adjacent to the normal tissue, which can represent the status of tumor invasion. The hypoechoic area inside the tumor is related to the tumor growth rate and malignancy. This proves that our model is reasonable.
Clinicians can use this model to evaluate esophageal fistula risk before or during treatment. For high-risk patients, the dose of chemotherapy or radiotherapy can be appropriately reduced with enhanced nutritional support. In addition, the examination should also be taken more frequently. Although it is generally believed that one of the adverse reactions of radiotherapy is esophageal fistula, some studies believe that radiotherapy can promote the healing of esophageal fistula, and further research on the frequency and dose of radiotherapy is needed.
This study has several limitations. First, deep learning has poor interpretability of clinical parameters, and it is difficult to analyze which clinical parameters have a greater impact on the esophageal fistula. Second, the study is a single-center study. Data from other regions and centers are required for further validation.
Conclusion
In this study, we developed a deep learning model to integrate CT imaging and clinical information for esophageal fistula prediction in EC patients. We suggest this study and the developed model can facilitate individualized treatment, leading to maximized therapeutic gain.
Data Availability Statement
The raw data supporting the conclusions of this article will be made available by the authors, without undue reservation.
Ethics Statement
The studies involving human participants were reviewed and approved by Institutional Review Board of Shandong Cancer Hospital and Institute, Shandong First Medical University, and Shandong Academy of Medical Sciences. The ethics committee waived the requirement of written informed consent for participation.
Author Contributions
YX, TD, and LW contributed to the conception and design of the manuscript. BZ, BF, and WL collected the data and CT images. YX and HC performed data analysis and drafted the manuscript. SW, XS, and JY revised the original manuscript. All authors contributed to the article and approved the submitted version.
Funding
This study was funded by the following grant: Natural Science Foundation of Shandong Province (Grant No. ZR2019LZL012), the Innovation Project of Shandong Academy of Medical Sciences (2019-04), and the Academic Promotion Program of Shandong First Medical University (2019ZL002).
Conflict of Interest
The authors declare that the research was conducted in the absence of any commercial or financial relationships that could be construed as a potential conflict of interest.
Publisher’s Note
All claims expressed in this article are solely those of the authors and do not necessarily represent those of their affiliated organizations, or those of the publisher, the editors and the reviewers. Any product that may be evaluated in this article, or claim that may be made by its manufacturer, is not guaranteed or endorsed by the publisher.
Acknowledgments
The authors thank all patients who participated in the study.
References
1. Lagergren J, Smyth E, Cunningham D, Lagergren P. Oesophageal Cancer. Lancet (London England) (2017) 390(10110):2383–96. doi: 10.1016/S0140-6736(17)31462-9
2. McGuire S. World Cancer Report 2014. Geneva, Switzerland: World Health Organization, International Agency for Research on Cancer, WHO Press, 2015. Adv Nutr (Bethesda Md) (2016) 7(2):418–9. doi: 10.3945/an.116.012211
3. Ferlay J, Colombet M, Soerjomataram I, Mathers C, Parkin DM, Pineros M, et al. Estimating the Global Cancer Incidence and Mortality in 2018: GLOBOCAN Sources and Methods. Int J Cancer (2019) 144(8):1941–53. doi: 10.1002/ijc.31937
4. Guan X, Liu C, Zhou T, Ma Z, Zhang C, Wang B, et al. Survival and Prognostic Factors of Patients With Esophageal Fistula in Advanced Esophageal Squamous Cell Carcinoma. Biosci Rep (2020) 40(1):BSR20193379. doi: 10.1042/BSR20193379
5. Zhang Y, Li Z, Zhang W, Chen W, Song Y. Risk Factors for Esophageal Fistula in Patients With Locally Advanced Esophageal Carcinoma Receiving Chemoradiotherapy. OncoTargets Ther (2018) 11:2311–7. doi: 10.2147/OTT.S161803
6. Kawakami T, Tsushima T, Omae K, Ogawa H, Shirasu H, Kito Y, et al. Risk Factors for Esophageal Fistula in Thoracic Esophageal Squamous Cell Carcinoma Invading Adjacent Organs Treated With Definitive Chemoradiotherapy: A Monocentric Case-Control Study. BMC Cancer (2018) 18(1):573. doi: 10.1186/s12885-018-4486-3
7. Paul G, Bohle W, Zoller W. Risk Factors for the Development of Esophagorespiratory Fistula in Esophageal Cancer. J Gastrointest Liver Dis JGLD (2019) 28(3):265–70. doi: 10.15403/jgld-271
8. Larue R, Klaassen R, Jochems A, Leijenaar RTH, Hulshof M, van Berge Henegouwen MI, et al. Pre-Treatment CT Radiomics to Predict 3-Year Overall Survival Following Chemoradiotherapy of Esophageal Cancer. Acta Oncol (Stockholm Sweden) (2018) 57(11):1475–81. doi: 10.1080/0284186X.2018.1486039
9. Wu L, Yang X, Cao W, Zhao K, Li W, Ye W, et al. Multiple Level CT Radiomics Features Preoperatively Predict Lymph Node Metastasis in Esophageal Cancer: A Multicentre Retrospective Study. Front Oncol (2019) 9:1548. doi: 10.3389/fonc.2019.01548
10. Luo HS, Huang SF, Xu HY, Li XY, Wu SX, Wu DH. A Nomogram Based on Pretreatment CT Radiomics Features for Predicting Complete Response to Chemoradiotherapy in Patients With Esophageal Squamous Cell Cancer. Radiat Oncol (London England) (2020) 15(1):249. doi: 10.1186/s13014-020-01692-3
11. Jansen T, Geleijnse G, Van Maaren M, Hendriks MP, Ten Teije A, Moncada-Torres A. Machine Learning Explainability in Breast Cancer Survival. Stud Health Technol Inf (2020) 270:307–11. doi: 10.3233/SHTI200172
12. Bi WL, Hosny A, Schabath MB, Giger ML, Birkbak NJ, Mehrtash A, et al. Artificial Intelligence in Cancer Imaging: Clinical Challenges and Applications. CA: Cancer J Clin (2019) 69(2):127–57. doi: 10.3322/caac.21552
13. Krizhevsky A, Sutskever I, Hinton GE. Imagenet Classification With Deep Convolutional Neural Networks. Commun ACM (2017) 60(6):84–90. doi: 10.1145/3065386
14. Naik N, Madani A, Esteva A, Keskar NS, Press MF, Ruderman D, et al. Deep Learning-Enabled Breast Cancer Hormonal Receptor Status Determination From Base-Level H&E Stains. Nat Commun (2020) 11(1):5727. doi: 10.1038/s41467-020-19334-3
15. Hao J, Kosaraju SC, Tsaku NZ, Song DH, Kang M. PAGE-Net: Interpretable and Integrative Deep Learning for Survival Analysis Using Histopathological Images and Genomic Data. Pac Symp Biocomput Pac Symp Biocomput (2020) 25:355–66. doi: 10.1142/9789811215636_0032
16. Xu Y, Wang L, He B, Li W, Wen Q, Wang S, et al. Development and Validation of a Risk Prediction Model for Radiotherapy-Related Esophageal Fistula in Esophageal Cancer. Radiat Oncol (London England) (2019) 14(1):181. doi: 10.1186/s13014-019-1385-y
17. Liu T, Guo Q, Lian C, Ren X, Liang S, Yu J, et al. Automated Detection and Classification of Thyroid Nodules in Ultrasound Images Using Clinical-Knowledge-Guided Convolutional Neural Networks. Med Image Anal (2019) 58:101555. doi: 10.1016/j.media.2019.101555
Keywords: esophageal cancer, esophageal fistula, radiomics, deep learning, prediction model
Citation: Xu Y, Cui H, Dong T, Zou B, Fan B, Li W, Wang S, Sun X, Yu J and Wang L (2021) Integrating Clinical Data and Attentional CT Imaging Features for Esophageal Fistula Prediction in Esophageal Cancer. Front. Oncol. 11:688706. doi: 10.3389/fonc.2021.688706
Received: 31 March 2021; Accepted: 05 November 2021;
Published: 23 November 2021.
Edited by:
Zsolt Kovács, Sciences and Technology of Târgu Mureş, RomaniaCopyright © 2021 Xu, Cui, Dong, Zou, Fan, Li, Wang, Sun, Yu and Wang. This is an open-access article distributed under the terms of the Creative Commons Attribution License (CC BY). The use, distribution or reproduction in other forums is permitted, provided the original author(s) and the copyright owner(s) are credited and that the original publication in this journal is cited, in accordance with accepted academic practice. No use, distribution or reproduction is permitted which does not comply with these terms.
*Correspondence: Jinming Yu, sdyujinming@163.com; Linlin Wang, wanglinlinatjn@163.com