- 1Department of Onco-Hematology Azienda Ospedaliera (AO) Cosenza, Biotechnology Research Unit, Cosenza, Italy
- 2Department of Hematology and Bone Marrow Transplant Unit, Augusta Victoria Hospital, Jerusalem, Israel
- 3Centro Nazionale Ricerca Istituto di Fisiologia Clinica (CNR-IFC), Research Unit of Reggio Calabria, Reggio Calabria, Italy
- 4Division of Hematology, Department of Translational Medicine, University of Eastern Piedmont, Novara, Italy
- 5Hematology, Department of Translational and Precision Medicine, ‘Sapienza’ University, Rome, Italy
- 6Department of Onco-Hematology AO Cosenza, Hematology Unit AO of Cosenza, Cosenza, Italy
- 7Mutagenesis and Cancer Prevention Unit, IRCCS Ospedale Policlinico San Martino, Genoa, Italy
- 8Division of Hematology, Policlinico, Department of Surgery and Medical Specialties, University of Catania, Catania, Italy
- 9Hematology, Oncology Institute of Southern Switzerland, Bellinzona, Switzerland
- 10Molecular Pathology Unit, IRCCS Ospedale Policlinico San Martino, Genova, Italy
- 11Department of Experimental Medicine, University of Genoa, Genoa, Italy
- 12Hematology Unit, Fondazione IRCCS Ca’ Granda, Ospedale Maggiore Policlinico, Milan, Italy
- 13Department of Hematology, Hospital Clinic, Institut d’Investigacions Biomèdiques August Pi i Sunyer (IDIBAPS), Barcelona, Spain
- 14Department of Oncology and Hemato-Oncology, University of Milan, Milan, Italy
The prognostic role of lymphocyte doubling time (LDT) in chronic lymphocytic leukemia (CLL) was recognized more than three decades ago when the neoplastic clone’s biology was almost unknown. LDT was defined as the time needed for the peripheral blood lymphocyte count to double the of the initial observed value. Herein, the LDT prognostic value for time to first treatment (TTFT) was explored in our prospective O-CLL cohort and validated in in two additional CLL cohorts. Specifically, newly diagnosed Binet stage A CLL patients from 40 Italian Institutions, representative of the whole country, were prospectively enrolled into the O-CLL1-GISL protocol (clinicaltrial.gov identifier: NCT00917540). Two independent cohorts of newly diagnosed CLL patients recruited respectively at the Division of Hematology in Novara, Italy, and at the Hospital Clinic in Barcelona, Spain, were utilized as validation cohorts. In the training cohort, TTFT of patients with LDT >12 months was significantly longer related to those with a shorter LDT. At Cox multivariate regression model, LDT ≤ 12 months maintained a significant independent relationship with shorter TTFT along with IGHV unmutated (IGHVunmut) status, 11q and 17p deletions, elevated β2M, Rai stage I-II, and NOTCH1 mutations. Based on these statistics, two regression models were constructed including the same prognostic factors with or without the LDT. The model with the LTD provided a significantly better data fitting (χ2 = 8.25, P=0.0041). The risk prediction developed including LDT had better prognostic accuracy than those without LDT. Moreover, the Harrell’C index for the scores including LDT were higher than those without LDT, although the accepted 0.70 threshold exceeded in both cases. These findings were also confirmed when the same analysis was carried out according to TTFT’s explained variation. When data were further analyzed based on the combination between LDT and IGHV mutational status in the training and validation cohorts, IGHVunmut and LDT>12months group showed a predominant prognostic role over IGHVmut LTD ≤ 12 months (P=0.006) in the O-CLL validation cohort. However, this predominance was of borden-line significance (P=0.06) in the Barcelona group, while the significant prognostic impact was definitely lost in the Novara group. Overall, in this study, we demonstrated that LDT could be re-utilized together with the more sophisticated prognostic factors to manage the follow-up plans for Binet stage A CLL patients.
Introduction
The heterogeneous course and outcome of chronic lymphocytic leukemia (CLL) are associated with clinical and laboratory parameters as well as the molecular and cytogenetic complexity of the leukemic clone can contribute to setting a prognosis (1–31). In the past, attention was centered primarily on the predictors of general outcome, intended as overall survival. In contrast, more recently, the focus also has included the definition of time to first treatment (TTFT). According to the current guidelines (32, 33), CLL is treated at progression. Many patients and clinicians often see the start of therapy as a partition between a healthy and a disease condition. Besides, an accurate prediction of the TTFT permits setting the appropriate follow-up strategy. Several methodologies have been proposed to predict the patient’s course and outcome, as well as the TTFT. Although the availability of a battery of cellular/molecular markers has opened the way to an always more refined prognostic stratification of patients, the current practice’s reality indicates difficulties in carrying out several sophisticated features, often confined to a research setting (3). Thus, simplifications introducing inexpensive tests suitable for the clinical setting would be more than welcome, and such prognostic indexes would have a high likelihood of broad applicability.
The breakthrough of novel biologic variables has led to several prognostic indexes to weigh TTFT in early-stage (Binet A) CLL patients (1, 2, 34–52). Indeed, over the past few years, there has been a great effort to use novel molecular markers in prognostic modeling. Yet, questions about their usefulness to improve clinical prediction have been recently debated (53). On the other hand, as the concern for novel molecular markers detected by cutting-edge technologies has soared, performance measures for statistically quantifying their prognostic added value have risen accordingly (52).
The German study group (53) has recently established the independent prognostic value of lymphocyte doubling time (LDT) in Binet stage A patients after more than 50 years following the recognition of a correlation between the lymphocyte proliferation pattern and clinical outcome in CLL (54). LDT, defined as the period needed for the peripheral blood lymphocyte count to reach a double value of that corresponding to the initial observation, is a simple parameter that is useful in arriving at an accurate prognosis in CLL. Whereas a high LDT (greater than 12 months) identifies a population with an excellent prognosis, a low LDT (less than or equal to 12 months) LDT predicts rapid disease progression in patients in the early clinical stages (53, 55, 56). The raising question is whether the re-introduction of LDT among the last generation prognostic factors could help determine the follow-up strategies for an early-stage patient, possibly improving or maintaining the prediction power of more hi-tech markers such as the IGHV gene status (51, 57).
Herein, we investigated the LDT predictive value for TTFT in our prospective O-CLL cohort. The results of LDT prognostication power in the O-CLL training cohort were validated in two additional CLL cohorts.
Materials and Methods
Lymphocyte Doubling Time
LDT was evaluated at diagnosis, also utilizing lymphocyte counts antecedent the enrollment, if available, and defined as the time needed for the peripheral blood lymphocyte count to reach a value double that of the initial observation (52, 55, 56). LDT was calculated as reported by Hoechstetter et al. (52) through a linear regression based on four blood lymphocyte measurements, each at an interval of a minimum of four weeks from the precedent one in no more than six months before enrollment.
O-CLL Training Cohort
Newly diagnosed CLL patients from 40 Italian Institutions were prospectively enrolled within 12 months of diagnosis into the O-CLL1-GISL protocol (clinicaltrial.gov identifier: NCT00917540). The ethics committees from each participating center approved this study. Informed consent was obtained from all subjects. Recruitment began in January 2007 and terminated in January 2012. According to the guidelines (32, 33), treatment was decided uniformly for all participating centers based on documented progressive and symptomatic disease. The present analysis was carried out in 498 out of 523 accrued cases where LDT was available.
All patients from the O-CLL cohort were studied for CD38, and ZAP-70 expression, IGHV mutational status, FISH assays, and NOTCH1 and SF3B1 gene mutations as previously described (18, 28, 29, 58, 59).
The contribution of the single institutions of the training cohort is shown in Supplementary Table 1.
Validation Cohorts
An independent cohort of newly diagnosed and prospectively followed CLL patients recruited since 2001 at the Division of Hematology, Department of Translational Medicine, UPO, Novara, Italy, was utilized as a first validation cohort. The present analysis was restricted to 276, with LDT available, out of 283 cases included in a previous paper (5). All the prognostic factors required in this study (IGHV mutational status, Rai stage, β2M, 17(p) and 11(q) deletions, NOTCH1 coding gene mutation, and LDT were available for 257 cases.
A further independent cohort of newly diagnosed and prospectively followed CLL patients recruited since 2001 at Hospital Clinic, Institute of Hematology and Oncology, University of Barcelona, Spain, was utilized as an additional validation cohort. The present analysis was performed in 414 cases; 355 were included in a recent paper (51). All the prognostic factors required in this study (IGHV mutational status, RAI stage, β2M, 17(p) and 11(q) deletions, NOTCH1 coding gene mutation, and LDT) were available for 247 cases.
Statistical Analysis
TTFT analyses (including the identification of risk factors for this endpoint) were performed using the Kaplan-Meier method followed by log-rank test. The prognostic impact of specific risk factors on the outcome variable was investigated by univariate and multiple Cox regression analysis. Results are expressed as hazard ratios (HR) and 95% confidence intervals (CI). The main prognostic factor in our study was the bScore. It was calculated by deriving a weight corresponding to the regression coefficients of each prognostic factor (b) (60). The regression coefficients of the independent prognostic factors were preliminarily summed up. Then, they were divided by this sum and multiplied by 100, thus deriving a weight ranging from 0 to a given percentage, These weights were summed up on an individual basis, thus deriving a score interpretable in a prognostic scale ranging from 0 to 100% (for patients exposed to all risk factors) (60). The predictive accuracy of the prognostic models was quantified by calculating the Harrell C-index (HC-index), ranging from 0.5 to 1.0, the explained variation on the outcome (i.e., an index combining calibration and discrimination) (61), and the Akaike weights (AIC) (62). The integrated discrimination improvement (IDI) (53) was also calculated to assess the gain in prognostic accuracy provided by LDT. IDI is an index of risk re-classification that quantifies whether a new variable offers a clinically relevant improvement in prediction beyond and above provided by a model based on a previous risk prediction rule and not including the same variable.
The fittings between two nested prognostic models (including and not including LDT) were compared by the -2 log likelihood statistics (63). Data analysis was performed by STATA for Windows v.9 and SPSS Statistics v.21.
Results
O-CLL Training Cohort
In the prospective O-CLL cohort, LDT was available in 498 Binet stage A CLL cases (median 72.4 months). Seventy-seven cases (15.5%) presented a LDT ≤ 12 months. Out of the 498 patients, 177 needed treatment, with a significantly higher percentage (P<0.0001) of cases requiring therapy detected among the group with LDT ≤ 12 months (54/77, 70.1%) as compared with that with LDT>12 months (123/431, 29.2%). TTFT of patients with LDT ≤12 months was significantly shorter (HR=2.9, 95% CI 2.1–4.0, P<0.0001) compared to those with a longer LDT (Figure 1). In the same cohort, when all the correlates of outcome determined by univariate analysis (Table 1) were introduced into the same multiple Cox regression model, only IGHV unmutated (IGHVumut) genes, 11q and 17p deletions, elevated β2M, Rai stage I-II, NOTCH1 mutations and LDT ≤ 12 months maintained a significant independent relationship with shorter TTFT (Figure 2).
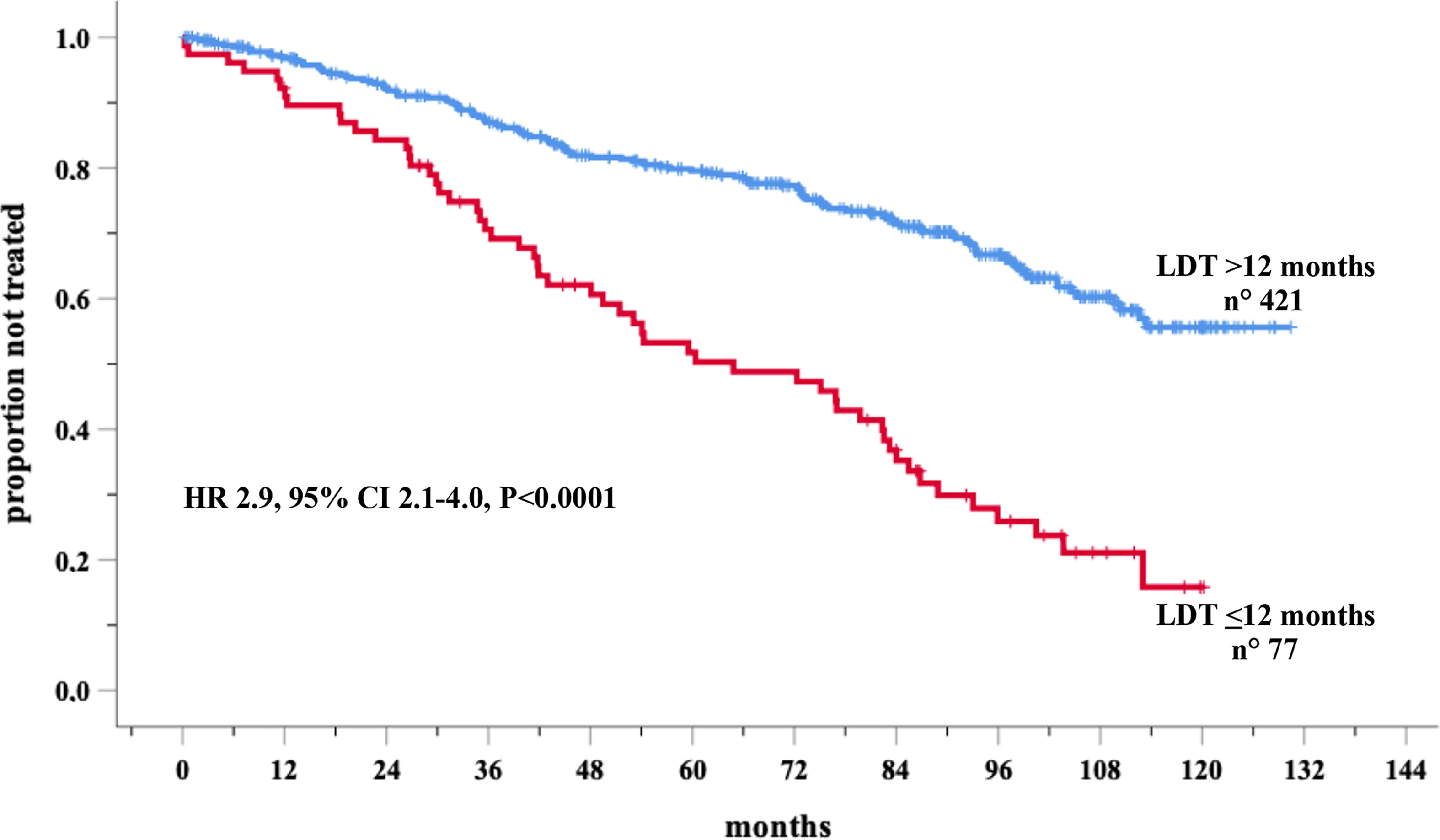
Figure 1 Kaplan-Meir curves of time to first treatment (TTFT) of patients stratified by lymphocyte doubling time (LDT) in the O-CLL training cohort.
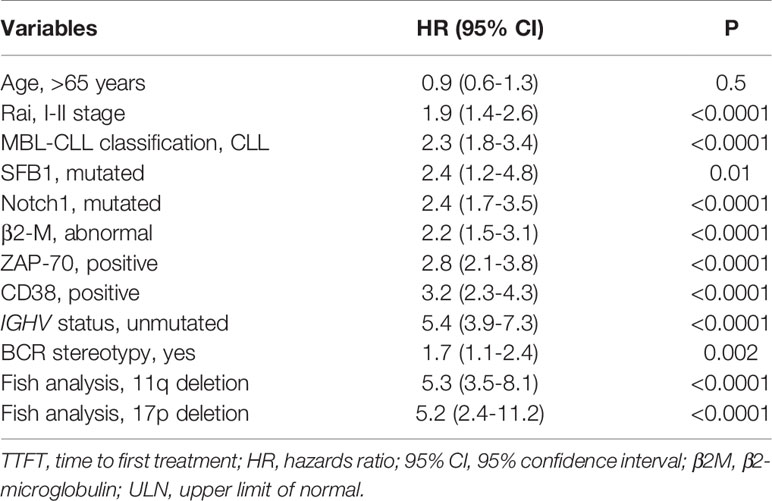
Table 1 Cox univariate analyses of several variables that significantly predict TTFT in the training O-CLL cohort.
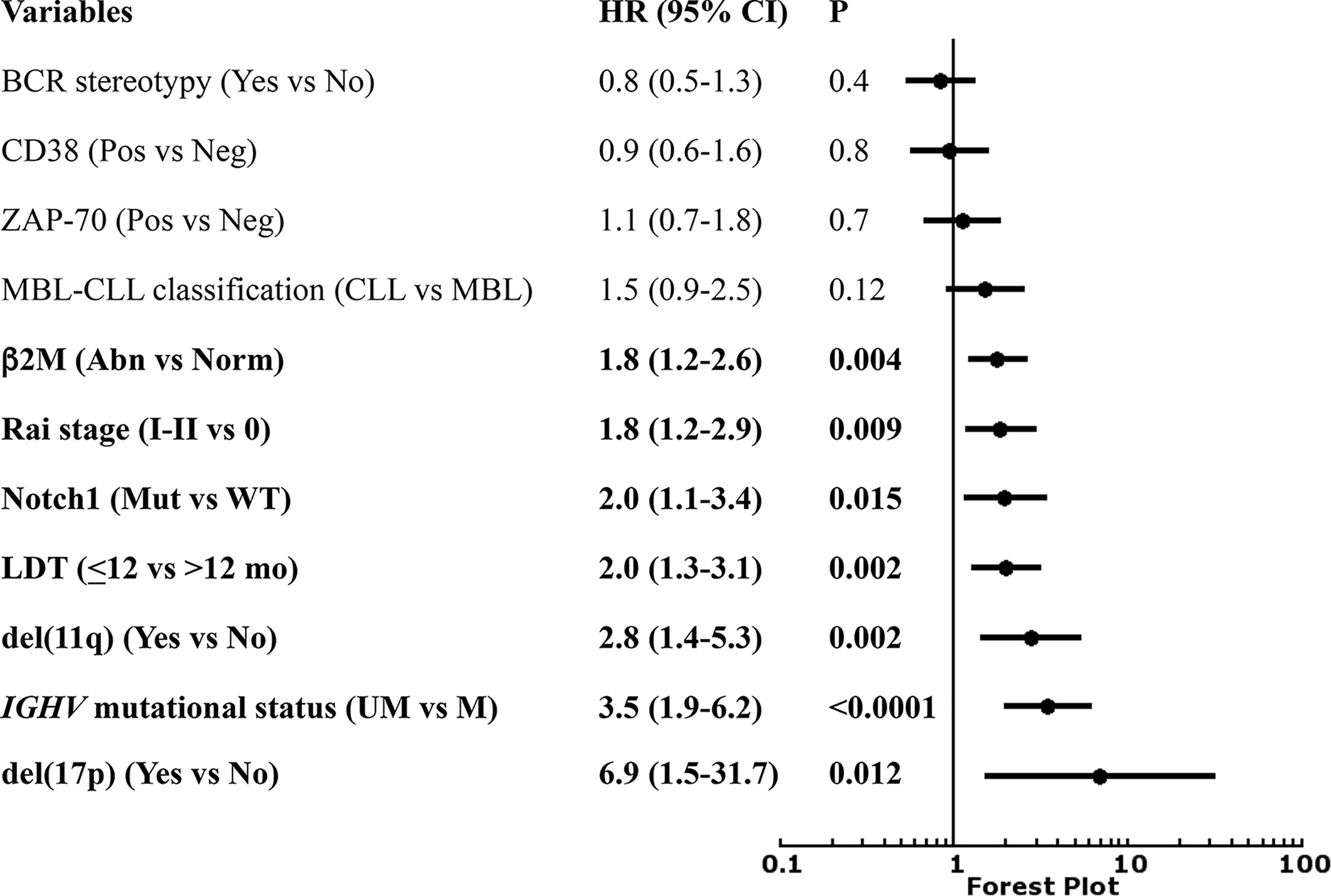
Figure 2 Forest plot of Cox multivariate analysis for time to first treatment (TTFT) according to several variables.
Starting from this analysis, two regression models were constructed, i.e., a Cox model including all significant and independent correlates of TTFT except LDT (Model 1, Table 2) and a Cox model including the same set of prognostic factors and LDT (Model 2, Table 2). Of note, Model 2 (including LDT) provided a significantly better data fitting (χ2 = 8.25, P=0.0041) than Model 1 (not including LDT). Moreover, by IDI calculations, we demonstrated that LDT increased the estimated risk of +3.8%, a result of high statistical significance (P<0.001). These analyses were carried out in 334 cases in which all the variables were available.
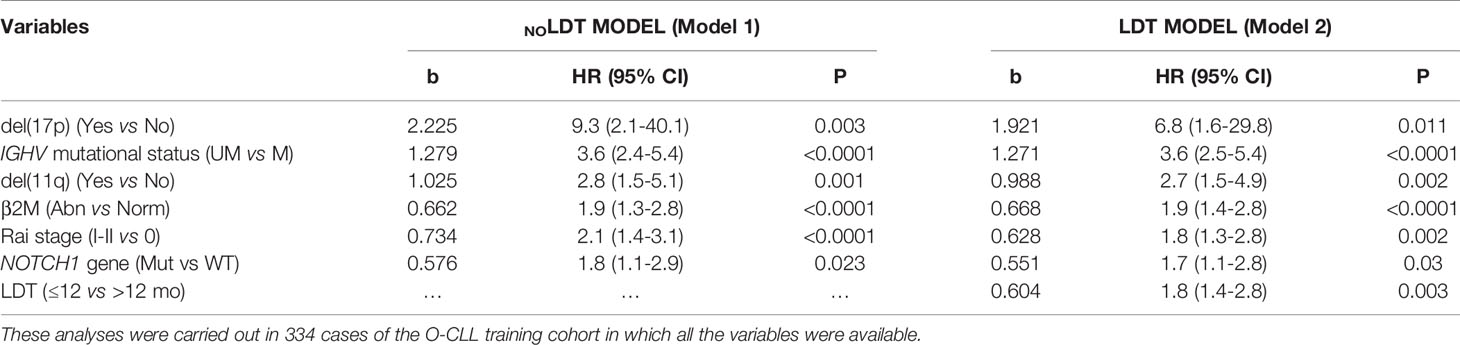
Table 2 Regression coefficients (b) derived from two multivariate models where lymphocyte doubling time (LDT) where excluded (NOLDT MODEL) or included (LDT MODEL).
Prediction Risk Scores in Training O-CLL Cohort
Based on Model 1 and Model 2, two risk prediction rules were developed, i.e. bScore LDT and bScore no LDT (Figure 3). bScore which included LDT, had better prognostic accuracy than that without LDT (Table 1, training set). Moreover, the HC-index including LDT, was higher than those without LDT (75.4 versus 74.7), although the accepted 0.70 threshold (25) exceeded in both cases (Table 3). This was also true when the same analysis was carried out according to the explained variation in TTFT, which combines the discrimination and the calibration abilities of a risk prediction rule (bScore LDT = 47.6% versus bScore no LDT = 45.0%; Table 3).
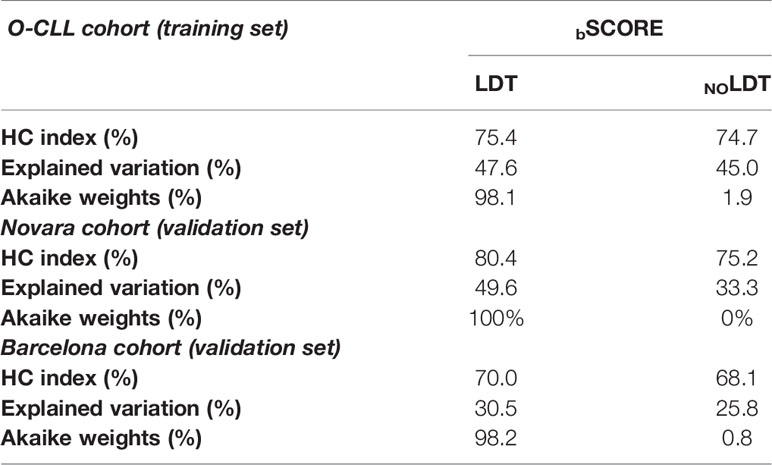
Table 3 Comparison between the NOLDT score and the LDT score for the prediction of TTFT in the O-CLL, in the O-CLL, Novara, and Barcelona cohorts by regression coefficients (BScore).
Prediction Risk Scores in Validation Novara and Barcelona Cohorts
These analyses were carried out in two validation cohorts. LDT was available in 276 and in 414 Binet stage A cases respectively in Novara and Barcelona cohorts. Eighty-six and 148 patients required therapy in the Novara and Barcelona cohorts, respectively. A significantly higher percentage of treated cases were recorded among the group with LDT ≤ 12 months in both the Novara (LDT ≤ 12 70/121, 57.9% versus LDT>12 months (16/155, 10.3%) and the Barcelona (LDT ≤ 12 months 30/39, 76.9% versus LDT>12 months cohort 119/375, 31.7%). In the Novara cohort, Cox univariate analysis showed a significantly increased risk of treatment for patients with LDT ≤12 months (155 cases) (HR=6.1, 95% CI 3.6–10.6, P<0.0001) compared to those with a longer LDT (121 patients) (Figure 4A). Similar results were detected in the Barcelona validation set, in which the 39 cases with LDT ≤12 months showed a risk of being treated 7.4 times higher (95% CI 4.9–11.2, P<0.0001) than the 375 cases with a longer LDT (HR=1) (Figure 4B).
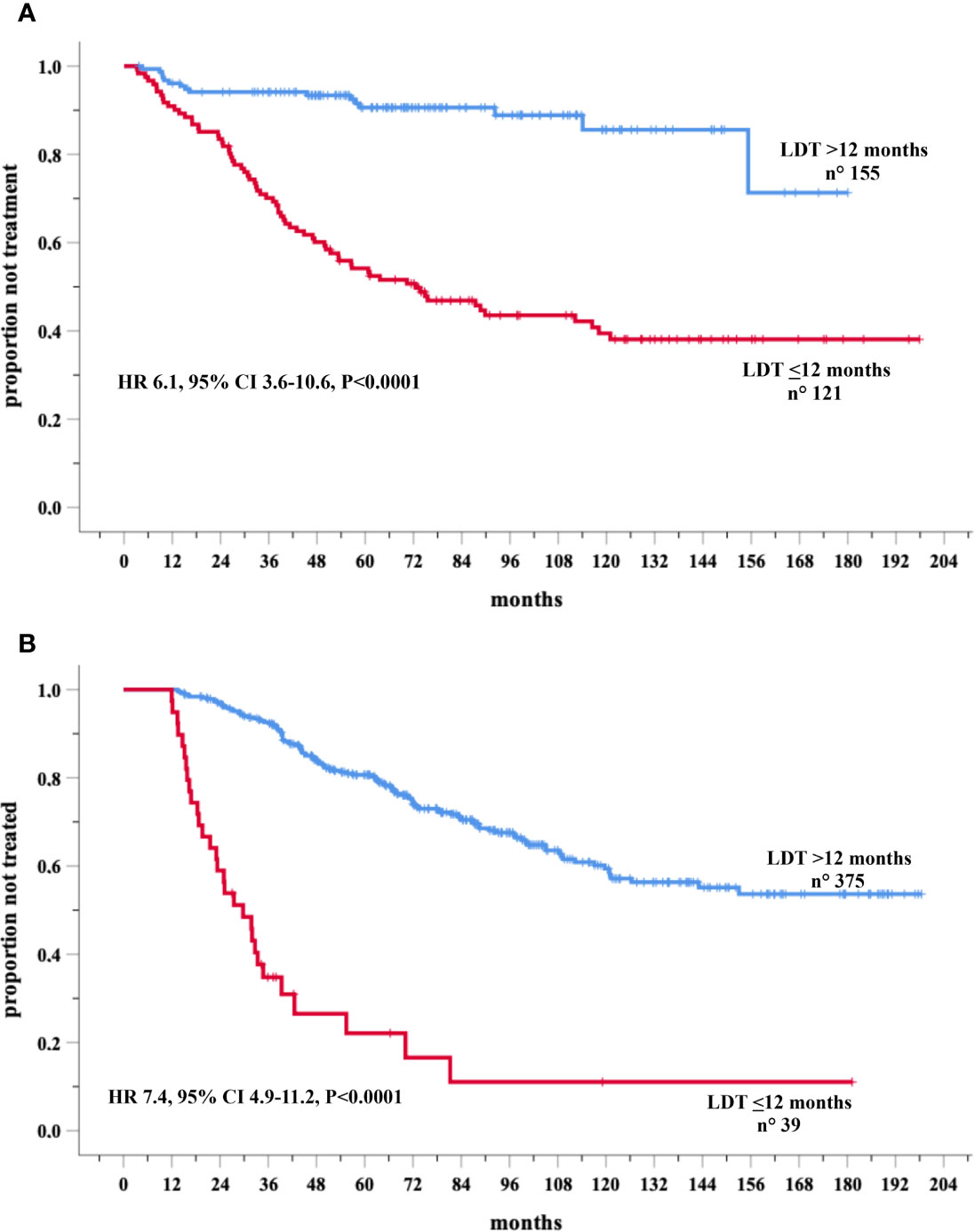
Figure 4 Kaplan-Meir curves of time to first treatment (TTFT) of patients stratified by lymphocyte doubling time (LDT) in the Novara (A) and Barcelona (B) validation cohorts.
Table 4 reports the clinical and biological variables significantly associated with TTFT in the multivariate models of training as well as both validation cohorts. Remarkably, the higher prognostic value, provided by risk prediction rule including LDT, found in the training cohort was fully confirmed in the two validation cohorts (Table 3). The HC-indexes and the explained variations in TTFT were consistently higher for bScore including LDT than for that excluding this variable (Table 3) in both Novara cohort (HC-Indexes 80.4 versus 75.2; explained variations 49.6 versus 33.3) and Barcelona cohort (HC-Indexes 70.0 versus 68.1; explained variations 30.5 versus 25.8). Finally, the Akaike weights coherently indicated that the risk scores including LDT had a chance to provide the best prognostic estimates ranging from 98.1% to 100% in both the training and in the two validation cohorts (Table 3).
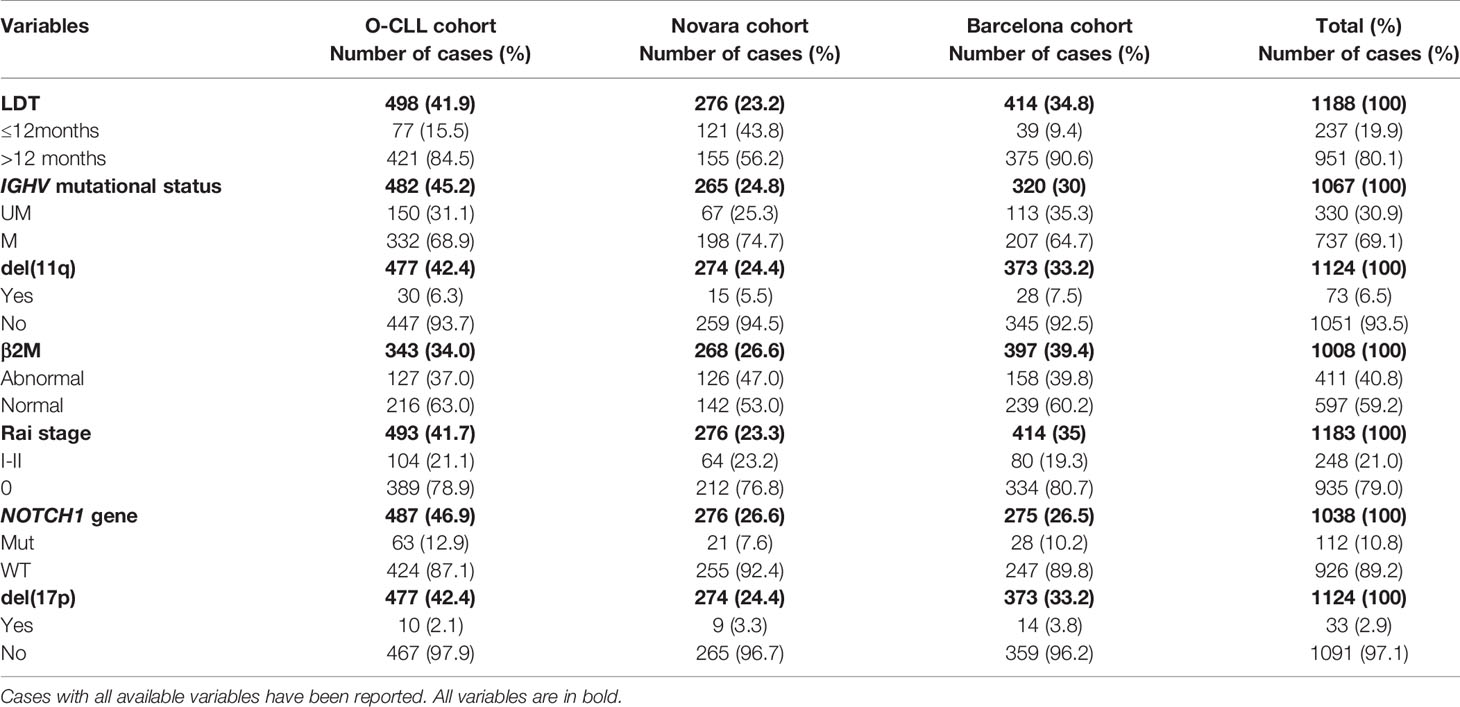
Table 4 Comparison of the clinical and biological variables of O-CLL (training), Novara and Barcelona (validation) cohorts resulted significantly associated to time to first treatment in the multivariate models.
Relationship Between LDT and IGHV Mutational Status
Data were further analyzed based on the combination between LDT and IGHV mutational status in the training and validation cohorts, in cases with both variables available As expected, LDT ≤12 months significantly maintained its negative prognostic power in both the IGHVmut and in the IGHVunmut patient groups in O-CLL (training) cohort (Figure 5A) as well as in both Novara (Figure 5B) and Barcelona (Figure 5C) validation cohorts, showing IGHVunmt & LDT ≤ 12 months and IGHVmut & LDT>12 months the shortest and the longest TTFT, respectively. Interestingly, the probability of remaining therapy-free at 6 years was 51% in the IGHVunmut and LDT>12months group and of 76% in the IGHVmut LTD ≤ 12 months, respectively (P=0.006) in the O-CLL validation cohort, confirming the predominant prognostic role of the IGHVumut status. However, this predominance was of borden-line significance (P=0.06) in the Barcelona group, while the significant prognostic impact was definitely lost in the Novara group. This discrepancy could be due to a different distribution of the above-mentioned subsets (IGHVunmut and LDT>12months and IGHVmut LTD ≤ 12 months) among Novara validation cohort (Supplementary Figure 1).
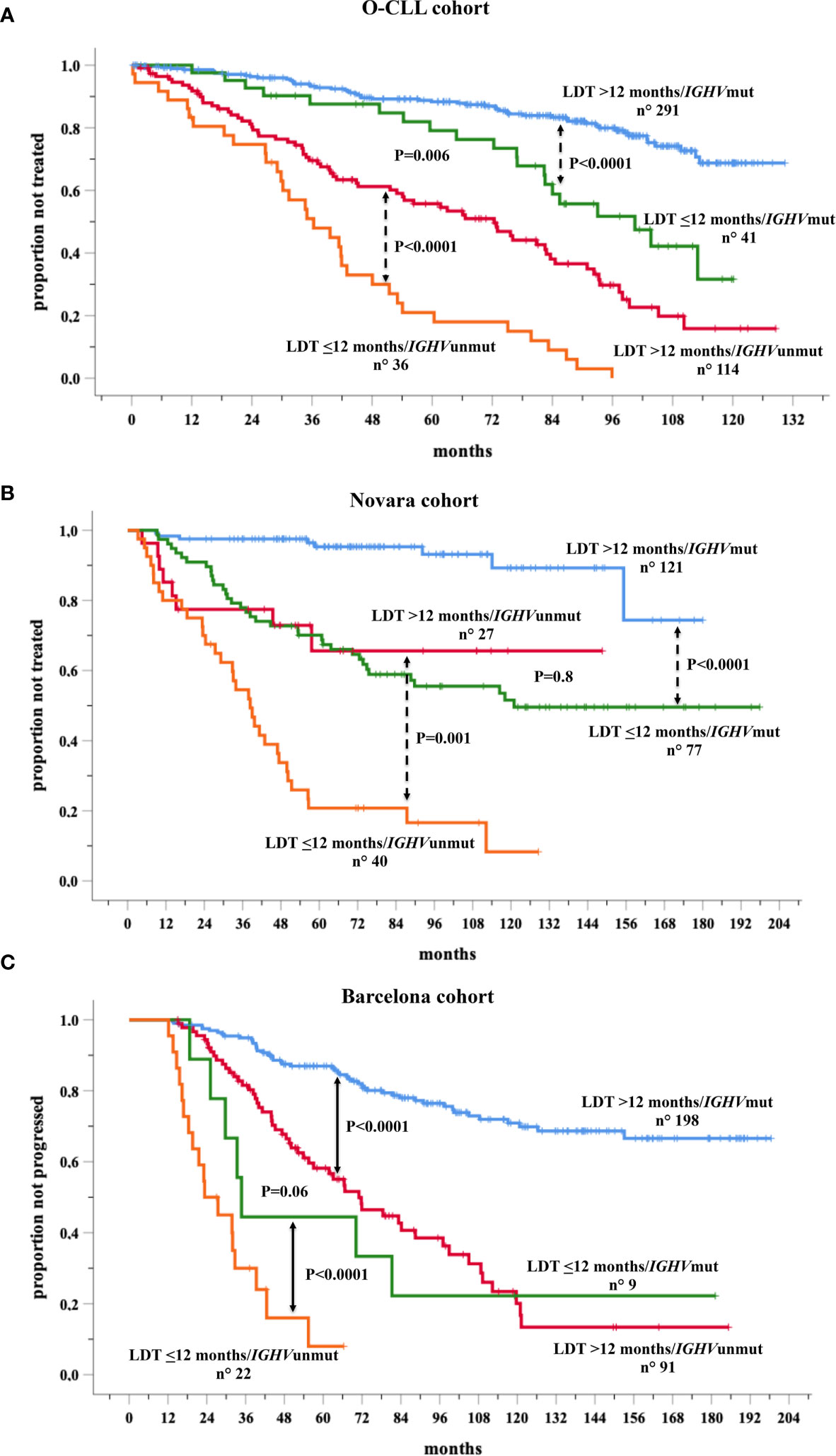
Figure 5 Kaplan-Meir curves of time to first treatment (TTFT) of patients stratified by the combined analysis of lymphocyte doubling time (LDT) and IGHV mutational status in the O-CLL training cohort (A) and Novara (B) and Barcelona (C) validation cohorts for whom both variables were available.
Discussion
The clinical course of early-stage CLL is hugely heterogeneous. While some patients need treatment at the onset of the diagnosis, others remain therapy-free for many years or even do not receive any treatment livelong (1, 2).
Several prognostic algorithms derived from multivariable models, nomograms and score systems have been developed to predict clinical outcomes accurately in early-stage CLL (64). The IGHV gene configuration is one of the most important single factors predicting therapy need, and it is recurrently incorporated in all prognostic models (65).
In contrast, the prognostic role of LDT in CLL was acknowledged more than 35 years ago by Montserrat et al. (55) and soon after by Molica et al. (56), when disease biology of the neoplastic cell remained weakly recognized. The raising question is whether the re-introduction of LDT among the last generation prognostic factors could help determine the follow-up strategies for an early-stage patient, possibly improving the prediction power of more sophisticated markers such as IGHV gene status. In the prospective O-CLL training cohort, a Cox regression model indicated IGHVumut genes, 11q and 17p deletions, elevated β2M, Rai stage I-II, NOTCH1 mutations and LDT ≤ 12 months as independently associated with shorter TTFT, thus confirmed the prognostic value of LDT in the era of new prognostic indicators. Our results fully agree with those of the German CLL Study Group, which endorses the prognostic benefit of determining LDT in the era of new markers (52). Specifically, the German prognostic model includes genetic features, i.e., 17p and 11q deletions, as well as IGHV mutational status. Similarly, the same prognostic indicators were demonstrated by our group to be independently associated with TTFT together with LDT. Unlike CLL1-PM, NOTCH1 remained significant in our analysis, confirming previous results (3, 5, 18).
Remarkably, the higher prognostic value, provided by risk prediction score including LDT, found in the training cohort was fully confirmed in the two validation cohorts. Specifically, the indicators of performance, such as the HC-indexes and the explained variations, were consistently higher for bScore including LDT than for that excluding this variable in the training and both validation cohorts. Finally, the Akaike weights coherently indicated that the risk scores including LDT had a chance to provide the best prognostic estimates ranging from 98.1% to 100% in both the training and the two validation cohorts. More recently, the prognostic significance of LDT was demonstrated to be independent for TTFT from the CLL-IPI and the Barcelona/Brno prognostic models in a real-life cohort of 848 Binet stage A patients (66).
Thus, the question of whether the re-introduction of LDT among the more sophisticated prognostic factors could help to determine the follow-up strategies for an early-stage patient has an affirmative answer. However, several caveats have to be considered. Although simple, LDT determinations require a precise timing and relative frequent accesses of patients to the clinic, thus preventing the setting of a definite ‘watch and wait’ strategy in concomitance with the work-up at diagnosis. Alternatively, more precise and possibly more rapid methodologies to measure lymphocyte proliferating potential, such as labeling with deuterated water, are too complex to be used routinely (67). Moreover, when data were further analyzed based on the combination between LDT and IGHV mutational status in the training and validation cohorts, IGHVunmut and LDT>12 months group showed a predominant prognostic role over IGHVmut LTD ≤ 12 months (P=0.006) in the O-CLL validation cohort. However, this predominance was of borden-line significance (P=0.06) in the Barcelona group, while the significant prognostic impact was definitely lost in the Novara group, incongruity possibly due to a different distribution of the above-mentioned subsets among Novara validation cohort. Thus, the IGHV mutation status could offer some, likely marginal, sensitivity advantage over the LDT determination. Finally, no information on the LDT stability overtime is available. Therefore, LDT values may vary over time, particularly in concomitance with the progression of the CLL towards a more aggressive form. These changes are likely to relate to a minority of cases and may not influence an entire cohort’s data but may have clinical relevance for the individual patients. These variations in time have been reported for cellular markers such as CD38 or ZAP-70 and have caused their subsequent obsolescence.
Data Availability Statement
The raw data supporting the conclusions of this article will be made available by the authors, without undue reservation.
Ethics Statement
The studies involving human participants were reviewed and approved by Comitato Etico Regionale Liguria n° OMC07.002. The patients/participants provided their written informed consent to participate in this study.
Author Contributions
FMo, MG, MF, and AN designed the study. FMo, GT, MG, and GD’A performed statistical analysis. FMo, MG, GT, DR, GG, EMo, MF, GC, MC, FF, FS, SB, SF, and AN analyzed and interpreted data, and wrote the manuscript. All authors contributed to the article and approved the submitted version.
Funding
Associazione Italiana Ricerca sul Cancro (AIRC) Grant 5 x 1000 n.9980 (to FM, MF, and AN); AIRC and Fondazione CaRiCal co-financed Multi-Unit Regional Grant 2014 n.16695 to FM; AIRC, Special Program Metastases (n. 21198) 5x1000 to RF and GG; RF-2018-12365790, MoH, Rome, Italy (to GG); AIRC IG-5506 to GF, IG-14326 (to MF), IG-15426 (to FF); Compagnia S. Paolo, Turin, Italy, Project 2017.0526 (to GF) and by the Ministry of Health (Project 5x1000, 2015 and 2016 and Current Research 2016 (to GF, GC, and FF); Swiss Cancer League, ID 3746, 4395 4660, and 4705, Bern, Switzerland; European Research Council (ERC) Consolidator Grant CLLCLONE, ID: 772051; Swiss National Science Foundation, ID 320030_169670/1 and 310030_192439, Berne, Switzerland; The Leukemia & Lymphoma Society, Translational Research Program, ID 6594-20, New York; Italian Ministry of Health_Ricerca Corrente 2021 to AN.
Conflict of Interest
The authors declare that the research was conducted in the absence of any commercial or financial relationships that could be construed as a potential conflict of interest.
The reviewer FA declared a shared affiliation with one of the authors, AN, to the handling editor at time of review.
Publisher’s Note
All claims expressed in this article are solely those of the authors and do not necessarily represent those of their affiliated organizations, or those of the publisher, the editors and the reviewers. Any product that may be evaluated in this article, or claim that may be made by its manufacturer, is not guaranteed or endorsed by the publisher.
Acknowledgments
In addition to the listed Authors, the following Investigators participated in this study as part of the Gruppo Italiano Studio Linfomi (GISL): Gianni Quintana, Divisione di Ematologia, Presidio Ospedaliero “A.Perrino”, Brindisi; Giovanni Bertoldero, Dipartimento di Oncologia, Ospedale Civile, Noale, Venezia; Paolo Di Tonno, Dipartimento di Ematologia, Ospedale di Venere, Bari; Robin Foà and Francesca R Mauro, Divisione di Ematologia, Università La Sapienza, Roma; Nicola Di Renzo, Unità di Ematologia, Ospedale Vito Fazzi, Lecce; Maria Cristina Cox, Ematologia, A.O. Sant’Andrea, Università La Sapienza, Roma; Stefano Molica, Dipartimento di Oncologia ed Ematologia, Pugliese-Ciaccio Hospital, Catanzaro; Attilio Guarini, Unità di Ematologia e Trapianto di Cellule Staminali, Istituto di Oncologia “Giovanni Paolo II”, Bari; Antonio Abbadessa, U.O.C. di Oncoematologia Ospedale “S. Anna e S. Sebastiano”, Caserta; Francesco Iuliano, U.O.C. di Oncologia, Ospedale Giannettasio, Rossano Calabro, Cosenza; Omar Racchi, Ospedale Villa Scassi Sampierdarena, Genova; Mauro Spriano, Ematologia, A.O. San Martino, Genova; Felicetto Ferrara, Divisione di Ematologia, Ospedale Cardarelli, Napoli; Monica Crugnola, Ematologia, CTMO, Azienda Ospedaliera Universitaria di Parma; Alessandro Andriani, Dipartimento di Ematologia, Ospedale Nuovo Regina Margherita, Roma; Nicola Cascavilla, Unità di Ematologia e Trapianto di Cellule Staminali, IRCCS Ospedale Casa Sollievo della Sofferenza, San Giovanni Rotondo; Lucia Ciuffreda, Unità di Ematologia, Ospedale San Nicola Pellegrino, Trani; Graziella Pinotti, U.O. Oncologia Medica, Ospedale di Circolo Fondazione Macchi, Varese; Anna Pascarella, Unità Operativa di Ematologia, Ospedale dell’Angelo, Venezia-Mestre; Maria Grazia Lipari, Divisione di Ematologia, Ospedale Policlinico, Palermo, Francesco Merli, Unità Operativa di Ematologia, A.O.S. Maria Nuova, Reggio Emilia; Luca Baldini Istituto di Ricovero e Cura a Carattere Scientifico Cà Granda-Maggiore Policlinico, Milano; Caterina Musolino, Divisione di Ematologia, Università di Messina; Agostino Cortelezzi, Ematologia and CTMO, Foundation IRCCS Ca’ Granda Ospedale Maggiore Policlinico, Milano; Francesco Angrilli, Dipartimento di Ematologia, Ospedale Santo Spirito, Pescara; Ugo Consoli, U.O.S. di Emato-Oncologia, Ospedale Garibaldi-Nesima, Catania; Gianluca Festini, Centro di Riferimento Ematologico-Seconda Medicina, Azienda Ospedaliero-Universitaria, Ospedali Riuniti, Trieste; Giuseppe Longo, Unità di Ematologia, Ospedale San Vincenzo, Taormina; Daniele Vallisa and Annalisa Arcari, Unità di Ematologia, Dipartimento di Onco-Ematologia, Guglielmo da Saliceto Hospital, Piacenza; Francesco Di Raimondo and Annalisa Chiarenza, Divisione di Ematologia, Università di Catania Ospedale Ferrarotto, Catania; Iolanda Vincelli, Unità di Ematologia, A.O. of Reggio Calabria; Donato Mannina, Divisione di Ematologia, Ospedale Papardo, Messina, Italy. For Each Institution, the Ethics Review Committee that approved the protocol is listed below. Unità di Ematologia e Trapianto di Cellule Staminali, Istituto di Oncologia “Giovanni Paolo II”, Bari comitato etico Istituto di Oncologia “Giovanni Paolo II”, di Bari, Italy; Dipartimento di Ematologia, Ospedale di Venere, Bari comitato etico Ospedale di Venere,Bari, Italy; U.O.C. Ematologia e Trapianto, Ospedale “Mons. R. Dimiccoli” -Barletta comitato etico Ospedale “Mons. R. Dimiccoli” -Barletta, Italy; Divisione di Ematologia, Presidio Ospedaliero “A. Perrino”, Brindisi comitato etico Presidio Ospedaliero “A. Perrino”, Brindisi, Italy; U.O.C. di Oncoematologia Ospedale “S. Anna e S. Sebastiano”, Caserta comitato etico Ospedale “S. Anna e S. Sebastiano”, Caserta, Italy; Divisione di Ematologia, Università di Catania Ospedale Ferrarotto, Catania comitato etico Ospedale Ferrarotto, Catania, Italy; U.O.S. di Emato-Oncologia, Ospedale Garibaldi-Nesima, Catania comitato etico Ospedale Garibaldi-Nesima, Catania, Italy, Dipartimento di Oncologia ed Ematologia, Pugliese-Ciaccio Hospital, Catanzaro comitato etico Pugliese-Ciaccio Hospital, Catanzaro, Italy; U.O.C di Ematologia, A.O. Cosenza comitato etico A.O. Cosenza, Italy; U.O.C. di Oncologia, Ospedale Giannettasio, Rossano Calabro, Cosenza comitato etico Ospedale Giannettasio, Rossano Calabro, Italy; Divisione di Ematologia, Ospedale Policlinico, Palermo, comitato etico Ospedale Policlinico, Palermo, Italy; Ematologia ospedale Goretti, Latina comitato etico ospedale Goretti, Latina, Italy; Clinica Ematologica, DIMI, Genova, comitato etico Ospedale San Martino, Genova, Italy; Oncologia medica C IRCCS Ospedale Policlinico San Martino, Genoa, Italy comitato etico IRCCS Ospedale Policlinico San Martino,Genova, Italy; Ospedale Villa Scassi Sampierdarena, Genova comitato etico Ospedale Villa Scassi, Genova, Italy; Ematologia, A.O. San Martino, Genova comitato etico A.O. San Martino, Genova, Italy; Unità di Ematologia, Ospedale Vito Fazzi, Lecce comitato etico Ospedale Vito Fazzi, Lecce, Italy; UOC di Ematologia Ospedale di Matera comitato etico Ospedale di Matera, Italy; Divisione di Ematologia, Ospedale Papardo, Messina, Italy. comitato etico Ospedale Papardo, Messina, Italy; Divisione di Ematologia, Università di Messina comitato etico Ospedale di Messina, Italy; Ematologia and CTMO, Foundation IRCCS Ca’ Granda Ospedale Maggiore Policlinico, Milano comitato etico Ospedale Maggiore Policlinico di Milano, Italy; Dipartimento di Oncologia, Ospedale Civile, Noale, Venezia comitato etico Ospedale Civile, Noale, Italy; Oncoematologia Policlinico di Modena comitato etico provinciale di Modena, Italy; Divisione di Ematologia, Ospedale Cardarelli, Napoli comitato etico Ospedale Cardarelli, Napoli, Italy; Ematologia, CTMO, Azienda Ospedaliera Universitaria di Parma comitato etico Azienda Ospedaliera Universitaria di Parma, Italy; Dipartimento di Ematologia, Ospedale Santo Spirito, Pescara comitato etico Ospedale Santo Spirito, Pescara, Italy; Unità di Ematologia, Dipartimento di Onco-Ematologia, Guglielmo da Saliceto Hospital, Piacenza comitato etico Guglielmo da Saliceto Hospital, Piacenza, Italy; Unità di Ematologia, A.O. of Reggio Calabria comitato etico A.O. of Reggio Calabria, Italy; Unità Operativa di Ematologia, A.O.S. Maria Nuova, Reggio Emilia comitato etico A.O.S. Maria Nuova, Reggio Emilia, Italy; S.C. di Ematologia e Trapianto di Cellule Staminali IRCCS-CROB di Rionero in Vulture, Potenza comitato etico IRCCS-CROB di Rionero in Vulture, Italy; Dipartimento di Ematologia, Ospedale Nuovo Regina Margherita, Roma comitato etico Ospedale Nuovo Regina Margherita, Roma, Italy; Ematologia, A.O. Sant’Andrea, Università La Sapienza, Roma comitato etico A.O. Sant’Andrea, Roma, Italy; Divisione di Ematologia, Università La Sapienza, Roma comitato etico Università La Sapienza, Roma, Italy; Unità di Ematologia e Trapianto di Cellule Staminali, IRCCS Ospedale Casa Sollievo della Sofferenza, San Giovanni Rotondo comitato etico IRCCS Ospedale Casa Sollievo della Sofferenza, San Giovanni Rotondo, Italy; Unità di Ematologia, Ospedale San Vincenzo, Taormina, comitato etico Ospedale San Vincenzo, Taormina, Italy;Unità di Ematologia, Ospedale San Nicola Pellegrino, Trani comitato etico Ospedale San Nicola Pellegrino, Trani, Italy; Centro di Riferimento Ematologico-Seconda Medicina, Azienda Ospedaliero-Universitaria, Ospedali Riuniti, Trieste comitato etico Ospedali Riuniti, Trieste, Italy; U.O. Oncologia Medica, Ospedale di Circolo Fondazione Macchi, Varese comitato etico Ospedale di Circolo Fondazione Macchi, Varese, Italy; Unità Operativa di Ematologia, Ospedale dell’Angelo, Venezia-Mestre comitato etico Ospedale dell’Angelo, Venezia-Mestre, Italy; Istituto di Ricovero e Cura a Carattere Scientifico Cà Granda-Maggiore Policlinico, Milano comitato etico Policlinico, Milano, Italy.
Supplementary Material
The Supplementary Material for this article can be found online at: https://www.frontiersin.org/articles/10.3389/fonc.2021.684621/full#supplementary-material
References
1. Hallek M. Chronic Lymphocytic Leukemia: 2020 Update on Diagnosis, Risk Stratification and Treatment. Am J Hematol (2019) 94(11):1266–87. doi: 10.1002/ajh.25595
2. Bosch F, Dalla-Favera R. Chronic Lymphocytic Leukaemia: From Genetics to Treatment. Nat Rev Clin Oncol (2019) 16(11):684–701. doi: 10.1038/s41571-019-0239-8
3. Monti P, Lionetti M, De Luca G, Menichini P, Recchia AG, Matis S, et al. Time to First Treatment and P53 Dysfunction in Chronic Lymphocytic Leukaemia: Results of the O-CLL1 Study in Early Stage Patients. Sci Rep (2020) 10(1):18427. doi: 10.1038/s41598-020-75364-3
4. Ronchetti D, Favasuli V, Monti P, Cutrona G, Fabris S, Silvestris I, et al. NEAT1 Long Isoform Is Highly Expressed in Chronic Lymphocytic Leukemia Irrespectively of Cytogenetic Groups or Clinical Outcome. Noncoding RNA (2020) 6(1):11. doi: 10.3390/ncrna6010011
5. Lionetti M, Barbieri M, Favasuli V, Taiana E, Fabris S, Favoino C, et al. Frequency and Clinical Relevance of Coding and Noncoding NOTCH1 Mutations in Early Stage Binet A Chronic Lymphocytic Leukemia Patients. Hematol Oncol (2020) 38(3):406–8. doi: 10.1002/hon.2722
6. Raponi S, Ilari C, Della Starza I, Cappelli LV, Cafforio L, Piciocchi A, et al. Redefining the Prognostic Likelihood of Chronic Lymphocytic Leukaemia Patients With Borderline Percentage of Immunoglobulin Variable Heavy Chain Region Mutations. Br J Haematol (2020) 189(5):853–9. doi: 10.1111/bjh.16434
7. Tissino E, Pozzo F, Benedetti D, Caldana C, Bittolo T, Rossi FM, et al. CD49d Promotes Disease Progression in Chronic Lymphocytic Leukemia: New Insights From CD49d Bimodal Expression. Blood (2020) 135(15):1244–54. doi: 10.1182/blood.2019003179
8. Autore F, Strati P, Innocenti I, Corrente F, Trentin L, Cortelezzi A, et al. Elevated Lactate Dehydrogenase Has Prognostic Relevance in Treatment-Naïve Patients Affected by Chronic Lymphocytic Leukemia With Trisomy 12. Cancers (Basel) (2019) 11(7):896. doi: 10.3390/cancers11070896
9. Morabito F, Shanafelt TD, Gentile M, Reda G, Mauro FR, Rossi D, et al. Immunoglobulin Heavy Chain Variable Region Gene (IGHV) and Prediction of Time to First Treatment (TTFT) in Patients With Chronic Lymphocytic Leukemia (CLL): Mutational Load or Mutational Status? Analysis of 1003 Cases. Am J Hematol (2018) 93(9):E216–9. doi: 10.1002/ajh.25206
10. Raponi S, Del Giudice I, Marinelli M, Wang J, Cafforio L, Ilari C, et al. Genetic Landscape of Ultra-Stable Chronic Lymphocytic Leukemia Patients. Ann Oncol (2018) 29(4):966–72. doi: 10.1093/annonc/mdy021
11. Ronchetti D, Manzoni M, Agnelli L, Vinci C, Fabris S, Cutrona G, et al. lncRNA Profiling in Early-Stage Chronic Lymphocytic Leukemia Identifies Transcriptional Fingerprints With Relevance in Clinical Outcome. Blood Cancer J (2016) 6(9):e468. doi: 10.1038/bcj.2016.77
12. Molica S, Digiesi G, D’Arena G, Mirabelli R, Antenucci A, Conti L, et al. Serum Levels of Soluble Calreticulin Predict for Time to First Treatment in Early Chronic Lymphocytic Leukaemia. Br J Haematol (2016) 175(5):983–5. doi: 10.1111/bjh.13907
13. Maura F, Mosca L, Fabris S, Cutrona G, Matis S, Lionetti M, et al. Insulin Growth Factor 1 Receptor Expression Is Associated With NOTCH1 Mutation, Trisomy 12 and Aggressive Clinical Course in Chronic Lymphocytic Leukaemia. PLoS One (2015) 10(3):e0118801. doi: 10.1371/journal.pone.0118801
14. Gentile M, Cutrona G, Molica S, Ilariucci F, Mauro FR, Di Renzo N, et al. Prospective Validation of Predictive Value of Abdominal Computed Tomography Scan on Time to First Treatment in Rai 0 Chronic Lymphocytic Leukemia Patients: Results of the Multicenter O-CLL1-GISL Study. Eur J Haematol (2016) 96(1):36–45. doi: 10.1111/ejh.12545
15. Morabito F, Cutrona G, Gentile M, Fabris S, Matis S, Vigna E, et al. Is ZAP70 Still a Key Prognostic Factor in Early Stage Chronic Lymphocytic Leukaemia? Results of the Analysis From a Prospective Multicentre Observational Study. Br J Haematol (2015) 168(3):455–9. doi: 10.1111/bjh.13117
16. Negrini M, Cutrona G, Bassi C, Fabris S, Zagatti B, Colombo M. microRNAome Expression in Chronic Lymphocytic Leukemia: Comparison With Normal B Cell Subsets and Correlations With Prognostic and Clinical Parameters. Clin Cancer Res (2014) 20(15):4141–53. doi: 10.1158/1078-0432.CCR-13-2497
17. Hoxha M, Fabris S, Agnelli L, Bollati V, Cutrona G, Matis S, et al. Relevance of Telomere/Telomerase System Impairment in Early Stage Chronic Lymphocytic Leukemia. Genes Chromosomes Cancer (2014) 53(7):612–21. doi: 10.1002/gcc.22171
18. Lionetti M, Fabris S, Cutrona G, Agnelli L, Ciardullo C, Matis S, et al. High-Throughput Sequencing for the Identification of NOTCH1 Mutations in Early Stage Chronic Lymphocytic Leukaemia: Biological and Clinical Implications. Br J Haematol (2014) 165(5):629–39. doi: 10.1111/bjh.12800
19. Ronchetti D, Tuana G, Rinaldi A, Agnelli L, Cutrona G, Mosca L, et al. Distinct Patterns of Global Promoter Methylation in Early Stage Chronic Lymphocytic Leukemia. Genes Chromosomes Cancer (2014) 53(3):264–73. doi: 10.1002/gcc.22139
20. Ronchetti D, Mosca L, Cutrona G, Tuana G, Gentile M, Fabris S, et al. Small Nucleolar RNAs as New Biomarkers in Chronic Lymphocytic Leukemia. BMC Med Genomics (2013) 6:27. doi: 10.1186/1755-8794-6-27
21. Gentile M, Cutrona G, Fabris S, Pesce EA, Baldini L, Di Raimondo F, et al. Total Body Computed Tomography Scan in the Initial Work-Up of Binet Stage a Chronic Lymphocytic Leukemia Patients: Results of the Prospective, Multicenter O-CLL1-GISL Study. Am J Hematol (2013) 88(7):539–44. doi: 10.1002/ajh.23448
22. Fabris S, Mosca L, Cutrona G, Lionetti M, Agnelli L, Ciceri G, et al. Chromosome 2p Gain in Monoclonal B-Cell Lymphocytosis and in Early Stage Chronic Lymphocytic Leukemia. Am J Hematol (2013) 88(1):24–31. doi: 10.1002/ajh.23340
23. Morabito F, De Filippi R, Laurenti L, Zirlik K, Recchia AG, Gentile M, et al. The Cumulative Amount of Serum-Free Light Chain Is a Strong Prognosticator in Chronic Lymphocytic Leukemia. Blood (2011) 118(24):6353–61. doi: 10.1182/blood-2011-04-345587
24. Fabris S, Scarciolla O, Morabito F, Cifarelli RA, Dininno C, Cutrona G, et al. Multiplex Ligation-Dependent Probe Amplification and Fluorescence In Situ Hybridization to Detect Chromosomal Abnormalities in Chronic Lymphocytic Leukemia: A Comparative Study. Genes Chromosomes Cancer (2011) 50(9):726–34. doi: 10.1002/gcc.20894
25. Fabris S, Bollati V, Agnelli L, Morabito F, Motta V, Cutrona G, et al. Biological and Clinical Relevance of Quantitative Global Methylation of Repetitive DNA Sequences in Chronic Lymphocytic Leukemia. Epigenetics (2011) 6(2):188–94. doi: 10.4161/epi.6.2.13528
26. Mosca L, Fabris S, Lionetti M, Morabito F, Motta V, Cutrona G, et al. Integrative Genomics Analyses Reveal Molecularly Distinct Subgroups of B-Cell Chronic Lymphocytic Leukemia Patients With 13q14 Deletion. Clin Cancer Res (2010) 16(23):5641–53. doi: 10.1158/1078-0432.CCR-10-0151
27. Gentile M, Cutrona G, Neri A, Molica S, Ferrarini M, Morabito F. Predictive Value of beta2-microglobulin (beta2-m) Levels in Chronic Lymphocytic Leukemia Since Binet A Stages. Haematologica (2009) 94(6):887–8. doi: 10.3324/haematol.2009.005561
28. Fabris S, Mosca L, Todoerti K, Cutrona G, Lionetti M, Intini D, et al. Molecular and Transcriptional Characterization of 17p Loss in B-Cell Chronic Lymphocytic Leukemia. Genes Chromosomes Cancer (2008) 47(9):781–93. doi: 10.1002/gcc.20579
29. Cutrona G, Colombo M, Matis S, Fabbi M, Spriano M, Callea V, et al. Clonal Heterogeneity in Chronic Lymphocytic Leukemia Cells: Superior Response to Surface IgM Cross-Linking in CD38, Zap-70-Positive Cells. Haematologica (2008) 93(3):413–22. doi: 10.3324/haematol.11646
30. Gentile M, Mauro FR, Guarini A, Foà R. New Developments in the Diagnosis, Prognosis and Treatment of Chronic Lymphocytic Leukemia. Curr Opin Oncol (2005) 17(6):597–604. doi: 10.1097/01.cco.0000181403.75460.c7
31. Gentile M, Mauro FR, Calabrese E, De Propris MS, Giammartini E, Mancini F, et al. The Prognostic Value of CD38 Expression in Chronic Lymphocytic Leukaemia Patients Studied Prospectively at Diagnosis: A Single Institute Experience. Br J Haematol (2005) 130(4):549–57. doi: 10.1111/j.1365-2141.2005.05659.x
32. Hallek M, Cheson BD, Catovsky D, Caligaris-Cappio F, Dighiero G, Döhner H, et al. Guidelines for the Diagnosis and Treatment of Chronic Lymphocytic Leukemia: A Report From the International Workshop on Chronic Lymphocytic Leukemia Updating the National Cancer Institute-Working Group 1996 Guidelines. Blood (2008) 111:5446–56. doi: 10.1182/blood-2007-06-093906
33. Hallek M, Cheson BD, Catovsky D, Caligaris-Cappio F, Dighiero G, Döhner H, et al. iwCLL Guidelines for Diagnosis, Indications for Treatment, Response Assessment, and Supportive Management of CLL. Blood (2018) 131(25):2745–60. doi: 10.1182/blood-2017-09-806398
34. Mato AR, Barrientos JC, Ghosh N, Pagel JM, Brander DM, Gutierrez M, et al. Prognostic Testing and Treatment Patterns in Chronic Lymphocytic Leukemia in the Era of Novel Targeted Therapies: Results From the informCLL Registry. Clin Lymphoma Myeloma Leuk (2020) 20(3):174–83.e3. doi: 10.1016/j.clml.2019.10.009
35. Montserrat E, Gale RP. Predicting the Outcome of Patients With Chronic Lymphocytic Leukemia: Progress and Uncertainty. Cancer (2019) 125(21):3699–705. doi: 10.1002/cncr.32353
36. Morabito F, Cutrona G, Gentile M, Matis S, Todoerti K, Colombo M, et al. Definition of Progression Risk Based on Combinations of Cellular and Molecular Markers in Patients With Binet Stage A Chronic Lymphocytic Leukaemia. Br J Haematol (2009) 146(1):44–53. doi: 10.1111/j.1365-2141.2009.07703.x
37. Molica S, Giannarelli D, Gentile M, Cutrona G, Di Renzo N, Di Raimondo F, et al. The Utility of Two Prognostic Models for Predicting Time to First Treatment in Early Chronic Lymphocytic Leukemia Patients: Results of a Comparative Analysis. Leuk Res (2013) 37(8):943–7. doi: 10.1016/j.leukres.2013.02.016
38. Molica S, Giannarelli D, Gentile M, Cutrona G, Di Renzo N, Di Raimondo F, et al. External Validation on a Prospective Basis of a Nomogram for Predicting the Time to First Treatment in Patients With Chronic Lymphocytic Leukemia. Cancer (2013) 119(6):1177–85. doi: 10.1002/cncr.27900
39. Molica S, Giannarelli D, Levato L, Mirabelli R, Gentile M, Lentini M, et al. A Prognostic Algorithm Including a Modified Version of MD Anderson Cancer Center (MDACC) Score Predicts Time to First Treatment of Patients With Clinical Monoclonal Lymphocytosis (cMBL)/Rai Stage 0 Chronic Lymphocytic Leukemia (CLL). Int J Hematol (2014) 100(3):290–5. doi: 10.1007/s12185-014-1634-7
40. Gentile M, Mauro FR, Rossi D, Vincelli I, Tripepi G, Recchia AG, et al. Italian External and Multicentric Validation of the MD Anderson Cancer Center Nomogram and Prognostic Index for Chronic Lymphocytic Leukaemia Patients: Analysis of 1502 Cases. Br J Haematol (2014) 167(2):224–32. doi: 10.1111/bjh.13032
41. Gentile M, Cutrona G, Mosca L, Matis S, Fabris S, Lionetti M, et al. Prospective Validation of a Risk Score Based on Biological Markers for Predicting Progression Free Survival in Binet Stage A Chronic Lymphocytic Leukemia Patients: Results of the Multicenter O-CLL1-GISL Study. Am J Hematol (2014) 89(7):743–50. doi: 10.1002/ajh.23729
42. Gentile M, Shanafelt TD, Rossi D, Laurenti L, Mauro FR, Molica S, et al. Validation of the CLL-IPI and Comparison With the MDACC Prognostic Index: Analysis of 1364 Newly Diagnosed Patients. Blood (2016) 128(16):2093–5. doi: 10.1182/blood-2016-07-728261
43. Molica S, Shanafelt TD, Giannarelli D, Gentile M, Mirabelli R, Cutrona G, et al. The Chronic Lymphocytic Leukemia International Prognostic Index Predicts Time to First Treatment in Early CLL: Independent Validation in a Prospective Cohort of Early Stage Patients. Am J Hematol (2016) 91(11):1090–5. doi: 10.1002/ajh.24493
44. Gentile M, Shanafelt TD, Cutrona G, Molica S, Tripepi G, Alvarez I, et al. A Progression-Risk Score to Predict Treatment Free Survival for Early Stage Chronic Lymphocytic Leukemia Patients. Leukemia (2015) 30(6):1440–3. doi: 10.1038/leu.2015.333
45. Molica S, Giannarelli D, Mirabelli R, Levato L, Russo A, Linardi M, et al. Unavailability of Thymidine Kinase Does Not Preclude the Use of German Comprehensive Prognostic Index. Results of an External Validation Analysis in Early Chonic Lymphocytic Leukemia and Comparison With Md Anderson Cancer Center Model. Eur J Haematol (2015) 96(1):72–7. doi: 10.1111/ejh.12550
46. Gentile M, Shanafelt TD, Mauro FR, Laurenti L, Rossi D, Molica S, et al. Comparison Between the Cll-Ipi and the Barcelona-Brno Prognostic Model: Analysis of 1299 Newly Diagnosed Cases. Am J Hematol (2017) 93(2):E35–7. doi: 10.1002/ajh.24960
47. Molica S, Giannarelli D, Mirabelli R, Levato L, Gentile M, Morabito F, et al. Reliability of Six Prognostic Models to Predict Time-to-First-Treatment in Patients With Chronic Lymphocytic Leukaemia in Early Phase. Am J Hematol (2017) 92(6):E91–3. doi: 10.1002/ajh.24707
48. Cohen JA, Rossi FM, Zucchetto A, Bomben R, Terzi-di-Bergamo L, Rabe KG, et al. A Laboratory-Based Scoring System Predicts Early Treatment in Rai 0 Chronic Lymphocytic Leukemia. Haematologica (2019) 105(6):1613–20. doi: 10.3324/haematol.2019.228171
49. Morabito F, Tripepi G, Vigna E, Bossio S, D'Arrigo G, Martino EA, et al. Validation of the Alternative International Prognostic Score-E (AIPS-E): Analysis of Binet Stage a Chronic Lymphocytic Patients Enrolled Into the O-CLL1-GISL Protocol. Eur J Haematol (2021) 106(6):831–5. doi: 10.1111/ejh.13614. Epub ahead of print
50. Pflug N, Bahlo J, Shanafelt TD, Eichhorst BF, Bergmann MA, Elter T, et al. Development of a Comprehensive Prognostic Index for Patients With Chronic Lymphocytic Leukemia. Blood (2014) 124(1):49–62. doi: 10.1182/blood-2014-02-556399
51. Condoluci A, Terzi di Bergamo L, Langerbeins P, Hoechstetter MA, Herling CD, De Paoli L, et al. International Prognostic Score for Asymptomatic Early-Stage Chronic Lymphocytic Leukemia. Blood (2020) 135(21):1859–69. doi: 10.1182/blood.2019003453
52. Hoechstetter MA, Busch R, Eichhorst B, Buhler A, Winkler D, Bahlo J, et al. Prognostic Model for Newly Diagnosed CLL Patients in Binet Stage A: Results of the Multicenter, Prospective CLL1 Trial of the German CLL Study Group. Leukemia (2020) 34(4):1038–51. doi: 10.1038/s41375-020-0727-y
53. Cook NR. Quantifying the Added Value of New Biomarkers: How and How Not. Diagn Progn Res (2018) 2:14. doi: 10.1186/s41512-018-0037-2
54. Galton DA. The Pathogenesis of Chronic Lymphocytic Leukemia. Can Med Assoc J (1966) 94(19):1005–10.
55. Montserrat E, Sanchez-Bisono J, Vinolas N, Rozman C. Lymphocyte Doubling Time in Chronic Lymphocytic Leukaemia: Analysis of Its Prognostic Significance. Br J Haematol (1986) 62(3):567–75. doi: 10.1111/j.1365-2141.1986.tb02969.x
56. Molica S, Alberti A. Prognostic Value of the Lymphocyte Doubling Time in Chronic Lymphocytic Leukemia. Cancer (1987) 60(11):2712–6. doi: 10.1002/1097-0142(19871201)60:11<2712::AID-CNCR2820601122>3.0.CO;2-1
57. Lad DP, Tejaswi V, Jindal N, Malhotra P, Khadwal A, Prakash G, et al. Modified CLL International Prognostic Index (CLL-LIPI) Using Lymphocyte Doubling Time (LDT) in Place of IgHV Mutation Status in Resource-Limited Settings Predicts Time to First Treatment and Overall Survival. Leuk Lymphoma (2020) 61(6):1512–5. doi: 10.1080/10428194.2020.1719099
58. Fais F, Ghiotto F, Hashimoto S, Sellars B, Valetto A, Allen SL, et al. Chronic Lymphocytic Leukemia B Cells Express Restricted Sets of Mutated and Unmutated Antigen Receptors. J Clin Invest (1998) 102(8):1515–25. doi: 10.1172/JCI3009
59. Rossi D, Bruscaggin A, Spina V, Rasi S, Khiabanian H, Messina M, et al. Mutations of the SF3B1 Splicing Factor in Chronic Lymphocytic Leukemia: Association With Progression and Fludarabine-Refractoriness. Blood (2011) 118(26):6904–8. doi: 10.1182/blood-2011-08-373159
60. Mehta HB, Mehta V, Girman CJ, Adhikari D, Johnson ML. Regression Coefficient-Based Scoring System Should be Used to Assign Weights to the Risk Index. J Clin Epidemiol (2016) 79:22–8. doi: 10.1016/j.jclinepi.2016.03.031
61. Tripepi G, Heinze G, Jager KJ, Stel VS, Dekker FW, Zoccali C. Risk Prediction Models. Nephrol Dial Transplant (2013) 28(8):1975–80. doi: 10.1093/ndt/gft095
62. Akaike H. A New Look at the Statistical Model Identification. IEEE Trans Automat Control (1974) 19(6):716–23. doi: 10.1109/TAC.1974.1100705
63. D’Arrigo G, Gori M, Pitino A, Torino C, Roumeliotis S, Tripepi G. Statistical Methods to Assess the Prognostic Value of Risk Prediction Rules in Clinical Research. Aging Clin Exp Res (2020) 33(2):279–83. doi: 10.1007/s40520-020-01542-y
64. Kipps TJ, Stevenson FK, Wu CJ, Croce CM, Packham G, Wierda WG, et al. Chronic Lymphocytic Leukemia. Nat Rev Dis Primers (2017) 3:17008. doi: 10.1038/nrdp.2016.96
65. Kreuzberger N, Damen JA, Trivella M, Estcourt LJ, Aldin A, Umlauff L, et al. Prognostic Models for Newly-Diagnosed Chronic Lymphocytic Leukaemia in Adults: A Systematic Review and Meta-Analysis. Cochrane Database Syst Rev (2020) 7:CD012022. doi: 10.1002/14651858.CD012022.pub2
66. Baumann T, Moia R, Gaidano G, Delgado J, Condoluci A, Villamor N, et al. Lymphocyte Doubling Time in Chronic Lymphocytic Leukemia Modern Era: A Real-Life Study in 848 Unselected Patients. Leukemia (2021). doi: 10.1038/s41375-021-01149-w
Keywords: CLL, prognosis, lymphocyte doubling time, TTFT, early stage
Citation: Morabito F, Tripepi G, Moia R, Recchia AG, Boggione P, Mauro FR, Bossio S, D’Arrigo G, Martino EA, Vigna E, Storino F, Fronza G, Di Raimondo F, Rossi D, Condoluci A, Colombo M, Fais F, Fabris S, Foa R, Cutrona G, Gentile M, Montserrat E, Gaidano G, Ferrarini M and Neri A (2021) Lymphocyte Doubling Time As A Key Prognostic Factor To Predict Time To First Treatment In Early-Stage Chronic Lymphocytic Leukemia. Front. Oncol. 11:684621. doi: 10.3389/fonc.2021.684621
Received: 23 March 2021; Accepted: 05 July 2021;
Published: 02 August 2021.
Edited by:
Annalisa Trama, Istituto Nazionale dei Tumori (IRCCS), ItalyReviewed by:
Federico Ambrogi, University of Milan, ItalyJohn Seymour, Peter MacCallum Cancer Centre, Australia
Copyright © 2021 Morabito, Tripepi, Moia, Recchia, Boggione, Mauro, Bossio, D’Arrigo, Martino, Vigna, Storino, Fronza, Di Raimondo, Rossi, Condoluci, Colombo, Fais, Fabris, Foa, Cutrona, Gentile, Montserrat, Gaidano, Ferrarini and Neri. This is an open-access article distributed under the terms of the Creative Commons Attribution License (CC BY). The use, distribution or reproduction in other forums is permitted, provided the original author(s) and the copyright owner(s) are credited and that the original publication in this journal is cited, in accordance with accepted academic practice. No use, distribution or reproduction is permitted which does not comply with these terms.
*Correspondence: Fortunato Morabito, Zi5tb3JhYml0bzUzQGdtYWlsLmNvbQ==