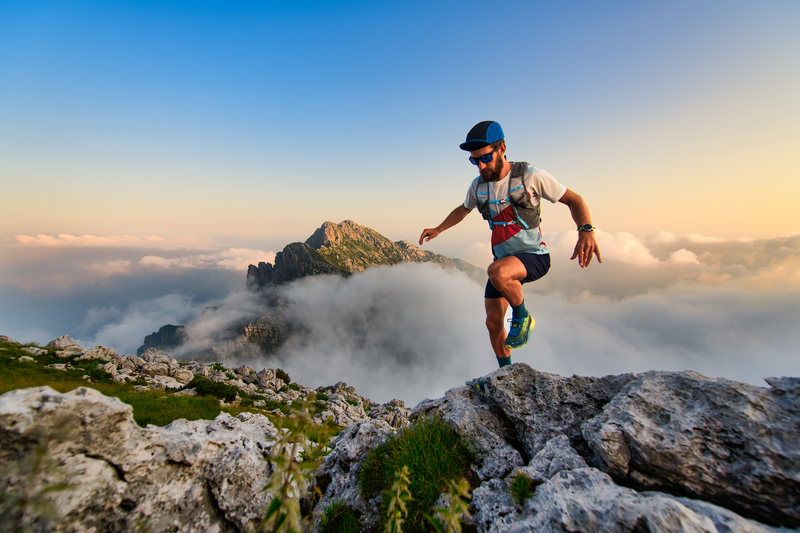
95% of researchers rate our articles as excellent or good
Learn more about the work of our research integrity team to safeguard the quality of each article we publish.
Find out more
SYSTEMATIC REVIEW article
Front. Oncol. , 04 June 2021
Sec. Neuro-Oncology and Neurosurgical Oncology
Volume 11 - 2021 | https://doi.org/10.3389/fonc.2021.665235
This article is part of the Research Topic Molecular and Immune Influences in the Progression of Gliomas View all 12 articles
Background: Gliomas are the most common and aggressive tumors of the central nervous system. A robust and widely used blood-based biomarker for glioma has not yet been identified. In recent years, a plethora of new research on blood-based biomarkers for glial tumors has been published. In this review, we question which molecules, including proteins, nucleic acids, circulating cells, and metabolomics, are most promising blood-based biomarkers for glioma diagnosis, prognosis, monitoring and other purposes, and align them to the seminal processes of cancer.
Methods: The Pubmed and Embase databases were systematically searched. Biomarkers were categorized in the identified biomolecules and biosources. Biomarker characteristics were assessed using the area under the curve (AUC), accuracy, sensitivity and/or specificity values and the degree of statistical significance among the assessed clinical groups was reported.
Results: 7,919 references were identified: 3,596 in PubMed and 4,323 in Embase. Following screening of titles, abstracts and availability of full-text, 262 articles were included in the final systematic review. Panels of multiple biomarkers together consistently reached AUCs >0.8 and accuracies >80% for various purposes but especially for diagnostics. The accuracy of single biomarkers, consisting of only one measurement, was far more variable, but single microRNAs and proteins are generally more promising as compared to other biomarker types.
Conclusion: Panels of microRNAs and proteins are most promising biomarkers, while single biomarkers such as GFAP, IL-10 and individual miRNAs also hold promise. It is possible that panels are more accurate once these are involved in different, complementary cancer-related molecular pathways, because not all pathways may be dysregulated in cancer patients. As biomarkers seem to be increasingly dysregulated in patients with short survival, higher tumor grades and more pathological tumor types, it can be hypothesized that more pathways are dysregulated as the degree of malignancy of the glial tumor increases. Despite, none of the biomarkers found in the literature search seem to be currently ready for clinical implementation, and most of the studies report only preliminary application of the identified biomarkers. Hence, large-scale validation of currently identified and potential novel biomarkers to show clinical utility is warranted.
Gliomas, and especially glioblastomas, are one of the most devastating primary tumors of the central nervous system with a dismal prognosis. Definite diagnosis of the disease is particularly dependent on tumor tissue assessment, though repetitive collection of tumor tissue to track tumor molecular evolution and/or tumor progression and regression is not desired. Part of such follow-up monitoring can be done via (advanced) imaging techniques. Also, the past years a plethora of research has been published in which blood-based biomarkers for glioma were utilized with various purposes. This is in line with the upcoming field of so-called ‘liquid biopsies’ in other (solid) tumor types. Blood-based biomarkers were found to be helpful as (early) diagnostic markers, including tumor grade and brain disease differentiating markers, prognostic, predictive, and monitoring markers (1–3) in glioma patients. Early diagnostic blood markers are biomarkers that can be utilized to predict development of glioma in individuals years before clinical or radiological signs can be noticed. These markers may be useful to screen patients with familial disorders such as neurofibromatosis type I, Li–Fraumeni syndrome and others that are at risk of development of a glioma (4). The term ‘diagnostic marker’ in this systematic review implies markers that were used to differentiate between healthy individuals and glioma patients. The terms ‘tumor grade and brain disease/tumor type-differentiating markers’ are employed to further classify glial tumors in glioma patients. Predictive markers can be employed to predict response to therapy and thus aid in correct therapy selection by examining the expression of histopathological features present in the glial tumor. Lastly, monitoring markers can be used to monitor tumor volume or monitor tumor progression as opposed to pseudoprogression after treatment. Tumor volume monitoring biomarkers that are stated in this review, were mainly used to predict tumor volume pre-treatment, but may also have use as volume monitoring markers after treatment. An example of how the different biomarker types may be employed during the clinical course of a typical glioma patient is detailed in Figure 1. Here, the timing of different biomarker types during and before treatment of future glioma patients is illustrated, along a timeline of clinical events in high- and low-grade glioma patients. Currently, it remains unclear which biomarkers or which combination of biomarkers will have most clinical utility. The aim of this systematic review is to identify and highlight the most promising and well-researched blood-based biomarkers for patients with glioma. Identification of a novel biomarker should start with the desired clinical groups to separate in mind. Distinguishing these groups should have clinical relevance, e.g. monitoring progression of lower-grade glioma patients to a secondary glioblastoma thereby tailoring treatment and providing prognostic information, or identification of patients with glioblastoma on treatment that develop tumor pseudo-progression as opposed to true-progression, thereby optimizing treatment schedules. With this, we believe that a promising biomarker should meet several criteria. First, the accuracy of the biomarker should be sufficiently high, measure exactly the difference between the clinically relevant groups without contribution from confounding variables, and adjusted towards its clinical context. For example, a diagnostic biomarker should be very precise, whereas predictive biomarkers should be very specific In order to not withhold patients potential therapeutic options. Second, a biomarker should be resistant to inter- and intra-individual factors, such as diurnal variation, body temperature, comorbidities, medication, radiation therapy, exercise, fasting, sex, and race. Following, the analytical devices that are used to measure the biomarker should be relatively cheap, easy to operate, sensitive in determining low concentrations of biomarker and specific for the biomarker, avoiding false-positive test results. Lastly, the biomarker should have been tested in several (preferably independent) studies with large patient populations, which include independent validation cohorts. Here, we provide a useful and easily accessible overview of the studies performed so far, after which we discuss the most promising markers that may deserve further validation. The review has been subdivided into several biosources and biomolecules as illustrated in Figure 2, and will close with a discussion of this dynamic field.
Figure 1 Timeline of clinical events for glioma patients and possible blood-based biomarkers that could be employed at different points in time. The straight lines indicate timelines for two example glioma patients [upper blue line for a lower-grade glioma (LGG) patient, lower red line for a high-grade glioma (HGG) patient]. Clinical events that occur on either timeline are indicated using dots and the clinical events are described in boxes connected to the dots. Early diagnostic markers have been found more than two decades before glioma diagnosis and could be used as a screening tool in the healthy population for patients older than 50 years. At the time of clinical or radiological findings that may indicate the growth of a glial tumor, diagnostic, tumor grade and disease differentiating biomarkers may be used to supplement the diagnostic procedure. Following, surgery (tumor tissue biopsy and/or tumor resection) may be performed, including either tumor resection or only a tumor tissue biopsy for definite histopathological diagnosis. At this point, the brain tumor is identified as a HGG or LGG. Following discussion of the case in a multidisciplinary tumor board, treatment may be initiated in patients with more malignant tumor types, while patients with less malignant tumor types may be subjected to frequent follow-up using monitoring markers and radiological imaging to monitor potential tumor progression. At the moment of tumor progression in patients with less malignant tumors or directly after surgical resection in patients with malignant tumors, predictive markers may provide additional information on the potential benefit of adjuvant treatment. Anti-tumor treatment with conventional chemo- and/or radiotherapy (CCR) is currently usually initiated at this point. Monitoring blood markers can detect tumor volume decrease over time. Patients with complete or partial response can be followed using radiological imaging and monitoring markers to distinguish between tumor progression or pseudoprogression. Patients with stable disease, progressive disease or tumor progression after complete or partial response may be admitted for experimental treatments. For each biomarker purpose, several potential blood-based biomarkers are listed aTocopherols; bmiR-21; cGFAP; dPanels of miRNAs, proteins and metabolites; eIL-10; fNLR; gYKL-40; hF-NLR; iF-NLR-AGR. Figure was adapted from “Cell Transfer Protocol”, by BioRender.com (2021). Retrieved from: https://app.biorender.com/biorender-templates.
Figure 2 Overview of possible blood-based biomarkers for glioma and their purposes. Schematic overview of the several biosources (plasma, serum, extracellular vesicles, blood platelets, circulating immune cells, and circulating glioma tumor cells) and biomolecules (proteins, nucleic acids, metabolomics and peptides) that are identified for patients with glioma. These biomolecules can be collected in a vial of blood, and employed as a diagnostic, prognostic, predictive, or therapy monitoring marker. Figure was created with BioRender.com.
We conducted systematic searches in the bibliographic databases PubMed and Embase from inception up to August 7, 2020. The following terms, including synonyms and closely related words, as index terms or free-text words were used: “Glioma”, “Blood”, “Biomarkers”. These were combined with possible purposes of biomarkers such as prognosis, diagnosis, monitoring and other related terms. Duplicate articles were excluded. The references of the identified articles were searched for relevant publications. The full search strategies for PubMed and Embase can be found in Supplemental Tables 1 and 2. Three authors independently screened all potentially relevant titles and abstracts for eligibility. If necessary, the full-text article was reassessed for the eligibility criteria. Differences in judgement were resolved through a consensus procedure. Studies were included if they met all of the following criteria: i) Histologically proven glial tumors; ii) Measured biomarker concentrations in whole blood, serum or plasma; iii) Correlation of the biomarkers with at least one of the following: glial tumor diagnosis, glial tumor grade, (glial) tumor type such as astrocytoma or oligodendroglioma, overall survival of patients, glial tumor manifestation prior to diagnosis, and tumor burden; iv) Included measures, such as Area Under the Curve (AUC), accuracy, hazard ratio (HR), sensitivity, specificity values and/or the degree of significance using a p-value. We excluded studies if they i) Reported on biomarkers found in CSF, tumor tissue or other non-hematogenous fluids such as cyst fluid; ii) Were of the following publication types: editorials, letters, interviews, case reports, animal studies, in vitro studies, pediatric studies, or (systematic) reviews; iii) Did not analyze biomarker value in a glioma-only (sub)group; iv) Reported on prognostic biomarkers when patients with glial tumors were treated with experimental treatments; v) Were published in languages other than English; vi) Described biomarker(s) which lacked substantial evidence relative to the biomarker categories. Substantial evidence is quantified as able to differentiate between clinically relevant groups in at least four independent studies. The process of retrieving all articles relevant to our systematic review is summarized in a Preferred Reporting Items for Systematic Reviews and Meta-Analyses (PRISMA) flowchart (see Figure 3).
Figure 3 PRISMA diagram showing the amount of records found through database searching and reference checking, the amount of records screened and removed using exclusion criteria and the amount of records included in the final qualitative synthesis.
Details per study (e.g. biosource and biomolecule), study population type (e.g. glioma or glioblastoma patients) and marker clinical group separating ability quantified as AUC, sensitivity, specificity, accuracy or hazard ratio can be found in the Supplementary Materials. In the Supplementary Tables, biomarkers are separated by purpose as diagnostic (Supplemental Tables 3–5), prognostic (Supplemental Table 6), predictive (Supplemental Table 7), and therapy monitoring (Supplemental Tables 8 and 9) markers. A separate table with panels of biomarkers and their potential function has been added as well (Supplemental Table 10). The summarized methodologies and results of included studies were used to critically assess the quality of the included studies. The evaluation of study quality is discussed in the results section.
The literature search generated a total of 7,919 references of which 3,596 were identified in PubMed and 4,323 in Embase, of which 262 studies were eligible for inclusion (Figure 3). A plethora of biomarkers were identified that could differentiate between clinically relevant patient groups. However, most markers were only found to be dysregulated in one group as compared to the other in only one or two studies. Therefore, we describe in this systematic review only markers that could differentiate between clinically relevant groups with significant results in at least four independent studies. We regarded these as the most promising biomarkers. Many studies did not include large patient or control populations of >100 patients or any validation cohort at all. Also, studies often did not report biomarker accuracies. The markers were divided into four relevant biomolecule groups: proteins, nucleic acids, circulating cells and metabolomics, and the most promising markers within these categories are discussed below. Due to word restrictions, we decided to report on glioma patients in general, and in most cases not per histopathological subtype separately, though we do understand that such separation is of clinical importance. The histopathological classification of gliomas is continuously developing with implementation of multiple (novel) molecular tissue markers (4). Hence, in retrospect it is not always possible to correlate the patients’ diagnoses as provided in the identified studies to the current standards. We decided to report the diagnosis as provided in the referenced studies.
Interleukins (ILs) are a group of cytokine proteins usually secreted by inflammatory cells by means of inter-inflammatory cell communication. Interleukins can promote or inhibit carcinogenesis. It is possible that glial tumors create a protumor environment by actively secreting (5, 6) and/or recruiting brain-resident cells such as microglia to stimulate the secretion of cytokines with pro-tumorigenic functions (7). Interleukins such as IL-1b (8–12), IL-6 (8–10, 13–17) and IL-10 (8, 10, 13, 18–23) have been found to be increased in glioma patients compared to healthy individuals. Accuracies of AUC = 0.9-1.0 (13) and a sensitivity 95% and specificity of 85% (19), have been found. However, IL-1b (21, 24) and IL-6 (21, 22, 25, 26) concentrations were also found to not be changed compared to controls or even decreased in glioma patients compared to controls. IL-1b (12) and IL-6 (17) levels may also be increased in patients with higher glioma grades, however other studies could not find a significant difference in IL-6 concentrations between patients with higher and lower glioma grades (25–27). Furthermore, IL-6 (17, 28) and IL-10 (21) have been found to be correlated with worse survival, but other studies could not confirm this for IL-6 (14, 25, 29–31). Thus, interleukins may be potential biomarkers, especially for glioma diagnosis.
Several S100-family members have been reported to contribute in vivo to tumor growth, metastasis, angiogenesis and immune invasion (32). Proteins from the S100 protein family including S100A8, S100A9 and S100B have been found to be increased in the blood of glioma patients compared to healthy individuals in multiple studies (33–39). However, it has also been reported that S100B is not changed in glioma patients compared to controls (40). Furthermore, it is unclear whether proteins of the S100-family are correlated with tumor grade (38, 40), tumor volume (39, 40) and survival (34, 41, 42). The accuracy of the inflammatory biomarker S100A8 is promising with a diagnostic AUC of 0.9 in glioblastoma patients (34). Glioblastoma and anaplastic astrocytoma patients could be differentiated with an AUC of 0.7 (34).
Tumor necrosis factor (TNF) was reported to be a major mediator of cancer-related inflammation and is elevated in cancer patients with poor prognosis (43). In vitro studies with glioma cells have shown that TNF can stimulate angiogenesis, downregulate the tumor suppressor gene PTEN and increase glioma cell invasiveness (44). Currently it is unclear whether TNF-alfa and TNF-beta are increased (9, 10, 14, 45), decreased (16, 20) or not changed in the blood of glioma patients (8, 13, 31).
Acute-phase (reactant) proteins (APRPs) are proteins that become increased (positive APRPs) or decreased (negative APRPs) in serum or plasma by at least 25% in response to an inflammatory stimulus (46). As gliomas and other cancers are characterized by chronic inflammation, it is possible that APRPs are altered in patients with cancer and can be employed as biomarkers. Indeed, in many other cancer types positive APRPs such as α1-antitrypsin and ceruloplasmin have been found to be increased, while negative APRPs such as kininogen and α2-HS glycoprotein are found to be decreased (47). Similarly, in glioma patients many positive APRPs such as haptoglobin (48–51) or CRP (14, 48, 52, 53) were increased compared to healthy individuals with AUCs around 0.8 (50, 52, 53) (see Supplemental Table 3). However, it is not clear whether negative APRPs and markers of reduced inflammation such as albumin, the prognostic nutritional index (PNI) and the albumin-globulin-ratio are decreased or remain unchanged (54, 55). Glioma tumor grade may be correlated with an increase of positive APRPs and a decrease of negative APRPs. The positive APRP fibrinogen was increased in patients with higher tumor grades (56–58) and similar results were also found for the related inflammatory marker F-NLR-AGR (57). The negative APRP marker albumin was decreased (56, 58) in patients with higher tumor grades, as is for the serum markers Albumin-Globulin-Ratio (AGR) and PNI (54–56, 58, 59), but these significant results were refuted in other studies (55, 57). The grade discriminative AUCs of both positive and negative APRPs were between 0.6 and 0.7 (54, 56, 58).
Several positive APRPs have been found to be dysregulated in patients with glioma with longer compared to shorter survival. The inflammatory marker CRP has been found to be decreased in the serum of patients with longer survival (52, 60, 61), but the prognostic value was low [(HR)=1.0] (52). However, significant results could not be confirmed elsewhere despite large patients series and multivariate analyses (14, 28, 62, 63). Furthermore, fibrinogen (56, 57, 64), fibrinogen-NLR score (64), F-NLR-AGR (57) and fibrinogen-albumin score (65) were all increased in glioma patients with worse survival. HRs were between 1.5-3.8 for fibrinogen and its related markers. Negative APRPs and markers of reduced inflammation such as albumin (56, 66–68), AGR (57, 69, 70), PNI (59, 69, 71), and the Sanbo Scoring System (72), were elevated in patients with prolonged survival compared to patients with shorter survival. However, other studies could not find a significant relationship between albumin (70, 71, 73, 74), AGR (75), PNI (74, 76) and survival. Lastly, APRPs may also have use as a marker to differentiate between glioma patients and patients with other intracranial diseases and as markers to detect IDH1-mutation and MGMT-methylation status. However, research on these topics is scarce at this moment. In all, it seems that positive APRPs and markers of increased inflammation are increased while negative APRPs and markers of reduced inflammation are decreased in patients with glial tumors and in particular patients with more malignant glial tumors (see Supplemental Tables 3–8).
Glial fibrillary acidic protein (GFAP) is a protein that is mainly expressed by astrocytes and aids in the maintenance of astrocytic structure and stability. Blood levels of GFAP can be increased after injury of the brain through strokes (77), traumatic brain injuries (78), and after brain surgery, including glioma resection (79–81). The blood levels are typically increased in the context of destruction of glial cells and opening of the blood-brain-barrier. As both usually do not occur in non-acute brain diseases such as multiple sclerosis or brain metastases, GFAP may be a specific marker for gliomas. Indeed, GFAP values (38, 80, 82–85) were found to be elevated in glioblastoma patients, but diagnostic sensitivities were rather variable: between 33% and 86% of glioblastoma had elevated GFAP concentrations (38, 82–86). GFAP diagnostic specificities were more uniform and ranged between 85-100% (80, 82, 84). However, GFAP concentrations were not elevated in the circulation of glioma patients with tumor grades lower than grade IV (80, 82, 85). Furthermore, GFAP was increased in patients with glioblastoma as compared to patients with lower tumor grades (38, 80, 82–85, 87) and in glioblastoma patients compared to patients with other brain pathologies such as brain metastases, meningioma or pituitary adenoma (38, 81, 82, 84–86, 88). Also, GFAP levels were increased in patients with worse survival (80, 84), greater tumor volume (40, 80, 82, 86, 88), higher Ki67 proliferation index and lack of IDH1-mutation (80). However, it was not always confirmed that circulating GFAP is correlated to tumor volume and survival (83). Thus, GFAP is a promising marker and might have value as biomarker for glioblastoma diagnosis, grade and tumor type differentiation.
YKL-40 is a glycoprotein that is secreted by macrophages, chondrocytes and several cancer cell types (89), including glioma cells (90). The exact functions of YKL-40 in cancer are unknown, however, it may stimulate angiogenesis, cell proliferation, prevent cell apoptosis (91), and aid in tissue remodeling during inflammation (89). YKL-40 is found to be increased in cancer and in inflammatory diseases such as Crohn’s disease, COPD, ulcerative colitis and others (89). YKL-40 was found to be increased in glioma patients as compared to healthy individuals (86, 92–95). The AUC in one study was 0.9 (93). Furthermore, YKL-40 is increased in patients with high-grade glioma compared to patients with low-grade glioma (93, 94, 96). Also, high baseline YKL-40 and increases in YKL-40 during treatment were correlated with worse survival in glioma patients with hazard ratios between 1-2.2 (25, 95–98). However, it was also found that YKL-40 was not correlated with survival (99) and tumor volume (94, 96, 97). In all, YKL-40 is an interesting marker, especially for predicting patient survival.
The vascular endothelial growth factor (VEGF) is one of the growth factors that aids in glioma neovascularization and a well-studied biomarker in glioma patients. VEGF has been researched extensively and has been found to be increased in glioma patients (10, 12, 14, 16, 100–107). However, multiple other studies did not find a significant difference (22, 24, 108–111). The same controversial results were also found in other studies when VEGF was used as a blood biomarker for other purposes such as tumor grade differentiating marker (12, 102, 103, 112, 113), tumor type differentiating marker for patients with glioblastoma and patients with intracranial metastases (103, 104, 114) and prognostic marker in patients that received several types of therapies (14, 19, 29, 31, 112, 115). It remains unclear why such differences have been found. It is possible that patient populations were too small to find a significant effect as both in studies with and without a significant effect of VEGF, most of the studies included small patient populations (<100 patients). Hence, VEGF does not seem to be a promising blood biomarker at this moment.
It is well known that cancer causes hypercoagulability that can result in venous thromboembolisms (VTE), disseminated intravascular coagulation and other coagulation disorders. The relation between brain cancer and thrombo-embolic events seems to be especially strong, as brain tumor patients had the second highest rate of thrombo-embolic events from malignancies in 18 organs (116). It is possible that this hypercoagulable state can be retraced in the blood of glioma patients if procoagulant factors are increased while anti-coagulant factors are decreased. It was seen that a multitude of coagulation markers and procoagulant factors were significantly increased in the circulation of glioma patients compared to healthy individuals such as prothrombin factor 1 + 2 (14), tissue factor (117), coagulation factor VII (19) and P-selectin (118). Procoagulant markers correlated with tumor grade and worse prognosis (see Supplemental Tables 4 and 6). Especially fibrinogen is well researched and often found to be correlated with grade (56–58) and survival in glioma (56, 57) and glioblastoma (56, 64) patients with moderate grade differentiating abilities (56, 58) and moderate prognostic abilities (HR=1.5) (64). Contrary, anti-clotting factors were also found to be increased (see Supplemental Tables 3, 4 and 6). Here, it may be possible that anti-clotting factors are reactively increased as a response to the prothrombotic state that is created by the tumor. However, it is also possible that the tumor stimulates the increase in anti-thrombotic proteins, as these may facilitate metastasis by degrading the extracellular matrix and allowing tumor cells to invade blood vessels (119).
Biomarker panels of two to over 100 markers were used with various purposes in glioma patients. In general, larger panels could differentiate between patients and controls or different patient groups with different grades, tumor types or survival with higher accuracies. Inflammation, immune response and cell proliferation related markers were dysregulated such as interleukins (13), TNF-alfa (13), CRP (52), YKL-40 (86) and FGF-basic (13). Functional analysis revealed enrichment of pathways that are dysregulated in cancer cells such as apoptosis pathways, immune function pathways and others (13, 52). Several protein and peptide panels could differentiate between glioma patients and healthy individuals with high accuracies with sensitivities and specificities >85% (13, 52, 86, 120–123). Only two panels had modest value as diagnostic markers with an AUC of 0.6 (16) and 74% accuracy (124). One protein panel (122) and several proteins or peptides from other panels (120, 123) could also differentiate between glioma patients with different tumor grades. Lastly, panels could differentiate between patients with better and worse prognosis (16, 125), and between patients with different intracranial tumors (123).
miRNAs are short, single-stranded RNAs of approximately 22 nucleotides in length, which bind and regulate translational repression or degradation of messenger and other RNAs (126). MicroRNAs may be ideal blood-based biomarkers as they are easily accessible in body fluids (127), are stable under harsh extrinsic conditions such as significant changes in temperature (128), and are protected from intrinsic conditions such as degradation by RNAses (129). Indeed, microRNAs can be found in biofluids such as serum, plasma, urine and cerebrospinal fluid and have shown to be deregulated in various cancer types such as renal cell carcinoma (130) and melanoma (131). Also, research is accumulating indicating that the blood of glioma patients has a unique miRNA expression pattern. However, it has been noted that miRNAs may not be good biomarkers as the brain has little influence on miRNA concentrations in blood as compared to other organs (132) and because differences in blood cell counts may more prominently influence variation in circulating miRNA profiles (133, 134). Despite that, miR-21 (135–143), miR-182 (144–148) and miR-222 (139, 149, 150) were all found to be increased in the blood of glioma patients as compared to healthy individuals. However, miR-21 (151, 152) and miR-222 (136) were also found to not have significantly different results in patients compared to controls. Diagnostic sensitivities and specificities of the miRNAs in glioma patients ranged from 47% to perfect accuracy (136, 139, 141, 148, 149). miR-21 (136, 139), miR-182 (146, 147) and miR-222 (139) were also correlated with tumor grade and an AUC of 0.8 was reported for miR-21 (139). Furthermore, miR-21 (143) and miR-222 (139) might also have use as a marker to differentiate between glial tumors and other intracranial tumors. Lastly, miR-21 (141), miR-182 (148, 153) and miR-222 (149, 150) may have value as prognostic markers and HRs of 1.3 (148) and 2.8 (149) have been reported. Remarkably, it was also found that miR-21 was upregulated years before glioma manifestation in patients (154).
In general, combination of microRNAs increased the accuracies of markers as compared to single microRNAs. Small panels of microRNAs which studied marker concentrations in two or three microRNAs could differentiate between glioma patients and controls with an AUC of 0.8 (139) and sensitivities and specificities between 70%-100% (138, 155–157). When larger miRNA panels were used, diagnostic accuracies of tests tended to increase. Using a panel of nine miRNAs as diagnostic markers, 50 and 90 glioma patients could be differentiated from healthy individuals with high accuracy with an AUC of 1.0 (151) and accuracy of 99.8% (137), respectively. However, a 180-miRNA panel in whole blood could distinguish between glioblastoma patients and healthy individuals with ‘just’ 81% accuracy (158). Patients with glioblastoma could be differentiated from lower grade patients with an AUC of 0.9 (159), also certain miRNA combinations were highly prognostic for glioma patients with HRs of 3.1 (151) and 0.4 (160), or could differentiate between patients with different brain tumors with an AUC of 0.8 (157). Lastly, the development of pulmonary embolisms could be predicted in glioma patients with an AUC of 0.8 (161).
Cell-free DNA (cfDNA) refers to fragmented DNA freely circulating outside of cells in blood plasma. cfDNA partly consists of DNA derived from tumor cells. cfDNA is often analyzed by examining circulating DNA from patients and searching whether there are tumor-specific mutations, deletions and/or amplifications present. The majority of cfDNA is released by non-tumor cells including (neighboring) inflammatory, stromal and other (healthy) cells, thereby searching for tumor-derived materials is considered to be a needle in a haystack. While both serum and plasma were used as biosource for cfDNA, it has been reported that serum contains around six times as much amounts of free cfDNA as compared to plasma with low levels of contaminating extraneous DNA released from leukocytes (162). Evidence is accumulating that the amount of cfDNA molecules and individual sequences of cfDNA can be employed as tumor biomarkers. First, it has been shown that total number of cfDNA molecules can be used as diagnostic marker to differentiate between glioma patients and controls (163, 164), tumor type differentiating marker (163), tumor progression marker (165), and prognostic marker (164). However, it remains unclear whether total cfDNA can also be used as a marker to estimate and monitor tumor burden (164–166). Furthermore, mutations and copy number variations in cfDNA can also be utilized to differentiate between glioma patients and controls (164, 166–170). Diagnostic sensitivities ranged from 50% to near perfect accuracies. Especially selection of cfDNA fragments between 90-150 base-pairs drastically improved detection accuracies. Moreover, mutations in therapeutically relevant genes such as TP53 and EGFR could also be found in cfDNA (164, 167) but were not always concordant with mutations in tumor tissue.
An alternative and highly potential biomarker may be methylation patterns in cfDNA of glioma patients. DNA methylation is one of three epigenetic mechanisms used to alter gene expression and can contribute to cancer development through regional hypermethylation and global hypomethylation (171). Methylation of tumor suppressor genes can silence tumor suppressor genes, while global hypomethylation of repetitive genomic elements can lead to elevated expression of oncogenes and chromosomal instability (171). In cfDNA of glioma patients, global hypomethylation of repetitive Alu elements and regional methylation of tumor suppressor genes such as MGMT were studied. Global hypomethylation of Alu elements was correlated with glioma diagnosis, higher tumor grade, shorter survival and lower Karnofsky Performance Score (172, 173). Also, it was recently reported that cfDNA methylation profiles have remarkable diagnostic capabilities in high-grade as well as in low-grade glioma with AUCs near 1.0. cfDNA methylation profiles also displayed high brain tumor differentiating capabilities with AUCs between 0.7-0.8 (174). Concordance of promoter methylation in tumor suppressor genes such as MGMT in cfDNA with their counterparts inside tumors, was observed with varying sensitivities 31%-80% but with high specificities all near 100% (175–182). Lastly, lack of MGMT promoter methylation in cfDNA could be used as a prognostic marker with hazard ratios between 2.0-2.2 (180, 182). While cfDNA methylation methods are of interest as markers with multiple purposes, so far the patient populations in which these methods were studied were often small (50 or less patients).
It has been suggested that circulating tumor cells (CTCs) are the driving cells of tumor metastasis. Extracranial metastases occur very rarely in patients with glioma and with an (estimated) incidence of less than 0.5% (183, 184). Despite this, several research efforts have been investigating the existence of circulating glial tumor cells (CGTCs) using a variety of methods, with highly variable results. Diagnostic sensitivities were reported between 21%-80% (185–192). Apart from diagnosis, CGTCs might also have other purposes. However, the cells were often not correlated with tumor grade (187, 190, 192), survival (188) or tumor burden (189) and they could not be used to differentiate between different glial tumor types (187). Interestingly, CGTCs have also been investigated as a tool to differentiate between tumor recurrence and radiation necrosis (192), and to differentiate between pseudoprogression and actual tumor progression (186, 190, 191), though such applications are definitely not ready for implementation in the current daily clinics.
It is well documented that platelets influence cancer cells in multiple ways, for example, platelets are known to promote tumor angiogenesis, tumor cell proliferation, metastasis and aid in immune surveillance escape of tumor cells (193). Because platelets stimulate tumor activities to a large degree, it is possible that platelet counts and content are altered as well in patients with glioma. However, currently platelet counts have variable results as biomarkers in glioma. Platelet counts were found to be increased in glioma patients (93, 100, 194) as well as non-significantly changed (54, 55, 118, 194) compared to healthy individuals. In most studies, platelets were observed to be non-significantly altered in patients with higher grade glioma compared to lower grade glioma (54, 58, 93, 195–197). Furthermore, there is overwhelming evidence that platelet counts are not correlated with patient prognosis in glioma patients (62, 70, 71, 93, 198–205). Moreover, platelet counts are not different in glioma patients as compared to other intracranial pathologies such as epilepsy and non-glial brain tumors (54, 206, 207). Aside platelet counts, researchers, amongst us, have noted that platelets may have altered protein content (208) and RNA content, due to sequestration of tumor-derived RNAs. The RNA content of these so-called ‘tumor-educated-platelets’ (TEPs) may be employed to distinguish cancer patients from healthy individuals (209). Also other research groups have confirmed that TEPs have good accuracy in distinguishing between healthy individuals and patients with various types of cancer (210–214). There are many obstacles that can interfere with the results of the TEPs such as age-related factors (215, 216), pre-analytical variables, and inflammatory and cardiovascular disease (217). Despite this, our data suggests that platelet RNA profiles may be employed for diagnostics of lower-grade glioma and glioblastoma, and potentially tumor treatment monitoring (209, 218, 219). Hence, platelets may contain promising information regarding the presence and treatment response of glioma.
In glioma patients it was often observed that WBC counts were increased compared to controls (21, 54, 55, 100, 118, 220). However, it was also found that leukocytes are not significantly changed in glioma patients compared to controls (21, 194), potentially due to dexamethasone use (221–224). Furthermore, it remains unclear whether WBCs are correlated with higher tumor grades (54, 55, 195, 201, 225, 226) and worse survival (23, 61, 62, 71, 201, 227) as many studies reported both statistically significant and non-significant results (144). Moreover, it was found that leukocyte counts were increased in glioma patients compared to patients with neuromas (54), non-lesional epilepsy (54) and meningioma (54, 161) and lack of IDH-mutation (201). However, it was also found that WBCs are not different in glioma as compared to meningioma patients (55). At this moment, total white blood cell counts are not considered promising as a blood-based marker for glioma.
Lymphocytes mainly consist out of three groups: T-cells (CD-3+), B-cells (CD-20+) and NK-cells (CD-56+). It was found in multiples studies that total lymphocyte numbers are not changed in glioma patients as compared to controls (21, 55, 100, 194, 228, 229). However, significant decreases in total lymphocytes were noted as well in glioma patients (54, 206, 228). This significant decrease might be attributed partly due to the use of dexamethasone (229). Total lymphocyte counts were lower in patients with higher tumor grades (54, 55, 196, 197, 201) and one study reported a tumor grade differentiating AUC of 0.6. Also, many studies reported that total lymphocyte numbers were not correlated with survival in glioma patients (62, 70, 71, 74, 198, 201, 202, 204, 205, 227, 230–233) though two studies reported that increased numbers of total lymphocytes were associated with prolonged survival (234, 235). Furthermore, total lymphocytes were not changed in glioma patients as compared to patients with brain metastases (206, 207), but it remains unclear whether total lymphocytes are changed in glioma patients as compared to meningioma (54, 55, 206) and epilepsy patients (54, 206). However, lymphocytes were also not correlated to tumor grade in two other studies (58, 196) or with IDH-1/2 mutation status (201, 236).
Total T-cells numbers were seen to be significantly decreased in glioma patients in multiple studies with high statistical significance (20, 21, 23, 229, 237, 238). Two other studies did not find a difference in malignant glioma patients (21, 24). Corticosteroids usually did not influence total T-cell counts (21, 23, 223), however, in one study corticosteroids did cause a significant decrease in CD3+-cell counts (229). Thus, more research is needed to determine whether CD3+ cells are altered in glioma patients. It may be possible that T-cells are decreased because of a decrease in CD4+-cells, which has often been reported in glioma patients (20, 21, 23, 229, 238–240). However, other studies did not find a significant difference between glioma patients and controls (21, 241, 242) in terms of CD4+-counts in blood. CD4+-cells have been found to be negatively correlated with glial tumor grade (20, 243) as well as to not be correlated with increasing tumor grades in glioma patients. Decrease in CD4+-cell counts was inversely related to survival in glioma patients (233, 243), but not related to IDH1-status (236).
There is little evidence for NK-cells as blood-based glioma biomarker. In several studies NK-cells (CD3+/CD56+, CD3-/CD56+ or CD16+/CD56+) were not significantly altered as compared to healthy individuals in glioma patients (23, 229, 244, 245). However, certain NK-cell populations were seen to be significantly decreased (23, 245) or increased (238) in glioma patients. Also, CD16+/CD56+-NK-cells had prognostic value (23). CD8+-cell counts were not altered in glioma patients in most studies (21, 238, 239, 242). It remains unclear whether CD8-cell counts are correlated with patient survival (23, 233) and lower tumor grades (20). There is a lot of controversial evidence concerning the value of lymphocytes and subpopulations of lymphocytes as biomarkers in glioma and glioblastoma patients. However, the majority of studies agree that total T-cells and CD4+-cells may be promising as a diagnostic marker.
Neutrophils were found to be increased in glioma patients compared to controls in the majority of the studies (21, 54, 55, 194, 220). Furthermore, higher-grade glioma patients were often reported to have increased neutrophil counts as compared to patients with lower-grade glioma (54, 55, 58, 196, 197, 201, 226, 246). Grade differentiating AUCs between 0.6-0.7 were reported (55, 58, 201). It remains unclear whether neutrophils are related to IDH mutation status (201, 236). Moreover, glioma patients had higher neutrophils compared to patients with a meningioma (54, 161), neuromas (54) or epilepsy (54, 206). It was also found that there was no difference between glioma or glioblastoma patients and meningioma patients (55, 206), between glioma or glioblastoma and metastases (206, 207) and grade III and grade IV glioma patients (227) in terms of neutrophil counts. Multiple studies reported that neutrophil counts had no prognostic value in glioma patients (62, 70, 71, 198, 204, 205, 230, 234), however, other studies found a negative correlation of neutrophil counts with survival in glioma (201, 227, 231, 247) with HRs around 1.6 (227, 231). Thus, neutrophil count might be valuable as diagnostic and grade differentiating marker.
It remains unclear whether monocyte counts (CD14+-cells and/or CD16+-cells) are changed in glioma patients compared to controls (54, 55, 106, 194, 229, 244, 248) or are related to tumor grade (54, 55, 58, 197, 225, 246). However, monocytes with reduced immune function and with mainly immunosuppressive functions such as M2-macrophages (245, 249, 250) and HLA-DR-low and HLA-DR-negative monocytes were significantly increased in glioma patients as compared to controls (21, 244, 251), but cell counts might be confounded by dexamethasone use (229). Also, less pro-inflammatory M1-macrophages were observed in glioma patients (249, 250). Total monocyte counts could not be correlated to prognosis (62, 71, 227, 231). Monocytes were found to not be different in glioblastoma patients as compared to patients with brain metastases (207) and increased in glioma patients compared to epilepsy (54), meningioma (54, 55), or acoustic neuroma (54).
The Neutrophil-lymphocyte-ratio (NLR) may be a promising marker for multiple types of cancers (252–255) and has the potential to fulfill various biomarker roles. It is unclear how the NLR can be dysregulated. However, a hypothesis is that tumors, including glioblastoma (256), secrete hematopoietic factors such as granulocyte-colony stimulating factor, granulocyte macrophage-colony stimulating factor and IL-1 and IL-6, which stimulate proliferation of neutrophils (257, 258). Also, tumors can secrete neutrophil attractant chemokines (259) and turn neutrophils from foe into friend via the secretion of TGF-beta (260). Tumor-associated neutrophils can stimulate vascularization of the tumor and inhibit lymphocyte function, weakening the antitumor response (261). NLR and the derived NLR (dNLR; absolute neutrophil count/(WBC count minus absolute neutrophil count) were increased in glioma patients compared to controls (54, 55, 194, 206, 262). Glioma patients with low NLR or derived NLR had longer survival in multiple studies with large patient populations (57, 63, 64, 69, 75, 93, 201, 203, 204, 225, 226, 230, 234, 262–269) with HRs mostly between 1.7 and 2.4. On the contrary, multiple studies including those with larger patient populations could not find a correlation between NLR and survival in glioma patients (62, 71, 198, 200, 205, 227, 247, 270). Furthermore, there is overwhelming evidence that NLR is significantly increased in patients with higher-grade glioma as compared to patients with lower-grade glioma (54–57, 93, 195, 197, 200, 201, 213, 266, 271, 272). The AUC to differentiate between patients with higher-grade and lower-grade glioma was mostly between 0.6 and 0.7. It remains unclear whether NLR values are increased in glioma patients compared to patients with meningioma (54, 55, 206) or intracerebral metastases (206, 207), although it may be increased as compared to patients with epilepsy (54, 206) or acoustic neuroma (54). Also, NLR values might be correlated with IDH-mutation status (56, 63, 69, 201, 225, 226, 267) and increased tissue Ki-67 expression (267, 271). Finally, high NLR correlated with tumor relapse (264), and decrease in NLR during treatment with radiotherapy and concomitant temozolomide was correlated with pseudoprogression (265). To conclude, NLR might be correlated with clinicopathological markers, survival and tumor grade. However, there is a lot of conflicting evidence for most of these markers. There is overwhelming evidence that NLR is related to tumor grade, but the accuracies reported are too limited to apply NLR as a definite diagnostics biomarker in the clinics.
It remains unclear whether the platelet-lymphocyte-ratio (PLR) and monocyte-lymphocyte-ratio may have use as a blood-based marker in glioma. Controversial results have been found for both PLR (54, 55, 206) and MLR (54, 194) as diagnostic markers to differentiate between glioma patients and controls. Similar controversial results have also been found for PLR (54–56, 197, 201, 263, 266, 271) and MLR (54, 58, 197, 266, 271) as tumor grade differentiating markers. PLR (63, 64, 69, 71, 75, 198, 204, 205, 263, 266, 267, 269) as well as MLR (69, 71, 75, 267, 271) were found to not be prognostic in glioma patients in the majority of studies. However, PLR and MLR might have some value as brain disease differentiating marker for glioma patients as these markers were significantly different in glioma patients as compared to patients with epilepsy (54, 206) and non-glial brain malignancies metastases (54, 55, 206, 207). Both markers were rarely correlated with tumor tissue IDH-mutation (56, 63, 69, 201, 267) or MGMT-methylation status, and Ki-67 proliferation index (267, 271).
The systemic immune inflammation (SII) index can be calculated as follows: platelets * (neutrophils/lymphocytes). A high SII-index was correlated with short survival in patients with different cancer types (273–275). In glioma, an increased SII-index was found in patients with higher tumor grades (58, 70, 195, 196) with AUCs of 0.6-0.8 (58, 196), respectively. The SII-index was correlated with poorer prognosis (70, 267), and patients with tumors with higher tissue Ki-67 proliferation index (196), but was not correlated with tumor size (70).
Dendritic cells are antigen presenting cells that can present antigens for example from tumor cells to T-cells, which subsequently activates these T-cells. Total dendritic cells and its subpopulations (myeloid/conventional dendritic cells and plasmacytoid dendritic cells) were found to be decreased in blood of glioma patients compared to controls (21, 243, 276), and these cell populations were also decreased in glioblastoma patients compared to patients with lower tumor grades (243). Furthermore, it was reported that an immature dendritic cell population with increased immunoinhibitory effects on cells (277) becomes increased in glioma patients, especially in patients with higher tumor grades (243). Therefore, glial tumors might actively weaken a patient’s immune system.
Myeloid-derived suppressor cells (MDSCs) are immunoinhibitory cells originating from monocytes. MDSCs might be formed during direct cell-cell contact with tumor cells possibly during infiltration of the glial tumor (106). There are variable results concerning in which glioma patients MDSC counts are changed. Total MDSCs (33, 278–280), monocytic MDSCs (21, 33, 280, 281) and granulocytic MDSCs (33, 278–281) were often significantly increased in glioblastoma patients but non-significantly altered in patients with lower grades. Furthermore, MDSCs were increased in patients with poor prognosis (30) and in glioblastoma patients as compared to other patients with intracranial tumors such as anaplastic glioma or meningioma (251).
Tregs are known for their immunosuppressive functions (282) and have been shown to be associated with poor patient prognosis in various cancer types (283). Tregs have been found to be significantly increased in the blood of glioblastoma patients as compared to healthy individuals (23, 229, 240, 284, 285). However, Tregs were also found to be non-significantly altered in glioma (21, 23, 241, 286) or even significantly decreased (237, 240).
Extracellular vesicles (EVs) are microparticles that are 30 to 10.000 nanometer in diameter. These vesicles are released by cells and can carry proteins, lipids and nucleic acids from one cell to another, thereby facilitating communication between cells (287). Extracellular vesicles can be released from the plasma membrane itself as microvesicles or can be released after fusion of endosomes inside a cell with the plasma membrane as exosomes (288). In glioma patients numbers of EVs (289, 290), microparticles (291) and exosomes (292) in blood were increased as compared to healthy individuals. EVs could potentially also be used as markers for tumor relapse (289) or tumor progression as opposed to pseudoprogression (293). Furthermore, the cargo of EVs can be employed as biomarkers. The protein cargo level – that is the total amount of protein loaded – from glioma patients might have value as diagnostic marker (294, 295). Also, the protein cargo itself is dysregulated and can be used to differentiate between a group of healthy individuals and patients with less malignant glial tumors, which have similar protein cargo, and patients with highly malignant glial tumors (296). EV protein cargo from glioblastoma patients was enriched in proteins that were associated with inflammation, immune response, members of the complement coagulation cascade and others (289). Other studies found a decrease in immune system related proteins IFN-γ, IL-10, and IL-3 within plasma exosomes from glioma patients (292). Furthermore, RNA inside exosomes may increase tumor cell invasion and repress apoptosis (297). Lastly, the surface protein profile of EVs are dysregulated (298, 299) and can be used as biomarkers to differentiate between glioma patients and healthy individuals with high accuracy.
Metabolomics is the analysis of small molecules in a biofluid, cell, tissue, organ or organism (300) and can be used to study metabolic pathways within the organism. Combinations of metabolites such as creatine, glucose and lactate could differentiate patients with brain tumors, glioblastoma, oligodendroglioma, glial tumor, or astrocytoma from healthy individuals with very high accuracy (AUC: 0.9-1.0) (301). Patients with higher grade and lower grade tumors could be differentiated with AUC of 0.7 (301) or 91% accuracy (302). Tumor type differentiating metabolomic panels had variable accuracies with AUCs between 0.4-0.8 (301, 303). Tumor tissue IDH-mutation status could be predicted with an accuracy of 94% (302). Single metabolites (303, 304) and metabolite combinations (303) could predict survival of glioma patients, even with near perfect accuracies. Remarkably, serum metabolites such as tocopherols were found in two studies that could predict glioblastoma up to 22 years before manifestation (305) and glioma patients up to 9 years before manifestation (306). The metabolic pathways that were dysregulated were often involved energy metabolism including amino acid metabolism (302, 303, 306, 307), lipid metabolism (303, 306, 307), nucleic acid metabolism (302) and carbohydrate metabolism (302, 306, 307). Glucose and lactate in particular are interesting markers and had value as blood biomarkers with several purposes, possibly due to their role in the Warburg effect. Glucose levels were reported to be increased in patients with higher tumor grades (308) or worse survival (308–311). One study reported that this was independent of the degree of disability, tumor grade, diabetes, prolonged dexamethasone use, or subsequent treatment modalities (309). Furthermore, it has been found that glucose was related to tumor progression and it was higher in patients with glial brain tumors such as glioblastoma and oligodendrogliomas, but not in meningioma, as compared to healthy individuals (301). Pre-treatment lactate levels (302, 312, 313) were increased in patients with high-grade glioma compared to low-grade glioma patients with AUCs of 0.7 (312) and 1.0 (313), and could potentially also be used as a diagnostic marker (307).
Using summaries of the methodology and results of the studies that we referenced here (see Supplemental Tables 3–9), we assessed risk of bias in the biomarker studies, similar to some degree to the QUADAS-2 (314) and REMARK (315) guidelines for quality assessment of diagnostic and prognostic biomarker studies. We noted several limitations in the studies that were reviewed concerning study population size, presentation of results and registration of effect of intrinsic and extrinsic factors that could influence marker levels. Apart from markers that are measured on a routine basis such as some APRPs or inflammatory cell populations in clinical chemistry labs, the study populations of markers are often small (often <100 individuals included). Also, small validation cohorts are used or validation cohorts were not included at all. Primarily glioblastoma patients are included in the studies that we referenced here. Patients with lower-grade gliomas are rarely included or comprise a small portion of the entire patient population. Therefore, it is unclear whether the biomarkers that we selected as being most promising, will be of value in particularly these patients. Furthermore, the majority of studies only reported the p-values of biomarkers and not the value of biomarkers quantified as accuracy, sensitivity, specificity and/or hazard ratio, and these could not be deduced from the available and presented data. Therefore, it is unclear what the clinical value of most biomarkers is. Lastly, it is largely unknown to what extent biomarkers are affected by extrinsic factors such as anti-tumor therapy, use of (co-)medication, choice of analytical methods, and by intra-individual factors such as race, comorbidities and others. Co-medication use by patients before sampling, such as corticosteroid use, is reported in some studies. Use of other medication, e.g. anti-diabetic and anti-epileptic drugs, is rarely reported. Most studies that reported use of these drugs did not (statistically) analyze the effect of these drugs on the biomarkers that were studied, a potential bias that should be taken into account when interpreting these data.
In order to assess the potential reproducibility, and ultimately clinical validation, of the most promising markers GFAP, IL-10, and miR-21, we precisely evaluated the available studies using dedicated guidelines. The MIQE guideline was employed for the miRNA (316), whereas we had to adjust existing guidelines to assess the studies for GFAP and IL-10 (Supplementary Table 12), as to the best of our knowledge no such guidelines are available for ELISA/immunoassays. As can be seen in Supplementary Figure 1, for GFAP and IL-10 essential factors of the study design such as the number of included patients, protein detection methods and kits were almost always mentioned. However, other important factors such as the used (analytical) instrumentation, sample storage and sample preparation procedures were rarely reported. Also, it was often not reported whether samples were quality controlled by evaluating intra-assay and inter-assay variability. Furthermore, test accuracy is often not reported which makes it unclear whether the tests will have value in the clinical settings. Lastly, factors such as comedication use, histopathological marker presence and tumor volume are rarely reported, which can have significant impact on biomarker concentrations. For miR-21, several categories of the guidelines were often sufficiently described such as experimental design, sample processing and storage. However, other categories such as ‘nucleic acid extraction’, ‘qPCR target information’ and ‘qPCR protocol’ were rarely sufficiently described or not described at all (Supplementary Figure 2). In all, it again highlights that adequate reporting of employed methods is of importance to ensure reproducibility of the identified biomarker.
Thus, it can be concluded that there is room for improvement in biomarker studies in multiple domains of methodology and results presentation, as has been reported by other (systematic) reviews (317–319). The biomarker studies that we referenced here may not be of the highest possible quality and cannot be used to determine immediately which biomarkers will have clinical value. However, they can still be used to determine which biomarkers are promising for further research, as markers that have shown great clinical group differentiating abilities in multiple studies may still hold clinical value despite the bias in results and methodologies present in the studies.
Glioma is still one of the most devastating diseases with high burden. Any additional information that can be obtained from the patient regarding tumor development, growth, behavior, and vulnerabilities, in a least minimally invasive way is desired. Many studies have been published, and included in this systematic review, that identified potential circulating biomarkers for patients with glioma, at each point in the glioma patients clinical course (Figure 1). Unfortunately, none of the biomarkers is in our opinion ready for direct clinical implementation.
As opposed to tumor tissue biomarkers, such as MGMT methylation, 1p/19q codeletion, IDH1-mutations, and the recently introduced methylation profiling (320) in glioma, blood-based markers often reflect local or systemic responses of the endogenous processes to the presence of a tumor. Direct measurement of glioma-derived circulating cells and genomic aberrations is an exception in this view. Cells, cell ratios and APRPs that are often measured in complete blood counts (CBCs), are attractive biomarkers as CBCs are regularly used in the clinic and extensive research has already been performed on their utility as biomarkers. However, they are possibly insufficiently accurate biomarkers for clinical utility as a single marker or in combination with other cells, cell ratios or APRPs. An explanation for the worse performance of single markers as opposed to panels of markers may be interpreted using the framework of hallmarks and enabling characteristics of cancer, as formulated in the seminal article by Hanahan and Weinberg (321). Hallmarks are traits unique to cancer cells and enabling characteristics are traits that lead to the development of such hallmarks. In this framework, blood biomarkers including VEGF, miR-182 and YKL-40 may be mediating factors that enable cancer cells to contain the hallmarks ‘inducing angiogenesis’, ‘resisting cell death’ and ‘tissue invasion’. Other biomarkers such as lactate concentrations and CGTC can be seen as an expression of the hallmarks or enabling characteristics ‘deregulated cellular energetics’ and ‘activating invasion and metastasis’. Inflammatory cells may be promoting the enabling characteristic ‘tumor promoting inflammation’ (see Supplemental Table 11 and Figure 4). As single blood markers have low to modest accuracies and value as biomarkers but panels of biomarkers often have higher accuracies, it can be hypothesized that screening of multiple markers involved in multiple hallmarks or enabling characteristics may improve biomarker accuracy. This hypothesis can be supported by the fact that diagnostic sensitivity of inflammatory cells such as NLR, PLR, neutrophils and others is limited with diagnostic and tumor grade and tumor type differentiating AUCs between 0.6-0.7 (54–56, 58, 201). Similar results also have been found for APRPs with AUCs between 0.5-0.7 (54–56, 58). Combination of inflammatory cell populations (54, 56, 58) or APRPs (56), as well as combination of inflammatory cell populations with APRPs (54, 56), does not increase accuracy in a meaningful way (144). Thus, it is possible that both APRPs as well as inflammatory cell populations already reflect alterations in the inflammation enabling characteristic and combination of these markers does not further improve marker accuracies. Also, panels of biomarkers often have higher accuracies than single biomarkers irrespective of the biomarker function and these panels contain biomarkers involved in multiple pathways related to the hallmarks of cancer and its enabling characteristics (13, 52, 155). Furthermore, our analysis indicates that biomarker levels become increasingly dysregulated as tumors increase in malignancy, as biomarker levels are often positively correlated with tumor grade, worse survival and/or more malignant tumor types. Therefore, cellular hallmarks might develop in more cancer cells as tumor malignancy increases and this may be reflected in the dysregulation of circulating biomarkers. With this in mind, we propose to introduce multi-biosource, multi-biomolecule-based blood tests for glioma patients. Keeping the criteria for biomarker test development as discussed above in mind, such tests may likely include (components of) a miRNA, protein, or platelet RNA panel, perhaps including the already promising single markers miR-21, IL-10, and GFAP. These panels likely include multiple components of the tumor progression, are less resistant to confounding variables due to its high dimensions, and far more accurate than a single measured biomarker. Also, complementary implementation of several biomarker types may make synergistically use of each other’s advantages, and perhaps at least partially reduce each other’s disadvantages (Table 1) (144).
Figure 4 Correlation of blood-based biomarkers in patients with glioma with seminal events in tumorigenesis. Blood biomarkers in glioma patients are implicated in the molecular pathways as detailed by Hanahan and Weinberg (321). Markers colored in green were mostly found to be increased in the circulation of glioma patients compared to healthy individuals, and in glioma patients with more malignant tumors compared to patients with less malignant tumors. Markers that were inversely correlated were colored in red. Markers without color were found to be either significantly increased or decreased in the formerly mentioned groups. The abbreviation “DC” indicates dendritic cells and “iDC” indicates immature dendritic cells. Adapted from “Hallmarks of Cancer: Circle”, by BioRender.com (2021). Retrieved from: https://app.biorender.com/biorender-templates.
Hence, additional validation of the currently most promising markers (Figure 4) is also required. Aside analysis of blood, other biofluids such as urine or perhaps cerebrospinal fluid may also be rich sources of biomarkers. Recent analysis has shown that tumor evolution could be tracked via repeated CSF samplings (322). Similarly, perhaps also other body fluids such as saliva, sputum, or breathing air may contain molecular information traceable to a primary glioma. We believe that blood-based biomarkers may currently only at maximum complement the current methods to diagnose and/or monitor a glioma, such as clinical symptoms, imaging, and tissue collection via tumor resection or (stereotactic) biopsy. It may very well be anticipated that blood-based biomarkers are included in a future setting in clinical decision making, for example in multidisciplinary tumor boards, once such biomarkers are thoroughly validated. For this, systematic biobanking of blood from glioma patients is required. Such biobanking requires research funds that support these efforts, as well as research project that in a dedicated way screen for relevant and valuable biomarkers in well-annotated, large, and homogeneous patient series. It is of importance that any future biomarker discovery or validation research is reported according to the highest standards, facilitating reproducibility of the found results. Alternatively, we believe that any clinical trial, even in a phase 1 stage, should include a blood-biomarker branch in the trial design, in order to at least aim to discover a companion diagnostics biomarker. Also, blood-based biomarkers that may complement current imaging methods for the identification of true tumor progression versus pseudo-tumor progression is required.
In all, the glioma research community should be encouraged towards additional identification and inclusion of blood-based biomarker research in a clinical setting. While currently at the stage of analytical validation and start of clinical validation, further studies should focus on demonstrating its clinical utility.
The original contributions presented in the study are included in the article/Supplementary Material. Further inquiries can be directed to the corresponding author.
HA, RH, and MGB developed the search strategy and developed the selection criteria. HA, RH, and MA selected studies for inclusion through title, abstract, and full-text screening. HA, TW, and MGB drafted the manuscript. All authors contributed to the article and approved the submitted version.
Financial support was provided by stichting STOPHersentumoren.nl (TW and MGB).
The authors declare that the research was conducted in the absence of any commercial or financial relationships that could be construed as a potential conflict of interest.
The Supplementary Material for this article can be found online at: https://www.frontiersin.org/articles/10.3389/fonc.2021.665235/full#supplementary-material
1. Best MG, Sol N, Zijl S, Reijneveld JC, Wesseling P, Wurdinger T. Liquid Biopsies in Patients With Diffuse Glioma. Acta Neuropathol (2015) 129:849–65. doi: 10.1007/s00401-015-1399-y
2. Zachariah MA, Oliveira-Costa JP, Carter BS, Stott SL, Nahed BV. Blood-Based Biomarkers for the Diagnosis and Monitoring of Gliomas. Neuro Oncol (2018) 20:1155–61. doi: 10.1093/neuonc/noy074
3. Holdhoff M, Yovino SG, Boadu O, Grossman SA. Blood-Based Biomarkers for Malignant Gliomas. J Neurooncol (2013) 113:345–52. doi: 10.1007/s11060-013-1144-0
4. Weller M, van den Bent M, Preusser M, Le Rhun E, Tonn JC, Minniti G, et al. EANO Guidelines on the Diagnosis and Treatment of Diffuse Gliomas of Adulthood. Nat Rev Clin Oncol (2020) 17:30. doi: 10.1038/s41571-020-00447-z
5. Tarassishin L, Casper D, Lee SC. Aberrant Expression of Interleukin-1β and Inflammasome Activation in Human Malignant Gliomas. PloS One (2014) 9:e103432. doi: 10.1371/journal.pone.0103432
6. Van Meir E, Sawamura Y, Diserens A-CC, Hamou M-FF, de Tribolet N. Human Glioblastoma Cells Release Interleukin 6 in Vivo and In Vitro. Cancer Res (1990) 50:6683–8.
7. a Dzaye OD, Hu F, Derkow K, Haage V, Euskirchen P, Harms C, et al. Glioma Stem Cells But Not Bulk Glioma Cells Upregulate IL-6 Secretion in Microglia/Brain Macrophages Via Toll-like Receptor 4 Signaling. J Neuropathol Exp Neurol (2016) 75:429–40. doi: 10.1093/jnen/nlw016
8. Nijaguna MB, Patil V, Urbach S, Shwetha SD, Sravani K, Hegde AS, et al. Glioblastoma-Derived Macrophage Colony-Stimulating Factor (MCSF) Induces Microglial Release of Insulin-Like Growth Factor-Binding Protein 1 (IGFBP1) to Promote Angiogenesis. J Biol Chem (2015) 290:23401–15. doi: 10.1074/jbc.M115.664037
9. Ren J, Jia P, Feng H, Li X, Zhao J, Sun Y. Involvement of Poly(Adp-Ribose) Polymerase-1 in Chinese Patients With Gliomaa Potential Target for Effective Patient Care. Int J Biol Markers (2018) 33:68–72. doi: 10.5301/ijbm.5000267
10. Albulescu R, Codrici E, Popescu ID, Mihai S, Necula LG, Petrescu D, et al. Cytokine Patterns in Brain Tumour Progression. Mediators Inflamm (2013) 2013:979748. doi: 10.1155/2013/979748
11. Gao Y, Zhang E, Liu B, Zhou K, He S, Feng L, et al. Integrated Analysis Identified Core Signal Pathways and Hypoxic Characteristics of Human Glioblastoma. J Cell Mol Med (2019) 23:6228–37. doi: 10.1111/jcmm.14507
12. Shamsdin SA, Mehrafshan A, Rakei SM, Mehrabanilare D. Evaluation of VEGF, FGF and PDGF and Serum Levels of Inflammatory Cytokines in Patients With Glioma and Meningioma in Southern Iran. Asian Pac J Cancer Prev (2019) 20:2883–90. doi: 10.31557/APJCP.2019.20.10.2883
13. Nijaguna MB, Patil V, Hegde AS, Chandramouli BA, Arivazhagan A, Santosh V, et al. An Eighteen Serum Cytokine Signature for Discriminating Glioma From Normal Healthy Individuals. PloS One (2015) 10:e0137524. doi: 10.1371/journal.pone.0137524
14. Reynés G, Vila V, Martín M, Parada A, Fleitas T, Reganon E, et al. Circulating Markers of Angiogenesis, Inflammation, and Coagulation in Patients With Glioblastoma. J Neurooncol (2011) 102:35–41. doi: 10.1007/s11060-010-0290-x
15. Deniz ÇD, Gürbilek M, Koç M. Prognostic Value of Interferon-Gamma, Interleukin-6, and Tumor Necrosis Factor-Alpha in the Radiation Response of Patients Diagnosed With Locally Advanced Non-Small-Cell Lung Cancer and Glioblastoma Multiforme. Turk J Med Sci (2018) 48:117–23. doi: 10.3906/sag-1611-77
16. Carlsson A, Persson O, Ingvarsson J, Widegren B, Salford L, Borrebaeck CAKK, et al. Plasma Proteome Profiling Reveals Biomarker Patterns Associated With Prognosis and Therapy Selection in Glioblastoma Multiforme Patients. Proteomics Clin Appl (2010) 4:591–602. doi: 10.1002/prca.200900173
17. Shan Y, He X, Song W, Han D, Niu J, Wang J. Role of IL-6 in the Invasiveness and Prognosis of Glioma. Int J Clin Exp Med (2015) 8:9114–20.
18. Hands JR, Abel P, Ashton K, Dawson T, Davis C, Lea RW, et al. Investigating the Rapid Diagnosis of Gliomas From Serum Samples Using Infrared Spectroscopy and Cytokine and Angiogenesis Factors. Anal Bioanal Chem (2013) 405:7347–55. doi: 10.1007/s00216-013-7163-z
19. Xu BJ, An QA, Gowda SS, Yan W, Pierce LA, Abel TW, et al. Identification of Blood Protein Biomarkers That Aid in the Clinical Assessment of Patients With Malignant Glioma. Int J Oncol (2012) 40:1995–2003. doi: 10.3892/ijo.2012.1355
20. Gousias K, Markou M, Arzoglou V, Voulgaris S, Vartholomatos G, Kostoula A, et al. Frequent Abnormalities of the Immune System in Gliomas and Correlation With the WHO Grading System of Malignancy. J Neuroimmunol (2010) 226:136–42. doi: 10.1016/j.jneuroim.2010.05.027
21. Löhr M, Freitag B, Technau A, Krauss J, Monoranu C-MM, Rachor J, et al. High-Grade Glioma Associated Immunosuppression Does Not Prevent Immune Responses Induced by Therapeutic Vaccines in Combination With Treg Depletion. Cancer Immunol Immunother (2018) 67:1545–58. doi: 10.1007/s00262-018-2214-0
22. Kmiecik J, Poli A, Brons NHC, Waha A, Eide GE, Enger PØ, et al. Elevated CD3+ and CD8+ Tumor-Infiltrating Immune Cells Correlate With Prolonged Survival in Glioblastoma Patients Despite Integrated Immunosuppressive Mechanisms in the Tumor Microenvironment and at the Systemic Level. J Neuroimmunol (2013) 264:71–83. doi: 10.1016/j.jneuroim.2013.08.013
23. Mostafa H, Pala A, Högel J, Hlavac M, Dietrich E, Westhoff MA, et al. Immune Phenotypes Predict Survival in Patients With Glioblastoma Multiforme. J Hematol Oncol (2016) 9:77. doi: 10.1186/s13045-016-0272-3
24. Bryant NL, Suarez-Cuervo C, Gillespie GY, Markert JM, Nabors LB, Meleth S, et al. Characterization and Immunotherapeutic Potential of γδ T-cells in Patients With Glioblastoma. Neuro Oncol (2009) 11:357–67. doi: 10.1215/15228517-2008-111
25. Holst CB, Christensen IJ, Skjøth-Rasmussen J, Hamerlik P, Poulsen HS, Johansen JS. Systemic Immune Modulation in Gliomas: Prognostic Value of Plasma IL-6, YKL-40, and Genetic Variation in YKL-40. Front Oncol (2020) 10:478. doi: 10.3389/fonc.2020.00478
26. Doroudchi M, Pishe ZG, Malekzadeh M, Golmoghaddam H, Taghipour M, Ghaderi A. Elevated Serum IL-17A But Not IL-6 in Glioma Versus Meningioma and Schwannoma. Asian Pac J Cancer Prev (2013) 14:5225–30. doi: 10.7314/APJCP.2013.14.9.5225
27. Hu J, Mao Y, Li M, Lu Y. The Profile of Th17 Subset in Glioma. Int Immunopharmacol (2011) 11:1173–9. doi: 10.1016/j.intimp.2011.03.015
28. Bunevicius A, Radziunas A, Tamasauskas S, Tamasauskas A, Laws ER, Iervasi G, et al. Prognostic Role of High Sensitivity C-reactive Protein and Interleukin-6 in Glioma and Meningioma Patients. J Neurooncol (2018) 138:351–8. doi: 10.1007/s11060-018-2803-y
29. Demirci U, Yaman M, Buyukberber S, Coskun U, Baykara M, Uslu K, et al. Prognostic Importance of Markers for Inflammation, Angiogenesis and Apoptosis in High Grade Glial Tumors During Temozolomide and Radiotherapy. Int Immunopharmacol (2012) 14:546–9. doi: 10.1016/j.intimp.2012.08.007
30. Marinari E, Allard M, Gustave R, Widmer V, Philippin G, Merkler D, et al. Inflammation and Lymphocyte Infiltration are Associated With Shorter Survival in Patients With High-Grade Glioma. Oncoimmunology (2020) 9:1779990. doi: 10.1080/2162402X.2020.1779990
31. Chiorean R, Berindan-Neagoe I, Braicu C, Florian IS, Leucuta D, Crisan D, et al. Quantitative Expression of Serum Biomarkers Involved in Angiogenesis and Inflammation, in Patients With Glioblastoma Multiforme: Correlations With Clinical Data. Cancer Biomark (2014) 14:185–94. doi: 10.3233/CBM-130310
32. Bresnick AR, Weber DJ, Zimmer DB. S100 Proteins in Cancer. Nat Rev Cancer (2015) 15:96–109. doi: 10.1038/nrc3893
33. Gielen PR, Schulte BM, Kers-Rebel ED, Verrijp K, Bossman SAJFH, Ter Laan M, et al. Elevated Levels of Polymorphonuclear Myeloid-Derived Suppressor Cells in Patients With Glioblastoma Highly Express S100A8/9 and Arginase and Suppress T Cell Function. Neuro Oncol (2016) 18:1253–64. doi: 10.1093/neuonc/now034
34. Arora A, Patil V, Kundu P, Kondaiah P, Hegde AS, Arivazhagan A, et al. Serum Biomarkers Identification by iTRAQ and Verification by MRM: S100A8/S100A9 Levels Predict Tumor-Stroma Involvement and Prognosis in Glioblastoma. Sci Rep (2019) 9:1–12. doi: 10.1038/s41598-019-39067-8
35. Popescu ID, Codrici E, Albulescu L, Mihai S, Enciu AM, Albulescu R, et al. Potential Serum Biomarkers for Glioblastoma Diagnostic Assessed by Proteomic Approaches. Proteome Sci (2014) 12:47. doi: 10.1186/s12953-014-0047-0
36. Gautam P, Nair SC, Gupta MK, Sharma R, Polisetty RV, Uppin MS, et al. Proteins With Altered Levels in Plasma From Glioblastoma Patients as Revealed by iTRAQ-Based Quantitative Proteomic Analysis. PloS One (2012) 7:e46153. doi: 10.1371/journal.pone.0046153
37. Zupancic K, Blejec A, Herman A, Veber M, Verbovsek U, Korsic M, et al. Identification of Plasma Biomarker Candidates in Glioblastoma Using an Antibody-Array-Based Proteomic Approach. Radiol Oncol (2014) 48:257–66. doi: 10.2478/raon-2014-0014
38. Lyubimova NV, Toms MG, Popova EE, Bondarenko YV, Krat VB, Kushlinskii NE, et al. Neurospecific Proteins in the Serum of Patients With Brain Tumors. Eksp Biol Med (2010) 150:678–81. doi: 10.1007/s10517-011-1236-9
39. Oktay K, Olguner SK, Sarac ME, Mazhar KÖ, Çetinalp NE, GEZERCAN4 Y, et al. Evaluation of Serum S100b Values in High Grade Glioma Patients. J Neurol Sci (2015) 32:738–46. doi: 10.1007/s11060-016-2204-z
40. Brommeland T, Rosengren L, Fridlund S, Hennig R, Isaksen V. Serum Levels of Glial Fibrillary Acidic Protein Correlate to Tumour Volume of High-Grade Gliomas. Acta Neurol Scand (2007) 116:380–4. doi: 10.1111/j.1600-0404.2007.00889.x
41. Vos MJ, Postma TJ, Martens F, Uitdehaag BMJ, Blankenstein MA, Vandertop WP, et al. Serum Levels of S-100B Protein and Neuron-specific Enolase in Glioma Patients: A Pilot Study. Anticancer Res (2004) 24:2511–4.
42. Holla FK, Postma TJ, Blankenstein MA, van Mierlo TJM, Vos MJ, Sizoo EM, et al. Prognostic Value of the S100B Protein in Newly Diagnosed and Recurrent Glioma Patients: A Serial Analysis. J Neurooncol (2016) 129:525–32. doi: 10.1007/s11060-016-2204-z
43. Balkwill F. Tumour Necrosis Factor and Cancer. Nat Rev Cancer (2009) 9:361–71. doi: 10.1038/nrc2628
44. Villeneuve J, Tremblay P, Vallières L. Tumor Necrosis Factor Reduces Brain Tumor Growth by Enhancing Macrophage Recruitment and Microcyst Formation. Cancer Res (2005) 65:3928–36. doi: 10.1158/0008-5472.CAN-04-3612
45. Rahbar A, Cederarv M, Wolmer-Solberg N, Tammik C, Stragliotto G, Peredo I, et al. Enhanced Neutrophil Activity is Associated With Shorter Time to Tumor Progression in Glioblastoma Patients. Oncoimmunology (2016) 5:e1075693. doi: 10.1080/2162402X.2015.1075693
46. Kushner AM I. Acute Phase Proteins as Disease Markers. Disease Markers J (1987) 5: (1):1–11. Pubmed.
47. Pang WW, Abdul-Rahman PS, Wan-Ibrahim WI, Hashim OH. Can the Acute-Phase Reactant Proteins be Used as Cancer Biomarkers? Int J Biol Markers (2010) 25:1–11. doi: 10.1177/172460081002500101
48. Weiss JF, Morantz RA, Bradley WP, Chretien PB. Serum Acute-Phase Proteins and Immunoglobulins in Patients With Gliomas. Cancer Res (1979) 39:542–4.
49. Matsuura H, Nakazawa S. Prognostic Significance of Serum Alpha 1-Acid Glycoprotein in Patients With Glioblastoma Multiforme: A Preliminary Communication. Neurosurg Psychiatry (1985) 48:835–7. doi: 10.1136/jnnp.48.8.835
50. Mohan Kumar D, Thota B, Vijay Shinde S, Prasanna KV, Hegde AS, Arivazhagan A, et al. Proteomic Identification of Haptoglobin Alpha2 as a Glioblastoma Serum Biomarker: Implications in Cancer Cell Migration and Tumor Growth. J Proteome Res (2010) 9:5557–67. doi: 10.1021/pr1001737
51. Gollapalli K, Ray S, Srivastava R, Renu D, Singh P, Dhali S, et al. Investigation of Serum Proteome Alterations in Human Glioblastoma Multiforme. Proteomics (2012) 12:2378–90. doi: 10.1002/pmic.201200002
52. Nijaguna MB, Schröder C, Patil V, Shwetha SD, Hegde AS, Chandramouli BA, et al. Definition of a Serum Marker Panel for Glioblastoma Discrimination and Identification of Interleukin 1β in the Microglial Secretome as a Novel Mediator of Endothelial Cell Survival Induced by C-reactive Protein. J Proteomics (2015) 128:251–61. doi: 10.1016/j.jprot.2015.07.026
53. Miyauchi E, Furuta T, Ohtsuki S, Tachikawa M, Uchida Y, Sabit H, et al. Identification of Blood Biomarkers in Glioblastoma by SWATH Mass Spectrometry and Quantitative Targeted Absolute Proteomics. PloS One (2018) 13:e0193799. doi: 10.1371/journal.pone.0193799
54. Zheng SH, Huang JL, Chen M, Wang BL, Ou QS, Huang SY. Diagnostic Value of Preoperative Inflammatory Markers in Patients With Glioma: A Multicenter Cohort Study. J Neurosurg (2018) 129:583–92. doi: 10.3171/2017.3.JNS161648
55. Liu S, Zhu Y, Zhang C, Meng X, Sun B, Zhang G, et al. The Clinical Significance of Soluble Programmed Cell Death-Ligand 1 (sPD-L1) in Patients With Gliomas. Front Oncol (2020) 10:9. doi: 10.3389/fonc.2020.00009
56. Wang PF, Meng Z, Song HW, Yao K, Duan ZJ, Yu CJ, et al. Preoperative Changes in Hematological Markers and Predictors of Glioma Grade and Survival. Front Pharmacol (2018) 9:886. doi: 10.3389/fphar.2018.00886
57. Wu Y, Song Z, Sun K, Rong S, Gao P, Wang F, et al. A Novel Scoring System Based on Peripheral Blood Test in Predicting Grade and Prognosis of Patients With Glioma. Onco Targets Ther (2019) 12:11413–23. doi: 10.2147/OTT.S236598
58. Huang Z, Wu L, Hou Z, Zhang P, Li G, Xie J. Eosinophils and Other Peripheral Blood Biomarkers in Glioma Grading: A Preliminary Study. BMC Neurol (2019) 19:313. doi: 10.1186/s12883-019-1549-2
59. He ZQ, Ke C, Al-Nahari F, Duan H, Guo CC, Wang Y, et al. Low Preoperative Prognostic Nutritional Index Predicts Poor Survival in Patients With Newly Diagnosed High-Grade Gliomas. J Neurooncol (2017) 132:239–47. doi: 10.1007/s11060-016-2361-0
60. Tadej Strojnik TŠ and TTL. Prognostic Value of Erythrocyte Sedimentation Rate and C-Reactive Protein in the Blood of Patients With Glioma. Anticancer Res (2014) 34:339–47.
61. Pierscianek D, Ahmadipour Y, Michel A, Chihi M, Oppong MD, Kebir S, et al. Prediction of Preoperative Survival in Patients With Glioblastoma by Routine Inflammatory Laboratory Parameters. Anticancer Res (2020) 40:1161–6. doi: 10.21873/anticanres.14058
62. Maas SLN, Draaisma K, Snijders TJ, Senders JT, Berendsen S, Seute T, et al. Routine Blood Tests Do Not Predict Survival in Patients With Glioblastoma—Multivariable Analysis of 497 Patients. World Neurosurg (2019) 126:e1081–91. doi: 10.1016/j.wneu.2019.03.053
63. Auezova R, Ivanova N, Akshulakov S, Zhetpisbaev B, Kozhakhmetova A, Ryskeldiyev N, et al. Isocitrate Dehydrogenase 1 Mutation is Associated With Reduced Levels of Inflammation in Glioma Patients. Cancer Manag Res (2019) 11:3227–36. doi: 10.2147/CMAR.S195754
64. Hao Y, Li X, Chen H, Huo H, Liu Z, Tian F, et al. A Cumulative Score Based on Preoperative Neutrophil-Lymphocyte Ratio and Fibrinogen in Predicting Overall Survival of Patients With Glioblastoma Multiforme. World Neurosurg (2019) 128:e427–33. doi: 10.1016/j.wneu.2019.04.169
65. He ZQ, Duan H, Ke C, Zhang XH, Guo CC, Al-Nahari F, et al. Evaluation of Cumulative Prognostic Score Based on Pretreatment Plasma Fibrinogen and Serum Albumin Levels in Patients With Newly Diagnosed High-Grade Gliomas. Oncotarget (2017) 8:49605–14. doi: 10.18632/oncotarget.17849
66. Han S, Huang Y, Li Z, Hou H, Wu A. The Prognostic Role of Preoperative Serum Albumin Levels in Glioblastoma Patients. BMC Cancer (2015) 15:108. doi: 10.1186/s12885-015-1125-0
67. Schwartzbaum JA, Lal P, Evanoff W, Mamrak S, Yates A, Barnett GH, et al. Presurgical Serum Albumin Levels Predict Survival Time From Glioblastoma Multiforme. J Neurooncol (1999) 43:35–41. doi: 10.1023/A:1006269413998
68. Borg N, Guilfoyle MR, Greenberg DC, Watts C, Thomson S. Serum Albumin and Survival in Glioblastoma Multiforme. J Neurooncol (2011) 105:77–81. doi: 10.1007/s11060-011-0562-0
69. Wang PF, Song HW, Cai HQ, Kong LW, Yao K, Jiang T, et al. Preoperative Inflammation Markers and IDH Mutation Status Predict Glioblastoma Patient Survival. Oncotarget (2017) 8:50117–23. doi: 10.18632/oncotarget.15235
70. Liang R, Li J, Tang X, Liu Y. The Prognostic Role of Preoperative Systemic Immune-Inflammation Index and Albumin/Globulin Ratio in Patients With Newly Diagnosed High-Grade Glioma. Clin Neurol Neurosurg (2019) 184:105397. doi: 10.1016/j.clineuro.2019.105397
71. Zhou XW, Dong H, Yang Y, Luo JW, Wang X, Liu YH, et al. Significance of the Prognostic Nutritional Index in Patients With Glioblastoma: A Retrospective Study. Clin Neurol Neurosurg (2016) 151:86–91. doi: 10.1016/j.clineuro.2016.10.014
72. Wang PF, Zhang J, Cai HQ, Meng Z, Yu CJ, Li SW, et al. Sanbo Scoring System, Based on Age and Pre-Treatment Hematological Markers, is a Non-Invasive and Independent Prognostic Predictor for Patients With Primary Glioblastomas: A Retrospective Multicenter Study. J Cancer (2019) 10:5654–60. doi: 10.7150/jca.33047
73. Xu WZ, Li F, Xu ZK, Chen X, Sun B, Cao JW, et al. Preoperative Albumin-to-Globulin Ratio and Prognostic Nutrition Index Predict Prognosis for Glioblastoma. Onco Targets Ther (2017) 10:725–33. doi: 10.2147/OTT.S127441
74. Rigamonti A, Imbesi F, Silvani A, Lamperti E, Agostoni E, Porcu L, et al. Prognostic Nutritional Index as a Prognostic Marker in Glioblastoma: Data From a Cohort of 282 Italian Patients. J Neurol Sci (2019) 400:175–9. doi: 10.1016/j.jns.2019.04.002
75. Zhang ZY, Zhan YB, Zhang FJ, Yu B, Ji YC, Zhou JQ, et al. Prognostic Value of Preoperative Hematological Markers Combined With Molecular Pathology in Patients With Diffuse Gliomas. Aging (Albany NY) (2019) 11:6252–72. doi: 10.18632/aging.102186
76. Ding JD, Yao K, Wang PF, Yan CX. Clinical Significance of Prognostic Nutritional Index in Patients With Glioblastomas. Medicine (United States) (2018) 97:e13218. doi: 10.1097/MD.0000000000013218
77. Vos PE, Jacobs B, Andriessen TMJC, Lamers KJB, Borm GF, Beems T, et al. GFAP and S100B are Biomarkers of Traumatic Brain Injury: An Observational Cohort Study. Neurology (2010) 75:1786–93. doi: 10.1212/WNL.0b013e3181fd62d2
78. Herrmann M, Vos P, Wunderlich MT, De Bruijn CHMM, Lamers KJB. Release of Glial Tissue-Specific Proteins After Acute Stroke: A Comparative Analysis of Serum Concentrations of Protein S-100B and Glial Fibrillary Acidic Protein. Stroke (2000) 31:2670–7. doi: 10.1161/01.STR.31.11.2670
79. Husain H, Savage W, Grossman SA, Ye X, Burger PC, Everett A, et al. Pre- and Post-Operative Plasma Glial Fibrillary Acidic Protein Levels in Patients With Newly Diagnosed Gliomas. J Neurooncol (2012) 109:123–7. doi: 10.1007/s11060-012-0874-8
80. Kiviniemi A, Gardberg M, Frantzén J, Parkkola R, Vuorinen V, Pesola M, et al. Serum Levels of GFAP and EGFR in Primary and Recurrent High-Grade Gliomas: Correlation to Tumor Volume, Molecular Markers, and Progression-Free Survival. J Neurooncol (2015) 124:237–45. doi: 10.1007/s11060-015-1829-7
81. Baumgarten P, Quick-Weller J, Gessler F, Wagner M, Tichy J, Forster MT, et al. Pre- and Early Postoperative GFAP Serum Levels in Glioma and Brain Metastases. J Neurooncol (2018) 139:541–6. doi: 10.1007/s11060-018-2898-1
82. Jung CS, Foerch C, Schänzer A, Heck A, Plate KH, Seifert V, et al. Serum GFAP is a Diagnostic Marker for Glioblastoma Multiforme | Brain | Oxford Academic. Brain (2007) 130:3336–41. doi: 10.1093/brain/awm263
83. Vietheer JM, Rieger J, Wagner M, Senft C, Tichy J, Foerch C. Serum Concentrations of Glial Fibrillary Acidic Protein (GFAP) do Not Indicate Tumor Recurrence in Patients With Glioblastoma. J Neurooncol (2017) 135:193–9. doi: 10.1007/s11060-017-2565-y
84. Lyubimova NV, Timofeev YS, Mitrofanov AA, Bekyashev AK, Goncharova ZA, Kushlinskii NE. Glial Fibrillary Acidic Protein in the Diagnosis and Prognosis of Malignant Glial Tumors. Bull Exp Biol Med (2020) 168:503–6. doi: 10.1007/s10517-020-04741-9
85. Ilhan-Mutlu A, Wagner L, Widhalm G, Wöhrer A, Bartsch S, Czech T, et al. Exploratory Investigation of Eight Circulating Plasma Markers in Brain Tumor Patients. Neurosurg Rev (2013) 36:45–56. doi: 10.1007/s10143-012-0401-6
86. Gállego Pérez-Larraya J, Paris S, Idbaih A, Dehais C, Laigle-Donadey F, Navarro S, et al. Diagnostic and Prognostic Value of Preoperative Combined GFAP, IGFBP-2, and YKL-40 Plasma Levels in Patients With Glioblastoma. Cancer (2014) 120:3972–80. doi: 10.1002/cncr.28949
87. Husain H, Savage W, Everett A, Ye X, Blair C, Romans KE, et al. The Role of Plasma GFAP as a Biomarker for Glioblastoma. J Clin Oncol (2011) 29:2095–5. doi: 10.1200/jco.2011.29.15_suppl.2095
88. Tichy J, Spechtmeyer S, Mittelbronn M, Hattingen E, Rieger J, Senft C, et al. Prospective Evaluation of Serum Glial Fibrillary Acidic Protein (GFAP) as a Diagnostic Marker for Glioblastoma. J Neurooncol (2015) 126:361–9. doi: 10.1007/s11060-015-1978-8
89. Llorens F, Thüne K, Tahir W, Kanata E, Diaz-Lucena D, Xanthopoulos K, et al. YKL-40 in the Brain and Cerebrospinal Fluid of Neurodegenerative Dementias. Mol Neurodegener (2017) 12:83. doi: 10.1186/s13024-017-0226-4
90. Horbinski C, Wang G, Wiley CA. YKL-40 is Directly Produced by Tumor Cells and is Inversely Linked to EGFR in Glioblastomas. Int J Clin Exp Pathol (2010) 3:226–37.
91. Schultz NA, Johansen JS. YKL-40-a Protein in the Field of Translational Medicine: A Role as a Biomarker in Cancer Patients? Cancers (Basel) (2010) 2:1453–91. doi: 10.3390/cancers2031453
92. Kazakova MH, Staneva DN, Koev IG, Staikov DG, Mateva N, Timonov PT, et al. Protein and mRNA levels of YKL-40 in high-grade glioma. Folia Biol (2014) 60:261–70.
93. Gandhi P, Khare R, VasudevGulwani H, Kaur S. Circulatory YKL-40 & NLR: Underestimated Prognostic Indicators in Diffuse Glioma. Int J Mol Cell Med (2018) 7:111–8. doi: 10.22088/IJMCM.BUMS.7.2.111
94. Tanwar MK, Gilbert MR, Holland EC. Gene Expression Microarray Analysis Reveals YKL-40 to be a Potential Serum Marker for Malignant Character in Human Glioma. Cancer Res (2002) 62:4364–8.
95. Bernardi D, Padoan A, Ballin A, Sartori M, Manara R, Scienza R, et al. Serum YKL-40 Following Resection for Cerebral Glioblastoma. J Neurooncol (2012) 107:299–305. doi: 10.1007/s11060-011-0762-7
96. Iwamoto FM, Hottinger AF, Karimi S, Riedel E, Dantis J, Jahdi M, et al. Serum YKL-40 is a Marker of Prognosis and Disease Status in High-Grade Gliomas. Neuro Oncol (2011) 13:1244–51. doi: 10.1093/neuonc/nor117
97. Hormigo A, Gu B, Karimi S, Riedel E, Panageas KS, Edgar MA, et al. YKL-40 and Matrix Metalloproteinase-9 as Potential Serum Biomarkers for Patients With High-Grade Gliomas. Clin Cancer Res (2006) 12:5698–704. doi: 10.1158/1078-0432.CCR-06-0181
98. Chandra A, Jahangiri A, Chen W, Nguyen AT, Yagnik G, Pereira MP, et al. Clonal ZEB1-driven Mesenchymal Transition Promotes Targetable Oncologic Antiangiogenic Therapy Resistance. Cancer Res (2020) 80:1498–511. doi: 10.1158/0008-5472.CAN-19-1305
99. van Linde ME, van der Mijn JC, Pham TV, Knol JC, Wedekind LE, Hovinga KE, et al. Evaluation of Potential Circulating Biomarkers for Prediction of Response to Chemoradiation in Patients With Glioblastoma. J Neurooncol (2016) 129:221–30. doi: 10.1007/s11060-016-2178-x
100. Corsini E, Ciusani E, Gaviani P, Silvani A, Canazza A, Bernardi G, et al. Decrease in Circulating Endothelial Progenitor Cells in Treated Glioma Patients. J Neurooncol (2012) 108:123–9. doi: 10.1007/s11060-012-0805-8
101. Salmaggi A, Eoli M, Frigerio S, Silvani A, Gelati M, Corsini E, et al. Intracavitary VEGF, bFGF, IL-8, IL-12 Levels in Primary and Recurrent Malignant Glioma. J Neurooncol (2003) 62:297–303. doi: 10.1023/A:1023367223575
102. Yang J, Zhao Z, Zhong X. Correlation Analysis of the Clinicopathological Features of Glioma and Expression of p53 and VEGF. Int J Clin Exp Med (2017) 10:3606–11.
103. Nowacka A, Smuczyński W, Rość D, Woźniak—Dąbrowska K, Śniegocki M. Serum VEGF-A Concentrations in Patients With Central Nervous System (CNS) Tumors. PloS One (2018) 13:e0192395. doi: 10.1371/journal.pone.0192395
104. Rafat N, Beck GC, Schulte J, Tuettenbeg J, Vajkoczy P. Circulating Endothelial Progenitor Cells in Malignant Gliomas. Clinical Article. J Neurosurg (2010) 112:43–9. doi: 10.3171/2009.5.JNS081074
105. Reynés G, Martínez-Sales V, Vila V, Balañá C, Pérez-Segura P, Vaz MA, et al. Phase II Trial of Irinotecan and Metronomic Temozolomide in Patients With Recurrent Glioblastoma. Anticancer Drugs (2016) 27:133–7. doi: 10.1097/CAD.0000000000000314
106. Rodrigues JC, Gonzalez GC, Zhang L, Ibrahim G, Kelly JJ, Gustafson MP, et al. Normal Human Monocytes Exposed to Glioma Cells Acquire Myeloid-Derived Suppressor Cell-Like Properties. Neuro Oncol (2010) 12:351–65. doi: 10.1093/neuonc/nop023
107. Labussière M, Cheneau C, Prahst C, Gállego Pérez-Larraya J, Farina P, Lombardi G, et al. Angiopoietin-2 May be Involved in the Resistance to Bevacizumab in Recurrent Glioblastoma. Cancer Invest (2016) 34:39–44. doi: 10.3109/07357907.2015.1088948
108. Ribom D, Larsson A, Pietras K, Smits A. Growth Factor Analysis of Low-Grade Glioma CSF: PDGF and VEGF are Not Detectable. Neurol Sci (2003) 24:70–3. doi: 10.1007/s100720300075
109. Takano S, Yoshii Y, Kondo S, Suzuki H, Maruno T, Shirai S, et al. Concentration of Vascular Endothelial Growth Factor in the Serum and Tumor Tissue of Brain Tumor Patients. Cancer Res (1996) 56:2185–90.
110. Stockhammer G, Obwegeser A, Kostron H, Schumacher P, Muigg A, Felber S, et al. Vascular Endothelial Growth Factor (VEGF) is Elevated in Brain Tumor Cysts and Correlates With Tumor Progression. Acta Neuropathol (2000) 100:101–5. doi: 10.1007/s004010051199
111. Crocker M, Ashley S, Giddings I, Petrik V, Hardcastle A, Aherne W, et al. Serum Angiogenic Profile of Patients With Glioblastoma Identifies Distinct Tumor Subtypes and Shows That TIMP-1 is a Prognostic Factor. Neuro Oncol (2011) 13:99–108. doi: 10.1093/neuonc/noq170
112. Peles E, Lidar Z, Simon AJ, Grossman R, Nass D, Ram Z. Angiogenic Factors in the Cerebrospinal Fluid of Patients With Astrocytic Brain Tumors. Neurosurgery (2004) 55:562–7. doi: 10.1227/01.NEU.0000134383.27713.9A
113. Nazari PMS, Marosi C, Moik F, Riedl J, Özer Ö, Berghoff AS, et al. Low Systemic Levels of Chemokine C-C Motif Ligand 3 (CCL3) are Associated With a High Risk of Venous Thromboembolism in Patients With Glioma. Cancers (Basel) (2019) 11:2020. doi: 10.3390/cancers11122020
114. Ilhan A, Gartner W, Neziri D, Czech T, Base W, Hörl WH, et al. Angiogenic Factors in Plasma of Brain Tumour Patients. Anticancer Res (2009) 29:731–6. Pubmed.
115. Tabouret E, Boudouresque F, Barrie M, Matta M, Boucard C, Loundou A, et al. Association of Matrix Metalloproteinase 2 Plasma Level With Response and Survival in Patients Treated With Bevacizumab for Recurrent High-Grade Glioma. Neuro Oncol (2014) 16:392–9. doi: 10.1093/neuonc/not226
116. Levitan N, Dowlati A, Remick SC, Tahsildar HI, Sivinski LD, Beyth R, et al. Rates of Initial and Recurrent Thromboembolic Disease Among Patients With Malignancy Versus Those Without Malignancy: Risk Analysis Using Medicare Claims Data. Medicine (Baltimore) (1999) 78:285–91. doi: 10.1097/00005792-199909000-00001
117. Rość D, Grabarczyk E, Bierwagen M, Wierciński M, Góralczyk K, Haor B, et al. A Preliminary Estimation of Tissue Factor Pathway Inhibitor (TFPI) and Protein C in Patients With Intracranial Tumors. Adv Clin Exp Med (2017) 26:1219–24. doi: 10.17219/acem/67760
118. Marx S, Splittstöhser M, Kinnen F, Moritz E, Joseph C, Paul S, et al. Platelet Activation Parameters and Platelet-Leucocyte-Conjugate Formation in Glioblastoma Multiforme Patients. Oncotarget (2018) 9:25860–76. doi: 10.18632/oncotarget.25395
119. Kwaan HC, Mazar AP, McMahon BJ. The Apparent uPA/PAI-1 Paradox in Cancer: More Than Meets the Eye. Semin Thromb Hemost (2013) 39:382–91. doi: 10.1055/s-0033-1338127
120. Li Z, Lu H, Yang J, Zeng X, Zhao L, Li H, et al. Analysis of the Raw Serum Peptidomic Pattern in Glioma Patients. Clin Chim Acta (2013) 425:221–6. doi: 10.1016/j.cca.2013.08.002
121. Villanueva J, Philip J, Entenberg D, Chaparro CA, Tanwar MK, Holland EC, et al. Serum Peptide Profiling by Magnetic Particle-Assisted, Automated Sample Processing and MALDI-TOF Mass Spectrometry. Anal Chem (2004) 76:1560–70. doi: 10.1021/ac0352171
122. Liu J, Zheng S, Yu Jk, Zhang Jm, Chen Z. Serum Protein Fingerprinting Coupled With Artificial Neural Network Distinguishes Glioma From Healthy Population or Brain Benign Tumor. J Zhejiang Univ Sci B (2005) 6:4–10. doi: 10.1631/jzus.2005.b0004
123. Zhang H, Wu G, Tu H, Huang F. Discovery of Serum Biomarkers in Astrocytoma by SELDI-TOF MS and Proteinchip Technology. J Neurooncol (2007) 84:315–23. doi: 10.1007/s11060-007-9376-5
124. Vaitkiene P, Urbanaviciute R, Grigas P, Steponaitis G, Tamasauskas A, Skiriutė D. Identification of Astrocytoma Blood Serum Protein Profile. Cells (2019) 9:16. doi: 10.3390/cells9010016
125. Zhenjiang L, Rao M, Luo X, Valentini D, von Landenberg A, Meng Q, et al. Cytokine Networks and Survivin Peptide-Specific Cellular Immune Responses Predict Improved Survival in Patients With Glioblastoma Multiforme. EBioMedicine (2018) 33:49–56. doi: 10.1016/j.ebiom.2018.06.014
126. Kim VN, Han J, Siomi MC. Biogenesis of Small RNAs in Animals. Nat Rev Mol Cell Biol (2009) 10:126–39. doi: 10.1038/nrm2632
127. Weber JA, Baxter DH, Zhang S, Huang DY, Huang KH, Lee MJ, et al. The microRNA Spectrum in 12 Body Fluids. Clin Chem (2010) 56:1733–41. doi: 10.1373/clinchem.2010.147405
128. Wang J, Che F, Zhang J, Zhang M, Xiao S, Liu Y, et al. Diagnostic and Prognostic Potential of Serum Cell-Free microRNA-214 in Glioma. World Neurosurg (2019) 125:e1217–25. doi: 10.1016/j.wneu.2019.02.009
129. Vickers KC, Palmisano BT, Shoucri BM, Shamburek RD, Remaley AT. MicroRNAs are Transported in Plasma and Delivered to Recipient Cells by High-Density Lipoproteins. Nat Cell Biol (2011) 13:423–35. doi: 10.1038/ncb2210
130. Fedorko M, Stanik M, Iliev R, Redova-Lojova M, Machackova T, Svoboda M, et al. Combination of MiR-378 and MiR-210 Serum Levels Enables Sensitive Detection of Renal Cell Carcinoma. Int J Mol Sci (2015) 16:23382–9. doi: 10.3390/ijms161023382
131. Kanemaru H, Fukushima S, Yamashita J, Honda N, Oyama R, Kakimoto A, et al. The Circulating microRNA-221 Level in Patients With Malignant Melanoma as a New Tumor Marker. J Dermatol Sci (2011) 61:187–93. doi: 10.1016/j.jdermsci.2010.12.010
132. Chen G, Wang J, Cui Q. Could Circulating miRNAs Contribute to Cancer Therapy? Trends Mol Med (2013) 19:71–3. doi: 10.1016/j.molmed.2012.10.006
133. Pritchard CC, Kroh E, Wood B, Arroyo JD, Dougherty KJ, Miyaji MM, et al. Blood Cell Origin of Circulating microRNAs: A Cautionary Note for Cancer Biomarker Studies. Cancer Prev Res (2012) 5:492–7. doi: 10.1158/1940-6207.CAPR-11-0370
134. Keller A, Leidinger P, Bauer A, Elsharawy A, Haas J, Backes C, et al. Toward the Blood-Borne miRNome of Human Diseases. Nat Methods (2011) 8:841–3. doi: 10.1038/nmeth.1682
135. Siegal T, Charbit H, Paldor I, Zelikovitch B, Canello T, Benis A, et al. Dynamics of Circulating Hypoxia-Mediated miRNAs and Tumor Response in Patients With High-Grade Glioma Treated With Bevacizumab. J Neurosurg (2016) 125:1008–15. doi: 10.3171/2015.8.JNS15437
136. Wang Q, Li P, Li A, Jiang W, Wang H, Wang J, et al. Plasma Specific miRNAs as Predictive Biomarkers for Diagnosis and Prognosis of Glioma. J Exp Clin Cancer Res (2012) 31:97. doi: 10.1186/1756-9966-31-97
137. Morokoff A, Jones J, Nguyen H, Ma C, Lasocki A, Gaillard F, et al. Serum microRNA is a Biomarker for Post-Operative Monitoring in Glioma. J Neurooncol (2020) 149:391–400. doi: 10.1007/s11060-020-03566-w
138. Ivo D’Urso P, Fernando D’Urso O, Damiano Gianfreda C, Mezzolla V, Storelli C, Marsigliante S. miR-15b and miR-21 as Circulating Biomarkers for Diagnosis of Glioma. Curr Genomics (2015) 16:304–11. doi: 10.2174/1389202916666150707155610
139. Santangelo A, Imbrucè P, Gardenghi B, Belli L, Agushi R, Tamanini A, et al. A microRNA Signature From Serum Exosomes of Patients With Glioma as Complementary Diagnostic Biomarker. J Neurooncol (2018) 136:51–62. doi: 10.1007/s11060-017-2639-x
140. ParvizHamidi M, Haddad G, Ostadrahimi S, Ostadrahimi N, Sadeghi S, Fayaz S, et al. Circulating miR-26a and miR-21 as Biomarkers for Glioblastoma Multiform. Biotechnol Appl Biochem (2019) 66:261–5. doi: 10.1002/bab.1707
141. Labib EM, Ezz LR, Arab E, Ghanem HM, Hassan RE, Swellam M. Relevance of Circulating MiRNA-21 and MiRNA-181 in Prediction of Glioblastoma Multiforme Prognosis Relevance of Circulating MiRNA-21 and MiRNA-181 in Prediction of Glioblastoma Multiforme Prognosis. Arch Physiol Biochem (2020) 21:1–6. doi: 10.1080/13813455.2020.1739716
142. Ilhan-Mutlu A, Wagner L, Wöhrer A, Furtner J, Widhalm G, Marosi C, et al. Plasma Microrna-21 Concentration may be a Useful Biomarker in Glioblastoma Patients. Cancer Invest (2012) 30:615–21. doi: 10.3109/07357907.2012.708071
143. Yang K, Wang S, Cheng Y, Tian Y, Hou J. Role of miRNA-21 in the Diagnosis and Prediction of Treatment Efficacy of Primary Central Nervous System Lymphoma. Oncol Lett (2019) 17:3475–81. doi: 10.3892/ol.2019.9941
144. Lu Z, Tang H, Wu D, Xia Y, Wu M, Yi X, et al. Amplified Voltammetric Detection of miRNA From Serum Samples of Glioma Patients Via Combination of Conducting Magnetic Microbeads and Ferrocene-Capped Gold Nanoparticle/Streptavidin Conjugates. Biosens Bioelectron (2016) 86:502–7. doi: 10.1016/j.bios.2016.07.010
145. Wang J, Yi X, Tang H, Han H, Wu M, Zhou F. Direct Quantification of MicroRNA At Low Picomolar Level in Sera of Glioma Patients Using a Competitive Hybridization Followed by Amplified Voltammetric Detection. Anal Chem (2012) 84:6400–6. doi: 10.1021/ac203368h
146. Wang J, Lu Z, Tang H, Wu L, Wang Z, Wu M, et al. Multiplexed Electrochemical Detection of MiRNAs From Sera of Glioma Patients At Different Stages Via the Novel Conjugates of Conducting Magnetic Microbeads and Diblock Oligonucleotide-Modified Gold Nanoparticles. Anal Chem (2017) 89:10834–40. doi: 10.1021/acs.analchem.7b02342
147. Li J, Yuan H, Xu H, Zhao H, Xiong N. Hypoxic Cancer-Secreted Exosomal Mir-182-5p Promotes Glioblastoma Angiogenesis by Targeting Kruppel-Like Factor 2 and 4. Mol Cancer Res (2020) 18:1218–31. doi: 10.1158/1541-7786.MCR-19-0725
148. Xiao Y, Zhang L, Song Z, Guo C, Zhu J, Li Z, et al. Potential Diagnostic and Prognostic Value of Plasma Circulating Microrna-182 in Human Glioma. Med Sci Monit (2016) 22:855–62. doi: 10.12659/MSM.897164
149. Zhang R, Pang B, Xin T, Guo H, Xing Y, Xu S, et al. Plasma miR-221/222 Family as Novel Descriptive and Prognostic Biomarkers for Glioma. Mol Neurobiol (2016) 53:1452–60. doi: 10.1007/s12035-014-9079-9
150. Swellam M, Ezz El Arab L, Al-Posttany AS, B. Said S. Clinical Impact of Circulating Oncogenic MiRNA-221 and MiRNA-222 in Glioblastoma Multiform. J Neurooncol (2019) 144:545–51. doi: 10.1007/s11060-019-03256-2
151. Zhi F, Shao N, Wang R, Deng D, Xue L, Wang Q, et al. Identification of 9 Serum microRNAs as Potential Noninvasive Biomarkers of Human Astrocytoma. Neuro Oncol (2015) 17:383–91. doi: 10.1093/neuonc/nou169
152. Shi R, Wang PY, Li XY, Chen JX, Li Y, Zhang XZ, et al. Exosomal Levels of miRNA-21 From Cerebrospinal Fluids Associated With Poor Prognosis and Tumor Recurrence of Glioma Patients. Oncotarget (2015) 6:26971–81. doi: 10.18632/oncotarget.4699
153. Zhao H, Shen J, Hodges TR, Song R, Fuller GN, Heimberger AB. Serum microRNA Profiling in Patients With Glioblastoma: A Survival Analysis. Mol Cancer (2017) 16:59. doi: 10.1186/s12943-017-0628-5
154. Ilhan-Mutlu A, Wagner L, Wöhrer A, Jungwirth S, Marosi C, Fischer P, et al. Blood Alterations Preceding Clinical Manifestation of Glioblastoma. Cancer Invest (2012) 30:625–9. doi: 10.3109/07357907.2012.725443
155. Ebrahimkhani S, Vafaee F, Hallal S, Wei H, Lee MYT, Young PE, et al. Deep Sequencing of Circulating Exosomal microRNA Allows non-Invasive Glioblastoma Diagnosis. NPJ Precis Oncol (2018) 2:1–9. doi: 10.1038/s41698-018-0071-0
156. Manterola L, Guruceaga E, Pérez-Larraya JG, González-Huarriz M, Jauregui P, Tejada S, et al. A Small Noncoding RNA Signature Found in Exosomes of GBM Patient Serum as a Diagnostic Tool. Neuro Oncol (2014) 16:520–7. doi: 10.1093/neuonc/not218
157. Ohno M, Matsuzaki J, Kawauchi J, Aoki Y, Miura J, Takizawa S, et al. Assessment of the Diagnostic Utility of Serum Microrna Classification in Patients With Diffuse Glioma. JAMA Netw Open (2019) 2:e1916953. doi: 10.1001/jamanetworkopen.2019.16953
158. Roth P, Wischhusen J, Happold C, Chandran PA, Hofer S, Eisele G, et al. A Specific miRNA Signature in the Peripheral Blood of Glioblastoma Patients. J Neurochem (2011) 118:449–57. doi: 10.1111/j.1471-4159.2011.07307.x
159. Regazzo G, Terrenato I, Spagnuolo M, Carosi M, Cognetti G, Cicchillitti L, et al. A Restricted Signature of Serum miRNAs Distinguishes Glioblastoma From Lower Grade Gliomas. J Exp Clin Cancer Res (2016) 35:124. doi: 10.1186/s13046-016-0393-0
160. Qian Z, Li Y, Fan X, Zhang C, Wang Y, Jiang T, et al. Prognostic Value of a microRNA Signature as a Novel Biomarker in Patients With Lower-Grade Gliomas. J Neurooncol (2018) 137:127–37. doi: 10.1007/s11060-017-2704-5
161. Oto J, Plana E, Solmoirago MJ, Fernández-Pardo Á, Hervás D, Cana F, et al. MicroRNAs and Markers of Neutrophil Activation as Predictors of Early Incidental Post-Surgical Pulmonary Embolism in Patients With Intracranial Tumors. Cancers (Basel) (2020) 12:1–19. doi: 10.3390/cancers12061536
162. Umetani N, Hiramatsu S, Hoon DSB. Higher Amount of Free Circulating DNA in Serum Than in Plasma is Not Mainly Caused by Contaminated Extraneous DNA During Separation. Ann N Y Acad Sci (2006) 1075:299–307. doi: 10.1196/annals.1368.040
163. Faria G, Silva E, Da Fonseca C, Quirico-Santos T. Circulating Cell-Free DNA as a Prognostic and Molecular Marker for Patients With Brain Tumors Under Perillyl Alcohol-Based Therapy. Int J Mol Sci (2018) 19:1610. doi: 10.3390/ijms19061610
164. Bagley SJ, Ali Nabavizadeh S, Mays JJ, Till JE, Ware JB, Levy S, et al. Clinical Utility of Plasma Cell-Free DNA in Adult Patients With Newly Diagnosed Glioblastoma: A Pilot Prospective Study. Clin Cancer Res (2020) 26:397–407. doi: 10.1158/1078-0432.CCR-19-2533
165. Nørøxe DS, Østrup O, Yde CW, Ahlborn LB, Nielsen FC, Michaelsen SR, et al. Cell-Free DNA in Newly Diagnosed Patients With Glioblastoma – A Clinical Prospective Feasibility Study. Oncotarget (2019) 10:4397–406. doi: 10.18632/oncotarget.27030
166. Nabavizadeh SA, Ware JB, Guiry S, Nasrallah MP, Mays JJ, Till JE, et al. Imaging and Histopathologic Correlates of Plasma Cell-Free DNA Concentration and Circulating Tumor DNA in Adult Patients With Newly Diagnosed Glioblastoma. Neurooncol Adv (2020) 2:1–9. doi: 10.1093/noajnl/vdaa016
167. Piccioni DE, Achrol AS, Kiedrowski LA, Banks KC, Boucher N, Barkhoudarian G, et al. Analysis of Cell-Free Circulating Tumor DNA in 419 Patients With Glioblastoma and Other Primary Brain Tumors. CNS Oncol (2019) 8:CNS34. doi: 10.2217/cns-2018-0015
168. Ahmed K, Govardhan H, Roy M, Naveen T, Siddanna P, Sridhar P, et al. Cell-Free Circulating Tumor DNA in Patients With High-Grade Glioma as Diagnostic Biomarker - A Guide to Future Directive. Indian J Cancer (2019) 56:65–9. doi: 10.4103/ijc.IJC_551_17
169. Mouliere F, Chandrananda D, Piskorz AM, Moore EK, Morris J, Ahlborn LB, et al. Enhanced Detection of Circulating Tumor DNA by Fragment Size Analysis. Sci Transl Med (2018) 10:4921. doi: 10.1126/scitranslmed.aat4921
170. Bettegowda C, Sausen M, Leary RJ, Kinde I, Wang Y, Agrawal N, et al. Detection of Circulating Tumor DNA in Early- and Late-Stage Human Malignancies. Sci Transl Med (2014) 6:24. doi: 10.1126/scitranslmed.3007094
171. Yoshida T, Yamashita S, Takamura-Enya T, Niwa T, Ando T, Enomoto S, et al. Alu and Satα Hypomethylation in Helicobacter Pylori-Infected Gastric Mucosae. Int J Cancer (2011) 128:33–9. doi: 10.1002/ijc.25534
172. Chen J, Gong M, Lu S, Liu F, Xia L, Nie D, et al. Detection of Serum Alu Element Hypomethylation for the Diagnosis and Prognosis of Glioma. J Mol Neurosci (2013) 50:368–75. doi: 10.1007/s12031-013-0014-8
173. Chen J, Huan W, Zuo H, Zhao L, Huang C, Liu X, et al. Alu Methylation Serves as a Biomarker for Non-Invasive Diagnosis of Glioma. Oncotarget (2016) 7:26099–106. doi: 10.18632/oncotarget.8318
174. Nassiri F, Chakravarthy A, Feng S, Shen SY, Nejad R, Zuccato JA, et al. Detection and Discrimination of Intracranial Tumors Using Plasma Cell-Free DNA Methylomes. Nat Med (2020) 26:1044–7. doi: 10.1038/s41591-020-0932-2
175. Lavon I, Refael M, Zelikovitch B, Shalom E, Siegal T. Serum DNA can Define Tumor-Specific Genetic and Epigenetic Markers in Gliomas of Various Grades. Neuro Oncol (2010) 12:173–80. doi: 10.1093/neuonc/nop041
176. Wang Z, Jiang W, Wang Y, Guo Y, Cong Z, Du F, et al. MGMT Promoter Methylation in Serum and Cerebrospinal Fluid as a Tumor-Specific Biomarker of Glioma. BioMed Rep (2015) 3:543–8. doi: 10.3892/br.2015.462
177. Majchrzak-Celińska A, Paluszczak J, Kleszcz R, Magiera M, Barciszewska AM, Nowak S, et al. Detection of MGMT, RASSF1A, p15INK4B, and p14ARF Promoter Methylation in Circulating Tumor-Derived DNA of Central Nervous System Cancer Patients. J Appl Genet (2013) 54:335–44. doi: 10.1007/s13353-013-0149-x
178. Weaver KD, Grossman SA, Herman JG. Methylated Tumor-Specific DNA as a Plasma Biomarker in Patients With Glioma. Cancer Invest (2006) 24:35–40. doi: 10.1080/07357900500449546
179. Estival A, Sanz C, Ramirez JL, Velarde JM, Domenech M, Carrato C, et al. Pyrosequencing Versus Methylation-Specific PCR for Assessment of MGMT Methylation in Tumor and Blood Samples of Glioblastoma Patients. Sci Rep (2019) 9:11125. doi: 10.1038/s41598-019-47642-2
180. Balañá C, Carrato C, Ramírez JL, Cardona AF, Berdiel M, Sánchez JJ, et al. Tumour and Serum MGMT Promoter Methylation and Protein Expression in Glioblastoma Patients. Clin Transl Oncol (2011) 13:677–85. doi: 10.1007/s12094-011-0714-x
181. Balaña C, Ramirez JL, Taron M, Roussos Y, Ariza A, Ballester R, et al. O6-methyl-guanine-DNA Methyltransferase Methylation in Serum and Tumor DNA Predicts Response to 1,3-Bis(2-Chloroethyl)-1-nitrosourea But Not to Temozolamide Plus Cisplatin in Glioblastoma Multiforme. Clin Cancer Res (2003) 9:1461–8. Pubmed.
182. Fiano V, Trevisan M, Trevisan E, Senetta R, Castiglione A, Sacerdote C, et al. MGMT Promoter Methylation in Plasma of Glioma Patients Receiving Temozolomide. J Neurooncol (2014) 117:347–57. doi: 10.1007/s11060-014-1395-4
183. Schuster H, Jellinger K, Gund A, Reǵele H. Extracranial Metastases of Anaplastic Cerebral Gliomas. Acta Neurochir (Wien) (1976) 35:247–59. doi: 10.1007/BF01406121
184. Campbell AN, Chan HSL, Becker LE, Daneman A, Park TS, Hoffman HJ. Extracranial Metastases in Childhood Primary Intracranial Tumors. A Report of 21 Cases and Review of the Literature. Cancer (1984) 53:974–81. doi: 10.1002/1097-0142(19840215)53:4<974::AID-CNCR2820530426>3.0.CO;2-C
185. Müller C, Holtschmidt J, Auer M, Heitzer E, Lamszus K, Schulte A, et al. Cancer: Hematogenous Dissemination of Glioblastoma Multiforme. Sci Transl Med (2014) 6:247. doi: 10.1126/scitranslmed.3009095
186. Sullivan JP, Nahed BV, Madden MW, Oliveira SM, Springer S, Bhere D, et al. Brain Tumor Cells in Circulation are Enriched for Mesenchymal Gene Expression. Cancer Discov (2014) 4:1299–309. doi: 10.1158/2159-8290.CD-14-0471
187. Bang-Christensen SR, Pedersen RS, Pereira MA, Clausen TM, Løppke C, Sand NT, et al. Capture and Detection of Circulating Glioma Cells Using the Recombinant VAR2CSA Malaria Protein. Cells (2019) 8:998. doi: 10.3390/cells8090998
188. Lynch D, Powter B, Po JW, Cooper A, Garrett C, Koh E-SS, et al. Isolation of Circulating Tumor Cells From Glioblastoma Patients by Direct Immunomagnetic Targeting. Appl Sci (2020) 10:3338. doi: 10.3390/app10093338
189. Krol I, Castro-Giner F, Maurer M, Gkountela S, Szczerba BM, Scherrer R, et al. Detection of Circulating Tumour Cell Clusters in Human Glioblastoma. Br J Cancer (2018) 119:487–91. doi: 10.1038/s41416-018-0186-7
190. Zhang W, Bao L, Yang S, Qian Z, Dong M, Yin L, et al. Tumor-Selective Replication Herpes Simplex Virus-Based Technology Significantly Improves Clinical Detection and Prognostication of Viable Circulating Tumor Cells. Oncotarget (2016) 7:39768–83. doi: 10.18632/oncotarget.9465
191. MacArthur KM, Kao GD, Chandrasekaran S, Alonso-Basanta M, Chapman C, Lustig RA, et al. Detection of Brain Tumor Cells in the Peripheral Blood by a Telomerase Promoter-Based Assay. Cancer Res (2014) 74:2152–9. doi: 10.1158/0008-5472.CAN-13-0813
192. Gao F, Cui Y, Jiang H, Sui D, Wang Y, Jiang Z, et al. Circulating Tumor Cell is a Common Property of Brain Glioma and Promotes the Monitoring System. Oncotarget (2016) 7:71330–40. doi: 10.18632/oncotarget.11114
193. Schlesinger M. Role of Platelets and Platelet Receptors in Cancer Metastasis 06 Biological Sciences 0601 Biochemistry and Cell Biology. J Hematol Oncol (2018) 11:1–15. doi: 10.1186/s13045-018-0669-2
194. Subeikshanan V, Dutt A, Basu D, Tejus MN, Maurya VP, Madhugiri VS. A Prospective Comparative Clinical Study of Peripheral Blood Counts and Indices in Patients With Primary Brain Tumors. J Postgrad Med (2016) 62:86–90. doi: 10.4103/0022-3859.180551
195. Xu W, Wang D, Zheng X, Ou Q, Huang L, Ou Q. Sex-Dependent Association of Preoperative Hematologic Markers With Glioma Grade and Progression. J Neurooncol (2018) 137:279–87. doi: 10.1007/s11060-017-2714-3
196. Liang R, Chen N, Li M, Wang X, Mao Q, Liu Y. Significance of Systemic Immune-Inflammation Index in the Differential Diagnosis of High- and Low-Grade Gliomas. Clin Neurol Neurosurg (2018) 164:50–2. doi: 10.1016/j.clineuro.2017.11.011
197. Kemerdere R, Akgun MY, Toklu S, Alizada O, Tanriverdi T. Preoperative Systemic Inflammatory Markers in Low- and High-Grade Gliomas: A Retrospective Analysis of 171 Patients. Heliyon (2019) 5:e01681. doi: 10.1016/j.heliyon.2019.e01681
198. Lopes M, Carvalho B, Vaz R, Linhares P. Influence of Neutrophil–Lymphocyte Ratio in Prognosis of Glioblastoma Multiforme. J Neurooncol (2018) 136:173–80. doi: 10.1007/s11060-017-2641-3
199. Brockmann MA, Giese A, Mueller K, Kaba FJ, Lohr F, Weiss C, et al. Preoperative Thrombocytosis Predicts Poor Survival in Patients With Glioblastoma. Neuro Oncol (2007) 9:335–42. doi: 10.1215/15228517-2007-013
200. Auezova R, Ryskeldiev N, Doskaliyev A, Kuanyshev Y, Zhetpisbaev B, Aldiyarova N, et al. Association of Preoperative Levels of Selected Blood Inflammatory Markers With Prognosis in Gliomas. Onco Targets Ther (2016) 9:6111–7. doi: 10.2147/OTT.S113606
201. Wang Z, Zhong L, Li G, Huang R, Wang Q, Wang Z, et al. Pre-Treatment Neutrophils Count as a Prognostic Marker to Predict Chemotherapeutic Response and Survival Outcomes in Glioma: A Single-Center Analysis of 288 Cases. Am J Transl Res (2020) 12:90–104.
202. Williams M, Zi, Liu W, Woolf D, Hargreaves S, Michalarea V, Menashy R, et al. Change in Platelet Levels During Radiotherapy With Concurrent and Adjuvant Temozolomide for the Treatment of Glioblastoma: A Novel Prognostic Factor for Survival. J Cancer Res Clin Oncol (2012) 138:1683–8. doi: 10.1007/s00432-012-1243-x
203. McNamara MG, Lwin Z, Jiang H, Templeton AJ, Zadeh G, Bernstein M, et al. Factors Impacting Survival Following Second Surgery in Patients With Glioblastoma in the Temozolomide Treatment Era, Incorporating Neutrophil/Lymphocyte Ratio and Time to First Progression. J Neurooncol (2014) 117:147–52. doi: 10.1007/s11060-014-1366-9
204. Han S, Liu Y, Li Q, Li Z, Hou H, Wu A. Pre-Treatment Neutrophil-to-Lymphocyte Ratio is Associated With Neutrophil and T-Cell Infiltration and Predicts Clinical Outcome in Patients With Glioblastoma. BMC Cancer (2015) 15:617. doi: 10.1186/s12885-015-1629-7
205. Yersal Ö, Odabaşi E, Özdemir Ö, Kemal Y. Prognostic Significance of Pre−Treatment Neutrophil−to−Lymphocyte Ratio and Platelet−to−Lymphocyte Ratio in Patients With Glioblastoma. Mol Clin Oncol (2018) 9:453. doi: 10.3892/mco.2018.1695
206. Kayhan A, Korkmaz TS, Baran O, Kemerdere R, Yeni SN, Tanriverdi T. Preoperative Systemic Inflammatory Markers in Different Brain Pathologies: An Analysis of 140 Patients. Turk Neurosurg (2019) 29:799–803. doi: 10.5137/1019-5149.JTN.24244-18.2
207. Baran O, Kemerdere R, Korkmaz TS, Kayhan A, Tanriverdi T. Can Preoperative Neutrophil to Lymphocyte, Lymphocyte to Monocyte, or Platelet to Lymphocyte Ratios Differentiate Glioblastoma From Brain Metastasis? Medicine (United States) (2019) 98:e18306. doi: 10.1097/MD.0000000000018306
208. Campanella R, Guarnaccia L, Cordiglieri C, Trombetta E, Caroli M, Carrabba G, et al. Tumor-Educated Platelets and Angiogenesis in Glioblastoma: Another Brick in the Wall for Novel Prognostic and Targetable Biomarkers, Changing the Vision From a Localized Tumor to a Systemic Pathology. Cells (2020) 9:294. doi: 10.3390/cells9020294
209. Best MG, Sol N, Kooi I, Tannous J, Westerman BA, Rustenburg F, et al. RNA-Seq of Tumor-Educated Platelets Enables Blood-Based Pan-Cancer, Multiclass, and Molecular Pathway Cancer Diagnostics. Cancer Cell (2015) 28:666–76. doi: 10.1016/j.ccell.2015.09.018
210. Liefaard MC, Lips E, Best M, Sol N, Veld SI‘T, Rookus M, et al. RNA Signatures From Tumor-Educated Platelets (TEP) Enable Detection of Early-Stage Breast Cancer. Ann Oncol (2019) 30. doi: 10.1093/annonc/mdz095.036
211. Sheng M, Dong Z, Xie Y. Identification of Tumor-Educated Platelet Biomarkers of Non-Small-Cell Lung Cancer. Onco Targets Ther (2018) 11:8143–51. doi: 10.2147/OTT.S177384
212. Xue L, Xie L, Song X, Song X. Expression and Significance of ACIN1 mRNA in Platelets of Lung Cancer. Chin J Lung Cancer (2018) 21:677–81. doi: 10.3779/j.issn.1009-3419.2018.09.05
213. Xing S, Zeng T, Xue N, He Y, Lai YZ, Li HL, et al. Development and Validation of Tumor-Educated Blood Platelets Integrin Alpha 2b (ITGA2B) RNA for Diagnosis and Prognosis of non-Small-Cell Lung Cancer Through RNA-Seq. Int J Biol Sci (2019) 15:1977–92. doi: 10.7150/ijbs.36284
214. Asghar S, Waqar W, Umar M, Manzoor S. Tumor Educated Platelets, a Promising Source for Early Detection of Hepatocellular Carcinoma: Liquid Biopsy an Alternative Approach to Tissue Biopsy. Clin Res Hepatol Gastroenterol (2020) 44:836–44. doi: 10.1016/j.clinre.2020.03.023
215. Campbell RA, Franks Z, Bhatnagar A, Rowley JW, Manne BK, Supiano MA, et al. Granzyme A in Human Platelets Regulates the Synthesis of Proinflammatory Cytokines by Monocytes in Aging. J Immunol (2018) 200:295–304. doi: 10.4049/jimmunol.1700885
216. Simon LM, Edelstein LC, Nagalla S, Woodley AB, Chen ES, Kong X, et al. Human Platelet microRNA-mRNA Networks Associated With Age and Gender Revealed by Integrated Plateletomics. Blood (2014) 123:e37–45. doi: 10.1182/blood-2013-12-544692
217. Best MG, Vancura A, Wurdinger T. Platelet RNA as a Circulating Biomarker Trove for Cancer Diagnostics. J Thromb Haemost (2017) 15:1295–306. doi: 10.1111/jth.13720
218. Sol N, In ‘t Veld GJG, Vancura A, Tjerkstra M, Leurs C, Rustenburg FO, et al. Tumor-Educated Platelet RNA for the Detection and (Pseudo)Progression Monitoring of Glioblastoma. Cell Rep Med (2020) 1:100101. doi: 10.1016/j.xcrm.2020.100101
219. Best MG, In ‘t Veld SGJG, Sol N, Wurdinger T. RNA Sequencing and Swarm Intelligence–Enhanced Classification Algorithm Development for Blood-Based Disease Diagnostics Using Spliced Blood Platelet RNA. Nat Protoc (2019) 14:1206–34. doi: 10.1038/s41596-019-0139-5
220. Fossati G, Ricevuti G, Edwards SW, Walker C, Dalton A, Rossi ML. Neutrophil Infiltration Into Human Gliomas. Acta Neuropathol (1999) 98:349–54. doi: 10.1007/s004010051093
221. Serra-Bonett N, Al Snih S, Rodriguez MA. Effect of Low-Dose Prednisone on Leukocyte Counts and Subpopulations in Patients With Rheumatoid Arthritis. J Clin Rheumatol (2009) 15:148–9. doi: 10.1097/RHU.0b013e3181a3ac2d
222. Shoenfeld Y, Gurewich Y, Gallant LA, Pinkhas J. Prednisone-Induced Leukocytosis. Influence of Dosage, Method and Duration of Administration on the Degree of Leukocytosis. Am J Med (1981) 71:773–8. doi: 10.1016/0002-9343(81)90363-6
223. Olnes MJ, Kotliarov Y, Biancotto A, Cheung F, Chen J, Shi R, et al. Effects of Systemically Administered Hydrocortisone on the Human Immunome. Sci Rep (2016) 6:1–15. doi: 10.1038/srep23002
224. Frenkel A, Kachko E, Novack V, Klein M, Brotfain E, Koyfman L, et al. The Association of Glucocorticosteroid Treatment With WBC Count in Patients With COPD Exacerbation. J Clin Med (2019) 8:1697. doi: 10.3390/jcm8101697
225. Wang ZL, Zhang CB, Liu YQ, Wang Z, Jiang T. Peripheral Blood Test Provides a Practical Method for Glioma Evaluation and Prognosis Prediction. CNS Neurosci Ther (2019) 25:876–83. doi: 10.1111/cns.13120
226. Weng W, Chen X, Gong S, Guo L, Zhang X. Preoperative Neutrophil–Lymphocyte Ratio Correlated With Glioma Grading and Glioblastoma Survival. Neurol Res (2018) 40:917–22. doi: 10.1080/01616412.2018.1497271
227. Schernberg A, Nivet A, Dhermain F, Ammari S, Escande A, Pallud J, et al. Neutrophilia as a Biomarker for Overall Survival in Newly Diagnosed High-Grade Glioma Patients Undergoing Chemoradiation. Clin Transl Radiat Oncol (2018) 10:47–52. doi: 10.1016/j.ctro.2018.04.002
228. Adachi-Hayama M, Adachi A, Shinozaki N, Matsutani T, Hiwasa T, Takiguchi M, et al. Circulating Anti-Filamin C Autoantibody as a Potential Serum Biomarker for Low-Grade Gliomas. BMC Cancer (2014) 14:452. doi: 10.1186/1471-2407-14-452
229. Gustafson MP, Lin Y, New KC, Bulur PA, O’Neill BP, Gastineau DA, et al. Systemic Immune Suppression in Glioblastoma: The Interplay Between CD14 +HLA-DR Lo/Neg Monocytes, Tumor Factors, and Dexamethasone. Neuro Oncol (2010) 12:631–44. doi: 10.1093/neuonc/noq001
230. Bambury RM, Teo MY, Power DG, Yusuf A, Murray S, Battley JE, et al. The Association of Pre-Treatment Neutrophil to Lymphocyte Ratio With Overall Survival in Patients With Glioblastoma Multiforme. J Neurooncol (2013) 114:149–54. doi: 10.1007/s11060-013-1164-9
231. Bertaut A, Truntzer C, Madkouri R, Kaderbhai CG, Derangère V, Vincent J, et al. Blood Baseline Neutrophil Count Predicts Bevacizumab Efficacy in Glioblastoma. Oncotarget (2016) 7:70948–58. doi: 10.18632/oncotarget.10898
232. Boonyawan K, Hess KR, Yang J, Long L, Wang Q, Ezhilarasan R, et al. A Relative Increase in Circulating Platelets Following Chemoradiation Predicts for Poor Survival of Patients With Glioblastoma. Oncotarget (2017) 8:90488–95. doi: 10.18632/oncotarget.21799
233. Gousias K, Voulgaris S, Vartholomatos G, Voulgari P, Kyritsis AP, Markou M. Prognostic Value of the Preoperative Immunological Profile in Patients With Glioblastoma. Surg Neurol Int (2014) 5:89. doi: 10.4103/2152-7806.134104
234. Gan Y, Zhou X, Niu X, Li J, Wang T, Zhang H, et al. Neutrophil/Lymphocyte Ratio is an Independent Prognostic Factor in Elderly Patients With High-Grade Gliomas. World Neurosurg (2019) 127:e261–7. doi: 10.1016/j.wneu.2019.03.085
235. Vaios EJ, Winter SF, Muzikansky A, Nahed BV, Dietrich J. Eosinophil and Lymphocyte Counts Predict Bevacizumab Response and Survival in Recurrent Glioblastoma. Neurooncol Adv (2020) 2:1–11. doi: 10.1093/noajnl/vdaa031
236. Capper D, von Deimling A, Brandes AA, Carpentier AF, Kesari S, Sepulveda-Sanchez JM, et al. Biomarker and Histopathology Evaluation of Patients With Recurrent Glioblastoma Treated With Galunisertib, Lomustine, or the Combination of Galunisertib and Lomustine. Int J Mol Sci (2017) 18:995. doi: 10.3390/ijms18050995
237. Wiencke JK, Accomando WP, Zheng S, Patoka J, Dou X, Phillips JJ, et al. Epigenetic Biomarkers of T-Cells in Human Glioma. Epigenetics (2012) 7:1391–402. doi: 10.4161/epi.22675
238. Bahador M, Gras Navarro A, Rahman MA, Dominguez-Valentin M, Sarowar S, Ulvestad E, et al. Increased Infiltration and Tolerised Antigen-Specific CD8+ TEM Cells in Tumor But Not Peripheral Blood Have No Impact on Survival of HCMV+ Glioblastoma Patients. Oncoimmunology (2017) 6:e1336272. doi: 10.1080/2162402X.2017.1336272
239. Bhondeley MK, Mehra RD, Mehra NK, Mohapatra AK, Tandon PN, Roy VB S. Imbalances in T Cell Subpopulations in Human Gliomas in. J Neurosurg (1988) 68:589–93. doi: 10.3171/jns.1988.68.4.0589
240. Fecci PE, Mitchell DA, Whitesides JF, Xie W, Friedman AH, Archer GE, et al. Increased Regulatory T-Cell Fraction Amidst a Diminished CD4 Compartment Explains Cellular Immune Defects in Patients With Malignant Glioma. Cancer Res (2006) 66:3294–302. doi: 10.1158/0008-5472.CAN-05-3773
241. Vasco C, Canazza A, Rizzo A, Mossa A, Corsini E, Silvani A, et al. Circulating T Regulatory Cells Migration and Phenotype in Glioblastoma Patients: An In Vitro Study. J Neurooncol (2013) 115:353–63. doi: 10.1007/s11060-013-1236-x
242. Mohme M, Schliffke S, Maire CL, Runger A, Glau L, Mende KC, et al. Immunophenotyping of Newly Diagnosed and Recurrent Glioblastoma Defines Distinct Immune Exhaustion Profiles in Peripheral and Tumor-Infiltrating Lymphocytes. Clin Cancer Res (2018) 24:4187–200. doi: 10.1158/1078-0432.CCR-17-2617
243. Gousias K, von Ruecker A, Voulgari P, Simon M. Phenotypical Analysis, Relation to Malignancy and Prognostic Relevance of ICOS + T Regulatory and Dendritic Cells in Patients With Gliomas. J Neuroimmunol (2013) 264:84–90. doi: 10.1016/j.jneuroim.2013.09.001
244. Ogden AT, Horgan D, Waziri A, Anderson D, Louca J, McKhann GM, et al. Defective Receptor Expression and Dendritic Cell Differentiation of Monocytes in Glioblastomas. Neurosurgery (2006) 59:902–10. doi: 10.1227/01.NEU.0000233907.03070.7B
245. Li X, Wang B, Gu L, Zhang J, Li X, Gao L, et al. Tim-3 Expression Predicts the Abnormal Innate Immune Status and Poor Prognosis of Glioma Patients. Clin Chim Acta (2018) 476:178–84. doi: 10.1016/j.cca.2017.11.022
246. Wilson JRF, Saeed F, Tyagi AK, Goodden JR, Sivakumar G, Crimmins D, et al. Pre-Operative Neutrophil Count and Neutrophil-Lymphocyte Count Ratio (NLCR) in Predicting the Histological Grade of Paediatric Brain Tumours: A Preliminary Study. Acta Neurochir (Wien) (2018) 160:793–800. doi: 10.1007/s00701-017-3388-5
247. Evans SM, Putt M, Yang X-Y, Lustig RA, Martinez-Lage M, Williams D, et al. Initial Evidence That Blood-Borne Microvesicles are Biomarkers for Recurrence and Survival in Newly Diagnosed Glioblastoma Patients. J Neurooncol (2016) 127:391–400. doi: 10.1007/s11060-015-2051-3
248. Pinton L, Masetto E, Vettore M, Solito S, Magri S, D’Andolfi M, et al. The Immune Suppressive Microenvironment of Human Gliomas Depends on the Accumulation of Bone Marrow-Derived Macrophages in the Center of the Lesion. J Immunother Cancer (2019) 7:58. doi: 10.1186/s40425-019-0536-x
249. Guo Y, Hong W, Zhang P, Han D, Fang Y, Tu J, et al. Abnormal Polarization of Macrophage-Like Cells in the Peripheral Blood of Patients With Glioma. Oncol Lett (2020) 20:947–54. doi: 10.3892/ol.2020.11602
250. Vidyarthi A, Agnihotri T, Khan N, Singh S, Tewari MK, Radotra BD, et al. Predominance of M2 Macrophages in Gliomas Leads to the Suppression of Local and Systemic Immunity. Cancer Immunol Immunother (2019) 68:1995–2004. doi: 10.1007/s00262-019-02423-8
251. Sippel TR, White J, Nag K, Tsvankin V, Klaassen M, Kleinschmidt-DeMasters BK, et al. Neutrophil Degranulation and Immunosuppression in Patients With GBM: Restoration of Cellular Immune Function by Targeting Arginase I. Clin Cancer Res (2011) 17:6992–7002. doi: 10.1158/1078-0432.CCR-11-1107
252. Templeton AJ, McNamara MG, Šeruga B, Vera-Badillo FE, Aneja P, Ocaña A, et al. Prognostic Role of Neutrophil-to-Lymphocyte Ratio in Solid Tumors: A Systematic Review and Meta-Analysis. J Natl Cancer Inst (2014) 106:dju124. doi: 10.1093/jnci/dju124
253. Chen J, Deng Q, Pan Y, He B, Ying H, Sun H, et al. Prognostic Value of Neutrophil-to-Lymphocyte Ratio in Breast Cancer. FEBS Open Bio (2015) 5:502–7. doi: 10.1016/j.fob.2015.05.003
254. Shimada H, Takiguchi N, Kainuma O, Soda H, Ikeda A, Cho A, et al. High Preoperative Neutrophil-Lymphocyte Ratio Predicts Poor Survival in Patients With Gastric Cancer. Gastric Cancer (2010) 13:170–6. doi: 10.1007/s10120-010-0554-3
255. Ferrucci PF, Ascierto PA, Pigozzo J, Vecchio MD, Maio M, Cappellini GCA, et al. Baseline Neutrophils and Derived Neutrophil-to-Lymphocyte Ratio: Prognostic Relevance in Metastatic Melanoma Patients Receiving Ipilimumab. Ann Oncol (2016) 27:732–8. doi: 10.1093/annonc/mdw016
256. Tweardy DJ, Cannizzaro LA, Palumbo AP, Shane S, Huebner K, Vantuinen P, et al. Molecular Cloning and Characterization of a cDNA for Human Granulocyte Colony-Stimulating Factor (G-CSF) From a Glioblastoma Multiforme Cell Line and Localization of the G-CSF Gene to Chromosome Band 17q21. Oncogene Res (1987) 1:209–20.
257. Teramukai S, Kitano T, Kishida Y, Kawahara M, Kubota K, Komuta K, et al. Pretreatment Neutrophil Count as an Independent Prognostic Factor in Advanced Non-Small-Cell Lung Cancer: An Analysis of Japan Multinational Trial Organisation LC00-03. Eur J Cancer (2009) 45:1950–8. doi: 10.1016/j.ejca.2009.01.023
258. Lechner MG, Liebertz DJ, Epstein AL. Characterization of Cytokine-Induced Myeloid-Derived Suppressor Cells From Normal Human Peripheral Blood Mononuclear Cells. J Immunol (2010) 185:2273–84. doi: 10.4049/jimmunol.1000901
259. Balkwill F, Mantovani A. Inflammation and Cancer: Back to Virchow? Lancet (2001) 357:539–45. doi: 10.1016/S0140-6736(00)04046-0
260. Fridlender ZG, Sun J, Kim S, Kapoor V, Cheng G, Ling L, et al. Polarization of Tumor-Associated Neutrophil Phenotype by TGF-β: “N1” Versus “N2” Tan. Cancer Cell (2009) 16:183–94. doi: 10.1016/j.ccr.2009.06.017
261. Uribe-Querol E, Rosales C. Neutrophils in Cancer: Two Sides of the Same Coin. J Immunol Res (2015) 2015:983698. doi: 10.1155/2015/983698
262. Wiencke JK, Koestler DC, Salas LA, Wiemels JL, Roy RP, Hansen HM, et al. Immunomethylomic Approach to Explore the Blood Neutrophil Lymphocyte Ratio (NLR) in Glioma Survival. Clin Epigenet (2017) 9:10. doi: 10.1186/s13148-017-0316-8
263. Wang J, Xiao W, Chen W, Hu Y. Prognostic Significance of Preoperative Neutrophil-to-Lymphocyte Ratio and Platelet-to-Lymphocyte Ratio in Patients With Glioma. EXCLI J (2019) 17:505–12. doi: 10.17179/excli2017-978
264. Tan Z, Shen L, Wu H, Deng L, Li Z, Huang X. Preoperative Neutrophil/Lymphocyte Ratio Is an Independent Prognostic Biomarker in Patients With Low-Grade Gliomas. World Neurosurg (2019) 132:e585–90. doi: 10.1016/j.wneu.2019.08.068
265. Huang Y, Ding H, Wu Q, Li Z, Li H, Li S, et al. Neutrophil–Lymphocyte Ratio Dynamics are Useful for Distinguishing Between Recurrence and Pseudoprogression in High-Grade Gliomas. Cancer Manag Res (2019) 11:6003–9. doi: 10.2147/CMAR.S202546
266. Bao Y, Yang M, Jin C, Hou S, Shi B, Shi J, et al. Preoperative Hematologic Inflammatory Markers as Prognostic Factors in Patients With Glioma. World Neurosurg (2018) 119:e710–6. doi: 10.1016/j.wneu.2018.07.252
267. Lv Y, Zhang S, Liu Z, Tian Y, Liang N, Zhang J. Prognostic Value of Preoperative Neutrophil to Lymphocyte Ratio is Superior to Systemic Immune Inflammation Index for Survival in Patients With Glioblastoma. Clin Neurol Neurosurg (2019) 181:24–7. doi: 10.1016/j.clineuro.2019.03.017
268. Alexiou G, Vartholomatos E, Voulgaris S, Zagorianakou P. Prognostic Significance of Neutrophil-to-Lymphocyte Ratio in Glioblastoma. Neuroimmunol Neuroinflamm (2014) 1:131–4. doi: 10.4103/2347-8659.143666
269. Kaya V, Yildirim M, Yazici G, Yalçin AY, Orhan N, Güzel A. Prognostic Significance of Indicators of Systemic Inflammatory Responses in Glioblastoma Patients. Asian Pac J Cancer Prev (2017) 18:3287–91. doi: 10.22034/APJCP.2017.18.12.3287
270. Brenner A, Friger M, Geffen DB, Kaisman-Elbaz T, Lavrenkov K. The Prognostic Value of the Pretreatment Neutrophil/Lymphocyte Ratio in Patients With Glioblastoma Multiforme Brain Tumors: A Retrospective Cohort Study of Patients Treated With Combined Modality Surgery, Radiation Therapy, and Temozolomide Chemotherapy. Oncology (2019) 97:255–63. doi: 10.1159/000500926
271. Weng Y, Zhang X, Han J, Ouyang L, Liang M, Shi Z, et al. Do Selected Blood Inflammatory Markers Combined With Radiological Features Predict Proliferation Index in Glioma Patients? World Neurosurg (2018) 118:e137–46. doi: 10.1016/j.wneu.2018.06.142
272. Zadora P, Dabrowski W, Czarko K, Smolen A, Kotlinska-Hasiec E, Wiorkowski K, et al. Preoperative Neutrophil-Lymphocyte Count Ratio Helps Predict the Grade of Glial Tumor – A Pilot Study. Neurol Neurochir Pol (2015) 49:41–4. doi: 10.1016/j.pjnns.2014.12.006
273. Hong X, Cui B, Wang M, Yang Z, Wang L, Xu Q. Systemic Immune-Inflammation Index, Based on Platelet Counts and Neutrophil-Lymphocyte Ratio, is Useful for Predicting Prognosis in Small Cell Lung Cancer. Tohoku J Exp Med (2015) 236:297–304. doi: 10.1620/tjem.236.297
274. Hu B, Yang XR, Xu Y, Sun YF, Sun C, Guo W, et al. Systemic Immune-Inflammation Index Predicts Prognosis of Patients After Curative Resection for Hepatocellular Carcinoma. Clin Cancer Res (2014) 20:6212–22. doi: 10.1158/1078-0432.CCR-14-0442
275. Chen Z, Shao Y, Wang K, Cao W, Xiong Y, Wu R, et al. Prognostic Role of Pretreatment Serum Albumin in Renal Cell Carcinoma: A Systematic Review and Meta-Analysi. Onco Targets Ther (2016) 9:6701–10. doi: 10.2147/OTT.S108469
276. Pinzon-Charry A, Ho CSK, Laherty R, Maxwell T, Walker D, Gardiner RA, et al. A Population of HLA-DR+ Immature Cells Accumulates in the Blood Dendritic Cell Compartment of Patients With Different Types of Cancer. Neoplasia (2005) 7:1112–22. doi: 10.1593/neo.05442
278. Raychaudhuri B, Ireland PRJ, Ko J, Rini B, Borden EC, Garcia J, et al. Myeloid-Derived Suppressor Cell Accumulation and Function in Patients With Newly Diagnosed Glioblastoma. Neuro Oncol (2011) 13:591–9. doi: 10.1093/neuonc/nor042
279. Gabrusiewicz K, Rodriguez B, Wei J, Hashimoto Y, Healy LM, Maiti SN, et al. Glioblastoma-Infiltrated Innate Immune Cells Resemble M0 Macrophage Phenotype. JCI Insight (2016) 1:e85841. doi: 10.1172/jci.insight.85841
280. Gielen PR, Schulte BM, Kers-Rebel ED, Verrijp K, Petersen-Baltussen HMJM, Ter Laan M, et al. Increase in Both CD14-Positive and CD15-Positive Myeloid-Derived Suppressor Cell Subpopulations in the Blood of Patients With Glioma But Predominance of CD15-Positive Myeloid-Derived Suppressor Cells in Glioma Tissue. J Neuropathol Exp Neurol (2015) 74:390–400. doi: 10.1097/NEN.0000000000000183
281. Dubinski D, Wölfer J, Hasselblatt M, Schneider-Hohendorf T, Bogdahn U, Stummer W, et al. CD4+ T Effector Memory Cell Dysfunction is Associated With the Accumulation of Granulocytic Myeloid-Derived Suppressor Cells in Glioblastoma Patients. Neuro Oncol (2016) 18:807–18. doi: 10.1093/neuonc/nov280
282. Sakaguchi S. Naturally Arising CD4+ Regulatory T Cells for Immunologic Self-Tolerance and Negative Control of Immune Responses. Annu Rev Immunol (2004) 22:531–62. doi: 10.1146/annurev.immunol.21.120601.141122
283. Facciabene A, Motz GT, Coukos G. T-Regulatory Cells: Key Players in Tumor Immune Escape and Angiogenesis. Cancer Res (2012) 72:2162–71. doi: 10.1158/0008-5472.CAN-11-3687
284. Andaloussi A, Lesniak MS. An Increase in CD4+CD25+FOXP3+ Regulatory T Cells in Tumor-Infiltrating Lymphocytes of Human Glioblastoma Multiforme. Neuro Oncol (2006) 8:234–43. doi: 10.1215/15228517-2006-006
285. Li Z, Liu X, Guo R, Wang P. CD4+Foxp3– Type 1 Regulatory T Cells in Glioblastoma Multiforme Suppress T Cell Responses Through Multiple Pathways and are Regulated by Tumor-Associated Macrophages. Int J Biochem Cell Biol (2016) 81:1–9. doi: 10.1016/j.biocel.2016.09.013
286. Jacobs JFM, Idema AJ, Bol KF, Nierkens S, Grauer OM, Wesseling P, et al. Regulatory T Cells and the PD-L1/PD-1 Pathway Mediate Immune Suppression in Malignant Human Brain Tumors. Neuro Oncol (2009) 11:394–402. doi: 10.1215/15228517-2008-104
287. Willms E, Cabañas C, Mäger I, Wood MJA, Vader P. Extracellular Vesicle Heterogeneity: Subpopulations, Isolation Techniques, and Diverse Functions in Cancer Progression. Front Immunol (2018) 9:1. doi: 10.3389/fimmu.2018.00738
288. Stahl PD, Raposo G. Extracellular Vesicles: Exosomes and Microvesicles, Integrators of Homeostasis. Physiology (Bethesda) (2019) 34:169–77. doi: 10.1152/physiol.00045.2018
289. Osti D, Bene MD, Rappa G, Santos M, Matafora V, Richichi C, et al. Clinical Significance of Extracellular Vesicles in Plasma From Glioblastoma Patients. Clin Cancer Res (2019) 25:266–76. doi: 10.1158/1078-0432.CCR-18-1941
290. Ricklefs FL, Maire CL, Reimer R, Dührsen L, Kolbe K, Holz M, et al. Imaging Flow Cytometry Facilitates Multiparametric Characterization of Extracellular Vesicles in Malignant Brain Tumours. J Extracell Vesicles (2019) 8:1588555. doi: 10.1080/20013078.2019.1588555
291. Reynés G, Vila V, Fleitas T, Reganon E, Font de Mora J, Jordá M, et al. Circulating Endothelial Cells and Procoagulant Microparticles in Patients With Glioblastoma: Prognostic Value. PloS One (2013) 8:e69034. doi: 10.1371/journal.pone.0069034
292. Cumba Garcia LM, Peterson TE, Cepeda MA, Johnson AJ, Parney IF. Isolation and Analysis of Plasma-Derived Exosomes in Patients With Glioma. Front Oncol (2019) 10:3338. doi: 10.3389/fonc.2019.00651
293. Koch CJ, Lustig RA, Yang XY, Jenkins WT, Wolf RL, Martinez-Lage M, et al. Microvesicles as a Biomarker for Tumor Progression Versus Treatment Effect in Radiation/Temozolomide-Treated Glioblastoma Patients. Transl Oncol (2014) 7:752–8. doi: 10.1016/j.tranon.2014.10.004
294. Muller L, Muller-Haegele S, Mitsuhashi M, Gooding W, Okada H, Whiteside TL. Exosomes Isolated From Plasma of Glioma Patients Enrolled in a Vaccination Trial Reflect Antitumor Immune Activity and Might Predict Survival. Oncoimmunology (2015) 4:e1008347. doi: 10.1080/2162402X.2015.1008347
295. Lei YY, Li YT, Hu QL, Wang J, Sui AX. Prognostic Impact of Neutrophil-to-Lymphocyte Ratio in Gliomas: A Systematic Review and Meta-Analysis. World J Surg Oncol (2019) 17:1–8. doi: 10.1186/s12957-019-1686-5
296. Hallal S, Azimi A, Wei H, Ho N, Lee MYT, Sim H-W, et al. A Comprehensive Proteomic SWATH-MS Workflow for Profiling Blood Extracellular Vesicles: A New Avenue for Glioma Tumour Surveillance. Int J Mol Sci (2020) 21:4754. doi: 10.3390/ijms21134754
297. Ding C, Yi X, Wu X, Bu X, Wang D, Wu Z, et al. Exosome-Mediated Transfer of circRNA CircNFIX Enhances Temozolomide Resistance in Glioma. Cancer Lett (2020) 479:1–12. doi: 10.1016/j.canlet.2020.03.002
298. Shao H, Chung J, Balaj L, Charest A, Bigner DD, Carter BS, et al. Protein Typing of Circulating Microvesicles Allows Real-Time Monitoring of Glioblastoma Therapy. Nat Med (2012) 18:1835–40. doi: 10.1038/nm.2994
299. Wang H, Jiang D, Li W, Xiang X, Zhao J, Yu B, et al. Evaluation of Serum Extracellular Vesicles as Noninvasive Diagnostic Markers of Glioma. Theranostics (2019) 9:5347–58. doi: 10.7150/thno.33114
300. Johnson CH, Patterson AD, Idle JR, Gonzalez FJ. Xenobiotic Metabolomics: Major Impact on the Metabolome. Annu Rev Pharmacol Toxicol (2012) 52:37–56. doi: 10.1146/annurev-pharmtox-010611-134748
301. Baranovičová E, Galanda T, Galanda M, Jozef H, Kolarovszki B, Richterová R, et al. Metabolomic Profiling of Blood Plasma in Patients With Primary Brain Tumours: Basal Plasma Metabolites Correlated With Tumour Grade and Plasma Biomarker Analysis Predicts Feasibility of the Successful Statistical Discrimination From Healthy Subjects - a P. IUBMB Life (2019) 71:1994–2002. doi: 10.1002/iub.2149
302. Zhao H, Heimberger AB, Lu Z, Wu X, Hodges TR, Song R, et al. Metabolomics Profiling in Plasma Samples From Glioma Patients Correlataes With Tumor Phenotypes. Oncotarget (2016) 7:20486–95. doi: 10.18632/oncotarget.7974
303. Mörén L, Tommy Bergenheim A, Ghasimi S, Brännström T, Johansson M, Antti H. Metabolomic Screening of Tumor Tissue and Serum in Glioma Patients Reveals Diagnostic and Prognostic Information. Metabolites (2015) 5:502–20. doi: 10.3390/metabo5030502
304. Shen J, Song R, Hodges TR, Heimberger AB, Zhao H. Identification of Metabolites in Plasma for Predicting Survival in Glioblastoma. Mol Carcinog (2018) 57:1078–84. doi: 10.1002/mc.22815
305. Björkblom B, Wibom C, Jonsson P, Mörén L, Andersson U, Johannesen TB, et al. Metabolomic Screening of Pre-Diagnostic Serum Samples Identifies Association Between α- and γ-Tocopherols and Glioblastoma Risk. Oncotarget (2016) 7:37043–53. doi: 10.18632/oncotarget.9242
306. Huang J, Weinstein SJ, Kitahara CM, Karoly ED, Sampson JN, Albanes D. A Prospective Study of Serum Metabolites and Glioma Risk. Oncotarget (2017) 8:70366–77. doi: 10.18632/oncotarget.19705
307. Kelimu A, Xie R, Zhang K, Zhuang Z, Mamtimin B, Sheyhidin I. Metabonomic Signature Analysis in Plasma Samples of Glioma Patients Based on 1H-Nuclear Magnetic Resonance Spectroscopy. Neurol India (2016) 64:246–51. doi: 10.4103/0028-3886.177606
308. Zhao S, Cai J, Li J, Bao G, Li D, Li Y, et al. Bioinformatic Profiling Identifies a Glucose-Related Risk Signature for the Malignancy of Glioma and the Survival of Patients. Mol Neurobiol (2017) 54:8203–10. doi: 10.1007/s12035-016-0314-4
309. McGirt MJ, Chaichana KL, Gathinji M, Attenello F, Than K, Ruiz AJ, et al. Persistent Outpatient Hyperglycemia is Independently Associated With Decreased Survival After Primary Resection of Malignant Brain Astrocytomas. Neurosurgery (2008) 63:286–91. doi: 10.1227/01.NEU.0000315282.61035.48
310. Mayer A, Vaupel P, Struss HG, Giese A, Stockinger M, Schmidberger H. Strong Adverse Prognostic Impact of Hyperglycemic Episodes During Adjuvant Chemoradiotherapy of Glioblastoma Multiforme. Strahlenther Onkol (2014) 190:933–8. doi: 10.1007/s00066-014-0696-z
311. Derr RL, Ye X, Islas MU, Desideri S, Saudek CD, Grossman SA. Association Between Hyperglycemia and Survival in Patients With Newly Diagnosed Glioblastoma. J Clin Oncol (2009) 27:1082–6. doi: 10.1200/JCO.2008.19.1098
312. Shih CC, Lee TS, Tsuang FY, Lin PL, Cheng YJ, Cheng HL, et al. Pretreatment Serum Lactate Level as a Prognostic Biomarker in Patients Undergoing Supratentorial Primary Brain Tumor Resection. Oncotarget (2017) 8:63715–23. doi: 10.18632/oncotarget.18891
313. Branco M, Linhares P, Carvalho B, Santos P, Costa BM, Vaz R. Serum Lactate Levels are Associated With Glioma Malignancy Grade. Clin Neurol Neurosurg (2019) 186:105546. doi: 10.1016/j.clineuro.2019.105546
314. Whiting PF, Rutjes AWS, Westwood ME, Mallett S, Deeks JJ, Reitsma JB, et al. Quadas-2: A Revised Tool for the Quality Assessment of Diagnostic Accuracy Studies. Ann Intern Med (2011) 155:529–36. doi: 10.7326/0003-4819-155-8-201110180-00009
315. McShane LM, Altman DG, Sauerbrei W, Taube SE, Gion M, Clark GM. Reporting Recommendations for Tumour MARKer Prognostic Studies (REMARK). Br J Cancer (2005) 93:387–91. doi: 10.1038/sj.bjc.6602678
316. Bustin SA, Benes V, Garson JA, Hellemans J, Huggett J, Kubista M, et al. The MIQE Guidelines: Minimum Information for Publication of Quantitative Real-Time PCR Experiments. Clin Chem (2009) 55:611–22. doi: 10.1373/clinchem.2008.112797
317. Iafolla MAJ, Picardo S, Aung K, Hansen AR. Systematic Review and REMARK Scoring of Renal Cell Carcinoma Prognostic Circulating Biomarker Manuscripts. PloS One (2019) 14:e0222359. doi: 10.1371/journal.pone.0222359
318. Raza IJ, Tingate CA, Gkolia P, Romero L, Tee JW, Hunn MK. Blood Biomarkers of Glioma in Response Assessment Including Pseudoprogression and Other Treatment Effects: A Systematic Review. Front Oncol (2020) 10:1191. doi: 10.3389/fonc.2020.01191
319. Bustin S, Nolan T. Talking the Talk, But Not Walking the Walk: RT-qPCR as a Paradigm for the Lack of Reproducibility in Molecular Research. Eur J Clin Invest (2017) 47:756–74. doi: 10.1111/eci.12801
320. Capper D, Jones DTW, Sill M, Hovestadt V, Schrimpf D, Sturm D, et al. DNA Methylation-Based Classification of Central Nervous System Tumours. Nature (2018) 555:469–74. doi: 10.1038/nature26000
321. Hanahan D, Weinberg RA. Hallmarks of Cancer: The Next Generation. Cell (2011) 144:646–74. doi: 10.1016/j.cell.2011.02.013
Keywords: diagnostics, liquid biopsy, blood, glioblastoma, glioma
Citation: Ali H, Harting R, de Vries R, Ali M, Wurdinger T and Best MG (2021) Blood-Based Biomarkers for Glioma in the Context of Gliomagenesis: A Systematic Review. Front. Oncol. 11:665235. doi: 10.3389/fonc.2021.665235
Received: 07 February 2021; Accepted: 18 May 2021;
Published: 04 June 2021.
Edited by:
Jose R. Pineda, University of the Basque Country, SpainReviewed by:
Lisa Shaw, University of Central Lancashire, United KingdomCopyright © 2021 Ali, Harting, de Vries, Ali, Wurdinger and Best. This is an open-access article distributed under the terms of the Creative Commons Attribution License (CC BY). The use, distribution or reproduction in other forums is permitted, provided the original author(s) and the copyright owner(s) are credited and that the original publication in this journal is cited, in accordance with accepted academic practice. No use, distribution or reproduction is permitted which does not comply with these terms.
*Correspondence: Myron G. Best, bS5nLmJlc3RAYW1zdGVyZGFtdW1jLm5s
Disclaimer: All claims expressed in this article are solely those of the authors and do not necessarily represent those of their affiliated organizations, or those of the publisher, the editors and the reviewers. Any product that may be evaluated in this article or claim that may be made by its manufacturer is not guaranteed or endorsed by the publisher.
Research integrity at Frontiers
Learn more about the work of our research integrity team to safeguard the quality of each article we publish.