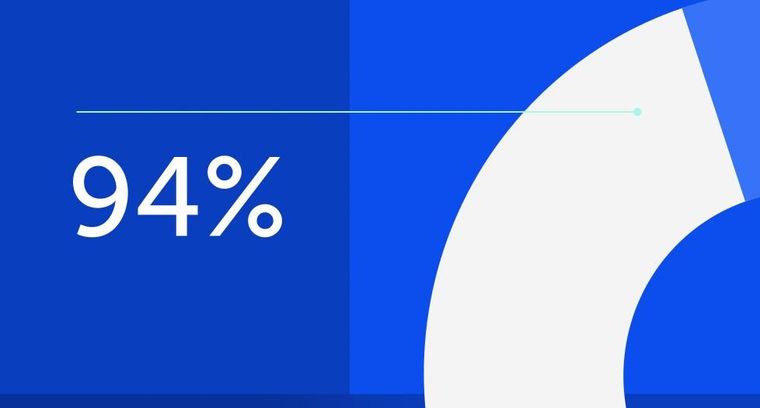
94% of researchers rate our articles as excellent or good
Learn more about the work of our research integrity team to safeguard the quality of each article we publish.
Find out more
ORIGINAL RESEARCH article
Front. Oncol., 29 November 2021
Sec. Neuro-Oncology and Neurosurgical Oncology
Volume 11 - 2021 | https://doi.org/10.3389/fonc.2021.657029
A correction has been applied to this article in:
Corrigendum: RAB42 promotes glioma pathogenesis via the VEGF signaling pathway
Angiogenesis plays an important role in tumor initiation and progression of glioma. Seeking for biomarkers associated with angiogenesis is important in enhancing our understanding of glioma biologically and identifying its new drug targets. RNA-sequencing (RNA-seq) data and matched clinical data were downloaded from the CGGA database. A series of filtering analyses were performed to screen for reliable genes: survival, multivariate Cox, ROC curve filtration, and clinical correlation analyses. After immunohistochemical verification, RAB42 was identified as a reliable gene for further single gene analysis. Afterwards, we performed gene set enrichment analysis (GSEA) and co-expression analysis to establish the related molecular mechanisms and signal pathways in glioma. Finally, the gene functions and the mechanisms were investigated in vitro experiments. A total of 23270 mRNA expression and 1018 glioma samples were included in this study. After the three filtering analyses, we selected ten genes for immunohistochemical verification: KLHDC8A, IKIP, HIST1H2BK, HIST1H2BJ, GNG5, FAM114A1, TMEM71, RAB42, CCDC18, and GAS2L3. Immunostaining demonstrated that RAB42 was significantly expressed on the membrane of glioma tissues but not in normal tissues. These results were verified and validated in GEPIA datasets, and the association between RAB42 with clinical features was also evaluated. Analysis of gene functions indicated that RAB42 activated VEGF signaling pathways and the mechanism was associated with natural killer cell mediated cytotoxicity, JAK-STAT signaling pathway and apoptosis pathways by PI3K/AKT in gliomas. Experiments in vitro suggested that the proliferation and invasion of glioma cells might be inhibited after downregulating of RAB42. And the tumorigenesis promotion of RAB42 may relate to the activation of VEGF signaling pathway. Taken together, this study shows that the overexpression of RAB42 is an independent prognostic factor of adverse prognosis. Its pro-oncogenic mechanism may be associated with the activation of VEGF signaling pathways.
Approximately 100,000 new cases of diffuse glioma are diagnosed globally every year (1, 2). According to morphological criteria of 2007 WHO guidelines for classifying gliomas, diffuse glioma is graded Stage II-IV. Glioblastoma (WHO IV, GBM) accounts for 70-75% of all diffuse gliomas. Despite the multiple treatment options including surgery, radiotherapy, and chemotherapy, the median overall survival of GBM is only 14-17 months (3).
Tumor angiogenesis is an important pathological process in the development of malignant tumors and refers to the formation of new blood vessels from pre-existing blood vessel networks (4). Glioblastoma is defined as lethal cancer by angiogenesis (5), which involves various mechanisms (6). Overlapping and intricate signaling pathways participate in the regulation of angiogenesis (7). As early as 25 years ago, the important role of vascular endothelial growth factor (VEGF) in vasculogenesis and angiogenesis had been identified, isolated, and cloned (8). Although VEGF has been demonstrated to be important in physiological vascular homeostasis, it contributes to the pathogenesis of malignant tumors by supporting growth and metastasis (9). The VEGF is overexpressed in the majority of human cancers and is correlated with vascular density, tumor invasiveness, metastasis, and prognosis (9). Several therapeutic approaches targeting the inhibition of the VEGF signaling pathway have been devised. Bevacizumab, a VEGF pathway inhibitor, has been approved for other malignancies in addition to metastatic colorectal cancer, including glioblastoma multiforme (8). However, Bevacizumab has displayed limited effectiveness in GBM as shown by relapse or progression of the tumor after 6 months of treatment (10). While numerous studies have increased the understanding of tumorigenesis and drug resistance, the mechanisms of glioma pathogenesis via the VEGF signaling pathway remain unclear.
With the development of high-throughput sequencing and bioinformatics, many tumor biomarkers of glioma, which is of great significance to the diagnosis and treatment of patients, have been identified (11). However, much more reliable biomarkers are needed in the diagnosis and decision-making processes. In this study, the filtering of the whole datasets of RNA-seq from the CGGA database allowed for the identification of 131 genes. Ten genes were screened for immunohistochemistry verification after bibliography data retrieval. The RAB42 was then screened for further identification. After a series of bioinformatics analysis and experiments in vitro, we found that RAB42 promotes glioma pathogenesis via the VEGF signaling pathway.
Both RNA-sequencing (RNA-seq) and clinical data were downloaded from the CGGA database (http://www.cgga.org.cn). The data included mRNA sequencing and corresponding clinical characteristics in glioma on 1,018 Chinese cohorts. There were two batches in all the RNA-seq data: mRNAseq 693 (batch 1) and mRNAseq 325 (batch 2). We performed batch correction and integration using the “sva” and “limma” package in R (version 4.0.2, https://www.r-project.org).
A series of filtering analyses were performed to screen for reliable genes. First, we performed Kaplan–Meier (K-M) (12) and univariate Cox analyses with data from the whole RNA-seq datasets using “survival” and “survminer” packages in R. Genes with a KM and cox P values < 0.001, and five years differences > 0.2 were considered statistically significant. The above results obtained from the survival analysis were further analyzed by multivariate Cox analysis with a “survival” package in R. And the screening criterion was p Filter < 0.001. We then screened for genes with survival differences and independent prognostic value. To predict the accuracy, we further performed receiver-operating-characteristic (ROC) curves on the expression of these genes, by the “survivalROC” package in R by defining the filter criterion as the area under the curve (AUC) > 0.7. Finally, the expression results of the three filtering and clinical information were applied to perform clinical correlation analysis. And P<0.05 was considered to be statistically significant.
After filtering analyses, we screened reliable mRNA from RNA-seq data. To further verify the expression of these genes in clinical tissue specimens, ten genes (KLHDC8A, IKIP, HIST1H2BK, HIST1H2BJ, GNG5, FAM114A1, TMEM71, RAB42, CCDC18, and GAS2L3) were selected for re-verification. The histological samples of different grades of glioma patients in Linyi People’s hospital were reviewed and selected for formalin-fixed paraffin-embedded (FFPE) tissue material for immunohistochemistry (13). Brain tissues after trauma embedded in paraffin were chosen as normal control. We randomly selected 30 glioma cases (10 cases each for grades II, III, and IV) and 10 cases of brain trauma patients as normal controls for the protein expression re-verification of the 10 genes. All the tissues were cut into 5μm thick sections for immunohistochemistry staining. All the slides were incubated at 70°C for at least 30 mins. This was followed by deparaffinization, rehydration, boiling with EDTA antigen retrieval buffer, and then incubation with 10% goat serum for 1 h to block the nonspecific binding sites. After the incubation with the primary antibodies at 37°C for 1 hour (anti-KLHDC8A, 1:200 dilution, Abcam; anti-IKIP, 1:200 dilution, Abcam; anti-HIST1H2BK,1:200 dilution, Invitrogen; HIST1H2BJ,1:200 dilution, Invitrogen; anti-GNG5, 1:200 dilution, SIGMA; anti-FAM114A1, 1:200 dilution, proteintech; anti-TMEM71, 1:200 dilution, Abcam; anti-RAB42, 1:200 dilution, USBiological; CCDC18, 1:200 dilution, SIGMA and anti-GAS2L3, 1:200 dilution, Abcam), the sections were incubated with appropriate secondary antibodies at 37°C for 30 mins (1:1000, ZSGB-Bio, Beijing, China). The slides were exposed to HRP substrate 3,3-diaminobenzidine (DAB) (Tiangen, Beijing, China) and then dehydrated by dipping sequentially through 70%, 95%, 100% ethanol, and xylene before mounting (Neutral balsam; Solarbio).
The reliable gene, RAB42, was identified for further analysis. RAB42 gene expression data and matched clinical information were used to perform survival, univariate, multivariate, and plot ROC curves analyses using “survival”, “survminer”, and “survivalROC” packages in R. The correlation between RAB42 gene expression and clinical traits was then validated. The “beeswarm” packages in R was used for graphic visualization. Further re-verification of RAB42 expression and survival analysis was performed in the Gene Expression Profiling Interactive Analysis (GEPIA; http://gepia2.cancer-pku.cn) and the Cancer Genome Atlas (TCGA) database. GEPIA is an interactive web server containing RNA sequencing data and clinical data based on 9,736 tumor and 8,587 normal samples from the TCGA and GTEx databases (14). In addition, we downloaded transcriptome expression profiles and clinical data from the TCGA database (https://portal.gdc.cancer.gov). Survival analysis was performed with the “survival” package in R(version 4.0.2, https://www.r-project.org).
Samples were divided into high- and low-risk population phenotypes based on RAB42 gene expression levels. Gene set enrichment analysis (GSEA) (https://www.gsea-msigdb.org/gsea/index.jsp) was then used to assess related molecular mechanisms and signal pathways in glioma patients (15). Gene sets with a nominal P < 0.05 and a false discovery rate (FDR) < 0.25 were considered statistically significant.
To further screen for the genes co-expressed with RAB42, we performed co-expression analysis using the “limma” package in R. Genes with p<0.001, and correlation coefficient >0.5 were considered as co-expressed genes. The gene sets of interest were previously identified through GSEA analysis. The overlapping genes between the co-expressed genes with RAB42 and genes in the designated Gene sets were chosen as function-related genes. Venn diagram was plotted using the “VennDiagram” package in R. Furthermore, the top 20 genes positively and negatively associated with RAB42 were exhibited in the heatmap using the “ pheatmap” package in R. The top 5 genes positively and negatively associated with RAB42 were used to generate a circular plot by “Corrplot” and “Circlize” packages in R.
All the glioblastoma cell lines SNB-19, KNS-89, CRT, U87, U251, A172, TG905 and SF295 were kindly provided by Central Laboratory of Linyi People’s Hospital. All the cell lines were free from mycoplasma contamination with a certificate of authentication with STR profiling. They were cultured in Dulbecco’s modified Eagle’s medium (DMEM, Gibco, Thermo Fisher Scientific, Shanghai, China), 10% FBS (Thermo Fisher Scientific), penicillin (100 U/mL), and streptomycin (100 µg/mL) at 37°C, and 5% CO2 air. Cells were digested using Trypsin‐EDTA (0.05%)(Beijing Soledad Bao Technology Co, Beijing, China) for cell passages.
The RAB42-knockdown stable cell lines (SNB19-sh-RAB42) were obtained by transfecting pSilencer 4.1-CMV-puro-RAB42 small interfering RNA (siRNA) vector into SNB-19 cells and selecting for stable clones in the presence of 0.5 mg/mL puromycin. And empty vectors were transfected as a control (SNB19-sh-NC).
Cell proliferation was tested using CCK8 cell proliferation assay kit (Beyotime Biotechnology, Shanghai, China). First, we plated cells at a density of 5,000 cells/well in 96‐well plates. Then, cells were allowed to attach 4 h, after which cells were collected at 0h, 1d, 2d, 3d, 4d and 5d. After adding 10 μl of CCK‐8 solution per well and incubating for 1 to 4h, we measured the absorbance at 450 nm using an enzyme calibrator (MolecularDevices, Sunnyvale, CA). The formula for calculating the cell viability was as follows: (mean OD treated wells)/(mean OD control wells) × 100%.
Transwell migration and transwell invasion assay were performed to analyze migration and invasion ability using transwell chambers (Corning Inc., Corning, USA). Cells were planted into the upper chamber in the serum‐free culture medium with the density of 1×105 cells/well. The lower chambers was added with 800 μl medium containing 20% FBS. After incubating for 24 h, cells were fixed with 4% PFA and stained with crystal. After wiping cells in the upper chamber with a cotton swab, the cells were observed under an inverted microscope and photographed. Cell invasion assays were performed in chambers precoated with matrigel (Corning Inc., Corning, USA) with the cells seeding density of 10×105 cells/well.
Cells were digested, resuspended and eeded onto six-well plates at the density of 200 cells per well. After 2 weeks of culturing in an incubator with 5% CO2 at 37°C, cells were fixed and stained using crystal violet. Then, colonies that contained more than 50 cells were counted and calculated.
RT-PCR was conducted to determine the gene expression of RAB42 and endogenous pluripotency genes. First, total RNA of cells was extracted by RNA extraction kit (GeneCopoeia, Rockville, MD, USA) following manufacturer’s instructions. Then, the concentration of the RNA was detected for further study. Next, cDNA was synthesized using a first-strand cDNA synthesis kit (GeneCopoeia, Rockville, MD, USA). Primers used for amplification of RAB42 gene and the reference gene, GAPDH, are described in Supplementary Table S5. Relative quantification of the gene expression level was calculated by 2−(ΔΔCt) method (16). The qRT-PCR analysis was repeated in three biological and three technical replications.
VEGFR PCR Array (WCgene, Biotechnology, Shanghai China) was utilized to the investigation of further molecular mechanism. The specific gene primers were designed by Wcgene Biotech (WCgene, Shanghai, China). RNA extraction and cDNA synthesis were performed as described. PCR reactions were performed using real-time 7500 PCR system (Applied Biosystems).And the PCR reaction procedure was as follows: 95°C For 10 min, then 40 cycles at 95°C for 15 sec and finally at 60°C for 1 min. The results were analyzed as previously described.
Western blot (WB) analysis using for detecting protein expressions was performed following standard techniques. Total protein was extracted using protein extraction kit (Solibao Technology Co. Ltd, Beijing, China). The protein concentration standard curve was plotted and then the protein concentrations were determined using BCA protein concentration assay kit (Biosharp, Hefei, China). After SDS-PAGE electrophoresis and wet transfer, the target protein was transferred to PDVF membrane (Solarbio, Beijing, China). Following blocking with 5% nonfat dry milk, the primary and secondary antibodies were incubated for 2 and 1 hour respectively at room temperature. Membranes were then washed and developed by ECL (Bio-Rad, 1705061).
All data of bioinformatics analysis were conducted by R language (version 4.0.1). Kaplan–Meier (K-M) and univariate Cox analyses was used to compare the survival differences between groups using “survival” and “survminer” packages in R. Genes with a KM and cox P values < 0.001, and five years differences > 0.2 were considered statistically significant. Spearman’s correlation was used to screen for the genes co-expressed with RAB42 by “limma” package in R. Genes with p<0.001, and correlation coefficient >0.5 were considered as co-expressed genes. All data of experiments in vitro are expressed as mean ± standard deviation (unless otherwise shown). Student’s t‐tests were used to determine the significance of differences between two groups, and one‐way ANOVA and Tukey’s post hoc test were used to determine differences among multiple groups. P<0.05 was considered to indicate a statistically significant difference.
The procedure of this study was presented in the flow chart (Figure 1A).
Figure 1 (A) Flow chart of data download, filtering, validation, and mechanism exploration; (B) Representative images of RAB42 in primary glioma patient tissues (Tumors were graded according to the WHO classification) and control normal tissues by immunohistochemical staining (magnification, 200×). (a) RAB42 expression was not visible in control normal tissues. (b) RAB42 was found clearly expressed on the membrane of glioma tissues(WHO, Grade II); (c) RAB42 was found strongly expressed on the membrane of glioma tissues(WHO, Grade III); (d) RAB42 was found strongly expressed on the membrane of glioma tissues(WHO, Grade IV).
A total of 23270 mRNA expression and 1018 glioma samples were included for subsequent analysis. After performing survival analysis, 5112 genes showing survival differences were obtained (Supplementary Table 1). By screening for the genes that could be used as independent prognostic factors using Multivariate Cox analysis, a total of 2630 genes were obtained (Supplementary Table 2). From the ROC curve filtration, using AUC>0.7 as the screening criterion, 131 reliable genes were identified to have good performance of survival prediction (Supplementary Table 3). The relationship between the 131 genes and clinical features, including Primary/Recurrent/Secondary type, LGG/GBM histology, WHO grade, Age, Grade, Radiotherapy status, Chemotherapy status, IDH mutation status, and 1p19q codeletion status, have been shown in Supplementary Table 4. Ten among these 131 genes (KLHDC8A, IKIP, HIST1H2BK, HIST1H2BJ, GNG5, FAM114A1, TMEM71, RAB42, CCDC18, and GAS2L3) were selected based on Bibliography Retrieval for subsequent immunohistochemical verification.
We performed immunohistochemistry staining for the ten selected genes on glioma and normal brain tissues. Eight of them showed no expression in tumor tissues. HIST1H2BK was strongly expressed in the nucleus of both glioma and normal brain tissues. RAB42 was markedly expressed on the membrane 429 of glioma tissues (Figures 1B–D, brown) particularly in 430 glioblastoma (Grade IV), and not on the normal brain tissues 431 (Figure 1A). Thus, we validated that RAB42 is differentially 432 expressed between tumor and normal tissues using 433 434 immunohistochemistry staining.
Figure 2 Single gene analysis of RAB42 using the CGGA database (LGG+GBM, n = 749). (A) Survival curve of glioma patients in high- and low- RAB42 groups. (B) Forest plot of univariate analysis. RAB42 (HR = 1.778; 95% CI = 1.36–1.933; P < 0.001), age, PRS type, histology, grade and chemo were independent poor prognostic factors, while IDH mutation and 1p19q codeletion were favorable prognostic factor. (C) Forest plot of multivariate analysis showing RAB42 as an independent prognostic factor (HR = 1.222; 95% CI= 1.100–1.357; P < 0.001). (D) ROC analysis of RAB42. AUC, the area under the curve. The area under the ROC curve (AUC) of 1-year, 3-year, and 5-year overall survival was 0.764, 0.760, and 0.727 respectively.
Based on our findings, we analyzed the tumor-promoting effect of RAB42 using bioinformatics. Survival analysis indicated that low RAB42 expression in CGGA glioma (including GBM and LGG) tissues predicted favorable survival outcome and vice versa (Kaplan-Meier survival analysis; P < 0.001; Figure 2A). As shown in results of the univariate Cox analysis, RAB42 (HR = 1.778; 95% CI = 1.36–1.933; P < 0.001), age, PRS type, histology, grade, and chemotherapy status were independent poor prognostic factors, while IDH mutation and 1p19q codeletion were well described prognostic factor (Figure 2B). RAB42 was still identified as an independent prognostic factor in a multivariable analysis (HR = 1.222; 95% CI= 1.100–1.357; P < 0.001), implying that it could be a powerful indicator of prognosis in glioma portending poor prognosis (Figure 2C). To depict the accuracy of RAB42 for predicting prognostic in glioma patients, we plotted ROC curves using the data downloaded from the CGGA database. The area under the ROC curve (AUC) is a measure of the accuracy of the marker prediction, where AUC = 0.50 means no discrimination, and AUC = 1.0 means perfect prediction (17). The ROC curve analysis indicated that RAB42 had high diagnostic accuracy, and the area under the ROC curve (AUC) of 1-year, 3-year, and 5-year overall survival was 0.764, 0.760, and 0.727, respectively (Figure 2D).
In GEPIA datasets, we found that the expressions of RAB42 in GBM and LGG were upregulated (Figure 3A). Survival curve indicated that the high expression of the RAB42 was associated with poor prognosis (Figure 3B). After collecting transcriptome expression profiles and clinical data, 695 samples were downloaded from the TCGA database, including 167 GBM tissues and 528 LGG samples. Multivariate Cox regression analysis showed RAB42 (HR = 2.96; 95% CI = 2.30–3.80; P < 0.001), PRS type (HR = 14.51; 95% CI = 9.17–23.00; P < 0.001) and age (HR = 1.05; 95% CI = 1.04–1.1; P < 0.001) were independent poor prognostic factors. This is consistent with CGGA results indicating that RAB42 could be a poor prognostic predictor in glioma (Figure 3C). Additionally, overall survival analysis of RAB42 expression in GBM and LGG revealed that patients with high expression of RAB42 had a significantly worse prognosis (Figure 3D). This is consistent with our previous analysis in CGGA database.
Figure 3 Relationship between RAB42 expression and prognosis of glioma patients based in the GEPIA and TCGA database. (A) RAB42 was significantly upregulated both in GBM and LGG in the GEPIA database. (B) Survival curve of RAB42 expression levels and overall survival in the GEPIA database (P < 0.001). (C) Forest plot of multivariate analysis showing RAB42 as an independent prognostic factor (HR = 2.96; 95% CI = 2.30–3.80; P < 0.001) in TCGA database. (D) Survival curve of GBM and LGG patients in high- and low- RAB42 groups in TCGA database *** means P < 0.001.
To establish the relationship between RAB42 expression and clinical traits, we analyzed their relevance using the 1,018 samples from the CGGA database. Results showed that the expression of RAB42 was closely related to 1p19q codeletion status, age, chemotherapy status, grade, IDH mutation status, PRS type, and histology (Figure 4).
Figure 4 The relationship between RAB42 expression and clinical traits using the CGGA database. Differential expression of RAB42 was significantly related to (A) 1p19q codeletion status (Codel, n = 155; Non-codel, n = 594), (B) age (≤41, n = 342; ≥41, n = 407), (C) chemotherapy status (No, n = 229; Yes, n = 520), (D) Gender (Female=322, Male=427), (E) Grade (WHO II, n = 218; WHO III, n = 240;WHO IV, n = 291), (F) IDH mutation status (Mutant, n = 410; Wildtype, n = 339), (G) PRS type (Primary, n = 502; Recurrent, n = 222; Secondary, n = 25) and (H) Histology (GBM=288;LGG=461).
The signaling pathways enrichment in high and low RAB42 expression groups were analyzed using GSEA software. MSigDB C2 collection (c2.cp.kegg.v7.1.symnols.gmt) was chosen and gene sets with significant differences (FDR < 0.05) downloaded. It turned out that 170 signaling pathways were enriched in the high-RAB42 expression group. Of these, 151 gene sets were upregulated and multiple signaling pathways seemed to be very closely related to cancer. Six pathways showing significant enrichment associated with cancer are shown in Figure 5A. RAB42 was positively correlated with apoptosis, natural killer cell mediated cytotoxicity, P53 signaling pathway, pathways in cancer, JAK-STAT signaling pathway and VEGF signaling pathway.
Figure 5 GSEA of RAB42 and identification of go-expression genes. (A) GSEA enrichment analysis of RAB42 (GBM LGG, n = 749). RAB42 positively correlates with apoptosis, natural killer cell mediated cytotoxicity, P53 signaling pathway, pathways in cancer, JAK-STAT signaling pathway and VEGF signaling pathway.(B) Correlation curves of RAB42 and PIK3CD, PIK3CG, PIK3R5, PLCG2, RAC2, and VEGFA.
Gene co-expression analysis with the full expression profile identified 1280 relevant genes that were closely correlated with RAB42. Heat maps of the top 20 negatively and positively correlated genes are shown in Supplementary Figure 1A and a circular plot of the top negatively and positively related genes shown in Supplementary Figure 1B. We performed a series of alignments with related genes of RAB42 and genes in each pathway to characterize downstream mechanisms and explored key genes. The intersection between the 1280 related genes and genes of pathways in cancer and VEGF signaling pathways highlighted six genes: PIK3CD, PIK3CG, PIK3R5, PLCG2, RAC2, and VEGFA. Further analyses on the relationship between these genes and apoptosis, natural killer cell mediated cytotoxicity and JAK-STAT signaling pathways showed that PIK3CD, PIK3CG, and PIK3R5 were common key genes (Supplementary Figure 2). These results indicated that RAB42 activated VEGF signaling pathways and was associated with apoptosis, natural killer cell mediated cytotoxicity and JAK-STAT signaling pathways by PIK3 signaling pathway in gliomas. Finally, we plotted the correlation curves of RAB42 and PIK3CD, PIK3CG, PIK3R5, PLCG2, RAC2, and VEGFA (Figure 5B). The expression of all the six genes were correlated positively with RAB42 expression (PIK3CD, P=1.103e-76; PIK3CG, P=7.534e-67; PIK3R5, P=4.563e-100; PLCG2, P=2.054e-70; RAC2, P=6.236e-174, and VEGFA, P=2.231e-113).
At the outset, we first detected the expression of RAB42 by RT-PCR in eight cell lines (SNB-19, KNS-89, CRT, U87, U251, A172, TG905 and SF295). As showcased in Figure 6A, the SNB-19 cells, which exhibiting the highest expression level of RAB42 among all the 8 cell lines, was selected as the targeted cell for further study. Efficient RAB42 knockdown had been confirmed by PCR and WB (Figures 6B, C). Results of CCK8 assays showed downregulation of RAB42 reduced cell proliferation of glioma cells (Figure 6D). As shown in transwell results (Figures 6E, F), RAB42 knockdown inhibited invasion and migration of glioma cells. Clonogenic assay of cells showed similar results in Figures 6G, H. The above results suggested that the proliferation and invasion of glioma cells might be inhibited after downregulating of RAB42, which also suggesting the tumor‐promoting role of RAB42 in glioma.
Figure 6 Validation of RAB42 gene functions in vitro. (A) Relative mRNA expression of SNB-19, KNS-89, CRT, U87, U251, A172, TG905 and SF295. (B) Relative expression of RAB42 in SNB19 cell lines after RAB42 knockdown (SNB19-sh-RAB42), parental cell line SNB19 (SNB19-Control) and control corresponding to the parental cell line transfected with the empty expression vector (SNB19-sh-NC). (C) Relative protein expression of SNB19-sh-RAB42, SNB19-Control and SNB19-sh-NC. (D) Cellular viability by CCK8 assay. (E) Quantification of invasion and migration assays. (F) Transwell invasion and migration assays. (G) Quantification of colony number in clonogenic assay. (H) Clonogenic assays. “ns” means “not significant”, *** means P < 0.001.
The stable RAB42 knockdown cells (SNB19-sh-RAB42), parental cell line SNB19 (SNB19-Control) and control corresponding to the parental cell line transfected with the empty expression vector (SNB19-sh-NC) were sent for sequencing. As shown in the Supplementary Table 6 and Supplementary Figure 3, after knocking down RAB42, the expression of VEGFD, PIK3CD, PIK3R3 and PIK3R1 changes due to the knockdown of RAB42 in SNB19 cells. Meanwhile, a PCR microarray was performed to investigate genetic alterations in VEGF signaling pathway after RAB42 knockdown in glioma. As shown in Figure 7A, 36 genes associated with VEGF signaling pathway were altered in SNB19-RAB42 cells. Among them, five genes (PLA2G3, PRKCB, PLCG2, RAC2 and SH2D2A) that showed positive correlation between RAB42, while eleven genes (PIK3CB, DUSP1, NRP1, PLCG1, PIK3CA, PLA2G12B, ATF4, VEGFA, NOS3, PLA2G4B and PXN) showed negative correlation (Figure 7B) with significantly statistical difference. The results confirmed that the tumorigenesis promotion of RAB42 may relate to the activation of VEGF signaling pathway. This is consistent with the former analyses.
Figure 7 PCR microarray (A) Heat map depicting genetic alterations in VEGF signaling pathway after RAB42 knockdown in glioma. (B) Selection of genes that were altered the most in SNB19-RAB42 cells.
Diffuse gliomas account for approximately 80% of malignant brain tumors (18, 19). Adult diffuse gliomas mainly include oligodendroglioma, oligoastrocytoma, astrocytoma, and glioblastoma according to histological features (20). In recent years, studies have shown how classical histopathology has failed to meet current clinical demand as compared with molecular reclassification (21, 22). These have resulted in a major revision of glioma classification in the 2016 WHO guideline by incorporating isocitrate dehydrogenase(IDH) status and 1p/19q codeletion diagnostics (23). Moreover, growing studies have demonstrated that a few functional molecules could be used as potential diagnostic and prognostic biomarkers in gliomas (24, 25). Therefore, the search for prognostic biomarkers that can improve the outcome in glioma is valuable for increasing our understanding and exploring therapeutic approaches to glioma.
The characterization efforts of the gene (for example, TCGA) have greatly enhanced our understanding of the biological process for glioma progression (26). The Chinese Glioma Genome Atlas (CGGA), is a web application for data storage and analysis for exploring brain tumors datasets over 2,000 samples from Chinese cohorts. More than 200 SCI papers have been published and more than 20 projects have been conducted using this database directly or indirectly. From the analysis of the gene expression data and clinical information of CGGA, Liu et al. found that HIST1H2BK could be a biomarker for LGG with poor prognosis (27). In this study, RNA-seq data of glioma and matched clinical data were downloaded from the CGGA database. After filtering by survival analysis, multivariate Cox, ROC curve, and clinical relevance, 131 genes were identified. All of the 131 genes significantly correlated with survival, and served as reliable independent predictors with high clinical relevance. Ten genes (KLHDC8A, IKIP, HIST1H2BK, HIST1H2BJ, GNG5, FAM114A1, TMEM71, RAB42, CCDC18, and GAS2L3) were then selected for immunohistochemical verification. As a result, KLHDC8A, IKIP, HIST1H2BJ, GNG5, FAM114A1, TMEM71, CCDC18, and GAS2L3 were not expressed both in glioma and normal tissues. HIST1H2BK was strongly expressed in the nucleus of both glioma and normal tissue cells. Contrary, RAB42 was significantly expressed on the membrane of glioma tissues but not in normal tissues.
RAB42 is a member of the mammalian Rab family small GTPases (28). Consistent with our findings, a previous study showed that RAB42 is one of the Seven genes used for the prognostic prediction in patients with glioma (29). This previous study mainly focused on the building of the seven genes prognostic model with minimum attention paid to RAB42. This study performed a single gene analysis of RAB42 and found that it had as a tumor-promoting role in glioma and could be a powerful indicator of prognosis in glioma portending poor prognosis. Besides, a strong association was found between the expression of RAB42 and multiple clinical features. To explore the possible mechanism of RAB42 cancer-promoting functions, we further performed GSEA and co-expression analysis. The results indicated that RAB42 activated VEGF signaling pathway and was associated with apoptosis, natural killer cell mediated cytotoxicity pathways and JAK-STAT signaling pathways by PI3K/AKT in gliomas.
Central malignant processes for glioma, neo-angiogenesis, and vascularity are important factors for glioma histopathological grading (30, 31). As an angiogenic growth factor produced by tumors, VEGF can often stimulate tumor angiogenesis (32). It has also been identified that VEGF plays a critical regulatory role in the angiogenic response of glioblastomas (33). Antiangiogenic therapy causes devascularization that inhibits tumor growth and VEGF is considered a functionally important drug target (34). Researchers have reported that PI3K/AKT pathway is involved in angiogenesis and that the suppression of the PI3K/AKT signal axis could inhibit new blood vessel formation by downregulating VEGF expression (35). Similar to previous studies, our findings indicated that RAB42 functioned as an oncogene in glioma, activated VEGF signaling pathways, and regulated PI3K/AKT signal axis. Furthermore, our study found that potential mechanisms of RAB42 were associated with apoptosis, natural killer cell mediated cytotoxicity pathways and JAK/STAT signaling pathway through the regulation of PI3K/AKT. The pleiotropic cascade mediated by JAK/STAT pathway is the principal mechanism for signal transduction of multiple cytokines and growth factors. It has been reported that the activation of the JAK/STAT pathway causes the inhibition of apoptosis and tumor initiation (36). Growing evidence suggests that angiogenesis and tumor progression are related to members of the innate immune system (37). As a part of the innate immunity, natural killer cell-mediated cytotoxicity is strongly associated with tumorigenesis and tumor development (38). In the present study, RAB42 showed cancer-promoting functions by regulating multiple tumor-related signaling pathways, which is consistent with previous findings.
We found that the pro-oncogenic mechanism of RAB42 could be through the activation of VEGF signaling pathways, which is novel and has potential clinical utility. In this study, RAB42 was selected from a large number of candidate genes as the target gene for further investigation. A large amount of bioinformatic analysis demonstrated that the tumorigenesis promotion of RAB42 may relate to the activation of VEGF signaling pathway. Subsequently, we experimentally validated that RAB42 promoted the proliferation, migration and invasion of glioma and the pro-oncogenic mechanism of RAB42 is associated with the activation of VEGF signaling pathways. However, this study had some limitations. We collected the RNA-seq data and clinical information from the CGGA dataset instead of getting our sequencing data from patients of our hospital. Besides, the sample size used for immunohistochemistry verification was not large enough and the information about clinical characters and survival data was not enough. In addition, the study mainly focused on bioinformatic analysis with limited experimental validation of the function and the specific mechanisms of action of RAB42. Therefore, more in-depth studies are needed to investigate the function and pro-oncogenic mechanism of RAB42.
In conclusion, we explored the biological function of RAB42 and mechanism in glioma. We used filtering analyses to screen for the reliable genes and performed immunohistochemical verification to identify the key gene, RAB42. Single gene analysis of RAB42 showed that RAB42 is an independent prognostic factor of adverse prognostic. RAB42 expression is associated with multiple clinical features. Furthermore, functional in vitro experiments indicated that RAB42 promoted the proliferation, migration and invasion of glioma and the pro-oncogenic mechanism of RAB42 is associated with the activation of VEGF signaling pathways.
The datasets presented in this study can be found in online repositories. The names of the repository/repositories and accession number(s) can be found in the article/Supplementary Material.
The studies involving human participants were reviewed and approved by the Human Research Ethic Committee of Linyi People’s hospital. The patients/participants provided their written informed consent to participate in this study.
BL conducted the experiments, wrote, and edited the manuscript. QS and BX provided experimental support. GZ provided glioma and traumatic brain tissue. LZ provided support during the experiment. JY reviewed and edited the manuscript. LW and FC contributed to analyze the data and draft the manuscript. XH contributed to the conception, design, and analysis of the manuscript. All authors contributed to the article and approved the submitted version.
This research is supported by the postdoctoral innovation project of Shandong Province in 2020 (No.20203068), Medical and Health Technology Development Program in Shandong Province (No. 2017WS499), Medical Health Science and Technology Development Plan of Shandong Province (No.2019WS133) and Technology Development Plan Program of Linyi (No.202020001).
The authors declare that the research was conducted in the absence of any commercial or financial relationships that could be construed as a potential conflict of interest.
All claims expressed in this article are solely those of the authors and do not necessarily represent those of their affiliated organizations, or those of the publisher, the editors and the reviewers. Any product that may be evaluated in this article, or claim that may be made by its manufacturer, is not guaranteed or endorsed by the publisher.
We thank the members of the Central Laboratory and Department of Pathology for their helpful in the experiment operations and manuscript writing.
The Supplementary Material for this article can be found online at: https://www.frontiersin.org/articles/10.3389/fonc.2021.657029/full#supplementary-material
Supplementary Table 1 | Results of survival analysis filtering.
Supplementary Table 2 | Results of independent prognostic analysis filtering.
Supplementary Table 3 | Results of ROC curve filtering.
Supplementary Table 4 | Results of clinical relevance analysis filtering.
Supplementary Table 5 | Primers used in the experiments
Supplementary Table 6 | The differential gene expression of SNB19-WT vs SNB19-RAB42
Supplementary Figure 1 | Identification of RAB 42 Co-expression Genes. (A) Heatmap of the top twenty genes positively and negatively correlated to RAB42. (B) A circular plot of the top five genes positively and negatively associated with RAB42. Red indicates a positive correlation whereas green indicates a negative correlation.
Supplementary Figure 2 | The Venn diagrams showed the common key genes of RAB42 related genes and genes in pathways in cancer, VEGF signaling pathway, apoptosis, natural killer cell mediated cytotoxicity and JAK-STAT signaling pathways.
Supplementary Figure 3 | Heatmap and Volcano Plot of the differential gene expression.
GBM, Glioblastoma; VEGF, vascular endothelial growth factor; RNA-seq, mRNA sequencing; CGGA, Chinese Glioma Genome Atlas; ROC, receiver-operating-characteristic; AUC, area under curve; FFPE, formalin-fixed paraffin-embedded; GSEA, Gene Set Enrichment Analysis; IHC, immunohistochemistry; GEPIA, Gene Expression Profiling Interactive Analysis; TCGA, Cancer Genome Atlas; RT-PCR, Real-time quantitative PCR; WB, Western blot.
1. Bray F, Ferlay J, Soerjomataram I, Siegel RL, Torre LA, Jemal A. Global Cancer Statistics 2018: GLOBOCAN Estimates of Incidence and Mortality Worldwide for 36 Cancers in 185 Countries. CA Cancer J Clin (2018) 68(6):394–424. doi: 10.3322/caac.21492
2. Ferlay J, Colombet M, Soerjomataram I, Mathers C, Parkin DM, Pineros M, et al. Estimating the Global Cancer Incidence and Mortality in 2018: GLOBOCAN Sources and Methods. Int J Cancer (2019) 144(8):1941–53. doi: 10.1002/ijc.31937
3. Molinaro AM, Taylor JW, Wiencke JK, Wrensch MR. Genetic and Molecular Epidemiology of Adult Diffuse Glioma. Nat Rev Neurol (2019) 15(7):405–17. doi: 10.1038/s41582-019-0220-2
4. Aalders KC, Tryfonidis K, Senkus E, Cardoso F. Anti-Angiogenic Treatment in Breast Cancer: Facts, Successes, Failures and Future Perspectives. Cancer Treat Rev (2017) 53:98–110. doi: 10.1016/j.ctrv.2016.12.009
5. Jin X, Kim L, Wu Q, Wallace L, Prager B, Sanvoranart T, et al. Targeting Glioma Stem Cells Through Combined BMI1 and EZH2 Inhibition. Nat Med (2017) 23(11):1352–61. doi: 10.1038/nm.4415
6. Cheng L, Huang Z, Zhou W, Wu Q, Donnola S, Liu JK, et al. Glioblastoma Stem Cells Generate Vascular Pericytes to Support Vessel Function and Tumor Growth. Cell (2013) 153(1):139–52. doi: 10.1016/j.cell.2013.02.021
7. Schulte JD, Aghi MK, Taylor JW. Anti-Angiogenic Therapies in the Management of Glioblastoma. Chin Clin Oncol (2020) 10(4):37. doi: 10.21037/cco.2020.03.06
8. Ferrara N, Adamis AP. Ten Years of Anti-Vascular Endothelial Growth Factor Therapy. Nat Rev Drug Discov (2016) 15(6):385–403. doi: 10.1038/nrd.2015.17
9. Ferrara N. VEGF and Intraocular Neovascularization: From Discovery to Therapy. Transl Vis Sci Technol (2016) 5(2):10. doi: 10.1167/tvst.5.2.10
10. Kovic B, Xie F. Economic Evaluation of Bevacizumab for the First-Line Treatment of Newly Diagnosed Glioblastoma Multiforme. J Clin Oncol (2015) 33(20):2296–302. doi: 10.1200/jco.2014.59.7245
11. Szopa W, Burley TA, Kramer-Marek G, Kaspera W. Diagnostic and Therapeutic Biomarkers in Glioblastoma: Current Status and Future Perspectives. BioMed Res Int (2017) 2017:8013575. doi: 10.1155/2017/8013575
12. Dudley W, Wickham R, Coombs N. An Introduction to Survival Statistics: Kaplan-Meier Analysis. J Adv Pract Oncol (2016) 7(1):91–100. doi: 10.6004/jadpro.2016.7.1.8
13. Touat M, Li YY, Boynton AN, Spurr LF, Iorgulescu JB, Bohrson CL, et al. Mechanisms and Therapeutic Implications of Hypermutation in Gliomas. Nature (2020) 580(7804):517–23. doi: 10.1038/s41586-020-2209-9
14. Tang Z, Li C, Kang B, Gao G, Li C, Zhang Z. GEPIA: A Web Server for Cancer and Normal Gene Expression Profiling and Interactive Analyses. Nucleic Acids Res (2017) 45(W1):W98–W102. doi: 10.1093/nar/gkx247
15. Subramanian A, Tamayo P, Mootha VK, Mukherjee S, Ebert BL, Gillette MA, et al. Gene Set Enrichment Analysis: A Knowledge-Based Approach for Interpreting Genome-Wide Expression Profiles. Proc Natl Acad Sci USA (2005) 102(43):15545–50. doi: 10.1073/pnas.0506580102
16. Zhu S, Shi W, Jie Y. Overexpression of BnPCS1, a Novel Phytochelatin Synthase Gene From Ramie (Boehmeria Nivea), Enhanced Cd Tolerance, Accumulation, and Translocation in Arabidopsis Thaliana. Front Plant Sci (2021) 12:639189. doi: 10.3389/fpls.2021.639189
17. Goldstein LJ, Gray R, Badve S, Childs BH, Yoshizawa C, Rowley S, et al. Prognostic Utility of the 21-Gene Assay in Hormone Receptor-Positive Operable Breast Cancer Compared With Classical Clinicopathologic Features. J Clin Oncol (2008) 26(25):4063–71. doi: 10.1200/JCO.2007.14.4501
18. Ruiz VY, Praska CE, Armstrong G, Kollmeyer TM, Yamada S, Decker PA, et al. Molecular Subtyping of Tumors From Patients With Familial Glioma. Neuro Oncol (2018) 20(6):810–7. doi: 10.1093/neuonc/nox192
19. Ceccarelli M, Barthel FP, Malta TM, Sabedot TS, Salama SR, Murray BA, et al. Molecular Profiling Reveals Biologically Discrete Subsets and Pathways of Progression in Diffuse Glioma. Cell (2016) 164(3):550–63. doi: 10.1016/j.cell.2015.12.028
20. Starossom SC, Campo Garcia J, Woelfle T, Romero-Suarez S, Olah M, Watanabe F, et al. Chi3l3 Induces Oligodendrogenesis in an Experimental Model of Autoimmune Neuroinflammation. Nat Commun (2019) 10(1):217. doi: 10.1038/s41467-018-08140-7
21. Dubbink HJ, Atmodimedjo PN, Kros JM, French PJ, Sanson M, Idbaih A, et al. Molecular Classification of Anaplastic Oligodendroglioma Using Next-Generation Sequencing: A Report of the Prospective Randomized EORTC Brain Tumor Group 26951 Phase III Trial. Neuro Oncol (2016) 18(3):388–400. doi: 10.1093/neuonc/nov182
22. Reuss DE, Sahm F, Schrimpf D, Wiestler B, Capper D, Koelsche C, et al. ATRX and IDH1-R132H Immunohistochemistry With Subsequent Copy Number Analysis and IDH Sequencing as a Basis for an “Integrated” Diagnostic Approach for Adult Astrocytoma, Oligodendroglioma and Glioblastoma. Acta Neuropathol (2015) 129(1):133–46. doi: 10.1007/s00401-014-1370-3
23. van den Bent MJ, Weller M, Wen PY, Kros JM, Aldape K, Chang S. A Clinical Perspective on the 2016 WHO Brain Tumor Classification and Routine Molecular Diagnostics. Neuro Oncol (2017) 19(5):614–24. doi: 10.1093/neuonc/now277
24. Fontana L, Tabano S, Bonaparte E, Marfia G, Pesenti C, Falcone R, et al. MGMT-Methylated Alleles Are Distributed Heterogeneously Within Glioma Samples Irrespective of IDH Status and Chromosome 10q Deletion. J Neuropathol Exp Neurol (2016) 75(8):791–800. doi: 10.1093/jnen/nlw052
25. Zhou K, Liu Y, Zhao Z, Wang Y, Huang L, Chai R, et al. ABCC8 mRNA Expression is an Independent Prognostic Factor for Glioma and can Predict Chemosensitivity. Sci Rep (2020) 10(1):12682. doi: 10.1038/s41598-020-69676-7
26. Alexandrov LB, Kim J, Haradhvala NJ, Huang MN, Tian Ng AW, Wu Y, et al. The Repertoire of Mutational Signatures in Human Cancer. Nature (2020) 578(7793):94–101. doi: 10.1038/s41586-020-1943-3
27. Liu W, Xu Z, Zhou J, Xing S, Li Z, Gao X, et al. High Levels of HIST1H2BK in Low-Grade Glioma Predicts Poor Prognosis: A Study Using CGGA and TCGA Data. Front Oncol (2020) 10:627. doi: 10.3389/fonc.2020.00627
28. Marubashi S, Fukuda M. Rab7B/42 Is Functionally Involved in Protein Degradation on Melanosomes in Keratinocytes. Cell Struct Funct (2020) 45(1):45–55. doi: 10.1247/csf.19039
29. Zhang GH, Zhong QY, Gou XX, Fan EX, Shuai Y, Wu MN, et al. Seven Genes for the Prognostic Prediction in Patients With Glioma. Clin Transl Oncol (2019) 21(10):1327–35. doi: 10.1007/s12094-019-02057-3
30. Togao O, Hiwatashi A, Yamashita K, Kikuchi K, Mizoguchi M, Yoshimoto K, et al. Differentiation of High-Grade and Low-Grade Diffuse Gliomas by Intravoxel Incoherent Motion MR Imaging. Neuro Oncol (2016) 18(1):132–41. doi: 10.1093/neuonc/nov147
31. Yadav VN, Altshuler D, Kadiyala P, Zamler D, Comba A, Appelman H, et al. Molecular Ablation of Tumor Blood Vessels Inhibits Therapeutic Effects of Radiation and Bevacizumab. Neuro Oncol (2018) 20(10):1356–67. doi: 10.1093/neuonc/noy055
32. Hosaka K, Yang Y, Seki T, Du Q, Jing X, He X, et al. Therapeutic Paradigm of Dual Targeting VEGF and PDGF for Effectively Treating FGF-2 Off-Target Tumors. Nat Commun (2020) 11(1):3704. doi: 10.1038/s41467-020-17525-6
33. Osterberg N, Ferrara N, Vacher J, Gaedicke S, Niedermann G, Weyerbrock A, et al. Decrease of VEGF-A in Myeloid Cells Attenuates Glioma Progression and Prolongs Survival in an Experimental Glioma Model. Neuro Oncol (2016) 18(7):939–49. doi: 10.1093/neuonc/now005
34. Wu HB, Yang S, Weng HY, Chen Q, Zhao XL, Fu WJ, et al. Autophagy-Induced KDR/VEGFR-2 Activation Promotes the Formation of Vasculogenic Mimicry by Glioma Stem Cells. Autophagy (2017) 13(9):1528–42. doi: 10.1080/15548627.2017.1336277
35. Bai C, Sun Y, Pan X, Yang J, Li X, Wu A, et al. Antitumor Effects of Trimethylellagic Acid Isolated From Sanguisorba Officinalis L. @ on Colorectal Cancer via Angiogenesis Inhibition and Apoptosis Induction. Front Pharmacol (2019) 10:1646. doi: 10.3389/fphar.2019.01646
36. Botto S, Streblow DN, DeFilippis V, White L, Kreklywich CN, Smith PP, et al. IL-6 in Human Cytomegalovirus Secretome Promotes Angiogenesis and Survival of Endothelial Cells Through the Stimulation of Survivin. Blood (2011) 117(1):352–61. doi: 10.1182/blood-2010-06-291245
37. Allavena P, Mantovani A. Immunology in the Clinic Review Series; Focus on Cancer: Tumour-Associated Macrophages: Undisputed Stars of the Inflammatory Tumour Microenvironment. Clin Exp Immunol (2012) 167(2):195–205. doi: 10.1111/j.1365-2249.2011.04515.x
Keywords: glioma, RAB42, vascular endothelial growth factor (VEGF), tumorigenesis, CGGA
Citation: Liu B, Su Q, Xiao B, Zheng G, Zhang L, Yin J, Wang L, Che F and Heng X (2021) RAB42 Promotes Glioma Pathogenesis via the VEGF Signaling Pathway. Front. Oncol. 11:657029. doi: 10.3389/fonc.2021.657029
Received: 26 January 2021; Accepted: 08 November 2021;
Published: 29 November 2021.
Edited by:
Kamalakannan Palanichamy, The Ohio State University, United StatesReviewed by:
Fatemeh Kazemi-Lomedasht, Pasteur Institute of Iran (PII), IranCopyright © 2021 Liu, Su, Xiao, Zheng, Zhang, Yin, Wang, Che and Heng. This is an open-access article distributed under the terms of the Creative Commons Attribution License (CC BY). The use, distribution or reproduction in other forums is permitted, provided the original author(s) and the copyright owner(s) are credited and that the original publication in this journal is cited, in accordance with accepted academic practice. No use, distribution or reproduction is permitted which does not comply with these terms.
*Correspondence: Lijuan Wang, d2FuZ2xqNzMwQDE2My5jb20=; Fengyuan Che, Y2hlMTk3MUAxMjYuY29t; Xueyuan Heng, eHVleXVhbmhlbmdAeWFob28uY29t
†Theses authors have contributed equally to this work
Disclaimer: All claims expressed in this article are solely those of the authors and do not necessarily represent those of their affiliated organizations, or those of the publisher, the editors and the reviewers. Any product that may be evaluated in this article or claim that may be made by its manufacturer is not guaranteed or endorsed by the publisher.
Research integrity at Frontiers
Learn more about the work of our research integrity team to safeguard the quality of each article we publish.