- 1PhD Program for Cancer Molecular Biology and Drug Discovery, College of Medical Science and Technology, Taipei Medical University and Academia Sinica, Taipei, Taiwan
- 2Graduate Institute for Cancer Biology & Drug Discovery, College of Medical Science and Technology, Taipei Medical University, Taipei, Taiwan
- 3Department of Orthopedics, Taipei Medical University Hospital, Taipei, Taiwan
- 4Department of Orthopaedics, School of Medicine, College of Medicine, Taipei Medical University, Taipei, Taiwan
- 5TMU Research Center of Cancer Translational Medicine, Taipei Medical University, Taipei, Taiwan
- 6The PhD Program of Translational Medicine, College of Science and Technology, Taipei Medical University, Taipei, Taiwan
- 7Clinical Research Center, Taipei Medical University Hospital, Taipei Medical University, Taipei, Taiwan
- 8Graduate Institute of Medical Sciences, National Defense Medical Center, Taipei, Taiwan
- 9School of Pharmacy, National Defense Medical Center, Taipei, Taiwan
- 10PhD Program in Biotechnology Research and Development, College of Pharmacy, Taipei Medical University, Taipei, Taiwan
Background: The application of computational and multi-omics approaches has aided our understanding of carcinogenesis and the development of therapeutic strategies. NSC765598 is a novel small molecule derivative of salicylanilide. This study aims to investigate the ligand-protein interactions of NSC765598 with its potential targets and to evaluate its anticancer activities in vitro.
Methods: We used multi-computational tools and clinical databases, respectively, to identify the potential drug target for NSC765598 and analyze the genetic profile and prognostic relevance of the targets in multiple cancers. We evaluated the in vitro anticancer activities against the National Cancer Institute 60 (NCI60) human tumor cell lines and used molecular docking to study the ligand-protein interactions. Finally, we used the DTP-COMPARE algorithm to compare the NSC765598 anticancer fingerprints with NCI standard agents.
Results: We identified mammalian target of rapamycin (mTOR)/epidermal growth factor receptor (EGFR)/inducible nitric oxide synthase (iNOS)/mitogen-activated protein 2 kinase 1 (MAP2K1)/fibroblast growth factor receptor (FGFR)/transforming growth factor-β1 (TGFB1) as potential targets for NSC765598. The targets were enriched in cancer-associated pathways, were overexpressed and were of prognostic relevance in multiple cancers. Among the identified targets, genetic alterations occurred most frequently in EGFR (7%), particularly in glioblastoma, esophageal squamous cell cancer, head and neck squamous cell cancer, and non–small-cell lung cancer, and were associated with poor prognoses and survival of patients, while other targets were less frequently altered. NSC765598 displayed selective antiproliferative and cytotoxic preferences for NSCLC (50% growth inhibition (GI50) = 1.12–3.95 µM; total growth inhibition (TGI) = 3.72–16.60 μM), leukemia (GI50 = 1.20–3.10 µM; TGI = 3.90–12.70 μM), melanoma (GI50 = 1.45–3.59 µM), and renal cancer (GI50 = 1.38–3.40 µM; TGI = 4.84–13.70 μM) cell lines, while panels of colon, breast, ovarian, prostate, and central nervous system (CNS) cancer cell lines were less sensitive to NSC765598. Interestingly, NSC765598 docked well into the binding cavity of the targets by conventional H-bonds, van der Waal forces, and a variety of π-interactions, with higher preferences for EGFR (ΔG = −11.0 kcal/mol), NOS2 (ΔG = −11.0 kcal/mol), and mTOR (ΔG = −8.8 kcal/mol). NSC765598 shares similar anti-cancer fingerprints with NCI standard agents displayed acceptable physicochemical values and met the criteria of drug-likeness.
Conclusion: NSC765598 displayed significant anticancer and potential multi-target properties, thus serve as a novel candidate worthy of further preclinical studies.
Introduction
Currently, cancer is one of the leading causes of global morbidity and the second leading cause of mortality worldwide (1), accounting for an estimated 19.3 million new cases and 9.9 million deaths in 2020 (2). One in five men or women develops cancer, while death occurs in 1 out of 8 men or 1 out of 11 women. However, due to increasing rates of predisposing factors, such as smoking, a sedentary lifestyle, being overweight, and increasing pollution associated with urbanization and industrial development, cancer is predicted to top the rank of leading causes of global mortality and be the most important independent dictator of a poor life expectancy in the 21st century (3). In 2020, female breast cancer has surpassed lung cancer as the most commonly diagnosed cancer accounting for 11.7% (2.3 million new cases) (2), and closely followed by lung (11.7%), colorectal (10.0%), prostate (7.3%), and stomach (7.7%) cancers, whereas colorectal (9.4% deaths), liver (8.3% death), stomach (7.7% deaths), and breast (6.9% deaths) cancers followed lung cancer (with an estimated 1.8 million deaths (18%) in terms of mortality rates (2), with higher incidences in males than in females (4). Among women, breast cancer is the most frequently diagnosed and a prominent cause of cancer deaths, followed by colorectal, lung, and cervical cancers (3). Unfortunately, the global cancer burden is expected to be 28.4 million cases in 2040, a 47% rise from 2020 (2). This projection is solely due to the growth and aging of the population and an increasing prevalence of risk factors.
The most common strategy for managing cancer is conventional therapies involving surgery and chemo- and radiotherapies. However, despite the early effectiveness demonstrated by these oncological strategies (5, 6), therapeutic resistance and toxicity, among other factors, have limited the success rates and long-term survival of cancer patients. Hence, research is needed on novel therapeutic options, which can offer better and more-specific multi-target antitumor activities (7), with minimal side effects to ultimately enhance the survival of cancer patients. Without a doubt, multitarget small molecules with anticancer activities represent alternative strategies with promising features for the realization of novel drugs that could target multiple aberrant signaling pathways (8, 9), offering fewer chances for drug resistance and thus better prognoses of cancer patients.
Several potential molecular targets exist for exploring the dysregulation of signaling pathways of cancer cells (10–12). Growth factors, including epidermal growth factor (EGF), fibroblast growth factor (FGF), transforming growth factor (TGF), and vascular endothelial growth factor (VEGF), are compact molecules that play important roles in regulating cellular communication, growth and differentiation, proliferation, survival, migration, and metastasis of cancer cells (13, 14). Upon receptor binding, EGF and TGF activate downstream mitogen-activated protein kinase (MAPK) and AKT-mammalian target of rapamycin (mTOR) pathways (15), which regulate apoptosis and cell proliferation (16), while inducible nitric oxide synthase (iNOS) modulates cellular bioenergetics pathways in cancer (17). Therefore, these signaling pathways are important for cancer initiation, progression, and metastasis and thus act as attractive targets for anticancer therapy exploration. Mapping these targets with corresponding drugs, therefore, has become a relevant prerequisite for drug discovery.
Over the years, rational drug design (RDD) based on computational biology and bioinformatics has not only changed the way drugs are designed but also speed up drug discovery and development processes while reducing costs and the wasting of resources (18). Computational and bioinformatics studies of cancer involve a variety of methods including mining and analysis of clinical data to give insights into the prognostic relevance of gene signatures, analyze geno-phenotypic responses to clinical drugs, and identify novel compounds with their potential targets before in vitro and in vivo validation studies. One such method is molecular modeling and docking studies of receptor-ligand interactions. Molecular docking is frequently employed to predict binding orientations of small molecules with their target proteins in order to calculate the affinities and activities of the small-molecule drug candidates (18, 19).
In our previous study, a salicylanilide analogue, NDMC101 (PubChem CID: 60202556), was synthesized, and we observed significant cytotoxic effects via downregulation of proliferative and inflammatory markers (20). A series of modifications of the salicylanilide core scaffold of the lead molecule were synthesized, and their preclinical anticancer activities against a variety of cancers were reported (9, 21–23). As a continuing effort to find and screen more active derivatives of NDMC101, in the present study, we reported a new derivative, NSC765598, for anticancer activities and identified mTOR/EGR receptor (EGFR)/iNOS/MAP2K/TGF-β1 (TGFB1)/fibroblast growth factor receptor-1 (FGFR1) as its potential targets.
Materials and Methods
Drug Likeness, ADMET Properties, and Target Identification of NSC765598
NSC765598 was synthesized as a derivative of diflunisal and salicylanilides (NDMC101) (Figure 1A) via reactions (SOCl2, anhydrous THF, reflux, 8 h; and aniline, anhydrous THF, reflux 14-16) described in our previous study (21). We analyzed the drug-likeness, pharmacokinetics, and medicinal chemistry of NSC765598 using ADMETLab (http://admet.scbdd.com/), SwissADME software (http://www.swissadme.ch) (24), and the Lipinski’s rule-of-five algorithm (http://www.scfbio-iitd.res.in/software/drugdesign/lipinski.jsp) which identifies drug candidates based on the principle that drug candidates must have a molecular weight of < 500 Da, fewer than five hydrogen bond donors, fewer than ten hydrogen bond acceptors, the lipophilicity of < 5 LogP, and a molar refractivity range of 40 to 130 (25). NSC765598’s targets were identified using the PharmMapper Server (http://lilab-ecust.cn/pharmmapper/index.html) (26), SwissADME software, and computer-aided Prediction of Biological Activity Spectra (PASS) web resources (http://way2drug.com/dr) (27).
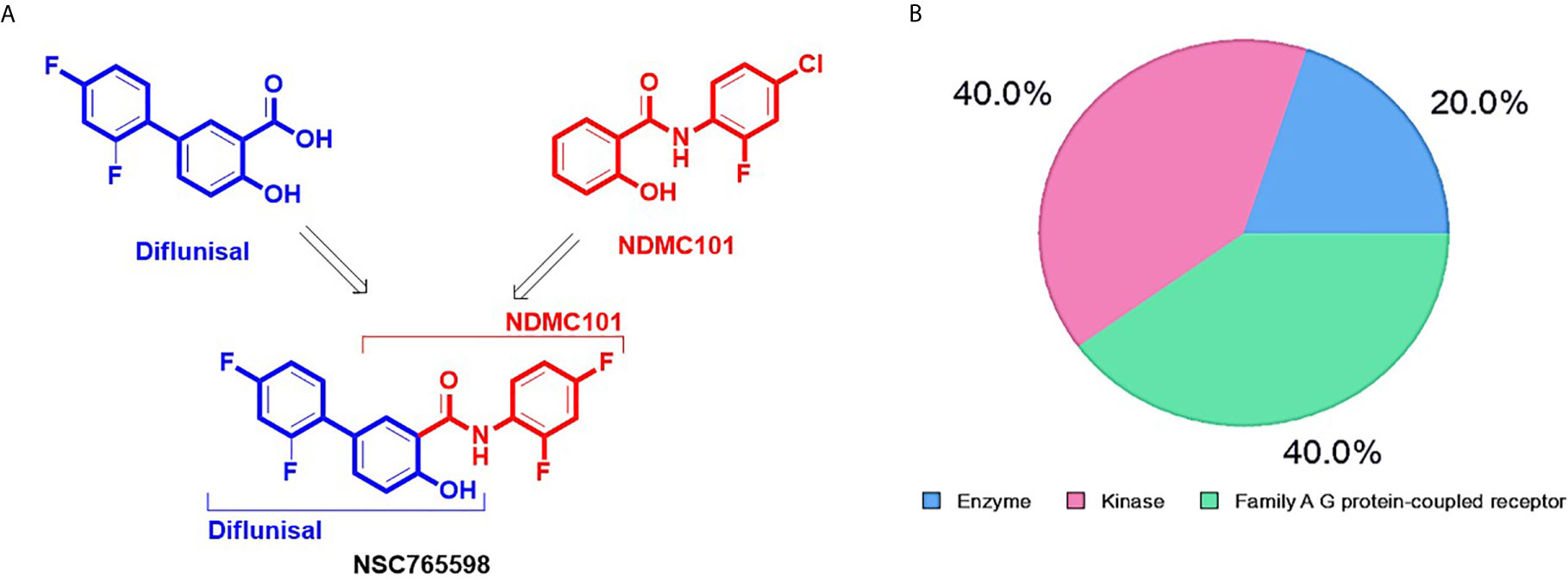
Figure 1 Synthetic protocol and potential drug target classification of N-(2,4-difluorophenyl)-2’,4’-difluoro-4-hydroxybiphenyl-3-carboxamide (NSC765598). (A) NSC765598 is a chemical derivative of diflunisal and NDMC101s (reaction conditions; SOCl2, anhydrous THF, reflux, 8 h; and aniline, anhydrous THF, reflux 14-16) (B). The SwissTarget identified top 20 targets of NSC765598 are classified into enzymes (20%), kinases (40%) and Family A G protein-coupled receptor (40%).
Clinical Data Mining of Databases
Differential gene expression profiles of the EGFR, iNOS, mTOR, FGF receptor (FGFR), TGF receptor (TGFR), and MAP2K1 across The Cancer Genome Atlas (TCGA) databases were analyzed using the Tumor IMmune Estimation Resource (TIMER2.0) resource (http://timer.cistrome.org/) (28). The prognostic relevance of the genes in 9736 tumor samples across 33 TCGA cancer types was analyzed using the Gene Expression Profiling Interactive Analysis (GEPIA) (http://gepia.cancer-pku.cn/) (29). We collected the RNA expression profile of the genes and then set the median expression as the expression threshold to split the patient samples into high‐expression and low‐expression groups, and used the Kaplan‐Meier survival plot to assess the overall survival (OS) with the hazard ratio (HR), a 95% confidence interval (CI), and a log‐rank test p‐value. We also explored the cancer genomic dataset using the cBioPortal tool (http://www.cbioportal.org/) to analyze genomic alterations and survival and perform group comparisons of EGFR, iNOS, mTOR, FGFR, TGFR, and MAP2K1 in 10,953 cancer patients (10,967 samples) from different cancer types (30, 31).
Protein-Protein Interactions (PPIs) and Functional Enrichment Analysis
PPI networks and functional enrichment analyses including Kyoto Encyclopedia of Genes and Genomes (KEGG) pathways and Gene Ontology (GO) biological processes of the selected NSC765598 target gene list were conducted using the Search Tool for Retrieval of Interacting Genes (STRING, vers. 10.5, (https://www.string-db.org/) with the adjusted threshold confidence set to 0.900 (32) and Enrich (https://maayanlab.cloud/Enrichr/enrich#) (33, 34). KEGG enrichment criteria were set to p < 10−5, gene count of ≥ 3, and strength of ≥ 2.
Receptor-Ligand Interaction Study
The crystal structures of MAPK2K (PDB:3FXW), FGF (PDB: 1IJT), the mTORdeltaN-mLST8 complex (PDB: 4JSN), tumor growth factor beta1 (PDB:1KLC), EGFR kinase (T790M/L858R) apo (PDB: 5EDP), and iNOS (PDB:2BHJ) were obtained from the Protein Data Bank (https://www.rcsb.org/ ) in protein data bank (PDB) file format and subsequently converted into the Auto Dock Pdbqt format using AutoDock Vina (vers. 0.8, the Scripps Research Institute, La Jolla, CA, USA) (35). The three-dimensional (3D) structure of NSC765598 was built out with the Avogadro molecular builder and visualization tool vers. 1.XX (http://avogadro.cc/) (36). The structure was retrieved in mol2 file format and was subsequently transformed into PDB format using the PyMOL Molecular Graphics System, vers. 1.2r3pre (Schrödinger; https://pymol.org/edu/?q=educational/) followed by conversion to pdbqt. The three-dimensional (3D) structures of standard drugs; Dactolisib (CID: 11977753), Mirdametinib (CID: 9826528), Gefitinib (CID: 123631), N-Iminoethyl-L-lysine dihydrochloride (CID: 2733505), Erdafitinib (CID: 67462786), and Galunisertib (CID: 10090485) were retrieved in SDF file format from the PubChem database and subsequently converted to PDB format using the PyMOL tool and then to pdbqt format using the AutoDock Vina. Receptors were prepared by pre-docking removal of water (H20) molecules, the addition of hydrogen atoms (polar only), and the addition of Kolmman charges (8). Molecular docking was performed using AutoDock Vina with all parameters set to default values, and all bonds in the ligand rotated freely while considering the receptor to be rigid. A grid box of 40 × 40 × 40 Å at X, Y, and Z dimensions and a spacing of 1.0 angstrom were used. All docking was performed at an exhaustiveness of 8. The docking outcome was analyzed in terms of the ligand’s affinity for the receptor and was expressed as binding energy values in Kcal/mol. The interactions in 2D conformation were visualized using the Discovery studio visualizer vers. 19.1.0.18287 (BIOVIA, San Diego, CA, USA) (37), while the hydrophobic contacts between the receptor-ligand complex were mapped out using the protein-ligand interaction profiler web tool (https://plip-tool.biotec.tu-dresden.de/plip-web/plip/index) (38).
In Vitro Anticancer Screening of NSC765598 Against NCI60 Panels of Human Tumor Cell Lines
NSC765598 was screened for anti-proliferative and cytotoxic activities against NCI60 panels of cancer cell lines at the National Cancer Institute (NCI). Protocols for in vitro screening of bioactive compounds against NCI60 panels of cancer cell lines are well documented (39, 40). Briefly, about 5000 to 40,000 cells were seeded into each well of 96-well plates for 24 h. Cells were treated with a single dose (10 μM) of NSC765598 and incubated at 37°C, 5% CO2, 95% air, and 100% relative humidity for 48 h. Cell viability was determined using the sulforhodamine B (SRB) solution protocol (41). Following single-dose testing, NSC765598, was further evaluated for dose-dependent activities at five concentrations (0, 0.1, 1.0, 10, and 100 μM). Using the seven absorbance measurements [time zero, (Tz), control growth, (C), and test growth in the presence of drug at the five concentration (Ti)], the percentage growth is calculated at each of the drug concentrations levels. The percentage growth inhibition is calculated as: [(Ti-Tz)/(C-Tz)] × 100 for concentrations for which Ti>/=Tz or [(Ti-Tz)/Tz] × 100 for concentrations for which Ti<Tz.
Three dose-response parameters including the drug concentration causing total growth inhibition (TGI), drug concentration causing a 50% reduction in the net protein increase in control cells during the drug incubation (GI50), and drug concentration causing 50% cell lethality (LC50), indicating a net loss of cells following treatment) (42) were computed. GI50 is calculated from [(Ti-Tz)/(C-Tz)] × 100 = 50, TGI is calculated from Ti = Tz, while the LC50 is calculated from [(Ti-Tz)/Tz] × 100 = −50. Values are calculated for each of these three parameters if the level of activity is reached; however, if the effect is not reached or is exceeded, the value for that parameter is expressed as greater or less than the maximum or minimum concentration tested.
DTP-COMPARE Analysis of NSC765598 Anticancer Fingerprints
The activity patterns (fingerprints) of NSC765598 were correlated to NCI synthetic compounds and standard agents using the DTP-COMPARE algorithms (43). The NSC numerical IDs were used as “seed” while GI50, TGI, and LC50 were set as the endpoints (8).
Data Analysis and Visualization
Data were visualized using GraphPad Prism 8 software. Overall survival of the cohorts was visualized in Kaplan-Meier (K-M) survival plots. The statistical significance of differentially expressed genes was evaluated using the Wilcoxon test. *p<0.05; **p<0.01; and ***p<0.001 indicate statistical significance. Growth inhibition was calculated relative to cells without drug treatment and the time-zero control. The growth inhibition by NSC765598 in the single-dose assay was obtained by subtracting the positive value on the plot from 100, i.e., a value of 60 would indicate 40% growth inhibition.
Results
Drug-Likeness, Physicochemical, and ADMET Properties of NSC765598
NSC765598 was synthesized as a white powder purity of > 95%. Our evaluation of the drug- properties revealed that NSC765598 exhibited acceptable physicochemical and ADMET properties, and satisfied Lipinski’s rule of five for drug-likeness. It has good synthetic accessibility (2.27), a high bioavailability score (0.607–0.87), and low toxicity (LD50 = 880.766 mg/kg). Interestingly, NSC765598 exhibited a high human intestinal absorption capability and was permeant to the blood-brain barrier (Table 1).
EGFR, mTOR, NOS2, TGFB1, MAP2K1, and FGFR1 Are Potential Drug Targets for NSC765598
Our PASS analysis of NSC765598 predicted among other activities, anti-inflammatory, antiangiogenic, and anti-proliferative activities, with Pa (probability of being active) values of 0.491, 0.443, and 0.205, respectively and low Pi (probability of being inactive) values of < 0.060, 0.029, and 0.092, respectively. NSC765598 was also predicted to be an antagonist of connective tissue growth factor (Pa = 0.796, Pi = 0.001), platelet-derived growth factor receptor kinase (Pa = 0.695, Pi = 0.007), VEGF (Pa = 0.141, Pi = 0.037), hepatocyte growth factor (HGF) (Pa = 0.132, Pi = 0.018), FGF (Pa = 0.099, Pi = 0.074), MAPK (Pa = 0.137, Pi = 0.005), EGFR (Pa = 0.139, Pi = 0.004), TGFB1 (Pa = 0.150, Pi = 0.051), iNOS (Pa = 0.232, Pi = 0.011), and tumor necrosis factor (TNF)-α (Pa = 0.150, Pi = 0.051) (Supplementary Table 1). Furthermore, NSC765598 target predictions using SwissTarget algorithm partitioned the top 20 targets into three classes: enzymes (20%), kinases (40%), and family A G protein-coupled receptors (40%) (Figure 1B). Coherent with the PASS target predictions, SwissTarget also identified EGFR, TGFB1, FGFR1, iNOS, VEGFR2, MAPK, and serine/threonine-protein kinase mTOR as top druggable targets of NSC765598 (Supplementary Table 2). Furthermore, we also used PharmMapper Server, an integrated pharmacophore matching platform with statistical methods to identify potential targets. Interestingly out of 1627 drug targets (459 of which were human protein targets) on PharmMapper, EGFR, TGF-βR1, FGFR1 and −2, VEGFR2, iNOS, MAPK, HGFR, insulin-like growth factor 1 receptor (IGF1R), B-Raf proto-oncogene serine/threonine-protein kinase, serine/threonine-protein kinase Chk1, and cyclin-dependent kinase 5 (CDK5) activator 1 were predicted to be among the top 20 targets for NSC765598. The top 20 predicted targets exhibited three or more total protein-ligand interactions, two or more hydrophobic interactions with a normalized fit score range of 0.7082 to 0.9998, and a z score range of 0.1056 to 0.641082 (Supplementary Table 3). Collectively, six genes, viz., iNOS, TGFB1, VEGFR2, FGFR, EGFR, and MAPK2 were predicted by the three algorithms (Figures 2A, B), while two genes, viz., signal transducer and activator of transcription (STAT) and serine/threonine-protein kinase (mTOR) were predicted to be NSC765598 top ranked target by 2 algorithms.
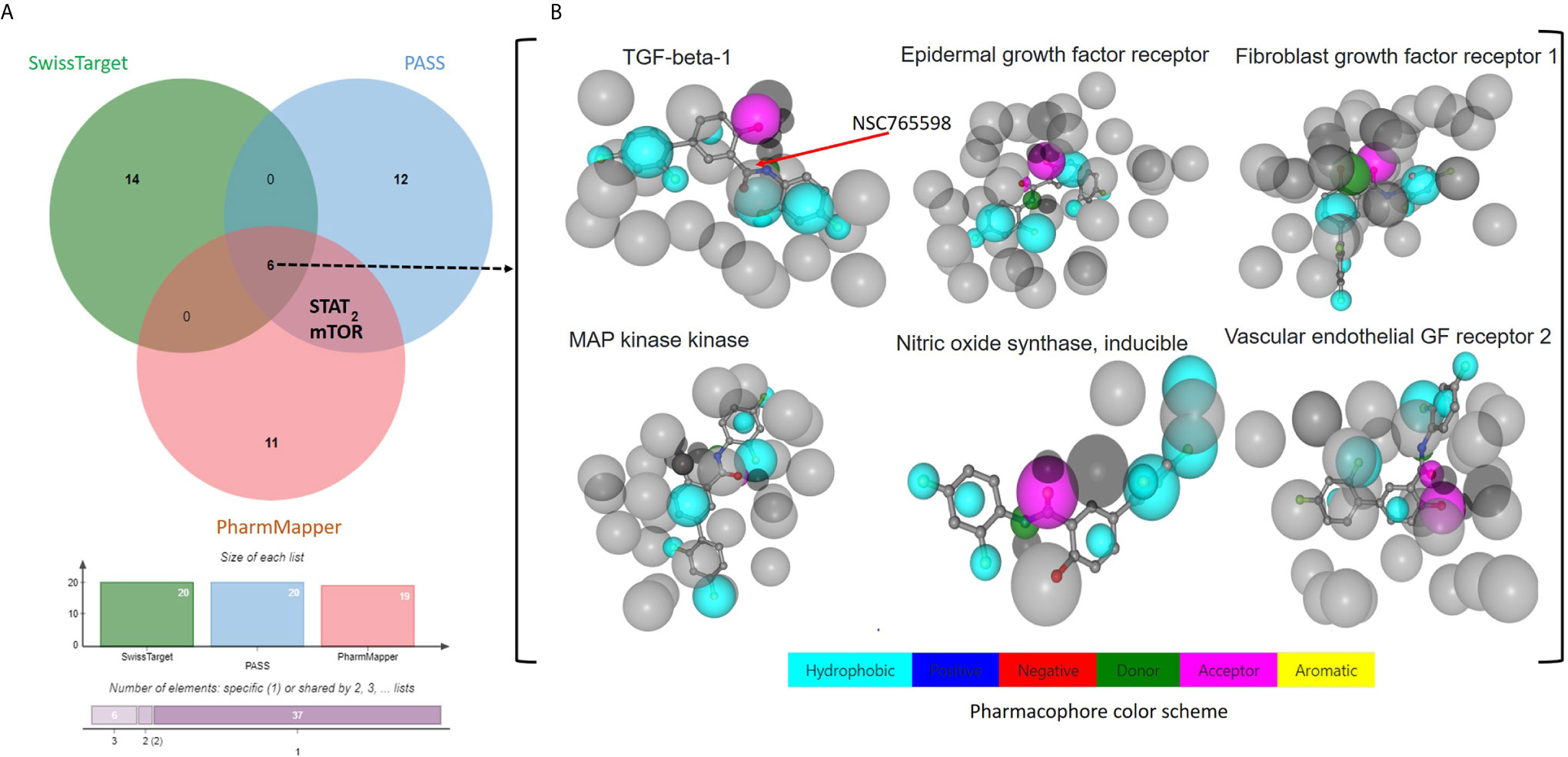
Figure 2 Potential targets for NSC765598. (A) Venn diagram showing common targets of NSC765598 as revealed by the SwissTarget, PASS, and PharmMapper algorithms. Six genes including iNOS, TGFB1, vascular endothelial growth factor receptor 2, FGFR, EDFR, and MAPK2 were predicted by the three algorithms (Figures 3A, B), while two genes, viz., signal transducer and activator of transcription and serine/threonine-protein kinase mTOR, were predicted to be top ranked targets of NSC765598 by the two algorithms. (B) Pharmacophore-based models of the six commonly identified NSC765598 target predictions via protein-receptor interactions.
NSC765598 Targets Are Enriched in Cancer-Associated Biological Processes and Signaling Pathways
Our second-order clustering of NSC765598 gene targets generated 26 nodes, 176 edges, 13.5 average node degrees, a 0.811 average local clustering coefficient, and a PPI enrichment p value of < 1.0e-16. Hub genes with the highest numbers of nodes were mTOR, MAP2K1, and EGFR with 23, 14, and 13 nodes, respectively, and connectivity scores that ranged 0.433 to 0.999 (mTOR), 0.433–0.999 (EGFR), and 0.402 to 0.999 (MAP2K1), while FGFR1 (0.433–0.836), TGFB1 (0.473–0.965), and NOS2 (0.473) had the fewest nodes with four, four, and one, respectively. Genes with the highest interaction scores (con. score = 0.999) with mTOR were RPS6KB1, RHEB, LAMTOR1/2/4/5, EIF4EBP1, MAPKAP1, RICTOR, and RPTOR; those with EGFR were GRB2 and EGF; and that with MAP2K1 was BRAF (Figure 3C and accompanying table). To gain insights into biological processes and pathways of the selected NSC765598 targets, KEGG pathway and GO enrichment analyses were conducted. As shown in Figure 3A, the top 10 KEGG pathways that were significantly enriched (p < 10−5, gene count of ≥ 3, and strength of ≥ 2) for selected NSC765598 targets included central carbon metabolism in cancer (p = 1.516e-9), pancreatic cancer (p = 2.720e-9), colorectal cancer (p = 4.747e-9), prostate cancer (p = 7.739e-9), the hypoxia-inducible factor (HIF)-1 signaling pathway (p = 8.756e-9), melanoma (p = 8.877e-7), glioma (p = 0.000001005), relaxin signaling pathway (p = 2.530e-8), choline metabolism in cancer (p = 0.000002328) and erbb signaling pathway (p = 0.000001468). The top 10 enriched biological processes (Figure 3B) were positive regulation of production of miRNAs involved in gene silencing by miRNA (p = 4.69E-06), salivary gland morphogenesis (p = 1.29E-05), positive regulation of protein kinase activity (p = 1.29E-05), positive regulation of epithelial cell proliferation (p = 1.54E-05), regulation of cardiocyte differentiation (p = 2.29E-05), positive regulation of MAPK activity (p = 3.20E-05), embryonic organ development (p = 8.89E-05), positive regulation of epithelial cell migration (p = 0.00016), glial cell differentiation (p = 0.00021), and positive regulation of the extracellular signal-regulated kinase 1 (ERK1) and ERK2 cascade (p = 0.00031). Collectively, our observations suggested that the selected NSC765598 targets were enriched in biological processes and pathways associated with growth development and cancer progression.
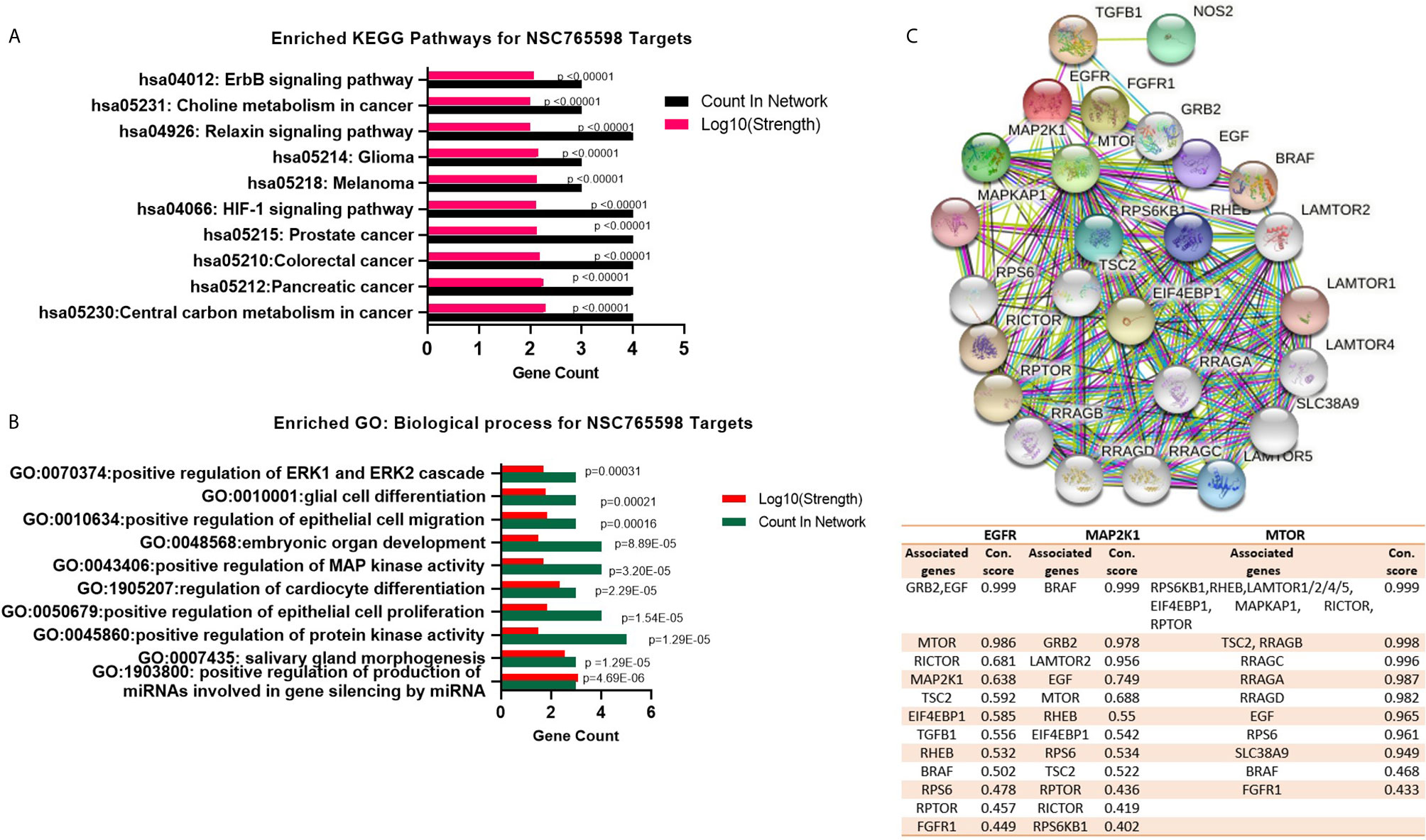
Figure 3 NSC765598 targets are enriched in cancer-associated biological processes and signaling pathways. (A) Enriched Kyoto Encyclopedia of Genes and Genomes (KEGG) pathway of NSC765598 targets. Enrichment was considered at p < 10-6, a gene count of ≥ 3, and a strength of ≥ 2. (B) Enriched Gene Ontology (GO) of NSC765598 targets. (C) Protein-protein interaction (PPI) network of mTOR/EGFR/iNOS/MAP2K1/FGFR/TGFB1. Second-order clustering of NSC765598 gene targets generated 26 nodes, 176 edges, 13.5 average node degrees, an 0.811 average local clustering coefficient, and a PPI enrichment p-value of < 1.0e-16. Hub genes with the highest nodes were mTOR, MAP2K1, and EGFR with 23, 14, and 13 nodes, respectively The accompanying table shows connection scores of nodes with the top three hub genes (mTOR, MAP2K1, and EGFR).
EGFR, mTOR, NOS2, TGFB1, MAP2K1, and FGFR1 Are Overexpressed and Are of Prognostic Relevance in Multiple Cancers
We analyzed differential expression profiles of EGFR between cancer and matched normal tissues across all TCGA tumors and found that cancer tissues expressed EGFR at significantly (p < 0.001) higher levels than did adjacent normal tissues in breast cancer (BRCA), colorectal adenocarcinoma (COAD), HNSC, kidney renal clear cell carcinoma (KIRC), lung squamous cell carcinoma (LUSC), prostate adenocarcinoma (PRAD), rectum adenocarcinoma (READ), and uterine corpus endometrial carcinoma (UCEC); NOS2 at higher levels in cholangiocarcinoma (CHOL), esophageal carcinoma (ESCA), kidney renal papillary cell carcinoma (KIRP), liver hepatocellular carcinoma (LICH), lung adenocarcinoma (LUAD), thyroid cancer (THCH), and UCEC; TGFB1 at higher levels in urothelial bladder carcinoma (BLCA), CHOL, HNSC, kidney chromophobe (KICH), KIRC, LICH, LUAD, LUSC, skin cancer (SKCM), and THCA; mTOR at higher levels in ESCA, HNSC, LICH, LUAD, LUSC, PRAD, stomach adenocarcinoma (STAD), and UECE; MAP2K1 at higher levels in BRCA, ESCA, HNSC, KIRP, LICH, LUSC, PRAD, STAD, LUAD, THCA, and UECE; and FGFR1 at higher levels in BLCA, BRCA, CHOL, COAD, LUAD, LUSC, PRAD, and THCA than adjacent normal tissues (Figure 4A). However, cancer tissues expressed mTOR at significantly (p < 0.001) lower levels than adjacent normal tissues in KICH, KIRC, and KIRP; FGFR1 at lower levels in READ and THCA; and NOS2 at lower levels in KICH than adjacent normal tissues. In addition, we explored the prognostic relevance of EGFR, mTOR, NOS2, TGFB1, mTOR, and FGFR1. First, we grouped the 9736 TCGA cancer cohorts into high or low RNA expressions of EGFR, mTOR, NOS2, TGFB1, mTOR, and FGFR1 and compared the survival of cohorts from the two groups. Results showed that cohorts with higher mRNA expressions of EGFR, MAP2K1, and NOS2 exhibited shorter overall survival (OS) than the cohort with lower expression profiles. However, there was no significant correlation (p > 0.05) with OS for TGFB1, mTOR, or FGFR1 expressions (Figure 4B). Higher EGFR expression was also found to be associated with poor DFS of the cohorts, whereas mTOR, NOS2, TGFB1, MAP2K1, and FGFR1 expressions were not associated with DFS of the cohorts (Supplementary Figure 1).
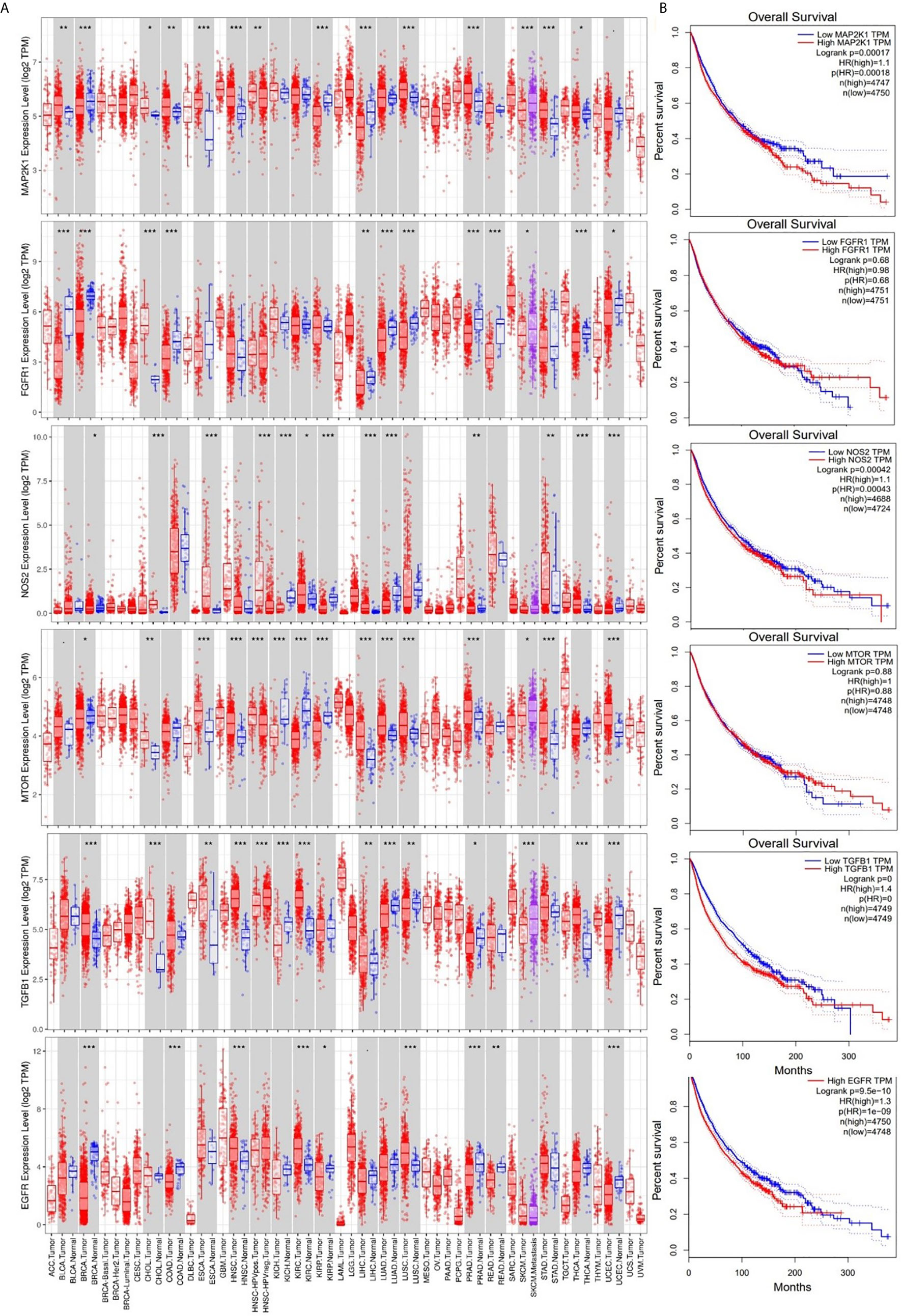
Figure 4 Expression levels and prognostic values of epidermal growth factor receptor (EGFR), mammalian target of rapamycin (mTOR), nitric oxide synthase 2 (NOS2), transforming growth factor-β1 (TGFB1), mitogen-activated protein 2 kinase 1 (MAP2K1), and fibroblast growth factor receptor 1 (FGFR1) in The Cancer Genome Atlas (TCGA) cancer cohorts. (A) Box plots showing differential gene expression levels (log2 TPM) of signal transduction and activator of transcription 3 (STAT3)/cyclin-dependent kinase 2 (CDK2)/4/6 between tumor and adjacent normal tissue samples across TCGA database. Blue labels indicate normal tissues, and red labels indicate tumor samples. (B) Kaplan-Meier curve of overall survival of cancer cohorts with low and cohorts with high RNA expression levels of EGFR, mTOR, NOS2, TGFB1, MAP2K1, and FGFR1. Higher RNA expression profiles of MAP2K1/NOS2/EGFR were correlated with low overall survival of cohorts. The statistical significance of differentially expressed genes was evaluated using the Wilcoxon test. * p<0.05; ** p<0.01; *** p<0.001.
Our correlation analysis revealed that EGFR expression was strongly correlated with expressions of mTOR (r= 0.262–0.496) and FGFR1 (r= 0.203–0.348) in liver, lung, kidney, glioblastoma, colon, head and neck, and breast cancers. MAP2K1 expression was strongly correlated with expressions of EGFR (r= 0.252–0.62) in liver, lung, kidney, colon, head and neck, and breast cancers but negatively correlated in glioblastoma (r = −0.062). EGFR expression was strongly correlated with expressions of NOS2 (r= 0.287–0.388) in liver cancer, kidney cancer and, breast cancer, poorly correlated in head and neck cancer (r=0.082) but show no association (r = −0.001) in colon and lung cancer (Figure 5).
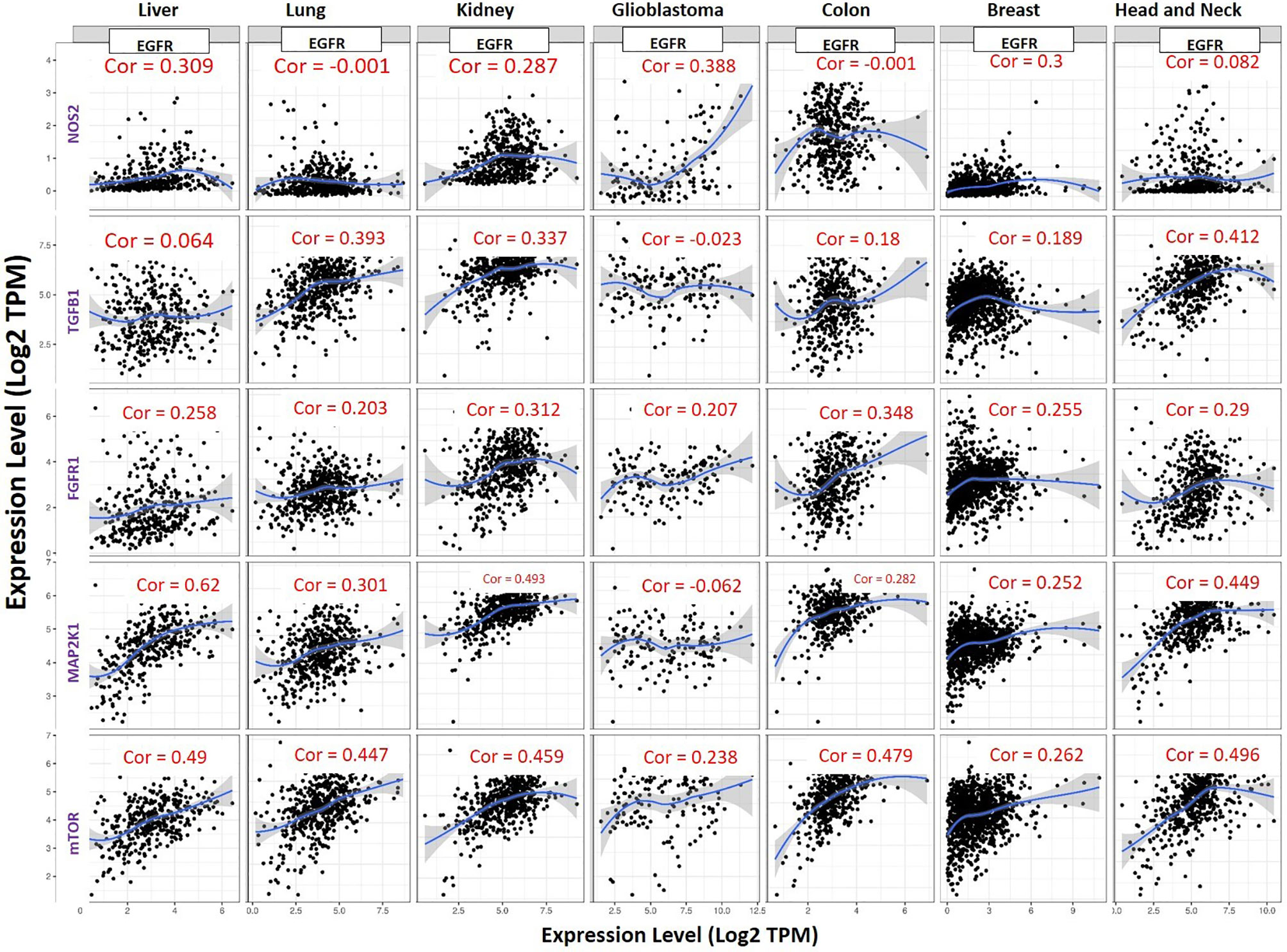
Figure 5 Expression scatterplots of EGFR correlations with NOS2, TGFB1, MAP2K1, mTOR and FGFR1 in multiple cancer types. The strength of correlations between the genes is reflected by the purity-adjusted partial spearman’s rho value, where a value of r= 1 means a perfect positive correlation and a value of r= −1 means a perfect negative correlation.
Genetic alterations of EGFR Are Associated With Poor Prognoses of Cancer Patients
We explored the cancer genomic dataset from the cBioPortal to analyze genomic alterations in EGFR, iNOS, mTOR, FGFR, TGFR, and MAP2K1 of 10,953 cancer patients (10,967 samples) from different cancer types. We found that of the 10,967 cancer cohorts representing 33 cancer types, genetic alterations in EGFR occurred in 821 (7%) cohorts of 26 cancer types (Figure 6A). EGFR mutations occurred most frequently in glioblastomas (47.3%), esophageal squamous cell carcinoma (17.89%), head and neck squamous cell carcinoma (12.62%), NSCLC (12.16%), esophagogastric adenocarcinomas (10.7%), and diffuse gliomas (10.53%), while pleural mesothelioma, seminoma, well-differentiated thyroid cancer, thymic epithelial tumor, ocular melanoma, and mature B cell neoplasm cohorts were devoid of EGFR mutations (Figure 6A). The most common alterations of EGFR gene were amplification and gene gain, while gene deletions were the least common alterations in EGFR genes (Figures 6A, E). These alterations in EGFR genes were associated with low OS (p < 10−10), disease-specific survival (p < 10−10), and disease-progression survival (p < 10−10) in those cohorts (Figures 6B–D). We also determine the gene alteration co-occurrence frequencies with respect to EGFR-altered and EGFR-unaltered cohorts and found a number of other gene mutations that co-occurred with alteration of EGFR (Figures 6F, G). The top ten gene mutations that were significantly (p value= 1.07 × 10−23 to 4.79 × 10−21 and q value 2.07 × 10−19 to 9.32 × 10−18) enriched in EGFR-altered cohorts were RICTOR, UGGT1, ATRNL1, AHNAK2, RIMS2, DSP, STXBP5L, DUOX2, PKD1L1, and MYH3 (Figure 6F, Table 2), while only two genes, viz., KRAS (p = 11.7 × 10−03 and q = 2.22 × 10−03) and IDH1 (p = 0.017 and q = 0.0194) were enriched in unaltered EGFR cohorts (Figure 6G, Table 2). However, 10 genes, including TP53 (46.78% and 36.01%), TTN (43.03% and 29.05%), MUC16 (30.56% and 18.43%), CSMD3 (29.19% and 12.15%), FLG (22.12% and 10.38%), RYR2 (22.12% and 11.92%), LRP1B (21.45% and 11.73%), SYNE1 (20.38% and 11.34%), PCLO (19.57% and 9.71%), and USH2A (19.17% and 10.18%) had higher mutation frequencies in both EGFR-altered and EGFR-unaltered cohorts, respectively (Figure 6H). However, only 6% (662/10953), 4% (478/10967), 3% (346/10967), 1% (141/10967), and 1% (133/10967) of cohorts had FGFR, mTOR, iNOS, TGFB1, and MAP2K1 gene alterations (Supplementary Figure 2) and were not associated (p > 0.05) with poor survival or prognosis (Supplementary Figures 3–7).
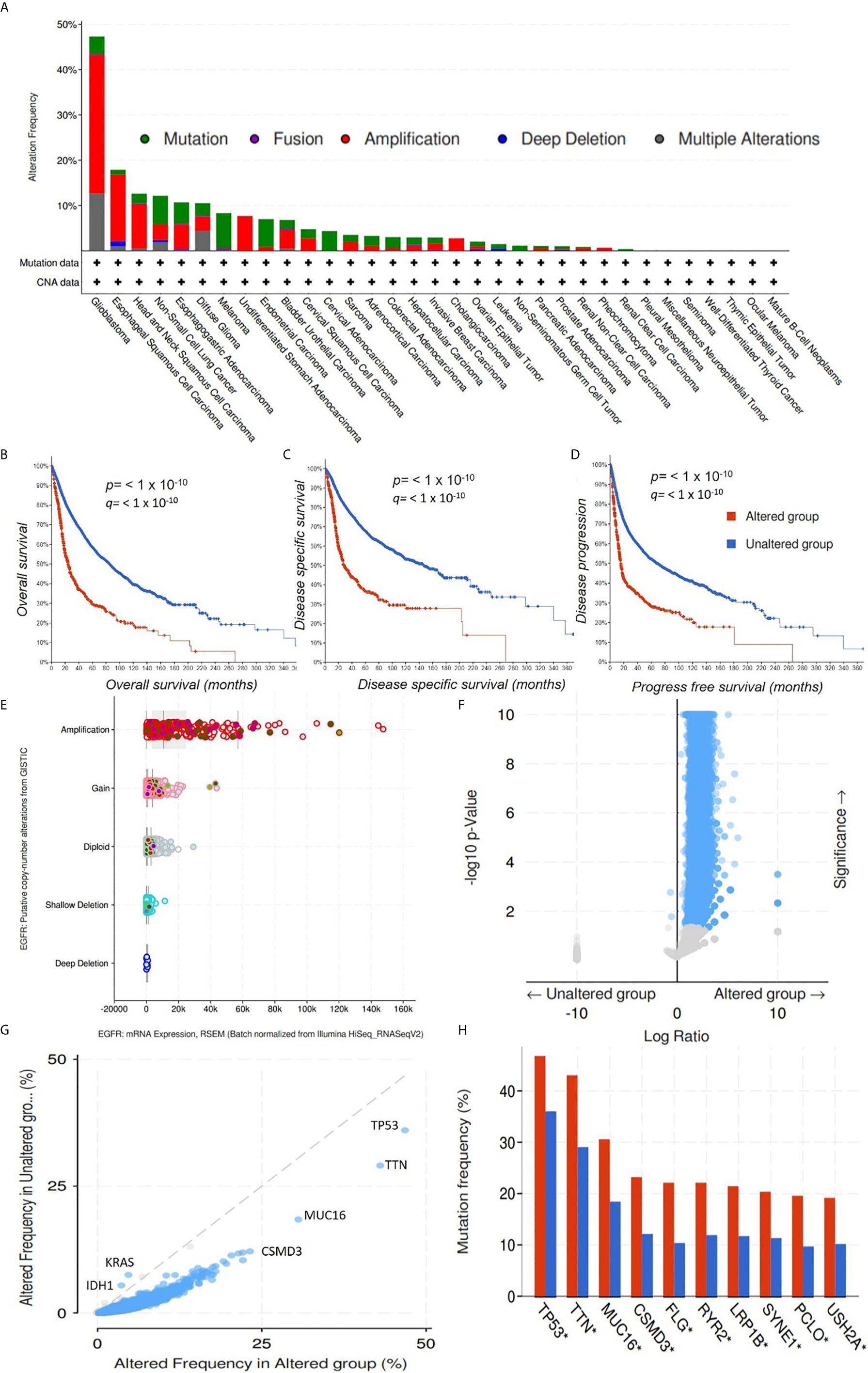
Figure 6 Genomic alterations in the epidermal growth factor receptor (EGFR) are associated with poor prognoses of cancer cohorts. (A) Bar graph showing alteration frequencies of the EGFR in cohorts of different cancer types. The most common alteration in the EGFR gene was amplification. Kaplan-Meir survival curve of (B) overall survival, (C) disease-specific survival, and (D) disease-progression survival of cancer cohorts with altered EGFR and unaltered EGFR genes. Cohorts with altered EGFR had low overall survival, disease-specific survival, and disease-progression survival. p < 10−10. (E). Bar showing the EGFR putative copy number alterations and messenger RNA expressions. (F) Heat map showing p values and significance levels of other gene mutations associated with cohorts with altered EGFR and cohorts with unaltered EGFR. (G) Line graph showing frequencies of altered genes in cancer cohorts with altered EGFR and cohorts with unaltered EGFR. (H) Bar graph showing gene mutations that were significantly enriched in both EGFR-altered and EGFR-unaltered cohorts.
Molecular Docking Revealed Unique Interactions of NSC765598 With EGFR, mTOR, NOS2, TGFB1, MAP2K1, and FGFR1
In order to evaluate the strength and nature of interactions between NSC765598 and the selected mapped pharmacophores, NSC765598 was docked into the active sites of EGFR, mTOR, NOS2, TGFB1, MAP2K1, and FGFR1.
Molecular Docking of NSC765598 With mTOR
NSC765598 demonstrated a –8.8 kcal/mol binding affinity for mTOR. The NSC765598- mTOR complex was bonded by conventional H-bonds with GLN1937, VAL2227, and ARG2224, C-H bonding with GLN2200, halogen bonding with GLN1937 and VAL2227, and multiple π-interactions. NSC765598 also forms 4 hydrophobic contacts with LEU1936, GLN1937, and GLN2200 residues of mTOR. Stabilization of NSC765598– mTOR complex was also supported by the Van der Waal forces between the ligand and ASP1933, PRO21146, ALA2226, GLU2196, MET2199, PRO1940, GLY2203, LEU2204, LEU1900, and ASN1899 residues of the receptor binding pocket (Figure 7A). However, a standard mTOR inhibitor, dactolisib exhibited a higher affinity for mTOR (−9.2 kcal/mol) than NSC765598 (Table 3).
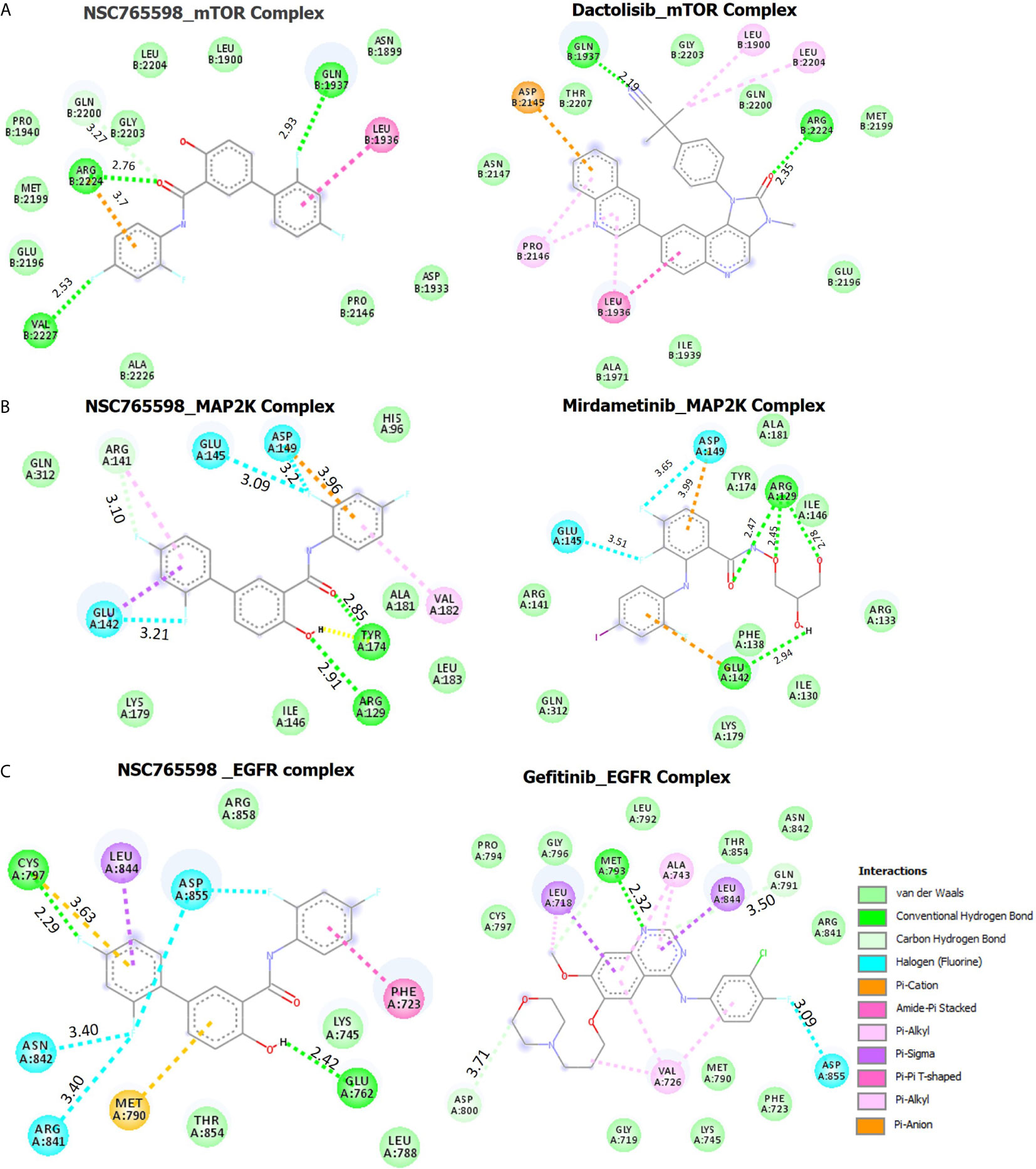
Figure 7 Docking profiles of mTOR/MAP2K/EGFR with NSC765598 and standard inhibitors. The two dimensional (2D) representations of ligand–receptor complexes, showing the interacting amino acid residues and the type of interactions, occurring between the ligands and (A) mTOR (B) MAP2K and (C) EGFR.
Molecular Docking of NSC765598 With MAP2K
Molecular docking studies revealed that NSC765598 docked well into the MAP2K binding cavity with more robust interactions, stronger binding affinity (−7.6 kcal/mol), and shorter interaction distances (2.85–3.96 Å) than the interaction observed between MAP2K and a standard inhibitor, mirdamatinib (−6.5 kcal/mol, 2.78–4.59 Ă). There NSC765598-MAP2K complex was bonded by conventional H-bonds with Arg129 and Tyr174, a carbon-hydrogen bond with Arg141, halogen bonding with Glu145, Glu142, and Asp149, and multiple π-interactions, including π-anion interaction with Asp149, π-sigma interaction with Glu142, and π-alkylation with Arg141. NSC765598 also forms 4 hydrophobic contacts with ARG141, GLU142 and GLU145 residue of MAP2K. Stabilization of the NSC765598-MAP2K complex was also supported by Van der Waal forces between the ligand and the amino acid residues (Gen312, Lys179, Ile146, Leu183, Ala181, and His96) in the receptor binding pocket (Figure 7B, Table 3).
Molecular Docking of NSC765598 With EGFR
Molecular docking of NSC765598 with EGFR revealed more robust interactions and higher binding affinity (−11 kcal/mol) to EGFR than do gefitinib (−6.8 kcal/mol) a standard EGFR inhibitor. The NSC765598-EGFR complex was bonded by strong H-bond interactions with GLU762 and CYS797, halogen bond interactions with ASN842, ARG841, and ASP855, and multiple π-interactions, including π-π interaction with PHE723, π-alkyl interaction with LEU844, and π-sulfur interactions with MET790 and CYS797 (Figure 7C). In terms of interaction distances between the ligand atoms and receptor atoms, the hydrogen bond interactions of GLU762 (2.42 Å) and CYS797 (2.29 Å) were the shortest, while π-interactions of LEU844 (5.49 Å), CYS797 (3.63 Å), and MET790 (5.43 Å) had longer distance interactions within the NSC765598-EGFR complex. NSC765598 also forms 5 hydrophobic contacts with PHE723 and LEU844 residues of EGFR. Stabilization of the NSC765598-EGFR complex was also supported by Van der Waal forces between the ligand and ARG858, LYS745, LEU788, and THR854 in receptor binding pockets (Figure 7C, Table 3).
Molecular Docking of NSC765598 With FGFR1
NSC765598 docked well with the binding pocket of FGFR1 with a binding affinity of −7.3 kcal/mol stronger than the interactions between FGFR and Erdafitinib (−5.7 Kcal/mol). The NSC765598-FGFR1 complex was stabilized by two H-bonds with SER119 (2.13 Å) and VAL121 (3.33 Å) as opposed to only single H-bond interaction (ARG134) between FGFR_Erdafitinib. In addition, halogen interactions (PRO120, 2.54 Ă and PRO149, 3.05 Ă), π-π stacking with the aromatic ring of PHE129, and Amide-Pi stacking with SER119 were also observed between the NSC765598_FGFR1 complex. NSC765598-FGFR1 complex was also supported by several Van der Waal forces created around the backbone of the ligand with respective amino acid residues (LYS81, GLU117, ARG134, PHE136, PHE151, PHE150, and SER127) of the receptor binding pocket (Figure 8A, Table 4).
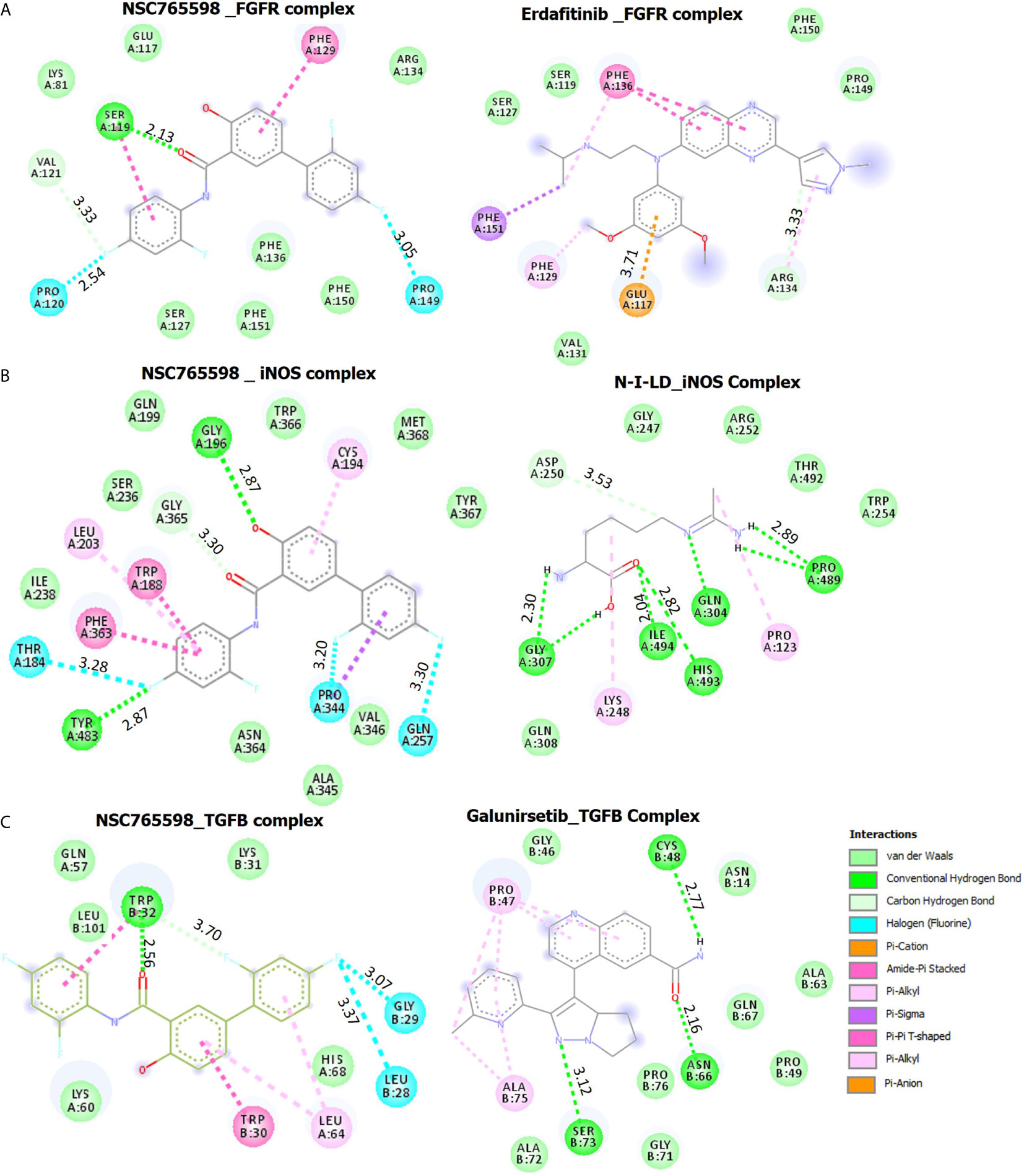
Figure 8 Docking profiles of iNOS/FGFR/TGFB with NSC765598 and standard inhibitors. The two-dimensional (2D) representations of ligand–receptor complexes, showing the interacting amino acid residues and the type of interactions, occurring between the ligands and (A) iNOS (B) FGFR and (C) TGFB.
Molecular Docking of NSC765598 With iNOS
Molecular docking of NSC765598 with iNOS revealed a binding affinity of −11.0 kcal/mol. NSC765598 interacts with iNOS by three H-bonds with TYR483, GLY196, and GLY363, and halogen interactions with GLN257, PRO344, and THR184. Binding interaction proximities in the NSC765598-iNOS complex ranged 2.65–3.28 Å; the shortest distance interactions were conventional H-bonds with TYR483 (2.65 Å) and GLY196 (2.87 Å). The NSC765598-iNOS complex was also supported by π-interactions; π-π stacking with aromatic rings of TRP188 and PHE363, and π-alkyl interactions with CYS194, LEU203, and PRO344. The interaction was also stabilized by Van der Waal forces created on the backbone with the following amino acids: ILE238, SER236, GLN199, TRP366, MET368, TYR367, ALA345, VAL346, and ASN364 (Figure 8B, Table 4). Furthermore, NSC765598 interaction with iNOS is more robust and with higher affinity than the interactions between iNOS and N-Iminoethyl-L-lysine dihydrochloride (−5.3 Kcal/mol), a standard iNOS inhibitor (Table 4). In addition, NSC765598 forms 4 hydrophobic contact with TRP188, PRO344, and PHE363 residue of iNOS as compared to 1 hydrophobic contact in the iNOS_N-Iminoethyl-L-lysine dihydrochloride complex
Molecular Docking of NSC765598 With TGF-β1
NSC765598 docked well to the binding cavity of TGF-β1 with an affinity of −7.2 kcal/mol comparable with the affinity that galunisertib (a standard TGF-β1 inhibitor) has for TGF-β1 (−7.1 kcal/mol). NSC765598 binds with TGF-β1 by single H-bond (TRY32) as against three (3) H-bonds (CYS48, ASN66, SER73) between galunisertib_TGF-β1 complex. In addition to the halogen interactions (GLY29 and LEU28), π-stacking with the aromatic ring of TRP30, and π-alkyl interaction with LEU64 residues of TGF-β1, NSC765598-TGF-β1 complex was also supported by Van der Waal forces created between the ligand backbone and amino acid residues (LEU101, GLN57, LYS31, and HIS68) of TGF-β1 binding pocket (Figure 8C, Table 4). In addition, NSC765598 forms 3 hydrophobic contact with TRP32 and LEU64 residues of TGFB as compared to 1 hydrophobic contact (PRO47) in the TGF-β1_ galunisertib complex.
Anti-Proliferative Activities of NSC765598 Against NCI60 Panels of Human Tumor Cell Lines
The percent growth inhibition (GI) caused by single-dose testing revealed that NSC765598 inhibited the growth of all of the NCI60 cell line panels of breast, prostate, renal, ovarian, colon, melanoma, CNS, leukemia, and non-small cell lung cancers. Single-dose (10 μM) treatment with NSC765598 exhibited more than 50% growth inhibition of all the NCI60 cell line panels except for two colon cancer cell lines (HT29 and COLO 205) which were completely insensitive to NSC765598 treatment (Figure 9). Furthermore, single-dose treatment with NSC765598 also demonstrated cytotoxic activities against leukemia cell lines of HL-60 (cell growth (CG) = −31.31%) and RPMI-8226 (CG = −26.88%), NSCLC cell lines of HOP-92 (CG = −29.23%) and NSC-H522 (CG = −4.38%), melanoma cell lines of MALME-3M (GI = −12.11%), SK-MEL-2 (CG = −19.27%), SK-MEL-5 (CG = −50.91%), and UACC-257 (CG = −29.80%), renal cell line of A498 (CG = −30.67%), prostate cell line of PC-3 (CG = −9.28%), and breast cancer cell line of MDA-MB-231/ATCC (CG = −24.07%). However, no cytotoxic activities were detected against the ovarian, colon, or CNS cancer cell lines (Figure 9). The primary single-dose results clearly indicated the anticancer activities of NSC765598 against different kinds of human cancer cell lines, and thus it is worthy of further evaluation for dose-dependent activities.
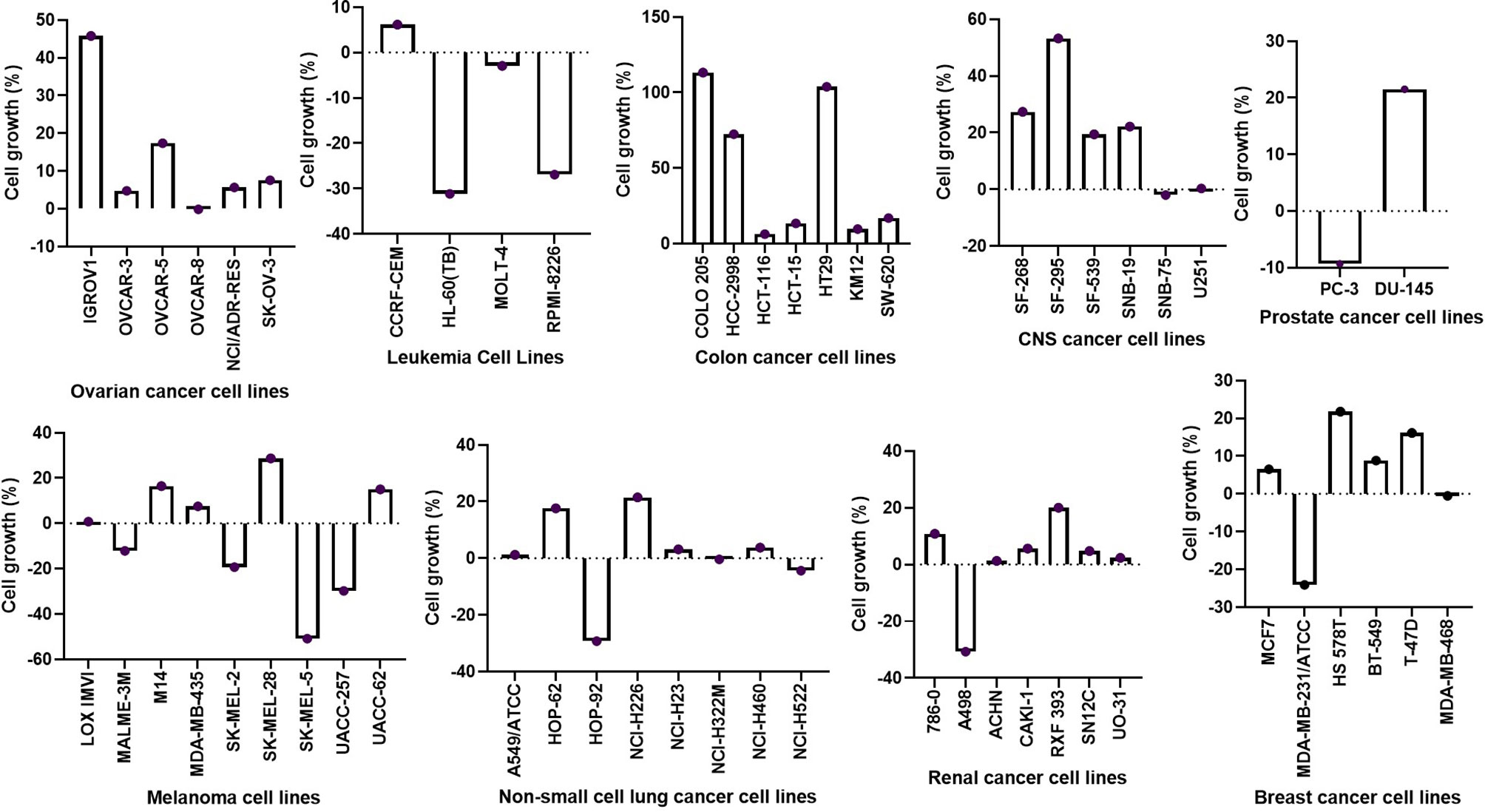
Figure 9 Inhibitory activities of NSC765598 against panels of 60 human cancer cell lines. Each cell line was treated with a single dose of 10 μM of NSC765598 for 48 h. The bar points above point zero on the y-axis indicate the mean percentage of cell growth relative to cells without drug treatment, while bar points below point zero indicate the percentage of cell death.
NSC765598 Exhibited Selective Cytotoxic Preferences for NSCLC, Leukemia, Melanoma, and Renal Cancer Cell Lines
In the five-dose assay screening, NSC765598 demonstrated selective dose-dependent cytotoxic effects against the panel of NCI60 human tumor cell lines (Figure 10). The GI50 values of NSC765598 (concentration of NSC765598 that causes 50% inhibition of cell growth) against individual cells of the NCI60 panels of human cell lines tested were lower than 5.0 µM, except for COLO 205, a colon cancer cell line with a GI50 of 16.0 µM (Figure 11). However, among the nine types of cancer involved in this assay, panels of NSCLC cell lines were the most responsive to growth inhibition by NSC765598 treatment (GI50 = 1.12–3.95 µM) followed by leukemia cell lines (GI50 = 1.20–3.10 µM), melanoma cell lines (GI50 = 1.45–3.59 µM), and renal cell lines (GI50 = 1.38–3.40 µM). Furthermore, NSC765598, displayed the least TGI (concentration causing 100% growth inhibition of cancer cells) against six panels of NSCLC cell lines (TGI =3.72–16.60 μM), six panels of leukemia cell lines (TGI = 3.90–12.70 μM), and eight panels of renal cancer cell lines (TGI = 4.84–13.70 μM), while the highest TGI (11.80 to > 100 μM) and perhaps the lowest activity were recorded for the seven panels of colon cancer cell lines. Furthermore, as revealed by the LC50 (concentration causing 50% lethality of cancer cells), cytotoxic activities of NSC765598 were more pronounced against NSCLC cell lines of HOP-92 (LC50 = 18.90 μM) and HOP-62 (LC50 = 92.0 μM), leukemia cell lines of HL-60 (LC50 = 20.30 μM), RPMI-8226 (LC50 = 29.50 μM), and MOLT-4 (LC50 = 71.10 μM), melanoma cell lines of SK-MEL-5 (LC50 = 7.68 μM) and SK-MEL-2 (LC50 = 23.50 μM), brain cancer cell lines of U251 (LC50 = 40.0 μM) and SNB-75 (LC50 = 65.70 μM), and renal cancer cell lines of RXF 393 (LC50 = 41.60 μM), 786-0 (LC50 = 67.0 μM), A498 (LC50 = 78.70 μM), and TK-10 (LC50 = 84.20 μM), while the two panels of prostate cancer cell lines (PC-3 and DU-145) were less responsive to the cytotoxic effects of NSC765598 (LC50 > 100 μM). However, contrary to the primary one-dose cell growth percent inhibition assay, the colon cancer cell line, COLO 205 was found to be the most sensitive to five-dose testing of NSC765598 than the other colon cancer cell lines, displaying total growth inhibition and 50% cytotoxic response at NSC765598 concentrations of 35.80 and 80.30 μM, respectively (Figure 11, Table 5).
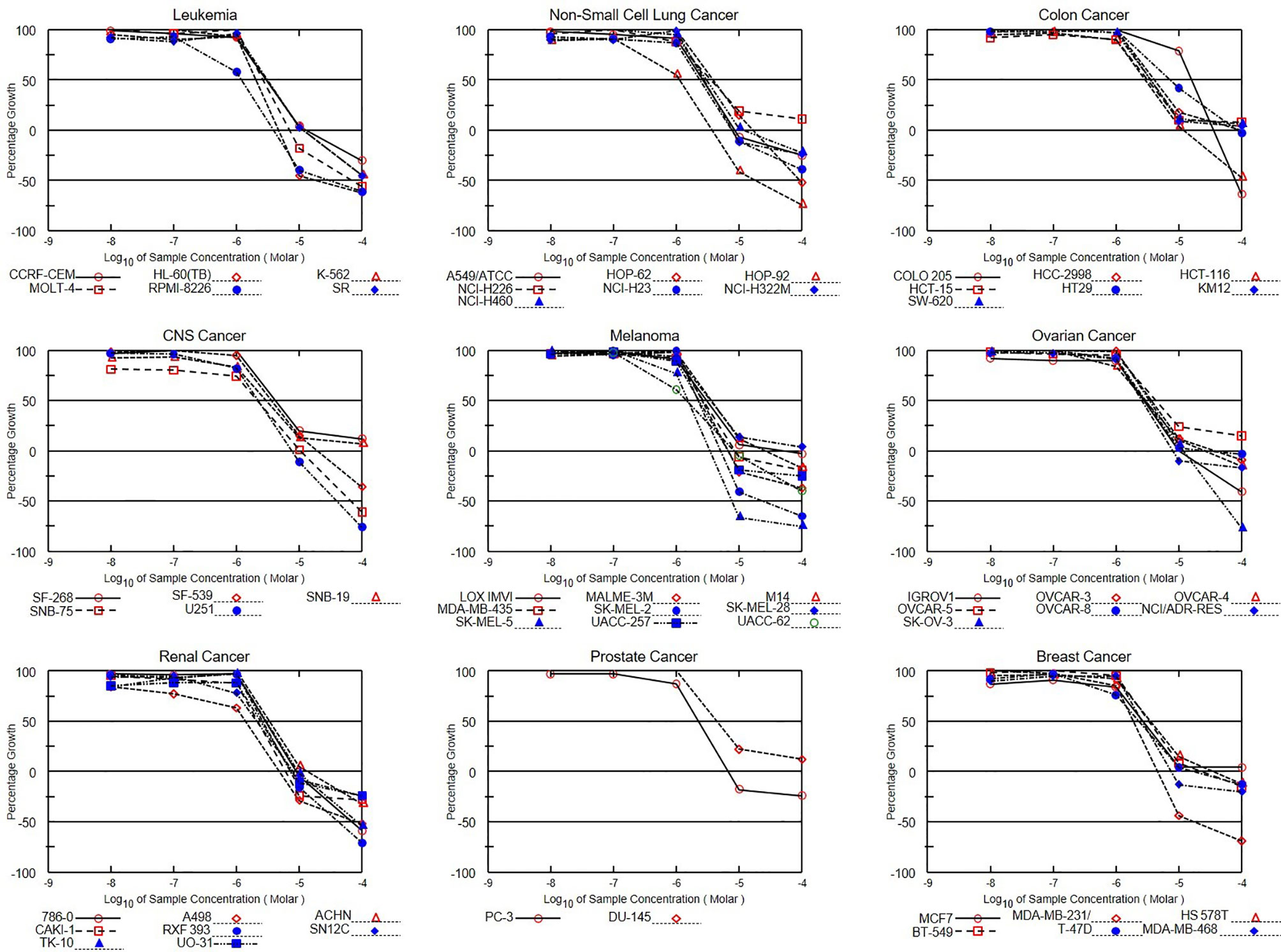
Figure 10 Dose-response curves of NCI60 human cancer cell lines to NSC765598 treatment. Growth percentage value of 100 on the y-axis represents the growth of untreated cells, the 0 value represents no net growth, while −100 represents complete death of cells.
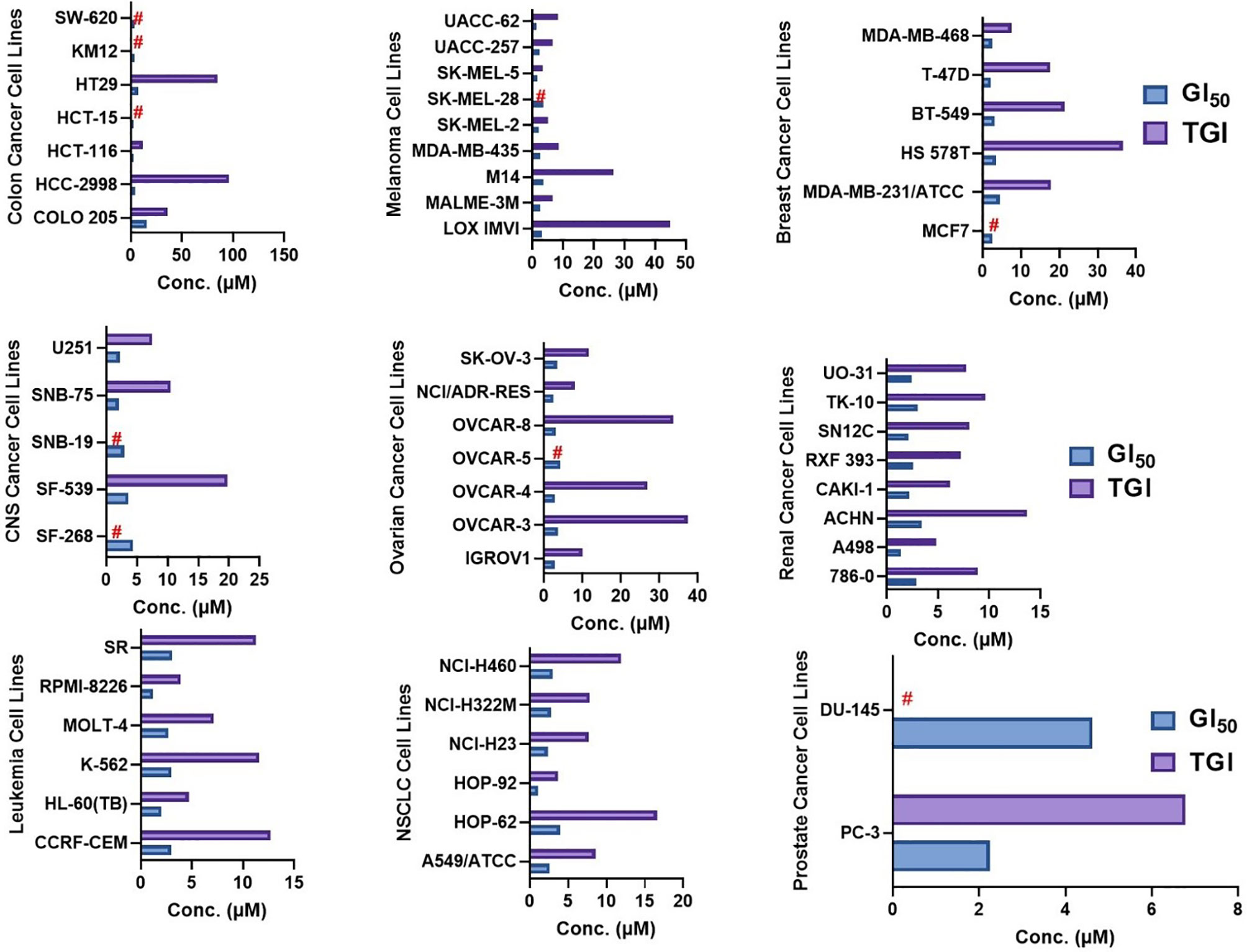
Figure 11 GI50 (50% inhibition of the cancer cell growth) and TGI (100% growth inhibition of the cancer cells) concentrations of NSC765598 against NCI60 panels of human cancer cell lines.
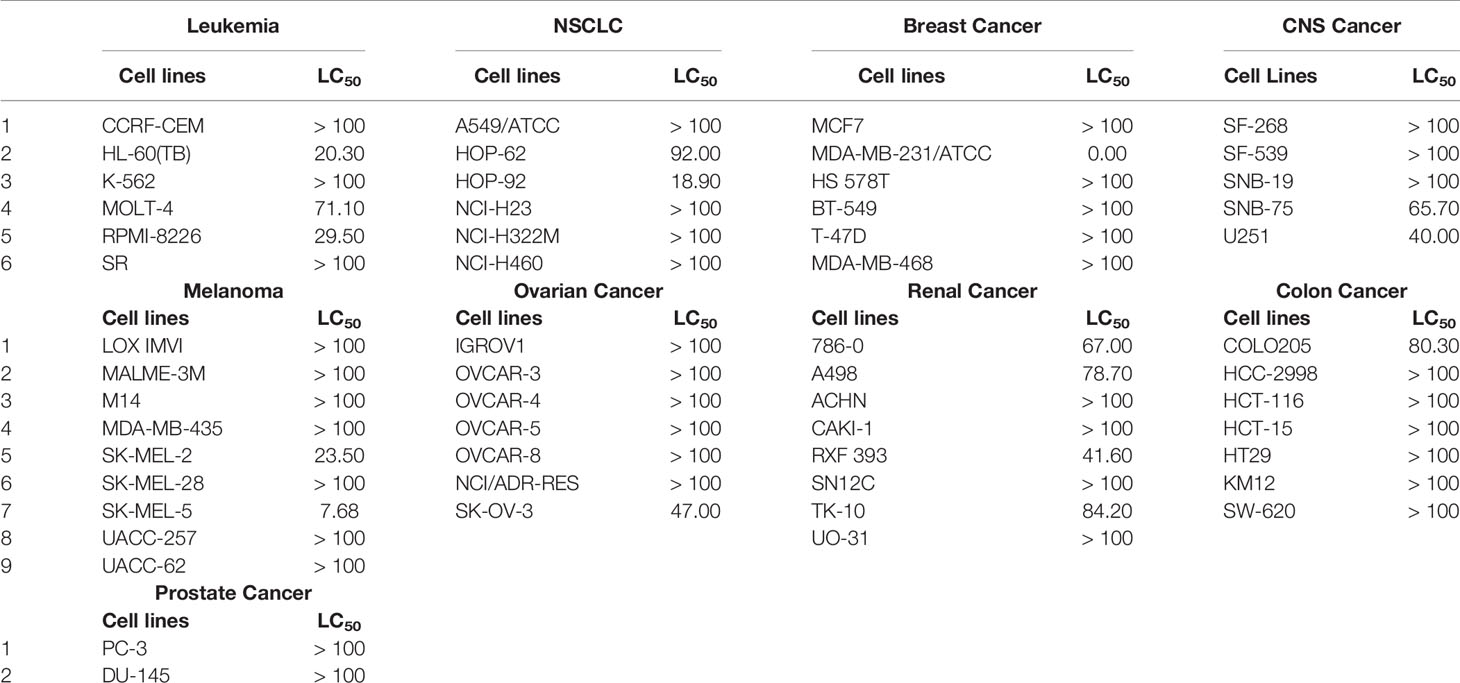
Table 5 LC50 (50% lethality of the cancer cells) concentrations of NSC765598 against NCI60 panels of human cancer cell lines.
NSC765598 Share a Similar Antitumor Fingerprint With NCI Synthetic Compounds and Standard Agents
DTP-COMPARE analysis indicated that NSC765598 share similar antitumor fingerprints with a number of NCI standard agents. The p-value and cell counts of the top 15 NCI correlated standard agents are shown in Table 6. All NCI synthetic compounds with NSC765598 similar antitumor fingerprints are small molecules (MW; 277.3–461.9 g/mol). The identity, p-value, cell counts, and molecular weight of the top 15 NCI synthetic compounds are also shown in Table 6.
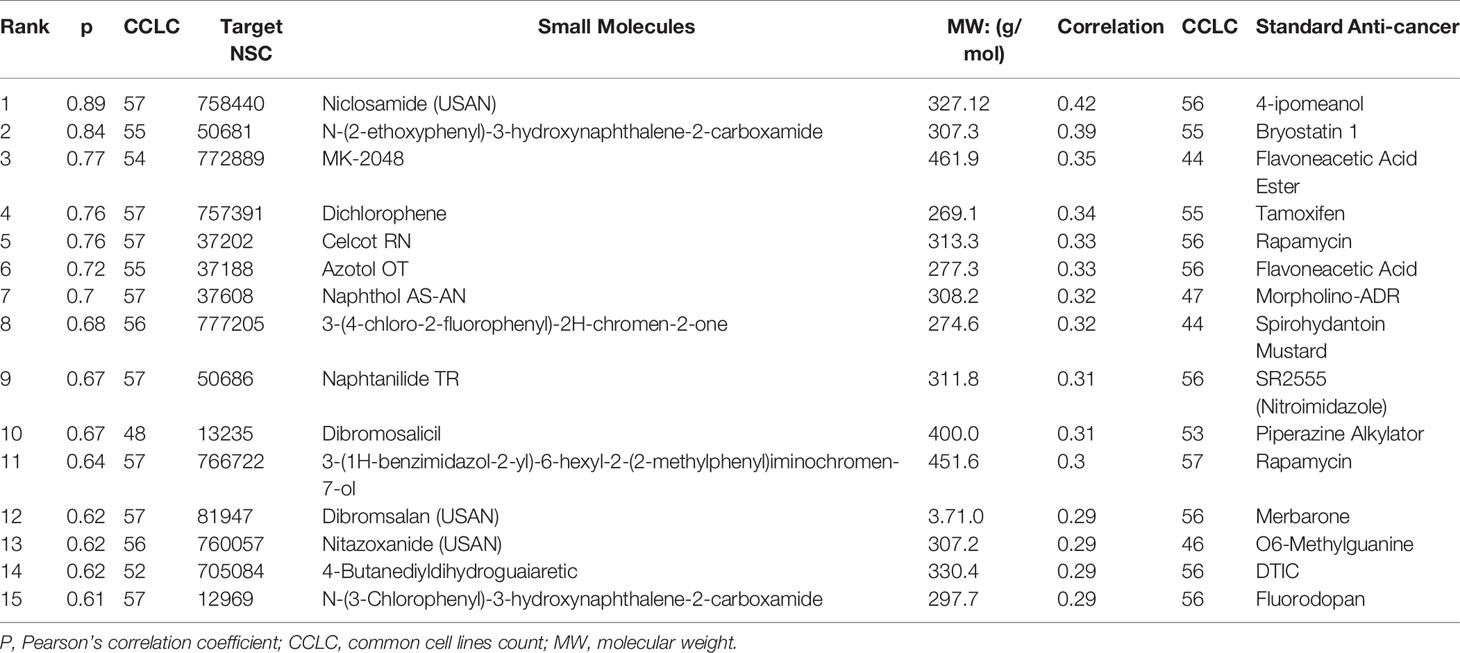
Table 6 NCI synthetic compounds and standard anticancer agent sharing similar anti-cancer fingerprints and mechanistic correlation with NSC765598.
Discussion
The application of multi-omics approaches has aided our understanding of carcinogenesis and the development of therapeutic strategies (44). Identifying drug targets for small molecules and their molecular mechanisms play important roles in drug design, discovery, and development (45). Drug targets can be identified via genetic interactions, biochemical methods, and computational studies (46). In this study, our collective computational approach identified EGFR/mTOR/NOS2/TGFB1/FGFR1/MAP2K1 as potential targets for NSC765598. The PPI network revealed that these gene targets interact strongly with other oncogenic proteins and were mainly enriched in pathways and processes associated with multiple cancers. In accordance with our observations, previous studies had identified these gene signatures as cancer-associated genes. For instance, Murugan et al. (47) reported that mTOR promotes cancer growth, drug resistance, and metastasis, while McCubrey et al. (48) reported that the EGFR/mTOR signaling pathways play prominent roles in malignant transformation, therapeutic resistance, cancer stemness, metastasis, and inhibition of apoptosis. MAP2K was implicated in hypoxia-mediated angiogenesis, cancer progression, metastasis, and drug resistance (49). Increasing evidence also implicated EGFR signaling pathways in promoting cancer growth and metastasis (50, 51). Taking together, these results strongly suggest the oncogenic roles of EGFR/mTOR/NOS2/TGFB1/FGFR1/MAP2K1 signatures in multiple cancers. Hence, we explored public databases to investigate the full prognostic relevance of these target genes in multiple cancers.
Comparative gene expression profiling of cancer and adjacent normal tissues facilitates an understanding of disease etiologies and enhances the design of appropriate therapeutic interventions (16). Our differential expression analysis revealed that mRNAs of EGFR, mTOR, NOS2, TGFB1, FGFR1, and MAP2K1 were overexpressed in multiple cancer types compared to corresponding adjacent healthy cohorts, while the survival analysis revealed that cohorts with higher expressions of EGFR, MAP2K1, and NOS2 exhibited shorter OS than cohorts with low expression profiles. Collectively, these data suggested that EGFR/mTOR/NOS2/TGFB1/FGFR1/MAP2K1 play important roles in the progression of tumors and serve as reliable biomarkers of poor prognosis
Using the cBioPortal, we also studied frequencies of genetic alterations of these signatures and their prognostic values in cancer patients. We found that genetic alterations in EGFR occurred in 7% of cancer cohorts, most frequently in GBM, ESCC, HNSCC, and NSCLC, and were associated with poor prognoses and survival of patients. Dong et al. (52) evaluated the alteration status of the top three genes identified as prognosticators of renal cell carcinoma and respectively found only 2%, 2%, and 1.7% genetic alterations in the FN1, COL1A2, and COL3A1 genes. Chen et al. (53) studied genetic alterations of 20 genes and found alteration frequencies ranging 0.8% to 3% for 19 individual genes, while only one gene (NBN) had an alteration frequency of 9%. Therefore, percentages of patients with genetic alterations in EGFR (7%), FGFR (6%), mTOR (4%), and iNOS (3%) as reported in this study were on the high side and thus strengthen our earlier observations that these signatures are very important in cancer progression and can thus serve as attractive targets worthy of further exploration.
Nevertheless, it is becoming increasingly evident that the initiation and progression of cancer cannot be ascribed to a single genetic mutation (54). Therefore, we evaluated the co-occurrence of genetically altered EGFR with other genetic mutations in the cancer cohorts, and we found its co-occurrence with genetically altered RICTOR, UGGT1, ATRNL1, AHNAK2, RIMS2, DSP, STXBP5L, DUOX2, PKD1L1, and MYH3. Alterations in these genes were found to be positively enriched in patients with altered EGFR, while alterations in KRAS and IDH1 were only enriched in patients without genetically altered EGFR. Intriguingly, we also observed that the most common genetic alterations in the EGFR gene were amplifications and gene gains, supporting the role of EGFR as an oncoprotein. These findings are also coherent with our earlier observations on the differential expression of EGFR mRNA in cancer cohorts compared to healthy cohorts. However, contrary to our earlier observations on the poor survival rates of cohorts with high mRNA expressions of EGFR, mTOR, FGFR iNOS, TGFB1, and MAP2K1, only genetic alterations of EGFR, but not those of mTOR, iNOS, TGFB1, or MAP2K1, were associated with poor prognoses and survival of patients.
Lung cancer is the most commonly diagnosed (often diagnosed in advanced stages) and the leading cause of global cancer mortality due to limited therapeutic success (3, 55). It is therefore plausible to say that genetic alterations in EGFR are a major contributor to this dilemma. In line with our observations, Ding et al (56). reported that the EGFR pathway is the most altered pathway in lung adenocarcinomas (26%). Gower et al (57). identified that alterations in EGFR are major oncogenic drivers of acquired resistance and predict poor survival in NSCLC, while Saadeh et al (58). reported high correlations of EGFR mutations with poor therapeutic responses and survival of glioblastoma patients. Although, treatments with EGFR tyrosine kinase inhibitors (TKIs) showed initial therapeutic responses, acquired resistance to 1st-, 2nd-, and recently approved 3rd-generation TKIs (osimertinib) owing to EGFR mutations has been well reported (59, 60). Taken together, patients with high EGFR mRNA expression are more likely to harbor genetically altered EGFR and experience worse prognoses. This necessitates alternative treatment strategies; hence, we evaluated NSC765598 for that purpose.
In order to facilitate the identification of potent anticancer small molecules from synthetic libraries, the National Cancer Institute (NCI) established the NCI60 cell panel screen to examine the activities of small molecules on cancer cell viability (61). Hence, we explored this avenue to evaluate the anticancer properties of NSC765598 against the NCI60 panel of cell lines. Interestingly we found that NSC765598 exhibited antiproliferative effects on all panels of breast cancer, prostate cancer, renal cancer, ovarian cancer, melanoma, CNS cancer, leukemia, and NSCLC cells with GI50 values of < 5 µM, but it showed lower anti-proliferative activities against colon cancer cell lines. Among the nine types of cancer involved in this assay, NSC765598 had some cytotoxic preference for NSCLC, leukemia, melanoma, and renal cancer cell lines. Interestingly, NSC765598 also exhibited activity against a drug-resistant cell line, NCI/ADR-RES (GI50 = 2.54 µM; TGI = 8.00 μM). However, the least amounts of cell lethality were found against panels of colon, breast, ovarian, prostate, and CNS cancer cell lines (TGI > 20 µM in most cases and LC50 >100 µM in almost all cases), suggesting that NSC765598 is not generally toxic to growing cell lines, but displays some degree of specificity to NSCLC, renal cancer, leukemia, and melanomas. The NCI60 cell lines used in this study have been well characterized for proteins, genes, microRNA expressions, mutations, and DNA methylation (62–64). Mutations in the EGFR TK domain have been reported in NSCLC and have been associated with response to gefitinib and erlotinib (65, 66). In addition, among the NCI60 cell lines, mutations in the EGFR gene have been identified in the leukemia cell line (RPMI-8226) and melanoma cell line (SK-MEL-28) and were found to be associated with the resistant of these cell lines to 12 tyrosine kinase inhibitors (TKI) including the erlotinib (67, 68). It is, therefore, noteworthy that NSC765598 demonstrated high activity on these cells line (Figure 11, Table 5).
COMPARE analysis indicated that NSC765598 shares a similar antitumor fingerprint with a number of NCI standard agents and a very strong correlation with NCI synthetic compounds (p-value 0.61~0.89). Importantly, the top most correlated synthetic compounds, niclosamide (p-value 0.89) is a known anticancer agent, which have been mechanistically reported to exhibit anticancer activities via suppression of Wnt/β-catenin, mTORC1, EGF, STAT3, NF-κB, and Notch signaling pathways (69, 70), while nitazoxanide (p-value 0.62) exhibit it anticancer activities via suppression of MAPK and mTOR pathways (71). The strong correlation of these compounds with the NSC765598 anticancer fingerprint strongly suggests a similar mechanism of action. Hence, it is very likely that anticancer activities demonstrated by NSC765598 could be attributed to suppression of EGF, MAPK, and mTOR signaling pathways, which is in line with the in silico target prediction and molecular docking. Collectively, this study has provided in vitro and in silico evidence for anticancer activities of NSC765598 and inhibition of EGFR, mTOR, NOS2, TGFB1, MAP2K1, and FGFR1 as the most probable mechanism of action. Hence we validated this hypothesis using molecular docking of ligand-protein interactions.
Molecular docking of a drug candidate with target proteins is very useful for identifying the strength of an association between a ligand and receptor and ultimately gives a prediction of the activity of the small molecule (18). We conducted a molecular docking study to elucidate likely binding affinities and binding interactions of NSC765598 with selected targets. We found that NSC765598 docked well into the binding cavity of EGFR, mTOR, NOS2, TGFB1, FGFR1, and MAP2K1 with binding affinities ranging −7.2 to −11.0 kcal/mol. Hydrogen bonds together with other non-covalent interactions, such as hydrophobic and ionic interactions and van der Waals forces, play important roles in stabilizing protein-ligand interactions (72). Interestingly, interactions of all targets with NSC765598 predominantly involved conventional hydrogen bonds, fluorine bonds, hydrophobic contacts, van der Waal forces, and a variety of π-interactions including π-π stacking, and π-anion and π-cation interactions, which consequently led to high binding affinities and strong ligand-NSC765598 complexes, and likely compromised the expression integrity of the proteins.
Among the targets, EGFR and iNOS demonstrated unique interactions with a higher binding affinity of −11 kcal/mol compared to other targets, thereby suggesting higher promising inhibitory effects of NSC765598 against EGFR and iNOS. The high binding affinities and unique stability of NSC765598 in binding pockets of iNOS and EGFR were chiefly attributed to three conventional H-bonds with TYR483, GLY196, and GLY363 of iNOS and two conventional H-bonds with GLU762 and CYS797 of EGFR; halogen interactions with GLN257, PRO344, and THR184 of iNOS, and ASN842, ARG841, and ASP855 of EGFR; and multiple hydrophobic and Pi interactions (π-stacking, π-alkyl, and π-sulfur). The large numbers of π-interactions, which mostly involve charge transfer, helped NSC765598 intercalate in binding cavities of the receptors. Furthermore, higher Van der Waal forces created on the NSC765598 backbone with the respective amino acids of ILE238, SER236, GLN199, TRP366, MET368, TYR367, ALA345, VAL346, and ASN364 in the binding pocket of iNOS; and ARG858, LYS745, LEU788, and THR854 in the binding pocket of EGFR created strong cohesive environments, thereby stabilizing the complexes formed (73).
The critical role of amino acids in docking has been well demonstrated (74). Alkylation of proteins is dependent on the biochemical properties of target amino acids to create nucleophilic sites (75). Therefore, the specific alkylation of the LEU844 residue of EGFR and alkylation of the three CYS194, LEU203, and PRO344 residues of iNOS by NSC765598 could mediate the modification of substrate binding sites and subsequently induce alkylation-dependent inhibition of target proteins (76). Collectively, the strong interactions observed between NSC765598 and EGFR/iNOS may compromise their activity/expression abilities and consequently affect the survival of cells that depend on these proteins for their vital activities. These findings, therefore, suggest that the anticancer activities demonstrated by NSC765598 could be attributed more to its dual inhibition of iNOS and EGFR. Specifically, the docking results corroborated our observations of NSCLC cell lines, which were observed to demonstrate higher responses to NSC765598 (GI50 = 1.12–3.95 µM; TGI = 3.72–16.60 μM). Therefore, stronger NSC765598 interactions with EGFR could be implicated in the higher activity of NSC765598 on NSCLC panels. Interestingly, NSC765598 demonstrated more robust interactions and higher binding affinities for MAP2K, EGFR, iNOS, and FGFR1 than does their respective standard drugs; mirdametinib, gefitinib, N-Iminoethyl-L-lysine dihydrochloride, and erdafitinib. However, it shows, lower binding affinity for mTOR than dactolisib, and its comparable to galunisertib in its affinity for TGFB1. Currently, there are no iNOS inhibitors approved for human use because promising results in animal studies have not translated to humans (77). Therefore, the results of the present study indicate that NSC765598 could serve as a novel iNOS inhibitor worthy of further preclinical and clinical developments.
Hydrophobic interactions play important role in the formation of ligand-receptor complexes. The number of contacts between hydrophobic atoms reflects the extent of the hydrophobic interactions between a ligand and receptor complex. NSC765598 forms several hydrophobic contacts with amino acid residues of the mTOR/EGFR/MAP2K/iNOS/FGFR/TGFB1 (Tables 3 and 4). These hydrophobic interactions are key players in stabilizing the energetically-favored ligands, in an open conformational environment of the proteins (78). It’s noteworthy that NSC765598 forms higher hydrophobic contacts with the receptors than do some of the standard inhibitors used for comparison in this study (Table 4). This higher number of hydrophobic contacts in the active core of the drug -target interface would further increase the biological activity of the drug lead. Collectively, this study suggested that NSC765598 has a potential for multi-target inhibition of EGFR/iNOS/mTOR/TGFB1/FGFR/MAP2K1 and could serve as a lead compound for developing new therapeutics for cancer treatment. Preclinical toxicity study is an important aspect of drug discovery and developments (79). Our computational study of toxicity indicated that NSC765598 displayed LD50 at high concentration and, thus may be safely used for acute administration, while the drug-likeness studies indicated that it met acceptable criteria of being used as a drug for therapeutic applications. Further in vitro and in vivo studies in tumor-bearing mice are ongoing to evaluate the full therapeutic efficacy of this novel small molecule.
Conclusion
The current study identified mTOR/EGFR/iNOS/MAP2K1/FGFR/TGFB1 as potential targets of NSC765598 with higher binding preferences for EGFR, iNOS, and mTOR. NSC765598 displayed anti-proliferative activities and selective cytotoxic preferences for NSCLC, leukemia, melanoma, and renal cancer cell lines. In addition, NSC765598 displayed favorable properties as a drug lead compound and thus could be considered a novel small molecule with potential for multi-target inhibition of EGFR/iNOS/mTOR/TGFB1/FGFR/MAP2K1 and could serve as a lead compound for developing new therapeutics for cancer treatment.
Data Availability Statement
The original contributions presented in the study are included in the article/Supplementary Material. Further inquiries can be directed to the corresponding authors.
Author Contributions
BL wrote the manuscript. C-YL, NM, and HK helped with data collection and analyses. H-SH and MS synthesized and provided NSC765598. H-SH and AW designed and oversaw the study. All authors contributed to the article and approved the submitted version.
Funding
H-SH is funded by the Ministry of Science and Technology (MOST109-2113-M-038-003). C-YL is funded by the Ministry of Science and Technology (MOST 109-2314-B-038-108).
Conflict of Interest
The authors declare that the research was conducted in the absence of any commercial or financial relationships that could be construed as a potential conflict of interest.
Acknowledgments
The NCI Developmental Therapeutics Program (DTP) for the 60-cancer-cell-line screening of selected compounds described in this paper, funded by the National Cancer Institute, National Institutes of Health (NIH-NCI).
Supplementary Material
The Supplementary Material for this article can be found online at: https://www.frontiersin.org/articles/10.3389/fonc.2021.656738/full#supplementary-material
References
1. Mathers CD. History of global burden of disease assessment at the World HealthOrganization. Arch Public Health (2020) 78:1–13. doi: 10.1186/s13690-020-00458-3
2. Sung H, Ferlay J, Siegel RL, Laversanne M, Soerjomataram I, Jemal A, et al. Global cancer statistics 2020: GLOBOCAN estimates of incidence and mortality worldwide for 36 cancers in 185 countries. CA Cancer J Clin (2021). doi: 10.3322/caac.21660
3. Bray F, Ferlay J, Soerjomataram I, Siegel RL, Torre LA, Jemal A. Global cancer statistics 2018: GLOBOCAN estimates of incidence and mortalityworldwide for 36 cancers in 185 countries. CA: Cancer J Clin (2018) 68:394–424. doi: 10.3322/caac.21492
4. Park B, Kim Y, Lee J, Lee N, Jang SH.Sex Difference and Smoking Effect of Lung Cancer Incidence in AsianPopulation. Cancers (Basel) (2021) 13:113. doi: 10.3390/cancers13010113
5. Stigliani S, Massarotti C, De Leo C, Maccarini E, Sozzi F, Cagnacci A, et al. Fifteen Year Regional Center Experience in Sperm Banking for Cancer Patients: Use and Reproductive Outcomes in Survivors. Cancers (Basel) (2021) 13(1):116. doi: 10.3390/cancers13010116
6. Smith SM, Wachter K, Burris HA, Schilsky RL, George DJ, Peterson DE, et al. Clinical Cancer Advances 2021: ASCO’s Report on Progress Against Cancer. J Clin Oncol (2021). doi: 10.1200/JCO.20.03420
7. Shi D, Khan F, Abagyan R. Extended Multitarget Pharmacology of Anticancer Drugs. J Chem Inf Model (2019) 59:3006–17. doi: 10.1021/acs.jcim.9b00031
8. Lawal B, Liu Y-L, Mokgautsi N, Khedkar H, Sumitra MR, Wu ATH, et al. Pharmacoinformatics and Preclinical Studies of NSC765690 and NSC765599, Potential STAT3/CDK2/4/6 Inhibitors with Antitumor Activities against NCI60 Human Tumor Cell Lines. Biomedicines (2021) 9:92. doi: 10.3390/biomedicines9010092
9. Yadav VK, Huang Y-J, George TA, Wei P-L, Sumitra MR, Ho C-L, et al. Preclinical Evaluation of the Novel Small-Molecule MSI-N1014 for Treating Drug-Resistant Colon Cancer via the LGR5/β-catenin/miR-142-3p Network and Reducing Cancer-Associated FibroblastTransformation. Cancers (Basel) (2020)12:1590. doi: 10.3390/cancers12061590
10. Fereidoonnezhad M, Faghih Z, Mojaddami A, Rezaei Z, Sakhteman AA. Comparative QSAR Analysis, Molecular Docking and PLIF Studies of Some N-arylphenyl-2, 2-Dichloroacetamide Analogues as Anticancer Agents. Iran J Pharm Res (2017) 16:981–98. doi: 10.22037/ijpr.2017.2064
11. Lawal B, Lin L-C, Lee J-C, Chen J-H, Bekaii-Saab TS, Wu ATH, et al. Multi-Omics Data Analysis of Gene Expressions and Alterations, Cancer-Associated Fibroblast and Immune Infiltrations, Reveals the Onco-Immune Prognostic Relevance of STAT3/CDK2/4/6in Human Malignancies. Cancers (Basel) (2021)13:954. doi: 10.3390/cancers13050954
12. Hanahan D, Weinberg RA. Hallmarks of cancer: the next generation. Cell (2011) 144:646–74. doi: 10.1016/j.cell.2011.02.013
13. Witsch E, Sela M, Yarden Y. Roles for Growth Factors in Cancer Progression. Physiology (2010) 25:85–101. doi: 10.1152/physiol.00045.2009
14. Ghosh S, Marrocco I, Yarden Y. Roles for receptor tyrosine kinases in tumor progression and implications for cancer treatment. Adv Cancer Res 147:1–57. doi: 10.1016/bs.acr.2020.04.002
15. Lastwika KJ, Wilson W, Li QK, Norris J, Xu H, Ghazarian SR, et al. Control of PD-L1 Expression by Oncogenic Activation of the AKT–mTOR Pathway in Non–Small Cell Lung Cancer. Cancer Res (2016) 76:227–38. doi: 10.1158/0008-5472.can-14-3362
16. Selvaraj G, Kaliamurthi S, Kaushik AC, Khan A, Wei Y-K, Cho WC, et al. Identification of target gene and prognostic evaluation for lung adenocarcinoma using gene expression meta-analysis, network analysis and neural network algorithms. J Biomed Inf (2018) 86:120–34. doi: 10.1016/j.jbi.2018.09.004
17. Chang CF, Diers AR, Hogg N. Cancer cell metabolism and the modulating effects of nitric oxide. Free Radical Biol Med (2015) 79:324–36. doi: 10.1016/j.freeradbiomed.2014.11.012
18. Sanghani H, Ganatra S, Pande R. Molecular—docking studies of potent anticancer agent. J Comput Sci Syst Biol (2012)5:12–5. doi: 10.4172/jcsb.1000085
19. Baskaran C, Ramachandran M. Computational molecular docking studies on anticancer drugs. Asian Pac J Trop Dis (2012) 2:S734–8. doi: 10.1016/S2222-1808(12)60254-0
20. Cheng C-P, Huang H-S, Hsu Y-C, Sheu M-J, Chang D-MA. Benzamide-linked Small Molecule NDMC101 Inhibits NFATc1 and NF-κB Activity: A Potential Osteoclastogenesis Inhibitor for Experimental Arthritis. J Clin Immunol (2012) 32:762–77. doi: 10.1007/s10875-012-9660-9
21. Lee C-C, Liu F-L, Chen C-L, Chen T-C, Chang D-M, Huang H-S. Discovery of 5-(2′,4′-difluorophenyl)-salicylanilides as new inhibitors of receptor activator of NF-κB ligand (RANKL)-induced osteoclastogenesis. Eur J med Chem (2015) 98:115–26. doi: 10.1016/j.ejmech.2015.05.015
22. Lee C-C, Liu F-L, Chen C-L, Chen T-C, Liu F-C, Ahmed Ali AA, et al. Novel inhibitors of RANKL-induced osteoclastogenesis: Design, synthesis, and biological evaluation of 6-(2,4-difluorophenyl)-3-phenyl-2H-benzo[e][1,3]oxazine-2,4(3H)-diones. Bioorganic Med Chem (2015) 23:4522–32. doi: 10.1016/j.bmc.2015.06.007
23. Lee J-C, Wu ATH, Chen J-H, Huang W-Y, Lawal B, Mokgautsi N, et al. HNC0014, a Multi-Targeted Small-Molecule, Inhibits Head and Neck Squamous CellCarcinoma by Suppressing c-Met/STAT3/CD44/PD-L1 Oncoimmune Signature and Eliciting Antitumor ImmuneResponses. Cancers (Basel) (2020) 12:3759. doi: 10.3390/cancers12123759
24. Daina A, Michielin O, Zoete V. SwissADME: a free web tool to evaluate pharmacokinetics, drug-likeness and medicinal chemistry friendliness of small molecules. Sci Rep (2017) 7:42717. doi: 10.1038/srep42717
25. Lipinski CA. Lead- and drug-like compounds: the rule-of-five revolution. Drug Discovery Today: Technol (2004) 1:337–41. doi: 10.1016/j.ddtec.2004.11.007
26. Liu X, Ouyang S, Yu B, Liu Y, Huang K, Gong J, et al. PharmMapper server: a web server for potential drug target identification using pharmacophore mapping approach. Nucleic Acids Res (2010) 38:W609–14. doi: 10.1093/nar/gkq300
27. Poroikov VV, Filimonov DA, Gloriozova TA, Lagunin AA, Druzhilovskiy DS, Rudik AV, et al. Computer-aided prediction of biological activity spectra for organic compounds: the possibilities and limitations. Russian Chem Bull (2019) 68:2143–54. doi: 10.1007/s11172-019-2683-0
28. Li T, Fu J, Zeng Z, Cohen D, Li J, Chen Q, et al. TIMER2.0 for analysis of tumor-infiltrating immune cells. Nucleic Acids Res (2020) 48:W509–14. doi: 10.1093/nar/gkaa407
29. Tang Z, Li C, Kang B, Gao G, Li C, Zhang Z. GEPIA: a web server for cancer and normal gene expression profiling and interactive analyses. Nucleic Acids Res (2017) 45:W98–102. doi: 10.1093/nar/gkx247
30. Cerami E, Gao J, Dogrusoz U, Gross BE, Sumer SO, Aksoy BA, et al. The cBio cancer genomics portal: an open platform for exploring multidimensional cancer genomics data. Cancer Discovery (2012) 2:401–4. doi: 10.1158/2159-8290.cd-12-0095
31. Gao J, Aksoy BA, Dogrusoz U, Dresdner G, Gross B, Sumer SO, et al. Integrative analysis of complex cancer genomics and clinical profiles using the cBioPortal. Sci Signal (2013) 6(269):p. l1. doi: 10.1126/scisignal.2004088
32. Szklarczyk D, Gable AL, Lyon D, Junge A, Wyder S, Huerta-Cepas J, et al. STRING v11: protein-protein association networks with increased coverage, supporting functional discovery in genome-wide experimental datasets. Nucleic Acids Res (2019) 47:D607–13. doi: 10.1093/nar/gky1131
33. Chen EY, Tan CM, Kou Y, Duan Q, Wang Z, Meirelles GV, et al. Enrichr: interactive and collaborative HTML5 gene list enrichment analysis tool. BMC Bioinf (2013) 14:128. doi: 10.1186/1471-2105-14-128
34. Kuleshov MV, Jones MR, Rouillard AD, Fernandez NF, Duan Q, Wang Z, et al. Enrichr: a comprehensive gene set enrichment analysis web server 2016 update. Nucleic Acids Res (2016) 44:W90–7. doi: 10.1093/nar/gkw377
35. Trott O, Olson AJ. AutoDock Vina: improving the speed and accuracy of docking with a new scoring function, efficient optimization, and multithreading. J Comput Chem (2010) 31:455–61. doi: 10.1002/jcc.21334
36. Marcus D Hanwell DEC, Lonie DC, Vandermeersch T.Eva Zurek and Geoffrey R Hutchison. Avogadro: An advanced semantic chemical editor,visualization, and analysis platform. J Cheminformatics(2012) 4:17. doi: 10.1186/1758-2946-4-17
37. Visualizer DS, BIOVIA. Dassault Systèmes, BIOVIA Workbook, Release 2020; BIOVIA Pipeline Pilot, Release 2020. San Diego: Dassault Systèmes (2020).
38. Salentin S, Schreiber S, Haupt VJ, Adasme MF, Schroeder M. PLIP: fully automated protein-ligand interaction profiler. Nucleic Acids Res (2015) 43:W443–7. doi: 10.1093/nar/gkv315
39. Shoemaker RH.The NCI60 human tumour cell line anticancer drug screen. NatRev Cancer (2006) 6:813–23. doi: 10.1038/nrc1951
40. Holbeck SL, Collins JM, Doroshow JH. Analysis of Food and Drug Administration-approved anticancer agents in the NCI60 panel of human tumor cell lines. Mol Cancer Ther (2010) 9:1451–60. doi: 10.1158/1535-7163.MCT-10-0106
41. Vichai V, Kirtikara K. Sulforhodamine B colorimetric assay for cytotoxicity screening. Nat Protoc (2006) 1:1112–6. doi: 10.1038/nprot.2006.179
42. Boyd MR, Paull KD. Some practical considerations and applications of the National Cancer Institute invitro anticancer drug discovery screen. Drug Dev Res (1995) 34:91–109. doi: 10.1002/ddr.430340203
43. Paull K, Shoemaker R, Hodes L, Monks A, Scudiero D, Rubinstein L, et al. Display and analysis of patterns of differential activity of drugs against humantumor cell lines: development of mean graph and COMPARE algorithm. JNCI: JNatl Cancer Inst (1989) 81:1088–92. doi: 10.1093/jnci/81.14.1088
44. Lu M, Zhan X. The crucial role of multiomic approach in cancer research and clinically relevant outcomes. EPMA J (2018) 9:77–102. doi: 10.1007/s13167-018-0128-8
45. Schenone M, Dančík V, Wagner BK, Clemons PA. Target identification and mechanism of action in chemical biology and drugdiscovery. Nat Chem Biol (2013) 9:232. doi: 10.1038/nchembio.1199
46. Gupta PP, Bastikar VA, Kuciauskas D, Kothari SL, Cicenas J, Valius M. Molecular modeling and structure-based drug discovery approach reveals protein kinases as off-targets for novel anticancer drug RH1. Med Oncol (2017) 34:176. doi: 10.1007/s12032-017-1011-5
47. Murugan AK. mTOR: Role in cancer, metastasis and drug resistance. Semin Cancer Biol (2019) 59:92–111. doi: 10.1016/j.semcancer.2019.07.003
48. McCubrey JA, Abrams SL, Fitzgerald TL, Cocco L, Martelli AM, Montalto G, et al. Roles of signaling pathways in drug resistance, cancer initiating cells and cancer progression and metastasis. Adv Biol Regul (2015) 57:75–101. doi: 10.1016/j.jbior.2014.09.016
49. Muz B, de la Puente P, Azab F, Azab AK. The role of hypoxia in cancer progression, angiogenesis, metastasis, and resistance to therapy. Hypoxia (Auckland N.Z.) (2015) 3:83–92. doi: 10.2147/hp.s93413
50. Sasaki T, Hiroki K, Yamashita Y. The Role of Epidermal Growth Factor Receptor in Cancer Metastasis and Microenvironment. BioMed Res Int (2013) 2013:546318. doi: 10.1155/2013/546318
51. Normanno N, De Luca A, Bianco C, Strizzi L, Mancino M, Maiello MR, et al. Epidermal growth factor receptor (EGFR) signaling in cancer. Gene (2006) 366:2–16. doi: 10.1016/j.gene.2005.10.018
52. Dong Y, Zhai W, Xu Y. Bioinformatic gene analysis for potential biomarkers and therapeutic targets ofdiabetic nephropathy associated renal cell carcinoma. Trans Andrology Urol (2020) 9:2555–71. doi:10.21037/tau-19-911
53. Chen Y-Z, Zuo D, Ren H-L, Fan S-J, Ying G. Bioinformatics Analysis of Expression and Alterations of BARD1 in Breast Cancer. Technol Cancer Res Treat (2019) 18:1533033819892260. doi: 10.1177/1533033819892260
54. Bendahou MA, Ibrahimi A, Boutarbouch M. Bioinformatics Analysis of Differentially Expressed Genes and miRNAs in Low-Grade Gliomas. Cancer Inf (2020) 19:1176935120969692. doi: 10.1177/1176935120969692
55. Beck TN, Chikwem AJ, Solanki NR, Golemis EA. Bioinformatic approaches to augment study of epithelial-to-mesenchymal transition in lung cancer. Physiol Genomics (2014) 46:699–724. doi: 10.1152/physiolgenomics.00062.2014
56. Ding L, Getz G, Wheeler DA, Mardis ER, McLellan MD, Cibulskis K, et al. Somatic mutations affect key pathways in lung adenocarcinoma. Nature (2008) 455:1069–75. doi: 10.1038/nature07423
57. Gower A, Wang Y, Giaccone G. Oncogenic drivers, targeted therapies, and acquired resistance in non-small-cell lung cancer. J Mol Med (Berlin Germany) (2014) 92:697–707. doi: 10.1007/s00109-014-1165-y
58. Saadeh FS, Mahfouz R, Assi HI. EGFR as a clinical marker in glioblastomas and other gliomas. Int J Biol Markers (2018) 33:22–32. doi: 10.5301/ijbm.5000301
59. Tang Z-H, Lu J-J. Osimertinib resistance in non-small cell lung cancer: Mechanisms and therapeutic strategies. Cancer Lett (2018) 420:242–6. doi: 10.1016/j.canlet.2018.02.004
60. Lazzari C, Gregorc V, Karachaliou N, Rosell R, Santarpia M.Mechanisms of resistance to osimertinib. J ThoracDis (2019) 12:2851–8. doi:10.21037/jtd.2019.08.30
61. Monks A, Zhao Y, Hose C, Hamed H, Krushkal J, Fang J, et al.The NCI transcriptional pharmacodynamics workbench: a tool to examine dynamicexpression profiling of therapeutic response in the NCI-60 cell line panel.Cancer Res (2018)78:6807–17. doi: 10.1158/0008-5472.CAN-18-0989
62. Sausville EA, Holbeck SL. Transcription profiling of gene expression in drug discovery and development: the NCIexperience. Eur J Cancer (2004) 40:2544–9. 10.1016/j.ejca.2004.08.006
63. Shankavaram UT, Reinhold WC, Nishizuka S, Major S, Morita D, Chary KK, et al. Transcript and protein expression profiles of the NCI-60 cancer cell panel: an integromic microarray study. Mol Cancer Ther (2007) 6:820–32. doi: 10.1158/1535-7163.MCT-06-0650
64. Kohn KW, Zeeberg BR, Reinhold WC, Sunshine M, Luna A, Pommier Y. Gene expression profiles of the NCI-60 human tumor cell lines define molecular interaction networks governing cell migration processes. PloS One (2012) 7:e35716. doi: 10.1371/journal.pone.0035716
65. Paez JG, Jänne PA, Lee JC, Tracy S, Greulich H, Gabriel S, et al. EGFR mutations in lung cancer: correlation with clinical response to gefitinib therapy. Science (2004) 304:1497–500. doi: 10.1126/science.1099314
66. Pao W, Miller VA, Politi KA, Riely GJ, Somwar R, Zakowski MF, et al. Acquired resistance of lung adenocarcinomas to gefitinib or erlotinib is associated with a second mutation in the EGFR kinase domain. PloS Med (2005) 2:e73. doi: 10.1371/journal.pmed.0020073
67. Liu W, Wu X, Zhang W, Montenegro RC, Fackenthal DL, Spitz JA, et al. Relationship of <em<EGFR</em< Mutations, Expression, Amplification, and Polymorphisms to Epidermal Growth Factor Receptor Inhibitors in the NCI60 Cell Lines. Clin Cancer Res (2007) 13:6788. doi: 10.1158/1078-0432.CCR-07-0547
68. Ikediobi ON, Davies H, Bignell G, Edkins S, Stevens C, O’Meara S, et al. Mutation analysis of 24 known cancer genes in the NCI-60 cell line set. Mol Cancer Ther (2006) 5:2606–12. doi: 10.1158/1535-7163.MCT-06-0433
69. Li Y, Li P-K, Roberts MJ, Arend RC, Samant RS, Buchsbaum DJ. Multi-targeted therapy of cancer by niclosamide: A new application for an old drug. Cancer Lett (2014) 349:8–14. doi: 10.1016/j.canlet.2014.04.003
70. Ren X, Duan L, He Q, Zhang Z, Zhou Y, Wu D, et al.Identification of Niclosamide as a New Small-Molecule Inhibitor of the STAT3Signaling Pathway. ACS Med Chem Lett (2010) 1:454–59. doi: 10.1021/ml100146z
71. Di Santo N, Ehrisman J. A functional perspective of nitazoxanide as a potential anticancer drug. Mutat Res/Fundam Mol Mech Mutagen (2014) 768:16–21. doi: 10.1016/j.mrfmmm.2014.05.005
72. Zhao H, Huang D. Hydrogen bonding penalty upon ligand binding. PloS One (2011) 6:e19923. doi: 10.1371/journal.pone.0019923
73. Arthur DE, Uzairu A. Molecular docking studies on the interaction of NCI anticancer analogues with human Phosphatidylinositol 4,5-bisphosphate 3-kinase catalytic subunit. J King Saud Univ - Sci (2019) 31:1151–66. doi: 10.1016/j.jksus.2019.01.011
74. Chelliah V, Blundell TL, Fernández-Recio J. Efficient restraints for protein-protein docking by comparison of observed amino acid substitution patterns with those predicted from local environment. J Mol Biol (2006) 357:1669–82. doi: 10.1016/j.jmb.2006.01.001
75. Oronsky BT, Reid T, Knox SJ, Scicinski JJ. The scarlet letter of alkylation: a mini review of selective alkylating agents. Trans Oncol (2012) 5:226–9. doi: 10.1593/tlo.12187
76. Vlahos CJ, Dekker EE.Active-site residues of 2-keto-4-hydroxyglutarate aldolase from Escherichia coli.Bromopyruvate inactivation and labeling of glutamate 45. J BiolChem (1990) 265:20384–9. doi:10.1016/S0021-9258(17)30515-X
77. Cinelli MA, Do HT, Miley GP, Silverman RB. Inducible nitric oxide synthase: Regulation, structure, and inhibition. Med Res Rev (2020) 40:158–89. doi: 10.1002/med.21599
78. Patil R, Das S, Stanley A, Yadav L, Sudhakar A, Varma AK. Optimized hydrophobic interactions and hydrogen bonding at the target-ligand interface leads the pathways of drug-designing. PloS One (2010) 5:e12029–9. doi: 10.1371/journal.pone.0012029
Keywords: NSC765598, multi-omics study, multi-target small molecule, anticancer activity, molecular docking, protein-ligand interaction
Citation: Lawal B, Lee C-Y, Mokgautsi N, Sumitra MR, Khedkar H, Wu ATH and Huang H-S (2021) mTOR/EGFR/iNOS/MAP2K1/FGFR/TGFB1 Are Druggable Candidates for N-(2,4-Difluorophenyl)-2′,4′-Difluoro-4-Hydroxybiphenyl-3-Carboxamide (NSC765598), With Consequent Anticancer Implications. Front. Oncol. 11:656738. doi: 10.3389/fonc.2021.656738
Received: 21 January 2021; Accepted: 08 March 2021;
Published: 26 March 2021.
Edited by:
Sonja M. Kessler, Martin Luther University of Halle-Wittenberg, GermanyReviewed by:
Charlotte Dahlem, Saarland University, GermanyAbdul Ajees Abdul Salam, Manipal Academy of Higher Education, India
Richard Barry Sessions, University of Bristol, United Kingdom
Copyright © 2021 Lawal, Lee, Mokgautsi, Sumitra, Khedkar, Wu and Huang. This is an open-access article distributed under the terms of the Creative Commons Attribution License (CC BY). The use, distribution or reproduction in other forums is permitted, provided the original author(s) and the copyright owner(s) are credited and that the original publication in this journal is cited, in accordance with accepted academic practice. No use, distribution or reproduction is permitted which does not comply with these terms.
*Correspondence: Alexander T.H. Wu, chaw1211@tmu.edu.tw; Hsu-Shan Huang, huanghs99@tmu.edu.tw
†These authors have contributed equally to this work