- 1Department of Radiology, West China Hospital, Sichuan University, Chengdu, China
- 2Department of Laboratory Medicine, State Key Laboratory of Biotherapy, West China Hospital, Sichuan University, Chengdu, China
Gastric cancer (GC) is one of the most common cancers and one of the leading causes of cancer-related death worldwide. Precise diagnosis and evaluation of GC, especially using noninvasive methods, are fundamental to optimal therapeutic decision-making. Despite the recent rapid advancements in technology, pretreatment diagnostic accuracy varies between modalities, and correlations between imaging and histological features are far from perfect. Artificial intelligence (AI) techniques, particularly hand-crafted radiomics and deep learning, have offered hope in addressing these issues. AI has been used widely in GC research, because of its ability to convert medical images into minable data and to detect invisible textures. In this article, we systematically reviewed the methodological processes (data acquisition, lesion segmentation, feature extraction, feature selection, and model construction) involved in AI. We also summarized the current clinical applications of AI in GC research, which include characterization, differential diagnosis, treatment response monitoring, and prognosis prediction. Challenges and opportunities in AI-based GC research are highlighted for consideration in future studies.
Introduction
As one of the most common cancers, gastric cancer (GC) ranks as the top three in terms of mortality rate (1). The American Joint Commission on Cancer (8th Edition) for Gastric Cancer recommends computed tomography (CT) and endoscopic ultrasound for pretreatment TNM classification, whereas magnetic resonance imaging (MRI) and Positron Emission Tomography – Computed Tomography (PET-CT) are effective alternatives for metastasis evaluation. Despite the introduction of new techniques, the pretreatment diagnostic accuracy of GC varies from 40.8% to 98.1% (2–4). Efforts have also been made toward the prediction of histological type such as tumor differentiation grade and Lauren classification, based on enhancement pattern analysis, perfusion analysis, and spectral analysis, which have moderate discriminating performance and area under the curve (AUC) ranging from 0.697 to 0.891 (5–7). Given the importance of accurate pretreatment imaging evaluation and prognostic value of histopathological features, there is an urgent need for better diagnostic methods for treatment planning.
Fortunately, there has been considerable progress in artificial intelligence (AI) during the past decade, which offers promise for meeting these needs. Of all the AI techniques, hand-crafted radiomics and deep learning (DL) are the two most frequently applied methods for medical imaging and have shown the powerful capacity for converting mass medical images into minable data. With the ability to detect features that are invisible to human readers, hand-crafted radiomics and DL have demonstrated promising performance in tumor detection, characterization, and monitoring (8).
Therefore, we reviewed the published AI methodologies utilized in studies on GC imaging to provide an overview of the latest developments. This included data acquisition, lesion segmentation, feature design, and model construction. Furthermore, we summarize the representative clinical applications, knowledge gaps, and future directions. A total of 47 published AI studies on gastric cancer imaging were selected through MEDLINE (June, 2021), of which 45 were retrospective in design (36 single-center and 9 multicenter studies), while the remaining two were single-center prospective studies (Table 1). Imaging modalities varied across the studies. Specifically, 39 studies were performed using CT with only one studies based on dual-energy CT, six used MRI, and two used PET-CT (Table 1).
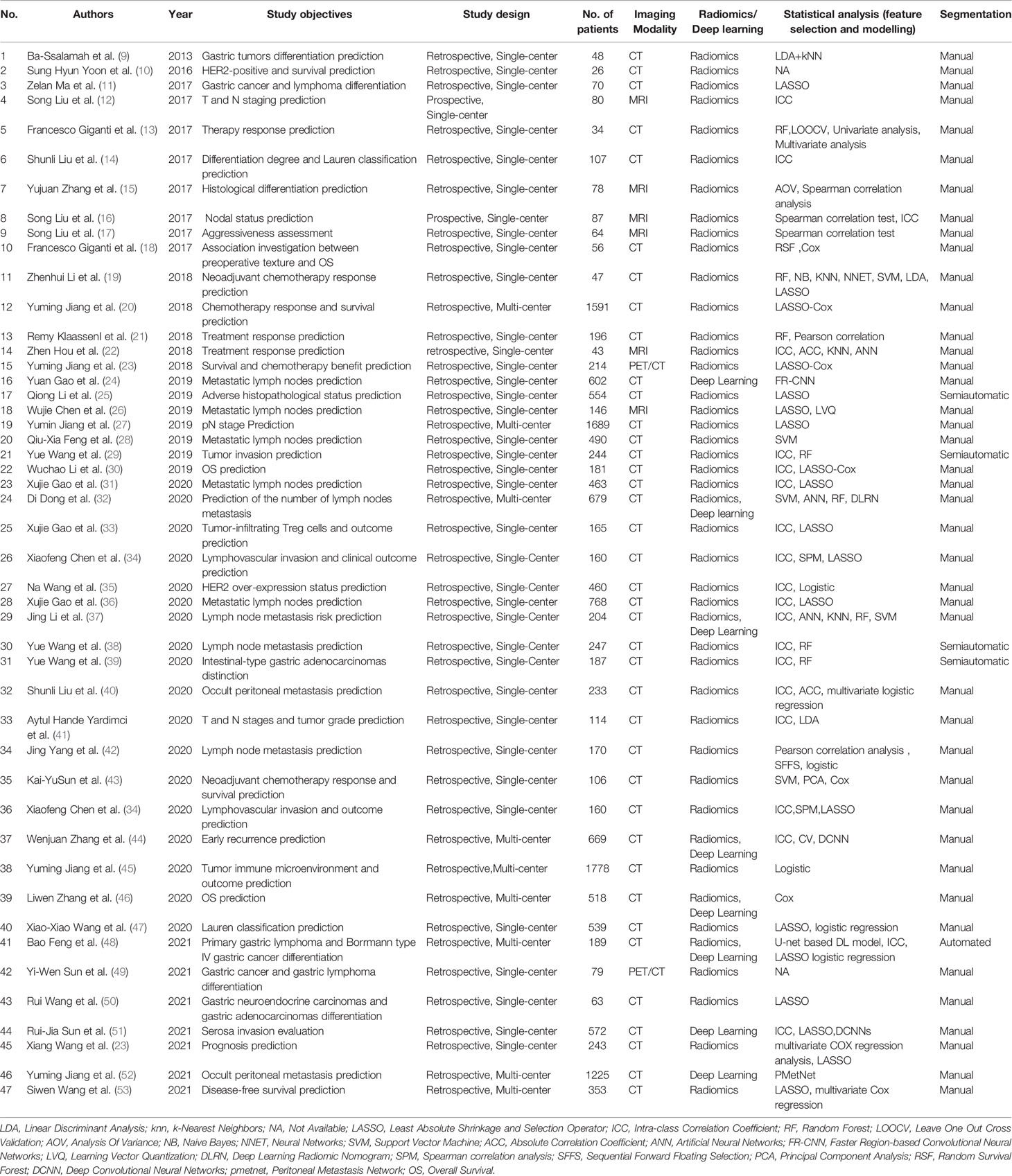
Table 1 Summary of published hand-crafted radiomics and deep learning studies on gastric cancer imaging.
Methodologies of Ai Studies On Gastric Cancer
Data Acquisition
Image preprocessing accounts for the substantial heterogeneity introduced by different imaging modalities, scanning protocols, machine types, and manufacturers. Image intensity normalization and resampling are two mathematical techniques that are used widely for this purpose. Specifically, image intensity normalization is performed to transform the original image into a standardized form to reduce data variability between cohorts and to generate appropriate inputs for quantitative radiomic feature calculation (20, 27). Resampling is used to adapt the input shape of the model by transforming the original image into the target size by upsampling or downsampling (32, 33, 36, 44).
In addition to imaging data, clinicopathological data also play an important role in AI-based modeling and can be used to improve model performance. These factors included patient age, gender, body mass index, cancer antigen 72-4 (CA72-4), CA199, CA242, carcinoembryonic antigen, alpha-fetoprotein, tumor location, tumor size, and TNM stages (9–13, 15, 17, 18, 20–22, 24–28, 30–36, 38–45, 54).
Lesion Segmentation
Segmentation of region of interests (ROIs) in AI analysis can be performed using manual, automatic, or semiautomatic methods. Among the included AI-based GC studies, 42 (89%) studies utilized manual segmentation methods, four (9%) applied semiautomatic methods (25, 30, 38, 39), and only one study (2%) used automatic method (48).
Manual segmentation, which is usually carried out by radiologists, involves placing rectangular/circular boxes that delineate the two-/three-dimensional (2D/3D) boundary of the whole lesion. In Di Dong et al.’s study, 2D ROIs were placed to cover the largest tumor area for predicting lymph node metastasis in locally advanced GC (32). Yue Wang et al. segmented the entire tumor and built a 3D-based hand-crafted radiomics model to diagnose intestinal-type gastric adenocarcinomas (39). In addition, Wenjuan Zhang et al. constructed a DL model on 18 layers of residual convolutional neural network (CNN) with squared segmentation of CT images to predict overall survival (OS) in GC patients (44). It is important to note that because subjective judgments regarding tumor boundaries can vary substantially among radiologists, manual segmentations by multiple radiologists at multiple time points are required to minimize intra- and inter-rater variability. In addition, intra- and interclass correlation coefficients and coefficients of variation are often calculated to evaluate the robustness and reproducibility of the extracted features (12, 14, 17, 22, 30, 31, 34, 36, 39, 41).
In contrast to manual segmentation, semiautomatic segmentation usually comprises two steps. First, several labeling points are marked by radiologists. Thereafter, the entire ROIs are generated automatically by computing devices, based on the labeling points. Satisfactory gastric lesion segmentation performance has been achieved using this approach (25, 30, 38, 39). All the four studies using semiautomatic segmentation employed the same software package (Frontier, Syngo via, Siemens healthcare), which applies a dichotomic classification algorithm to semiautomatically segment lesions from perinormal areas.
Feature Extraction
After lesion segmentation, quantitative handcrafted engineer features can be calculated to profile the intrinsic characteristics of the ROI. Handcrafted engineer features can be categorized as first-order statistics, shape-based, or texture-based features. First-order statistics are used to describe the distribution of pixel/voxel intensities in the ROIs, shape-based features show the geometric properties of the ROIs, and texture-based features are gray level matrices that represent textural patterns in an image region. Commonly used manual engineered features are presented in Table 2.
As opposed to handcrafted features, DL features are derived directly from the artificial neural networks, which encode medical images into a series of feature maps to extract features that represent high-dimensional information that cannot be detected by human readers. Using this method, Yuan Gao et al. achieved a mean average precision value and AUC of 0.7801 and 0.9541 in predicting perigastric lymph node metastasis, based on faster region-based CNN (24).
Handcrafted features describe the morphology, intensity, and textural patterns of ROIs, whereas deep learning network can automatically learn non-handcrafted feature representations from sample images.
Furthermore, studies combining handcrafted engineer and DL features have been carried out to maximize model efficiency. In Wenjuan Zhang et al.’s study, three handcrafted features, six DL features, and several clinical factors were combined to construct a nomogram, which demonstrated AUCs of 0.806-0.831 in predicting postoperative early recurrence in GC patients (44).
Feature Selection
Most commonly used feature selection methods are categorized into the filter, wrapper, or embedded methods. Among these approaches, filter-based methods (e.g., correlation analysis, analysis of variance) are the simplest methods and select features according to a mutual information criterion (12, 14, 42, 55). Wrappers (e.g., recursive feature elimination, sequential feature selection algorithms, and genetic algorithms) extract useful features based on classifier performance. Filters and wrappers are frequently combined to improve feature selection ability. Using Pearson correlation analysis and the sequential forward floating selection algorithm, Jing Yang et al. obtained optimal tumor and nodal hand-crafted radiomics features to construct a model, which demonstrated good predictive performance for GC metastasis (42). Embedded methods perform variable selection during the model training process. The least absolute shrinkage and selection operator (LASSO) is a classical and widely applied embedded method (11, 19, 25, 27, 31, 33, 34, 36, 45). Unlike the aforementioned methods, LASSO regression adds a penalty against complexity, which can enable the construction of a simple, yet effective model with a small number of features.
Model Construction
Regarding modeling strategy, logistic regression models (e.g., multivariate logistic regression, LASSO regression) have been widely used in AI-based GC studies. Random forest and support vector machines (SVM) are also effective alternatives for model construction (19, 28, 32, 36, 43). In a multicenter study, Di Dong et al. proposed an AI model that integrated DL, hand-crafted radiomics, and clinical factors. Their model used various modeling methods, including SVM, artificial neural networks, random forest, Spearman’s correlation analysis, logistic regression analysis, and linear regression analysis, and demonstrated good predictive performance for lymph node metastasis in locally advanced GC (32).
The above workflow and key methodologies of AI techniques in GC imaging are summarized in Figure 1.
Clinical Applications of Hand-Crafted Radiomics and Deep Learning In Gastric Cancer
Major clinical applications of AI in GC research are shown in Figure 2.
Characterization
The TNM classification is the most widely used staging system in GC, and pretreatment CT/MRI is vital for making optimal treatment decisions (56, 57). Considering its widespread application, most hand-crafted radiomics and DL studies have utilized CT images for preoperative prediction of TNM stages (24, 27, 28, 31, 32, 36–38, 40–42, 51, 52). Precise pretreatment TNM staging of lymph node metastasis is plagued by major obstacles because of discrepancies in traditional imaging features, such as shape, size, and enhancement patterns. Therefore, many researchers have been developing AI-based models to accurately predict lymph node status in GC patients (24, 27, 28, 31, 32, 36–38, 41, 42). While Yang et al.’s study combined tumor and nodal hand-crafted radiomics features (42), other studies selected only the tumor for the ROI (24, 27, 28, 31, 32, 36–38, 41). Of the 10 studies focusing on lymph node status, seven were designed to discriminate between N+ and N- (28, 31, 36–38, 41, 42), two to discriminate specific N stages (N0–3) (27, 32), and one with ambiguous lymph node status (24). Models based on hand-crafted radiomics, DL, or the combination of the two have shown AUC and C-indices of 0.79–0.95 in the training and 0.76–0.89 in the validation cohorts, respectively (24, 27, 28, 31, 32, 36–38, 41, 42). Three studies tested model efficacies for T stage prediction, where two aimed to discriminate T1/2 from T3/4 (29, 30, 41), and one to classify all T1–4 stages (25), with all yielding good discriminatory performance with AUCs ranging from 0.82 to 0.90. Liu et al. investigated venous CT images of primary tumors in advanced GC and built a hand-crafted radiomics model to predict occult peritoneal metastasis (40). Because of the popularity of CT, MRI has been used less frequently in GC patients, with only four studies focused on MRI-based prediction of TNM staging (12, 16, 17, 26). Using hand-crafted radiomics analysis, the authors found that diffusion-weighted imaging and apparent diffusion coefficient maps demonstrate potential in preoperative T and N staging for GC.
Using histopathological results as a reference, six studies explored the correlation between AI-based models and prognosis-related factors of tumor differentiation grade (9, 14, 15, 25), Lauren classification (14, 39, 47), and lymphovascular and neural invasion (14, 17, 25, 34). Two studies were based on MRI images (15, 17) and four were on CT images (14, 25, 34, 39), and all models exhibited good predictive ability for GC before operation. In addition, researchers carrying out immunohistochemistry studies have developed hand-crafted radiomics models to predict human epidermal receptor 2 status, which could serve as a noninvasive prediction tool for GC for selecting candidates suitable for Herceptin (10, 35). Furthermore, Gao’s hand-crafted radiomics model showed good performance in estimating tumor-infiltrating regulatory T (TITreg) cells, with AUCs of 0.85–0.88 in various cohorts (33).
Differential Diagnosis
Five studies were conducted to differentiate between different gastric tumors (9, 11, 48–50). By applying texture analysis, Ba-Ssalamah et al. classified adenocarcinomas, lymphomas, and gastrointestinal stromal tumors from artery and portal venous CT images, respectively, and misclassification rates ranged from 0%–10% (9). Ma, Feng and Sun et al. focused specifically on differentiating Borrmann type IV GC from primary gastric lymphoma. By combining hand-crafted radiomics signatures, subjective CT findings, age, and gender, Ma’s model achieved a diagnostic accuracy of 87.1% (11). All these models demonstrated potential for accurate gastric tumor discrimination.
Treatment Response and Prognosis
Neoadjuvant chemotherapy (NAC) can decrease tumor size and reduce mortality (58) and is recommended for potentially resectable advanced GCs. However, response rates of NAC vary among studies (59). In patients who do not benefit from NAC, the delay in surgery can lead to tumor progression and poor prognosis. Therefore, noninvasive selection of NAC responders before treatment is crucial for treating patients with advanced GCs. Three studies have utilized CT-based hand-crafted radiomics analysis build models to predict non-responders, which have yielded AUCs of 0.65–0.82 (13, 19, 43). Notably, Sun et al. demonstrated that their hand-crafted radiomics model performed better for NAC response prediction compared with a clinical model (43).
Chemotherapy and radiation therapy are two mainstays for advanced GCs. Three studies have been carried out to predict chemotherapy response (20, 21, 23). Jiang et al.’s model showed that higher scores of their CT-based hand-crafted radiomics signature indicated a favorable response to chemotherapy for stage II-III patients (20). Similarly, Jiang et al. built a Rad-score system based on hand-crafted radiomics features from PET images, where higher scores indicated chemotherapy responders (23). Klaassen et al. focused on individual liver metastases in esophagogastric cancers and developed a CT-based hand-crafted radiomics model to predict responsive lesions; the resulting AUCs ranged between 0.65–0.87 in various cohorts (21). Only one study tested model efficacy for radiotherapy responders in GC patients with abdominal cavity metastasis. Based on pretreatment CT images, Hou et al. constructed two prediction models with high accuracies ranging from 0.71 to 0.82 (22).
Prognosis is the research highlight of AI-based studies and numerous researchers have explored the potential of hand-crafted radiomics and DL features for prognosis prediction (10, 18, 20, 23, 25, 30, 33, 34, 43–46, 53, 60). Nine studies directly correlated hand-crafted radiomics and DL features with prognosis (10, 18, 20, 23, 30, 44, 46, 53, 60), and five constructed AI-based models to predict certain clinicopathological features, which were shown to be related to prognosis (25, 33, 34, 43, 45). Only one study extracted hand-crafted radiomics features from PET images (23), whereas all others used CT images (10, 13, 18–22, 25, 30, 33, 34, 43–46, 53, 60). Earlier studies reported OS-related hand-crafted radiomics features (10, 18), with later studies building hand-crafted radiomics and DL models that integrated hand-crafted radiomics features with and without clinicopathological features; these achieved good performance in OS, disease-free survival (DFS), and early recurrence prediction (20, 23, 30, 33, 44). Furthermore, hand-crafted radiomics models to predict clinicopathological features, such as TITreg cells, lymphovascular invasion, adverse histopathological status, tumor immune microenvironment, and NAC response have also been developed, which have yielded AUCs of 0.75–0.89 for predicting OS, DFS, and progression-free survival (25, 33, 34, 43, 45).
Future Challenges and Opportunities
To date, numerous studies have demonstrated the prediction potential of hand-crafted radiomics and DL in GC characterization, differential diagnosis, treatment response, and prognosis. Despite the frequent application of MRI in clinical practice, it is not routinely recommended for GC evaluation. Most studies have focused on CT images and few have used MRI images. Considering its excellent resolution of soft tissue, MRI images may reveal more intrinsic tumor features and improve prediction. Therefore, future investigations should aim to include more patients undergoing MRI examinations for GC evaluation. Lymph node metastasis status is a key component of pretreatment and postoperative evaluation, and many studies have developed methods for pretreatment AI-based prediction, which include prediction of the existence of lymph node metastasis and N stage. However, there have not been any studies that have focused on individual lymph nodes, which is fundamental for precise pretreatment N stage evaluation and treatment plan modification during follow-up. We encourage future studies to focus on individual lymph node metastasis status prediction based on rigid pathological correlations. Moreover, few studies have analyzed the relationship between imaging features and treatment response. There is still a considerable knowledge gap in this field; further research is needed to improve patient selection and develop better treatment plans.
In addition, future efforts should continue to be actively pursued regarding the methodologies of AI. More intensive and standardized quality controls throughout the entire workflow of AI are warranted to meet this requirement. By analyzing a total of 77 hand-crafted radiomics-based oncology researches, Park et al. reported insufficient overall scientific quality of current hand-crafted radiomics studies (61). Similar dilemmas arose at every stage of GC from data acquisition, segmentation, feature extraction, feature selection, model construction to model performance reporting. In this context, compliance with widely-accepted quality systems [e.g. Hand-crafted radiomics Quality Score (RQS) (62), Transparent Reporting of a multivariable prediction model for Individual Prognosis Or Diagnosis (TRIPOD) (63), etc.] may offer appeal. In addition, prospective multi-institutional collaborations to establish well-curated databases and networks are encouraged in future studies. Furthermore, considering the inherent capacity of AI in analyzing parallel streams of information, including clinical and genomics characteristics (64–66), multi-omics studies which integrate these data may pave the way for better personalized and precision medicine. Collectively, we hope the fruit of these efforts could help to shift the landscape of AI in GC from exploratory research settings to routine clinical settings.
Conclusion
GC has a high incidence and mortality rate, which have been the clinical research emphasis over the past decades. Hand-crafted radiomics and DL are emerging quantitative subsets of AI that have been widely utilized in medicine. The exploration of GC using hand-crafted radiomics and DL has led to promising results for every step of the clinical pathway. However, most studies have been retrospective, conducted in a single center, and analyzed using a single image modality, which have limited the utility of the constructed AI models. Therefore, further prospective and multicenter studies are needed to validate the models. Moreover, other imaging modalities, such as endoscopic ultrasound may be integrated into the models to further improve model efficacy.
Author Contributions
BS and NH designed and supervised this study. YQ, YD, and HJ conducted the literature search, article selection, data extraction, data analyses and data interpretation. YQ and YD contributed to the conception of the study and drafted the manuscript. All authors contributed to writing of the manuscript and approved the final manuscript. YQ and YD contributed equally to this work. All authors contributed to the article and approved the submitted version.
Funding
This work was supported by grants from the National Natural Science Foundation of China (82002569, 81902437); 1.3.5 Project for Disciplines of Excellence, West China Hospital, Sichuan University (ZYJC21006, ZYYC20003).
Conflict of Interest
The authors declare that the research was conducted in the absence of any commercial or financial relationships that could be construed as a potential conflict of interest.
Supplementary Material
The Supplementary Material for this article can be found online at: https://www.frontiersin.org/articles/10.3389/fonc.2021.631686/full#supplementary-material
References
1. Bray F, Ferlay J, Soerjomataram I, Siegel RL, Torre LA, Jemal A. Global Cancer Statistics 2018: GLOBOCAN Estimates of Incidence and Mortality Worldwide for 36 Cancers in 185 Countries. CA Cancer J Clin (2018) 68(6):394–424. doi: 10.3322/caac.21492
2. Li J, Fang M, Wang R, Dong D, Tian J, Liang P, et al. Diagnostic Accuracy of Dual-Energy CT-Based Nomograms to Predict Lymph Node Metastasis in Gastric Cancer. Eur Radiol (2018) 28(12):5241–9. doi: 10.1007/s00330-018-5483-2
3. Yang L, Zheng R, Wang N, Yuan Y, Liu S, Li H, et al. Incidence and Mortality of Stomach Cancer in China, 2014. Chin J Cancer Res (2018) 30(3):291–8. doi: 10.21147/j.issn.1000-9604.2018.03.01
4. Seevaratnam R, Cardoso R, McGregor C, Lourenco L, Mahar A, Sutradhar R, et al. How Useful Is Preoperative Imaging for Tumor, Node, Metastasis (TNM) Staging of Gastric Cancer? A Meta-Analysis. Gastric Cancer (2011) 15(S1):3–18. doi: 10.1007/s10120-011-0069-6
5. Tsurumaru D, Miyasaka M, Muraki T, Asayama Y, Nishie A, Oki E, et al. Diffuse-Type Gastric Cancer: Specific Enhancement Pattern on Multiphasic Contrast-Enhanced Computed Tomography. Jpn J Radiol (2017) 35(6):289–95. doi: 10.1007/s11604-017-0631-1
6. Li R, Li J, Wang X, Liang P, Gao J. Detection of Gastric Cancer and Its Histological Type Based on Iodine Concentration in Spectral CT. Cancer Imaging (2018) 18(1):42. doi: 10.1186/s40644-018-0176-2
7. Lee DH, Kim SH, Joo I, Han JK. CT Perfusion Evaluation of Gastric Cancer: Correlation With Histologic Type. Eur Radiol (2017) 28(2):487–95. doi: 10.1007/s00330-017-4979-5
8. Bi WL, Hosny A, Schabath MB, Giger ML, Birkbak NJ, Mehrtash A, et al. Artificial Intelligence in Cancer Imaging: Clinical Challenges and Applications. CA: A Cancer J Clin (2019) 69(2):127–57. doi: 10.3322/caac.21552
9. Ba-Ssalamah A, Muin D, Schernthaner R, Kulinna-Cosentini C, Bastati N, Stift J, et al. Texture-Based Classification of Different Gastric Tumors at Contrast-Enhanced CT. Eur J Radiol (2013) 82(10):e537–43. doi: 10.1016/j.ejrad.2013.06.024
10. Yoon SH, Kim YH, Lee YJ, Park J, Kim JW, Lee HS, et al. Tumor Heterogeneity in Human Epidermal Growth Factor Receptor 2 (HER2)-Positive Advanced Gastric Cancer Assessed by CT Texture Analysis: Association With Survival After Trastuzumab Treatment. PloS One (2016) 11(8):e0161278. doi: 10.1371/journal.pone.0161278
11. Ma Z, Fang M, Huang Y, He L, Chen X, Liang C, et al. CT-Based Radiomics Signature for Differentiating Borrmann Type IV Gastric Cancer From Primary Gastric Lymphoma. Eur J Radiol (2017) 91:142–7. doi: 10.1016/j.ejrad.2017.04.007
12. Liu S, Zhang Y, Chen L, Guan W, Guan Y, Ge Y, et al. Whole-Lesion Apparent Diffusion Coefficient Histogram Analysis: Significance in T and N Staging of Gastric Cancers. BMC Cancer (2017) 17(1):665. doi: 10.1186/s12885-017-3622-9
13. Giganti F, Marra P, Ambrosi A, Salerno A, Antunes S, Chiari D, et al. Pre-Treatment MDCT-Based Texture Analysis for Therapy Response Prediction in Gastric Cancer: Comparison With Tumour Regression Grade at Final Histology. Eur J Radiol (2017) 90:129–37. doi: 10.1016/j.ejrad.2017.02.043
14. Liu S, Liu S, Ji C, Zheng H, Pan X, Zhang Y, et al. Application of CT Texture Analysis in Predicting Histopathological Characteristics of Gastric Cancers. Eur Radiol (2017) 27(12):4951–9. doi: 10.1007/s00330-017-4881-1
15. Zhang Y, Chen J, Liu S, Shi H, Guan W, Ji C, et al. Assessment of Histological Differentiation in Gastric Cancers Using Whole-Volume Histogram Analysis of Apparent Diffusion Coefficient Maps. J Magn Reson Imaging (2017) 45(2):440–9. doi: 10.1002/jmri.25360
16. Liu S, Zhang Y, Xia J, Chen L, Guan W, Guan Y, et al. Predicting the Nodal Status in Gastric Cancers: The Role of Apparent Diffusion Coefficient Histogram Characteristic Analysis. Magn Reson Imaging (2017) 42:144–51. doi: 10.1016/j.mri.2017.07.013
17. Liu S, Zheng H, Zhang Y, Chen L, Guan W, Guan Y, et al. Whole-Volume Apparent Diffusion Coefficient-Based Entropy Parameters for Assessment of Gastric Cancer Aggressiveness. J Magn Reson Imaging (2018) 47(1):168–75. doi: 10.1002/jmri.25752
18. Giganti F, Antunes S, Salerno A, Ambrosi A, Marra P, Nicoletti R, et al. Gastric Cancer: Texture Analysis From Multidetector Computed Tomography as a Potential Preoperative Prognostic Biomarker. Eur Radiol (2017) 27(5):1831–9. doi: 10.1007/s00330-016-4540-y
19. Li Z, Zhang D, Dai Y, Dong J, Wu L, Li Y, et al. Computed Tomography-Based Radiomics for Prediction of Neoadjuvant Chemotherapy Outcomes in Locally Advanced Gastric Cancer: A Pilot Study. Chin J Cancer Res (2018) 30(4):406–14. doi: 10.21147/j.issn.1000-9604.2018.04.03
20. Jiang Y, Chen C, Xie J, Wang W, Zha X, Lv W, et al. Radiomics Signature of Computed Tomography Imaging for Prediction of Survival and Chemotherapeutic Benefits in Gastric Cancer. EBioMedicine (2018) 36:171–82. doi: 10.1016/j.ebiom.2018.09.007
21. Tian J, Klaassen R, Larue RTHM, Mearadji B, van der Woude SO, Stoker J, et al. Feasibility of CT Radiomics to Predict Treatment Response of Individual Liver Metastases in Esophagogastric Cancer Patients. PloS One (2018) 13(11):e0207362. doi: 10.1371/journal.pone.0207362
22. Hou Z, Yang Y, Li S, Yan J, Ren W, Liu J, et al. Radiomic Analysis Using Contrast-Enhanced CT: Predict Treatment Response to Pulsed Low Dose Rate Radiotherapy in Gastric Carcinoma With Abdominal Cavity Metastasis. Quant Imaging Med Surg (2018) 8(4):410–20. doi: 10.21037/qims.2018.05.01
23. Jiang Y, Yuan Q, Lv W, Xi S, Huang W, Sun Z, et al. Radiomic Signature of (18)F Fluorodeoxyglucose PET/CT for Prediction of Gastric Cancer Survival and Chemotherapeutic Benefits. Theranostics (2018) 8(21):5915–28. doi: 10.7150/thno.28018
24. Gao Y, Zhang ZD, Li S, Guo YT, Wu QY, Liu SH, et al. Deep Neural Network-Assisted Computed Tomography Diagnosis of Metastatic Lymph Nodes From Gastric Cancer. Chin Med J (Engl) (2019) 132(23):2804–11. doi: 10.1097/CM9.0000000000000532
25. Li Q, Qi L, Feng QX, Liu C, Sun SW, Zhang J, et al. Machine Learning-Based Computational Models Derived From Large-Scale Radiographic-Radiomic Images Can Help Predict Adverse Histopathological Status of Gastric Cancer. Clin Transl Gastroenterol (2019) 10(10):e00079. doi: 10.14309/ctg.0000000000000079
26. Chen W, Wang S, Dong D, Gao X, Zhou K, Li J, et al. Evaluation of Lymph Node Metastasis in Advanced Gastric Cancer Using Magnetic Resonance Imaging-Based Radiomics. Front Oncol (2019) 9:1265. doi: 10.3389/fonc.2019.01265
27. Jiang Y, Wang W, Chen C, Zhang X, Zha X, Lv W, et al. Radiomics Signature on Computed Tomography Imaging: Association With Lymph Node Metastasis in Patients With Gastric Cancer. Front Oncol (2019) 9:340. doi: 10.3389/fonc.2019.00340
28. Feng QX, Liu C, Qi L, Sun SW, Song Y, Yang G, et al. An Intelligent Clinical Decision Support System for Preoperative Prediction of Lymph Node Metastasis in Gastric Cancer. J Am Coll Radiol (2019) 16(7):952–60. doi: 10.1016/j.jacr.2018.12.017
29. Wang Y, Liu W, Yu Y, Liu J-J, Jiang L, Xue H-D, et al. Prediction of the Depth of Tumor Invasion in Gastric Cancer: Potential Role of CT Radiomics. Acad Radiol (2019) 27(8):1077–84. doi: 10.1016/j.acra.2019.10.020
30. Li W, Zhang L, Tian C, Song H, Fang M, Hu C, et al. Prognostic Value of Computed Tomography Radiomics Features in Patients With Gastric Cancer Following Curative Resection. Eur Radiol (2019) 29(6):3079–89. doi: 10.1007/s00330-018-5861-9
31. Gao X, Ma T, Cui J, Zhang Y, Wang L, Li H, et al. A CT-Based Radiomics Model for Prediction of Lymph Node Metastasis in Early Stage Gastric Cancer. Acad Radiol (2020) 28(6):e155–64. doi: 10.1016/j.acra.2020.03.045
32. Dong D, Fang MJ, Tang L, Shan XH, Gao JB, Giganti F, et al. Deep Learning Radiomic Nomogram can Predict the Number of Lymph Node Metastasis in Locally Advanced Gastric Cancer: An International Multicenter Study. Ann Oncol (2020) 31(7):912–20. doi: 10.1016/j.annonc.2020.04.003
33. Gao X, Ma T, Bai S, Liu Y, Zhang Y, Wu Y, et al. A CT-Based Radiomics Signature for Evaluating Tumor Infiltrating Treg Cells and Outcome Prediction of Gastric Cancer. Ann Transl Med (2020) 8(7):469. doi: 10.21037/atm.2020.03.114
34. Chen X, Yang Z, Yang J, Liao Y, Pang P, Fan W, et al. Radiomics Analysis of Contrast-Enhanced CT Predicts Lymphovascular Invasion and Disease Outcome in Gastric Cancer: A Preliminary Study. Cancer Imaging (2020) 20(1):24. doi: 10.1186/s40644-020-00302-5
35. Wang N, Wang X, Li W, Ye H, Bai H, Wu J, et al. Contrast-Enhanced CT Parameters of Gastric Adenocarcinoma: Can Radiomic Features Be Surrogate Biomarkers for HER2 Over-Expression Status? Cancer Manag Res (2020) 12:1211–9. doi: 10.2147/CMAR.S230138
36. Gao X, Ma T, Cui J, Zhang Y, Wang L, Li H, et al. A Radiomics-Based Model for Prediction of Lymph Node Metastasis in Gastric Cancer. Eur J Radiol (2020) 129:109069. doi: 10.1016/j.ejrad.2020.109069
37. Li J, Dong D, Fang M, Wang R, Tian J, Li H, et al. Dual-Energy CT–Based Deep Learning Radiomics Can Improve Lymph Node Metastasis Risk Prediction for Gastric Cancer. Eur Radiol (2020) 30(4):2324–33. doi: 10.1007/s00330-019-06621-x
38. Wang Y, Liu W, Yu Y, Liu JJ, Xue HD, Qi YF, et al. CT Radiomics Nomogram for the Preoperative Prediction of Lymph Node Metastasis in Gastric Cancer. Eur Radiol (2020) 30(2):976–86. doi: 10.1007/s00330-019-06398-z
39. Wang Y, Liu W, Yu Y, Han W, Liu JJ, Xue HD, et al. Potential Value of CT Radiomics in the Distinction of Intestinal-Type Gastric Adenocarcinomas. Eur Radiol (2020) 30(5):2934–44. doi: 10.1007/s00330-019-06629-3
40. Liu S, He J, Liu S, Ji C, Guan W, Chen L, et al. Radiomics Analysis Using Contrast-Enhanced CT for Preoperative Prediction of Occult Peritoneal Metastasis in Advanced Gastric Cancer. Eur Radiol (2020) 30(1):239–46. doi: 10.1007/s00330-019-06368-5
41. Yardimci AH, Sel I, Bektas CT, Yarikkaya E, Dursun N, Bektas H, et al. Computed Tomography Texture Analysis in Patients With Gastric Cancer: A Quantitative Imaging Biomarker for Preoperative Evaluation Before Neoadjuvant Chemotherapy Treatment. Jpn J Radiol (2020) 38(6):553–60. doi: 10.1007/s11604-020-00936-2
42. Yang J, Wu Q, Xu L, Wang Z, Su K, Liu R, et al. Integrating Tumor and Nodal Radiomics to Predict Lymph Node Metastasis in Gastric Cancer. Radiother Oncol (2020) 150:89–96. doi: 10.1016/j.radonc.2020.06.004
43. Sun K-Y, Hu H-T, Chen S-L, Ye J-N, Li G-H, Chen L-D, et al. CT-Based Radiomics Scores Predict Response to Neoadjuvant Chemotherapy and Survival in Patients With Gastric Cancer. BMC Cancer (2020) 20(1):468. doi: 10.1186/s12885-020-06970-7
44. Zhang W, Fang M, Dong D, Wang X, Ke X, Zhang L, et al. Development and Validation of a CT-Based Radiomic Nomogram for Preoperative Prediction of Early Recurrence in Advanced Gastric Cancer. Radiother Oncol (2020) 145:13–20. doi: 10.1016/j.radonc.2019.11.023
45. Jiang Y, Wang H, Wu J, Chen C, Yuan Q, Huang W, et al. Noninvasive Imaging Evaluation of Tumor Immune Microenvironment to Predict Outcomes in Gastric Cancer. Ann Oncol (2020) 31(6):760–8. doi: 10.1016/j.annonc.2020.03.295
46. Zhang L, Dong D, Zhang W, Hao X, Fang M, Wang S, et al. A Deep Learning Risk Prediction Model for Overall Survival in Patients With Gastric Cancer: A Multicenter Study. Radiother Oncol (2020) 150:73–80. doi: 10.1016/j.radonc.2020.06.010
47. Wang XX, Ding Y, Wang SW, Dong D, Li HL, Chen J, et al. Intratumoral and Peritumoral Radiomics Analysis for Preoperative Lauren Classification in Gastric Cancer. Cancer Imaging (2020) 20(1):83. doi: 10.1186/s40644-020-00358-3
48. Feng B, Huang L, Li C, Quan Y, Chen Y, Xue H, et al. A Heterogeneity Radiomic Nomogram for Preoperative Differentiation of Primary Gastric Lymphoma From Borrmann Type IV Gastric Cancer. J Comput Assist Tomogr (2021) 45(2):191–202. doi: 10.1097/RCT.0000000000001117
49. Sun YW, Ji CF, Wang H, He J, Liu S, Ge Y, et al. Differentiating Gastric Cancer and Gastric Lymphoma Using Texture Analysis (TA) of Positron Emission Tomography (PET). Chin Med J (Engl) (2020) 134(4):439–47. doi: 10.1097/CM9.0000000000001206
50. Wang R, Liu H, Liang P, Zhao H, Li L, Gao J. Radiomics Analysis of CT Imaging for Differentiating Gastric Neuroendocrine Carcinomas From Gastric Adenocarcinomas. Eur J Radiol (2021) 138:109662. doi: 10.1016/j.ejrad.2021.109662
51. Sun RJ, Fang MJ, Tang L, Li XT, Lu QY, Dong D, et al. CT-Based Deep Learning Radiomics Analysis for Evaluation of Serosa Invasion in Advanced Gastric Cancer. Eur J Radiol (2020) 132:109277. doi: 10.1016/j.ejrad.2020.109277
52. Jiang Y, Liang X, Wang W, Chen C, Yuan Q, Zhang X, et al. Noninvasive Prediction of Occult Peritoneal Metastasis in Gastric Cancer Using Deep Learning. JAMA Netw Open (2021) 4(1):e2032269. doi: 10.1001/jamanetworkopen.2020.32269
53. Wang S, Feng C, Dong D, Li H, Zhou J, Ye Y, et al. Preoperative Computed Tomography-Guided Disease-Free Survival Prediction in Gastric Cancer: A Multicenter Radiomics Study. Med Phys (2020) 47(10):4862–71. doi: 10.1002/mp.14350
54. Parmar C, Rios Velazquez E, Leijenaar R, Jermoumi M, Carvalho S, Mak RH, et al. Robust Radiomics Feature Quantification Using Semiautomatic Volumetric Segmentation. PloS One (2014) 9(7):e102107. doi: 10.1371/journal.pone.0102107
55. Guyon I, Elisseeff A. An Introduction to Variable and Feature Selection. J Mach Learn Res (2003) 3:1157–82. doi: 10.1162/153244303322753616
56. Amin MB, Greene FL, Edge SB, Compton CC, Gershenwald JE, Brookland RK, et al. The Eighth Edition AJCC Cancer Staging Manual: Continuing to Build a Bridge From a Population-Based to a More “Personalized” Approach to Cancer Staging. CA: Cancer J Clin (2017) 67(2):93–9. doi: 10.3322/caac.21388
57. In H, Solsky I, Palis B, Langdon-Embry M, Ajani J, Sano T. Validation of the 8th Edition of the AJCC TNM Staging System for Gastric Cancer Using the National Cancer Database. Ann Surg Oncol (2017) 24(12):3683–91. doi: 10.1245/s10434-017-6078-x
58. Coccolini F, Nardi M, Montori G, Ceresoli M, Celotti A, Cascinu S, et al. Neoadjuvant Chemotherapy in Advanced Gastric and Esophago-Gastric Cancer. Meta-Analysis of Randomized Trials. Int J Surg (2018) 51:120–7. doi: 10.1016/j.ijsu.2018.01.008
59. Kodera Y. Neoadjuvant Chemotherapy for Gastric Adenocarcinoma in Japan. Surg Today (2017) 47(8):899–907. doi: 10.1007/s00595-017-1473-2
60. Wang X, Sun J, Zhang W, Yang X, Zhu C, Pan B, et al. Use of Radiomics to Extract Splenic Features to Predict Prognosis of Patients With Gastric Cancer. Eur J Surg Oncol (2020) 46(10 Pt A):1932–40. doi: 10.1016/j.ejso.2020.06.021
61. Park JE, Kim D, Kim HS, Park SY, Kim JY, Cho SJ, et al. Quality of Science and Reporting of Radiomics in Oncologic Studies: Room for Improvement According to Radiomics Quality Score and TRIPOD Statement. Eur Radiol (2020) 30(1):523–36. doi: 10.1007/s00330-019-06360-z
62. Lambin P, Leijenaar RTH, Deist TM, Peerlings J, de Jong EEC, van Timmeren J, et al. Radiomics: The Bridge Between Medical Imaging and Personalized Medicine. Nat Rev Clin Oncol (2017) 14(12):749–62. doi: 10.1038/nrclinonc.2017.141
63. Moons KG, Altman DG, Reitsma JB, Ioannidis JP, Macaskill P, Steyerberg EW, et al. Transparent Reporting of a Multivariable Prediction Model for Individual Prognosis or Diagnosis (TRIPOD): Explanation and Elaboration. Ann Intern Med (2015) 162(1):W1–73. doi: 10.7326/M14-0698
64. Shu Y, Zhang W, Hou Q, Zhao L, Zhang S, Zhou J, et al. Prognostic Significance of Frequent CLDN18-ARHGAP26/6 Fusion in Gastric Signet-Ring Cell Cancer. Nat Commun (2018) 9(1):1–11. doi: 10.1038/s41467-018-04907-0
65. Network CGAR. Comprehensive Molecular Characterization of Gastric Adenocarcinoma. Nature (2014) 513(7517):202. doi: 10.1038/nature13480
Keywords: gastric cancer, artificial intelligence, deep learning, hand-crafted radiomics, methodologies, clinical applications and challenges
Citation: Qin Y, Deng Y, Jiang H, Hu N and Song B (2021) Artificial Intelligence in the Imaging of Gastric Cancer: Current Applications and Future Direction. Front. Oncol. 11:631686. doi: 10.3389/fonc.2021.631686
Received: 20 November 2020; Accepted: 07 July 2021;
Published: 21 July 2021.
Edited by:
Changqiang Wu, North Sichuan Medical College, ChinaReviewed by:
Subathra Adithan, Jawaharlal Institute of Postgraduate Medical Education and Research (JIPMER), IndiaHsin Wu Tseng, University of Arizona, United States
Copyright © 2021 Qin, Deng, Jiang, Hu and Song. This is an open-access article distributed under the terms of the Creative Commons Attribution License (CC BY). The use, distribution or reproduction in other forums is permitted, provided the original author(s) and the copyright owner(s) are credited and that the original publication in this journal is cited, in accordance with accepted academic practice. No use, distribution or reproduction is permitted which does not comply with these terms.
*Correspondence: Na Hu, bmFodV93Y2hAMTYzLmNvbQ==; Bin Song, YW5pY2Vzb25nQHZpcC5zaW5hLmNvbQ==
†These authors have contributed equally to this work