- 1Department of Radiation Oncology, The Netherlands Cancer Institute, Amsterdam, Netherlands
- 2Department of Radiation Oncology, University of California, Los Angeles, CA, United States
MRI-guided radiotherapy systems have the potential to bring two important concepts in modern radiotherapy together: adaptive radiotherapy and biological targeting. Based on frequent anatomical and functional imaging, monitoring the changes that occur in volume, shape as well as biological characteristics, a treatment plan can be updated regularly to accommodate the observed treatment response. For this purpose, quantitative imaging biomarkers need to be identified that show changes early during treatment and predict treatment outcome. This review provides an overview of the current evidence on quantitative MRI measurements during radiotherapy and their potential as an imaging biomarker on MRI-guided radiotherapy systems.
Introduction
At the turn of the century, two novel concepts were introduced in radiation oncology that acknowledged the complexity of tumor biology and that presented the challenges that must be met to improve the outcome of radiotherapy. Recognizing that tumors can respond rapidly to fractionated treatment, Yan et al. introduced the concept of adaptive radiation therapy (1). Instead of delivering the entire treatment with a single treatment plan based on pre-treatment imaging, the proposal was to create a closed-loop process where the treatment plan could be modified based on observed changes in the patient. To date, with state-of-the-art linear accelerators, on-board imaging equipment and software for image processing and treatment planning, we see this concept come to fruition (2, 3). The second concept, introduced by Ling et al., addressed the biological heterogeneity of a tumor (4). Using biological images that reveal metabolic, functional, physiological, genotypic, and phenotypic data, a biological target volume could be defined. This could be used to ‘paint’ a dose distribution that matched the biological heterogeneity. Since then, many imaging biomarker studies have been conducted, essentially trying to establish how radiosensitivity can be visualized non-invasively (5). It was shown that while tumors indeed are quite heterogeneous, this heterogeneity changes during the course of fractionated radiotherapy (6, 7).
At this stage, it becomes clear that, considering the biological characteristics of the tumor as well as its dynamic nature during treatment, the two concepts of biological targeting and adaptive radiotherapy need to be merged. Based on frequent imaging, monitoring the changes that occur in volume, shape as well as biological characteristics, a treatment plan can be updated regularly to accommodate the observed response (8). While the logistical challenges for biological image-guided adaptive radiotherapy (BIGART) made the concept almost infeasible to carry out in practice, the emergence of MRI-guided radiotherapy (MRIgRT) platforms may be a game changer (9, 10).
For this purpose, imaging biomarkers need to be identified that show changes early during treatment and predict treatment outcome. Quantitative MRI (qMRI) techniques can be used to assess tumor morphology, biology and function. Therefore, they are promising imaging biomarkers for BIGART (9). In this review, we summarize the current evidence on repeated qMRI measurements during radiotherapy and the potential for such an approach with MRIgRT systems.
Quantitative Manetic Resonance Imaging Biomarkers
The majority of MRI biomarker studies investigate the potential of a measurement prior to the onset of treatment to predict outcome (11–13). In addition, promising evidence has emerged showing changes in qMRI values during radiotherapy. This suggests that qMRI parameters are prognostic for outcome and might be potential biomarkers for BIGART (9). In this section the literature is discussed in which measurements during the course of radiotherapy were reported (Table 1). Studies with only pre- and post-treatment measurements were out of the scope of this review.
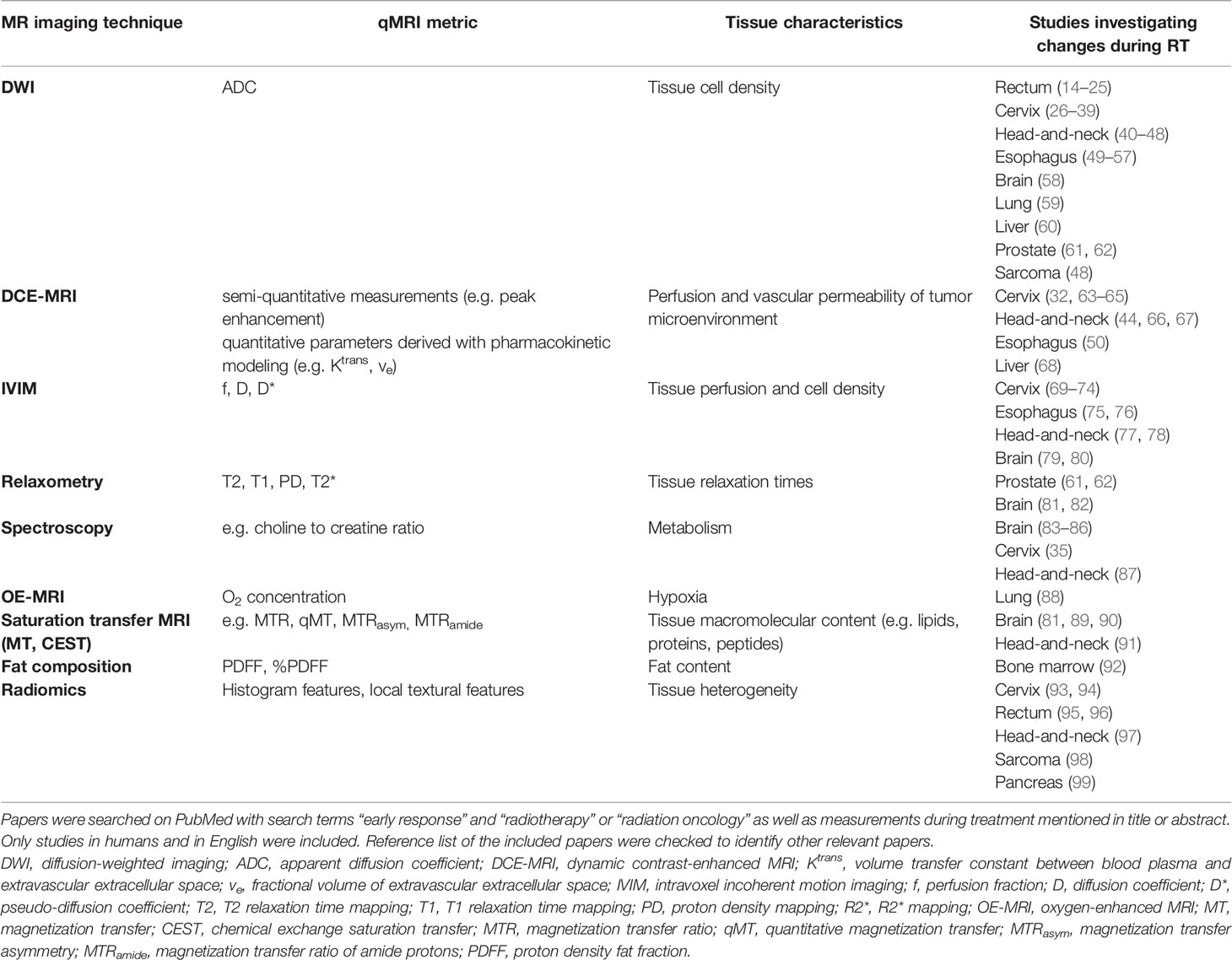
Table 1 Summary of MR imaging techniques for which changes during the course of radiotherapy have been investigated.
Diffusion weighted imaging (DWI) has been the most investigated technique so far. The apparent diffusion coefficient (ADC) derived from DWI data has been associated with the cell density of the tissue. Radiotherapy results in breakdown of cellular membranes and finally necrosis (13, 100). As a result the cell density is reduced, which will be observed as an increase in ADC. For many tumor sites, changes in ADC parameters early during radiotherapy have been reported, including rectal cancer (14–20), cervical cancer (26–38), head and neck cancer (40–47), esophageal cancer (49–56), brain cancer (58), lung cancer (59), and liver cancer (60). The majority of the studies report a larger increase in average ADC values for responders compared to non-responders (15–20, 29, 33, 36, 41, 44–47, 49, 51, 54–56, 60). Some studies observed a significant increase for responders and not for non-responders (14, 35, 43). Only a few studies did not observe a significant difference in the changes in ADC values between responders and non-responders (34, 52). For example, in a study with 108 cervical cancer patients there was no difference in the increase in ADC values between complete and partial responders (34).
Dynamic contrast-enhanced (DCE-) MRI indirectly measures the tissue perfusion and vascular permeability of the tumor microenvironment and has been proposed as a biomarker for radiotherapy (101, 102). The enhancement reflects the abnormal microvasculature in tumors (102). Changes during treatment in DCE-MRI have been investigated to a lesser extent than DWI. Most studies have been performed for cervical cancer (32, 63–65). One of the first studies showed with a semi-quantitative analysis that an increase in enhancement early during treatment was predictive for local recurrence (63). Gong et al. observed similar results, as they found a significant relation between the change in mean enhancement and tumor regression rate (64). This was confirmed in a larger patient population showing that patients with an improved perfusion during treatment have a more favorable outcome (65). Quantitative analysis of DCE-MRI data showed an increase in Ktrans (volume transfer constant between blood plasma and extravascular extracellular space) and ve (fractional volume of extravascular extracellular space) during treatment, both in week 1 and week 4 (32). Ktrans decreased 1 month after treatment again. The changes in Ktrans and ve during treatment were not correlated to changes in tumor volume. In a small group of head and neck cancer patients a larger increase in Ktrans and ve was observed in responders than in non-responders (44). Similarly Baer et al. reported that changes in Ktrans and the area under the curve were predictive for survival (66). In addition, patients that have large persistent subvolumes with low blood volume within the primary tumor have a higher probability of local failure (67). For esophageal cancer, a decrease in Ktrans was reported in complete responders (50). For liver metastases, an increase in slope and peak at week 2 was associated with an improved local response (68).
A limitation of DCE is that contrast agent needs to be injected intravenously. This could present logistical challenges and might not be amenable for repeated imaging. Alternatively, intravoxel incoherent motion (IVIM), based on multi-b-value diffusion, has been investigated for probing microscopic perfusion (103). By modeling the diffusion data with a perfusion component that predominantly affects low b-value data, a surrogate for tissue perfusion can be calculated (104). Studies in cervical cancer have reported changes in IVIM parameters during treatment (69–74). The perfusion fraction (f) first increased early during treatment and decreased later during treatment (72). Early increases in f have been associated with good response (70, 73). In esophageal cancers, responders showed a larger mid-treatment increase in the diffusion coefficient (D) of the tumor compared to non-responders (75, 76). Head-and-neck cancer patients with regional failure showed higher D values and larger reductions in f than patients with regional control (77).
For other qMRI techniques changes during treatment have been investigated only on a small scale so far. Spectroscopy has mainly been applied in brain (83–86). Changes in choline and lactate metrics during treatment were significantly related to outcome in patients with glioblastoma (83) and glioma (84). In two other studies only changes after treatment were significantly related to outcome (85, 86). For cervical cancer, changes in choline metrics could not predict treatment outcome (35). For head-and-neck cancer, choline metrics were stable in the first two weeks of treatment in responders and non-responders (87). Magnetization transfer (MT) and chemical exchange saturation transfer imaging (CEST) can be used to characterize the macromolecular content of tissue (105, 106). Changes during treatment have been investigated in glioblastoma (81, 89, 90) and head-and-neck cancer (91). All studies demonstrate the promising value of MT or CEST parameters as possible biomarkers for BIGART. Another promising technique is oxygen-enhanced MRI requiring an oxygen challenge (107). This technique was used in lung cancer patients to assess the hypoxic volume in the tumor (88). In the second week of the treatment the hypoxic volume was smaller than before treatment. Fat quantification could be useful to assess changes in tissue composition. For example, changes in fat fraction were correlated with changes in bone marrow composition induced by radiotherapy (92), which could be useful to assess hematologic toxicity. A few studies have looked into the potential of radiomics, where textural features derived from anatomical or functional images were tested (93–95, 97). Recently, deep learning approaches have been applied to extract information from images during treatment for response prediction (95, 108).
The evidence so far is mostly based on one or two measurements during treatment. Only a few studies used more than two measurements during treatment (Table 2). The study of Sun et al. showed in a population with mixed tumor sites that changes in ADC were correlated with treatment response and independent of tumor location (21). After the first week of treatment significant differences between responders and non-responders were observed, while a change in tumor size was not visible that early. In a study with cervical cancer patients, measurement of ADC at two weeks seemed optimal for monitoring early treatment response (39). Similar results were found for esophageal cancer (57) and rectal cancer (22). In contrast, a study with nine rectal cancer patients reported a decrease in ADC from week 2 onwards (23). Two studies investigated weekly changes in T2 and ADC values during treatment of prostate cancer (61, 62). While there were differences in overall treatment duration between the two studies, both studies did not observe early changes in either T2 or ADC. Only late ADC changes for the tumor were observed. However, the relation with treatment outcome was not assessed. A study in head-and-neck cancer patients investigated whether changes in IVIM parameters were visible during treatment (78). They showed a significant increase in ADC and D during treatment for patients with complete response. No significant differences were observed for the other IVIM parameters in the complete responding or non-responding patients.
Up to this moment, only three studies performed daily measurements during treatment in humans (24, 79, 80). Mahmood et al. performed daily IVIM measurements in patients with brain metastases. They showed that the mean ADC increased for patients with responding brain metastases and decreased for non-responding metastases (79). From fraction seven onwards the distinction between responders and non-responders became more pronounced. The IVIM parameters, perfusion fraction f and pseudo-diffusion coefficient D*, did not show significant prognostic value. In another study, they showed that the size of the viable tumor delineated on DWI images and the ADC value of the viable tumor are a better predictor for outcome than the change in tumor size delineated on anatomical images (80). In a small, but unique, study with 8 rectal cancer patients, ADC values during treatment overlapped between complete and partial responders (24). Therefore, no significant differences in ADC dynamics were observed between the two groups.
The small number of studies with multiple measurements per patient may be explained by logistical challenges and the cost of MRI exams beyond standard-of-care. Here, the MRIgRT systems provide an opportunity. For patients who are treated on an MRIgRT system the logistical barrier is much lower as it only requires some prolonged time for imaging on the table (9). In fact, as the online adaptive workflow on MRIgRT systems takes up some time, quantitative imaging can be acquired during this time period, avoiding an increase in overall time on the table. As MRIgRT systems have been introduced in clinical practice recently (10), only a few qMRI studies have been performed so far. Feasibility of qMRI on MRIgRT systems was first demonstrated in a pilot DWI study. In this study, longitudinal DWI was acquired from a cohort of patients with head-and-neck cancer and sarcoma every 2-5 fractions throughout their treatment courses with different ADC change patterns observed (48). In a similar way, the feasibility of DWI for response assessment was shown in three rectal cancer patients (25). A pilot with four patients with brain tumors showed that changes in T1, R2* and proton density maps were detectable during the course of treatment (82). In addition, a few studies assessed the feasibility of using radiomic features to monitor response during treatment (96, 98, 99). For sarcoma patients it was shown that radiomic features derived from longitudinal DWI can be used to predict post-surgery tumor necrosis score after radiotherapy (98). The study of Boldrini et al. illustrated that changes in radiomic features during treatment have the potential to predict clinical complete response in rectal cancer (96). In addition, a pilot study showed that radiomic features could predict outcome for patients with pancreatic cancer treated with stereotactic ablative body radiotherapy on an MRIgRT system (99).
Technical Validation
To integrate an MRI and a linear accelerator, modifications have been made to the MRI scanners in these systems. As a result, their technical specifications differ considerably from those of diagnostic systems. For the MRIdian (Viewray Technologies Inc. USA), the on-board MRI is a split bore superconducting magnet with a field strength of 0.35 T (109, 110). There is a 28 cm gap in between to reduce the number of MR components being in the radiation beam pathway. In case of the Unity system (Elekta AB, Sweden), the field strength is 1.5 T, but the gradient coils are physically split to create a radiation window (111). The 2 x 4 channel receive coil is radiolucent with all electronic components at the edges of the coil (111). The reduced signal-to-noise ratio and gradient performance for both systems put constraints on the acquisition protocols and the performance of qMRI measurements. Therefore, first efforts have been taken to assess the performance of these measurements on MRIgRT systems with phantoms (48, 82, 112–114). For the MRIdian 0.35T MRI, a few DWI studies have been performed, demonstrating the ADC accuracy and reproducibility, as well as improving DWI spatial integrity (48, 112). Studies of Nejad-Davarini et al. (82) and Bydder et al. (113) also explored feasibility and accuracy of T1 mapping, R2* mapping, proton density mapping, and proton density fat fraction using MRIdian. A multicenter study showed that consistent ADC, T1, T2, and DCE values can be measured across institutes with a Unity system (114). The accuracy of the techniques was similar to previously reported literature on diagnostic scanners. In addition, the feasibility of these qMRI techniques was demonstrated for a prostate cancer patient. Phantom measurements showed that accurate ADC values can be obtained within a 7 cm radius of the iso-center (115). Outside this region, ADC values deviated more than 5%. To increase the time window during which qMRI data can be acquired, the effect of image acquisition during irradiation has also been investigated. Phantom images acquired during gantry rotation were negligibly different from images with a static gantry (116). However, bulk shifts in the order of one pixel were observed and the extent of the phantom was gantry angle dependent. Therefore, DWI with an echo planar imaging sequence may require special attention to geometrical shifts and distortions. With test-retest measurements in prostate cancer patients it was shown that the rotating gantry did not affect the repeatability of ADC measurements (115).
Discussion
With BIGART two important concepts in radiotherapy are brought together. Recognizing the dynamic heterogeneity of a tumor during radiotherapy and adapting the treatment to the changing characteristics may widen the therapeutic window between tumor control and treatment-related toxicity. Although the two concepts have been around for over two decades, only now the technology is available to integrate daily biological imaging with online treatment adaptation. While many qMRI biomarker studies have been conducted, many more steps need to be taken before BIGART on MRIgRT systems becomes routine practice.
From a clinical perspective, the first step will be to investigate daily changes in qMRI values in different tumor sites. Multicenter observational trials should be initiated to validate these findings. In particular, it is important to investigate which qMRI techniques are suitable candidates for BIGART (117, 118). Based on the current and mostly consistent evidence, DWI seems to be a logical first choice to investigate further. The potential of DCE needs to be established, but might be very useful in certain applications (102). IVIM is an attractive alternative to study perfusion as it avoids administration of a contrast agent. Although previous studies observed a weak to moderate correlation between DCE and IVIM parameters (119–123), for BIGART it might be sufficient if similar trends are visible in the IVIM and DCE parameters. Other qMRI techniques are also promising, but must be investigated with larger populations. As different qMRI techniques reflect different aspects of tumor biology, a combination of techniques might give complimentary information with a higher predictive value for early treatment response (50, 53). Another open issue is the time scale at which changes in qMRI values happen during treatment. Some studies have reported changes early during treatment, others later. Monitoring changes on a daily basis, will help characterize this further. In addition, this will also reveal whether changes are homogeneous at group level (e.g. responder or non-responder groups), whether the time scale of the changes differs on patient-level or even differs within the tumor of the same patient. Furthermore, the relevance of observed changes in relation to treatment outcome (e.g. survival, recurrence, toxicity) needs to be established in order to identify if a biomarker potentially is predictive and suitable for BIGART.
Technical validation (124–126) of qMRI measurements on MRIgRT systems is required to ensure that the results are also relevant outside the MRIgRT domain, in particular because the MR-part of the MRIgRT systems is different from diagnostic systems. Digital and physical phantoms can be used to assess the accuracy and reproducibility of the qMRI measurements (127–134). Furthermore, to know which changes in qMRI values can be attributed to the effect of the treatment, assessment of the repeatability of the measurements should be performed with test-retest studies (125). Standardization of qMRI protocols could assist to improve reproducibility across participating centers (115).
In conclusion, MRIgRT systems have the potential to bring adaptive radiotherapy and biological targeting together in practice. The first step will be to investigate daily changes in qMRI values in different tumor sites, validated in a multicenter setting. Then, interventional studies become feasible to investigate the potential of qMRI as a biomarker for BIGART.
Author Contributions
PH and UH contributed to the conception and design of the review paper. All authors contributed to the article and approved the submitted version.
Conflict of Interest
The Netherlands Cancer Institute is a member of the Elekta Unity consortium and receives research funding from Elekta AB (Sweden) and Philips Healthcare (the Netherlands).
The authors declare that the research was conducted in the absence of any commercial or financial relationships that could be construed as a potential conflict of interest.
References
1. Yan D, Vicini F, Wong J, Martinez A. Adaptive radiation therapy. Phys Med Biol (1997) 42:123–32. doi: 10.1088/0031-9155/42/1/008
2. Morgan HE, Sher DJ. Adaptive radiotherapy for head and neck cancer. Cancers Head Neck (2020) 5:1. doi: 10.1186/s41199-019-0046-z
3. Yan D. Adaptive Radiotherapy: Merging Principle Into Clinical Practice. Semin Radiat Oncol (2010) 20:79–83. doi: 10.1016/j.semradonc.2009.11.001
4. Ling CC, Humm J, Larson S, Amols H, Fuks Z, Leibel S, et al. Towards multidimensional radiotherapy (MD-CRT): biological imaging and biological conformality. Int J Radiat Oncol Biol Phys (2000) 47:551–60. doi: 10.1016/s0360-3016(00)00467-3
5. Baumann M, Krause M, Overgaard J, Debus J, Bentzen SM, Daartz J, et al. Radiation oncology in the era of precision medicine. Nat Rev Cancer (2016) 16:234–49. doi: 10.1038/nrc.2016.18
6. van der Heide UA, Thorwarth D. Quantitative Imaging for Radiation Oncology. Int J Radiat Oncol Biol Phys (2018) 102:683–6. doi: 10.1016/j.ijrobp.2018.06.012
7. Thorwarth D, Alber M. Implementation of hypoxia imaging into treatment planning and delivery. Radiother Oncol (2010) 97:172–5. doi: 10.1016/j.radonc.2010.05.012
8. Grégoire V, Guckenberger M, Haustermans K, Lagendijk JJW, Ménard C, Pötter R, et al. Image guidance in radiation therapy for better cure of cancer. Mol Oncol (2020) 14:1470–91. doi: 10.1002/1878-0261.12751
9. Hall WA, Paulson ES, van der Heide UA, Fuller CD, Raaymakers BW, Lagendijk JJW, et al. The transformation of radiation oncology using real-time magnetic resonance guidance: A review. Eur J Cancer (2019) 122:42–52. doi: 10.1016/j.ejca.2019.07.021
10. Pollard JM, Wen Z, Sadagopan R, Wang J, Ibbott GS. The future of image-guided radiotherapy will be MR guided. Br J Radiol (2017) 90:20160667. doi: 10.1259/bjr.20160667
11. Noij DP, de Jong MC, Mulders LGM, Marcus JT, de Bree R, Lavini C, et al. Contrast-enhanced perfusion magnetic resonance imaging for head and neck squamous cell carcinoma: A systematic review. Oral Oncol (2015) 51:124–38. doi: 10.1016/j.oraloncology.2014.10.016
12. Schurink NW, Lambregts DMJ, Beets-Tan RGH. Diffusion-weighted imaging in rectal cancer: current applications and future perspectives. Br J Radiol (2019) 92:20180655. doi: 10.1259/bjr.20180655
13. Tsien C, Cao Y, Chenevert T. Clinical Applications for Diffusion Magnetic Resonance Imaging in Radiotherapy. Semin Radiat Oncol (2014) 24:218–26. doi: 10.1016/j.semradonc.2014.02.004
14. Musio D, De Felice F, Magnante AL, Ciolina M, De Cecco CN, Rengo M, et al. Diffusion-weighted magnetic resonance application in response prediction before, during, and after neoadjuvant radiochemotherapy in primary rectal cancer carcinoma. BioMed Res Int (2013) 2013:1–5. doi: 10.1155/2013/740195
15. Barbaro B, Vitale R, Valentini V, Illuminati S, Vecchio FM, Rizzo G, et al. Diffusion-weighted magnetic resonance imaging in monitoring rectal cancer response to neoadjuvant chemoradiotherapy. Int J Radiat Oncol Biol Phys (2012) 83:594–9. doi: 10.1016/j.ijrobp.2011.07.017
16. Sun YS, Zhang XP, Tang L, Ji JF, Gu J, Cai Y, et al. Locally advanced rectal carcinoma treated with preoperative chemotherapy and radiation therapy: Preliminary analysis of diffusion-weighted MR imaging for early detection of tumor histopathologic downstaging. Radiology (2010) 254:170–8. doi: 10.1148/radiol.2541082230
17. Lambrecht M, Vandecaveye V, De Keyzer F, Roels S, Penninckx F, Van Cutsem E, et al. Value of diffusion-weighted magnetic resonance imaging for prediction and early assessment of response to neoadjuvant radiochemotherapy in rectal cancer: Preliminary results. Int J Radiat Oncol Biol Phys (2012) 82:863–70. doi: 10.1016/j.ijrobp.2010.12.063
18. Jacobs L, Intven M, van Lelyveld N, Philippens M, Burbach M, Seldenrijk K, et al. Diffusion-weighted MRI for Early Prediction of Treatment Response on Preoperative Chemoradiotherapy for Patients With Locally Advanced Rectal Cancer. Ann Surg (2016) 263:522–8. doi: 10.1097/SLA.0000000000001311
19. Delli Pizzi A, Cianci R, Genovesi D, Esposito G, Timpani M, Tavoletta A, et al. Performance of diffusion-weighted magnetic resonance imaging at 3.0T for early assessment of tumor response in locally advanced rectal cancer treated with preoperative chemoradiation therapy. Abdom Radiol (2018) 43:2221–30. doi: 10.1007/s00261-018-1457-8
20. Lambrecht M, Van Herck H, De Keyzer F, Vandecaveye V, Slagmolen P, Suetens P, et al. Redefining the target early during treatment. Can we visualize regional differences within the target volume using sequential diffusion weighted MRI? Radiother Oncol (2014) 110:329–34. doi: 10.1016/j.radonc.2013.09.023
21. Sun YS, Cui Y, Tang L, Qi LP, Wang N, Zhang XY, et al. Early evaluation of cancer response by a new functional biomarker: Apparent diffusion coefficient. Am J Roentgenol (2011) 197:23–9. doi: 10.2214/AJR.10.4912
22. Cai G, Xu Y, Zhu J, Gu WL, Zhang S, Ma XJ, et al. Diffusion-weighted magnetic resonance imaging for predicting the response of rectal cancer to neoadjuvant concurrent chemoradiation. World J Gastroenterol (2013) 19:5520–7. doi: 10.3748/wjg.v19.i33.5520
23. Hein PA, Kremser C, Judmaier W, Griebel J, Pfeiffer KP, Kreczy A, et al. Diffusion-weighted magnetic resonance imaging for monitoring diffusion changes in rectal carcinoma during combined, preoperative chemoradiation: Preliminary results of a prospective study. Eur J Radiol (2003) 45:214–22. doi: 10.1016/S0720-048X(02)00231-0
24. Bostel T, Dreher C, Wollschläger D, Mayer A, König F, Bickelhaupt S, et al. Exploring MR regression patterns in rectal cancer during neoadjuvant radiochemotherapy with daily T2- And diffusion-weighted MRI. Radiat Oncol (2020) 15:1–12. doi: 10.1186/s13014-020-01613-4
25. Shaverdian N, Yang Y, Hu P, Hart S, Sheng K, Lamb J, et al. Feasibility evaluation of diffusion-weighted imaging using an integrated MRI-radiotherapy system for response assessment to neoadjuvant therapy in rectal cancer. Br J Radiol (2017) 90:20160739. doi: 10.1259/bjr.20160739
26. Haack S, Tanderup K, Kallehauge JF, Mohamed SMI, Lindegaard JC, Pedersen EM, et al. Diffusion-weighted magnetic resonance imaging during radiotherapy of locally advanced cervical cancer - Treatment response assessment using different segmentation methods. Acta Oncol (Madr) (2015) 54:1535–42. doi: 10.3109/0284186X.2015.1062545
27. Meng J, Zhu L, Zhu L, Wang H, Liu S, Yan J, et al. Apparent diffusion coefficient histogram shape analysis for monitoring early response in patients with advanced cervical cancers undergoing concurrent chemo-radiotherapy. Radiat Oncol (2016) 11:1–9. doi: 10.1186/s13014-016-0715-6
28. Kim HS, Kim CK, Park BK, Huh SJ, Kim B. Evaluation of therapeutic response to concurrent chemoradiotherapy in patients with cervical cancer using diffusion-weighted MR imaging. J Magn Reson Imaging (2013) 37:187–93. doi: 10.1002/jmri.23804
29. Somoye G, Harry V, Semple S, Plataniotis G, Scott N, Gilbert FJ, et al. Early diffusion weighted magnetic resonance imaging can predict survival in women with locally advanced cancer of the cervix treated with combined chemo-radiation. Eur Radiol (2012) 22:2319–27. doi: 10.1007/s00330-012-2496-0
30. Liu Y, Bai R, Sun H, Liu H, Zhao X, Li Y. Diffusion-weighted imaging in predicting and monitoring the response of uterine cervical cancer to combined chemoradiation. Clin Radiol (2009) 64:1067–74. doi: 10.1016/j.crad.2009.07.010
31. Meng J, Zhu L, Zhu L, Ge Y, He J, Zhou Z, et al. Histogram analysis of apparent diffusion coefficient for monitoring early response in patients with advanced cervical cancers undergoing concurrent chemo-radiotherapy. Acta radiol (2017) 58:1400–8. doi: 10.1177/0284185117694509
32. Park JJ, Kim CK, Park SY, Simonetti AW, Kim EJ, Park BK, et al. Assessment of early response to concurrent chemoradiotherapy in cervical cancer: Value of diffusion-weighted and dynamic contrast-enhanced MR imaging. Magn Reson Imaging (2014) 32:993–1000. doi: 10.1016/j.mri.2014.05.009
33. Kuang F, Yan Z, Wang J, Rao Z. The value of diffusion-weighted MRI to evaluate the response to radiochemotherapy for cervical cancer. Magn Reson Imaging (2014) 32:342–9. doi: 10.1016/j.mri.2013.12.007
34. Valentini AL, Miccò M, Gui B, Giuliani M, Rodolfino E, Telesca AM, et al. The price study: The role of conventional and diffusion-weighted magnetic resonance imaging in assessment of locally advanced cervical cancer patients administered by chemoradiation followed by radical surgery. Eur Radiol (2018) 28:2425–35. doi: 10.1007/s00330-017-5233-x
35. Rizzo S, Buscarino V, Origgi D, Summers P, Raimondi S, Lazzari R, et al. Evaluation of diffusion-weighted imaging (DWI) and MR spectroscopy (MRS) as early response biomarkers in cervical cancer patients. Radiol Med (2016) 121:838–46. doi: 10.1007/s11547-016-0665-y
36. Makino H, Kato H, Furui T, Morishige KI, Kanematsu M. Predictive value of diffusion-weighted magnetic resonance imaging during chemoradiotherapy for uterine cervical cancer. J Obstet Gynaecol Res (2014) 40:1098–104. doi: 10.1111/jog.12276
37. Zhang Y, Chen JY, Xie CM, Mo YX, Liu XW, Liu Y, et al. Diffusion-weighted magnetic resonance imaging for prediction of response of advanced cervical cancer to chemoradiation. J Comput Assist Tomogr (2011) 35:102–7. doi: 10.1097/RCT.0b013e3181f6528b
38. Harry VN, Semple SI, Gilbert FJ, Parkin DE. Diffusion-weighted magnetic resonance imaging in the early detection of response to chemoradiation in cervical cancer. Gynecol Oncol (2008) 111:213–20. doi: 10.1016/j.ygyno.2008.07.048
39. Liu Y, Sun H, Bai R, Ye Z. Time-window of early detection of response to concurrent chemoradiation in cervical cancer by using diffusion-weighted MR imaging: A pilot study. Radiat Oncol (2015) 10:185. doi: 10.1186/s13014-015-0493-6
40. Hoang JK, Choudhury KR, Chang J, Craciunescu OI, Yoo DS, Brizel DM. Diffusion-weighted imaging for head and neck squamous cell carcinoma: Quantifying repeatability to understand early treatment-induced change. Am J Roentgenol (2014) 203:1104–8. doi: 10.2214/AJR.14.12838
41. King AD, Chow KK, Yu KH, Mo FKF, Yeung DKW, Yuan J, et al. Head and neck squamous cell carcinoma: Diagnostic performance of diffusion-weighted MR imaging for the prediction of treatment response. Radiology (2013) 266:531–8. doi: 10.1148/radiol.12120167
42. Schouten CS, de Bree R, van der Putten L, Noij DP, Hoekstra OS, Comans EFI, et al. Diffusion-weighted EPI- and HASTE-MRI and 18F-FDG-PET-CT early during chemoradiotherapy in advanced head and neck cancer. Quant Imaging Med Surg (2014) 4:239–50. doi: 10.3978/j.issn.2223-4292.2014.07.15
43. Galbán CJ, Mukherji SK, Chenevert TL, Meyer CR, Hamstra DA, Bland PH, et al. A Feasibility Study of Parametric Response Map Analysis of Diffusion-Weighted Magnetic Resonance Imaging Scans of Head and Neck Cancer Patients for Providing Early Detection of Therapeutic Efficacy. Transl Oncol (2009) 2:184–90. doi: 10.1593/tlo.09175
44. Wong KH, Panek R, Dunlop A, Mcquaid D, Riddell A, Welsh LC, et al. Changes in multimodality functional imaging parameters early during chemoradiation predict treatment response in patients with locally advanced head and neck cancer. Eur J Nucl Med Mol Imaging (2018) 45:759–67. doi: 10.1007/s00259-017-3890-2
45. Kim S, Loevner L, Quon H, Sherman E, Weinstein G, Kilger A, et al. Diffusion-weighted magnetic resonance imaging for predicting and detecting early response to chemoradiation therapy of squamous cell carcinomas of the head and neck. Clin Cancer Res (2009) 15:986–94. doi: 10.1158/1078-0432.CCR-08-1287
46. Hatakenaka M, Shioyama Y, Nakamura K, Yabuuchi H, Matsuo Y, Sunami S, et al. Apparent diffusion coefficient calculated with relatively high b-values correlates with local failure of head and neck squamous cell carcinoma treated with radiotherapy. Am J Neuroradiol (2011) 32:1904–10. doi: 10.3174/ajnr.A2610
47. Matoba M, Tuji H, Shimode Y, Toyoda I, Kuginuki Y, Miwa K, et al. Fractional change in apparent diffusion coefficient as an imaging biomarker for predicting treatment response in head and neck cancer treated with chemoradiotherapy. Am J Neuroradiol (2014) 35:379–85. doi: 10.3174/ajnr.A3706
48. Yang Y, Cao M, Sheng K, Gao Y, Chen A, Kamrava M, et al. Longitudinal diffusion MRI for treatment response assessment: Preliminary experience using an MRI-guided tri-cobalt 60 radiotherapy system. Med Phys (2016) 43:1369–73. doi: 10.1118/1.4942381
49. Chen Y, Xie T, Ye Z, Wang F, Long D, Jiang M, et al. ADC correlation with Sirtuin1 to assess early chemoradiotherapy response of locally advanced esophageal carcinoma patients. Radiat Oncol (2019) 14:1–9. doi: 10.1186/s13014-019-1393-y
50. Xie T, Ye Z, Pang P, Shao G. Quantitative Multiparametric MRI May Augment the Response to Radiotherapy in Mid-Treatment Assessment of Patients with Esophageal Carcinoma. Oncol Res Treat (2019) 42:326–33. doi: 10.1159/000499322
51. Borggreve AS, Goense L, van Rossum PSN, Heethuis SE, van Hillegersberg R, Lagendijk JJW, et al. Preoperative Prediction of Pathologic Response to Neoadjuvant Chemoradiotherapy in Patients With Esophageal Cancer Using 18F-FDG PET/CT and DW-MRI: A Prospective Multicenter Study. Int J Radiat Oncol Biol Phys (2020) 106:998–1009. doi: 10.1016/j.ijrobp.2019.12.038
52. Kwee RM, Dik AK, Sosef MN, Berendsen RCM, Sassen S, Lammering G, et al. Interobserver reproducibility of diffusion-weighted MRI in monitoring tumor response to neoadjuvant therapy in esophageal cancer. PloS One (2014) 9:e92211. doi: 10.1371/journal.pone.0092211
53. Heethuis SE, Goense L, van Rossum PSN, Borggreve AS, Mook S, Voncken FEM, et al. DW-MRI and DCE-MRI are of complementary value in predicting pathologic response to neoadjuvant chemoradiotherapy for esophageal cancer. Acta Oncol (Madr) (2018) 57:1201–8. doi: 10.1080/0284186X.2018.1473637
54. Van Rossum PSN, Van Lier ALHMW, Van Vulpen M, Reerink O, Lagendijk JJW, Lin SH, et al. Diffusion-weighted magnetic resonance imaging for the prediction of pathologic response to neoadjuvant chemoradiotherapy in esophageal cancer. Radiother Oncol (2015) 115:163–70. doi: 10.1016/j.radonc.2015.04.027
55. Imanishi S, Shuto K, Aoyagi T, Kono T, Saito H, Matsubara H. Diffusion-weighted magnetic resonance imaging for predicting and detecting the early response to Chemoradiotherapy of advanced esophageal Squamous cell carcinoma. Dig Surg (2013) 30:240–8. doi: 10.1159/000351435
56. Fang P, Musall BC, Son JB, Moreno AC, Hobbs BP, Carter BW, et al. Multimodal Imaging of Pathologic Response to Chemoradiation in Esophageal Cancer. Int J Radiat Oncol Biol Phys (2018) 102:996–1001. doi: 10.1016/j.ijrobp.2018.02.029
57. Wang L, Liu L, Han C, Liu S, Tian H, Li Z, et al. The diffusion-weighted magnetic resonance imaging (DWI) predicts the early response of esophageal squamous cell carcinoma to concurrent chemoradiotherapy. Radiother Oncol (2016) 121:246–51. doi: 10.1016/j.radonc.2016.10.021
58. Farjam R, Tsien CI, Feng FY, Gomez-Hassan D, Hayman JA, Lawrence TS, et al. Investigation of the diffusion abnormality index as a new imaging biomarker for early assessment of brain tumor response to radiation therapy. Neuro Oncol (2014) 16:131–9. doi: 10.1093/neuonc/not153
59. Weiss E, Ford JC, Olsen KM, Karki K, Saraiya S, Groves R, et al. Apparent diffusion coefficient (ADC) change on repeated diffusion-weighted magnetic resonance imaging during radiochemotherapy for non-small cell lung cancer: A pilot study. Lung Cancer (2016) 96:113–9. doi: 10.1016/j.lungcan.2016.04.001
60. Eccles CL, Haider EA, Haider MA, Fung S, Lockwood G, Dawson LA. Change in diffusion weighted MRI during liver cancer radiotherapy: Preliminary observations. Acta Oncol (Madr) (2009) 48:1034–43. doi: 10.1080/02841860903099972
61. Foltz WD, Wu A, Chung P, Catton C, Bayley A, Milosevic M, et al. Changes in apparent diffusion coefficient and T2 relaxation during radiotherapy for prostate cancer. J Magn Reson Imaging (2013) 37:909–16. doi: 10.1002/jmri.23885
62. van Schie MA, van Houdt PJ, Ghobadi G, Pos FJ, Walraven I, de Boer HCJ, et al. Quantitative MRI Changes During Weekly Ultra-Hypofractionated Prostate Cancer Radiotherapy With Integrated Boost. Front Oncol (2019) 9:1264. doi: 10.3389/fonc.2019.01264
63. Mayr NA, Yuh WTC, Magnotta VA, Ehrhardt JC, Wheeler JA, Sorosky JI, et al. Tumor perfusion studies using fast magnetic resonance imaging technique in advanced cervical cancer: A new noninvasive predictive assay. Int J Radiat Oncol Biol Phys (1996) 36:623–33. doi: 10.1016/S0360-3016(97)85090-0
64. Gong QY, Brunt JNH, Romaniuk CS, Oakley JP, Tan LT, Roberts N, et al. Contrast enhanced dynamic MRI of cervical carcinoma during radiotherapy: Early prediction of tumour regression rate. Br J Radiol (1999) 72:1177–84. doi: 10.1259/bjr.72.864.10703475
65. Mayr NA, Wang JZ, Zhang D, Grecula JC, Lo SS, Jaroura D, et al. Longitudinal Changes in Tumor Perfusion Pattern during the Radiation Therapy Course and its Clinical Impact in Cervical Cancer. Int J Radiat Oncol Biol Phys (2010) 77:502–8. doi: 10.1016/j.ijrobp.2009.04.084
66. Baer AH, Hoff BA, Srinivasan A, Galba CJ. Feasibility Analysis of the Parametric Response Map as an Early Predictor of Treatment Efficacy in Head and Neck Cancer. AJNR Am J Neuroradiol (2015) 36(4):757–62. doi: 10.3174/ajnr.A4296
67. Wang P, Popovtzer A, Eisbruch A, Cao Y. An approach to identify, from DCE MRI, significant subvolumes of tumors related to outcomes in advanced head-and-neck cancer. Med Phys (2012) 39:5277–85. doi: 10.1118/1.4737022
68. Liang P-C, Chang H-J, Hsu C, Tseng SS, Shih TTF, Wu Liu T. Dynamic MRI signals in the second week of radiotherapy relate to treatment outcomes of hepatocellular carcinoma: a preliminary result. Liver Int (2007) 27:516–28. doi: 10.1111/j.1478-3231.2007.01456.x
69. Gao S, Du S, Lu Z, Xin J, Gao S, Sun H. Multiparametric PET/MR (PET and MR-IVIM) for the evaluation of early treatment response and prediction of tumor recurrence in patients with locally advanced cervical cancer. Eur Radiol (2020) 30:1191–201. doi: 10.1007/s00330-019-06428-w
70. Kato H, Esaki K, Yamaguchi T, Tanaka H, Kajita K, Furui T, et al. Predicting early response to chemoradiotherapy for uterine cervical cancer using intravoxel incoherent motion mr imaging. Magn Reson Med Sci (2019) 18:293–8. doi: 10.2463/mrms.tn.2018-0138
71. Xu C, Sun H, Du S, Xin J. Early treatment response of patients undergoing concurrent chemoradiotherapy for cervical cancer: An evaluation of integrated multi-parameter PET-IVIM MR. Eur J Radiol (2019) 117:1–8. doi: 10.1016/j.ejrad.2019.05.012
72. Zhu L, Zhu L, Wang H, Yan J, Liu B, Chen W, et al. Predicting and early monitoring treatment efficiency of cervical cancer under concurrent chemoradiotherapy by intravoxel incoherent motion magnetic resonance imaging. J Comput Assist Tomogr (2017) 41:422–9. doi: 10.1097/RCT.0000000000000550
73. Bian H, Liu F, Chen S, Li G, Song Y, Sun M, et al. Intravoxel incoherent motion diffusion-weighted imaging evaluated the response to concurrent chemoradiotherapy in patients with cervical cancer. Med (Baltimore) (2019) 98:e17943. doi: 10.1097/MD.0000000000017943
74. Zhu L, Zhu L, Shi H, Wang H, Yan J, Liu B, et al. Evaluating early response of cervical cancer under concurrent chemo-radiotherapy by intravoxel incoherent motion MR imaging. BMC Cancer (2016) 16:1–8. doi: 10.1186/s12885-016-2116-5
75. Zheng H, Ren W, Pan X, Zhang Q, Liu B, Liu S, et al. Role of intravoxel incoherent motion MRI in early assessment of the response of esophageal squamous cell carcinoma to chemoradiotherapy: A pilot study. J Magn Reson Imaging (2018) 48:349–58. doi: 10.1002/jmri.25934
76. Li FP, Wang H, Hou J, Tang J, Lu Q, Wang LL, et al. Utility of intravoxel incoherent motion diffusion-weighted imaging in predicting early response to concurrent chemoradiotherapy in oesophageal squamous cell carcinoma. Clin Radiol (2018) 73:756.e17–756.e26. doi: 10.1016/j.crad.2018.03.015
77. Marzi S, Piludu F, Sanguineti G, Marucci L, Farneti A, Terrenato I, et al. The prediction of the treatment response of cervical nodes using intravoxel incoherent motion diffusion-weighted imaging. Eur J Radiol (2017) 92:93–102. doi: 10.1016/j.ejrad.2017.05.002
78. Paudyal R, Oh JH, Riaz N, Venigalla P, Li J, Hatzoglou V, et al. Intravoxel incoherent motion diffusion-weighted MRI during chemoradiation therapy to characterize and monitor treatment response in human papillomavirus head and neck squamous cell carcinoma. J Magn Reson Imaging (2017) 45:1013–23. doi: 10.1002/jmri.25523
79. Mahmood F, Johannesen HH, Geertsen P, Hansen RH. Repeated diffusion MRI reveals earliest time point for stratification of radiotherapy response in brain metastases. Phys Med Biol (2017) 62:2990–3002. doi: 10.1088/1361-6560/aa5249
80. Mahmood F, Hjorth Johannesen H, Geertsen P, Hansen RH. Diffusion MRI outlined viable tumour volume beats GTV in intra-treatment stratification of outcome. Radiother Oncol (2020) 144:121–6. doi: 10.1016/j.radonc.2019.11.012
81. Chan RW, Chen H, Myrehaug S, Atenafu EG, Stanisz GJ, Stewart J, et al. Quantitative CEST and MT at 1.5T for monitoring treatment response in glioblastoma: early and late tumor progression during chemoradiation. J Neurooncol (2020). doi: 10.1007/s11060-020-03661-y
82. Nejad-Davarani SP, Zakariaei N, Chen Y, Haacke EM, Hurst NJ, Salim Siddiqui M, et al. Rapid multicontrast brain imaging on a 0.35T MR-linac. Med Phys (2020) 47(9):4064–76. doi: 10.1002/mp.14251
83. Muruganandham M, Clerkin PP, Smith BJ, Anderson CM, Morris A, Capizzano AA, et al. 3-dimensional magnetic resonance spectroscopic imaging at 3 Tesla for early response assessment of glioblastoma patients during external beam radiation therapy. Int J Radiat Oncol Biol Phys (2014) 90:181–9. doi: 10.1016/j.ijrobp.2014.05.014
84. Alexander A, Murtha A, Abdulkarim B, Mehta V, Wheatley M, Murray B, et al. Prognostic significance of serial magnetic resonance spectroscopies over the course of radiation therapy for patients with malignant glioma. Clin Invest Med (2006) 29:301–11. doi: 10.1016/j.lungcan.2016.04.001
85. Nelson SJ, Li Y, Lupo JM, Olson M, Crane JC, Molinaro A, et al. Serial analysis of 3D H-1 MRSI for patients with newly diagnosed GBM treated with combination therapy that includes bevacizumab. J Neurooncol (2016) 130:171–9. doi: 10.1007/s11060-016-2229-3
86. Quon H, Brunet B, Alexander A, Murtha A, Abdulkarim B, Fulton D, et al. Changes in serial magnetic resonance spectroscopy predict outcome in high-grade glioma during and after postoperative radiotherapy. Anticancer Res (2011) 31:3559–65.
87. King AD, Yeung DKW, Yu KH, Mo FKF, Bhatia KS, Tse GMK, et al. Pretreatment and early intratreatment prediction of clinicopathologic response of head and neck cancer to chemoradiotherapy using 1H-MRS. J Magn Reson Imaging (2010) 32:199–203. doi: 10.1002/jmri.22224
88. Salem A, Little RA, Latif A, Featherstone AK, Babur M, Peset I, et al. Oxygen-enhanced MRI is feasible, repeatable, and detects radiotherapy-induced change in hypoxia in xenograft models and in patients with non-small cell lung cancer. Clin Cancer Res (2019) 25:3818–29. doi: 10.1158/1078-0432.CCR-18-3932
89. Mehrabian H, Myrehaug S, Soliman H, Sahgal A, Stanisz GJ. Quantitative Magnetization Transfer in Monitoring Glioblastoma (GBM) Response to Therapy. Sci Rep (2018) 8:1–11. doi: 10.1038/s41598-018-20624-6
90. Mehrabian H, Myrehaug S, Soliman H, Sahgal A, Stanisz GJ. Evaluation of Glioblastoma Response to Therapy With Chemical Exchange Saturation Transfer. Int J Radiat Oncol Biol Phys (2018) 101:713–23. doi: 10.1016/j.ijrobp.2018.03.057
91. Qamar S, King AD, Ai QY, Law BKH, Chan JSM, Poon DMC, et al. Amide proton transfer MRI detects early changes in nasopharyngeal carcinoma: providing a potential imaging marker for treatment response. Eur Arch Oto Rhino Laryngol (2019) 276:505–12. doi: 10.1007/s00405-018-5231-x
92. Carmona R, Pritz J, Bydder M, Gulaya S, Zhu H, Williamson CW, et al. Fat composition changes in bone marrow during chemotherapy and radiation therapy. Int J Radiat Oncol Biol Phys (2014) 90:155–63. doi: 10.1016/j.ijrobp.2014.05.041
93. Bowen SR, Yuh WTC, Hippe DS, Wu W, Partridge SC, Elias S, et al. Tumor radiomic heterogeneity: Multiparametric functional imaging to characterize variability and predict response following cervical cancer radiation therapy. J Magn Reson Imaging (2018) 47:1388–96. doi: 10.1002/jmri.25874
94. Prescott JW, Zhang D, Wang JZ, Mayr NA, Yuh WTC, Saltz J, et al. Temporal analysis of tumor heterogeneity and volume for cervical cancer treatment outcome prediction: Preliminary evaluation. J Digit Imaging (2010) 23:342–57. doi: 10.1007/s10278-009-9179-7
95. Shi L, Zhang Y, Nie K, Sun X, Niu T, Yue N, et al. Machine learning for prediction of chemoradiation therapy response in rectal cancer using pre-treatment and mid-radiation multi-parametric MRI. Magn Reson Imaging (2019) 61:33–40. doi: 10.1016/j.mri.2019.05.003
96. Boldrini L, Cusumano D, Chiloiro G, Casà C, Masciocchi C, Lenkowicz J, et al. Delta radiomics for rectal cancer response prediction with hybrid 0.35 T magnetic resonance-guided radiotherapy (MRgRT): a hypothesis-generating study for an innovative personalized medicine approach. Radiol Med (2019) 124:145–53. doi: 10.1007/s11547-018-0951-y
97. Scalco E, Marzi S, Sanguineti G, Vidiri A, Rizzo G. Characterization of cervical lymph-nodes using a multi-parametric and multi-modal approach for an early prediction of tumor response to chemo-radiotherapy. Phys Med (2016) 32:1672–80. doi: 10.1016/j.ejmp.2016.09.003
98. Gao Y, Kalbasi A, Hsu W, Ruan D, Fu J, Shao J, et al. Treatment effect prediction for sarcoma patients treated with preoperative radiotherapy using radiomics features from longitudinal diffusion-weighted MRI. Phys Med Biol (2020) 65(17):175006. doi: 10.1088/1361-6560/ab9e58
99. Simpson G, Spieler B, Dogan N, Portelance L, Mellon EA, Kwon D, et al. Predictive value of 0.35 T magnetic resonance imaging radiomic features in stereotactic ablative body radiotherapy of pancreatic cancer: A pilot study. Med Phys (2020) 47:3682–90. doi: 10.1002/mp.14200
100. Hamstra DA, Lee KC, Moffat BA, Chenevert TL, Rehemtulla A, Ross BD. Diffusion magnetic resonance imaging: An imaging treatment response biomarker to chemoradiotherapy in a mouse model of squamous cell cancer of the head and neck. Transl Oncol (2008) 1:187–94. doi: 10.1593/tlo.08166
101. Cao Y. The promise of dynamic contrast-enhanced imaging in radiation therapy. Semin Radiat Oncol (2011) 21:147–56. doi: 10.1016/j.semradonc.2010.11.001
102. Zahra MA, Hollingsworth KG, Sala E, Lomas DJ, Tan LT. Dynamic contrast-enhanced MRI as a predictor of tumour response to radiotherapy. Lancet Oncol (2007) 8:63–74. doi: 10.1016/S1470-2045(06)71012-9
103. Federau C. Intravoxel incoherent motion MRI as a means to measure in vivo perfusion: A review of the evidence. NMR BioMed (2017) 30:1–15. doi: 10.1002/nbm.3780
104. Iima M, Le Bihan D. Clinical Intravoxel Incoherent Motion and Diffusion MR Imaging: Past, Present, and Future. Radiology (2016) 278:13–32. doi: 10.1148/radiol.2015150244
105. Wu B, Warnock G, Zaiss M, Lin C, Chen M, Zhou Z, et al. An overview of CEST MRI for non-MR physicists. EJNMMI Phys (2016) 3:19. doi: 10.1186/s40658-016-0155-2
106. Mehrabian H, Detsky J, Soliman H, Sahgal A, Stanisz GJ. Advanced magnetic resonance imaging techniques in management of brain metastases. Front Oncol (2019) 9:440. doi: 10.3389/fonc.2019.00440
107. O’Connor JPB, Rose CJ, Waterton JC, Carano RAD, Parker GJM, Jackson A. Imaging Intratumor Heterogeneity: Role in Therapy Response, Resistance, and Clinical Outcome. Clin Cancer Res (2015) 21:249–57. doi: 10.1158/1078-0432.CCR-14-0990
108. Ferrari R, Mancini-Terracciano C, Voena C, Rengo M, Zerunian M, Ciardiello A, et al. MR-based artificial intelligence model to assess response to therapy in locally advanced rectal cancer. Eur J Radiol (2019) 118:1–9. doi: 10.1016/j.ejrad.2019.06.013
109. Mutic S, Dempsey JF. The ViewRay System: Magnetic Resonance-Guided and Controlled Radiotherapy. Semin Radiat Oncol (2014) 24:196–9. doi: 10.1016/j.semradonc.2014.02.008
110. Klüter S. Technical design and concept of a 0.35 T MR-Linac. Clin Transl Radiat Oncol (2019) 18:98–101. doi: 10.1016/j.ctro.2019.04.007
111. Lagendijk JJW, Raaymakers BW, van Vulpen M. The Magnetic Resonance Imaging-Linac System. Semin Radiat Oncol (2014) 24:207–9. doi: 10.1016/j.semradonc.2014.02.009
112. Gao Y, Han F, Zhou Z, Cao M, Kaprealian T, Kamrava M, et al. Distortion-free diffusion MRI using an MRI-guided Tri-Cobalt 60 radiotherapy system: Sequence verification and preliminary clinical experience. Med Phys (2017) 44:5357–66. doi: 10.1002/mp.12465
113. Bydder M, Kouzehkonan VG, Gao Y, Robson MD, Yang Y, Hu P. Constraints in estimating the proton density fat fraction. Magn Reson Imaging (2020) 66:1–8. doi: 10.1016/j.mri.2019.11.009
114. Kooreman ES, van Houdt PJ, Nowee ME, van Pelt VWJ, Tijssen RHN, Paulson ES, et al. Feasibility and accuracy of quantitative imaging on a 1.5 T MR-linear accelerator. Radiother Oncol (2019) 133:156–62. doi: 10.1016/j.radonc.2019.01.011
115. Kooreman ES, van Houdt PJ, Keesman R, Pos FJ, van Pelt VWJ, Nowee ME, et al. ADC measurements on the unity MR-linac – a recommendation on behalf of the elekta unity MR-linac consortium. Radiother Oncol (2020) 153:106–13. doi: 10.1016/j.radonc.2020.09.046
116. Jackson S, Glitzner M, Tijssen RHN, Raaymakers BW. MRI B 0 homogeneity and geometric distortion with continuous linac gantry rotation on an Elekta Unity MR-linac. Phys Med Biol (2019) 64:0–7. doi: 10.1088/1361-6560/ab231a
117. Datta A, Aznar MC, Dubec M, Parker GJM, O’Connor JPB. Delivering Functional Imaging on the MRI-Linac: Current Challenges and Potential Solutions. Clin Oncol (2018) 30:702–10. doi: 10.1016/j.clon.2018.08.005
118. Press RH, Shu HKG, Shim H, Mountz JM, Kurland BF, Wahl RL, et al. The Use of Quantitative Imaging in Radiation Oncology: A Quantitative Imaging Network (QIN) Perspective. Int J Radiat Oncol Biol Phys (2018) 102:1219–35. doi: 10.1016/j.ijrobp.2018.06.023
119. Pang Y, Turkbey B, Bernardo M, Kruecker J, Kadoury S, Merino MJ, et al. Intravoxel incoherent motion MR imaging for prostate cancer: an evaluation of perfusion fraction and diffusion coefficient derived from different b-value combinations. Magn Reson Med (2013) 69:553–62. doi: 10.1002/mrm.24277
120. Ioannidis GS, Nikiforaki K, Karantanas A. Statistical and spatial correlation between diffusion and perfusion MR imaging parameters: A study on soft tissue sarcomas. Phys Med (2019) 65:59–66. doi: 10.1016/j.ejmp.2019.08.007
121. Wang H, Zhu L, Li G, Zuo M, Ma X, Wang J. Perfusion parameters of intravoxel incoherent motion based on tumor edge region of interest in cervical cancer: evaluation of differentiation and correlation with dynamic contrast-enhanced MRI. Acta radiol (2020) 61:1087–95. doi: 10.1177/0284185119890086
122. Sun H, Xu Y, Xu Q, Duan J, Zhang H, Liu T, et al. Correlation Between Intravoxel Incoherent Motion and Dynamic Contrast-Enhanced Magnetic Resonance Imaging Parameters in Rectal Cancer. Acad Radiol (2019) 26:e134–40. doi: 10.1016/j.acra.2018.08.012
123. Yang X, Xiao X, Lu B, Chen Y, Wen Z, Yu S. Perfusion-sensitive parameters of intravoxel incoherent motion MRI in rectal cancer: evaluation of reproducibility and correlation with dynamic contrast-enhanced MRI. Acta radiol (2019) 60:569–77. doi: 10.1177/0284185118791201
124. O’Connor JPB, Aboagy EO, Adams JE, Aerts HJWL, Barrington SF, Beer AJ, et al. Imaging biomarker roadmap for cancer studies. Nat Rev Clin Oncol (2016) 14:169–86. doi: 10.1038/nrclinonc.2016.162
125. Shukla-Dave A, Obuchowski NA, Chenevert TL, Jambawalikar S, Schwartz LH, Malyarenko D, et al. Quantitative imaging biomarkers alliance (QIBA) recommendations for improved precision of DWI and DCE-MRI derived biomarkers in multicenter oncology trials. J Magn Reson Imaging (2019) 49:e101–21. doi: 10.1002/jmri.26518
126. Kurz C, Buizza G, Landry G, Kamp F, Rabe M, Paganelli C, et al. Medical physics challenges in clinical MR-guided radiotherapy. Radiat Oncol (2020) 15:1–16. doi: 10.1186/s13014-020-01524-4
127. Keenan KE, Biller JR, Delfino JG, Boss MA, Does MD, Evelhoch JL, et al. Recommendations towards standards for quantitative MRI (qMRI) and outstanding needs. J Magn Reson Imaging (2019) 49:e26–39. doi: 10.1002/jmri.26598
128. Ford J, Dogan N, Young L, Yang F. Quantitative Radiomics: Impact of Pulse Sequence Parameter Selection on MRI-Based Textural Features of the Brain. Contrast Media Mol Imaging (2018) 2018:1–9. doi: 10.1155/2018/1729071
129. Bianchini L, Santinha J, Loução N, Figueiredo M, Botta F, Origgi D, et al. A multicenter study on radiomic features from T2-weighted images of a customized MR pelvic phantom setting the basis for robust radiomic models in clinics. Magn Reson Med (2021) 85(3):1713–26. doi: 10.1002/mrm.28521
130. Malyarenko D, Galbán CJ, Londy FJ, Meyer CR, Johnson TD, Rehemtulla A, et al. Multi-system repeatability and reproducibility of apparent diffusion coefficient measurement using an ice-water phantom. J Magn Reson Imaging (2013) 37:1238–46. doi: 10.1002/jmri.23825
131. van Houdt PJ, Kallehauge JF, Tanderup K, Nout R, Zaletelj M, Tadic T, et al. Phantom-based quality assurance for multicenter quantitative MRI in locally advanced cervical cancer. Radiother Oncol (2020) 153:114–21. doi: 10.1016/j.radonc.2020.09.013
132. Rai R, Holloway LC, Brink C, Field M, Christiansen RL, Sun Y, et al. Multicenter evaluation of MRI-based radiomic features: A phantom study. Med Phys (2020) 47:3054–63. doi: 10.1002/mp.14173
133. Yang F, Dogan N, Stoyanova R, Ford JC. Evaluation of radiomic texture feature error due to MRI acquisition and reconstruction: A simulation study utilizing ground truth. Phys Med (2018) 50:26–36. doi: 10.1016/j.ejmp.2018.05.017
Keywords: quantitative magnetic resonance imaging (MRI), biological image-guided adaptive radiotherapy, magnetic resonance imaging (MRI)-guided radiotherapy, functional magnetic resonance imaging (MRI), treatment response
Citation: van Houdt PJ, Yang Y and van der Heide UA (2021) Quantitative Magnetic Resonance Imaging for Biological Image-Guided Adaptive Radiotherapy. Front. Oncol. 10:615643. doi: 10.3389/fonc.2020.615643
Received: 09 October 2020; Accepted: 08 December 2020;
Published: 29 January 2021.
Edited by:
Vincenzo Valentini, Catholic University of the Sacred Heart, ItalyReviewed by:
F. Yang, University of Miami, United StatesYaacov Lawrence, Sheba Medical Center, Israel
Copyright © 2021 van Houdt, Yang and van der Heide. This is an open-access article distributed under the terms of the Creative Commons Attribution License (CC BY). The use, distribution or reproduction in other forums is permitted, provided the original author(s) and the copyright owner(s) are credited and that the original publication in this journal is cited, in accordance with accepted academic practice. No use, distribution or reproduction is permitted which does not comply with these terms.
*Correspondence: Petra J. van Houdt, cC52LmhvdWR0QG5raS5ubA==