- 1Department of Breast and Thyroid Surgery, Renmin Hospital of Wuhan University, Wuhan, China
- 2School of Computer Science, Wuhan University, Wuhan, China
- 3Institute of Artificial Intelligence, Wuhan University, Wuhan, China
Thyroid cancers (TC) have increasingly been detected following advances in diagnostic methods. Risk stratification guided by refined information becomes a crucial step toward the goal of personalized medicine. The diagnosis of TC mainly relies on imaging analysis, but visual examination may not reveal much information and not enable comprehensive analysis. Artificial intelligence (AI) is a technology used to extract and quantify key image information by simulating complex human functions. This latent, precise information contributes to stratify TC on the distinct risk and drives tailored management to transit from the surface (population-based) to a point (individual-based). In this review, we started with several challenges regarding personalized care in TC, for example, inconsistent rating ability of ultrasound physicians, uncertainty in cytopathological diagnosis, difficulty in discriminating follicular neoplasms, and inaccurate prognostication. We then analyzed and summarized the advances of AI to extract and analyze morphological, textural, and molecular features to reveal the ground truth of TC. Consequently, their combination with AI technology will make individual medical strategies possible.
Introduction
Thyroid cancers (TC) have emerged in popularity over the past decades, with indolent TC accounting for the majority (1–3). For advanced TC (1, 2) and aggressive papillary thyroid carcinomas (PTC) (4), the incidence and mortality rates are also steadily increasing, which makes it imperative to adopt more effective strategies for managing such changes. In the era of personalized medicine, precise and efficient risk stratification is important before, during, and after treatment, to choose and adjust its type and intensity. The foremost step is to discover key information that reveals the biological behavior of TC. There are abundant anatomical structures (texture, internal architecture, and spatial distribution) and molecular components (gene variation, protein expression, etc.) within TC. So far, TC’s diagnosis mainly relies on image analysis (e.g., ultrasound images, cell smears, and tissue sections), but information obtained only by our naked eyes hardly enables a comprehensive analysis of the tumors (5). Given patients and their disease features, primary human cell cultures both from surgical biopsies and from fine-needle aspiration (FNA) samples foster the targeted therapies (6). However, many tough challenges still hinder a clear break of personalized treatment such as inconsistent rating ability of ultrasound (US) physicians (7), uncertainty in cytopathological diagnosis (8), difficulty in discriminating follicular neoplasms (9, 10), and inaccurate prognostication.
Artificial intelligence (AI) is a series of technologies combined to mimic human interaction (Figure 1). In some tasks, it matches or exceeds human perception (11, 12). AI deals with various sorts of omics information in parallel, easily identifying and modeling a complicated nonlinear relationship in the image (13, 14). Several studies have demonstrated that AI classifier is comparable to radiologists while qualitatively analyzing thyroid nodules (TN) (15–18). Furthermore, AI can extract and quantify key image information, whereby image diagnosis converts from a subjective qualitative task to objective quantitative analysis. This more detailed and precise information is conducive to special risk stratification and propels tailored management to transit from the surface (population-based) to a point (individual-based).
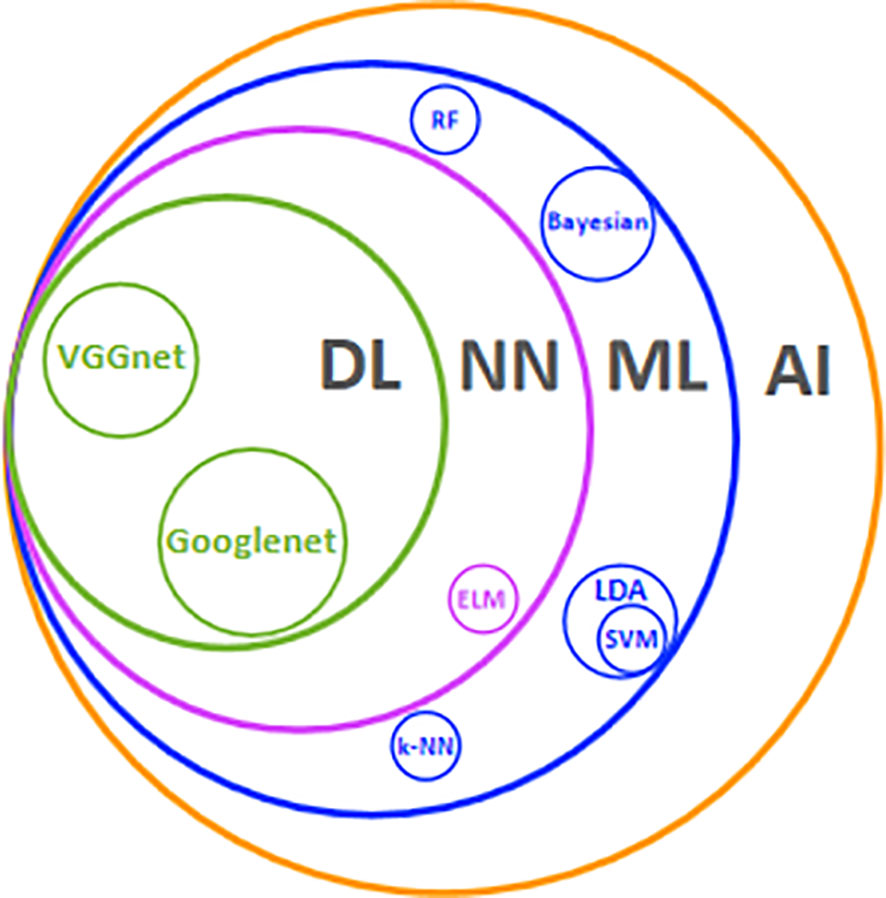
Figure 1 Main AI technologies and their relationships. AI. Artificial intelligence; ML, machine learning; NN, neural network; DL, deep learning; LDA, linear discriminant analysis; ELM, extreme learning machine; RF, random forest; SVM, support vector machine; k-NN, k-nearest neighbor.
In this review, we aimed to summarize the use of AI for extracting and analyzing morphological, textural, and molecular features to reveal detailed information and personalize therapies for TC patients (Figure 2).
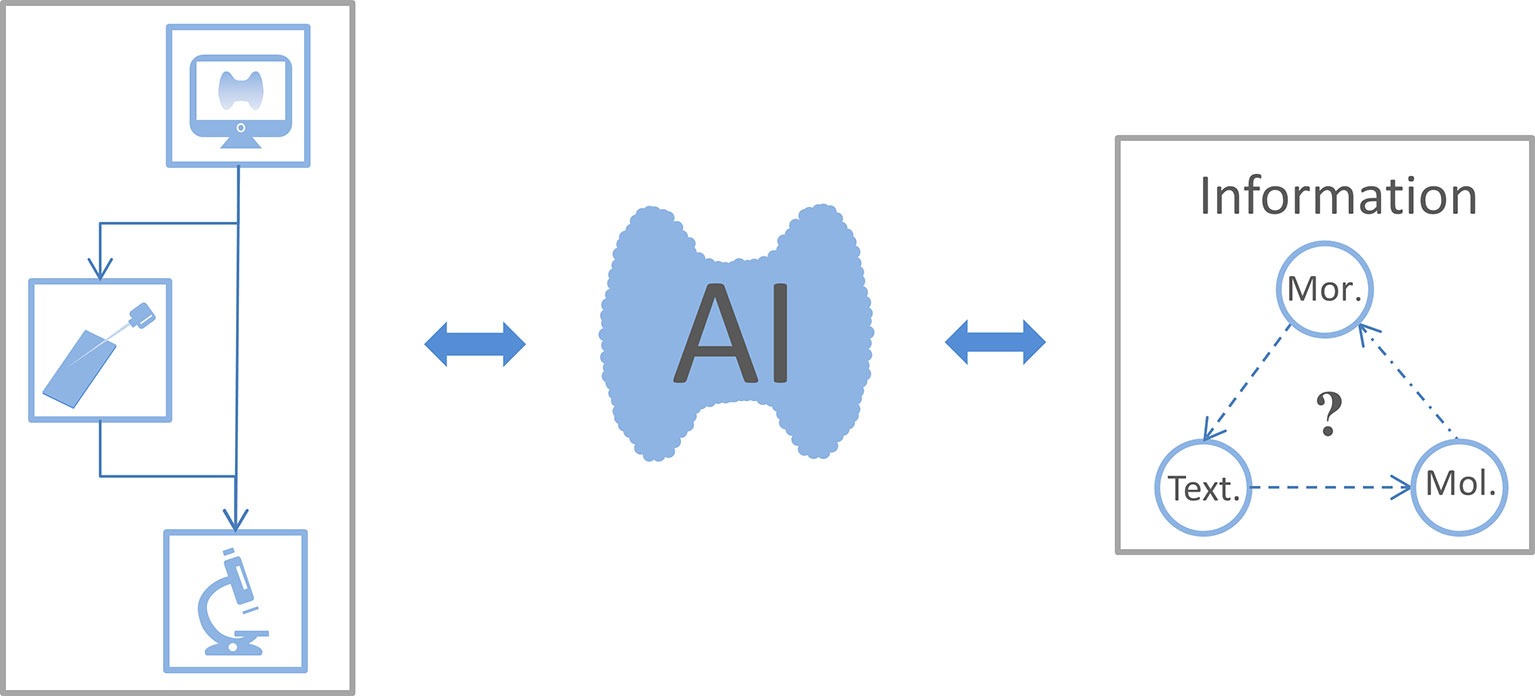
Figure 2 The connection between the focus reviewed. Thyroid ultrasound is the preferred imaging examination for patients with thyroid nodules. When sonographers consider certain thyroid nodules as malignant, the patient could choose fine-needle aspiration biopsy or surgery for a confirmed diagnosis. Artificial intelligence (AI) uses ultrasound images, cell smears, and tissue slices to extract morphological, textural, and molecular features. This information is fed back into the AI classifier to improve its performance and thus optimize thyroid cancer diagnosis and treatment workflow. As expected, whether these morphological (Mor.), textural (Text.), and molecular (Mol.) features are related to each other warrants further study.
Applications of AI in the US Diagnosis of TN
TN with several typical ultrasound features implies an increased risk of malignancy, such as solid composition, hypoechogenicity, irregular margin, microcalcification, and taller-than-wide shape. However, these properties can neither confirm nor exclude the diagnosis of TC (19). The observer’s agreement among multiple centers is poorly satisfactory in assessing these features (7). Thyroid Imaging Reporting and Data Systems (TI-RADS) are enormously valuable to PTC as risk stratification systems, while relatively less to FTC, MTC, and other malignancies (20). Interestingly, the AI model appears to be a promising tool to facilitate a better knowledge of TN via quantitative analysis of typical US features and introduction of texture features.
Performance of Typical US Features
Wildman-Tobriner et al. (21) developed an AI TI-RADS based on the American College of Radiology (ARC) TI-RADS. This system optimized the evaluation task through reassigned values for eight ultrasound features, highlighting the status of hypoechogenicity or marked hypoechogenicity. The novel AI TI-RADS had better accuracy than ARC TI-RADS when performed by inexperienced radiologists (55% vs. 48%) and experts (65% vs. 47%). Similar to other studies, ARC TI-RADS-based classifiers had higher sensitivity and slightly lower specificity (21–24). Wu et al. (25) evaluated quantitative echoic indexes for detecting malignant TN, which showed higher accuracy than typical ultrasound hypoechogenicity (>60% vs. 54.01%). We summarized the outcomes of the ultrasound features employed by AI for classification in Table 1 and found the most widely used features were shape, margin, echogenicity, calcification, composition, and size. In other words, these discriminative features seem to be the focus for the AI model to learn (31, 37). Particularly, Choi et al. (30) demonstrated several new calcification features associated with TN malignancy, including shorter calcification distance ratio, smaller amounts of calcification, and dimmer calcification. Chen et al. (28) quantified TN malignant risk through the calcification index. These new features boosted diagnostic accuracy by combining qualitative and quantitative methods (30, 38). Current AI classifiers focus on benign and malignant TN dichotomy, and certain of them like the S-Detect series have already become commercially available (32, 34). Furthermore, they are expected to predict more tumor-biological behaviors such as lymph node metastasis (39, 40) and pathological subtypes (41).
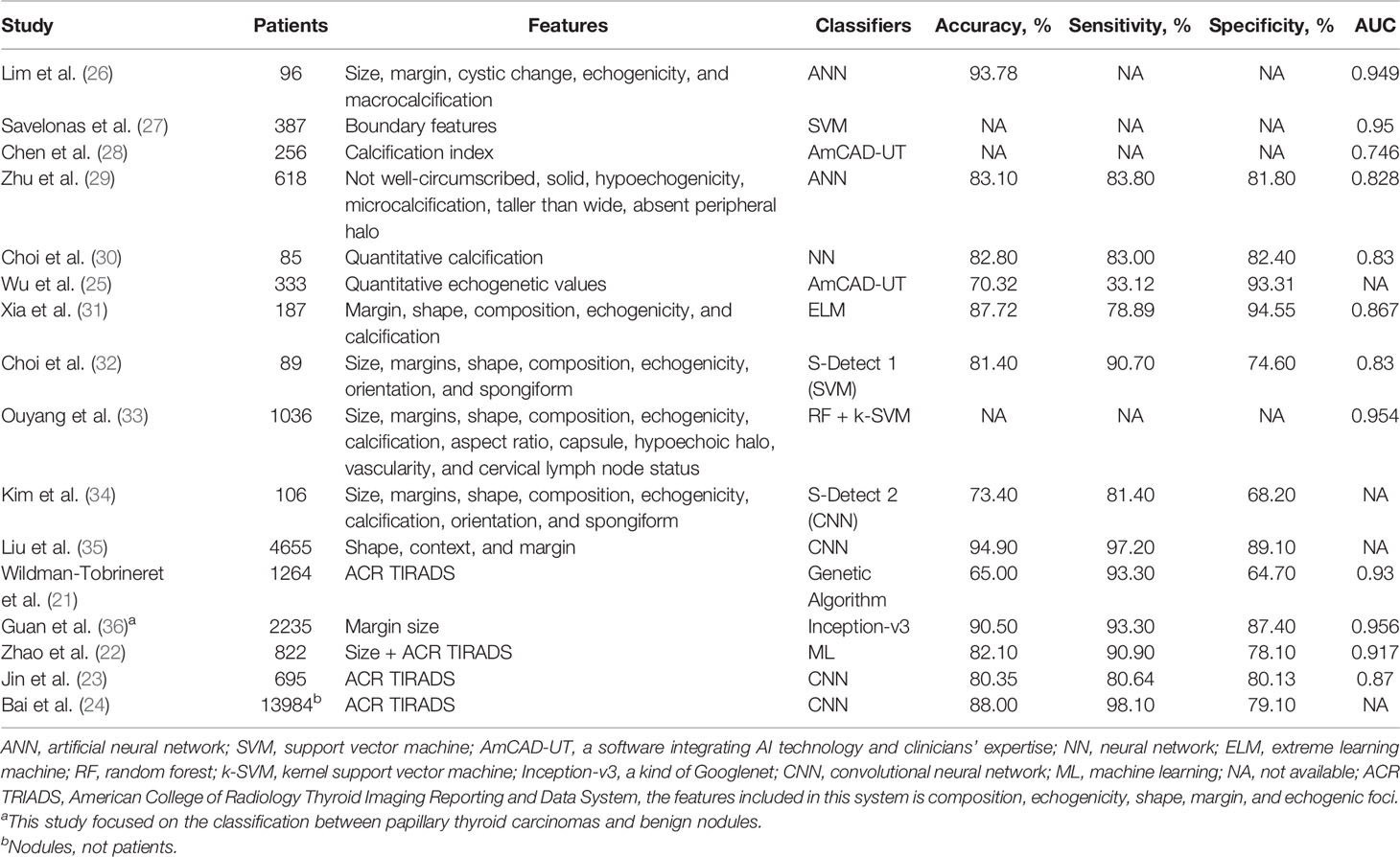
Table 1 Summary of key studies on the outcome of ultrasound features in artificial intelligence classifier identifying benign and malignant thyroid nodules.
Performance of Texture Features
A meta-analysis suggested that a taller-than-wide shape displays TN’s variation in space and orientation growth, and it is defined as the most suggestive feature for malignancy (42). Texture features refer to the characterization of spatial distribution and surface orientation with numerical features (43). Thus, texture analysis as a powerful alternative will make it possible for radiologists to comprehend the TN in depth and gain a correct diagnosis. Raghavendra et al. (44) integrated spatial and fractal texture features and screened two features with an excellent area under the curve in diagnostic practice (94.45%). Prochazka et al. (45) used AI to extract texture features from US images independent of the direction of the US probe and achieved better accuracy (94.64%). Yu et al. (46) performed a numerical transformation of two US features, unregulated shape and long/short-axis ratio into the perimeter2/area and the angle between the long axis and the horizontal axis. These new features showed excellent sensitivity and specificity (100% and 87.88%, respectively) combined with 65 texture features. Collectively, AI mode has a role in integrating typical ultrasonic and texture features, and this fusion might sharply reduce the differences in judgments among US professionals. Despite the mounting advantages of the AI model in optimizing and even creating workflows, many remarkable factors hold its ultimate practice back in the real world. The three main factors are as follows: (i) poor availability of large-high-quality datasets to guarantee great robustness (17); (ii) lack of explainability for conclusions from a black-box algorithm to solidify the trust between physicians and patients (47, 48); (iii) financial burden from specific equipment and research costs (48).
Applications of AI in Cytopathological Evaluation From FNA
FNA is a primary preoperative examination to evaluate TN. Its report system, the Bethesda System for Reporting Thyroid Cytopathology (TBSRTC), is a state-of-the-art and category-based method for clinicians’ decision-making. While TBSRTC includes six diagnostic categories on the estimated risk of malignancy (ROM) (Table 2), 15%–30% of TN continues to be classified as indeterminate TN (ITN), most frequently TBSRTC categories III, IV, and V (8). Recent studies showed excellent consistency between machine learning (ML) models and cytologists in malignancy prediction (49–51), in which the ROM of TBSRTC III determined by the ML model was considerably lower than by manual classification (4.2% vs. 18.8%) (51). It’s worth noting that morphological and genetic classifications assisted by the AI model are fairly accurate at distinguishing malignancy from benign TN (52–54) (Table 3).
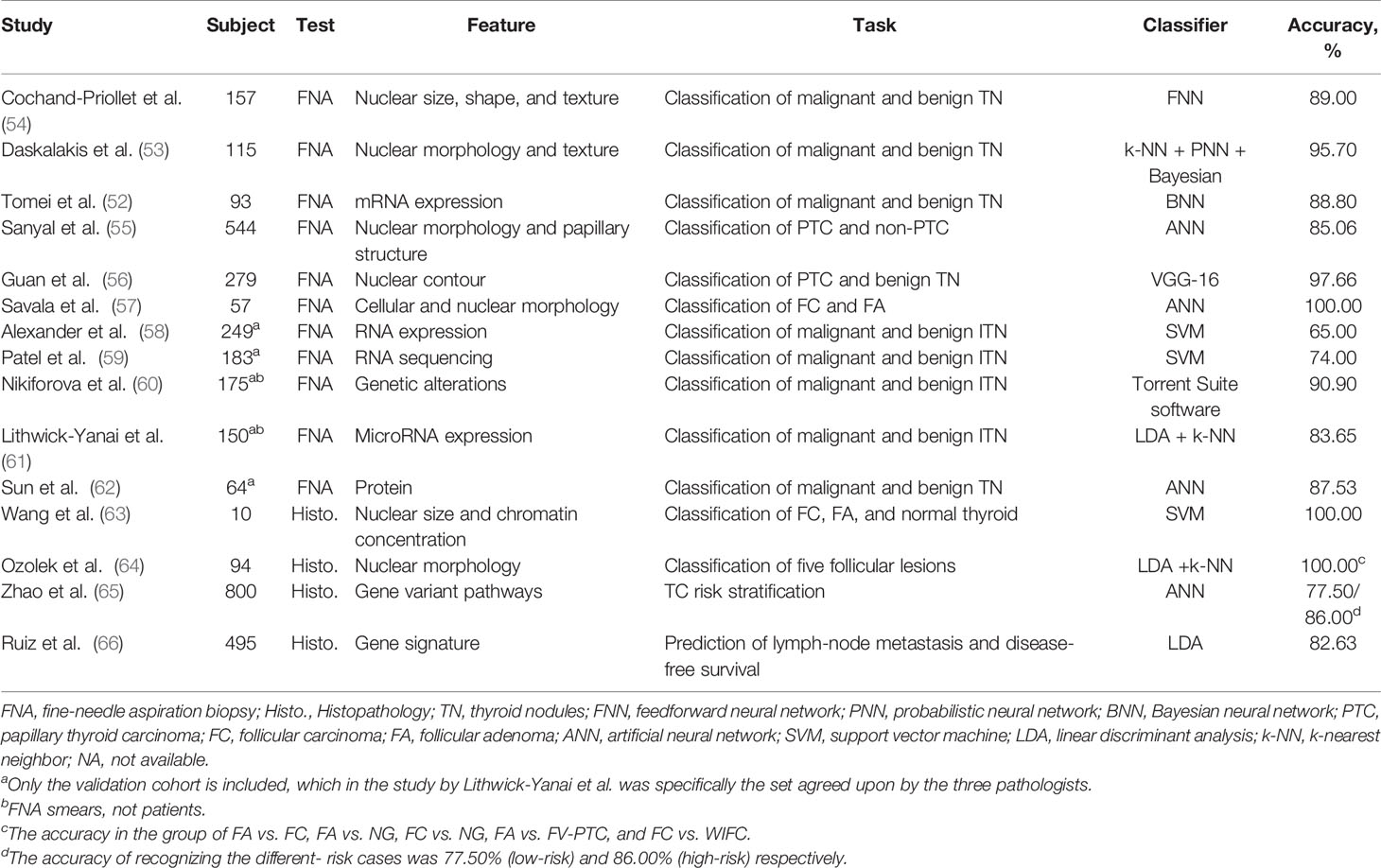
Table 3 The main performance of artificial intelligence using pathological information in different task.
Performance of Morphological Features
PTC, the most common TC (>80%), arises from abnormal growth of thyroid epithelial cells (28, 38). In recent years, AI models with quantitative morphological features have tried to improve follicular lesions’ recognition capacity (55–57). Sanyal et al. (55) obtained the nuclear morphology and papillary structure of PTC under two magnifications (×10 and ×40). CNN model selected PTC from colloid goiter, follicular neoplasms, and lymphocytic thyroiditis by right of these features. Guan et al. (56) developed a new AI cytological classification based on nuclear size and staining information (the contours, perimeter, area, and means of pixel intensity), whose results showed high accuracy (97.66%) to differentiate PTC from benign nodules. Another research group also confirmed this performance (57). They first derived nuclear pleomorphism and area information and then reported the weight of 17 cytological and morphological features. Finally, their model successfully discriminated follicular carcinoma (FC) from follicular adenoma (FA) (57) (Table 3).
The major difference between FC and FA is the occurrence of capsular or vascular invasion (67). Preoperative examinations of both US and FNA have difficulty in making a reliable diagnosis. A highly vascularized tumor protrusion on the US strongly indicates FC, which is rather rare yet (68). Seo et al. (69) took full advantage of this difference by collecting information about the tumor edge in the US images. The overall accuracy was 89.51% for distinguishing FC and FA. Yang et al. (70) segmented the whole lesions of follicular neoplasms; as a result, the classification accuracy was significantly improved to 96%. This clarified the importance of internal information and affirmed the study’s reliability by Savala et al. (57). Similarly, the diagnosis of MTC and ATC is histology dependent (71, 72), yet now no studies to our knowledge have answered the hope of AI in their ultrasound and cytopathological diagnosis.
Performance of Biomolecules
For patients with ITN, repeat FNA or lobectomy might be performed because management guidelines are more flexible (8, 73). Fortunately, molecular tests provide a noninvasive and accurate option to reduce clinical and healthy uncertainty (8, 67). Each genome contains as much information as 100,000 photographs (74). Next-generation sequencing (NGS) can perform high-speed analysis of multiple genes parallelly in a single operation, producing billions of molecular fragments (74, 75). It has always been a crucial component of big data due to its large volume of data, the astonishing velocity of the sequencing methods, and the result output’s veracity. Traditional information systems are less competent to analyze large and complex datasets (76, 77). AI as a big data algorithm can integrate multi-omic data in a different learning task, and automatically realize high-level features’ detection or classification (77). Some genetic classifiers have played their strengths in TN such as the Afirma gene expression classifier (GEC) (58), gene sequence classifier (GSC) (59), gene mutation-based classifier (ThyroSeq) (60, 78), and microRNA-based classifier (RosettaGX Reveal) (61, 79). The GEC involved 167 genes that displayed high sensitivity (92%) and positive predictive value (PPV: 93%) but limited by its relatively low specificity (52%) and negative predictive value (NPV: 47%) (58). GSC expanded the gene spectrum to 10,196 genes by RNA-enhanced NGS. Compared to GEC in the same samples, it made progress in screening for benign nodules (sensitivity: 91.1% and specificity: 68.3%) (59) (Table 3). These two classifiers are the most broadly accepted methods to rule out malignant nodules. In general, ThyroSeq and RosettaGX Reveal are more like rule-in entities. Nikiforova et al. (60) achieved a robust sensitivity of 98% and a hopeful specificity of 81.8% by employing the latest version of ThyroSeq (ThyroSeq V3) to recognize a few cancers from most benign tumors. Steward et al. (78) drew similar conclusions in a prospective blinded multicenter study and reported 94% sensitivity and 82% specificity. RosettaGX Reveal showed 98% sensitivity and 78% specificity when validated in independent cases with all three pathologists’ agreement on the histopathological diagnosis (61) (Table 3). However, whether the mentioned classifiers could consolidate and complement each other remains so ambiguous that we need to further investigate the precise application strategy.
The multi-gene analysis is able to enhance diagnostic performance, but it may be limited due to key genes’ deletion or their reduced expression. Of note, the number of thyroglobulins has been considered as a predictor of postoperative disease progression (67). Therefore, the key proteins might provide some added information for personalized therapy. Recent research has confirmed that proteins are more stable than RNA in clinical tissues (80). Sun et al. (62) completed a 14 protein-based ANN classifier for TN classification. This model realized the accuracy of 90.62% and 87.53% in multicenter retrospective and prospective samples respectively (Table 3). Some molecular alterations such as BRAF mutations (81) are diagnostic of cancer, but most of the other alterations (82, 83) show overlap in both benign and malignant lesions. Therefore, assessing the risk of malignancy by molecular testing should depend on knowledge of the prior cytological appearance.
Applications of AI in Histopathological Analysis
Upon reliable evidence obtained by the US and FNA examination, tumor information from the resected specimens is significant for pathologists to diagnosis TC such as tumor size, pathologic types, and degree of malignancy. Molecular patterns in the tumor microenvironment like cytokines, chemokines, and adipocytokines interconnect the units of immune-inflammatory responses (e.g., macrophages, neutrophils, lymphocytes) and tumor nest (e.g., epithelial cancer cells, fibroblasts, endothelial cells) (84). The more detailed information the pathologists provide, the more precise the treatment strategies physicians take. The combination of AI, morphology, and molecular markers is expected to provide more information for TC management at a patient’s level.
Performance of Morphological Features
The morphological feature is the final station of biological behavior and genetic variation of TN. The morphological performance supported by AI might be beneficial for the accurate diagnosis of TN. Wang et al. (63) successfully classified FA, FC, and normal tissues according to nuclear size and chromatin concentration. Ozolek et al. (64) achieved nearly perfect accuracy based on nine nuclear morphological features for discriminating five thyroid follicular lesions: FA, FC, follicular variant of PTC, nodular goiter, and the widely invasive FC (Table 3). However, further validation of these models is required due to tumor complicated heterogeneity, which was also turned out in a recent study for classifying TC, normal tissues, nodular goiter, and adenomas using a deep learning model (85).
Morphologically, FV-PTC is a mixed entity for typical PTC nuclear features and entirely or almost entirely follicular growth patterns. FV-PTC includes two major subtypes: encapsulated (EFV-PTC) and non-encapsulated or infiltrative variants (IFV-PTC) (86). The former generally have RAS mutations like follicular tumors, the latter often presents extrathyroidal extension (ETE), lymphatic metastasis, and BRAF mutations like classical PTC (cPTC) (87). Likewise, EFV-PTC usually appears invasive or non-invasive, and the noninvasive encapsulate tumor was redefined from carcinoma to borderline tumor, noninvasive follicular thyroid neoplasm with papillary-like nuclear features (NIFTP) (86). Up to a point, the invasive EFV-PTC behaves more aggressively like FC, whereas NIFTP is with indolent clinical behaviors like FA (87). It is believed that invasive EFV-PTC might develop from NIFTP (88). Borrelli et al. (89) revealed a significant difference in miRNA expression of FA, NIFTP, and IFV-PTC. In particular, just two miRNA (miR-10a-5p and miR-320e) enable us to differentiate NIFTP from IFV-PTC. In another study by Selvaggi (90), none of the multinucleated giant cells (MGCs) were observed in 20 NIFTP cases, while the amount of MGCs varied from 1 to 4 in 88% of the FVPTC cases (both IFV-PTC and invasive EFV-PTC). When utilizing computer quantitative analysis to classify FV-PTC, Chain et al. (91) demonstrated the NIFTP nuclear area (mean, 54.8 μm2) and elongation was smaller than PTC (mean, 77.2 μm2); Hsieh et al. (92) addressed PD-L1 expression in NIFTP was lower than in invasive EFV-PTC. These quantitative morphological characteristics and definite molecular alterations contribute to FV-PTC classification.
As FV-PTC’s definition stated, the coexistence of papillary and variable follicular structures is so common in cancer nests that we hold a positive view about more transitional or intermediate categories between the cPTC and FV-PTC. Undoubtedly, the clearer the learning exemplars, the easier it is to learn for the AI model because it receives fewer error messages (13). For greater efficiency, it’s essential to accurately classify the training set and refine the output target.
Performance of Genetic Parameters
The American Thyroid Association risk stratification system and the American Joint Committee on Cancer TNM staging system are used to guide postoperative treatment and predict post-treatment outcomes, which incorporate several parameters including age, ETE, anatomic location, number, and size of metastatic lymph nodes, aggressive variants, vascular invasion, and distant metastasis. Nonetheless, these systems fail to routinely recommend a genetic determination to guide individual management (67, 93). Zhao et al. (65) selected 10 gene variant pathways that involved inflammatory and immune responses to determine the TC patients’ risk level. Based on these pathways, the patients were divided into the high-risk and low-risk groups whose survival time was significantly better than the former. Ruiz et al. (66) demonstrated a 25-gene panel related to molecular pathways, cell structure, and function was an independent prognostic factor for lymphatic metastasis and disease-free survival (Table 3). Further evidence is still warranted to address the value of this genetic information to TN’s triage and biological behaviors. As AI and gene testing technology upgrade, the cooperation of traditional clinic-pathological parameters and gene molecules might yield more precise therapeutic implications.
Conclusion
The future development of personalized medicine in TC still faces several challenges like inconsistent rating ability of US physicians, uncertainty in cytopathological diagnosis, difficulty in discriminating follicular lesions, and inaccurate prognostication. AI’s application has improved the efficiency and accuracy of diagnosis and treatment in other tumors (94–96). A growing amount of medical information can be extracted and analyzed through AI technology. This review has innovatively offered ideas for the ultrasonic and pathological testing out of these dilemmas in terms of morphological, textural, and molecular features. As more key parameters are explored from the tumor and its microenvironment, the AI-aided combination of morphological and molecular features will pave the way for TC’s protocol at the individual level.
Author Contributions
L-RL: study design, literature review, article writing, and revision. BD: literature review. H-QL: literature review. CC: study design, article revision. All authors contributed to the article and approved the submitted version.
Funding
This research was supported by the grants from the Fundamental Research Funds for the Central Universities (2042019kf0229) and the Science and Technology Major Project of Hubei Province (Next-Generation AI Technologies) (2019AEA170).
Conflict of Interest
The authors declare that the research was conducted in the absence of any commercial or financial relationships that could be construed as a potential conflict of interest.
Acknowledgments
Sincere thanks to the teachers, classmates, and editors who worked together on this research.
References
1. Lim H, Devesa SS, Sosa JA, Check D, Kitahara CM. Trends in thyroid cancer incidence and mortality in the United States, 1974-2013. JAMA (2017) 317(13):1338–48. doi: 10.1001/jama.2017.2719
2. Jegerlehner S, Bulliard JL, Aujesky D, Rodondi N, Germann S, Konzelmann I, et al. Overdiagnosis and overtreatment of thyroid cancer: A population-based temporal trend study. PloS One (2017) 12(6):e0179387. doi: 10.1371/journal.pone.0179387
3. La Vecchia C, Malvezzi M, Bosetti C, Garavello W, Bertuccio P, Levi F, et al. Thyroid cancer mortality and incidence: a global overview. Int J Cancer (2015) 136(9):2187–95. doi: 10.1002/ijc.29251
4. Ho AS, Luu M, Barrios L, Chen I, Melany M, Ali N, et al. Incidence and Mortality risk spectrum across aggressive variants of papillary thyroid Carcinoma. JAMA Oncol (2020) 6(5):706–13. doi: 10.1001/jamaoncol.2019.6851
5. Koelzer VH, Sirinukunwattana K, Rittscher J, Mertz KD. Precision immunoprofiling by image analysis and artificial intelligence. Virchows Archiv (2019) 474(4):511–22. doi: 10.1007/s00428-018-2485-z
6. Fallahi P, Ferrari SM, Elia G, Ragusa F, Patrizio A, Paparo SR, et al. Primary cell cultures for the personalized therapy in aggressive thyroid cancer of follicular origin. Semin Cancer Biol (2020). doi: 10.1016/j.semcancer.2020.06.013
7. Persichetti A, Di Stasio E, Coccaro C, Graziano F, Bianchini A, Di Donna V, et al. Inter- and intraobserver agreement in the assessment of thyroid nodule ultrasound features and classification systems: a blinded multicenter study. Thyroid (2020) 30(2):237–42. doi: 10.1089/thy.2019.0360
8. Cibas ES, Ali SZ. The 2017 Bethesda System for Reporting Thyroid Cytopathology. Thyroid (2017) 27(11):1341–6. doi: 10.1089/thy.2017.0500
9. Baloch ZW, Livolsi VA. Follicular-patterned lesions of the thyroid: the bane of the pathologist. Am J Clin Pathol (2002) 117(1):143–50. doi: 10.1309/8vl9-ecxy-nvmx-2rqf
10. LiVolsi VA, Baloch ZW. Follicular neoplasms of the thyroid: view, biases, and experiences. Adv Anat Pathol (2004) 11(6):279–87. doi: 10.1097/01.pap.0000138143.34505.02
11. Acs B, Rantalainen M, Hartman J. Artificial intelligence as the next step towards precision pathology. J Intern Med (2020) 288(1):62–81. doi: 10.1111/joim.13030
12. Cao C, Liu F, Tan H, Song D, Shu W, Li W, et al. Deep learning and its applications in biomedicine. Genomics Proteomics Bioinf (2018) 16(1):17–32. doi: 10.1016/j.gpb.2017.07.003
13. Manning T, Sleator RD, Walsh P. Biologically inspired intelligent decision making: a commentary on the use of artificial neural networks in bioinformatics. Bioengineered (2014) 5(2):80–95. doi: 10.4161/bioe.26997
14. Tu JV. Advantages and disadvantages of using artificial neural networks versus logistic regression for predicting medical outcomes. J Clin Epidemiol (1996) 49(11):1225–31. doi: 10.1016/s0895-4356(96)00002-9
15. Sun C, Zhan Y, Chang Q, Liu T, Zhang S, Wang X, et al. Evaluation of a deep learning-based computer-aided diagnosis system for distinguishing benign from malignant thyroid nodules in ultrasound images. Med Phys (2020) 47(9):3952–60. doi: 10.1002/mp.14301
16. Wang L, Yang S, Yang S, Zhao C, Tian G, Gao Y, et al. Automatic thyroid nodule recognition and diagnosis in ultrasound imaging with the YOLOv2 neural network. World J Surg Oncol (2019) 17(1):12. doi: 10.1186/s12957-019-1558-z
17. Li X, Zhang S, Zhang Q, Wei X, Pan Y, Zhao J, et al. Diagnosis of thyroid cancer using deep convolutional neural network models applied to sonographic images: a retrospective, multicohort, diagnostic study. Lancet Oncol (2019) 20(2):193–201. doi: 10.1016/s1470-2045(18)30762-9
18. Buda M, Wildman-Tobriner B, Hoang JK, Thayer D, Tessler FN, Middleton WD, et al. Management of Thyroid Nodules Seen on US Images: Deep Learning May Match Performance of Radiologists. Radiology (2019) 292(3):695–701. doi: 10.1148/radiol.2019181343
19. Remonti LR, Kramer CK, Leitao CB, Pinto LC, Gross JL. Thyroid ultrasound features and risk of carcinoma: a systematic review and meta-analysis of observational studies. Thyroid (2015) 25(5):538–50. doi: 10.1089/thy.2014.0353
20. Trimboli P, Castellana M, Piccardo A, Romanelli F, Grani G, Giovanella L, et al. The ultrasound risk stratification systems for thyroid nodule have been evaluated against papillary carcinoma. A meta-analysis. Rev Endocr Metab Disord (2020). doi: 10.1007/s11154-020-09592-3
21. Wildman-Tobriner B, Buda M, Hoang JK, Middleton WD, Thayer D, Short RG, et al. Using artificial intelligence to revise ACR TI-RADS risk stratification of thyroid nodules: diagnostic accuracy and utility. Radiology (2019) 292(1):112–9. doi: 10.1148/radiol.2019182128
22. Zhao CK, Ren TT, Yin YF, Shi H, Wang HX, Zhou BY, et al. A comparative analysis of two machine learning-based diagnostic patterns with thyroid imaging reporting and data system for thyroid nodules: diagnostic performance and unnecessary biopsy rate. Thyroid (2020). doi: 10.1089/thy.2020.0305
23. Jin Z, Zhu Y, Zhang S, Xie F, Zhang M, Zhang Y, et al. Ultrasound Computer-aided diagnosis (CAD) based on the thyroid imaging reporting and data system (TI-RADS) to distinguish benign from malignant thyroid nodules and the diagnostic performance of radiologists with different diagnostic experience. Med Sci Monit (2020) 26:e918452. doi: 10.12659/msm.918452
24. Bai Z, Chang L, Yu R, Li X, Wei X, Yu M, et al. Thyroid nodules risk stratification through deep learning based on ultrasound images. Med Phys (2020) 47(12):6355–65. doi: 10.1002/mp.14543
25. Wu MH, Chen CN, Chen KY, Ho MC, Tai HC, Wang YH, et al. Quantitative analysis of echogenicity for patients with thyroid nodules. Sci Rep (2016) 6:35632. doi: 10.1038/srep35632
26. Lim KJ, Choi CS, Yoon DY, Chang SK, Kim KK, Han H, et al. Computer-aided diagnosis for the differentiation of malignant from benign thyroid nodules on ultrasonography. Acad Radiol (2008) 15(7):853–8. doi: 10.1016/j.acra.2007.12.022
27. Savelonas M, Maroulis D. Sangriotis M. A computer-aided system for malignancy risk assessment of nodules in thyroid US images based on boundary features. Comput Methods Prog BioMed (2009) 96(1):25–32. doi: 10.1016/j.cmpb.2009.04.001
28. Chen KY, Chen CN, Wu MH, Ho MC, Tai HC, Huang WC, et al. Computerized detection and quantification of microcalcifications in thyroid nodules. Ultrasound Med Biol (2011) 37(6):870–8. doi: 10.1016/j.ultrasmedbio.2011.03.002
29. Zhu LC, Ye YL, Luo WH, Su M, Wei HP, Zhang XB, et al. A model to discriminate malignant from benign thyroid nodules using artificial neural network. PloS One (2013) 8(12):e82211. doi: 10.1371/journal.pone.0082211
30. Choi WJ, Park JS, Kim KG, Kim SY, Koo HR, Lee YJ. Computerized analysis of calcification of thyroid nodules as visualized by ultrasonography. Eur J Radiol (2015) 84(10):1949–53. doi: 10.1016/j.ejrad.2015.06.021
31. Xia J, Chen H, Li Q, Zhou M, Chen L, Cai Z, et al. Ultrasound-based differentiation of malignant and benign thyroid Nodules: An extreme learning machine approach. Comput Methods Prog BioMed (2017) 147:37–49. doi: 10.1016/j.cmpb.2017.06.005
32. Choi YJ, Baek JH, Park HS, Shim WH, Kim TY, Shong YK, et al. A computer-aided diagnosis system using artificial intelligence for the diagnosis and characterization of thyroid nodules on ultrasound: initial clinical assessment. Thyroid (2017) 27(4):546–52. doi: 10.1089/thy.2016.0372
33. Ouyang FS, Guo BL, Ouyang LZ, Liu ZW, Lin SJ, Meng W, et al. Comparison between linear and nonlinear machine-learning algorithms for the classification of thyroid nodules. Eur J Radiol (2019) 113:251–7. doi: 10.1016/j.ejrad.2019.02.029
34. Kim HL, Ha EJ, Han M. Real-world performance of computer-aided diagnosis system for thyroid nodules using ultrasonography. Ultrasound Med Biol (2019) 45(10):2672–8. doi: 10.1016/j.ultrasmedbio.2019.05.032
35. Liu T, Guo Q, Lian C, Ren X, Liang S, Yu J, et al. Automated detection and classification of thyroid nodules in ultrasound images using clinical-knowledge-guided convolutional neural networks. Med Image Anal (2019) 58:101555. doi: 10.1016/j.media.2019.101555
36. Guan Q, Wang Y, Du J, Qin Y, Lu H, Xiang J, et al. Deep learning based classification of ultrasound images for thyroid nodules: a large scale of pilot study. Ann Transl Med (2019) 7(7):137. doi: 10.21037/atm.2019.04.34
37. Wei X, Zhu J, Zhang H, Gao H, Yu R, Liu Z, et al. Visual interpretability in computer-assisted diagnosis of thyroid nodules using ultrasound images. Med Sci Monit (2020) 26:e927007. doi: 10.12659/msm.927007
38. Chambara N, Ying M. The Diagnostic Efficiency of ultrasound computer-aided diagnosis in differentiating thyroid nodules: a systematic review and narrative synthesis. Cancers (Basel) (2019) 11(11):1759. doi: 10.3390/cancers11111759
39. Yu J, Deng Y, Liu T, Zhou J, Jia X, Xiao T, et al. Lymph node metastasis prediction of papillary thyroid carcinoma based on transfer learning radiomics. Nat Commun (2020) 11(1):4807. doi: 10.1038/s41467-020-18497-3
40. Lee JH, Baek JH, Kim JH, Shim WH, Chung SR, Choi YJ, et al. Deep Learning-Based Computer-Aided Diagnosis System for Localization and Diagnosis of Metastatic Lymph Nodes on Ultrasound: A Pilot Study. Thyroid (2018) 28(10):1332–8. doi: 10.1089/thy.2018.0082
41. Wu T, Sultan LR, Tian J, Cary TW, Sehgal CM. Machine learning for diagnostic ultrasound of triple-negative breast cancer. Breast Cancer Res Treat (2019) 173(2):365–73. doi: 10.1007/s10549-018-4984-7
42. Brito JP, Gionfriddo MR, Al Nofal A, Boehmer KR, Leppin AL, Reading C, et al. The accuracy of thyroid nodule ultrasound to predict thyroid cancer: systematic review and meta-analysis. J Clin Endocrinol Metab (2014) 99(4):1253–63. doi: 10.1210/jc.2013-2928
43. Sollini M, Cozzi L, Chiti A, Kirienko M. Texture analysis and machine learning to characterize suspected thyroid nodules and differentiated thyroid cancer: Where do we stand? Eur J Radiol (2018) 99:1–8. doi: 10.1016/j.ejrad.2017.12.004
44. Raghavendra U, Rajendra Acharya U, Gudigar A, Hong Tan J, Fujita H, Hagiwara Y, et al. Fusion of spatial gray level dependency and fractal texture features for the characterization of thyroid lesions. Ultrasonics (2017) 77:110–20. doi: 10.1016/j.ultras.2017.02.003
45. Prochazka A, Gulati S, Holinka S, Smutek D. Classification of thyroid nodules in ultrasound images using direction-independent features extracted by two-threshold binary decomposition. Technol Cancer Res Treat (2019) 18:1533033819830748. doi: 10.1177/1533033819830748
46. Yu Q, Jiang T, Zhou A, Zhang L, Zhang C, Xu P. Computer-aided diagnosis of malignant or benign thyroid nodes based on ultrasound images. Eur Arch Otorhinolaryngol (2017) 274(7):2891–7. doi: 10.1007/s00405-017-4562-3
47. Thomas J, Haertling T. AIBx, artificial intelligence model to risk stratify thyroid nodules. Thyroid (2020) 30(6):878–84. doi: 10.1089/thy.2019.0752
48. Martín Noguerol T, Paulano-Godino F, Martín-Valdivia MT, Menias CO, Luna A. Strengths, Weaknesses, Opportunities, and Threats Analysis of Artificial Intelligence and Machine Learning Applications in Radiology. J Am Coll Radiol (2019) 16(9 Pt B):1239–47. doi: 10.1016/j.jacr.2019.05.047
49. Zoulias EA, Asvestas PA, Matsopoulos GK, Tseleni-Balafouta S. A decision support system for assisting fine needle aspiration diagnosis of thyroid malignancy. Anal Quant Cytol Histol (2011) 33(4):215–22.
50. Margari N, Mastorakis E, Pouliakis A, Gouloumi AR, Asimis E, Konstantoudakis S, et al. Classification and regression trees for the evaluation of thyroid cytomorphological characteristics: A study based on liquid based cytology specimens from thyroid fine needle aspirations. Diagn Cytopathol (2018) 46(8):670–81. doi: 10.1002/dc.23977
51. Elliott Range DD, Dov D, Kovalsky SZ, Henao R, Carin L, Cohen J. Application of a machine learning algorithm to predict malignancy in thyroid cytopathology. Cancer Cytopathol (2020) 128(4):287–95. doi: 10.1002/cncy.22238
52. Tomei S, Marchetti I, Zavaglia K, Lessi F, Apollo A, Aretini P, et al. A molecular computational model improves the preoperative diagnosis of thyroid nodules. BMC Cancer (2012) 12:396. doi: 10.1186/1471-2407-12-396
53. Daskalakis A, Kostopoulos S, Spyridonos P, Glotsos D, Ravazoula P, Kardari M, et al. Design of a multi-classifier system for discriminating benign from malignant thyroid nodules using routinely H&E-stained cytological images. Comput Biol Med (2008) 38(2):196–203. doi: 10.1016/j.compbiomed.2007.09.005
54. Cochand-Priollet B, Koutroumbas K, Megalopoulou TM, Pouliakis A, Sivolapenko G, Karakitsos P. Discriminating benign from malignant thyroid lesions using artificial intelligence and statistical selection of morphometric features. Oncol Rep (2006) 15 Spec no:1023–6. doi: 10.3892/or.15.4.1023
55. Sanyal P, Mukherjee T, Barui S, Das A, Gangopadhyay P. Artificial intelligence in cytopathology: A neural network to identify papillary carcinoma on thyroid fine-needle aspiration cytology smears. J Pathol Inform (2018) 9:43. doi: 10.4103/jpi.jpi_43_18
56. Guan Q, Wang Y, Ping B, Li D, Du J, Qin Y, et al. Deep convolutional neural network VGG-16 model for differential diagnosing of papillary thyroid carcinomas in cytological images: a pilot study. J Cancer (2019) 10(20):4876–82. doi: 10.7150/jca.28769
57. Savala R, Dey P, Gupta N. Artificial neural network model to distinguish follicular adenoma from follicular carcinoma on fine needle aspiration of thyroid. Diagn Cytopathol (2018) 46(3):244–9. doi: 10.1002/dc.23880
58. Alexander EK, Kennedy GC, Baloch ZW, Cibas ES, Chudova D, Diggans J, et al. Preoperative diagnosis of benign thyroid nodules with indeterminate cytology. New Engl J Med (2012) 367(8):705–15. doi: 10.1056/NEJMoa1203208
59. Patel KN, Angell TE, Babiarz J, Barth NM, Blevins T, Duh QY, et al. Performance of a genomic sequencing classifier for the preoperative diagnosis of cytologically indeterminate thyroid nodules. JAMA Surg (2018) 153(9):817–24. doi: 10.1001/jamasurg.2018.1153
60. Nikiforova MN, Mercurio S, Wald AI, Barbi de Moura M, Callenberg K, Santana-Santos L, et al. Analytical performance of the ThyroSeq v3 genomic classifier for cancer diagnosis in thyroid nodules. Cancer (2018) 124(8):1682–90. doi: 10.1002/cncr.31245
61. Lithwick-Yanai G, Dromi N, Shtabsky A, Morgenstern S, Strenov Y, Feinmesser M, et al. Multicentre validation of a microRNA-based assay for diagnosing indeterminate thyroid nodules utilising fine needle aspirate smears. J Clin Pathol (2017) 70(6):500–7. doi: 10.1136/jclinpath-2016-204089
62. Sun Y, Selvarajan S, Zang Z, Liu W, Zhu YJ, Zhang H, et al. Protein classifier for thyroid nodules learned from rapidly acquired proteotypes. medRxiv (2020). doi: 10.1101/2020.04.09.20059741
63. Wang W, Ozolek JA, Rohde GK. Detection and classification of thyroid follicular lesions based on nuclear structure from histopathology images. Cytometry A (2010) 77(5):485–94. doi: 10.1002/cyto.a.20853
64. Ozolek JA, Tosun AB, Wang W, Chen C, Kolouri S, Basu S, et al. Accurate diagnosis of thyroid follicular lesions from nuclear morphology using supervised learning. Med Image Anal (2014) 18(5):772–80. doi: 10.1016/j.media.2014.04.004
65. Zhao Y, Zhao L, Mao T, Zhong L. Assessment of risk based on variant pathways and establishment of an artificial neural network model of thyroid cancer. BMC Med Genet (2019) 20(1):92. doi: 10.1186/s12881-019-0829-4
66. Ruiz EML, Niu T, Zerfaoui M, Kunnimalaiyaan M, Friedlander PL, Abdel-Mageed AB, et al. A novel gene panel for prediction of lymph-node metastasis and recurrence in patients with thyroid cancer. Surgery (2020) 167(1):73–9. doi: 10.1016/j.surg.2019.06.058
67. Haugen BR, Alexander EK, Bible KC, Doherty GM, Mandel SJ, Nikiforov YE, et al. 2015 American thyroid association management guidelines for adult patients with thyroid nodules and differentiated thyroid cancer: The american thyroid association guidelines task force on thyroid nodules and differentiated thyroid cancer. Thyroid (2016) 26(1):1–133. doi: 10.1089/thy.2015.0020
68. Kobayashi K, Hirokawa M, Yabuta T, Masuoka H, Fukushima M, Kihara M, et al. Tumor protrusion with intensive blood signals on ultrasonography is a strongly suggestive finding of follicular thyroid carcinoma. Med Ultrason (2016) 18(1):25–9. doi: 10.11152/mu.2013.2066.181.kok
69. Seo JK, Kim YJ, Kim KG, Shin I, Shin JH, Kwak JY. Differentiation of the Follicular Neoplasm on the Gray-Scale US by Image Selection Subsampling along with the Marginal Outline Using Convolutional Neural Network. BioMed Res Int (2017) 2017:3098293. doi: 10.1155/2017/3098293
70. Yang B, Yan M, Yan Z, Zhu C, Xu D, Dong F. Segmentation and classification of thyroid follicular neoplasm using cascaded convolutional neural network. Phys Med Biol (2020). doi: 10.1088/1361-6560/abc6f2
71. Smallridge RC, Ain KB, Asa SL, Bible KC, Brierley JD, Burman KD, et al. American Thyroid Association guidelines for management of patients with anaplastic thyroid cancer. Thyroid (2012) 22(11):1104–39. doi: 10.1089/thy.2012.0302
72. Wells SA Jr., Asa SL, Dralle H, Elisei R, Evans DB, Gagel RF, et al. Revised American Thyroid Association guidelines for the management of medullary thyroid carcinoma. Thyroid (2015) 25(6):567–610. doi: 10.1089/thy.2014.0335
73. Misiakos EP, Margari N, Meristoudis C, Machairas N, Schizas D, Petropoulos K, et al. Cytopathologic diagnosis of fine needle aspiration biopsies of thyroid nodules. World J Clin Cases (2016) 4(2):38–48. doi: 10.12998/wjcc.v4.i2.38
74. Phillips KA, Trosman JR, Kelley RK, Pletcher MJ, Douglas MP, Weldon CB. Genomic sequencing: assessing the health care system, policy, and big-data implications. Health Aff (2014) 33(7):1246–53. doi: 10.1377/hlthaff.2014.0020
75. Schmidt B, Hildebrandt A. Next-generation sequencing: big data meets high performance computing. Drug Discovery Today (2017) 22(4):712–7. doi: 10.1016/j.drudis.2017.01.014
76. Mayer-Schönberger V, Ingelsson E. Big Data and medicine: a big deal? J Intern Med (2018) 283(5):418–29. doi: 10.1111/joim.12721
77. Hamamoto R, Komatsu M, Takasawa K, Asada K, Kaneko S. Epigenetics analysis and integrated analysis of multiomics data, including epigenetic data, using artificial intelligence in the era of precision medicine. Biomolecules (2019) 10(1):62. doi: 10.3390/biom10010062
78. Steward DL, Carty SE, Sippel RS, Yang SP, Sosa JA, Sipos JA, et al. Performance of a multigene genomic classifier in thyroid nodules with indeterminate cytology: a prospective blinded multicenter study. JAMA Oncol (2019) 5(2):204–12. doi: 10.1001/jamaoncol.2018.4616
79. Benjamin H, Schnitzer-Perlman T, Shtabsky A, VandenBussche CJ, Ali SZ, Kolar Z, et al. Analytical validity of a microRNA-based assay for diagnosing indeterminate thyroid FNA smears from routinely prepared cytology slides. Cancer Cytopathol (2016) 124(10):711–21. doi: 10.1002/cncy.21731
80. Shao W, Guo T, Toussaint NC, Xue P, Wagner U, Li L, et al. Comparative analysis of mRNA and protein degradation in prostate tissues indicates high stability of proteins. Nat Commun (2019) 10(1):2524. doi: 10.1038/s41467-019-10513-5
81. Xu X, Quiros RM, Gattuso P, Ain KB, Prinz RA. High prevalence of BRAF gene mutation in papillary thyroid carcinomas and thyroid tumor cell lines. Cancer Res (2003) 63(15):4561–7.
82. Zou M, Shi Y, Farid NR, al-Sedairy ST, Paterson MC. FHIT gene abnormalities in both benign and malignant thyroid tumours. Eur J Cancer (1999) 35(3):467–72. doi: 10.1016/s0959-8049(98)00370-0
83. Angell TE. RAS-positive thyroid nodules. Curr Opin Endocrinol Diabetes Obes (2017) 24(5):372–6. doi: 10.1097/med.0000000000000354
84. Ferrari SM, Fallahi P, Galdiero MR, Ruffilli I, Elia G, Ragusa F, et al. Immune and inflammatory cells in thyroid cancer microenvironment. Int J Mol Sci (2019) 20(18):4413. doi: 10.3390/ijms20184413
85. Wang Y, Guan Q, Lao I, Wang L, Wu Y, Li D, et al. Using deep convolutional neural networks for multi-classification of thyroid tumor by histopathology: a large-scale pilot study. Ann Transl Med (2019) 7(18):468. doi: 10.21037/atm.2019.08.54
86. Kakudo K, Bychkov A, Bai Y, Li Y, Liu Z, Jung CK. The new 4th edition World Health Organization classification for thyroid tumors, Asian perspectives. Pathol Int (2018) 68(12):641–64. doi: 10.1111/pin.12737
87. Daniels GH. Follicular variant of papillary thyroid carcinoma: hybrid or mixture? Thyroid (2016) 26(7):872–4. doi: 10.1089/thy.2016.0244
88. Hodak S, Tuttle RM, Maytal G, Nikiforov YE, Randolph G. Changing the cancer diagnosis: the case of follicular variant of papillary thyroid cancer-primum non nocere and NIFTP. Thyroid (2016) 26(7):869–71. doi: 10.1089/thy.2016.0205
89. Borrelli N, Denaro M, Ugolini C, Poma AM, Miccoli M, Vitti P, et al. miRNA expression profiling of ‘noninvasive follicular thyroid neoplasms with papillary-like nuclear features’ compared with adenomas and infiltrative follicular variants of papillary thyroid carcinomas. Mod Pathol (2017) 30(1):39–51. doi: 10.1038/modpathol.2016.157
90. Selvaggi SM. The presence of multinucleated giant cells: Noninvasive follicular thyroid neoplasm with papillary-like nuclear features vs the follicular variant of papillary thyroid carcinoma. Diagn Cytopathol (2019) 47(10):1007–10. doi: 10.1002/dc.24259
91. Chain K, Legesse T, Heath JE, Staats PN. Digital image-assisted quantitative nuclear analysis improves diagnostic accuracy of thyroid fine-needle aspiration cytology. Cancer Cytopathol (2019) 127(8):501–13. doi: 10.1002/cncy.22120
92. Hsieh AM, Polyakova O, Fu G, Chazen RS, MacMillan C, Witterick IJ, et al. Programmed death-ligand 1 expression by digital image analysis advances thyroid cancer diagnosis among encapsulated follicular lesions. Oncotarget (2018) 9(28):19767–82. doi: 10.18632/oncotarget.24833
93. Perrier ND, Brierley JD, Tuttle RM. Differentiated and anaplastic thyroid carcinoma: Major changes in the American Joint Committee on Cancer eighth edition cancer staging manual. CA Cancer J Clin (2018) 68(1):55–63. doi: 10.3322/caac.21439
94. Gardezi SJS, Elazab A, Lei B, Wang T. Breast cancer detection and diagnosis using mammographic data: systematic review. J Med Internet Res (2019) 21(7):e14464. doi: 10.2196/14464
95. Lui TKL, Guo CG, Leung WK. Accuracy of artificial intelligence on histology prediction and detection of colorectal polyps: a systematic review and meta-analysis. Gastrointest Endosc (2020) 92(1):11–22. doi: 10.1016/j.gie.2020.02.033
Keywords: artificial intelligence, thyroid cancer, biomarker, personalized medicine, histopathology, fine-needle aspiration biopsy, ultrasound
Citation: Li L-R, Du B, Liu H-Q and Chen C (2021) Artificial Intelligence for Personalized Medicine in Thyroid Cancer: Current Status and Future Perspectives. Front. Oncol. 10:604051. doi: 10.3389/fonc.2020.604051
Received: 08 September 2020; Accepted: 21 December 2020;
Published: 09 February 2021.
Edited by:
Randy Yeh, Memorial Sloan Kettering Cancer Center, United StatesReviewed by:
Vasyl Vasko, Uniformed Services University of the Health Sciences, United StatesBarbara Maria Jarzab, Maria Skłodowska-Curie National Research Institute of Oncology, Gliwice Branch, Poland
Alessandro Antonelli, University of Pisa, Italy
Copyright © 2021 Li, Du, Liu and Chen. This is an open-access article distributed under the terms of the Creative Commons Attribution License (CC BY). The use, distribution or reproduction in other forums is permitted, provided the original author(s) and the copyright owner(s) are credited and that the original publication in this journal is cited, in accordance with accepted academic practice. No use, distribution or reproduction is permitted which does not comply with these terms.
*Correspondence: Chuang Chen, Y2hlbmMyNDY5QDE2My5jb20=