- 1Royal Veterinary College, London, United Kingdom
- 2North Downs Specialist Referrals, Bletchingley, United Kingdom
- 3Fitzpatrick Referrals, Guildford, United Kingdom
- 4Wellcome Centre for Human Genetics, University of Oxford, Oxford, United Kingdom
- 5Barts Cancer Institute, Queen Mary University of London, London, United Kingdom
- 6School of Veterinary Medicine, University of Pennsylvania, Philadelphia, PA, United States
Gene expression profiling has revealed molecular heterogeneity of diffuse large B cell lymphoma (DLBCL) in both humans and dogs. Two DLBCL subtypes based on cell of origin are generally recognized, germinal center B (GCB)-like and activated B cell (ABC)-like. A pilot study to characterize the transcriptomic phenotype of 11 dogs with multicentric BCL yielded two molecular subtypes distinguished on the basis of genes important in oxidative phosphorylation. We propose a metabolic classification of canine BCL that transcends cell of origin and shows parallels to a similar molecular phenotype in human DLBCL. We thus confirm the validity of this classification scheme across widely divergent mammalian taxa and add to the growing body of literature suggesting cellular and molecular similarities between human and canine non-Hodgkin lymphoma. Our data support a One Health approach to the study of DLBCL, including the advancement of novel therapies of relevance to both canine and human health.
Introduction
Diffuse large B cell lymphoma (DLBCL), an aggressive malignancy of mature B lymphocytes, comprises the most common subtype of non-Hodgkin lymphoma (NHL) (1). Variable clinical characteristics and treatment response of patients with DLBCL have prompted investigations into its molecular heterogeneity (1–3). Gene expression profiling has classified DLBCL into multiple subtypes: the cell-of-origin (COO) classification identifies three subsets named germinal center B (GCB)-like, activated B cell (ABC)-like, and “undefined,” while the consensus cluster classification (CCC) identifies three subsets named “oxidative phosphorylation (OxPhos),” “B cell receptor/proliferation,” and “host response” (2, 3). The COO and CCC classification schemes yield complementary information and differentially stratify patients, with little correlation or overlap (3, 4).
Dogs have been increasingly gaining traction as an animal model in comparative oncology, attributed to their spontaneous development of cancer, intact immune system, and shared living environment with humans, with common exposure to xenobiotics (5). Canine DLBCL mirrors human DLBCL in clinical presentation, therapeutic modalities, and molecular pathogenesis (6, 7). A number of studies have set out to dissect the molecular signature of canine DLBCL, aiming to better diagnose this cancer and predict treatment outcome for patients of both species. For instance, our previous study showed that overexpression of FoxP3 by intratumoral T cells in canine multicentric BCL correlates with shorter survival (8). A gene profiling study has revealed GCB-like and ABC-like subtypes in canine DLBCL, the latter of which shows less favorable survival, thus resembling the human disease (9). The same study also proposed a list of 1,180 genes to distinguish GCB-DLBCL and ABC-DLBCL in dogs, prompting us to question whether a shorter list of selective classifiers may serve the same purpose. We therefore undertook a pilot study to analyze the transcriptomic phenotype of dogs with multicentric BCL, all with the cytological characteristics of DLBCL, hypothesizing that molecular subtype may be identified by a selective subset of gene identifiers.
Materials and Methods
Sample Collection
Samples of 11 canine lymphoma cases (Supplementary Data 1) were collected in a sterile fashion by qualified veterinarians prior to chemotherapy, with signed informed consent of the owner and approval of the Royal Veterinary College (RVC) Ethics and Welfare Committee (Permit Number: URN 2014 1285) in the United Kingdom (UK). Dogs of any age, breed, gender, or neutering status were recruited to minimize selection bias. Cells aspirated from enlarged peripheral lymph nodes were flushed into 100% fetal bovine serum (Biosera, East Sussex, UK) for immunophenotyping by flow cytometry. Board-certified clinical pathologists undertook contemporaneous cytological review. All 11 cases were confirmed to be BCL, with cytological features of DLBCL.
Cell Isolation
Aspirated lymph node cells were labeled by a mixture of R-phycoerythrin (PE)-conjugated anti-dog CD5 (clone YKIX322.3; Bio-Rad, UK), Alexa Fluor 647®-conjugated anti-dog CD21 (clone CA2.1D6; Bio-Rad) and 4′,6-diamidino-2-phenylindole (DAPI; BioLegend, San Diego, CA, USA). CD5−CD21+ cells, the majority of which comprised neoplastic B cells, were isolated using fluorescence-activated cell sorting (FACS™). RNA extraction, sequencing, and read processing of the isolated B cells were performed in accordance with our previous protocols (10).
RNA Extraction and Sequencing
Total RNA was extracted from FACS™-isolated CD5−CD21+ cells using RNA Bee (AMS Biotechnology, Abingdon, UK) and Direct-zol™ RNA MicroPrep Kit (Zymo Research, Irvine, CA, USA), according to the manufacturer's instructions. All samples were treated with DNase I during extraction and examined by Agilent 2100 Bioanalyzer (Agilent Technologies, Santa Clara, CA, USA), with an RNA Integrity Number of greater than or equal to 6.5. Seventy-five-bp, paired-end RNA sequencing (RNA-seq) was performed using the HiSeq.4000 System (Illumina, San Diego, CA, USA) at the Oxford Genomics Centre, University of Oxford (Oxford, UK). RNA-seq read processing and expression quantification followed methods used in our previous study (10). Quality control was performed by the Oxford Genomics Centre to assess sample processing and data integrity. Transcript reads were mapped and annotated to the canine genome, CanFam3.1 (Ensembl Genes, release 91). Read counts were all normalized to transcripts per million (TPM) values for subsequent data analyses.
Data Analyses
Hierarchical cluster analysis was conducted on Morpheus (Broad Institute, USA) using the one minus Pearson correlation and average linkage method. Principal component analysis (PCA), volcano plot creation, and survival analysis were all undertaken in R (version 3.4.2; R code provided in Supplementary Data 2). Differential expression analysis was performed using the Bioconductor package edgeR (Bioconductor version 3.6) (11). Differentially expressed genes with a false discovery rate (FDR) of <0.05 were input into the software Ingenuity Pathway Analysis (IPA; Ingenuity Systems Inc., Redwood City, CA, USA) to identify biological pathways affected by the altered expression of these genes (p < 0.05, |Z| score ≥ 2). Overall survival time post-diagnosis was estimated for nine of the 11 dogs using Kaplan-Meier curves and Cox regression analysis in R. (Dogs 7 and 11 received no chemotherapy for BCL and were excluded from survival analysis.)
Comparison With Human Dataset
Processed microarray data of 203 human DLBCL samples analyzed by Affymetrix Human Genome U133 Plus 2.0 Array (GEO accession number GSE11318) were downloaded (12). To combine the 11 canine RNA-seq and 203 human microarray data, a list of 14,224 consensus genes were identified between the two datasets based on annotated gene symbols. Canine RNA-seq data were z-transformed, and human microarray data were log2-transformed followed by z-transformation. Normalized expression data of 22 genes were retrieved from the combined datasets on the basis of the previously identified 27-gene classifiers that distinguish human GCB- and ABC-DLBCL (2, 9), and analyzed on Morpheus using K-means (K = 2) and average linkage hierarchical clustering.
Results
Gene Expression Profiling Reveals Two Molecular Subtypes of Canine B Cell Lymphoma With Distinct Metabolic Signatures
Unsupervised hierarchical clustering using genome-wide expression data of the neoplastic B cells segregated the 11 cases into two major clusters (Figure 1A). Their distinct expression signatures were confirmed by PCA (Figure 1B). We then set out to analyze genes differentially expressed between the two clusters and identified a list of 527 genes (FDR <0.05), of which 162 were of lower transcript abundance and 365 were of higher transcript abundance in cluster 2 compared to cluster 1 (Figure 1C). One hundred and forty-six (~28%) of the 527 genes are involved in mitochondrial metabolism or function, of which 50 were of lower transcript abundance and 96 were of higher transcript abundance in cluster 2 vs. cluster 1 (Supplementary Data 3). To identify genes with interesting functional implications, we narrowed down this list of 527 genes on the basis of two criteria. We first identified genes differentially expressed with high significance (FDR <0.01). We then identified genes in this curated list that were most variably expressed, i.e., in the top or bottom third of the list. As a result, five genes of lower transcript abundance and six genes of higher transcript abundance in cluster 2 were selected (Figure 1C). Of the 11 selected genes, uchl1, s100a8, and s100a12 are of particular interest, all correlating with oxidative stress (13, 14). Seven of the remaining eight genes have been associated with various cancer contexts, consonant with their association with subsets of canine DLBCL. Hence, ccr4 (15, 16), myoz2 (17), and clec3b (18, 19) are adverse prognosis biomarkers, while vwa5a (20) and sult1b1 (21) serve a tumor suppressor role in human solid tumors. The gene ffar2 encodes a short chain fatty acid receptor that may inhibit metastasis of human breast cancer cells (22), and cathepsin-G encoded by ctsg is a neutrophil protease that facilitates cell adhesion in human breast cancer cells (23). The remaining gene slc6a5 encodes a glycine transporter protein whose loss of function is implicated in human hyperekplexia (24, 25).
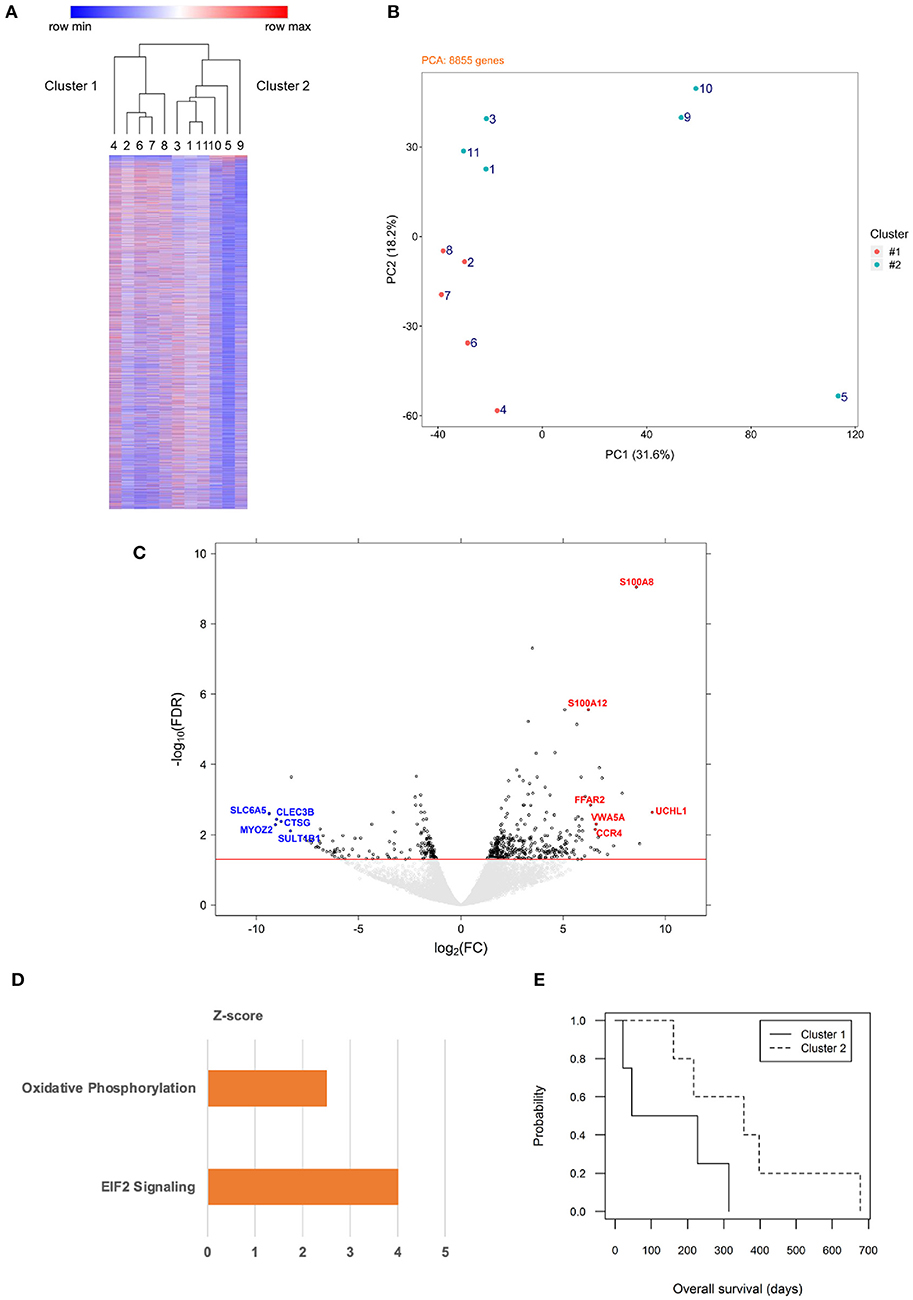
Figure 1. Gene expression profiling of canine B cell lymphoma with distinct metabolic signatures distinguished by oxidative phosphorylation. (A) Unsupervised hierarchical clustering classifies genome-wide expression data of 11 dogs with multicentric BCL into two major clusters. Each row represents one gene, color intensity positively correlating with TPM values presented by rows in relative value from 0 to 1. Numbers 1–11 represent each of the dogs. The two major clusters are designated 1 (left) and 2 (right). (B) Principal component analysis of genome-wide expression data of 8,855 genes from the same 11 dogs confirms spatial segregation of the two major clusters (cluster 1: orange; cluster 2: teal). (C) A volcano plot of expression data of differentially expressed genes of dogs in cluster 2 (n = 6) vs. dogs in cluster 1 (n = 5) identifies genes of particular interest. The threshold line (red) indicates a false detection rate of 0.05; each dot represents one gene (to facilitate visualization, gene symbols are designated in upper case). (D) Ingenuity Pathway Analysis of differentially expressed genes (cluster 2 vs. cluster 1) reveals significant enrichment of two biological pathways (p < 0.05), one focusing on oxidative phosphorylation (orange color represents activated status). (E) Overall survival time post-diagnosis was compared between clusters 1 and 2 using Kaplan-Meier curves and Cox regression analysis (p = 0.12).
Ingenuity Pathway Analysis of the 527 differentially expressed genes revealed that both OxPhos and eukaryotic initiation factor (EIF) 2 signaling pathways were enriched and activated in cluster 2 (Figure 1D). Overall survival time post-diagnosis was analyzed: dogs from cluster 1 had an estimated median survival time of 137 days, while those from cluster 2 had a median survival time of 356 days, with a hazard ratio of 0.26 (cluster 2 vs. cluster 1; 95% confidence interval: 0.05–1.44; p = 0.12; Figure 1E). Furthermore, among the 131 genes functionally annotated in “OxPhos” in the human database (Systematic name: M19540, Gene Set Enrichment Analysis, Broad Institute), 80 genes were annotated in the canine genome (CanFam3.1), of which six were of higher transcript abundance and four were of lower transcript abundance in canine cluster 2 vs. cluster 1 (Supplementary Data 4). These results collectively suggest that the 11 dogs with multicentric BCL in our study fell into two molecular subgroups with distinct metabolic signatures characterized by OxPhos, with no significant difference in survival. We therefore designated cluster 1 as “non-OxPhos” and cluster 2 as “OxPhos” subgroups in the remainder of this study.
Cell of origin and Metabolic Molecular Classification Schemes of B Cell Lymphoma in Dogs Differentially Segregate Cases
We then compared our 527 genes with the published 1,180 canine DLBCL COO subtype-classifiers, following the exclusion of redundant and un-annotated data (Figure 2A). The paucity of consensus genes, numbering only 16, suggested limited overlap between the two lists (Supplementary Data 5). Unsupervised hierarchical clustering using the published 1,180 classifiers failed to segregate our transcriptomic data into two major clusters (Figure 2B), further confirming the lack of consensus and inability of the COO classification scheme to stratify our cases. We speculated that this could have reflected the limited number of cases, with a majority bias toward one COO subtype or the other. Therefore, we co-clustered our 11 canine RNA-seq and 203 human DLBCL microarray data on the basis of the previously identified 27-gene classifiers that distinguish human GCB- and ABC-DLBCL (2, 9). Five of the 27 genes were excluded from this analysis: tbc1d27 had no canine homolog; c1orf186, mme, and serpina9 were expressed in none or fewer than two of the 11 dogs; and ighm had a predominant expression in some of the dogs that biased the clustering (9). K-means (K = 2) clustering partitioned the combined 11 canine and 203 human samples into two groups: two dogs co-clustered with 106 human samples in group 1, and the other nine dogs co-clustered with remaining 97 human samples in group 2 (Figure 2C). Hierarchical clustering on the basis of the 22 human GCB/ABC classifier genes revealed distinct expression patterns of the two groups: genes highly expressed in GCB-DLBCL were enriched in group 1, while genes highly expressed in ABC-DLBCL were enriched in group 2 (Figure 2C). Taken together, these results suggested that the two dogs in group 1 were more likely to be of GCB phenotype, while the remaining nine dogs in group 2 were more likely to be of ABC phenotype, consistent with a skewed COO phenotypic distribution of the canine patients.
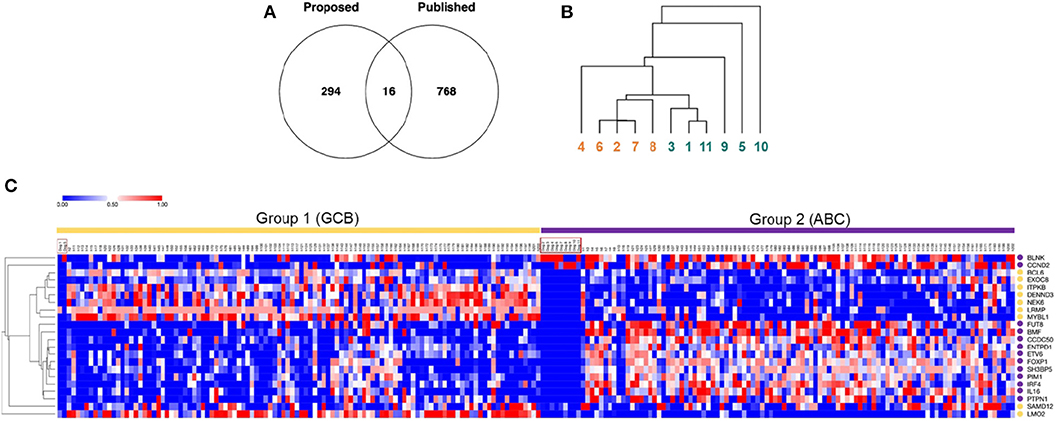
Figure 2. Cell of Origin and Metabolic molecular classification schemes of B cell lymphoma in dogs differentially segregate cases. (A) A Venn diagram of common and distinct differentially expressed genes between our dataset (n = 527 genes) and the published canine GCB/ABC-DLBCL gene classifier dataset (n = 1,180 genes) reveals minimal overlap of the two classification methods. (B) Hierarchical cluster analysis of B cell gene expression data from dogs recruited in this study using the published 1,180 canine GCB/ABC-DLBCL gene classifiers fails to stratify the cases into two major subtypes, suggesting a lack of distinction on the basis of cell of origin (numbers 1–11 represent each of the dogs. Colors indicate the original cluster of the 11 dogs, as identified in Figure 1. Redundant and un-annotated genes were excluded from all analyses). (C) Co-clustering of B cell gene expression data from 11 canine RNA-seq data in this study and published human microarray data (n = 203). The heatmap was generated via clustering combined datasets by columns using K-means (K = 2) and by rows using average linkage hierarchical clustering. Each column represents one sample, and each row represents one gene (color intensity positively correlating with z-transformed expression values presented by value from 0 to 1; gene symbols are designated in upper case to facilitate visualization). Sample names are annotated on top of the heatmap, with the 11 canine samples highlighted by red boxes. Dogs 1 and 5 co-clustered with 106 human samples falling into group 1, whereas the remaining nine dogs co-clustered with 97 human samples falling into group 2. Yellow circles annotate subtype-classifying genes highly expressed in human GCB-DLBCL, and purple circles annotate genes highly expressed in human ABC-DLBCL.
Discussion
This pilot study set out to characterize the transcriptomic signature of 11 dogs with DLBCL, aiming to refine the previously published canine DLBCL-subtyping COO classifier list. Our results suggest that multicentric BCL in dogs may be stratified in an alternative, non-overlapping manner based on metabolic signatures rather than COO characteristics. One of the two identified subtypes resembles the “OxPhos”-DLBCL subtype in human patients (4). We therefore propose a novel, complementary molecular classification of canine BCL, named OxPhos and non-OxPhos, with an understanding of several caveats to be addressed by future work. First, the small sample size of cases in our study may not adequately represent the diversity of canine DLBCL. Distinction of the two subtypes needs prospective validation in a larger cohort of cases using the 527 differentially expressed genes. Second, genes involved in oxidative metabolism are not all enriched in the proposed canine OxPhos subtype, as they are in the human counterpart. Third, the COO classification scheme has been refined in human DLBCL: four gene expression signatures, termed as “germinal center B cell,” “proliferation,” “major histocompatibility complex (MHC) class II,” and “lymph node” correlate with prognosis of patients treated by CHOP chemotherapy (26). The parallelism of these molecular subtypes in the two species therefore needs further, rigorous investigation. Survival times between the two subgroups showed no significant difference in this study, although the small sample size introduced the possibility of a type II error. Moreover, several dogs received non-CHOP chemotherapy, further confounding analysis of survival. Correlation of metabolic signature with prognosis is still unclear in human DLBCL: one study showed similar five-year survival between patients with OxPhos- and non-OxPhos-DLBCL (3), whereas another revealed poor response to rituximab (R)-CHOP treatment in human OxPhos-DLBCL (27). The prognosis of the OxPhos signature in canine DLBCL, and its resemblance to the human counterpart, therefore needs further characterization in a larger and more homogeneous cohort of cases.
Neoplastic cells reprogram their metabolism to sustain high proliferation (28, 29) and to resist apoptosis induced by oxidative stress (30). Numerous studies have been conducted to investigate the metabolic reprogramming of neoplastic cells, aiming to identify potential therapeutic targets (31). Major ATP production shifts from mitochondrial respiration to aerobic glycolysis in a number of tumors, a process termed the Warburg phenomenon (32, 33). However, some tumors rely on oxidative metabolism as their main energy source in certain contexts (34), including melanomas (35), lymphomas (36), and pancreatic (37) and pulmonary carcinomas (38). A variety of common Food and Drug Administration-approved drugs, such as metformin, arsenic trioxide, and atovaquone, act as OxPhos inhibitors and have potential as anti-neoplastic drugs, especially in the setting of tumors with unregulated oxidative phosphorylation (39). Moreover, mammalian target of rapamycin (mTOR) is a pivotal serine/threonine kinase upstream of metabolic pathways, including OxPhos, and thus also constitutes a novel therapeutic target (40, 41). One of its complex forms, mTORC1, is deregulated in DLBCL (42). mTORC1 inhibitors, known as rapalogs, kill DLBCL cell lines in vitro, but unfortunately show limited efficacy in patients with DLBCL, underlining the continuing unmet need for novel treatments targeting this molecular pathway (42). The three genes uchl1, s100a8, and s100a12, enriched in the canine OxPhos subgroup of our study, have interesting functional implications. Uchl1 encodes ubiquitin C-terminal hydrolase L1 (UCHL1), a de-ubiquinating enzyme that plays a pivotal role in maintaining cellular homeostasis under physiological conditions of oxidative stress (13). UCHL1 has variable roles in cancers, depending on histotype: for instance, the expression of UCHL1 correlates with poor prognosis in patients with multiple myeloma (43), mammary carcinoma (44), and pulmonary carcinoma (44), but has tumor suppressive properties in nasopharyngeal (45) and prostatic carcinoma (46). Both S100A8 and S100A12 are members of the calcium-binding S100 protein family (47), expression of which correlates with inflammation-associated carcinogenesis (48). However, S100A8 may also be anti-inflammatory and acts as an oxidant scavenger during oxidative stress and inflammation (14). Moreover, high expression of S100A8 and S100A12 has a positive prognostic impact on oropharyngeal squamous cell carcinoma (48). The functions of S100A8 and S100A12 are therefore broad and context-dependent. Taken together, these three genes may be potential biomarkers and therapeutic targets of OxPhos-DLBCL in both dogs and humans, given their prognostic impact in other cancers and correlation with oxidative stress imposed by an abundance of reactive oxygen species as a consequence of vigorous OxPhos (49). Furthermore, EIF2 signaling is induced by various cellular stresses, including those of oxidative origin, consolidating the view that this molecular subtype is distinguished by overactive OxPhos.
Future work will aim to provide additional insight into the genes uchl1, s100a8, and s100a12 in both canine and human DLBCL to confirm observations made in the current pilot study. First, distinction of OxPhos- and non-OxPhos-DLBCL will be validated in a larger cohort of canine cases using the 527 differentially expressed genes. Second, protein expression of the three genes will be examined in OxPhos- vs. non-OxPhos-DLBCL in both species, using immunohistochemistry in conjunction with flow cytometry and Western blots. The genes with a conserved expression pattern may be investigated for their functional impact in knockdown studies performed in vitro and in vivo, the latter requiring rodent models. Third, expression of the three genes may also be examined in DLBCL subtypes identified by the COO scheme to further interrogate the degree of overlap and prognostic significance of these two classification schemes. For instance, high expression of uchl1 identifies human GCB-DLBCL patients likely to have a poor outcome (50). Meticulous longitudinal studies in both species will ultimately yield a better understanding of the prognostic impact of uchl1, s100a8, and s100a12 in DLBCL.
In summary, our study has revealed hitherto unrecognized metabolic heterogeneity of multicentric BCL in dogs that resembles that of human DLBCL. These data yield potentially interesting therapeutic targets for canine lymphoma and substantiate the dog as a model for human NHL, further validating its use in the advancement of novel therapies of relevance to human health.
Data Availability Statement
Raw and processed RNA-seq data of the 11 canine samples in this study have been deposited to Gene Expression Omnibus (GEO), accession number GSE140603.
Ethics Statement
The animal study was reviewed and approved by Royal Veterinary College (RVC) Ethics and Welfare Committee (Permit Number: URN 2014 1285) in the United Kingdom. Written informed consent was obtained from the owners for the participation of their animals in this study.
Author Contributions
YW conducted the entire study and wrote the initial draft of the manuscript. Y-MC performed survival analysis and volcano plot gene annotation. GP, AS, NB, KK, and MM recruited and sampled clinical cases. BS and LP conducted cytological review of all clinical cases and making the definitive diagnoses. AS and SP co-supervised YW on project conduct and data interpretation. SS followed up outcome of canine patients at the RVC. ES performed initial RNA-seq analysis and provided scripts for basic transcriptomic analysis. MG helped with initial outcome data input in survival analysis. JG co-supervised YW and co-funded the study. DX contributed expertise and intellectual input on all the transcriptomic data interpretation, and co-supervised YW in the last year of the study. OG conceived and funded the entire study, served as the principal supervisor of YW, and edited the initial manuscript. All authors reviewed and approved the final manuscript.
Funding
This study was supported by the Petplan Charitable Trust, Royal Veterinary College, and Barts Cancer Institute.
Conflict of Interest
The authors declare that the research was conducted in the absence of any commercial or financial relationships that could be construed as a potential conflict of interest.
Acknowledgments
We are grateful to all the owners who volunteered their dogs to this study. We wish to thank North Downs Specialist Referrals, the Queen Mother Hospital for Animals, and Fitzpatrick Referrals for recruiting and sampling all of the clinical cases. We also wish to thank the Oxford Genomics Centre at the Wellcome Centre for Human Genetics (funded by Wellcome Trust grant reference 203141/Z/16/Z) for performing RNA sequencing and initial data analysis.
Supplementary Material
The Supplementary Material for this article can be found online at: https://www.frontiersin.org/articles/10.3389/fonc.2020.00307/full#supplementary-material
References
1. Alizadeh AA, Eisen MB, Davis RE, Ma C, Lossos IS, Rosenwald A, et al. Distinct types of diffuse large B-cell lymphoma identified by gene expression profiling. Nature. (2000) 403:503–11. doi: 10.1038/35000501
2. Wright G, Tan B, Rosenwald A, Hurt EH, Wiestner A, Staudt LM. A gene expression-based method to diagnose clinically distinct subgroups of diffuse large B cell lymphoma. Proc Natl Acad Sci USA. (2003) 100:9991–6. doi: 10.1073/pnas.1732008100
3. Monti S, Savage KJ, Kutok JL, Feuerhake F, Kurtin P, Mihm M, et al. Molecular profiling of diffuse large B-cell lymphoma identifies robust subtypes including one characterized by host inflammatory response. Blood. (2005) 105:1851–61. doi: 10.1182/blood-2004-07-2947
4. Caro P, Kishan AU, Norberg E, Stanley IA, Chapuy B, Ficarro SB, et al. Metabolic signatures uncover distinct targets in molecular subsets of diffuse large B cell lymphoma. Cancer Cell. (2012) 22:547–60. doi: 10.1016/j.ccr.2012.08.014
5. Garden OA, Pinheiro D, Cunningham F. All creatures great and small: regulatory T cells in mice, humans, dogs and other domestic animal species. Int Immunopharmacol. (2011) 11:576–88. doi: 10.1016/j.intimp.2010.11.003
6. Marconato L, Gelain ME, Comazzi S. The dog as a possible animal model for human non-Hodgkin lymphoma: a review. Hematol Oncol. (2013) 31:1–9. doi: 10.1002/hon.2017
7. Richards KL, Suter SE. Man's best friend: what can pet dogs teach us about non-Hodgkin's lymphoma? Immunol. Rev. (2015) 263:173–91. doi: 10.1111/imr.12238
8. Pinheiro D, Chang YM, Bryant H, Szladovits B, Dalessandri T, Davison LJ, et al. Dissecting the regulatory microenvironment of a large animal model of non-Hodgkin lymphoma: evidence of a negative prognostic impact of FOXP3+ T cells in canine B cell lymphoma. PLoS ONE. (2013) 9:e105027.doi: 10.1371/journal.pone.0105027
9. Richards KL, Motsinger-Reif AA, Chen HW, Fedoriw Y, Fan C, Nielsen DM, et al. Gene profiling of canine B-cell lymphoma reveals germinal center and postgerminal center subtypes with different survival times, modeling human DLBCL. Cancer Res. (2013) 73:5029–39. doi: 10.1158/0008-5472.CAN-12-3546
10. Wu Y, Chang YM, Stell AJ, Priestnall SL, Sharma E, Goulart MR, et al. Phenotypic characterisation of regulatory T cells in dogs reveals signature transcripts conserved in humans and mice. Sci Rep. (2019) 9:13478. doi: 10.1038/s41598-019-50065-8
11. Robinson MD, McCarthy DJ, Smyth GK. EdgeR: a Bioconductor package for differential expression analysis of digital gene expression data. Bioinformatics. (2010) 26:139–40. doi: 10.1093/bioinformatics/btp616
12. Lenz G, Wright GW, Emre NC, Kohlhammer H, Dave SS, Davis RE, et al. Molecular subtypes of diffuse large B-cell lymphoma arise by distinct genetic pathways. Proc Natl Acad Sci USA. (2008) 105:13520–5. doi: 10.1073/pnas.0804295105
13. Shen H, Sikorska M, Leblanc J, Walker PR, Liu QY. Oxidative stress regulated expression of ubiquitin Carboxyl-terminal Hydrolase-L1: role in cell survival. Apoptosis. (2006) 11:1049–59. doi: 10.1007/s10495-006-6303-8
14. Gomes LH, Raftery MJ, Yan WX, Goyette JD, Thomas PS, Geczy CL. S100A8 and S100A9-oxidant scavengers in inflammation. Free Radic Biol Med. (2013) 58:170–86. doi: 10.1016/j.freeradbiomed.2012.12.012
15. Cheng X, Wu H, Jin ZJ, Ma D, Yuen S, Jing XQ, et al. Up-regulation of chemokine receptor CCR4 is associated with Human Hepatocellular Carcinoma malignant behavior. Sci Rep. (2017) 7:12362. doi: 10.1038/s41598-017-10267-4
16. Karasaki T, Qiang G, Anraku M, Sun Y, Shinozaki-Ushiku A, Sato E, et al. High CCR4 expression in the tumor microenvironment is a poor prognostic indicator in lung adenocarcinoma. J Thorac Dis. (2018) 10:4741–50. doi: 10.21037/jtd.2018.07.45
17. Li YF, Zhu GY, Ma Y, Qu HY. Expression and prognosis of MYOZ2 in gastric cancer. Eur Rev Med Pharmacol Sci. (2018) 22:5920–27. doi: 10.26355/eurrev_201809_15921
18. Zhang X, Wan JX, Ke ZP, Wang F, Chai HX, Liu JQ. TMEM88, CCL14 and CLEC3B as prognostic biomarkers for prognosis and palindromia of human hepatocellular carcinoma. Tumour Biol. (2017) 39:1010428317708900. doi: 10.1177/1010428317708900
19. Zhu HF, Zhang XH, Gu CS, Zhong Y, Long T, Ma YD, et al. Cancer-associated fibroblasts promote colorectal cancer progression by secreting CLEC3B. Cancer Biol Ther. (2019) 20:967–78. doi: 10.1080/15384047.2019.1591122
20. Ghanta KS, Li D-Q, Eswaran J, Kumar R. Gene profiling of Mta1 identifies novel gene targets and functions. PLoS ONE. (2011) 6:e17135. doi: 10.1371/journal.pone.0017135
21. Yoshida T, Kobayashi T, Itoda M, Muto T, Miyaguchi K, Mogushi K, et al. Clinical omics analysis of colorectal cancer incorporating copy number aberrations and gene expression data. Cancer Inform. (2010) 29:147–61. doi: 10.4137/CIN.S3851
22. Thirunavukkarasan M, Wang C, Rao A, Hind T, Teo YR, Siddiquee AA, et al. Short-chain fatty acid receptors inhibit invasive phenotypes in breast cancer cells. PLoS ONE. (2017) 12:e0186334. doi: 10.1371/journal.pone.0186334
23. Kudo T, Kigoshi H, Hagiwara T, Takino T, Yamazaki M, Yui S. Cathepsin G, a neutrophil protease, induces compact cell-cell adhesion in MCF-7 human breast cancer cells. Mediators Inflamm. (2009) 2009:850940. doi: 10.1155/2009/850940
24. Kandasamy P, Gyimesi G, Kanai Y, Hediger MA. Amino acid transporters revisited: new views in health and disease. Trends Biochem Sci. (2018) 43:752–89. doi: 10.1016/j.tibs.2018.05.003
25. Carta E, Chung SK, James VM, Robinson A, Gill JL, Remy N, et al. Mutations in the GlyT2 gene (SLC6A5) are a second major cause of startle disease. J Biol Chem. (2012) 287:28975–85. doi: 10.1074/jbc.M112.372094
26. Lenz G, Wright G, Dave SS, Xiao W, Powell J, Zhao H, et al. Stromal gene signatures in large-B-cell lymphomas. N Engl J Med. (2008) 359:2313–23. doi: 10.1056/NEJMoa0802885
27. Chiche J, Reverso-Meinietti J, Mouchotte A, Rubio-Patiño C, Mhaidly R, Villa E, et al. GAPDH expression predicts the response to R-CHOP, the tumor metabolic status, and the response of DLBCL patients to metabolic inhibitors. Cell Metab. (2019) 29:1243–57.e10. doi: 10.1016/j.cmet.2019.02.002
28. DeBerardinis RJ, Lum JJ, Hatzivassiliou G, Thompson CB. The biology of cancer: metabolic reprogramming fuels cell growth and proliferation. Cell Metab. (2008) 7:11–20. doi: 10.1016/j.cmet.2007.10.002
29. Tennant DA, Duran RV, Boulahbel H, Gottlieb E. Metabolic transformation in cancer. Carcinogenesis. (2009) 30:1269–80. doi: 10.1093/carcin/bgp070
30. King A, Gottlieb E. Glucose metabolism and programmed cell death: an evolutionary and mechanistic perspective. Curr Opin Cell Biol. (2009) 21:885–93. doi: 10.1016/j.ceb.2009.09.009
31. Tennant DA, Duran RV, Gottlieb E. Targeting metabolic transformation for cancer therapy. Nat Rev Cancer. (2010) 10:267–77. doi: 10.1038/nrc2817
32. Weinhouse S, Warburg O, Burk D, Schade AL. On respiratory impairment in cancer cells. Science. (1956) 124:267–72. doi: 10.1126/science.124.3215.267
33. Vander Heiden MG, Cantley LC, Thompson CB. Understanding the Warburg effect: the metabolic requirements of cell proliferation. Science. (2009) 324:1029–33. doi: 10.1126/science.1160809
34. Koppenol WH, Bounds PL, Dang CV. Otto Warburg's contributions to current concepts of cancer metabolism. Nat Rev Cancer. (2011) 11:325–37. doi: 10.1038/nrc3038
35. Vazquez F, Lim JH, Chim H, Bhalla K, Girnun G, Pierce K, et al. PGC1alpha expression defines a subset of human melanoma tumors with increased mitochondrial capacity and resistance to oxidative stress. Cancer Cell. (2013) 23:287–301. doi: 10.1016/j.ccr.2012.11.020
36. Deberardinis RJ. A mitochondrial power play in lymphoma. Cancer Cell. (2012) 22:423–4. doi: 10.1016/j.ccr.2012.09.023
37. Viale A, Pettazzoni P, Lyssiotis CA, Ying H, Sánchez N, Marchesini M, et al. Oncogene ablation-resistant pancreatic cancer cells depend on mitochondrial function. Nature. (2014) 514:628–32. doi: 10.1038/nature13611
38. Hensley CT, Faubert B, Yuan Q, Lev-Cohain N, Jin E, Kim J, et al. Metabolic heterogeneity in human lung tumors. Cell. (2016) 164:681–94. doi: 10.1016/j.cell.2015.12.034
39. Ashton TM, McKenna WG, Kunz-Schughart LA, Higgins GS. Oxidative phosphorylation as an emerging target in cancer therapy. Clin Cancer Res. (2018) 24:2482–90. doi: 10.1158/1078-0432.CCR-17-3070
40. Cunningham JT, Rodgers JT, Arlow DH, Vazquez F, Mootha VK, Puigserver P. mTOR controls mitochondrial oxidative function through a YY1-PGC-1alpha transcriptional complex. Nature. (2007) 450:736–40. doi: 10.1038/nature06322
41. Morita M, Gravel SP, Chénard V, Sikström K, Zheng L, Alain T, et al. mTORC1 controls mitochondrial activity and biogenesis through 4E-BP-dependent translational regulation. Cell Metab. (2013) 18:698–711. doi: 10.1016/j.cmet.2013.10.001
42. Ricci JE, Chiche J. Metabolic reprogramming of non-Hodgkin's B-cell lymphomas and potential therapeutic strategies. Front Oncol. (2018) 8:556. doi: 10.3389/fonc.2018.00556
43. Hussain S, Bedekovics T, Chesi M, Bergsagel PL, Galardy PJ. UCHL1 is a biomarker of aggressive multiple myeloma required for disease progression. Oncotarget. (2015) 6:40704–18. doi: 10.18632/oncotarget.5727
44. Goto Y, Zeng L, Yeom CJ, Zhu Y, Morinibu A, Shinomiya K, et al. UCHL1 provides diagnostic and antimetastatic strategies due to its deubiquitinating effect on HIF-1alpha. Nat Commun. (2015) 6:6153. doi: 10.1038/ncomms7153
45. Li L, Tao Q, Jin H, van Hasselt A, Poon FF, Wang X, et al. The tumor suppressor UCHL1 forms a complex with p53/MDM2/ARF to promote p53 signaling and is frequently silenced in nasopharyngeal carcinoma. Clin Cancer Res. (2010) 16:2949–58. doi: 10.1158/1078-0432.CCR-09-3178
46. Ummanni R, Jost E, Braig M, Lohmann F, Mundt F, Barett C, et al. Ubiquitin carboxyl-terminal hydrolase 1 (UCHL1) is a potential tumour suppressor in prostate cancer and is frequently silenced by promoter methylation. Mol Cancer. (2011) 10:29. doi: 10.1186/1476-4598-10-129
47. Donato R, Cannon BR, Sorci G, Riuzzi F, Hsu K, Weber DJ, et al. Functions of S100 proteins. Curr Mol Med. (2013) 13:24–57. doi: 10.2174/156652413804486214
48. Funk S, Mark R, Bayo P, Flechtenmacher C, Grabe N, Angel P, et al. High S100A8 and S100A12 protein expression is a favorable prognostic factor for survival of oropharyngeal squamous cell carcinoma. Int J Cancer. (2015) 136:2037–46. doi: 10.1002/ijc.29262
49. Nita M, Grzybowski A. The role of the reactive oxygen species and oxidative stress in the pathomechanism of the age-related ocular diseases and other pathologies of the anterior and posterior eye segments in adults. Oxid Med Cell Longev. (2016) 2016:3164734. doi: 10.1155/2016/3164734
Keywords: diffuse large B cell lymphoma, dog, animal model, gene expression, metabolism, oxidative phosphorylation
Citation: Wu Y, Chang Y-M, Polton G, Stell AJ, Szladovits B, Macfarlane M, Peters LM, Priestnall SL, Bacon NJ, Kow K, Stewart S, Sharma E, Goulart MR, Gribben J, Xia D and Garden OA (2020) Gene Expression Profiling of B Cell Lymphoma in Dogs Reveals Dichotomous Metabolic Signatures Distinguished by Oxidative Phosphorylation. Front. Oncol. 10:307. doi: 10.3389/fonc.2020.00307
Received: 25 November 2019; Accepted: 20 February 2020;
Published: 06 March 2020.
Edited by:
Laurence de Leval, Lausanne University Hospital (CHUV), SwitzerlandReviewed by:
Yi Miao, First Affiliated Hospital, Nanjing Medical University, ChinaYasodha Natkunam, Stanford University, United States
Copyright © 2020 Wu, Chang, Polton, Stell, Szladovits, Macfarlane, Peters, Priestnall, Bacon, Kow, Stewart, Sharma, Goulart, Gribben, Xia and Garden. This is an open-access article distributed under the terms of the Creative Commons Attribution License (CC BY). The use, distribution or reproduction in other forums is permitted, provided the original author(s) and the copyright owner(s) are credited and that the original publication in this journal is cited, in accordance with accepted academic practice. No use, distribution or reproduction is permitted which does not comply with these terms.
*Correspondence: Oliver A. Garden, b2dhcmRlbkB1cGVubi5lZHU=
†ORCID: Ying Wu, orcid.org/0000-0002-2264-438X
Yu-Mei Chang, orcid.org/0000-0001-6388-9626
Balazs Szladovits, orcid.org/0000-0002-1926-3455
Laureen M. Peters, orcid.org/0000-0002-6302-4240
Simon L. Priestnall, orcid.org/0000-0002-6027-1879
Nicholas J. Bacon, orcid.org/0000-0001-5190-5342
Eshita Sharma, orcid.org/0000-0002-8663-7365
Michelle R. Goulart, orcid.org/0000-0001-8333-3908
John Gribben, orcid.org/0000-0002-8505-7430
Dong Xia, orcid.org/0000-0003-4571-2776
Oliver A. Garden, orcid.org/0000-0002-4133-9487
‡Present address: Ying Wu, School of Veterinary Medicine, University of Pennsylvania, Philadelphia, PA, United States
Kelvin Kow, Port City Veterinary Referral Hospital, Portsmouth, NH, United States
Michelle R. Goulart, Barts Cancer Institute, Queen Mary University of London, London, United Kingdom
§These authors share senior authorship