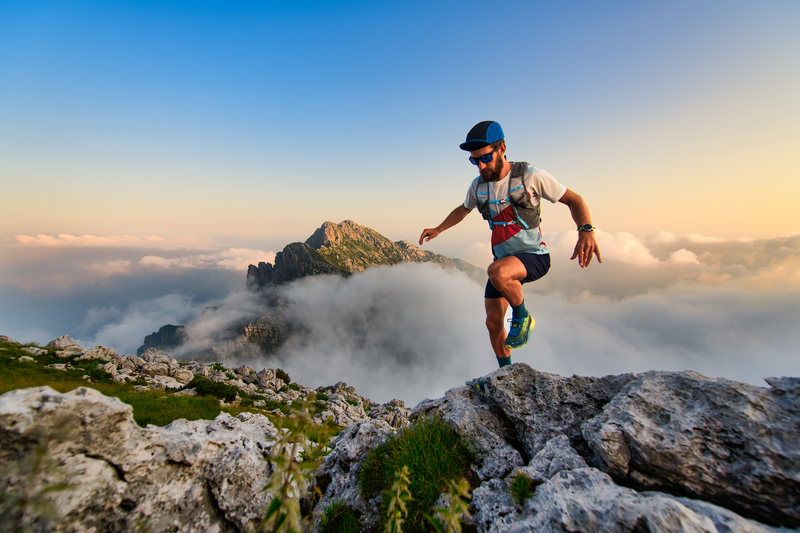
94% of researchers rate our articles as excellent or good
Learn more about the work of our research integrity team to safeguard the quality of each article we publish.
Find out more
MINI REVIEW article
Front. Oncol. , 30 September 2019
Sec. Radiation Oncology
Volume 9 - 2019 | https://doi.org/10.3389/fonc.2019.00983
This article is part of the Research Topic Magnetic Resonance Imaging for Radiation Therapy View all 17 articles
Magnetic resonance imaging provides a sea of quantitative and semi-quantitative data. While radiation oncologists already navigate a pool of clinical (semantic) and imaging data, the tide will swell with the advent of hybrid MRI/linear accelerator devices and increasing interest in MRI-guided radiotherapy (MRIgRT), including adaptive MRIgRT. The variety of MR sequences (of greater complexity than the single parameter Hounsfield unit of CT scanning routinely used in radiotherapy), the workflow of adaptive fractionation, and the sheer quantity of daily images acquired are challenges for scaling this technology. Biomedical informatics, which is the science of information in biomedicine, can provide helpful insights for this looming transition. Funneling MRIgRT data into clinically meaningful information streams requires committing to the flow of inter-institutional data accessibility and interoperability initiatives, standardizing MRIgRT dosimetry methods, streamlining MR linear accelerator workflow, and standardizing MRI acquisition and post-processing. This review will attempt to conceptually ford these topics using clinical informatics approaches as a theoretical bridge.
Use of magnetic resonance imaging (MRI) rather than computed tomography (CT) for radiotherapy (RT) planning can be highly desirable because MRI visualizes soft tissues with superior contrast and resolution (1), introduces unique sequences and contrast agents for delineating specific tumors and anatomic subsites (1, 2), and permits daily adaptive radiotherapy (ART) without added CT radiation dose (3–5). MRI-guided ART (MRIgART) machines have advanced from low-field (0.35 Tesla) magnets with Cobalt-60 radiation sources (6) to diagnostic-strength magnetic fields (1.5 Tesla) fully integrated with linear accelerators (7, 8) in <5 years. Over the coming decade, MRI-guided RT (MRIgRT) may change clinical practice paradigms (9). The earliest adopter of MRIgART, Washington University in St. Louis (WUSTL), has already altered its management of breast and abdominal malignancies (10). However, to scale MRIgRT, workflow and standardization challenges that do not exist in CT-guided planning need be resolved.
First, MR scan reproducibility is more complicated than for CT. Consider a T1-weighted scan: pixel intensities are predominately derived from longitudinal relaxation time (T1), an intrinsic tissue property. Nevertheless, proton density (H) and transverse relaxation time (T2) (which are also intrinsic tissue properties) may greatly influence overall signal intensity (11) depending on repetition time (TR) and echo time (TE) parameters (see Equation 1).
These parameters are not standardized across institutions or vendors, so a T1-weighted scan acquired by a given vendor's machine is not necessarily equivalent in terms of observed intensity as one acquired by another manufacturer. Similarly, MRI acquisition suffers from geometric distortions that are model-, vendor-, software-, shim-, and coil-dependent. Proper correction also depends on variable user-driven acquisition parameters (12, 13).
Second, there are more steps in MRIgRT planning than CT-guided planning. MRI does not convey electron density information necessary for standard photon dosimetry, so either (1) MRI data must be registered to CT Hounsfield unit values (14–17), or (2) a synthetic CT (sCT) must be algorithmically generated from MRI (18, 19), or (3) tissue types must be assigned a single, indiscriminate density (18) (Figure 1). Additionally, MRIgART fractionation requires far more time of the patient, radiation oncologist, and staff than traditional RT treatment courses.
Figure 1. RT planning with MRI requires assignment of electron density to pixels or tissues to calculate dose, a step that is not part of CT-guided RT planning.
Third, RT generates seas of imaging data (20, 21) and structured and unstructured clinical data (22–24) that will deepen with multiparametric MRI sequences, unique contrast agents, and radiomics features and MRIgART daily images, contours, and plans (Figure 2). At our institution, MRIgRT generates roughly four times as many bytes of data as CT-guided RT (1 Gb per patient per day vs. 250 Mb). Not all data are fit for clinical decision-making or scientific inquiry. For example, MRIgART could quantitatively track soft tissue tumor shrinkage, but the results would only be clinically actionable if the segmentation method were systematic and reproduceable. Interpretability and reproducibility of MRI data across institutions and vendors is not a given.
Figure 2. MRI-guided radiotherapy may introduce a deluge of new image sequences, optimization needs, image post-processing needs, contrast agents, prognostic and predictive radiomics features, and adaptive imaging and clinical data.
Effective use of “biomedical data, information, and knowledge for scientific inquiry, problem-solving and decision making” formally defines the field of biomedical informatics (BMI) (25). The raison d'être of BMI is to reduce data (which are meaningless symbols) into information (which is data plus meaning), and further into knowledge (which is information that is justifiably believed to be true) (26). This paper considers BMI concepts in the context of scaling MRIgRT (see Table 1) and critiques existing literature from the perspective of how it increases information and knowledge to streamline MRIgRT workflow and ensure the consistency and usability of MRIgRT data.
MRI is already an established modality for image-guided RT of nasopharynx, brain, spine, liver, pancreas, prostate, and female genital tract cancers (1, 2, 32). In each case, standardization preserves the integrity of critical decision-making information. Consider MRIgRT for prostate cancer (33, 34). Radiology standards exist for MRI acquisition, interpretation, and reporting (35). These improve reporting among radiologists of varying experience levels (36), lest anatomic delineation suffer poor consistency and patient outcomes comparison data be meaningless. At the MR-CT co-registration step, co-registration between limited field-of-view images is the recommended standard because error is increased when the field-of-view includes the anatomically variable bladder and rectum (37). At the RT planning step, guidelines from the European Society for Radiotherapy and Oncology (ESTRO) (38) and Radiation Therapy Oncology Group (RTOG) (39) standardize MRI-based clinical target and organ-at-risk (OAR) contour volumes. Ostensibly, these steps culminate in more conformal prostate RT, but MRIgRT has proved only modest decreases in OAR toxicity compared to CT-guided RT (40, 41), especially with the development of rectal spacer hydrogel (42). Evaluating data quality and the assumptions used to establish the clinical value of MRIgRT will be a critical BMI task in the coming decade, one that should exploit emerging consumer health informatics approaches.
As already noted, MRIgRT requires either MRI-CT co-registration, sCT generation, or bulk density assignment to calculate tissue radiation dose. MR-only workflows employ either the second or third approach (with the caveat that atlas-based sCT generation techniques employ MRI-CT co-registration to generate an MRI atlas). Improving MR-only RT is strongly motivated by the desire to simplify adaptive workflow for integrated magnetic resonance linear accelerators (MRLs). Figure 3 exemplifies an imputed electron density map in a patient treated on an MRL. We refer the reader to Table 1 in (34) for an overview of current MRL platforms.
Figure 3. This patient was reirradiated for a rectal adenocarcinoma recurrence on a hybrid magnetic resonance-guided linear accelerator. The planning image (top) is an electron density imputation based on the simulation MRI (bottom).
Homogenous bulk density override is crude but achieves reasonable dosimetric accuracy if specific structures (e.g., cortical bone) are contoured by a radiation oncologist and separately assigned a unique density (43–45). In contrast, sCT generation by voxel-based or atlas-based methods obviates the need for time-intensive contouring and therefore may be preferred. Johnstone et al. extensively discussed sCT generation methods in a systematic review (18). Many sCT results appear clinically comparable to CT. In the brain, sCT-derived digitally reconstructed radiographs were as geometrically robust as those derived from CT (46). In the prostate, sCT gamma passing rates have been comparable with CT gamma passing rates (median 1%/1 mm pass rate of 100% for almost all regions of interest across 29 patient scans) (47). Nevertheless, MR-only workflow introduces unique BMI considerations. For example, prostate RT plans are more precise with setup to intraprostatic gold or titanium fiducial implants (48), but these are visualized as signal void on conventional MRI sequences and poorly differentiated from calcifications. Maspero et al. (49) reported that 3/48 fiducial implants were imprecisely and inaccurately identified by five radiation technologists when visualized only on MRI. On the other hand, new setup techniques based on MR daily imaging might obviate the need for fiducials. Thoughtful consideration of parameters like these are needed to ensure not only the safety of the method but the quality and reproducibility of the data. Consensus is also needed to establish the standard metrics by which sCT quality should be gauged (18).
MRIs can be registered to CT rigidly (without warping the MRI) or by a deformation vector field. Deformable registration confers a more concordant result than rigid registration between diagnostic CT and simulation CT (50–53), but recent work from our group did not demonstrate the same advantage between simulation MRI and simulation CT, at least in the head and neck (17). This should not imply that rigid registration is adequately accurate, since we also found that the registration error (whether by deformable or rigid means) may not be within the target tolerance recommended by the American Association of Physicists in Medicine (AAPM) Task Group 132 (Dice similarity coefficient > 0.8) (54). Perhaps registration was poor because of MR geometric distortion, or perhaps because not all OARs are clearly delineated on both CT and MRI. Regardless, this informs our view that sCT may be preferred to CT-MRI co-registration for RT dose deposition calculation, pending needed standardizations as discussed above.
The “holy grail” of MRL RT is to see the target at setup, adapt the plan as needed, and gate by watching anatomic movement while the beam is on. The experience of the Department of Radiation Oncology at WUSTL, which introduced the first 0.35 T tri-cobalt-60 MRIgRT system (ViewRay, Oakwood Village, OH, USA) in the USA (6), provides great insight into adaptive MRL clinical informatics challenges. In a Phase I trial intended to demonstrate the temporal feasibility of MRI-guided stereotactic body radiation (SBRT), median on-table time per fraction was 79 min and consisted of MR set-up, physician arrival, patient localization, re-segmentation, re-planning, quality assurance (QA), and beam on-time (3). Almost all fractions (81/97) were adapted based on the patient's anatomy-of-day to avoid irradiating OARs. Despite fear that patients would not tolerate fractions longer than 80 min, all 20 patients completed their treatments as prescribed.
MRL RT has evolved into a dominant indication for abdominal and breast cancers at WUSTL, primarily because motion gating and daily adaptation prevent OAR dose constraint violations (10). The MRL has also prevented violations in hypofractionated lung tumor stereotactic radiotherapy, and enabled adaptive GTV reductions by as much as 65% (55). However, adaptation remains time-intensive. Current systems require physician attendance during every fraction (56), which would not be sustainable at sites that lack sufficient physician and support staff. Three studies from the University of Alberta examined whether automated ROI segmentation can decrease the burden on physician time. In the first, a pulse-coupled neural network (PCNN) was developed to segment lung tumors in the context of adaptive MRL RT (57). The PCNN achieved a strong Dice Similarity Index (DSI) of 0.87–0.92, but it required training on a unique dataset of manually-generated contours per patient. A follow-up study improved DSI (58) with a pre-segmentation deformable registration methodology, but still required a physician to segment lung tumor across multiple image frames. In the third study, DSI and other conformality metrics improved using a fully convolutional neural network (FCNN), but the FCNN still needed to be trained on 30 manual contours per patient. While these studies demonstrate that automated segmentations of lung tumors for MRL RT can achieve high fidelity, they may not hasten adaptive, online MRL workflow. In contrast, a WUSTL novel tri-convolutional neural network architecture capable of segmenting liver, kidneys, stomach, bowel, and duodenum did reduce manual segmentation time by 75% at WUSTL (59).
Intra and inter-observer variation in segmentation quality has been documented using many imaging modalities in pelvic (60, 61), lung (62), breast (63), head-and-neck (60, 64), and brain (65) RT planning. In the specific context of MRL for lung stereotactic body RT, Wee et al. (66) found no significant intra or inter-observer variation in manual segmentations of images acquired on a 0.35 T MRL. However, only two radiation oncologist observers were compared, for only one ROI (gross tumor volume) (66), limiting the generalizability of the study conclusion.
To hasten MRL re-planning, WUSTL simplified the number of planning objectives by grouping OAR structures into a single structure (67). This both increased PTV coverage and simplified re-planning by reducing the computational burden of satisfying a greater number of competing objectives. This work was specific to pancreatic cancer planning objectives, but the approach may be amenable to re-planning for other sites.
Intrafraction motion management/gating is a hotly anticipated MRL advantage. Han et al. (68) applied 3D-Rotating Cartesian K-space MRI (4D-ROCK-MRI) in an MRL RT workflow to improve lung tumor motion tracking. 4D-ROCK-MRI improved image quality and motion tracking and decreased lung cancer GTV variability compared with 4D-CT, which suffers from 2D-slice “stitching” artifact. The authors reason that it might capture motion better than 2D-CINE MRI because it acquires data over a 7 min interval, while the latter screens less than a minute of data. Cusumano et al. (69) compared 4D-CT and 2D-cine MR motion data acquired at the time of simulation with complete 2D-cine MR datasets acquired over entire MRIgRT treatment courses. Simulation 2D-cine MR appeared better than simulation 4D-CT, though not significantly. Patients with large motion amplitudes at the time of simulation tended to have more variable amplitudes throughout their treatment course, but even targets with steady amplitudes frequently drifted from the motion trajectory calculated at simulation. Drift was as severe as 1.6 cm craniocaudally and 1.2 cm anteroposteriorly, which highlights the importance of continual IGRT monitoring throughout treatment. Palacios et al. (70) tracked adrenal metastases and discovered that one-third of the time anatomy positioning violated OAR or target dose constraints. van Sornsen de Koste et al. (71) followed lung, adrenal, and pancreatic tumor GTVs with 2D-cine MRI. In 90% of cases these tumors oscillated no more than 6 mm anteroposteriorly and 9 mm craniocaudally. Mean coverage was better than 94% of the GTV volume for all three tumor types (coverage was defined as a 3 mm isotropic GTV expansion).
ART discussions encompass many other considerations beyond the scope of this paper, but we highlight one more: both US commercial hybrid MRL systems use what Heukelom et al. (5) define as “serial ART” (i.e., daily images are registered to a planning scan serially without interval dose accumulation) but can conceivably be utilized for “triggered ART” (when fixed interval re-planning offline occurs) or “cascade ART” (when serial deformed dose is integrated from prior treatments). Consequently, a need for new ways of visualizing and reporting dose and morphometric alterations will soon arise. Centers that lack MRL machines but are interested in MRIgRT for abdominal cancers may find the workflow outlined in Heerkens et al. (72) informative. This phase I trial demonstrated a favorable toxicity profile (no treatment-attributable grade 3 acute or late toxicities) in 20 patients with unresectable pancreatic cancer who received 24 Gy/3 fx SBRT planned with multiparametric MRI sequences and sagittal cine MRI.
The use of imaging biomarkers for diagnosis and prognosis is the field known as radiomics (73), or radiogenomics if the biomarkers are both radiomic and genomic (74, 75). MRI radiomics features have predicted tumor histopathology (76, 77), improved region-of-interest (ROI) auto-segmentation (78), automated radiotherapy planning (79) and predicted outcomes (e.g., survival, toxicity) (80–82). However, standardization of radiomics feature parameters is needed across radiation oncology, radiology, and nuclear medicine disciplines (83). In a systematic review of MRI radiomics applications, Jethanandani et al. concluded that MRI radiomics studies suffer from lack of standardization at multiple stages of image acquisition and processing, including MRI scanner sequence, scanner vendor, and scan acquisition parameters. There is currently no way to reliably compare between MRI radiomics studies (84). MRI has not nearly enjoyed the attention given to CT and PET radiomics standardization. Traverso et al. (85) systematically reviewed studies that assessed the repeatability and reproducibility radiomics features, finding only 1/41 papers (86) that investigated MRI.
Radiomics models should be commissioned from their ideation with a clinical decision support use case in mind (87). Studies designed to maximize the likelihood of a statistically significant finding at the expense of clinical generalizability ignore that practical implementation is a greater obstacle than discovery. To illustrate, one study that discriminated triple-negative from other breast cancer types using radiomics features ostensibly aspires to be a diagnostic alternative to biopsy (88), but would need to be less expensive yet no less accurate—a steep challenge.
Radiomics feature stability should be benchmarked on public, multi-institutional datasets (85, 89). For example, Bakas et al. (90) publicly provided radiomics features manually extracted from neuroradiologist segmentations of glioblastomas and low-grade gliomas for benchmarking future studies of these cancers. Stability should be benchmarked per anatomic site, since features that are repeatable and reproduceable at one site may degrade in the context of another.
Inter-institutional findable, accessible, interoperable, reusable (FAIR) (91) and high-quality data is essential for establishing the clinical value of MRIgRT. However, political, financial, and legal obstacles silo data within institutions (92) and ethical questions surrounding health data analytics, particularly by tech institutions currently not subject to the same patient privacy laws as healthcare institutions, are unresolved (93, 94). The need for FAIR data is not exclusive to MRIgRT: FAIR data are critical for achieving the vision for machine learning in healthcare widely (95–97).
A recent AAPM council observed that RT data increase as cancer patients survive longer and genomic data move toward mainstream clinical use (98). The council predicted, “Whereas success in medical research in the past has favored very large single institutions that can develop a critical mass of knowledge and resources in close physical proximity, diffuse networks of institutions able to generate and share information will have an advantage in the future” (emphasis added). We now conclude with a discussion of two emerging initiatives for FAIRer data: “distributed learning,” a method for inter-institutional machine learning, and Fast Healthcare Interoperability Resources (FHIR, pronounced “fire”) a healthcare data standard.
Distributed learning refers to training machine learning models on multi-institutional data without sharing the data (99–101). The key is that the statistical weights and parameters of the machine learning model travel between institutions, not the data. Distributed learning is an option method for generating statistical models for emerging technologies, such as MRLs (7, 8). Distributed learning is possible between horizontally-partitioned data (same features, different patients) or vertically-partitioned data (different features, same patients) (101, 102).
Conceived in 2014, FHIR is a specification for health data formatting (i.e., XML and JSON) and messaging (i.e., RESTful application programming interfaces). FHIR-conforming data are retrievable between health information technology softwares (103), and FHIR may soon be a mandated EHR specification (104). FHIR provides a standard for storing and querying radiotherapy data objects independent of vendor, such as total dose, or DICOM-RT structure sets. Conformity with FHIR also makes it possible to build applications that integrate health information technologies. For example, Substitutable Medical Applications and Reusable Technologies (SMART) is an EHR app platform built on FHIR (105). SMART delineates authorization, authentication, and user interface specifications for FHIR-conforming apps. Because RT treatment planning and information systems are usually separate from EHRs (98, 106), initiatives like SMART on FHIR envision a future where it is possible to build RT task-specific apps into EHRs (107). Open-source, FHIR-conforming applications may be one platform for scaling MRIgRT software.
KK and CF proposed the idea for this manuscript. KK drafted the manuscript. KK, CF, JW, and BS iteratively revised the manuscript.
CF has received funding from the National Institute for Dental and Craniofacial Research Award (1R01DE025248-01/R56DE025248) and Academic-Industrial Partnership Award (R01 DE028290), the National Science Foundation (NSF), Division of Mathematical Sciences, Joint NIH/NSF Initiative on Quantitative Approaches to Biomedical Big Data (QuBBD) Grant (NSF 1557679), the NIH Big Data to Knowledge (BD2K) Program of the National Cancer Institute (NCI) Early Stage Development of Technologies in Biomedical Computing, Informatics, and Big Data Science Award (1R01CA214825), the NCI Early Phase Clinical Trials in Imaging and Image-Guided Interventions Program (1R01CA218148), the NIH/NCI Cancer Center Support Grant (CCSG) Pilot Research Program Award from the UT MD Anderson CCSG Radiation Oncology and Cancer Imaging Program (P30CA016672), the NIH/NCI Head and Neck Specialized Programs of Research Excellence (SPORE) Developmental Research Program Award (P50 CA097007) and the National Institute of Biomedical Imaging and Bioengineering (NIBIB) Research Education Program (R25EB025787) as well as direct industry grant support, speaking honoraria and travel funding from Elekta AB. BS has received funding from the Cancer Prevention & Research Institute of Texas (RP160674), NIH R01 CA207216-01 and is an Andrew Sabin Family Fellow. JW has received research funding from NIH, Elekta AB, and GE Medical.
CF has received direct industry grant support, speaking honoraria, and travel funding from Elekta AB. BS has intellectual property licensed to Oncora Medical.
The remaining authors declare that the research was conducted in the absence of any commercial or financial relationships that could be construed as a potential conflict of interest.
1. Chandarana H, Wang H, Tijssen RHN, Das IJ. Emerging role of MRI in radiation therapy. J Magn Reson Imaging. (2018) 48:1468–78. doi: 10.1002/jmri.26271
2. McWilliam A, Rowland B, van Herk M. The challenges of using MRI during Radiotherapy. Clin Oncol. (2018) 30:680–5. doi: 10.1016/j.clon.2018.08.004
3. Henke L, Kashani R, Robinson C, Curcuru A, DeWees T, Bradley J, et al. Phase I trial of stereotactic MR-guided online adaptive radiation therapy (SMART) for the treatment of oligometastatic or unresectable primary malignancies of the abdomen. Radiother Oncol. (2018) 126:519–26. doi: 10.1016/j.radonc.2017.11.032
4. Lim-Reinders S, Keller BM, Al-Ward S, Sahgal A, Kim A. Online adaptive radiation therapy. Int J Radiat Oncol Biol Phys. (2017) 99:994–1003. doi: 10.1016/j.ijrobp.2017.04.023
5. Heukelom J, Fuller CD. Head and neck cancer Adaptive Radiation Therapy (ART): conceptual considerations for the informed clinician. Semin Radiat Oncol. (2019) 29:258–73. doi: 10.1016/j.semradonc.2019.02.008
6. Mutic S, Dempsey JF. The ViewRay system: magnetic resonance–guided and controlled radiotherapy. Semin Radiat Oncol. (2014) 24:196–9. doi: 10.1016/j.semradonc.2014.02.008
7. Raaymakers BW, Jürgenliemk-Schulz IM, Bol GH, Glitzner M, Kotte ANTJ, van Asselen B, et al. First patients treated with a 1.5 T MRI-LINAC: clinical proof of concept of a high-precision, high-field MRI guided radiotherapy treatment. Phys Med Biol. (2017) 62:L41–50. doi: 10.1088/1361-6560/aa9517
8. Wendler R. Cutting-Edge MRI-Guided Radiation Therapy Provides Real-Time View of Tumors. Houston, TX: The University of Texas MD Anderson Cancer Center (2019). Available online at:https://www.mdanderson.org/publications/cancer-frontline/2019/collaboration-leads-to-fda-approval-of-cutting-edge-radiation-tr.html
9. Bayouth JE, Low DA, Zaidi H. MRI-linac systems will replace conventional IGRT systems within 15 years. Med Phys. (2019) 46:3753–6. doi: 10.1002/mp.13657
10. Henke LE, Contreras JA, Green OL, Cai B, Kim H, Roach MC, et al. Magnetic resonance image-guided radiotherapy (MRIgRT): A 4.5-year clinical experience. Clin Oncol. (2018) 30:720–7. doi: 10.1016/j.clon.2018.08.010
11. Nitz WR, Reimer P. Contrast mechanisms in MR imaging. Eur Radiol. (1999) 9:1032–46. doi: 10.1007/s003300050789
12. Weygand J, Fuller CD, Ibbott GS, Mohamed AS, Ding Y, Yang J, et al. Spatial precision in magnetic resonance imaging-guided radiation therapy: the role of geometric distortion. Int J Radiat Oncol Biol Phys. (2016) 95:1304–16. doi: 10.1016/j.ijrobp.2016.02.059
13. Mohamed ASR, Hansen C, Weygand J, Ding Y, Frank SJ, Rosenthal DI, et al. Prospective analysis of in vivo landmark point-based MRI geometric distortion in head and neck cancer patients scanned in immobilized radiation treatment position: Results of a prospective quality assurance protocol. Clin Transl Radiat Oncol. (2017) 7:13–9. doi: 10.1016/j.ctro.2017.09.003
14. Wang X, Li L, Hu C, Qiu J, Xu Z, Feng Y. A comparative study of three CT and MRI registration algorithms in nasopharyngeal carcinoma. J Appl Clin Med Phys. (2009) 10:2906. doi: 10.1120/jacmp.v10i2.2906
15. Fortunati V, Verhaart RF, Angeloni F, Van Der Lugt A, Niessen WJ, Veenland JF, et al. Feasibility of multimodal deformable registration for head and neck tumor treatment planning. Int J Radiat Oncol Biol Phys. (2014) 90:85–93. doi: 10.1016/j.ijrobp.2014.05.027
16. Webster GJ, Kilgallon JE, Ho KF, Rowbottom CG, Slevin NJ, Mackay RI. A novel imaging technique for fusion of high-quality immobilised MR images of the head and neck with CT scans for radiotherapy target delineation. Br J Radiol. (2009) 82:497–503. doi: 10.1259/bjr/50709041
17. Kiser KJ, Meheissen MAM, Mohamed ASR, Kamal M, Ng SP, Elhalawani H, et al. Prospective quantitative quality assurance and deformation estimation of MRI-CT image registration in simulation of head and neck radiotherapy patients. Clin Transl Radiat Oncol. (2019) 18:120–7. doi: 10.1016/j.ctro.2019.04.018
18. Johnstone E, Wyatt JJ, Henry AM, Short SC, Sebag-Montefiore D, Murray L, et al. Systematic review of synthetic computed tomography generation methodologies for use in magnetic resonance imaging-only radiation therapy. Int J Radiat Oncol Biol Phys. (2018) 100:199–217. doi: 10.1016/j.ijrobp.2017.08.043
19. Edmund JM, Nyholm T. A review of substitute CT generation for MRI-only radiation therapy. Radiat Oncol. (2017) 12:28–42. doi: 10.1186/s13014-016-0747-y
20. Law MY, Liu B, Chan LW. Informatics in radiology: DICOM-RT-based electronic patient record information system for radiation therapy. Radiographics. (2009) 29:961–72. doi: 10.1148/rg.294085073
21. Thariat J, Marcy PY, Lacout A, Ramus L, Girinsky T, Pointreau Y, et al. Radiotherapy and radiology: joint efforts for modern radiation planning and practice. Diagn Interv Imaging. (2012) 93:342–50. doi: 10.1016/j.diii.2012.02.004
22. Kreimeyer K, Foster M, Pandey A, Arya N, Halford G, Jones SF, et al. Natural language processing systems for capturing and standardizing unstructured clinical information: a systematic review. J Biomed Inform. (2017) 73:14–29. doi: 10.1016/j.jbi.2017.07.012
23. Dreisbach C, Koleck TA, Bourne PE, Bakken S. A systematic review of natural language processing and text mining of symptoms from electronic patient-authored text data. Int J Med Inform. (2019) 125:37–46. doi: 10.1016/j.ijmedinf.2019.02.008
24. Mayo CS, Moran JM, Bosch W, Xiao Y, McNutt T, Popple R, et al. American association of physicists in medicine task group 263: standardizing nomenclatures in radiation oncology. Int J Radiat Oncol Biol Phys. (2018) 100:1057–66. doi: 10.1016/j.ijrobp.2017.12.013
25. Kulikowski CA, Shortliffe EH, Currie LM, Elkin PL, Hunter LE, Johnson TR, et al. AMIA Board white paper: definition of biomedical informatics and specification of core competencies for graduate education in the discipline. J Am Med Inform Assoc. (2012) 19:931–8. doi: 10.1136/amiajnl-2012-001053
26. Bernstam EV, Smith JW, Johnson TR. What is biomedical informatics? J Biomed Inform. (2010) 43:104–10. doi: 10.1016/j.jbi.2009.08.006
27. Rowley J. The wisdom hierarchy: representations of the DIKW hierarchy. J Inform Sci. (2007) 33:163–80. doi: 10.1177/0165551506070706
28. Shortliffe EH, Blois MS. The computer meets medicine and biology: emergence of a discipline. In: Shortliffe EH, Cimino JJ, editors. Biomedical Informatics: Computer Applications in Health Care and Biomedicine, 3rd ed. New York, NY: Springer Science + Business Media, LLC (2006). doi: 10.1007/0-387-36278-9-1
29. Anonymous. ISO/IEC Guide 2: Standardization and Related Activities – General Vocabulary. Geneva: International Organization for Standardization (2004).
30. Anonymous. Standards Glossary: Interoperability: Institute of Electrical and Electronics Engineers. (2016). Available online at: https://www.standardsuniversity.org/article/standards-glossary/ (accessed June 27, 2019).
31. Hersh WR. Standards and Interoperability. Health Informatics: Practical Guide, 7th ed. Informatics Education. (2018). Available online at: lulu.com (accessed June 27, 2019).
32. Fraass BA, Eisbruch A, Feng M. Intensity-modulated and image-guided radiation therapy. In: Gunderson LL, Tepper JE, editors. Clinical Radiation Oncology, 4th ed. Philadelphia, PA: Elsevier (2016). p. 294–324. doi: 10.1016/B978-0-323-24098-7.00016-2
33. Gkika E, Oehlke O, Bunea H, Wiedenmann N, Adebahr S, Nestle U, et al. Biological imaging for individualized therapy in radiation oncology: part II medical and clinical aspects. Future Oncol. (2018) 14:751–69. doi: 10.2217/fon-2017-0465
34. Pathmanathan AU, van As NJ, Kerkmeijer LGW, Christodouleas J, Lawton CAF, Vesprini D, et al. Magnetic resonance imaging-guided adaptive radiation therapy: a “Game Changer” for prostate treatment? Int J Radiat Oncol Biol Phys. (2018) 100:361–73. doi: 10.1016/j.ijrobp.2017.10.020
35. Weinreb JC, Barentsz JO, Choyke PL, Cornud F, Haider MA, Macura KJ, et al. PI-RADS prostate imaging - reporting and data system: 2015, version 2. Eur Urol. (2016) 69:16–40. doi: 10.1016/j.eururo.2015.08.052
36. Greer MD, Shih JH, Lay N, Barrett T, Bittencourt L, Borofsky S, et al. Interreader variability of prostate imaging reporting and data system version 2 in detecting and assessing prostate cancer lesions at prostate MRI. AJR Am J Roentgenol. (2019) 212:1–8. doi: 10.2214/AJR.18.20536
37. Menard C, Paulson E, Nyholm T, McLaughlin P, Liney G, Dirix P, et al. Role of prostate MR imaging in radiation oncology. Radiol Clin North Am. (2018) 56:319–25. doi: 10.1016/j.rcl.2017.10.012
38. Salembier C, Villeirs G, De Bari B, Hoskin P, Pieters BR, Van Vulpen M, et al. ESTRO ACROP consensus guideline on CT- and MRI-based target volume delineation for primary radiation therapy of localized prostate cancer. Radiother Oncol. (2018) 127:49–61. doi: 10.1016/j.radonc.2018.01.014
39. Contouring atlases: Radiation Therapy Oncology Group (RTOG). RTOG Contouring Consensus Guidelines for Various Anatomic Sites. (2019). Available online at: https://www.rtog.org/CoreLab/ContouringAtlases.aspx (accessed April 4, 2019).
40. Ali AN, Rossi PJ, Godette KD, Martin D, Liauw S, Vijayakumar S, et al. Impact of magnetic resonance imaging on computed tomography-based treatment planning and acute toxicity for prostate cancer patients treated with intensity modulated radiation therapy. Pract Radiat Oncol. (2013) 3:e1–9. doi: 10.1016/j.prro.2012.04.005
41. Sander L, Langkilde NC, Holmberg M, Carl J. MRI target delineation may reduce long-term toxicity after prostate radiotherapy. Acta Oncol. (2014) 53:809–14. doi: 10.3109/0284186X.2013.865077
42. Hamstra DA, Mariados N, Sylvester J, Shah D, Karsh L, Hudes R, et al. Continued benefit to rectal separation for prostate radiation therapy: final results of a phase III trial. Int J Radiat Oncol Biol Phys. (2017) 97:976–85. doi: 10.1016/j.ijrobp.2016.12.024
43. Karotki A, Mah K, Meijer G, Meltsner M. Comparison of bulk electron density and voxel-based electron density treatment planning. J Appl Clin Med Phys. (2011) 12:3522. doi: 10.1120/jacmp.v12i4.3522
44. Jonsson JH, Karlsson MG, Karlsson M, Nyholm T. Treatment planning using MRI data: an analysis of the dose calculation accuracy for different treatment regions. Radiat Oncol. (2010) 5:62. doi: 10.1186/1748-717X-5-62
45. Lambert J, Greer PB, Menk F, Patterson J, Parker J, Dahl K, et al. MRI-guided prostate radiation therapy planning: Investigation of dosimetric accuracy of MRI-based dose planning. Radiother Oncol. (2011) 98:330–4. doi: 10.1016/j.radonc.2011.01.012
46. Morris ED, Price RG, Kim J, Schultz L, Siddiqui MS, Chetty I, et al. Using synthetic CT for partial brain radiation therapy: impact on image guidance. Pract Radiat Oncol. (2018) 8:342–50. doi: 10.1016/j.prro.2018.04.001
47. Christiansen RL, Jensen HR, Brink C. Magnetic resonance only workflow and validation of dose calculations for radiotherapy of prostate cancer. Acta Oncol. (2017) 56:787–91. doi: 10.1080/0284186X.2017.1290275
48. Zaorsky NG, Showalter TN, Ezzell GA, Nguyen PL, Assimos DG, D'Amico AV, et al. ACR Appropriateness Criteria for external beam radiation therapy treatment planning for clinically localized prostate cancer, part II of II. Adv Radiat Oncol. (2017) 2:437–54. doi: 10.1016/j.adro.2017.03.003
49. Maspero M, Seevinck PR, Willems NJW, Sikkes GG, de Kogel GJ, de Boer HCJ, et al. Evaluation of gold fiducial marker manual localisation for magnetic resonance-only prostate radiotherapy. Radiat Oncol. (2018) 13:105. doi: 10.1186/s13014-018-1029-7
50. Mohamed AS, Ruangskul MN, Awan MJ, Baron CA, Kalpathy-Cramer J, Castillo R, et al. Quality assurance assessment of diagnostic and radiation therapy-simulation CT image registration for head and neck radiation therapy: anatomic region of interest-based comparison of rigid and deformable algorithms. Radiology. (2015) 274:752–63. doi: 10.1148/radiol.14132871
51. Guy CL, Weiss E, Che S, Jan N, Zhao S, Rosu-Bubulac M. Evaluation of image registration accuracy for tumor and organs at risk in the thorax for compliance with TG 132 recommendations. Adv Radiat Oncol. (2019) 4:177–85. doi: 10.1016/j.adro.2018.08.023
52. Sarudis S, Karlsson A, Bibac D, Nyman J, Back A. Evaluation of deformable image registration accuracy for CT images of the thorax region. Phys Med. (2019) 57:191–9. doi: 10.1016/j.ejmp.2018.12.030
53. Motegi K, Tachibana H, Motegi A, Hotta K, Baba H, Akimoto T. Usefulness of hybrid deformable image registration algorithms in prostate radiation therapy. J Appl Clin Med Phys. (2019) 20:229–36. doi: 10.1002/acm2.12515
54. Brock KK, Mutic S, McNutt TR, Li H, Kessler ML. Use of image registration and fusion algorithms and techniques in radiotherapy: report of the AAPM radiation therapy committee task Group No. 132. Med Phys. (2017) 44:e43–76. doi: 10.1002/mp.12256
55. Henke LE, Kashani R, Hilliard J, DeWees TA, Curcuru A, Przybysz D, et al. In silico trial of mr-guided midtreatment adaptive planning for hypofractionated stereotactic radiation therapy in centrally located thoracic tumors. Int J Radiat Oncol Biol Phys. (2018) 102:987–95. doi: 10.1016/j.ijrobp.2018.06.022
56. Kashani R, Olsen JR. Magnetic resonance imaging for target delineation and daily treatment modification. Semin Radiat Oncol. (2018) 28:178–84. doi: 10.1016/j.semradonc.2018.02.002
57. Yun J, Yip E, Gabos Z, Wachowicz K, Rathee S, Fallone BG. Neural-network based autocontouring algorithm for intrafractional lung-tumor tracking using Linac-MR. Med Phys. (2015) 42:2296–310. doi: 10.1118/1.4916657
58. Tahmasebi N, Boulanger P, Yun J, Fallone BG, Punithakumar K. Tracking tumor boundary using point correspondence for adaptive radio therapy. Comput Methods Programs Biomed. (2018) 165:187–95. doi: 10.1016/j.cmpb.2018.08.002
59. Fu Y, Mazur TR, Wu X, Liu S, Chang X, Lu Y, et al. A novel MRI segmentation method using CNN -based correction network for MRI -guided adaptive radiotherapy. Med Phys. (2018) 45:5129–37. doi: 10.1002/mp.13221
60. Riegel AC, Antone JG, Zhang H, Jain P, Raince J, Rea A, et al. Deformable image registration and interobserver variation in contour propagation for radiation therapy planning. J Appl Clin Med Phys. (2016) 17:347–57. doi: 10.1120/jacmp.v17i3.6110
61. Hellebust TP, Tanderup K, Lervag C, Fidarova E, Berger D, Malinen E, et al. Dosimetric impact of interobserver variability in MRI-based delineation for cervical cancer brachytherapy. Radiother Oncol. (2013) 107:13–9. doi: 10.1016/j.radonc.2012.12.017
62. Persson GF, Nygaard DE, Hollensen C, Munck af Rosenschold P, Mouritsen LS, Due AK, et al. Interobserver delineation variation in lung tumour stereotactic body radiotherapy. Br J Radiol. (2012) 85:e654–60. doi: 10.1259/bjr/76424694
63. Yang J, Woodward WA, Reed VK, Strom EA, Perkins GH, Tereffe W, et al. Statistical modeling approach to quantitative analysis of interobserver variability in breast contouring. Int J Radiat Oncol Biol Phys. (2014) 89:214–21. doi: 10.1016/j.ijrobp.2014.01.010
64. Mukesh M, Benson R, Jena R, Hoole A, Roques T, Scrase C, et al. Interobserver variation in clinical target volume and organs at risk segmentation in post-parotidectomy radiotherapy: can segmentation protocols help? Br J Radiol. (2012) 85:e530–6. doi: 10.1259/bjr/66693547
65. Stanley J, Dunscombe P, Lau H, Burns P, Lim G, Liu HW, et al. The effect of contouring variability on dosimetric parameters for brain metastases treated with stereotactic radiosurgery. Int J Radiat Oncol Biol Phys. (2013) 87:924–31. doi: 10.1016/j.ijrobp.2013.09.013
66. Wee CW, An HJ, Kang HC, Kim HJ, Wu HG. Variability of gross tumor volume delineation for stereotactic body radiotherapy of the lung with Tri-(60)Co magnetic resonance image-guided radiotherapy system (viewray): a comparative study with magnetic resonance- and computed tomography-based target delineation. Technol Cancer Res Treat. (2018) 17:1533033818787383. doi: 10.1177/1533033818787383
67. Olberg S, Green O, Cai B, Yang D, Rodriguez V, Zhang H, et al. Optimization of treatment planning workflow and tumor coverage during daily adaptive magnetic resonance image guided radiation therapy (MR-IGRT) of pancreatic cancer. Radiat Oncol. (2018) 13:51. doi: 10.1186/s13014-018-1000-7
68. Han F, Zhou Z, Du D, Gao Y, Rashid S, Cao M, et al. Respiratory motion-resolved, self-gated 4D-MRI using Rotating Cartesian K-space (ROCK): initial clinical experience on an MRI-guided radiotherapy system. Radiother Oncol. (2018) 127:467–73. doi: 10.1016/j.radonc.2018.04.029
69. Cusumano D, Dhont J, Boldrini L, Chiloiro G, Teodoli S, Massaccesi M, et al. Predicting tumour motion during the whole radiotherapy treatment: a systematic approach for thoracic and abdominal lesions based on real time MR. Radiother Oncol. (2018) 129:456–62. doi: 10.1016/j.radonc.2018.07.025
70. Palacios MA, Bohoudi O, Bruynzeel AME, van Sorsen de Koste JR, Cobussen P, Slotman BJ, et al. Role of daily plan adaptation in MR-guided stereotactic ablative radiation therapy for adrenal metastases. Int J Radiat Oncol Biol Phys. (2018) 102:426–33. doi: 10.1016/j.ijrobp.2018.06.002
71. van Sornsen de Koste JR, Palacios MA, Bruynzeel AME, Slotman BJ, Senan S, Lagerwaard FJ. MR-guided gated stereotactic radiation therapy delivery for lung, adrenal, and pancreatic tumors: a geometric analysis. Int J Radiat Oncol Biol Phys. (2018) 102:858–66. doi: 10.1016/j.ijrobp.2018.05.048
72. Heerkens HD, van Vulpen M, Erickson B, Reerink O, Intven MP, van den Berg CA, et al. MRI guided stereotactic radiotherapy for locally advanced pancreatic cancer. Br J Radiol. (2018) 91:20170563. doi: 10.1259/bjr.20170563
73. Aerts HJ, Velazquez ER, Leijenaar RT, Parmar C, Grossmann P, Carvalho S, et al. Decoding tumour phenotype by noninvasive imaging using a quantitative radiomics approach. Nat Commun. (2014) 5:4006. doi: 10.1038/ncomms5644
74. Peeken JC, Nusslin F, Combs SE. “Radio-oncomics”: the potential of radiomics in radiation oncology. Strahlenther Onkol. (2017) 193:767–79. doi: 10.1007/s00066-017-1175-0
75. Andreassen CN, Schack LM, Laursen LV, Alsner J. Radiogenomics - current status, challenges and future directions. Cancer Lett. (2016) 382:127–36. doi: 10.1016/j.canlet.2016.01.035
76. Dang M, Lysack JT, Wu T, Matthews TW, Chandarana SP, Brockton NT, et al. MRI texture analysis predicts p53 status in head and neck squamous cell carcinoma. AJNR Am J Neuroradiol. (2015) 36:166–70. doi: 10.3174/ajnr.A4110
77. Meyer HJ, Schob S, Hohn AK, Surov A. MRI texture analysis reflects histopathology parameters in thyroid cancer - a first preliminary study. Transl Oncol. (2017) 10:911–6. doi: 10.1016/j.tranon.2017.09.003
78. Yang X, Wu N, Cheng G, Zhou Z, Yu DS, Beitler JJ, et al. Automated segmentation of the parotid gland based on atlas registration and machine learning: a longitudinal MRI study in head-and-neck radiation therapy. Int J Radiat Oncol Biol Phys. (2014) 90:1225–33. doi: 10.1016/j.ijrobp.2014.08.350
79. Shiradkar R, Podder TK, Algohary A, Viswanath S, Ellis RJ, Madabhushi A. Radiomics based targeted radiotherapy planning (Rad-TRaP): a computational framework for prostate cancer treatment planning with MRI. Radiat Oncol. (2016) 11:148. doi: 10.1186/s13014-016-0718-3
80. Zhang B, Ouyang F, Gu D, Dong Y, Zhang L, Mo X, et al. Advanced nasopharyngeal carcinoma: pre-treatment prediction of progression based on multi-parametric MRI radiomics. Oncotarget. (2017) 8:72457–65. doi: 10.18632/oncotarget.19799
81. Zhang B, He X, Ouyang F, Gu D, Dong Y, Zhang L, et al. Radiomic machine-learning classifiers for prognostic biomarkers of advanced nasopharyngeal carcinoma. Cancer Lett. (2017) 403:21–7. doi: 10.1016/j.canlet.2017.06.004
82. Thor M, Tyagi N, Hatzoglou V, Apte A, Saleh Z, Riaz N, et al. A magnetic resonance Imaging-based approach to quantify radiation-induced normal tissue injuries applied to trismus in head and neck cancer. Phys Imaging Radiat Oncol. (2017) 1:34–40. doi: 10.1016/j.phro.2017.02.006
83. Gainey M, Carles M, Mix M, Meyer PT, Bock M, Grosu AL, et al. Biological imaging for individualized therapy in radiation oncology: part I physical and technical aspects. Future Oncol. (2018) 14:737–49. doi: 10.2217/fon-2017-0464
84. Jethanandani A, Lin TA, Volpe S, Elhalawani H, Mohamed ASR, Yang P, et al. Exploring applications of radiomics in magnetic resonance imaging of head and neck cancer: a systematic review. Front Oncol. (2018) 8:131. doi: 10.3389/fonc.2018.00131
85. Traverso A, Wee L, Dekker A, Gillies R. Repeatability and reproducibility of radiomic features: a systematic review. Int J Radiat Oncol Biol Phys. (2018) 102:1143–58. doi: 10.1016/j.ijrobp.2018.05.053
86. Guan Y, Li W, Jiang Z, Chen Y, Liu S, He J, et al. Whole-lesion apparent diffusion coefficient-based entropy-related parameters for characterizing cervical cancers: initial findings. Acad Radiol. (2016) 23:1559–67. doi: 10.1016/j.acra.2016.08.010
87. McNutt TR, Benedict SH, Low DA, Moore K, Shpitser I, Jiang W, et al. Using big data analytics to advance precision radiation oncology. Int J Radiat Oncol Biol Phys. (2018) 101:285–91. doi: 10.1016/j.ijrobp.2018.02.028
88. Wang J, Kato F, Oyama-Manabe N, Li R, Cui Y, Tha KK, et al. Identifying triple-negative breast cancer using background parenchymal enhancement heterogeneity on dynamic contrast-enhanced mri: a pilot radiomics study. PLoS ONE. (2015) 10:e0143308. doi: 10.1371/journal.pone.0143308
89. Bi WL, Hosny A, Schabath MB, Giger ML, Birkbak NJ, Mehrtash A, et al. Artificial intelligence in cancer imaging: clinical challenges and applications. CA Cancer J Clin. (2019) 69:127–57. doi: 10.3322/caac.21552
90. Bakas S, Akbari H, Sotiras A, Bilello M, Rozycki M, Kirby JS, et al. Advancing the cancer genome atlas glioma MRI collections with expert segmentation labels and radiomic features. Sci Data. (2017) 4:170117. doi: 10.1038/sdata.2017.117
91. Wilkinson MD, Dumontier M, Aalbersberg IJ, Appleton G, Axton M, Baak A, et al. The FAIR Guiding Principles for scientific data management and stewardship. Sci Data. (2016) 3:160018. doi: 10.1038/sdata.2016.18
92. Kiser KJ, Fuller CD, Reed VK. Artificial intelligence in radiation oncology treatment planning: a brief overview. J Med Artif Intell. (2019) 10:e2475. doi: 10.21037/jmai.2019.04.02
93. Parasidis E, Pike E, McGraw D. A belmont report for health data. N Engl J Med. (2019) 380:1493–5. doi: 10.1056/NEJMp1816373
94. Rajkomar A, Hardt M, Howell MD, Corrado G, Chin MH. Ensuring fairness in machine learning to advance health equity. Ann Intern Med. (2018) 169:866–72. doi: 10.7326/M18-1990
95. Rajkomar A, Dean J, Kohane I. Machine learning in medicine. N Engl J Med. (2019) 380:1347–58. doi: 10.1056/NEJMra1814259
96. Esteva A, Robicquet A, Ramsundar B, Kuleshov V, DePristo M, Chou K, et al. A guide to deep learning in healthcare. Nat Med. (2019) 25:24–9. doi: 10.1038/s41591-018-0316-z
97. Hinton G. Deep learning-a technology with the potential to transform health care. JAMA. (2018) 320:1101–2. doi: 10.1001/jama.2018.11100
98. Mayo CS, Phillips M, McNutt TR, Palta J, Dekker A, Miller RC, et al. Treatment data and technical process challenges for practical big data efforts in radiation oncology. Med Phys. (2018) 45:e793–810. doi: 10.1002/mp.13114
99. Jochems A, Deist TM, van Soest J, Eble M, Bulens P, Coucke P, et al. Distributed learning: developing a predictive model based on data from multiple hospitals without data leaving the hospital – a real life proof of concept. Radiother Oncol. (2016) 121:459–67. doi: 10.1016/j.radonc.2016.10.002
100. Jochems A, Deist TM, El Naqa I, Kessler M, Mayo C, Reeves J, et al. Developing and validating a survival prediction model for NSCLC patients through distributed learning across 3 countries. Int J Radiat Oncol Biol Phys. (2017) 99:344–52. doi: 10.1016/j.ijrobp.2017.04.021
101. Wu Y, Jiang X, Kim J, Ohno-Machado L. Grid Binary LOgistic REgression (GLORE): building shared models without sharing data. J Am Med Inform Assoc. (2012) 19:758–64. doi: 10.1136/amiajnl-2012-000862
102. Li Y, Jiang X, Wang S, Xiong H, Ohno-Machado L. VERTIcal Grid lOgistic regression (VERTIGO). J Am Med Inform Assoc. (2016) 23:570–9. doi: 10.1093/jamia/ocv146
103. Welcome to FHIR [Web Page]. HL7 International. (2018). Available online at: http://hl7.org/fhir/ (accessed December 27, 2018).
104. HHS Proposes New Rules to Improve the Interoperability of Electronic Health Information. New Innovations in Technology Promote Patient Access and Could Make No-Cost Health Data Exchange a Reality for Millions. Washington, DC: US Department of Health & Human Services. (2019). Available online at: https://www.hhs.gov/about/news/2019/02/11/hhs-proposes-new-rules-improve-interoperability-electronic-health-information.html
105. Cannon R. SMART on FHIR: The Basics [Video]: InterSystems Developer Community (2018). Available online at: https://www.youtube.com/watch?v=MMfe181tmwU&t=6s/ (accessed April 20, 2019).
106. Pan HY, Mazur LM, Martin NE, Mayo CS, Santanam L, Pawlicki T, et al. Radiation oncology health information technology: is it working for or against us? Int J Radiat Oncol Biol Phys. (2017) 98:259–62. doi: 10.1016/j.ijrobp.2017.02.018
Keywords: MRI, MRI-guided radiotherapy, MR LINAC, informatics, biomedical informatics, clinical informatics, imaging informatics, radiomics
Citation: Kiser KJ, Smith BD, Wang J and Fuller CD (2019) “Après Mois, Le Déluge”: Preparing for the Coming Data Flood in the MRI-Guided Radiotherapy Era. Front. Oncol. 9:983. doi: 10.3389/fonc.2019.00983
Received: 12 July 2019; Accepted: 16 September 2019;
Published: 30 September 2019.
Edited by:
Jing Cai, Hong Kong Polytechnic University, Hong KongReviewed by:
Sunyoung Jang, Princeton Radiation Oncology Center, United StatesCopyright © 2019 Kiser, Smith, Wang and Fuller. This is an open-access article distributed under the terms of the Creative Commons Attribution License (CC BY). The use, distribution or reproduction in other forums is permitted, provided the original author(s) and the copyright owner(s) are credited and that the original publication in this journal is cited, in accordance with accepted academic practice. No use, distribution or reproduction is permitted which does not comply with these terms.
*Correspondence: Clifton D. Fuller, CDFuller@mdanderson.org
Disclaimer: All claims expressed in this article are solely those of the authors and do not necessarily represent those of their affiliated organizations, or those of the publisher, the editors and the reviewers. Any product that may be evaluated in this article or claim that may be made by its manufacturer is not guaranteed or endorsed by the publisher.
Research integrity at Frontiers
Learn more about the work of our research integrity team to safeguard the quality of each article we publish.