- 1The University of Queensland Diamantina Institute, Translational Research Institute, The University of Queensland, Brisbane, QLD, Australia
- 2Discipline of Dermatology, University of Sydney, Sydney, NSW, Australia
Drug resistance constitutes a major challenge in designing melanoma therapies. Microenvironment-driven tumor heterogeneity and plasticity play a key role in this phenomenon. Melanoma is highly heterogeneous with diverse genomic alterations and expression of different biological markers. In addition, melanoma cells are highly plastic and capable of adapting quickly to changing microenvironmental conditions. These contribute to variations in therapy response and durability between individual melanoma patients. In response to changing microenvironmental conditions, like hypoxia and nutrient starvation, proliferative melanoma cells can switch to an invasive slow-cycling state. Cells in this state are more aggressive and metastatic, and show increased intrinsic drug resistance. During continuous treatment, slow-cycling cells are enriched within the tumor and give rise to a new proliferative subpopulation with increased drug resistance, by exerting their stem cell-like behavior and phenotypic plasticity. In melanoma, the proliferative and invasive states are defined by high and low microphthalmia-associated transcription factor (MITF) expression, respectively. It has been observed that in MITFhigh melanomas, inhibition of MITF increases the efficacy of targeted therapies and delays the acquisition of drug resistance. Contrarily, MITF is downregulated in melanomas with acquired drug resistance. According to the phenotype switching theory, the gene expression profile of the MITFlow state is predominantly regulated by WNT5A, AXL, and NF-κB signaling. Thus, different combinations of therapies should be effective in treating different phases of melanoma, such as the combination of targeted therapies with inhibitors of MITF expression during the initial treatment phase, but with inhibitors of WNT5A/AXL/NF-κB signaling during relapse.
Introduction
The development of targeted therapies for metastatic melanoma using small molecule MAPK pathway inhibitors (MAPKi) or immune checkpoint antagonists (ICi) has revolutionized dermatological oncology. However, first-generation MAPKi only works in approximately 35–50% of cases as a BRAFV600 mutation must be present (1, 2). ICi show response rates of up to 60%, depending on drug or combination, and many of these are durable effects (3). Yet, drug resistance constitutes a major challenge for effective cancer treatment with melanoma being no exception. Rapid resistance to MAPKi is common and has also been reported for ICi (4–9). Although the molecular mechanisms leading to inherent and acquired drug resistance have been discussed extensively in the literature, the dynamics leading to resistance are poorly understood but yet critical to designing better treatments. Besides genetic and epigenetic factors, other contributors to drug resistance are microenvironment-driven tumor heterogeneity and plasticity (10–16).
Mechanisms of Intrinsic and Acquired Drug Resistance in Melanoma
Intrinsic refers to a pre-existent drug resistance of the entire population or a subpopulation of cancer cells before exposure to the drug. For example, intrinsically resistant cancer cells do not harbor the targeted mutation or are not dependent on the pathway inhibited by the drug. In the case of acquired drug resistance, the tumor responds initially to the treatment but relapses and progresses later. However, it is difficult to distinguish between intrinsic and acquired resistance as a small subpopulation of intrinsically resistant cancer cells subsequently enriched, may also explain initial response and later relapse (17–20). Causative factors that contribute to MAPKi resistance can be broadly classified into three categories: mutational events, non-mutational events, and changes in the surrounding microenvironment (21). Mutational and non-mutational events that contribute to the development of drug resistance have been discussed previously (21, 22) and are not the focus of this review. In brief, the mechanisms linked with these events predominantly lead to MAPK pathway reactivation and/or activation of parallel signaling pathways (e.g., PI3K/AKT/mTOR) (21, 23). Besides mutational and non-mutational events which are intrinsic to tumor cells, the tumor microenvironment contributes to the development of drug resistance by influencing the crosstalk between distinct cellular compartments. Solid tumors are comprised of tumor cells and stromal cells (e.g., fibroblasts, endothelial cells, and lymphocytes) that form an organ-like structure which is embedded within the extracellular matrix (ECM) and nourished by a vascular network. Each of these components show varying distribution within the tumor resulting in a highly complex and heterogeneous tumor microenvironment (24). In melanoma, secretion of tumor necrosis factor-α (25, 26), hepatocyte growth factor (HGF) (27), Wnt antagonist, sFRP2 (28), and increased production of ECM (29) by stromal cells in the tumor can cause resistance to MAPKi. Thus, the density of stromal cells in different parts of the tumor plays a key role in determining response and resistance to MAPKi. In addition, the distribution of the vasculature plays a crucial role in the acquisition of varying drug resistance mechanisms in different parts of the tumor, due to differences in the levels of nutrients and oxygen. Hypoxia can induce resistance to MAPKi by mediating upregulation of HGF/MET signaling (30), increasing SNAIL, and decreasing E-cadherin expression (31).
Tumor Heterogeneity and Plasticity
Tumor heterogeneity refers to the presence of subpopulations of cells that differ phenotypically and/or by biological behavior, either within a tumor (intra-tumoral) or between tumors of the same histopathological subtype within a patient (inter-tumoral) or between patients (inter-patient) (32). Melanoma heterogeneity plays a key role in the response to MAPKi (5, 20). At the molecular level, the features of different subpopulations are conferred by alterations of the genome, transcriptome, epigenome, and proteome (33, 34). Melanoma is one of the most heterogeneous cancers (35), harboring diverse genomic alterations, including gain of function mutations (e.g., NRAS, BRAF, KIT, CDK4, and MITF), loss of function mutations (e.g., CDKN2A, PTEN, ARID2, and NF), and epigenetic changes (e.g., PTEN, CDKN2A, RAC1, and P53) (36). In addition, various biological markers of melanoma (e.g., CD20, CD133, ABCB5, CD271, JARID1B, and ALDH1) show differential expression patterns in different regions within a tumor (36).
There are three tumor heterogeneity models (37). The well-accepted clonal evolution model (38) refers to acquired additional genetic mutations in cancer cells that contribute to their altered phenotype and malignant potential. This results in a Darwinian-style selection of clones during disease progression (38). The stem cell model suggests that only a small fraction of tumor cells have the potential for maintaining the tumor and drive progression (39). These cancer stem cells have self-renewal capability and can be differentiated into “non-stem cancer cells” that lose their tumorigenic potential by acquiring stable epigenetic changes and occupy the largest fraction of the tumor (37, 39, 40). These two models are complementary to each other, rather than mutually exclusive (41). Their common feature is the unidirectional, irreversible nature of the molecular changes that lead to tumor heterogeneity (37). An alternative model is “phenotypic plasticity” or “phenotype switching.” This model suggests that tumor cells with different phenotypic and functional behavior can dynamically shift between different transcriptional programs (42–44). The different phenotypic states, described in terms of differential gene expression patterns, have been termed “proliferative” and “invasive” signatures (45). In this model, molecular changes resulting in tumor heterogeneity are reversible, unlike the clonal evolution and stem cell models. These changes are predominantly regulated by cues from the surrounding microenvironment, e.g., hypoxia, stroma-derived factors like HGF, TGF-β. For example, in response to hypoxia, proliferative melanoma cells can switch to the invasive phenotype by altering their gene expression profile (10, 46).
Microenvironment-Driven Dynamic Heterogeneity in Melanoma
“Tumor microenvironment” is a broad term, which includes (1) the tumor stroma composed of fibroblasts, endothelial cells, immune cells, soluble molecules, and the ECM, (2) the epidermal microenvironment where the tumor had originated from, and (3) different subcompartments within the tumor itself (47). Interactions between tumor cells and the microenvironment contribute to the malignant behavior of tumor cells, e.g., progression, metastasis, angiogenesis, migration, and invasion (48, 49). In addition, microenvironmental stress signals in response to nutrient starvation and inflammation drive phenotypic plasticity and invasion and determine therapeutic outcome (16, 50). Similarly, a pre-existing immune-active tumor microenvironment is necessary for a favorable response to ipilimumab, and potentially other ICi (51–53).
We have developed a 3D melanoma spheroid model, which recapitulates the in vivo tumor microenvironment and architecture (54, 55), that combined with the fluorescent ubiquitination-based cell cycle indicator (56) is a useful tool to study the microenvironment in vitro (57, 58). This model is being complemented constantly, e.g., by including DRAQ7 as a real-time cell death marker (59) or by applying mathematical algorithms to predict spatial and temporal patterns of cell density and cell cycle (60, 61). Due to an oxygen and nutrient gradient, melanoma spheroids segregate into a continuously proliferating subpopulation in the periphery and a G1-arrested subpopulation in the center (12). A similar phenomenon is observed in human melanoma xenografts in mice, where clusters of cycling cells are located near blood vessels and quiescent cells in central tumor zones (12). After isolating these two subpopulations from spheroids and plating them in 2D culture separately, within 24 h G1-arrested central cells recommence their cell cycle and become indistinguishable from the proliferating peripheral subpopulation (12). This supports the phenotypic plasticity model (10, 23). The cell cycle phase can also contribute to drug sensitivity (13, 62, 63) and can be targeted for cell cycle-tailored melanoma therapy (64). For example, bortezomib preferentially kills melanoma cells in the S/G2/M phase of the cell cycle (15). By contrast, cell cycle arrest can confer tolerance to drugs (14, 64, 65).
The Role of a Slow-Cycling Subpopulation in Melanoma Therapy Resistance
Although dysregulated proliferation is a hallmark of cancer (66, 67), a quiescent or slow-cycling cell subpopulation is reported in many solid cancers, including melanoma. This slow-cycling subpopulation is a major determinant of treatment resistance to targeted therapies (68–70). Increased level of oxidative phosphorylation in slow cycling compared to normal cells (69, 71) contributes to drug resistance in many cancers including melanoma (72–74). MAPKi are predominantly effective in targeting rapidly proliferating cells, while the slow-cycling cells are not readily responsive to MAPKi (69, 75, 76). Thus, cells in the slow-cycling state or cells that switch to this state due to therapeutic stress, can evade the action of MAPKi.
Various mechanisms are utilized by this slow-cycling subpopulation to contribute to drug resistance. First, clonal expansion of the residual slow-cycling cells, that have survived initial treatment, results in their enrichment within the tumor. A recent study suggested that these slow-cycling cells are highly aggressive with increased metastatic potential (77). Second, the slow-cycling subpopulation also displays increased cancer stem cell-like behavior (78). Consistent with the stem cell theory, in melanoma, these slow-cycling cells comprise only 0.5–5% of all tumor cells with self-renewal potential and are defined by the expression of the H3K4 demethylase JARID1B (23). In addition, JARID1B-positive cells are essential for maintaining tumor growth (23). During continuous treatment, slow-cycling cells can gain the potential to differentiate into other cell types with an increased proliferation rate and drug resistance, subsequently resulting in relapse. The cells experience a high level of “therapeutic stress,” forcing them to employ several drug resistance mechanisms. Thus, overtime highly resistant drug tolerant cells are enriched within the tumor and contribute to the highly aggressive and drug resistant nature of metastatic melanoma after relapse. JARID1B-positive cells can give rise to JARID1B-negative cells and also vice versa (23). This supports the phenotype switching theory which indicates the plastic nature of tumor cells that is predominantly influenced by changing microenvironmental conditions (Figure 1). In addition to JARID1B, PGC1α defines another distinct slow-cycling state in melanoma with increased treatment resistance (71, 73).
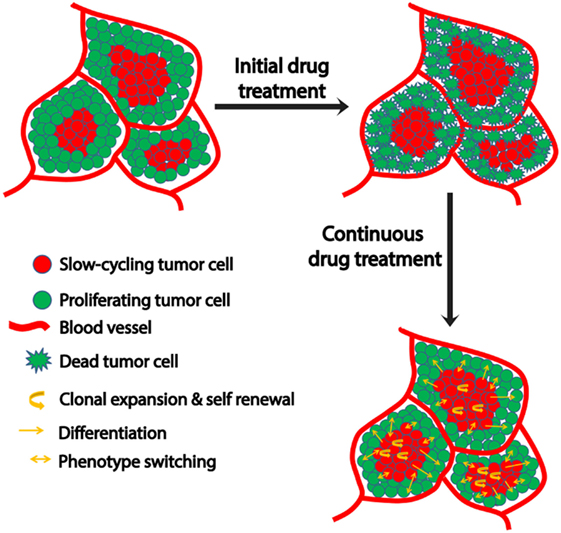
Figure 1. Schematic representation of microenvironment-driven dynamic heterogeneity and phenotypic plasticity as a mechanism of melanoma therapy resistance. Tumor cells close to the blood vessels proliferate, while those away from blood vessels experience hypoxia and nutrient starvation that contribute to their slow-cycling phenotype. While treatment readily targets proliferating cells, slow-cycling cells can evade drug action and survive. Upon continuous treatment, this slow-cycling subpopulation is enriched within the tumor by clonal expansion. Due to their inherent cancer stem cell-like property, they are capable of self-renewal or differentiation into a proliferative tumor cells with increased drug resistance. In addition to this, the slow-cycling cells can switch their phenotype to fast proliferating cells upon exposure to oxygen and nutrients after replacing the original peripheral fast proliferating cells that had been killed by the therapy. These phenotype-switched cells might be more drug resistant too, as they might have acquired resistance during their slow-cycling phase.
Taken together, slow-cycling cells play a pivotal role in developing therapy resistance and cancer progression. Thus, it is crucial to understand the underlying biology of the slow-cycling phenotype to improve the current therapy regimens in melanoma.
The Role of Microphthalmia-Associated Transcription Factor (MITF) in Melanoma Plasticity and Therapy Resistance
Microphthalmia-associated transcription factor is the master regulator of both normal melanocyte and melanoma biology (79, 80). In melanoma, MITF acts as a molecular switch that determines whether the cell will differentiate, proliferate, or become quiescent with increased migratory behavior (44, 81–84). The proliferative and invasive phenotypes of melanoma cells are defined by high and low levels of MITF, respectively, and melanoma cells are capable of switching between these two states, influenced by changing microenvironmental conditions (10, 45).
Depletion of MITF can reduce proliferation through G1-arrest (42, 68, 81, 85) with increased expression of cancer stem cell markers (68, 86). In response to hypoxia, MITF expression is downregulated (87). These properties are attributes of slow-cycling JARID1B-positive melanoma cells (20), supported by a negative correlation of MITF and JARID1B/SerpinE2 (77). Thus, in response to stress, e.g., hypoxia and/or nutrient starvation, melanoma cells switch from a proliferative MITFhigh to an invasive MITFlow slow-cycling phenotype. However, these subpopulations are not mutually exclusive, as within a tumor there can be MITFhigh and MITFlow cells, reflecting tumor heterogeneity as discussed above. In contrast to the proliferative MITFhigh phenotype, the invasive MITFlow phenotype is mainly governed by receptor tyrosine kinases (e.g., AXL, EGFR, and ERB3), WNT5A or NF-κB signaling, and the BRN2–NFIB–EZH2 axis (46, 88–90). Single cell expression analysis revealed that some MITFhigh cells also express the gene signature of the invasive MITFlow phenotype (91, 92). These and other studies indicate the presence of a third subpopulation in melanoma that expresses MITF, AXL, and WNT5A simultaneously (88, 93, 94). Consistent with this, we showed by using a 3D melanoma spheroid model that indeed melanoma cells can proliferate and invade simultaneously (12). In addition, another study has shown that invasive MITFlow and poorly invasive MITFhigh cells cooperate to invade into the surrounding matrix (95).
The role of MITF in drug resistance is controversial and the underlying mechanisms are yet to be understood. For instance, the presence of MITF is a marker for responsiveness to MAPKi treatment, but when MITF expression is upregulated, it can confer resistance to MAPKi (96). This might reflect the extreme end of the MITF rheostat model defined by differentiation, slow cycling (42), high PGC1α expression, and therapy resistance (20). Augmenting MITF levels in melanoma cells should switch the invasive slow-cycling phenotype to a proliferative phenotype. This would increase drug sensitivity because MAPKi predominantly act on rapidly proliferating cells. In addition, over-expression of MITF will inhibit the switching of proliferative cells to the invasive slow-cycling phenotype in response to stress by maintaining MITF levels constant. However, MITF is also reported as a driver of melanoma progression (97–99) and long-term MITF depletion induces senescence in melanoma cells and/or promotes apoptosis (81, 100, 101). Melanoma cells upregulate MITF expression to recover the loss of MAPK signaling upon exposure to MAPKi, enabling the cells to tolerate MAPKi (102). Downregulation of MITF increases the cytotoxic effects of MAPKi on melanoma cells and also reduces the acquisition of drug resistance (101, 103, 104). Upregulation of MITF has also been seen in several MAPKi acquired resistant cell lines (89). However, the same study reports that another population of resistant cell lines has lost MITF expression. MITF is downregulated in the acquired drug resistant phase and makes the cells more invasive (89). Thus, further investigation of these signaling pathways is required to determine in which combination these signaling pathways can be targeted along with the inhibition of MAPK signaling, to improve the outcomes of melanoma patients with disease relapse.
However, the situation appears to be even more complex, as in heterogeneous tumors MITFhigh and AXLhigh populations can co-exist (33, 102). Nevertheless, it has been shown that these subpopulations benefit from endothelin-1 in the presence of MAPKi, as inhibiting endothelin-1 signaling can effectively inhibit the growth of such heterogeneous tumors (105). More comprehensive studies are required to determine how MITF expression levels are altered in relation to the tumor’s response to MAPKi during ongoing treatment. Combination of MITF inhibitors with MAPKi should improve the efficacy of MAPKi in treating phases with high MITF expression. On the contrary, inhibitors of WNT5A/AXL/NF-κB in combination with MAPKi should improve the efficacy of MAPKi in treating phases with low MITF expression (Figure 2). Indeed, targeting AXL and BRAF/MEK simultaneously in a patient-derived xenograft model confers an increased survival advantage to the mice compared to monotherapy with either AXL or combination therapy with BRAF/MEK inhibitors (106).
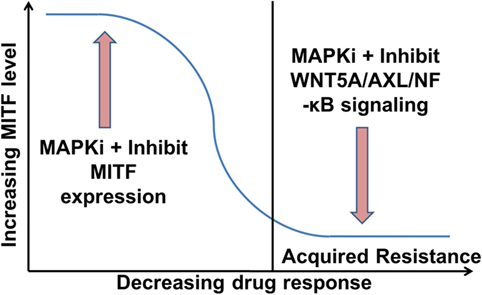
Figure 2. Proposed role of microphthalmia-associated transcription factor (MITF) and WNT5A/AXL/NF-κB signaling in melanoma therapy. MITFhigh melanomas could be treated initially with a combination of an MAPK pathway inhibitors (MAPKi) and an inhibitor of MITF expression. This should increase the efficacy of the MAPKi and delay the acquisition of drug resistance (104). Once in the resistance state with low MITF levels, the therapy could comprise a combination of a MAPKi and an inhibitor of WNT5A/AXL/NF-κB signaling.
Conclusion
Tumor microenvironment-driven dynamic heterogeneity is a major determinant of drug resistance in melanoma. This is mainly exerted by regulating the level of the master regulator MITF which is the major determinant of the dynamic phenotypic states in melanoma. A moderate MITF level determines the proliferative state of melanoma which is readily targetable with MAPKi. Both low and extremely high MITF levels give rise to two distinct slow-cycling states of melanoma (e.g., MITFlow/JARID1B-positive and MITFhigh/PGC1α-positive) with increased oxidative phosphorylation that results in treatment resistance. Thus, targeting this slow-cycling subpopulation by modulating MITF levels can be a potential strategy to overcome drug resistance in melanoma. However, MITF biology is highly complex and the downstream effects of MITF are extremely diverse (107). In addition, mechanisms that regulate MITF expression and activity are also numerous (80). Thus, modulation of MITF expression and activity can have diverse effects on melanoma cell biology. Considering the dynamic expression of MITF in response to changing microenvironmental conditions at various phases of melanomagenesis, MITF levels can be considered as a predictive marker for a suitable therapy regimen for treating a particular melanoma phase. We have developed an in vitro 3D melanoma spheroid model that mimics dynamic tumor heterogeneity to study the biology of microenvironment-driven tumor heterogeneity and plasticity and as these dynamic changes are difficult, time-consuming, and expensive to study in vivo.
Author Contributions
NH and FA wrote the manuscript together.
Conflict of Interest Statement
The authors declare that the research was conducted in the absence of any commercial or financial relationships that could be construed as a potential conflict of interest.
Funding
NH is a Cameron fellow of the Melanoma and Skin Cancer Research Institute, Australia, and currently funded by the National Health and Medical Research Council (APP1084893). FA holds a University of Queensland International Scholarship for Ph. D. in Biomedical Research.
References
1. Hodis E, Watson IR, Kryukov GV, Arold ST, Imielinski M, Theurillat JP, et al. A landscape of driver mutations in melanoma. Cell (2012) 150:251–63. doi:10.1016/j.cell.2012.06.024
2. The Cancer Genome Atlas Network. Genomic classification of cutaneous melanoma. Cell (2015) 161:1681–96. doi:10.1016/j.cell.2015.05.044
3. Carlino MS, Long GV. Ipilimumab combined with nivolumab: a standard of care for the treatment of advanced melanoma? Clin Cancer Res (2016) 22(16):3992–8. doi:10.1158/1078-0432.CCR-15-2944
4. Rizos H, Menzies AM, Pupo GM, Carlino MS, Fung C, Hyman J, et al. BRAF inhibitor resistance mechanisms in metastatic melanoma: spectrum and clinical impact. Clin Cancer Res (2014) 20:1965–77. doi:10.1158/1078-0432.CCR-13-3122
5. Shi H, Hugo W, Kong X, Hong A, Koya RC, Moriceau G, et al. Acquired resistance and clonal evolution in melanoma during BRAF inhibitor therapy. Cancer Discov (2014) 4:80–93. doi:10.1158/2159-8290.CD-13-0642
6. Van Allen EM, Wagle N, Sucker A, Treacy DJ, Johannessen CM, Goetz EM, et al. The genetic landscape of clinical resistance to RAF inhibition in metastatic melanoma. Cancer Discov (2014) 4:94–109. doi:10.1158/2159-8290.CD-13-0617
7. Welsh SJ, Rizos H, Scolyer RA, Long GV. Resistance to combination BRAF and MEK inhibition in metastatic melanoma: where to next? Eur J Cancer (2016) 62:76–85. doi:10.1016/j.ejca.2016.04.005
8. Zaretsky JM, Garcia-Diaz A, Shin DS, Escuin-Ordinas H, Hugo W, Hu-Lieskovan S, et al. Mutations associated with acquired resistance to PD-1 blockade in melanoma. N Engl J Med (2016) 375:819–29. doi:10.1056/NEJMoa1604958
9. Gide TN, Wilmott JS, Scolyer RA, Long GV. Primary and acquired resistance to immune checkpoint inhibitors in metastatic melanoma. Clin Cancer Res (2018) 24(6):1260–70. doi:10.1158/1078-0432.CCR-17-2267
10. Hoek KS, Eichhoff OM, Schlegel NC, Dobbeling U, Kobert N, Schaerer L, et al. In vivo switching of human melanoma cells between proliferative and invasive states. Cancer Res (2008) 68:650–6. doi:10.1158/0008-5472.CAN-07-2491
11. Hoek KS, Goding CR. Cancer stem cells versus phenotype-switching in melanoma. Pigment Cell Melanoma Res (2010) 23:746–59. doi:10.1111/j.1755-148X.2010.00757.x
12. Haass NK, Beaumont KA, Hill DS, Anfosso A, Mrass P, Munoz MA, et al. Real-time cell cycle imaging during melanoma growth, invasion, and drug response. Pigment Cell Melanoma Res (2014) 27:764–76. doi:10.1111/pcmr.12274
13. Haass NK. Dynamic tumor heterogeneity in melanoma therapy: how do we address this in a novel model system? Melanoma Manag (2015) 2:93–5. doi:10.2217/mmt.15.1
14. Ravindran Menon D, Das S, Krepler C, Vultur A, Rinner B, Schauer S, et al. A stress-induced early innate response causes multidrug tolerance in melanoma. Oncogene (2015) 34:4448–59. doi:10.1038/onc.2014.372
15. Beaumont KA, Hill DS, Daignault SM, Lui GY, Sharp DM, Gabrielli B, et al. Cell cycle phase-specific drug resistance as an escape mechanism of melanoma cells. J Invest Dermatol (2016) 136:1479–89. doi:10.1016/j.jid.2016.02.805
16. Kim IS, Heilmann S, Kansler ER, Zhang Y, Zimmer M, Ratnakumar K, et al. Microenvironment-derived factors driving metastatic plasticity in melanoma. Nat Commun (2017) 8:14343. doi:10.1038/ncomms14343
17. Hartsough E, Shao Y, Aplin AE. Resistance to RAF inhibitors revisited. J Invest Dermatol (2014) 134:319–25. doi:10.1038/jid.2013.358
18. Roesch A. Tumor heterogeneity and plasticity as elusive drivers for resistance to MAPK pathway inhibition in melanoma. Oncogene (2015) 34:2951–7. doi:10.1038/onc.2014.249
19. Tolk H, Satzger I, Mohr P, Zimmer L, Weide B, Schad S, et al. Complete remission of metastatic melanoma upon BRAF inhibitor treatment – what happens after discontinuation? Melanoma Res (2015) 25:362–6. doi:10.1097/CMR.0000000000000169
20. Ahn A, Chatterjee A, Eccles MR. The slow cycling phenotype: a growing problem for treatment resistance in melanoma. Mol Cancer Ther (2017) 16:1002–9. doi:10.1158/1535-7163.MCT-16-0535
21. Winder M, Viros A. Mechanisms of drug resistance in melanoma. Handb Exp Pharmacol (2017). doi:10.1007/164_2017_17
22. Manzano JL, Layos L, Buges C, De Los Llanos Gil M, Vila L, Martinez-Balibrea E, et al. Resistant mechanisms to BRAF inhibitors in melanoma. Ann Transl Med (2016) 4:237. doi:10.21037/atm.2016.06.07
23. Roesch A, Fukunaga-Kalabis M, Schmidt EC, Zabierowski SE, Brafford PA, Vultur A, et al. A temporarily distinct subpopulation of slow-cycling melanoma cells is required for continuous tumor growth. Cell (2010) 141:583–94. doi:10.1016/j.cell.2010.04.020
24. Tredan O, Galmarini CM, Patel K, Tannock IF. Drug resistance and the solid tumor microenvironment. J Natl Cancer Inst (2007) 99:1441–54. doi:10.1093/jnci/djm135
25. Gray-Schopfer VC, Karasarides M, Hayward R, Marais R. Tumor necrosis factor-alpha blocks apoptosis in melanoma cells when BRAF signaling is inhibited. Cancer Res (2007) 67:122–9. doi:10.1158/0008-5472.CAN-06-1880
26. Smith MP, Sanchez-Laorden B, O’brien K, Brunton H, Ferguson J, Young H, et al. The immune microenvironment confers resistance to MAPK pathway inhibitors through macrophage-derived TNFalpha. Cancer Discov (2014) 4:1214–29. doi:10.1158/2159-8290.CD-13-1007
27. Straussman R, Morikawa T, Shee K, Barzily-Rokni M, Qian ZR, Du J, et al. Tumour micro-environment elicits innate resistance to RAF inhibitors through HGF secretion. Nature (2012) 487:500–4. doi:10.1038/nature11183
28. Kaur A, Webster MR, Marchbank K, Behera R, Ndoye A, Kugel CH III, et al. sFRP2 in the aged microenvironment drives melanoma metastasis and therapy resistance. Nature (2016) 532:250–4. doi:10.1038/nature17392
29. Hirata E, Girotti MR, Viros A, Hooper S, Spencer-Dene B, Matsuda M, et al. Intravital imaging reveals how BRAF inhibition generates drug-tolerant microenvironments with high integrin beta1/FAK signaling. Cancer Cell (2015) 27:574–88. doi:10.1016/j.ccell.2015.03.008
30. Qin Y, Roszik J, Chattopadhyay C, Hashimoto Y, Liu C, Cooper ZA, et al. Hypoxia-driven mechanism of vemurafenib resistance in melanoma. Mol Cancer Ther (2016) 15:2442–54. doi:10.1158/1535-7163.MCT-15-0963
31. Liu S, Kumar SM, Martin JS, Yang R, Xu X. Snail1 mediates hypoxia-induced melanoma progression. Am J Pathol (2011) 179:3020–31. doi:10.1016/j.ajpath.2011.08.038
32. Fisher R, Pusztai L, Swanton C. Cancer heterogeneity: implications for targeted therapeutics. Br J Cancer (2013) 108:479–85. doi:10.1038/bjc.2012.581
33. Tirosh I, Izar B, Prakadan SM, Wadsworth MH II, Treacy D, Trombetta JJ, et al. Dissecting the multicellular ecosystem of metastatic melanoma by single-cell RNA-seq. Science (2016) 352:189–96. doi:10.1126/science.aad0501
34. Grzywa TM, Paskal W, Wlodarski PK. Intratumor and intertumor heterogeneity in melanoma. Transl Oncol (2017) 10:956–75. doi:10.1016/j.tranon.2017.09.007
35. Andor N, Graham TA, Jansen M, Xia LC, Aktipis CA, Petritsch C, et al. Pan-cancer analysis of the extent and consequences of intratumor heterogeneity. Nat Med (2016) 22:105–13. doi:10.1038/nm.3984
36. Shannan B, Perego M, Somasundaram R, Herlyn M. Heterogeneity in melanoma. Cancer Treat Res (2016) 167:1–15. doi:10.1007/978-3-319-22539-5_1
37. Shackleton M. Moving targets that drive cancer progression. N Engl J Med (2010) 363:885–6. doi:10.1056/NEJMcibr1006328
38. Nowell PC. The clonal evolution of tumor cell populations. Science (1976) 194:23–8. doi:10.1126/science.959840
39. Reya T, Morrison SJ, Clarke MF, Weissman IL. Stem cells, cancer, and cancer stem cells. Nature (2001) 414:105–11. doi:10.1038/35102167
40. Toh TB, Lim JJ, Chow EK. Epigenetics in cancer stem cells. Mol Cancer (2017) 16:29. doi:10.1186/s12943-017-0596-9
41. Marusyk A, Polyak K. Tumor heterogeneity: causes and consequences. Biochim Biophys Acta (2010) 1805:105–17. doi:10.1016/j.bbcan.2009.11.002
42. Carreira S, Goodall J, Denat L, Rodriguez M, Nuciforo P, Hoek KS, et al. MITF regulation of dia1 controls melanoma proliferation and invasiveness. Genes Dev (2006) 20:3426–39. doi:10.1101/gad.406406
43. Hill RP. Identifying cancer stem cells in solid tumors: case not proven. Cancer Res (2006) 66:1891–5; discussion 1890. doi:10.1158/0008-5472.CAN-05-3450
44. Hoek KS, Schlegel NC, Eichhoff OM, Widmer DS, Praetorius C, Einarsson SO, et al. Novel MITF targets identified using a two-step DNA microarray strategy. Pigment Cell Melanoma Res (2008) 21:665–76. doi:10.1111/j.1755-148X.2008.00505.x
45. Hoek KS, Schlegel NC, Brafford P, Sucker A, Ugurel S, Kumar R, et al. Metastatic potential of melanomas defined by specific gene expression profiles with no BRAF signature. Pigment Cell Res (2006) 19:290–302. doi:10.1111/j.1600-0749.2006.00322.x
46. O’Connell MP, Marchbank K, Webster MR, Valiga AA, Kaur A, Vultur A, et al. Hypoxia induces phenotypic plasticity and therapy resistance in melanoma via the tyrosine kinase receptors ROR1 and ROR2. Cancer Discov (2013) 3:1378–93. doi:10.1158/2159-8290.CD-13-0005
47. Brandner JM, Haass NK. Melanoma’s connections to the tumour microenvironment. Pathology (2013) 45:443–52. doi:10.1097/PAT.0b013e328363b3bd
48. Mintz B, Illmensee K. Normal genetically mosaic mice produced from malignant teratocarcinoma cells. Proc Natl Acad Sci U S A (1975) 72:3585–9. doi:10.1073/pnas.72.9.3585
49. Park CC, Bissell MJ, Barcellos-Hoff MH. The influence of the microenvironment on the malignant phenotype. Mol Med Today (2000) 6:324–9. doi:10.1016/S1357-4310(00)01756-1
50. Falletta P, Sanchez-Del-Campo L, Chauhan J, Effern M, Kenyon A, Kershaw CJ, et al. Translation reprogramming is an evolutionarily conserved driver of phenotypic plasticity and therapeutic resistance in melanoma. Genes Dev (2017) 31:18–33. doi:10.1101/gad.290940.116
51. Hamid O, Schmidt H, Nissan A, Ridolfi L, Aamdal S, Hansson J, et al. A prospective phase II trial exploring the association between tumor microenvironment biomarkers and clinical activity of ipilimumab in advanced melanoma. J Transl Med (2011) 9:204. doi:10.1186/1479-5876-9-204
52. Ji RR, Chasalow SD, Wang L, Hamid O, Schmidt H, Cogswell J, et al. An immune-active tumor microenvironment favors clinical response to ipilimumab. Cancer Immunol Immunother (2012) 61:1019–31. doi:10.1007/s00262-011-1172-6
53. Callahan MK. Immune checkpoint therapy in melanoma. Cancer J (2016) 22:73–80. doi:10.1097/PPO.0000000000000183
54. Santiago-Walker A, Li L, Haass NK, Herlyn M. Melanocytes: from morphology to application. Skin Pharmacol Physiol (2009) 22:114–21. doi:10.1159/000178870
55. Beaumont KA, Mohana-Kumaran N, Haass NK. Modeling melanoma in vitro and in vivo. Healthcare (Basel) (2013) 2:27–46. doi:10.3390/healthcare2010027
56. Sakaue-Sawano A, Kurokawa H, Morimura T, Hanyu A, Hama H, Osawa H, et al. Visualizing spatiotemporal dynamics of multicellular cell-cycle progression. Cell (2008) 132:487–98. doi:10.1016/j.cell.2007.12.033
57. Beaumont KA, Anfosso A, Ahmed F, Weninger W, Haass NK. Imaging- and flow cytometry-based analysis of cell position and the cell cycle in 3D melanoma spheroids. J Vis Exp (2015) 106:e53486. doi:10.3791/53486
58. Spoerri L, Beaumont KA, Anfosso A, Haass NK. Real-time cell cycle imaging in a 3D cell culture model of melanoma. Methods Mol Biol (2017) 1612:401–16. doi:10.1007/978-1-4939-7021-6_29
59. Kienzle A, Kurch S, Schloder J, Berges C, Ose R, Schupp J, et al. Dendritic mesoporous silica nanoparticles for pH-stimuli-responsive drug delivery of TNF-alpha. Adv Healthc Mater (2017) 6:1700012. doi:10.1002/adhm.201700012
60. Simpson MJ, Jin W, Vittadello ST, Tambyah T, Ryan J, Gunasingh G, et al. Stochastic models of cell invasion with fluorescent cell cycle indicators. BioRxiv (2018). doi:10.1101/273995
61. Vittadello ST, Mccue SW, Gunasingh G, Haass NK, Simpson MJ. Mathematical models for cell migration with real-time cell cycle dynamics. Biophys J (2018) 114:1241–53. doi:10.1016/j.bpj.2017.12.041
62. Minchinton AI, Tannock IF. Drug penetration in solid tumours. Nat Rev Cancer (2006) 6:583–92. doi:10.1038/nrc1893
63. Haass NK, Schumacher U. Melanoma never says die. Exp Dermatol (2014) 23:471–2. doi:10.1111/exd.12400
64. Haass NK, Gabrielli B. Cell cycle-tailored targeting of metastatic melanoma: challenges and opportunities. Exp Dermatol (2017) 26:649–55. doi:10.1111/exd.13303
65. Mitchison TJ. The proliferation rate paradox in antimitotic chemotherapy. Mol Biol Cell (2012) 23:1–6. doi:10.1091/mbc.E10-04-0335
66. Hanahan D, Weinberg RA. The hallmarks of cancer. Cell (2000) 100:57–70. doi:10.1016/S0092-8674(00)81683-9
67. Hanahan D, Weinberg RA. Hallmarks of cancer: the next generation. Cell (2011) 144:646–74. doi:10.1016/j.cell.2011.02.013
68. Cheli Y, Giuliano S, Botton T, Rocchi S, Hofman V, Hofman P, et al. MITF is the key molecular switch between mouse or human melanoma initiating cells and their differentiated progeny. Oncogene (2011) 30:2307–18. doi:10.1038/onc.2010.598
69. Roesch A, Vultur A, Bogeski I, Wang H, Zimmermann KM, Speicher D, et al. Overcoming intrinsic multidrug resistance in melanoma by blocking the mitochondrial respiratory chain of slow-cycling JARID1B(high) cells. Cancer Cell (2013) 23:811–25. doi:10.1016/j.ccr.2013.05.003
70. Sun C, Wang L, Huang S, Heynen GJ, Prahallad A, Robert C, et al. Reversible and adaptive resistance to BRAF(V600E) inhibition in melanoma. Nature (2014) 508:118–22. doi:10.1038/nature13121
71. Vazquez F, Lim JH, Chim H, Bhalla K, Girnun G, Pierce K, et al. PGC1alpha expression defines a subset of human melanoma tumors with increased mitochondrial capacity and resistance to oxidative stress. Cancer Cell (2013) 23:287–301. doi:10.1016/j.ccr.2012.11.020
72. Corazao-Rozas P, Guerreschi P, Jendoubi M, Andre F, Jonneaux A, Scalbert C, et al. Mitochondrial oxidative stress is the Achille’s heel of melanoma cells resistant to Braf-mutant inhibitor. Oncotarget (2013) 4:1986–98. doi:10.18632/oncotarget.1420
73. Haq R, Shoag J, Andreu-Perez P, Yokoyama S, Edelman H, Rowe GC, et al. Oncogenic BRAF regulates oxidative metabolism via PGC1alpha and MITF. Cancer Cell (2013) 23:302–15. doi:10.1016/j.ccr.2013.02.003
74. Viale A, Corti D, Draetta GF. Tumors and mitochondrial respiration: a neglected connection. Cancer Res (2015) 75:3685–6. doi:10.1158/0008-5472.CAN-15-0491
75. Moore N, Houghton J, Lyle S. Slow-cycling therapy-resistant cancer cells. Stem Cells Dev (2012) 21:1822–30. doi:10.1089/scd.2011.0477
76. Haferkamp S, Borst A, Adam C, Becker TM, Motschenbacher S, Windhovel S, et al. Vemurafenib induces senescence features in melanoma cells. J Invest Dermatol (2013) 133:1601–9. doi:10.1038/jid.2013.6
77. Perego M, Maurer M, Wang JX, Shaffer S, Muller AC, Parapatics K, et al. A slow-cycling subpopulation of melanoma cells with highly invasive properties. Oncogene (2018) 37:302–12. doi:10.1038/onc.2017.341
78. Moore N, Lyle S. Quiescent, slow-cycling stem cell populations in cancer: a review of the evidence and discussion of significance. J Oncol (2011) 2011:396076. doi:10.1155/2011/396076
79. Levy C, Khaled M, Fisher DE. MITF: master regulator of melanocyte development and melanoma oncogene. Trends Mol Med (2006) 12:406–14. doi:10.1016/j.molmed.2006.07.008
80. Hartman ML, Czyz M. MITF in melanoma: mechanisms behind its expression and activity. Cell Mol Life Sci (2015) 72:1249–60. doi:10.1007/s00018-014-1791-0
81. Giuliano S, Cheli Y, Ohanna M, Bonet C, Beuret L, Bille K, et al. Microphthalmia-associated transcription factor controls the DNA damage response and a lineage-specific senescence program in melanomas. Cancer Res (2010) 70:3813–22. doi:10.1158/0008-5472.CAN-09-2913
82. Goding CR. Commentary. A picture of MITF in melanoma immortality. Oncogene (2011) 30:2304–6. doi:10.1038/onc.2010.641
83. Yajima I, Kumasaka MY, Thang ND, Goto Y, Takeda K, Iida M, et al. Molecular network associated with MITF in skin melanoma development and progression. J Skin Cancer (2011) 2011:730170. doi:10.1155/2011/730170
84. Widmer DS, Cheng PF, Eichhoff OM, Belloni BC, Zipser MC, Schlegel NC, et al. Systematic classification of melanoma cells by phenotype-specific gene expression mapping. Pigment Cell Melanoma Res (2012) 25:343–53. doi:10.1111/j.1755-148X.2012.00986.x
85. Du J, Widlund HR, Horstmann MA, Ramaswamy S, Ross K, Huber WE, et al. Critical role of CDK2 for melanoma growth linked to its melanocyte-specific transcriptional regulation by MITF. Cancer Cell (2004) 6:565–76. doi:10.1016/j.ccr.2004.10.014
86. He S, Li CG, Slobbe L, Glover A, Marshall E, Baguley BC, et al. PAX3 knockdown in metastatic melanoma cell lines does not reduce MITF expression. Melanoma Res (2011) 21:24–34. doi:10.1097/CMR.0b013e328341c7e0
87. Feige E, Yokoyama S, Levy C, Khaled M, Igras V, Lin RJ, et al. Hypoxia-induced transcriptional repression of the melanoma-associated oncogene MITF. Proc Natl Acad Sci U S A (2011) 108:E924–33. doi:10.1073/pnas.1106351108
88. Konieczkowski DJ, Johannessen CM, Abudayyeh O, Kim JW, Cooper ZA, Piris A, et al. A melanoma cell state distinction influences sensitivity to MAPK pathway inhibitors. Cancer Discov (2014) 4:816–27. doi:10.1158/2159-8290.CD-13-0424
89. Muller J, Krijgsman O, Tsoi J, Robert L, Hugo W, Song C, et al. Low MITF/AXL ratio predicts early resistance to multiple targeted drugs in melanoma. Nat Commun (2014) 5:5712. doi:10.1038/ncomms6712
90. Fane ME, Chhabra Y, Hollingsworth DE, Simmons JL, Spoerri L, Oh TG, et al. NFIB mediates BRN2 driven melanoma cell migration and invasion through regulation of EZH2 and MITF. EBioMedicine (2017) 16:63–75. doi:10.1016/j.ebiom.2017.01.013
91. Ennen M, Keime C, Kobi D, Mengus G, Lipsker D, Thibault-Carpentier C, et al. Single-cell gene expression signatures reveal melanoma cell heterogeneity. Oncogene (2015) 34:3251–63. doi:10.1038/onc.2014.262
92. Ennen M, Keime C, Gambi G, Kieny A, Coassolo S, Thibault-Carpentier C, et al. MITF-High and MITF-low cells and a novel subpopulation expressing genes of both cell states contribute to intra- and intertumoral heterogeneity of primary melanoma. Clin Cancer Res (2017) 23:7097–107. doi:10.1158/1078-0432.CCR-17-0010
93. Dissanayake SK, Olkhanud PB, O’connell MP, Carter A, French AD, Camilli TC, et al. Wnt5A regulates expression of tumor-associated antigens in melanoma via changes in signal transducers and activators of transcription 3 phosphorylation. Cancer Res (2008) 68:10205–14. doi:10.1158/0008-5472.CAN-08-2149
94. Wellbrock C, Arozarena I. Microphthalmia-associated transcription factor in melanoma development and MAP-kinase pathway targeted therapy. Pigment Cell Melanoma Res (2015) 28:390–406. doi:10.1111/pcmr.12370
95. Chapman A, Fernandez Del Ama L, Ferguson J, Kamarashev J, Wellbrock C, Hurlstone A. Heterogeneous tumor subpopulations cooperate to drive invasion. Cell Rep (2014) 8:688–95. doi:10.1016/j.celrep.2014.06.045
96. Arozarena I, Wellbrock C. Overcoming resistance to BRAF inhibitors. Ann Transl Med (2017) 5:387. doi:10.21037/atm.2017.06.09
97. Garraway LA, Widlund HR, Rubin MA, Getz G, Berger AJ, Ramaswamy S, et al. Integrative genomic analyses identify MITF as a lineage survival oncogene amplified in malignant melanoma. Nature (2005) 436:117–22. doi:10.1038/nature03664
98. Regad T. Molecular and cellular pathogenesis of melanoma initiation and progression. Cell Mol Life Sci (2013) 70:4055–65. doi:10.1007/s00018-013-1324-2
99. Kumar SM, Dai J, Li S, Yang R, Yu H, Nathanson KL, et al. Human skin neural crest progenitor cells are susceptible to BRAF(V600E)-induced transformation. Oncogene (2014) 33:832–41. doi:10.1038/onc.2012.642
100. McGill GG, Haq R, Nishimura EK, Fisher DE. c-Met expression is regulated by MITF in the melanocyte lineage. J Biol Chem (2006) 281:10365–73. doi:10.1074/jbc.M513094200
101. Wellbrock C, Rana S, Paterson H, Pickersgill H, Brummelkamp T, Marais R. Oncogenic BRAF regulates melanoma proliferation through the lineage specific factor MITF. PLoS One (2008) 3:e2734. doi:10.1371/journal.pone.0002734
102. Smith MP, Brunton H, Rowling EJ, Ferguson J, Arozarena I, Miskolczi Z, et al. Inhibiting drivers of non-mutational drug tolerance is a salvage strategy for targeted melanoma therapy. Cancer Cell (2016) 29:270–84. doi:10.1016/j.ccell.2016.02.003
103. Smith MP, Ferguson J, Arozarena I, Hayward R, Marais R, Chapman A, et al. Effect of SMURF2 targeting on susceptibility to MEK inhibitors in melanoma. J Natl Cancer Inst (2013) 105:33–46. doi:10.1093/jnci/djs471
104. Arozarena I, Smith MP, Wellbrock C. Targeting MITF in the tolerance-phase. Oncotarget (2016) 7:54094–5. doi:10.18632/oncotarget.9423
105. Smith MP, Rowling EJ, Miskolczi Z, Ferguson J, Spoerri L, Haass NK, et al. Targeting endothelin receptor signalling overcomes heterogeneity driven therapy failure. EMBO Mol Med (2017) 9:1011–29. doi:10.15252/emmm.201607156
106. Boshuizen J, Koopman LA, Krijgsman O, Shahrabi A, Van Den Heuvel EG, Ligtenberg MA, et al. Cooperative targeting of melanoma heterogeneity with an AXL antibody-drug conjugate and BRAF/MEK inhibitors. Nat Med (2018) 24:203–12. doi:10.1038/nm.4472
Keywords: melanoma, tumor heterogeneity, clonality, cancer drug resistance, tumor microenvironment, microphthalmia-associated transcription factor, tumor plasticity, slow-cycling tumor cells
Citation: Ahmed F and Haass NK (2018) Microenvironment-Driven Dynamic Heterogeneity and Phenotypic Plasticity as a Mechanism of Melanoma Therapy Resistance. Front. Oncol. 8:173. doi: 10.3389/fonc.2018.00173
Received: 02 March 2018; Accepted: 03 May 2018;
Published: 24 May 2018
Edited by:
Petranel Theresa Ferrao, University of Melbourne, AustraliaReviewed by:
Patricia A. Possik, Instituto Nacional de Câncer (INCA), BrazilRodabe N. Amaria, University of Texas MD Anderson Cancer Center, United States
Copyright: © 2018 Ahmed and Haass. This is an open-access article distributed under the terms of the Creative Commons Attribution License (CC BY). The use, distribution or reproduction in other forums is permitted, provided the original author(s) and the copyright owner are credited and that the original publication in this journal is cited, in accordance with accepted academic practice. No use, distribution or reproduction is permitted which does not comply with these terms.
*Correspondence: Nikolas K. Haass, n.haass1@uq.edu.au