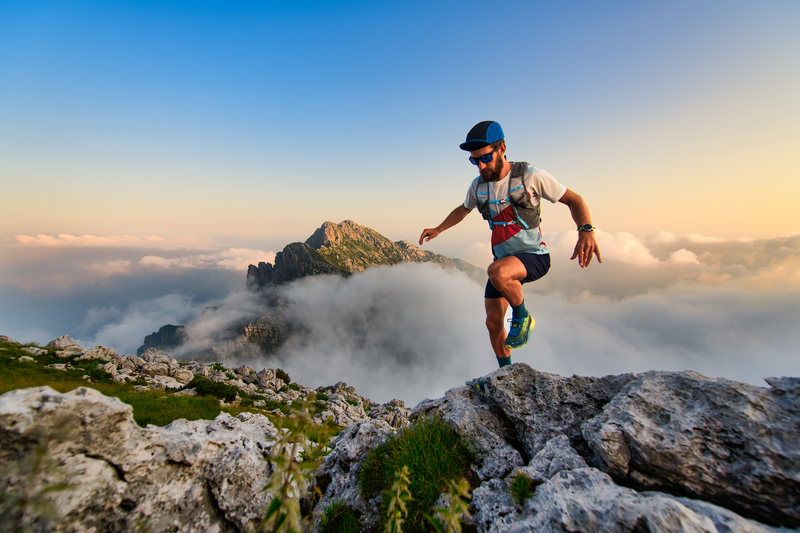
94% of researchers rate our articles as excellent or good
Learn more about the work of our research integrity team to safeguard the quality of each article we publish.
Find out more
REVIEW article
Front. Oncol. , 31 January 2017
Sec. Cancer Imaging and Image-directed Interventions
Volume 7 - 2017 | https://doi.org/10.3389/fonc.2017.00003
This article is part of the Research Topic Exploring cancer metabolic reprogramming through molecular imaging View all 23 articles
Tumors are often characterized by hypoxia, vascular abnormalities, low extracellular pH, increased interstitial fluid pressure, altered choline-phospholipid metabolism, and aerobic glycolysis (Warburg effect). The impact of these tumor characteristics has been investigated extensively in the context of tumor development, progression, and treatment response, resulting in a number of non-invasive imaging biomarkers. More recent evidence suggests that cancer cells undergo metabolic reprograming, beyond aerobic glycolysis, in the course of tumor development and progression. The resulting altered metabolic content in tumors has the ability to affect cell signaling and block cellular differentiation. Additional emerging evidence reveals that the interaction between tumor and stroma cells can alter tumor metabolism (leading to metabolic reprograming) as well as tumor growth and vascular features. This review will summarize previous and current preclinical, non-invasive, multimodal imaging efforts to characterize the tumor microenvironment, including its stromal components and understand tumor–stroma interaction in cancer development, progression, and treatment response.
The TME (Figure 1A), composed of tumor cells and stroma, is often characterized by hypoxia, vascular abnormalities, low extracellular pH (pHe), increased interstitial fluid pressure (1–7), increased aerobic glycolysis (Warburg effect) (8, 9), glutamine addiction (10–13), and altered choline-phospholipid metabolism (14–19). Recent evidence suggests that metabolic reprograming in the course of tumor development and progression increases in more aggressive cancer cells/tumors the ability to easily adapt metabolism to the most advantageous pathways, beyond the Warburg effect, in order to ensure their growth and survival in response to varying environmental stimuli, such as hypoxia or limited nutrient supply (20–24). Altered metabolic content in tumors may affect cell signaling and degree of cellular differentiation (11, 25–27).
Figure 1. The tumor microenvironment (TME). (A) Components and in vivo imaging of the TME. Immune cells include tumor-associated macrophages, antigen-presenting cells, myeloid-derived suppressor cells, and lymphocytes; CAFs, cancer-associated fibroblasts; MSCs, mesenchymal stem cells; ECM, extracellular matrix, consisting of collagens, laminins, and other matrix proteins, which is remodeled by ECM-degrading proteases; endothelial cells, pericytes, and vascular ECM compose the tumor blood and lymph vasculature. (B) Preclinical in vivo imaging of the TME. MRI, magnetic resonance imaging; PET, positron emission tomography; SPECT, single photon emission computer tomography; CT, computer tomography; US, ultrasound.
While previous research focused extensively on the tumor cells, over the last two decades or so, further evidence emerged that the tumor stroma is altered during tumor development/progression and that the tumor–stroma interaction plays an essential role in tumor metabolism (Figure 2), development, progression, and treatment response (2, 22, 23, 26, 28–37).
Figure 2. Models of cancer cell–cancer-associated fibroblast (CAF) metabolic cooperation in the tumor microenvironment, promoting survival, growth, and metastases (38, 39).
The stroma in solid tumors consists of extracellular matrix (ECM), and stromal cells, including fibroblasts, endothelial cells, pericytes, and various immune cells, such as macrophages, neutrophils, mast cells, myeloid progenitors, and lymphocytes (Figure 1A), with cancer cells playing an active role in the recruitment and metabolic reprograming of stromal cells (Figure 2) (22, 26, 40) and the dynamic remodeling of ECM by tumor and stromal cells promoting tumor progression (41–44).
Multiple preclinical imaging techniques (Table 1; Figure 1B) have been developed to visualize and quantify specific characteristics of the TME (5, 45, 46). This review summarizes the efforts to image and characterize non-invasively the TME (Figure 1), including its stromal components, and tumor–stroma interaction (Figures 2–7) in preclinical cancer. Stromal components and their imaging are described in the context of preclinical cancer in Section “The Tumor Stroma and Its Imaging.” Section “Non-invasive Multimodal Imaging of Tumor–Stroma Interaction” focuses on the more recent attempts to assess the interaction of stromal components with cancer cells by non- or minimally invasive preclinical multimodal imaging.
Table 1. Summary of modalities for in vivo imaging of the tumor microenvironment in preclinical (small animal) tumor models.
Figure 3. Intravital microscopy of the tumor microenvironment. (A) Epifluorescence microscopy was used to monitor and quantify tumor growth in a human fibrosarcoma xenograft model. The invasion of tumor into the surrounding tissue during growth can be visualized (white arrowheads). Bar 50 µm. Adapted with permission from Ref. (66). (B) Tumor morphology, vascularization, proliferation, and apoptosis in a human fibrosarcoma xenograft, as detected by intravital microscopy: tumor cells express cytoplasmic DsRed2 and nuclear histone 2B (H2B)-EGFP. Collagen fibers are detected by second-harmonic generation. Non-disrupted vessels are detected from the fluorescence signal of i.v.-administered Alexa660-Dextran. Bar 50 µm. Nuclear morphology including mitotic (white arrowheads) and apoptotic figures (black arrowhead) can be derived and quantified from imaging H2B-EGFP and DsRed2. Insets show prophase (P), metaphase (M), late anaphase (LA), and apoptotis (A). Bar 50 µm. Adapted with permission from Ref. (66).
Figure 4. Different motility and invasion of low-metastatic, GFP-expressing (green) and high-metastatic, CFP-expressing (white) mammary tumor cells within the collagen network (purple) was imaged by in vivo intravital microscopy with FL and second-harmonic generation. Bars 25 µm. (A) Time series demonstrating the migration of GFP-expressing (arrow head) and CFP-expressing (arrow) tumor cells along collagen fibers. (B) Metastatic growth of color-coded cells in the lung. (C) Protruding filopod (arrow, left) and lamellapod (arrow, right) of CFP-expressing cell near GFP-expressing cells. (D) Overall, the high-metastatic cells (outlined in white) move more frequently (see orange arrow path) than the low-metastatic cells (green). Adapted with permission from Ref. (67).
Figure 5. Intravital microscopy of the tumor blood vessels, lymph vasculature, and vascular response to treatment. (A) Top: Z-stack of lymphatics detected by near-infrared fluorescence (NIRF) multiphoton microscopy of FITC-tagged LyP-1 peptide (green), collagen fibers detected by second-harmonic generation, and tumor cells imaged by epifluorescence of cytoplasmic DsRed2 (red) and nuclear histone 2B (H2B)-EGFP (green) shows lymph vessels at the tumor margin. Bottom: intralymphatic (white arrowheads) and perilymphatic (black arrowheads) invasion of fibrosarcoma cells expressing cytoplasmic DsRed2 (red) and H2B-EGFP (green). Bars 100 µm. Adapted with permission from Ref. (66). (B) In vivo optical frequency domain imaging of blood [depth denoted from red (up to 2 mm deep) to yellow (superficial)] and lymph (blue) vessels in control and DC101-treated tumors, depicting the antivascular effect of VEGFR-2 inhibition. Adapted with permission from Ref. (68).
Figure 6. In vivo immune cell imaging. (A,B) Specificity of in vivo imaging of immune cells in 4T1 mammary breast tumors by fluorescence-reflectance imaging with a Cy5.5-labeled polyclonal antibody against murine S100A9 (aS100A9-C5.5). (C) Fluorescence molecular tomography of coinjected rabIgG-Cy7 and aS100A9-C5.5 demonstrates homogeneous perfusion (left) and immune cell distribution (right), respectively. (D) Ex vivo validation shoe S100A+ cells in the tumor periphery corresponding to F4/80+ TAMs. Adapted with permission from Ref. (69) © by the Society of Nuclear Medicine and Molecular Imaging, Inc.
Figure 7. In a carcinoembryonic antigen (CEA)-expressing colorectal adenocarcinoma model, simultaneous magnetic resonance imaging (MRI)/positron emission tomography (PET) at 4 h and 20 h after the injection of a radiolabeled antibody against CEA (64Cu-DOTA-NHS-M5A) demonstrates its accumulation in the tumor over time, while the apparent diffusion coefficient across the tumor remained largely unchanged. Adapted with permission from Ref. (70) © by the Society of Nuclear Medicine and Molecular Imaging, Inc.
In this chapter, we describe briefly the stromal components and their imaging with its strengths and limitations.
The ECM, a complex structure composed of laminins, collagens, proteoglycans, fibronectin, elastin, etc. (71), changes its composition during cancer progression (41, 72, 73). Many of its components are regulated by matrix metalloproteinases (MMPs) which are involved in growth signaling [by proteolytic activation of the transforming growth factor-β (TGF-β) pathway], apoptosis, and angiogenesis (73–76).
Available imaging methods focus on targeting the ECM component itself or the enzymes that degrade it, typically, by using activatable imaging probes (Figure 1B; Table 1). Imaging of cell–matrix adhesion can elucidate the dynamic interplay of cells and surrounding tissue during ECM remodeling, immune cell recruitment, wound healing, and cancer metastasis (77).
Methods, such as colorimetry (78), weight measurements (79), atomic force microscopy (80–82), and immunostaining (83–85), to image collagen structures risk their destruction and are limited by their in vivo translatability. The dorsal skinfold (window) chamber setup allows optical measurements by replacing skin with glass but may lead to collagen structural changes due to inflammation and mechanotransduction by the glass (86). The advances in ultrafast optics significantly improved the ability to image fibrillar collagen (the predominant structural protein in mammalian ECM and mostly type I) by second-harmonic generation (SHG) or third-harmonic generation (61) microscopy in vivo and ex vivo (87–91). The strength of SHG imaging is its specificity to fibrillar collagen (62, 87, 89, 92) and that it can be fairly easily combined with other optical imaging methods, in vivo (Figures 3–5A) and ex vivo (49, 90, 93–95). Ability for clinical translation has been demonstrated in breast cancer patients by combining SHG and bright-field high-resolution microscopy with large field of view to design a semi-automated technique to predict survival based on collagen fiber classifications (93). Recently, confocal microscopy has been used in vivo to detect collagen turnover after introduction of fluorescent fibrillar collagen into the dermis of live mice (96). However, all optical imaging methods suffer from their limited imaging depth, rendering them often an invasive tool and limiting their clinical translation (49, 57). Thus, the diagnosis and treatment of pathologies related to collagen remodeling has benefited greatly from the development of collagen-binding or hybridizing peptides, bearing an imaging contrast agent (CA) for, e.g., magnetic resonance imaging (MRI) or fluorescence imaging, or theranostic agents, to image triple-helical, intact, and/or unfolded, denatured collagen and treatment response (97). Other imaging modalities [e.g., ultrasound (US) (98, 99), optical coherence tomography (OCT) (100, 101), Fourier transform infrared spectroscopic imaging (53), or multispectral photoacoustic imaging (PAI) (102)], and various collagen-targeted agents, e.g., quantum dots (84, 85, 103, 104) or collagen-mimetic peptide-based imaging agents (105, 106) are being developed/applied to improve collagen imaging and to measure collagen turnover during tissue remodeling.
The key role that various MMPs play during cancer initiation and progression, with clear links to tumor invasion and metastasis (107), make them desirable treatment and cancer imaging targets (108). Targeted probes to image MMPs in vivo by optical imaging (fluorescence and bioluminescence), positron emission tomography (PET), single photon emission computer tomography (SPECT), and MRI have been developed (108–110), with a “broad-spectrum” MMP-activatable fluorescence probe available commercially (MMPSense, PerkinElmer, Akron, OH, USA). Each modality and imaging probe displays strengths and weaknesses in effectively imaging MMP activity (108, 110). However, optical imaging has limited penetration depth (108) and, while tomography is possible, anatomical information is lacking. Targeted, inhibitor-based PET and SPECT probes harness the excellent sensitivity of radioactive tracers and may be theranostic, but their synthesis may be difficult and, so far, it has not been possible to quantify proteolytic activity in vivo due to non-specific binding (108, 109, 111). Recently, an 18F-labeled MMP-activatable PET probe has been developed to overcome the lack of specificity of inhibitor-based probes (112). Photoacoustic tomography (PAT or PAI) (58, 113, 114) combines ultrasonic resolution with electromagnetic-enhanced contrast to obtain quantitative information on tissue structure, blood flow, and perfusion, and, through targeted probes (59), on receptor status or enzyme activity. For example, a photoacoustic probe activated by MMP-2 and MMP-9 demonstrated sensing of MMP-2/-9 activity in a follicular thyroid carcinoma model (115). With an imaging depth of >30 mm, depending on setup and desired spatial resolution (58), PAT expands on the tissue penetration of up to 20 mm typical for optical imaging (51, 108) and is thus suitable to monitor non-invasively tumor characteristics in orthotopic preclinical cancer models. The lack of anatomical/morphological information inherent to optical imaging, PAT/PAI, and PET/SPECT can be overcome by multimodal imaging. Using fluorescence molecular tomography (FMT) (51) coregistered with MRI, Salaun et al. (116) found increasing MMP-13 levels as lung tumors progressed. In skin squamous cell carcinoma xenografts, MMP-2, -3, -7, -9, -12, and -13 activities correlated with degree of angiogenesis and tumor invasion, as imaged by FMT combined with μCT (117). PAT/PAI, combined with US and OCT, can provide non-invasively morphological and functional tumor characteristics (60). Molecular MRI to measure MMP activity using protease-modulated CAs is still emerging and is hampered by its insensitivity, requiring long acquisition times but is potentially quantitative, and anatomical information can be obtained in the same setting (108, 109). Of note is that the interpretation of MMP images obtained with the typically broad-spectrum probes (110) is further complicated by the function of MMPs in biological processes beyond ECM remodeling (111).
Another major ECM constitutent, the proteoglycan hyaluronan [hyaluronic acid (HA), hyaluronate] is a high molecular weight glycosaminoglycan with a significant role in tumor growth and metastasis (118, 119), acting as tumor suppressor or promoter depending on its molecular weight (120).
It is degraded by hyaluronidases (Hyals), with hyaluronidase-1, -2 (Hyal1, Hyal2) currently being the most studied in cancer (119). Hyal1 overexpression has been associated with more aggressive tumors in a variety of epithelial cancers (e.g., bladder, colorectal, breast, and ovary), while Hyal2 may function as a tumor suppressor or promoter (119). The development of various HA probes to image HA turnover and clearance and of theranostic HA probes where encapsulated drugs are released in response to Hyal activity (119, 121, 122) has expanded greatly in recent years, and a detailed review is beyond the scope of this paper. HA probes often exploit the high specificity of HA for the CD44 receptor, a transmembrane receptor overexpressed in many tumor cell types (120, 121, 123–134). Single moiety, HA-based CAs have been used to image Hyals activity by MRI (135) and NIRF (124). Fluorescence correlation spectroscopy and Forster resonance energy transfer of HA-conjugated probes have shown promise in quantitative bladder cancer staging by detecting shedded Hyals in urine samples (119). Also, fluorescent HA probes may be used in an intraoperative to assess Hyal activity or drug delivery of theranostic probes (119). Often, HA probes contain more than one CA moiety to harness the strength of multimodal imaging, such as MRI/optical imaging (123, 136), MRI/computer tomography (CT) (137), NIRF/CT (138), or NIRF/PA imaging (139–141) to improve diagnostic capability and monitoring of therapeutic efficacy (121, 142).
Only a few studies report the in vivo imaging of other ECM constituents, such as fibronectin or laminins, in cancer. Fibronectin, whose expression increases with epithelial–mesenchymal transition, is typically targeted to image tumor-associated angiogenesis (143, 144). Laminins are a family of glycoproteins which interact with other ECM proteins, assuring the ECM organization, and are involved in cellular signal transduction pathways (145), cell adhesion, migration, and proliferation (146) and thus affect in cancer, tumor invasion, angiogenesis, and metastasis (145, 147). While laminins and their function have been studied extensively in vitro or ex vivo (29, 148–150), in vivo studies directly imaging laminins have been limited. Cuesta et al. developed a fluorescent trimerbody recognizing an angiogenesis-associated laminin epitope, accumulating in tumors (151). Other studies have used imaging agents targeting laminin cell surface receptors directly or indirectly to detect or treat tumors (152–154).
By tumor cells recruited adult, multipotent, non-hematopoietic stem cells (mesenchymal stromal (stem) cells, MSCs), typically derived from adipose tissue and bone marrow, have been found to differentiate into osteoblasts, CAFs, and pericytes among other cell types (155). In tumors, MSCs may contribute to tumor initiation, progression, angiogenesis, and metastasis, while also impacting immune function (155).
Cancer-associated fibroblasts are fibroblasts that reside within the tumor or tumor margins (156). They promote tumorigenic features, such as drug resistance, ECM modulation, chronic inflammation, and invasiveness (156). They may originate from normal fibroblasts or smooth muscle cells (altered by tumor cells), bone marrow-derived stem cells (mesenchymal stromal (stem) cells, MSCs), recruited and altered by tumor cells, or epithelial cells through transdifferentiation to myofibroblasts, or endothelial cells through endothelial-to-mesenchymal transition (156–158).
The TME (Figure 1) includes various immune cells: innate [tumor-associated macrophages (TAMs)], neutrophils, mast cells, myeloid-derived suppressor, dendritic, and natural killer cells, and adaptive (T and B lymphocytes), with TAMs and T cells the most prevalent cell types (159). Immune cells may enhance tumor growth and metastasis or exhibit antitumor immunity by modulating the immune and inflammatory milieu in the TME through paracrine and autocrine cell interactions, and thus, affecting the production of pro-angiogenic and growth factors, proteases, recruitment of other hematopoietic cells, or release of reactive oxygen or nitrogen species (159, 160). Immunotherapy aims at enhancing the antitumor activity of tumor-associated immune cells (161).
In preclinical cancer models, the preferential homing of ex vivo cultured MSCs to tumor tissue and metastasis has been imaged non-invasively in vivo after intravenous/arterial injection of MSCs, pre-(multi-)labeled with bioluminescence (162–167), fluorescence (168), MRI (169–171), PET (170–173), or SPECT (171, 174) imaging probes (175). The tumor effects and/or localization of MSCs, pre-labeled with an imaging probe and coinjected with tumor cells, have been monitored in vivo with MRI (176) and bioluminescence (177–180) imaging. The preferential accumulation of MSCs at sites of inflammation and tumors makes them an ideal vehicle for treatment delivery (155, 181, 182), and combined treatment/imaging MSC moieties are being developed for cell tracking and treatment monitoring (175, 177, 178, 183). While overall safe clinically (175, 184), ex vivo culture and pre-labeling of MSCs may lead to secondary tumors (185) and/or impact functionality (186). Using fluorescent, transgenic mice (187), potentially avoids ex vivo culture and pre-labeling of MSCs, with the disadvantage that typically all cell types express the imaging marker, limiting the in vivo identification of different stromal cell types, unless pre-labeled.
Various markers, such as α-smooth muscle actin (α-SMA), vimentin, and fibroblast-activation protein α (FAP), have been used to identify CAFs (188). In preclinical models, CAFs have been shown to promote breast tumor growth and metastasis by enhancing the recruitment of immune suppressor cells and TAMs (189) and to mediate collagen remodeling (190). The high expression of FAP in CAFs (191, 192) makes it a desirable target for diagnostic and therapeutic imaging, although it may also be expressed in some other tissues and tumor cells (192–194). One difficulty for the development of FAP-targeted in vivo imaging probes is that FAP shares peptide substrates with other post-prolyl peptidases, resulting in non-specific binding in vivo (192). Thus to track CAFs in vivo by MRI or NIRF imaging, Granot et al. took advantage of caveolae-mediated endocytosis in fibroblasts by pre-labeling CAFs in vitro with the CAs biotin-bovine serum albumin-gadolinium diethylenetriaminepentaacetic acid, Feridex, or 1,1′-dioctadecyl-3,3,3-tetramethylindotricarbocyanine iodide (195, 196). Recently developed FAP-specific, activatable NIR fluorescence probes (193, 194) show promise in in vivo imaging of FAP-expressing tumors. And novel cancer treatments based on the depletion of FAP-expressing stromal cells would greatly benefit from monitoring response in vivo with improved multimodal imaging probes (197).
Multiple recent reviews summarize the imaging techniques applied to track various immune cell types in vivo and to monitor immunotherapy response, including the ability to optimize administration route of therapeutic immune cells (45, 47, 61, 63, 198–202).
To visualize immune cells in vivo, cells may be labeled ex vivo with paramagnetic, fluorescent, or radiochemical probes for MRI, FLI, or PET/SPECT respectively or transfected with reporter genes for PET, SPECT, bioluminescence imaging (BLI), and/or FLI (or FMT) before injection into the host (45, 198, 201, 203–206). Tumor-infiltrating lymphocytes, dendritic cells (DCs), or TAMs can be imaged by FLI or PET/SPECT, using fluorescently labeled (Figure 6) or radiolabeled antibodies or antibody fragments targeting cell-type specific surface receptors (198, 199, 201). Delivery of full-size antibodies may be affected by vascular dysfunction in tumors, thus, potentially affecting image quality and interpretation [false positives, reduced specificity (50)]. Targeted US microbubbles have been used to track B7-H3 expressing TAMs and tumor cells (198). The high endocytic activity of TAMs facilitates their imaging by MRI, PET, and to a lesser extent by CT, FLI, US, Raman imaging, or PAI, using nanoparticles, either uncoated (e.g., ultra-small superparamagnetic iron-oxide nanoparticles for MRI) or coated to increase macrophage affinity (199, 200). Nanoparticle uptake may vary across different macrophage populations and may be detected also in other cell types (e.g., tumor cells) to a variable degree, confounding potentially macrophage tracking, but has been used successfully in clinical lymph node cancer staging by MRI (200). Cellular MRI of DC migration using CAs (iron oxide-based nanoparticles, perfluorocarbon emulsions) permits repeated monitoring (a limitation in PET/SPECT) and does not require pre-labeling with reporter genes, as in BLI or FLI, but has lower sensitivity (63). Intravital microscopy of stroma–tumor cell dynamics (207) has been an essential tool in assessing lymphocytic interactions in tumors and draining lymph nodes by optical imaging (208). The clinical translatability of these imaging methods has been reviewed previously (202, 209), with optical methods currently limited to intraoperative imaging (50).
Endothelial cells line the inside of tumor blood vessels and lymphatics and interact with pericytes and vascular smooth muscle cells in the vessel wall (Figure 1) (210–212). Cancer-associated endothelial cells often display an enhanced angiogenic potential (211), due to proangionenic factors secreted from cancer cells and/or tumor–stroma cells, forming heterogeneous neovasculature of enhanced permeability (6). The role of pericytes in tumor vascular development is still largely unexplored (212).
Tumors outgrowing their vascular supply lead to constant vascular remodeling, acute and permanent hypoxic tumor areas, and nutrient deprivation (23, 45, 213), increasing treatment resistance (due to, e.g., reduced radical formation in hypoxic tumor areas affecting radiotherapy and limiting drug delivery of chemotherapeutics) (214–216).
Vascular function and distribution has been assessed in vivo non-invasively by MRI (45, 217–226), CT (μCT) (45, 52, 227, 228), H215O or 11C PET (45, 217), US (45, 52, 229) [clinical (230)], PAI (45, 58, 114), and intravital optical imaging methods (231), such as FLI (48), second-generation OCT [optical frequency domain imaging (OFDI)] (Figure 5) (45, 48, 52, 68), and FMT (45).
In vascular MRI, five acquisition methods are used to measure the enhancement of exogenous (i, ii) or endogenous (iii–v) contrast dynamically: (i) Dynamic contrast-enhanced (DCE)-MRI, which exploits the shortening of the T1 relaxation time of water protons near a CA, typically Gd-based and low molecular weight (223, 229), (ii) dynamic susceptibility contrast (DSC)-MRI, which measures the effect of the CA (e.g., Gd-based CA or superparamagnetic iron oxide particles) on the T2 and T2* relaxation time of nearby water protons (218, 222), (iii) arterial spin labeling (ASL)-MRI, where the dynamic measurement of the in- and out-flow of magnetically labeled water protons, which serve as endogenous “CA,” characterizes the vasculature in a region of interest (223, 232), (iv) blood oxygen level dependent MRI (BOLD-MRI) where oxyhemoglobin confers diamagnetic and deoxyhemoglobin paramagnetic contrast, respectively (223), and (v) diffusion-weighted MRI (DW-MRI), where intravoxel incoherent motion (IVIM) reflects vascular perfusion (64, 224, 229, 233, 234).
In DCE- and DSC-MRI, the first pass of the exogenous CA uptake is characterized by the venous or arterial input function (219, 220, 222, 235–237), after which the CA distributes throughout the vasculature, extravasates at sites of leaky blood vessels into the interstitium, and is ultimately cleared from the body. Rate and path (e.g., liver and kidney) of tissue and vascular clearance are dependent on the specific CA (e.g., size and type of CA) used (223, 225, 238). Hemodynamic parameters, such as vessel density, vascular permeability, vascular perfusion, extravascular space, vessel size and plasma volume, etc., are either derived from semiquantitative measures of signal enhancement (220, 239–241) or from pharmacokinetic modeling of signal-versus-time curves (222, 242) with the underlying principle based on standard tracer-kinetic theory of linear and stationary systems (221, 243) and the model adjusted to account for variations of CA characteristics, e.g., low versus high molecular weight (impacting the ability of the CA to extravasate), receptor targeted (e.g., ανβ3-integrin), extra- or intracellular or both, with exchange (218, 223, 235, 244). More recently vascular feature-based analyses have been developed to assess intratumor vascular heterogeneity (245, 246).
Hemodynamic parameters conferred from ASL- and BOLD-MRI are tissue blood flow and volume (223, 232). Tissue perfusion and diffusivity are obtained from IVIM DW-MRI by fitting data with a bi-exponential model (224).
Similarly to DCE-MRI, DCE-CT has been used clinically to obtain blood flow, blood volume, and permeability with the disadvantage of radioactivity limiting serial monitoring and often worse spatial resolution than DCE-MRI (227, 228). In preclinical models, the relatively high radiation dose of μCT limits its in vivo use (45), and vascular networks have been assessed ex vivo (247). Viscosity of CA for DCE-CT may also result in complications, such as vessel rupture, and DCE-μCT still suffers from artifacts generated from, e.g., bones or large vessels (52). Generally, functional vascular parameters similar to DCE-MRI can be extracted from DCE-μCT data (52, 248).
While H215O PET measures specifically tissue perfusion (217), the short half-life of 15O limits the applicability of vascular perfusion measurements by PET (45). Vascular permeability can be obtained from dynamic PET using the macromolecular CAs 68Gd-DOTA-albumin (45), 11C-methylalbumin (limited by the 20.4 min short half-life of 11C), or 68Ga-transferrin (217). However, lack of accompanying anatomical information and ionizing radiation limits (especially serial) vascularity measurements by PET, despite its potential higher sensitivity than DCE-MRI and its ability to directly measure tissue perfusion.
Tumor angiogenesis, i.e., perfusion and vascular density have been successfully measured in small animals by Doppler or contrast-enhanced US with microbubbles (non-targeted and targeted to, e.g., VEGFR2 (249), ανβ3-integrin, or endoglin) with a spatial resolution of ~50–100 μm (45, 52, 229). One advantage of the combining contrast-enhanced and non-contrast-enhanced high-frequency volumetric power Doppler US is the ability to distinguish mature and immature vessels (52). While US is cheaper than DCE-MRI, it is not suitable for whole-body imaging, has limited soft tissue contrast, and is to some extent user dependent, limiting its applicability, especially for monitoring of antiangiogenic treatments (52).
In PAI of tumor angiogenesis, the intrinsic contrast from hemoglobin permits visualization of microvasculature and quantification of blood oxygen saturation (45, 52), with its usefulness recently extended to flow imaging (114). Submillimeter resolution is achieved, albeit restricted to depth of a few centimeters (58). Reporter genes or endogenous targeted CAs extend the ability to visualize and quantify tumor angiogenesis in vivo (52). The hemodynamic response to external stimuli or treatment is quantified from contrast changes after image reconstruction (52, 58, 114).
Blood vessel diameter, surface area, and branching pattern have been assessed with intravital optical imaging methods during tumor growth and/or treatment (Figure 5) (45, 48, 52, 68, 231).
Imaging of the lymphatic system is here summarized only briefly, as is has been reviewed in detail previously (50, 250). The lymphatic system (Figure 1) drains lymph fluid from interstitial space to the venous circulation, thus, maintaining tissue fluid homoeostatis, transports immune cells to lymphoid organs, and plays a role in lipid absorption (50, 250). While the lymphatic vasculature near tumors provides a route for metastatic dissemination of cancer cells, its role has only been explored by non-invasive imaging methods over the past decade (50, 250). Lymphangiography traces the drainage of a CA for X-ray, CT, MRI, US, PAI, or optical imaging but is lacking specificity, requiring direct injection into a lymph vessel (difficult to perform in preclinical models) or intradermal injection near sites draining into the dermal capillary plexus (50, 250). Identifying sentinel lymph nodes containing cancer cells has been achieved with intravenously injected CAs that identify blocked drainage or directly target cancer cells (50). In vivo OCT (or OFDI) and laser speckle imaging permit CA-free visualization of lymphatics (Figure 5) (68, 250). The most specific imaging approach to identify and characterize the lymphatic system is to use CAs, targeted to lymphatic vascular-specific molecules, i.e., vascular endothelial growth factor receptor-3 (VEGFR-3), lymphatic vessel hyaluronan receptor-1 (LYVE-1), podoplanin, or prospero-related homeodomain transcription factor PROX1, with LYVE-1 the most widely used lymphatic endothelial cell marker (250). LYVE-1-targeted CAs have been developed for PET (251) and optical imaging (252). Preclinically, fluorescent or bioluminescent gene reporters in transgenic mouse models have been also been used to visualize lymphatics, typically by intravital imaging methods (50).
Metabolic reprograming during tumor development and progression leads to a characteristic in vivo tumor metabolic phenotype (see Section “Introduction—The Tumor Microenvironment”).
Changes in choline-phospholipid metabolism have typically been assessed preclinically (and clinically) by non-invasive 31P and 1H magnetic resonance spectroscopy or spectroscopic imaging (MRS or MRSI) (14–16, 18, 253). Choline uptake and metabolic conversion have also been assessed with high sensitivity by 18F-fluoro-, 3H-, or 11C-choline PET and successfully translated to the clinic (254–257). As with any PET tracer though, radiolabeled choline PET does not discriminate between different metabolites (256), limiting its value for pathway studies.
Tumor hypoxia has been imaged non-invasively and visualized directly by hypoxia markers accumulating in hypoxic cells using PET, electron paramagnetic resonance (EPR), or 19F MRS (65, 248, 258–266). Delivery of a specific hypoxia marker to less vascularized regions, which are typically associated with hypoxia, may impact the intensity of the accumulating hypoxia marker (246, 267). Since evolution of chronic or acute hypoxia in tissue is linked to the vascular delivery of oxygen, several indirect MRI methods based on vascular features associated with tumor hypoxia, such as BOLD, TOLD, or DCE-MRI, have been developed to identify hypoxic areas or hypoxia changes in tumors (64, 246, 268–271). Tissue oxygen tensions have been mapped by 19F MRI oxymetry using perfluorocarbons; as with any exogenous tracer, these measurements are vascular delivery dependent, and thus, potentially biased toward well-perfused tumor regions (271). While carbonic anhydrase-IX (CA-IX) has been proposed as an intrinsic hypoxia marker in tumors, CA-IX expression, measured by immunohistochemistry, correlated to hypoxia in some and not in other studies (260). Nevertheless, attempts are underway to image CA-IX expression in vivo by PET (272), NIRF (273), and SPECT (274).
Tumor glycolysis is typically assessed by in vivo PET using the cellular entrapment of 18F-FDG, after uptake of 18F-FDG by glucose transporters (GLUT-1, GLUT-3; often overexpressed in cancer) and subsequent phosphorylation by hexokinase II (256, 275). While it is quite insensitive, 13C MRS has been applied preclinically to evaluate glycolysis and the 13C labeling of downstream metabolites (275–277). Detection sensitivity of 13C MRS can be significantly improved by magnetization transfer techniques or indirect inverse detection (276, 277). Hyperpolarization of 13C-labeled substrates increases detection sensitivity up to 10,000-fold, with the caveats that the hyperpolarization is short lived and currently limited to few substrates, including glucose (64, 275, 276, 278, 279). Compressed sensing can further improve acquisition speed and spatial resolution in hyperpolarized (HP) 13C MRSI (280). Lactate production from precursors, such as 13C-labeled pyruvate or glucose, can be rapidly assessed globally and localized by HP 13C MRSI (278, 279). Steady state levels of tumor lactate have been assessed by 1H MRS and MRSI, using spectral editing methods to suppress the high lipid signal overlapping lactate (64, 275, 281–285).
Measuring tumor tissue pH non-invasively is challenging, and various methods have been and are being developed to measure preclinically extracellular and/or intracellular pH (pHe or pHi) (64, 286).
Tumor pHe and pHi distributions can be obtained by 31P MRS/MRSI using 3-aminopropylphosphonate (287, 288). However, insensitivity of 31P MRSI limits its spatial resolution and restricts broad applicability. Thus, 1H MRSI pHe markers have been developed to improve detection sensitivity (288–291). To shorten acquisition times and improve sensitivity further, with potential for clinical translation, HP 13C MRSI of injected bicarbonate has been proposed for pH imaging, with pH (predominantly pHi, though it does not distinguish between pHi and pHe) calculated from the signal intensity ratio of hyperpolarized to 13CO2 (292, 293). However, the reaction is dependent on CA-IX activity in the tissue, thus, calibration has to be performed for each tissue type separately, restricting its applicability (294). Chemical exchange saturation transfer (CEST) MRI detects the pH-dependent chemical exchange between an amide proton and surrounding water molecules (291, 295). In acidoCEST, the pH dependence of the CEST effect ratio of a CEST agent with two amide protons, generating two CEST effects, is used to measure pHe (295–297). Challenges to measure pH by acidoCEST include its low sensitivity, requiring optimization of experimental parameters (295), and it may not always be a given that the CEST effect is solely visible for the amide protons of the selected CEST agent and only affected by pH (291).
Recently, the pH-dependency of cellular membrane insertion of radiolabeled pH (low) insertion peptides has been used to image tumor pH (at the intra-/extracellular interface) preclinically with PET (298–301).
Other non-invasive imaging modalities assessing pH in vivo include optical imaging with pH-sensitive dyes (302–304) or a pH-sensitive reporter gene (305), ratiometric PAI with pH-sensitive nanoprobes (306, 307), MRI using a CA with pH-sensitive (and concentration-dependent) relaxivity, with the difficulty of measuring in vivo the CA concentration (291), and EPR spectroscopy (308, 309).
Additionally, pH-sensitive probes are being developed as theranostic agents, combining treatment with diagnostic and monitoring ability (310–314). Of note is that all exogenous pH markers are delivery dependent and may not be clinically translatable, adding further challenges to pHe/pHi imaging.
Here, after a brief overview and some examples of recently recognized tumor–stroma interactions (see Section “Tumor–Stroma Interactions”), ongoing efforts to apply directly non-invasive multimodal imaging to characterize and understand tumor–stroma interaction in the context of tumor development, progression, and treatment will be summarized (see Section “Non-invasive In Vivo Imaging of Tumor–Stroma Interactions”). As is evident from the comparably fewer studies (see Section “Non-invasive In Vivo Imaging of Tumor–Stroma Interactions”), it is much more challenging to image directly and non-invasively the tumor–stroma interaction in in vivo cancer animal models (237, 315) and to confirm in vitro and ex vivo findings.
Tumor stroma interactions focus on the complex crosstalk between cancer and stromal cells and cell interactions with the ECM (316–320). These interactions are mediated by chemokines, soluble factors from enzymes, growth factors, extracellular vesicles (e.g., exosomes) and/or microRNAs, etc., and regulate enzymes activities, expression of genes and proteins, and metabolic pathways involved in tumor growth, metastases, survival, and drug resistance (37, 188, 211, 319–325). In this section, we present selected examples of in vitro, ex vivo, and in vivo tumor growth studies that highlight tumor–stroma interactions by using preclinical models that attempt to incorporate/simulate microenvironmental conditions of ultimately clinical relevance.
Various in vitro models mimicking the TME, such as cocultures between stromal and tumor cells or CAF-derived exosomes and cancer cells (326), 3D culture systems (327), bioreactors for live cell studies (20, 277, 328–330) have been developed to understand the nature and mechanisms behind tumor–stroma interactions by, e.g., gene expression microarrays from cocultures (331).
For example, in in vitro 2D and 3D cultures of the two breast cancer cell lines MDA-MB-231 and MCF-7 cocultured with CAFs or control fibroblasts, CAFs promoted invasion and proliferation in both MDA-MB-231 and MCF-7, and the more invasive MDA-MB-231 increased α-smooth muscle actin (α-SMA, a marker of fibroblast-to-myofibroblast transition) expression of CAFs contrary to the non-invasive MCF-7 (332), demonstrating reciprocal interaction. In cocultures of the cervical cancer cell line CSCC7 with CAFs or control fibroblasts, increased CSCC7 migration was associated with a CAF-induced decrease and partial replacement of fibrillar ECM components with laminin-1 (148). In 3D cocultures of oral tongue squamous cancer cells and CAFs in matrigel, CAFs (and CAF-conditioned medium) promoted growth, proliferation, migration, and epithelial-to-mesenchymal transition of the cancer cells (333). As observed by OCT, 3D cocultures of breast cancer cells and immortalized fibroblasts induced larger and more spherical acini with increased lumen size than cocultures using immortalized breast cells (101). Besides CAFs, the presence of TAMs has been shown also to affect ECM remodeling (334). For example, excretion of MMPs into the supernatant increased significantly in coculture of two breast cancer cell lines and macrophages, enhancing tumor cell invasiveness, and not in the benign breast cell line/macrophage coculture (335).
Tumor cells and CAFs also interact metabolically (Figure 2). As shown in vitro, CAFs take up and metabolize extracellular lactate (38) and export pyruvate which is taken up and metabolized by cancer cells (336) (Figure 2). Other research implies that epithelial cancer cells use metabolites, such as lactate, ketone bodies, and glutamine, excreted by CAFs in response to cancer cell-induced oxidative stress (39, 334, 337) (Figures 1 and 2). Glycolysis and glutamine-dependent reductive carboxylation increased in cancer cells following oxidative phosphorylation (OXPHOS) inhibition induced by exposure to CAF-derived exosomes (326). Additionally, immune cells and adipocytes may further impact the metabolic tumor phenotype (334). Closer to the in vivo scenario, ex vivo tumor/stroma immunostaining, molecular profiling from tissue microarrays of excised tumors (338), or multiplexed staining and in situ transcriptome profiling techniques (339) improve further our understanding of tumor–stroma interaction. For example, Choi et al. (338) classified breast cancer subtypes of patient tumors into four subgroups defined by the ex vivo expression of the glycolysis markers Glut-1 and/or CA-IX in the tumor and stroma, respectively: Warburg type (tumor: GLUT-1 and/or CA-IX positive; stroma: Glut-1 and CAIX negative), reverse Warburg type (tumor: Glut-1 and CAIX negative; stroma: GLUT-1 and/or CA-IX positive), mixed type (tumor and stroma: GLUT-1 and/or CA-IX positive), and null type (tumor and stroma: Glut-1 and CAIX negative). The Warburg and mixed type were predominantly associated with triple-negative breast cancer, while the reverse Warburg and null-types predominantly associated with luminal breast cancer (338).
These data/models of metabolic interaction between cancer cells and CAFs or other stromal cells highlight the complexities of metabolic crosstalk and the need for further research to understand how metabolic plasticity of tumor and stromal cells benefit tumor progression and evasion of treatment.
While MSCs can dedifferentiate into various stromal cells after recruitment to tumors, many questions about the mechanisms of MSC homing and MSC–cancer cell interaction are still topics for future research (155). In a recent study, MSCs promoted in vivo growth of subcutaneous colorectal tumor models by a β1-integrin-dependent interaction of MSCs and cancer cells (340). Coinjection of breast or prostate cancer cells with either normal fibroblasts or CAFs into animal models showed that, compared to normal fibroblasts, the presence of CAFs enhanced tumor growth (341, 342) and, as shown for the breast model, increased angiogenesis through elevated stromal cell-derived factor 1 via recruitment of endothelial progenitor cells (341). As demonstrated by in vivo fluorescence imaging and caliper tumor volume measurements, coinjection of human endometrial cancer cells with CAFs into nude mice increased tumor growth compared to tumor initiation without coinjection of CAFs (343). It was shown that the proliferation of endometrial cancer cells was increased in the presence of CAFs through the activation of JAK/STAT3/c-myc pathway (343).
Tumor–stroma interactions may sensitize tumors to treatment or be a source of treatment resistance across a wide range of therapeutics (320). And targeting tumor–stroma interactions by targeting its mediators, such as chemokines, may improve treatment response. For example, as observed with BLI, treatment of a prostate cancer model with the CXCR4-specific inhibitor AMD3100 in combination with docetaxel significantly reduced tumor growth compared to docetaxel alone (344). As a high-throughput alternative to in vivo models, an in vitro tumor cell-specific bioluminescence imaging (CS-BLI) assay for tumor–stroma cell cocultures has been proposed (345). Using this assay, it was shown that multiple myeloma cells exhibited chemoresistance to dexamethasone and doxorubicin when cocultured with bone marrow stromal cells, while effectiveness of reversine was enhanced by the presence of stromal cells (345).
Novel treatments, targeting tumor–stroma interaction by therapeutic targeting of adhesion, proteolysis, and/or signaling pathways, may improve on current treatment regimens and overcome treatment resistance (2, 346).
Studying tumor–stroma interactions in vivo enables the comprehensive characterization of the TME and its impact on treatment efficacy, potentially leading to improved diagnosis, to the identification of new treatment targets, and closing further the gap between preclinical and clinical studies (320). While single imaging methods have been used to image different aspects of the TME, only recently multimodal imaging has become more frequent. One major challenge of imaging the TME is that tumor and stromal cells use common pathways (286), necessitating cell-type-specific labeling and ideally imaging with cellular resolution. Localized, high-resolution imaging or combining multiple imaging modalities may to some extent overcome this inherent challenge. Intravital microscopy (66), which is considered a minimally invasive imaging modality, provides high-resolution imaging, including imaging of cellular processes (86, 208, 347), and has been to date the method of choice to study cancer cell interaction with the TME (Figures 3–5).
By using transgenic mice expressing green fluorescent protein (GFP) in all cells or in specific organs or driven by a cell marker and tumor cells expressing red fluorescent protein (RFP) (237, 348) or GFP in the nucleus and RFP in the cytoplasm (349), whole-body fluorescence imaging has been used to study tumor–TME interactions. While morphology and location of cells may help to identify what type of stromal cell may be involved in a specific biological process (237), specificity is lacking as all cell types of the host express the same fluorescence and ex vivo studies are needed for confirmation (349). By ex vivo validation of cell types, it was confirmed in a GFP-expressing mammary tumor model and a host with GFP-expressing macrophages, that both, cancer cells and macrophages migrated into microneedles filled with EGF, TGF-alpha, and CSF-1, as detected by multiphoton intravital microscopy (350). Using these techniques, it has been shown that paracrine loops associated with macrophage and tumor cell interaction impact tumor cell migration, intravasation, and dissemination (351).
Using intravital microscopy with multiphoton laser scanning microcopy (LSM) and SHG imaging of a human soft tissue sarcoma in VEGF-GFP mice, increased ECM remodeling by CAFs after exposure to relaxin has been imaged in vivo, with the involvement of CAFs confirmed by ex vivo cell typing (352). Using human, DsRed2- and nuclear histone 2B (H2B)-EGFP-expressing fibrosarcoma cells implanted into deep dermis of nude mice, tumor growth and tumor cell invasion into the surrounding tissue could be imaged by epifluorescence microscopy (Figure 3A) (66). In the same tumors, morphology (including collagen fibers), neoangiogenesis, cancer cell mitosis, and apoptosis were assessed in vivo during tumor growth by intravital microscopy with FLI and SHG (Figure 3B) (66). Multiphoton LSM combined with collagen (SHG) imaging of murine mammary tumors grown from a mix of a low-metastatic cell line expressing GFP and a high-metastatic subline transfected expressing CFP (cyan fluorescence protein) in the cytoplasm has been used to track and visualize cell shape, subcellular structures, and behavior in vivo (67) (Figure 4). The motility of the cells with the larger metastatic potential was about 4.5-fold higher than in the cells with low-metastatic potential with migration along collagen fibers (67).
Beyond migration and imaging of vasculature and collagen structures, the redox ratio based on endogenous NADH/(FAD + NADH) had been imaged by intravital microscopy with multiphoton fluorescence lifetime microscopy (FLIM), and redox ratio changes have been found to relate to changes observed by 18F-FDG PET, and, in ovarian cancer, were related to disease risk (49). As fluorescence lifetime changes with binding state and TME of metabolic enzymes (49), multiphoton FLIM, combined with other imaging modalities and intravital microscopy, is uniquely qualified to observe such changes in vivo, with the limitation of imaging depth.
Tumor vascularization, lymph vasculature, and vascular response to treatment have also been evaluated by intravital microscopy within the context of tumor growth and collagen structures (Figure 5) (66, 68, 86). Alexander et al. (66) imaged the intra- and perilymphatic invasion of fluorescent fibrosarcoma cells, indicative of a potential route of metastatic dissemination via the lymph vasculature located at the tumor margin (Figure 5A). Using intrinsic contrast, Vakoc et al. (68) imaged the antivascular effect of an antiangiogenic agent inhibiting VEGFR-2 on the tumor vasculature in vivo at the microscopic level, depicting lymph and blood vessels (Figure 5B). They found in response to VEGFR-2 blockade that intratumor vessel length and mean vessel diameter decreased, as tumor growth was delayed (68).
Nakasone et al. (353, 354) showed by intravital microscopy with a microlensed spinning-disk confocal microscope (355) of tumors in MMTV-PyMT mice expressing ACTB-ECFP in all host cells and c-fms-EGFP in myeloid cells, respectively, that vascular permeability and innate immune cell infiltration impact response to doxorubicin. The accumulation of macrophages with tumor growth as well as increased macrophage infiltration with increased metastatic ability have been imaged non-invasively in breast cancer models by fluorescence-reflectance imaging using a fluorescently labeled specific probe for alarmin S100A9, a calcium-binding protein secreted by monocytes/macrophages with the protein complex S100A8/A9 acting as mediator between tumor and immune cells (69) (Figure 6).
The fairly recent development of MRI/PET instrumentation permits the simultaneous imaging of metabolic, anatomical, and dynamic information, including cell tracking using appropriate labeled probes, during tumor progression and in response to treatment (70, 356–358) (Figure 7). The ability to effectively observe intratumoral function and heterogeneity over time by simultaneous MRI/PET has been demonstrated in a carcinoembryonic antigen-expressing colorectal adenocarcinoma model (Figure 7) (70). A recent study showed that microvessel volume and density index (determined from MRI) were significantly lower for glioblastoma tumors treated with bevacicumab and the PI3K/mTOR inhibitor BEZ235 combined than for tumors treated with bevacicumab alone, while 18F-FET (O-(2-[18F]Fluoroethyl)-l-tyrosine) uptake, a PET tracer to assess vessel amino acid transport, remained unchanged between the two treatments (359). Further, tumor growth, as determined from MRI, and cell proliferation, as determined from 18F-FLT PET, were the same for the bevacicumab/BEZ235 combination therapy and the bevacicumab alone treatment groups (359). The in vivo results were validated by ex vivo studies (359).
While still significant more research needs to be done, these studies show the potential of harnessing the strengths of different imaging modalities to image tumor–stroma interaction within the TME in vivo, and thus, enhancing our understanding of its impact on tumor growth and treatment response.
The strengths of optical imaging are its high sensitivity for CAs, ability to use a wide range of probes, including activatable probes and reporter genes, and compared to other imaging modalities, such as MRI and PET, low cost. However, optical imaging is typically semiquantitative, limited by penetration depth, small field of view, and, depending on method, high background signals and lack of tomographic information. Some of these limitations are overcome by PAI, which permits real-time quantitative imaging but is hampered by the range of available CAs. Ultrasound imaging is a low cost, rapid, real-time imaging modality with high temporal and spatial resolution, but has a limited field of view with low soft tissue contrast, and is typically semiquantitative and user dependent. Computer tomography is rapid, permits whole-body imaging, has high spatial resolution, is user independent, and mostly low cost, but is limited by its low sensitivity to CAs, lack of endogenous soft tissue contrast and exposure to radiation. Scanners for MRI (MRSI), PET, and SPECT are high in cost with the distinct advantage of whole-body imaging capabilities. While MRI has excellent soft tissue contrast, high spatial resolution, and has a wide range of methods available for tissue imaging and vessel characterization, it is limited by its low sensitivity and the fairly long acquisitions, the latter particularly prominent in spectroscopic imaging. To overcome these challenges, new methods, such as hyperpolarized 13C MRSI, are being actively developed. The high sensitivity of PET and SPECT, respectively, is offset by their low resolution (1–2 mm), lack of morphological information and radiation exposure from the radioactive tracers, whose half-lives range from 75 s (Rb-82) to 4.18 days (I-124) for PET radioisotopes and from 6 h (Tc-99m) to 59 days (I-125) for SPECT tracers. With the advancement of MRI/PET, the power of various MRS and MRI methods beyond anatomy and DCE-MRI, such as MRS(I), can be harnessed for future studies, distinguishing itself from PET/CT with reduced radiation exposure, the latter making MRI/PET a powerful tool for serial monitoring.
While CT, MRI (MRSI), PET, SPECT, and US are already standard imaging tools in the clinic, for localized applications, e.g., detecting cancer cells at tumor margins during surgery (360), optical imaging is being assiduously developed. Aside from physical parameters specific to each imaging modality, clinical imaging of tumor–stroma interaction will also be in part defined by the successful development of safe tracers/CAs.
In the majority of preclinical studies, specific aspects of the TME and its stromal components have been investigated separately (a few aspects at a time), selecting the non-invasive preclinical imaging modality best suited for the task. However, recent strong evidence pointing to the importance of the interaction between tumor cells and multiple components of the TME in tumor development, growth, metastases, and treatment response, including drug resistance, has generated a strong interest to further develop imaging technologies to investigate tumor–stroma interactions non-invasively in vivo. Despite recent research efforts, the comprehensive characterization (including serial monitoring) of the TME and tumor–stroma interactions non-invasively in vivo requires further advancement and to take advantage of the strengths of multimodal imaging tools for preclinical studies, and ultimately for clinical translation.
EA and NR: conception, design, and writing of review article.
The authors declare that the research was conducted in the absence of any commercial or financial relationships that could be construed as a potential conflict of interest.
The authors would like to thank Dr. Radka Stoyanova and Dr. Avigdor Leftin for their helpful reading of the manuscript.
We acknowledge salary support for Dr. N. Ramamonjisoa from a Cycle for Sarcoma grant and an Imaging and Radiation Sciences grant from MSKCC, as well as salary support for Dr. E. Ackerstaff from grants R01 CA172846 (NIH), R01 CA163980 (NIH), P50 CA092629 (NIH), and PC120233 (DOD). We also acknowledge the Memorial Sloan Kettering Cancer Center support grant P30 CA008748
1. Vaupel P, Kallinowski F, Okunieff P. Blood flow, oxygen and nutrient supply, and metabolic microenvironment of human tumors: a review. Cancer Res (1989) 49(23):6449–65.
2. Liotta LA, Kohn EC. The microenvironment of the tumour-host interface. Nature (2001) 411(6835):375–9. doi:10.1038/35077241
3. Swietach P, Vaughan-Jones RD, Harris AL. Regulation of tumor pH and the role of carbonic anhydrase 9. Cancer Metastasis Rev (2007) 26(2):299–310. doi:10.1007/s10555-007-9064-0
4. Pathak AP, Artemov D, Ward BD, Jackson DG, Neeman M, Bhujwalla ZM. Characterizing extravascular fluid transport of macromolecules in the tumor interstitium by magnetic resonance imaging. Cancer Res (2005) 65(4):1425–32. doi:10.1158/0008-5472.CAN-04-3682
5. LeBleu VS. Imaging the tumor microenvironment. Cancer J (2015) 21(3):174–8. doi:10.1097/PPO.0000000000000118
6. Payne SJ, Jones L. Influence of the tumor microenvironment on angiogenesis. Future Oncol (2011) 7(3):395–408. doi:10.2217/fon.11.13
7. Pouyssegur J, Dayan F, Mazure NM. Hypoxia signalling in cancer and approaches to enforce tumour regression. Nature (2006) 441(7092):437–43. doi:10.1038/nature04871
9. Warburg O, Wind F, Negelein E. The metabolism of tumors in the body. J Gen Physiol (1927) 8(6):519–30. doi:10.1085/jgp.8.6.519
10. Daye D, Wellen KE. Metabolic reprogramming in cancer: unraveling the role of glutamine in tumorigenesis. Semin Cell Dev Biol (2012) 23(4):362–9. doi:10.1016/j.semcdb.2012.02.002
11. De Vitto H, Perez-Valencia J, Radosevich JA. Glutamine at focus: versatile roles in cancer. Tumour Biol (2016) 37(2):1541–58. doi:10.1007/s13277-015-4671-9
12. Wise DR, Thompson CB. Glutamine addiction: a new therapeutic target in cancer. Trends Biochem Sci (2010) 35(8):427–33. doi:10.1016/j.tibs.2010.05.003
13. DeBerardinis RJ, Mancuso A, Daikhin E, Nissim I, Yudkoff M, Wehrli S, et al. Beyond aerobic glycolysis: transformed cells can engage in glutamine metabolism that exceeds the requirement for protein and nucleotide synthesis. Proc Natl Acad Sci U S A (2007) 104(49):19345–50. doi:10.1073/pnas.0709747104
14. Ackerstaff E, Glunde K, Bhujwalla ZM. Choline phospholipid metabolism: a target in cancer cells? J Cell Biochem (2003) 90(3):525–33. doi:10.1002/jcb.10659
15. Glunde K, Ackerstaff E, Mori N, Jacobs MA, Bhujwalla ZM. Choline phospholipid metabolism in cancer: consequences for molecular pharmaceutical interventions. Mol Pharm (2006) 3(5):496–506. doi:10.1021/mp060067e
16. Podo F. Tumour phospholipid metabolism. NMR Biomed (1999) 12(7):413–39. doi:10.1002/(SICI)1099-1492(199911)12:7<413::AID-NBM587>3.3.CO;2-L
17. Ridgway ND. The role of phosphatidylcholine and choline metabolites to cell proliferation and survival. Crit Rev Biochem Mol Biol (2013) 48(1):20–38. doi:10.3109/10409238.2012.735643
18. Glunde K, Bhujwalla ZM, Ronen SM. Choline metabolism in malignant transformation. Nat Rev Cancer (2011) 11(12):835–48. doi:10.1038/nrc3162
19. Awwad HM, Geisel J, Obeid R. The role of choline in prostate cancer. Clin Biochem (2012) 45(18):1548–53. doi:10.1016/j.clinbiochem.2012.08.012
20. Simoes RV, Serganova IS, Kruchevsky N, Leftin A, Shestov AA, Thaler HT, et al. Metabolic plasticity of metastatic breast cancer cells: adaptation to changes in the microenvironment. Neoplasia (2015) 17(8):671–84. doi:10.1016/j.neo.2015.08.005
21. Yoshida GJ. Metabolic reprogramming: the emerging concept and associated therapeutic strategies. J Exp Clin Cancer Res (2015) 34:111. doi:10.1186/s13046-015-0221-y
22. Xing Y, Zhao S, Zhou BP, Mi J. Metabolic reprogramming of the tumour microenvironment. FEBS J (2015) 282(20):3892–8. doi:10.1111/febs.13402
23. Polet F, Feron O. Endothelial cell metabolism and tumour angiogenesis: glucose and glutamine as essential fuels and lactate as the driving force. J Intern Med (2013) 273(2):156–65. doi:10.1111/joim.12016
24. DeBerardinis RJ, Lum JJ, Hatzivassiliou G, Thompson CB. The biology of cancer: metabolic reprogramming fuels cell growth and proliferation. Cell Metab (2008) 7(1):11–20. doi:10.1016/j.cmet.2007.10.002
25. Marchiq I, Pouyssegur J. Hypoxia, cancer metabolism and the therapeutic benefit of targeting lactate/H(+) symporters. J Mol Med (Berl) (2016) 94(2):155–71. doi:10.1007/s00109-015-1307-x
26. Hanahan D, Weinberg RA. Hallmarks of cancer: the next generation. Cell (2011) 144(5):646–74. doi:10.1016/j.cell.2011.02.013
27. Romero-Garcia S, Moreno-Altamirano MM, Prado-Garcia H, Sanchez-Garcia FJ. Lactate contribution to the tumor microenvironment: mechanisms, effects on immune cells and therapeutic relevance. Front Immunol (2016) 7:52. doi:10.3389/fimmu.2016.00052
28. Park CC, Bissell MJ, Barcellos-Hoff MH. The influence of the microenvironment on the malignant phenotype. Mol Med Today (2000) 6(8):324–9. doi:10.1016/S1357-4310(00)01756-1
29. Mahadevan D, Von Hoff DD. Tumor-stroma interactions in pancreatic ductal adenocarcinoma. Mol Cancer Ther (2007) 6(4):1186–97. doi:10.1158/1535-7163.MCT-06-0686
30. Kopfstein L, Christofori G. Metastasis: cell-autonomous mechanisms versus contributions by the tumor microenvironment. Cell Mol Life Sci (2006) 63(4):449–68. doi:10.1007/s00018-005-5296-8
31. Jodele S, Blavier L, Yoon JM, DeClerck YA. Modifying the soil to affect the seed: role of stromal-derived matrix metalloproteinases in cancer progression. Cancer Metastasis Rev (2006) 25(1):35–43. doi:10.1007/s10555-006-7887-8
32. Farnsworth WE. Prostate stroma: physiology. Prostate (1999) 38(1):60–72. doi:10.1002/(SICI)1097-0045(19990101)38:1<60::AID-PROS8>3.0.CO;2-3
33. Romero IL, Mukherjee A, Kenny HA, Litchfield LM, Lengyel E. Molecular pathways: trafficking of metabolic resources in the tumor microenvironment. Clin Cancer Res (2015) 21(4):680–6. doi:10.1158/1078-0432.CCR-14-2198
34. Martinez-Outschoorn UE, Pavlides S, Howell A, Pestell RG, Tanowitz HB, Sotgia F, et al. Stromal-epithelial metabolic coupling in cancer: integrating autophagy and metabolism in the tumor microenvironment. Int J Biochem Cell Biol (2011) 43(7):1045–51. doi:10.1016/j.biocel.2011.01.023
35. Ratnikov B, Jeon YJ, Smith JW, Ronai ZA. Right on TARGET: glutamine metabolism in cancer. Oncoscience (2015) 2(8):681–3. doi:10.18632/oncoscience.205
36. Lisanti MP, Martinez-Outschoorn UE, Sotgia F. Oncogenes induce the cancer-associated fibroblast phenotype: metabolic symbiosis and “fibroblast addiction” are new therapeutic targets for drug discovery. Cell Cycle (2013) 12(17):2723–32. doi:10.4161/cc.25695
37. Correia AL, Bissell MJ. The tumor microenvironment is a dominant force in multidrug resistance. Drug Resist Updat (2012) 15(1–2):39–49. doi:10.1016/j.drup.2012.01.006
38. Rattigan YI, Patel BB, Ackerstaff E, Sukenick G, Koutcher JA, Glod JW, et al. Lactate is a mediator of metabolic cooperation between stromal carcinoma associated fibroblasts and glycolytic tumor cells in the tumor microenvironment. Exp Cell Res (2012) 318(4):326–35. doi:10.1016/j.yexcr.2011.11.014
39. Pavlides S, Vera I, Gandara R, Sneddon S, Pestell RG, Mercier I, et al. Warburg meets autophagy: cancer-associated fibroblasts accelerate tumor growth and metastasis via oxidative stress, mitophagy, and aerobic glycolysis. Antioxid Redox Signal (2012) 16(11):1264–84. doi:10.1089/ars.2011.4243
40. Hanahan D, Coussens LM. Accessories to the crime: functions of cells recruited to the tumor microenvironment. Cancer Cell (2012) 21(3):309–22. doi:10.1016/j.ccr.2012.02.022
41. Pickup MW, Mouw JK, Weaver VM. The extracellular matrix modulates the hallmarks of cancer. EMBO Rep (2014) 15(12):1243–53. doi:10.15252/embr.201439246
42. Hagedorn HG, Bachmeier BE, Nerlich AG. Synthesis and degradation of basement membranes and extracellular matrix and their regulation by TGF-beta in invasive carcinomas (Review). Int J Oncol (2001) 18(4):669–81. doi:10.3892/ijo.18.4.669
43. Turley SJ, Cremasco V, Astarita JL. Immunological hallmarks of stromal cells in the tumour microenvironment. Nat Rev Immunol (2015) 15(11):669–82. doi:10.1038/nri3902
44. Friedl P, Brocker EB. The biology of cell locomotion within three-dimensional extracellular matrix. Cell Mol Life Sci (2000) 57(1):41–64. doi:10.1007/s000180050498
45. Narunsky L, Oren R, Bochner F, Neeman M. Imaging aspects of the tumor stroma with therapeutic implications. Pharmacol Ther (2014) 141(2):192–208. doi:10.1016/j.pharmthera.2013.10.003
46. Kobayashi H, Longmire MR, Ogawa M, Choyke PL. Rational chemical design of the next generation of molecular imaging probes based on physics and biology: mixing modalities, colors and signals. Chem Soc Rev (2011) 40(9):4626–48. doi:10.1039/c1cs15077d
47. Youn H, Hong KJ. In vivo non invasive molecular imaging for immune cell tracking in small animals. Immune Netw (2012) 12(6):223–9. doi:10.4110/in.2012.12.6.223
48. Zhou ZN, Boimel PJ, Segall JE. Tumor-stroma: in vivo assays and intravital imaging to study cell migration and metastasis. Drug Discov Today Dis Models (2011) 8(2–3):95–112. doi:10.1016/j.ddmod.2011.07.003
49. Provenzano PP, Eliceiri KW, Keely PJ. Multiphoton microscopy and fluorescence lifetime imaging microscopy (FLIM) to monitor metastasis and the tumor microenvironment. Clin Exp Metastasis (2009) 26(4):357–70. doi:10.1007/s10585-008-9204-0
50. Sevick-Muraca EM, Kwon S, Rasmussen JC. Emerging lymphatic imaging technologies for mouse and man. J Clin Invest (2014) 124(3):905–14. doi:10.1172/JCI71612
51. Stuker F, Ripoll J, Rudin M. Fluorescence molecular tomography: principles and potential for pharmaceutical research. Pharmaceutics (2011) 3(2):229–74. doi:10.3390/pharmaceutics3020229
52. Ehling J, Lammers T, Kiessling F. Non-invasive imaging for studying anti-angiogenic therapy effects. Thromb Haemost (2013) 109(3):375–90. doi:10.1160/TH12-10-0721
53. Noreen R, Chien CC, Chen HH, Bobroff V, Moenner M, Javerzat S, et al. FTIR spectro-imaging of collagen scaffold formation during glioma tumor development. Anal Bioanal Chem (2013) 405(27):8729–36. doi:10.1007/s00216-013-7337-8
54. Bhargava R. Infrared spectroscopic imaging: the next generation. Appl Spectrosc (2012) 66(10):1091–120. doi:10.1366/12-06801
55. Bellisola G, Sorio C. Infrared spectroscopy and microscopy in cancer research and diagnosis. Am J Cancer Res (2012) 2(1):1–21.
56. Lasch P, Naumann D. Spatial resolution in infrared microspectroscopic imaging of tissues. Biochim Biophys Acta (2006) 1758(7):814–29. doi:10.1016/j.bbamem.2006.06.008
57. Dhawan AP, D’Alessandro B, Fu X. Optical imaging modalities for biomedical applications. IEEE Rev Biomed Eng (2010) 3:69–92. doi:10.1109/RBME.2010.2081975
58. Xu MH, Wang LHV. Photoacoustic imaging in biomedicine. Rev Sci Instrum (2006) 77(4). doi:10.1063/1.2195024
59. Wu D, Huang L, Jiang MS, Jiang H. Contrast agents for photoacoustic and thermoacoustic imaging: a review. Int J Mol Sci (2014) 15(12):23616–39. doi:10.3390/ijms151223616
60. Mallidi S, Luke GP, Emelianov S. Photoacoustic imaging in cancer detection, diagnosis, and treatment guidance. Trends Biotechnol (2011) 29(5):213–21. doi:10.1016/j.tibtech.2011.01.006
61. Weigelin B, Bakker GJ, Friedl P. Third harmonic generation microscopy of cells and tissue organization. J Cell Sci (2016) 129(2):245–55. doi:10.1242/jcs.152272
62. Strupler M, Pena AM, Hernest M, Tharaux PL, Martin JL, Beaurepaire E, et al. Second harmonic imaging and scoring of collagen in fibrotic tissues. Opt Express (2007) 15(7):4054–65. doi:10.1364/OE.15.004054
63. Dekaban GA, Hamilton AM, Fink CA, Au B, de Chickera SN, Ribot EJ, et al. Tracking and evaluation of dendritic cell migration by cellular magnetic resonance imaging. Wiley Interdiscip Rev Nanomed Nanobiotechnol (2013) 5(5):469–83. doi:10.1002/wnan.1227
64. Bokacheva L, Ackerstaff E, LeKaye HC, Zakian K, Koutcher JA. High-field small animal magnetic resonance oncology studies. Phys Med Biol (2014) 59(2):R65–127. doi:10.1088/0031-9155/59/2/R65
65. Vikram DS, Zweier JL, Kuppusamy P. Methods for noninvasive imaging of tissue hypoxia. Antioxid Redox Signal (2007) 9(10):1745–56. doi:10.1089/ars.2007.1717
66. Alexander S, Koehl GE, Hirschberg M, Geissler EK, Friedl P. Dynamic imaging of cancer growth and invasion: a modified skin-fold chamber model. Histochem Cell Biol (2008) 130(6):1147–54. doi:10.1007/s00418-008-0529-1
67. Sahai E, Wyckoff J, Philippar U, Segall JE, Gertler F, Condeelis J. Simultaneous imaging of GFP, CFP and collagen in tumors in vivo using multiphoton microscopy. BMC Biotechnol (2005) 5:14. doi:10.1186/1472-6750-5-14
68. Vakoc BJ, Lanning RM, Tyrrell JA, Padera TP, Bartlett LA, Stylianopoulos T, et al. Three-dimensional microscopy of the tumor microenvironment in vivo using optical frequency domain imaging. Nat Med (2009) 15(10):1219–23. doi:10.1038/nm.1971
69. Becker A, Grosse Hokamp N, Zenker S, Flores-Borja F, Barzcyk K, Varga G, et al. Optical in vivo imaging of the alarmin S100A9 in tumor lesions allows for estimation of the individual malignant potential by evaluation of tumor-host cell interaction. J Nucl Med (2015) 56(3):450–6. doi:10.2967/jnumed.114.146688
70. Ng TS, Bading JR, Park R, Sohi H, Procissi D, Colcher D, et al. Quantitative, simultaneous PET/MRI for intratumoral imaging with an MRI-compatible PET scanner. J Nucl Med (2012) 53(7):1102–9. doi:10.2967/jnumed.111.099861
71. Mecham RP. Overview of extracellular matrix. Curr Protoc Cell Biol (2012) Chapter 10:Unit 10.1. doi:10.1002/0471143030.cb1001s57
72. Lu P, Weaver VM, Werb Z. The extracellular matrix: a dynamic niche in cancer progression. J Cell Biol (2012) 196(4):395–406. doi:10.1083/jcb.201102147
73. Lu P, Takai K, Weaver VM, Werb Z. Extracellular matrix degradation and remodeling in development and disease. Cold Spring Harb Perspect Biol (2011) 3(12):a005058. doi:10.1101/cshperspect.a005058
74. Kessenbrock K, Plaks V, Werb Z. Matrix metalloproteinases: regulators of the tumor microenvironment. Cell (2010) 141(1):52–67. doi:10.1016/j.cell.2010.03.015
75. Verma RP, Hansch C. Matrix metalloproteinases (MMPs): chemical-biological functions and (Q)SARs. Bioorg Med Chem (2007) 15(6):2223–68. doi:10.1016/j.bmc.2007.01.011
76. Page-McCaw A, Ewald AJ, Werb Z. Matrix metalloproteinases and the regulation of tissue remodelling. Nat Rev Mol Cell Biol (2007) 8(3):221–33. doi:10.1038/nrm2125
77. Worth DC, Parsons M. Advances in imaging cell-matrix adhesions. J Cell Sci (2010) 123(Pt 21):3629–38. doi:10.1242/jcs.064485
78. Valderrama R, Navarro S, Campo E, Camps J, Gimenez A, Pares A, et al. Quantitative measurement of fibrosis in pancreatic tissue. Evaluation of a colorimetric method. Int J Pancreatol (1991) 10(1):23–9.
79. Taskiran D, Taskiran E, Yercan H, Kutay FZ. Quantification of total collagen in rabbit tendon by the sirius red method. Tr J Med Sci (1999) 29:7–9.
80. Plodinec M, Loparic M, Aebi U. Imaging collagen II using atomic force microscopy (AFM). Cold Spring Harb Protoc (2010) 2010(10):db.rot5501. doi:10.1101/pdb.prot5501
81. Maller O, Hansen KC, Lyons TR, Acerbi I, Weaver VM, Prekeris R, et al. Collagen architecture in pregnancy-induced protection from breast cancer. J Cell Sci (2013) 126(Pt 18):4108–10. doi:10.1242/jcs.121590
82. Stamov DR, Stock E, Franz CM, Jahnke T, Haschke H. Imaging collagen type I fibrillogenesis with high spatiotemporal resolution. Ultramicroscopy (2015) 149:86–94. doi:10.1016/j.ultramic.2014.10.003
83. Dang TT, Prechtl AM, Pearson GW. Breast cancer subtype-specific interactions with the microenvironment dictate mechanisms of invasion. Cancer Res (2011) 71(21):6857–66. doi:10.1158/0008-5472.CAN-11-1818
84. Peng CW, Liu XL, Chen C, Liu X, Yang XQ, Pang DW, et al. Patterns of cancer invasion revealed by QDs-based quantitative multiplexed imaging of tumor microenvironment. Biomaterials (2011) 32(11):2907–17. doi:10.1016/j.biomaterials.2010.12.053
85. Fang M, Peng CW, Yuan JP, Zhang ZL, Pang DW, Li Y. Coevolution of the tumor microenvironment revealed by quantum dot-based multiplexed imaging of hepatocellular carcinoma. Future Oncol (2013) 9(7):1029–37. doi:10.2217/fon.13.63
86. Alexander S, Weigelin B, Winkler F, Friedl P. Preclinical intravital microscopy of the tumour-stroma interface: invasion, metastasis, and therapy response. Curr Opin Cell Biol (2013) 25(5):659–71. doi:10.1016/j.ceb.2013.07.001
87. Wu PC, Hsieh TY, Tsai ZU, Liu TM. In vivo quantification of the structural changes of collagens in a melanoma microenvironment with second and third harmonic generation microscopy. Sci Rep (2015) 5:8879. doi:10.1038/srep08879
88. Williams RM, Zipfel WR, Webb WW. Multiphoton microscopy in biological research. Curr Opin Chem Biol (2001) 5(5):603–8. doi:10.1016/S1367-5931(00)00241-6
89. Zoumi A, Yeh A, Tromberg BJ. Imaging cells and extracellular matrix in vivo by using second-harmonic generation and two-photon excited fluorescence. Proc Natl Acad Sci U S A (2002) 99(17):11014–9. doi:10.1073/pnas.172368799
90. Keikhosravi A, Bredfeldt JS, Sagar AK, Eliceiri KW. Second-harmonic generation imaging of cancer. Methods Cell Biol (2014) 123:531–46. doi:10.1016/B978-0-12-420138-5.00028-8
91. Raja AM, Xu S, Sun W, Zhou J, Tai DC, Chen CS, et al. Pulse-modulated second harmonic imaging microscope quantitatively demonstrates marked increase of collagen in tumor after chemotherapy. J Biomed Opt (2010) 15(5):056016. doi:10.1117/1.3497565
92. Drifka CR, Tod J, Loeffler AG, Liu Y, Thomas GJ, Eliceiri KW, et al. Periductal stromal collagen topology of pancreatic ductal adenocarcinoma differs from that of normal and chronic pancreatitis. Mod Pathol (2015) 28(11):1470–80. doi:10.1038/modpathol.2015.97
93. Bredfeldt JS, Liu Y, Conklin MW, Keely PJ, Mackie TR, Eliceiri KW. Automated quantification of aligned collagen for human breast carcinoma prognosis. J Pathol Inform (2014) 5:28. doi:10.4103/2153-3539.139707
94. Kirkpatrick ND, Andreou S, Hoying JB, Utzinger U. Live imaging of collagen remodeling during angiogenesis. Am J Physiol Heart Circ Physiol (2007) 292(6):H3198–206. doi:10.1152/ajpheart.01234.2006
95. Pfeffer CP, Olsen BR, Ganikhanov F, Legare F. Multimodal nonlinear optical imaging of collagen arrays. J Struct Biol (2008) 164(1):140–5. doi:10.1016/j.jsb.2008.07.002
96. Madsen DH, Bugge TH. Imaging collagen degradation in vivo highlights a key role for M2-polarized macrophages in extracellular matrix degradation. Oncoimmunology (2013) 2(12):e27127. doi:10.4161/onci.27127
97. Wahyudi H, Reynolds AA, Li Y, Owen SC, Yu SM. Targeting collagen for diagnostic imaging and therapeutic delivery. J Control Release (2016) 240:323–31. doi:10.1016/j.jconrel.2016.01.007
98. Mercado KP, Helguera M, Hocking DC, Dalecki D. Noninvasive quantitative imaging of collagen microstructure in three-dimensional hydrogels using high-frequency ultrasound. Tissue Eng Part C Methods (2015) 21(7):671–82. doi:10.1089/ten.TEC.2014.0527
99. Riggin CN, Sarver JJ, Freedman BR, Thomas SJ, Soslowsky LJ. Analysis of collagen organization in mouse achilles tendon using high-frequency ultrasound imaging. J Biomech Eng (2014) 136(2):021029. doi:10.1115/1.4026285
100. Ring HC, Mogensen M, Hussain AA, Steadman N, Banzhaf C, Themstrup L, et al. Imaging of collagen deposition disorders using optical coherence tomography. J Eur Acad Dermatol Venereol (2015) 29(5):890–8. doi:10.1111/jdv.12708
101. Chhetri RK, Phillips ZF, Troester MA, Oldenburg AL. Longitudinal study of mammary epithelial and fibroblast co-cultures using optical coherence tomography reveals morphological hallmarks of pre-malignancy. PLoS One (2012) 7(11):e49148. doi:10.1371/journal.pone.0049148
102. Wang P, Wang P, Wang HW, Cheng JX. Mapping lipid and collagen by multispectral photoacoustic imaging of chemical bond vibration. J Biomed Opt (2012) 17(9):96010–1. doi:10.1117/1.JBO.17.9.096010
103. Fang M, Yuan JP, Peng CW, Pang DW, Li Y. Quantum dots-based in situ molecular imaging of dynamic changes of collagen IV during cancer invasion. Biomaterials (2013) 34(34):8708–17. doi:10.1016/j.biomaterials.2013.07.069
104. Zheng HM, Chen C, Wu XH, Chen J, Sun S, Sun JZ, et al. Quantum dot-based in situ simultaneous molecular imaging and quantitative analysis of EGFR and collagen IV and identification of their prognostic value in triple-negative breast cancer. Tumour Biol (2016) 37(2):2509–18. doi:10.1007/s13277-015-4079-6
105. Jin HE, Farr R, Lee SW. Collagen mimetic peptide engineered M13 bacteriophage for collagen targeting and imaging in cancer. Biomaterials (2014) 35(33):9236–45. doi:10.1016/j.biomaterials.2014.07.044
106. Li Y, Foss CA, Pomper MG, Yu SM. Imaging denatured collagen strands in vivo and ex vivo via photo-triggered hybridization of caged collagen mimetic peptides. J Vis Exp (2014) 83:e51052. doi:10.3791/51052
107. Brown GT, Murray GI. Current mechanistic insights into the roles of matrix metalloproteinases in tumour invasion and metastasis. J Pathol (2015) 237(3):273–81. doi:10.1002/path.4586
108. Scherer RL, McIntyre JO, Matrisian LM. Imaging matrix metalloproteinases in cancer. Cancer Metastasis Rev (2008) 27(4):679–90. doi:10.1007/s10555-008-9152-9
109. Yang Y, Hong H, Zhang Y, Cai W. Molecular imaging of proteases in cancer. Cancer Growth Metastasis (2009) 2:13–27.
110. Lebel R, Lepage M. A comprehensive review on controls in molecular imaging: lessons from MMP-2 imaging. Contrast Media Mol Imaging (2014) 9(3):187–210. doi:10.1002/cmmi.1555
111. Shay G, Lynch CC, Fingleton B. Moving targets: emerging roles for MMPs in cancer progression and metastasis. Matrix Biol (2015) 44-46:200–6. doi:10.1016/j.matbio.2015.01.019
112. Chuang CH, Chuang KH, Wang HE, Roffler SR, Shiea JT, Tzou SC, et al. In vivo positron emission tomography imaging of protease activity by generation of a hydrophobic product from a noninhibitory protease substrate. Clin Cancer Res (2012) 18(1):238–47. doi:10.1158/1078-0432.CCR-11-0608
113. Cox B, Laufer JG, Arridge SR, Beard PC. Quantitative spectroscopic photoacoustic imaging: a review. J Biomed Opt (2012) 17(6):061202. doi:10.1117/1.JBO.17.6.061202
114. van den Berg PJ, Daoudi K, Steenbergen W. Review of photoacoustic flow imaging: its current state and its promises. Photoacoustics (2015) 3(3):89–99. doi:10.1016/j.pacs.2015.08.001
115. Levi J, Kothapalli SR, Bohndiek S, Yoon JK, Dragulescu-Andrasi A, Nielsen C, et al. Molecular photoacoustic imaging of follicular thyroid carcinoma. Clin Cancer Res (2013) 19(6):1494–502. doi:10.1158/1078-0432.CCR-12-3061
116. Salaun M, Peng J, Hensley HH, Roder N, Flieder DB, Houlle-Crepin S, et al. MMP-13 in-vivo molecular imaging reveals early expression in lung adenocarcinoma. PLoS One (2015) 10(7):e0132960. doi:10.1371/journal.pone.0132960
117. Al Rawashdeh W, Arns S, Gremse F, Ehling J, Knuchel-Clarke R, Kray S, et al. Optical tomography of MMP activity allows a sensitive noninvasive characterization of the invasiveness and angiogenesis of SCC xenografts. Neoplasia (2014) 16(3):235–46,246.e1. doi:10.1016/j.neo.2014.03.005
118. Toole BP. Hyaluronan promotes the malignant phenotype. Glycobiology (2002) 12(3):37R–42R. doi:10.1093/glycob/12.3.37R
119. McAtee CO, Barycki JJ, Simpson MA. Emerging roles for hyaluronidase in cancer metastasis and therapy. Adv Cancer Res (2014) 123:1–34. doi:10.1016/B978-0-12-800092-2.00001-0
120. Choi KY, Saravanakumar G, Park JH, Park K. Hyaluronic acid-based nanocarriers for intracellular targeting: interfacial interactions with proteins in cancer. Colloids Surf B Biointerfaces (2012) 99:82–94. doi:10.1016/j.colsurfb.2011.10.029
121. Tripodo G, Trapani A, Torre ML, Giammona G, Trapani G, Mandracchia D. Hyaluronic acid and its derivatives in drug delivery and imaging: recent advances and challenges. Eur J Pharm Biopharm (2015) 97(Pt B):400–16. doi:10.1016/j.ejpb.2015.03.032
122. Veiseh M, Turley EA. Hyaluronan metabolism in remodeling extracellular matrix: probes for imaging and therapy of breast cancer. Integr Biol (Camb) (2011) 3(4):304–15. doi:10.1039/c0ib00096e
123. Cho HJ, Yoon HY, Koo H, Ko SH, Shim JS, Cho JH, et al. Hyaluronic acid-ceramide-based optical/MR dual imaging nanoprobe for cancer diagnosis. J Control Release (2012) 162(1):111–8. doi:10.1016/j.jconrel.2012.06.011
124. Swierczewska M, Choi KY, Mertz EL, Huang X, Zhang F, Zhu L, et al. A facile, one-step nanocarbon functionalization for biomedical applications. Nano Lett (2012) 12(7):3613–20. doi:10.1021/nl301309g
125. Park J, Ku M, Kim E, Park Y, Hong Y, Haam S, et al. CD44-specific supramolecular hydrogels for fluorescence molecular imaging of stem-like gastric cancer cells. Integr Biol (Camb) (2013) 5(4):669–72. doi:10.1039/c3ib20203h
126. Park JH, Cho HJ, Yoon HY, Yoon IS, Ko SH, Shim JS, et al. Hyaluronic acid derivative-coated nanohybrid liposomes for cancer imaging and drug delivery. J Control Release (2014) 174:98–108. doi:10.1016/j.jconrel.2013.11.016
127. Lim EK, Kim HO, Jang E, Park J, Lee K, Suh JS, et al. Hyaluronan-modified magnetic nanoclusters for detection of CD44-overexpressing breast cancer by MR imaging. Biomaterials (2011) 32(31):7941–50. doi:10.1016/j.biomaterials.2011.06.077
128. Li J, He Y, Sun W, Luo Y, Cai H, Pan Y, et al. Hyaluronic acid-modified hydrothermally synthesized iron oxide nanoparticles for targeted tumor MR imaging. Biomaterials (2014) 35(11):3666–77. doi:10.1016/j.biomaterials.2014.01.011
129. Yoon HY, Koo H, Choi KY, Lee SJ, Kim K, Kwon IC, et al. Tumor-targeting hyaluronic acid nanoparticles for photodynamic imaging and therapy. Biomaterials (2012) 33(15):3980–9. doi:10.1016/j.biomaterials.2012.02.016
130. Yoon HY, Koo H, Choi KY, Chan Kwon I, Choi K, Park JH, et al. Photo-crosslinked hyaluronic acid nanoparticles with improved stability for in vivo tumor-targeted drug delivery. Biomaterials (2013) 34(21):5273–80. doi:10.1016/j.biomaterials.2013.03.050
131. Thomas RG, Moon MJ, Lee H, Sasikala AR, Kim CS, Park IK, et al. Hyaluronic acid conjugated superparamagnetic iron oxide nanoparticle for cancer diagnosis and hyperthermia therapy. Carbohydr Polym (2015) 131:439–46. doi:10.1016/j.carbpol.2015.06.010
132. Ravar F, Saadat E, Gholami M, Dehghankelishadi P, Mahdavi M, Azami S, et al. Hyaluronic acid-coated liposomes for targeted delivery of paclitaxel, in-vitro characterization and in-vivo evaluation. J Control Release (2016) 229:10–22. doi:10.1016/j.jconrel.2016.03.012
133. Yang RM, Fu CP, Li NN, Wang L, Xu XD, Yang DY, et al. Glycosaminoglycan-targeted iron oxide nanoparticles for magnetic resonance imaging of liver carcinoma. Mater Sci Eng C Mater Biol Appl (2014) 45:556–63. doi:10.1016/j.msec.2014.09.038
134. Song S, Qi H, Xu J, Guo P, Chen F, Li F, et al. Hyaluronan-based nanocarriers with CD44-overexpressed cancer cell targeting. Pharm Res (2014) 31(11):2988–3005. doi:10.1007/s11095-014-1393-4
135. Shiftan L, Israely T, Cohen M, Frydman V, Dafni H, Stern R, et al. Magnetic resonance imaging visualization of hyaluronidase in ovarian carcinoma. Cancer Res (2005) 65(22):10316–23. doi:10.1158/0008-5472.CAN-04-3947
136. Hou L, Yang X, Ren J, Wang Y, Zhang H, Feng Q, et al. A novel redox-sensitive system based on single-walled carbon nanotubes for chemo-photothermal therapy and magnetic resonance imaging. Int J Nanomedicine (2016) 11:607–24. doi:10.2147/IJN.S98476
137. Li J, Hu Y, Yang J, Wei P, Sun W, Shen M, et al. Hyaluronic acid-modified Fe3O4@Au core/shell nanostars for multimodal imaging and photothermal therapy of tumors. Biomaterials (2015) 38:10–21. doi:10.1016/j.biomaterials.2014.10.065
138. Jin Y, Ma X, Feng S, Liang X, Dai Z, Tian J, et al. Hyaluronic acid modified tantalum oxide nanoparticles conjugating doxorubicin for targeted cancer theranostics. Bioconjug Chem (2015) 26(12):2530–41. doi:10.1021/acs.bioconjchem.5b00551
139. Li W, Zheng C, Pan Z, Chen C, Hu D, Gao G, et al. Smart hyaluronidase-actived theranostic micelles for dual-modal imaging guided photodynamic therapy. Biomaterials (2016) 101:10–9. doi:10.1016/j.biomaterials.2016.05.019
140. Feng Q, Zhang Y, Zhang W, Shan X, Yuan Y, Zhang H, et al. Tumor-targeted and multi-stimuli responsive drug delivery system for near-infrared light induced chemo-phototherapy and photoacoustic tomography. Acta Biomater (2016) 38:129–42. doi:10.1016/j.actbio.2016.04.024
141. Uthaman S, Bom JS, Kim HS, John JV, Bom HS, Kim SJ, et al. Tumor homing indocyanine green encapsulated micelles for near infrared and photoacoustic imaging of tumors. J Biomed Mater Res B Appl Biomater (2016) 104(4):825–34. doi:10.1002/jbm.b.33607
142. Swierczewska M, Han HS, Kim K, Park JH, Lee S. Polysaccharide-based nanoparticles for theranostic nanomedicine. Adv Drug Deliv Rev (2016) 99(Pt A):70–84. doi:10.1016/j.addr.2015.11.015
143. Sollini M, Boni R, Traino AC, Lazzeri E, Pasqualetti F, Modeo L, et al. New approaches for imaging and therapy of solid cancer. Q J Nucl Med Mol Imaging (2015) 59(2):168–83.
144. Abou-Elkacem L, Wilson KE, Johnson SM, Chowdhury SM, Bachawal S, Hackel BJ, et al. Ultrasound molecular imaging of the breast cancer neovasculature using engineered fibronectin scaffold ligands: a novel class of targeted contrast ultrasound agent. Theranostics (2016) 6(11):1740–52. doi:10.7150/thno.15169
145. Givant-Horwitz V, Davidson B, Reich R. Laminin-induced signaling in tumor cells. Cancer Lett (2005) 223(1):1–10. doi:10.1016/j.canlet.2004.08.030
147. Patarroyo M, Tryggvason K, Virtanen I. Laminin isoforms in tumor invasion, angiogenesis and metastasis. Semin Cancer Biol (2002) 12(3):197–207. doi:10.1016/S1044-579X(02)00023-8
148. Fullar A, Dudas J, Olah L, Hollosi P, Papp Z, Sobel G, et al. Remodeling of extracellular matrix by normal and tumor-associated fibroblasts promotes cervical cancer progression. BMC Cancer (2015) 15:256. doi:10.1186/s12885-015-1272-3
149. Mazzocca A, Coppari R, De Franco R, Cho JY, Libermann TA, Pinzani M, et al. A secreted form of ADAM9 promotes carcinoma invasion through tumor-stromal interactions. Cancer Res (2005) 65(11):4728–38. doi:10.1158/0008-5472.CAN-04-4449
150. Moilanen JM, Kokkonen N, Loffek S, Vayrynen JP, Syvaniemi E, Hurskainen T, et al. Collagen XVII expression correlates with the invasion and metastasis of colorectal cancer. Hum Pathol (2015) 46(3):434–42. doi:10.1016/j.humpath.2014.11.020
151. Cuesta AM, Sanchez-Martin D, Sanz L, Bonet J, Compte M, Kremer L, et al. In vivo tumor targeting and imaging with engineered trivalent antibody fragments containing collagen-derived sequences. PLoS One (2009) 4(4):e5381. doi:10.1371/journal.pone.0005381
152. Koliakos G, Trontzos C, Kouzi-Koliakos K, Kanellaki M, Grammaticos P. Lung carcinoma imaging using a synthetic laminin derivative radioiodinated peptide YIGSR. J Nucl Med (1997) 38(12):1940–4.
153. Fujita M, Lee BS, Khazenzon NM, Penichet ML, Wawrowsky KA, Patil R, et al. Brain tumor tandem targeting using a combination of monoclonal antibodies attached to biopoly(beta-l-malic acid). J Control Release (2007) 122(3):356–63. doi:10.1016/j.jconrel.2007.05.032
154. Stelter L, Tseng JC, Torosjan A, Levin B, Longo VA, Pillarsetty N, et al. Tumor-specific targeting with modified Sindbis viral vectors: evaluation with optical imaging and positron emission tomography in vivo. Mol Imaging Biol (2013) 15(2):166–74. doi:10.1007/s11307-012-0585-8
155. Droujinine IA, Eckert MA, Zhao W. To grab the stroma by the horns: from biology to cancer therapy with mesenchymal stem cells. Oncotarget (2013) 4(5):651–64. doi:10.18632/oncotarget.1040
156. Madar S, Goldstein I, Rotter V. ‘Cancer associated fibroblasts’ – more than meets the eye. Trends Mol Med (2013) 19(8):447–53. doi:10.1016/j.molmed.2013.05.004
157. Xing F, Saidou J, Watabe K. Cancer associated fibroblasts (CAFs) in tumor microenvironment. Front Biosci (Landmark Ed) (2010) 15:166–79. doi:10.2741/3613
158. Shiga K, Hara M, Nagasaki T, Sato T, Takahashi H, Takeyama H. Cancer-associated fibroblasts: their characteristics and their roles in tumor growth. Cancers (Basel) (2015) 7(4):2443–58. doi:10.3390/cancers7040902
159. Grivennikov SI, Greten FR, Karin M. Immunity, inflammation, and cancer. Cell (2010) 140(6):883–99. doi:10.1016/j.cell.2010.01.025
160. Pollard JW. Tumour-educated macrophages promote tumour progression and metastasis. Nat Rev Cancer (2004) 4(1):71–8. doi:10.1038/nrc1256
161. Coussens LM, Zitvogel L, Palucka AK. Neutralizing tumor-promoting chronic inflammation: a magic bullet? Science (2013) 339(6117):286–91. doi:10.1126/science.1232227
162. Kidd S, Spaeth E, Dembinski JL, Dietrich M, Watson K, Klopp A, et al. Direct evidence of mesenchymal stem cell tropism for tumor and wounding microenvironments using in vivo bioluminescent imaging. Stem Cells (2009) 27(10):2614–23. doi:10.1002/stem.187
163. Klopp AH, Spaeth EL, Dembinski JL, Woodward WA, Munshi A, Meyn RE, et al. Tumor irradiation increases the recruitment of circulating mesenchymal stem cells into the tumor microenvironment. Cancer Res (2007) 67(24):11687–95. doi:10.1158/0008-5472.CAN-07-1406
164. Compte M, Cuesta AM, Sanchez-Martin D, Alonso-Camino V, Vicario JL, Sanz L, et al. Tumor immunotherapy using gene-modified human mesenchymal stem cells loaded into synthetic extracellular matrix scaffolds. Stem Cells (2009) 27(3):753–60. doi:10.1634/stemcells.2008-0831
165. Xiang J, Tang J, Song C, Yang Z, Hirst DG, Zheng QJ, et al. Mesenchymal stem cells as a gene therapy carrier for treatment of fibrosarcoma. Cytotherapy (2009) 11(5):516–26. doi:10.1080/14653240902960429
166. Kidd S, Caldwell L, Dietrich M, Samudio I, Spaeth EL, Watson K, et al. Mesenchymal stromal cells alone or expressing interferon-beta suppress pancreatic tumors in vivo, an effect countered by anti-inflammatory treatment. Cytotherapy (2010) 12(5):615–25. doi:10.3109/14653241003631815
167. Doucette T, Rao G, Yang Y, Gumin J, Shinojima N, Bekele BN, et al. Mesenchymal stem cells display tumor-specific tropism in an RCAS/Ntv-a glioma model. Neoplasia (2011) 13(8):716–25. doi:10.1593/neo.101680
168. Ke CC, Liu RS, Suetsugu A, Kimura H, Ho JH, Lee OK, et al. In vivo fluorescence imaging reveals the promotion of mammary tumorigenesis by mesenchymal stromal cells. PLoS One (2013) 8(7):e69658. doi:10.1371/journal.pone.0069658
169. Wu X, Hu J, Zhou L, Mao Y, Yang B, Gao L, et al. In vivo tracking of superparamagnetic iron oxide nanoparticle-labeled mesenchymal stem cell tropism to malignant gliomas using magnetic resonance imaging. Laboratory investigation. J Neurosurg (2008) 108(2):320–9. doi:10.3171/JNS/2008/108/2/0320
170. Huang X, Zhang F, Wang H, Niu G, Choi KY, Swierczewska M, et al. Mesenchymal stem cell-based cell engineering with multifunctional mesoporous silica nanoparticles for tumor delivery. Biomaterials (2013) 34(7):1772–80. doi:10.1016/j.biomaterials.2012.11.032
171. Belmar-Lopez C, Mendoza G, Oberg D, Burnet J, Simon C, Cervello I, et al. Tissue-derived mesenchymal stromal cells used as vehicles for anti-tumor therapy exert different in vivo effects on migration capacity and tumor growth. BMC Med (2013) 11:139. doi:10.1186/1741-7015-11-139
172. Hung SC, Deng WP, Yang WK, Liu RS, Lee CC, Su TC, et al. Mesenchymal stem cell targeting of microscopic tumors and tumor stroma development monitored by noninvasive in vivo positron emission tomography imaging. Clin Cancer Res (2005) 11(21):7749–56. doi:10.1158/1078-0432.CCR-05-0876
173. Knoop K, Kolokythas M, Klutz K, Willhauck MJ, Wunderlich N, Draganovici D, et al. Image-guided, tumor stroma-targeted 131I therapy of hepatocellular cancer after systemic mesenchymal stem cell-mediated NIS gene delivery. Mol Ther (2011) 19(9):1704–13. doi:10.1038/mt.2011.93
174. Dwyer RM, Ryan J, Havelin RJ, Morris JC, Miller BW, Liu Z, et al. Mesenchymal stem cell-mediated delivery of the sodium iodide symporter supports radionuclide imaging and treatment of breast cancer. Stem Cells (2011) 29(7):1149–57. doi:10.1002/stem.665
175. Shah K. Mesenchymal stem cells engineered for cancer therapy. Adv Drug Deliv Rev (2012) 64(8):739–48. doi:10.1016/j.addr.2011.06.010
176. Loebinger MR, Kyrtatos PG, Turmaine M, Price AN, Pankhurst Q, Lythgoe MF, et al. Magnetic resonance imaging of mesenchymal stem cells homing to pulmonary metastases using biocompatible magnetic nanoparticles. Cancer Res (2009) 69(23):8862–7. doi:10.1158/0008-5472.CAN-09-1912
177. Sasportas LS, Kasmieh R, Wakimoto H, Hingtgen S, van de Water JA, Mohapatra G, et al. Assessment of therapeutic efficacy and fate of engineered human mesenchymal stem cells for cancer therapy. Proc Natl Acad Sci U S A (2009) 106(12):4822–7. doi:10.1073/pnas.0806647106
178. Uchibori R, Okada T, Ito T, Urabe M, Mizukami H, Kume A, et al. Retroviral vector-producing mesenchymal stem cells for targeted suicide cancer gene therapy. J Gene Med (2009) 11(5):373–81. doi:10.1002/jgm.1313
179. Jia XH, Du Y, Mao D, Wang ZL, He ZQ, Qiu JD, et al. Zoledronic acid prevents the tumor-promoting effects of mesenchymal stem cells via MCP-1 dependent recruitment of macrophages. Oncotarget (2015) 6(28):26018–28. doi:10.18632/oncotarget.4658
180. Meleshina AV, Cherkasova EI, Shirmanova MV, Klementieva NV, Kiseleva EV, Snopova LB, et al. Influence of mesenchymal stem cells on metastasis development in mice in vivo. Stem Cell Res Ther (2015) 6:15. doi:10.1186/s13287-015-0003-7
181. Ozawa K, Sato K, Oh I, Ozaki K, Uchibori R, Obara Y, et al. Cell and gene therapy using mesenchymal stem cells (MSCs). J Autoimmun (2008) 30(3):121–7. doi:10.1016/j.jaut.2007.12.008
182. Caimi PF, Reese J, Lee Z, Lazarus HM. Emerging therapeutic approaches for multipotent mesenchymal stromal cells. Curr Opin Hematol (2010) 17(6):505–13. doi:10.1097/MOH.0b013e32833e5b18
183. Mader EK, Maeyama Y, Lin Y, Butler GW, Russell HM, Galanis E, et al. Mesenchymal stem cell carriers protect oncolytic measles viruses from antibody neutralization in an orthotopic ovarian cancer therapy model. Clin Cancer Res (2009) 15(23):7246–55. doi:10.1158/1078-0432.CCR-09-1292
184. Centeno CJ, Schultz JR, Cheever M, Robinson B, Freeman M, Marasco W. Safety and complications reporting on the re-implantation of culture-expanded mesenchymal stem cells using autologous platelet lysate technique. Curr Stem Cell Res Ther (2010) 5(1):81–93. doi:10.2174/157488810790442796
185. Tolar J, Nauta AJ, Osborn MJ, Panoskaltsis Mortari A, McElmurry RT, Bell S, et al. Sarcoma derived from cultured mesenchymal stem cells. Stem Cells (2007) 25(2):371–9. doi:10.1634/stemcells.2005-0620
186. Boddington SE, Sutton EJ, Henning TD, Nedopil AJ, Sennino B, Kim A, et al. Labeling human mesenchymal stem cells with fluorescent contrast agents: the biological impact. Mol Imaging Biol (2011) 13(1):3–9. doi:10.1007/s11307-010-0322-0
187. Myers JT, Petrosiute A, Huang AY. Utilization of multiphoton imaging for real-time fate determination of mesenchymal stem cells in an immunocompetent mouse model. J Stem Cell Res Ther (2014) 4(7):1000217. doi:10.4172/2157-7633.1000217
188. Orimo A, Weinberg RA. Heterogeneity of stromal fibroblasts in tumors. Cancer Biol Ther (2007) 6(4):618–9. doi:10.4161/cbt.6.4.4255
189. Liao D, Luo Y, Markowitz D, Xiang R, Reisfeld RA. Cancer associated fibroblasts promote tumor growth and metastasis by modulating the tumor immune microenvironment in a 4T1 murine breast cancer model. PLoS One (2009) 4(11):e7965. doi:10.1371/journal.pone.0007965
190. Hanley CJ, Noble F, Ward M, Bullock M, Drifka C, Mellone M, et al. A subset of myofibroblastic cancer-associated fibroblasts regulate collagen fiber elongation, which is prognostic in multiple cancers. Oncotarget (2016) 7(5):6159–74. doi:10.18632/oncotarget.6740
191. Koczorowska MM, Tholen S, Bucher F, Lutz L, Kizhakkedathu JN, De Wever O, et al. Fibroblast activation protein-alpha, a stromal cell surface protease, shapes key features of cancer associated fibroblasts through proteome and degradome alterations. Mol Oncol (2016) 10(1):40–58. doi:10.1016/j.molonc.2015.08.001
192. Brennen WN, Isaacs JT, Denmeade SR. Rationale behind targeting fibroblast activation protein-expressing carcinoma-associated fibroblasts as a novel chemotherapeutic strategy. Mol Cancer Ther (2012) 11(2):257–66. doi:10.1158/1535-7163.MCT-11-0340
193. Li J, Chen K, Liu H, Cheng K, Yang M, Zhang J, et al. Activatable near-infrared fluorescent probe for in vivo imaging of fibroblast activation protein-alpha. Bioconjug Chem (2012) 23(8):1704–11. doi:10.1021/bc300278r
194. Ruger R, Tansi FL, Rabenhold M, Steiniger F, Kontermann RE, Fahr A, et al. In vivo near-infrared fluorescence imaging of FAP-expressing tumors with activatable FAP-targeted, single-chain Fv-immunoliposomes. J Control Release (2014) 186:1–10. doi:10.1016/j.jconrel.2014.04.050
195. Granot D, Addadi Y, Kalchenko V, Harmelin A, Kunz-Schughart LA, Neeman M. In vivo imaging of the systemic recruitment of fibroblasts to the angiogenic rim of ovarian carcinoma tumors. Cancer Res (2007) 67(19):9180–9. doi:10.1158/0008-5472.CAN-07-0684
196. Granot D, Kunz-Schughart LA, Neeman M. Labeling fibroblasts with biotin-BSA-GdDTPA-FAM for tracking of tumor-associated stroma by fluorescence and MR imaging. Magn Reson Med (2005) 54(4):789–97. doi:10.1002/mrm.20628
197. Lo A, Wang LC, Scholler J, Monslow J, Avery D, Newick K, et al. Tumor-promoting desmoplasia is disrupted by depleting FAP-expressing stromal cells. Cancer Res (2015) 75(14):2800–10. doi:10.1158/0008-5472.CAN-14-3041
198. Juergens RA, Zukotynski KA, Singnurkar A, Snider DP, Valliant JF, Gulenchyn KY. Imaging biomarkers in immunotherapy. Biomark Cancer (2016) 8(Suppl 2):1–13. doi:10.4137/BIC.S31805
199. Freise AC, Wu AM. In vivo imaging with antibodies and engineered fragments. Mol Immunol (2015) 67(2 Pt A):142–52. doi:10.1016/j.molimm.2015.04.001
200. Weissleder R, Nahrendorf M, Pittet MJ. Imaging macrophages with nanoparticles. Nat Mater (2014) 13(2):125–38. doi:10.1038/nmat3780
201. Malviya G, Galli F, Sonni I, Signore A. Imaging T-lymphocytes in inflammatory diseases: a nuclear medicine approach. Q J Nucl Med Mol Imaging (2014) 58(3):237–57.
202. Aarntzen EH, Srinivas M, Radu CG, Punt CJ, Boerman OC, Figdor CG, et al. In vivo imaging of therapy-induced anti-cancer immune responses in humans. Cell Mol Life Sci (2013) 70(13):2237–57. doi:10.1007/s00018-012-1159-2
203. Ottobrini L, Martelli C, Trabattoni DL, Clerici M, Lucignani G. In vivo imaging of immune cell trafficking in cancer. Eur J Nucl Med Mol Imaging (2011) 38(5):949–68. doi:10.1007/s00259-010-1687-7
204. Dubey P. Reporter gene imaging of immune responses to cancer: progress and challenges. Theranostics (2012) 2(4):355–62. doi:10.7150/thno.3903
205. Singh AS, Radu CG, Ribas A. PET imaging of the immune system: immune monitoring at the whole body level. Q J Nucl Med Mol Imaging (2010) 54(3):281–90.
206. Jha P, Golovko D, Bains S, Hostetter D, Meier R, Wendland MF, et al. Monitoring of natural killer cell immunotherapy using noninvasive imaging modalities. Cancer Res (2010) 70(15):6109–13. doi:10.1158/0008-5472.CAN-09-3774
207. Lohela M, Werb Z. Intravital imaging of stromal cell dynamics in tumors. Curr Opin Genet Dev (2010) 20(1):72–8. doi:10.1016/j.gde.2009.10.011
208. Zal T, Chodaczek G. Intravital imaging of anti-tumor immune response and the tumor microenvironment. Semin Immunopathol (2010) 32(3):305–17. doi:10.1007/s00281-010-0217-9
209. Srinivas M, Aarntzen EH, Bulte JW, Oyen WJ, Heerschap A, de Vries IJ, et al. Imaging of cellular therapies. Adv Drug Deliv Rev (2010) 62(11):1080–93. doi:10.1016/j.addr.2010.08.009
210. Armulik A, Abramsson A, Betsholtz C. Endothelial/pericyte interactions. Circ Res (2005) 97(6):512–23. doi:10.1161/01.RES.0000182903.16652.d7
211. Kohlhapp FJ, Mitra AK, Lengyel E, Peter ME. MicroRNAs as mediators and communicators between cancer cells and the tumor microenvironment. Oncogene (2015) 34(48):5857–68. doi:10.1038/onc.2015.89
212. Armulik A, Genove G, Betsholtz C. Pericytes: developmental, physiological, and pathological perspectives, problems, and promises. Dev Cell (2011) 21(2):193–215. doi:10.1016/j.devcel.2011.07.001
213. Casazza A, Di Conza G, Wenes M, Finisguerra V, Deschoemaeker S, Mazzone M. Tumor stroma: a complexity dictated by the hypoxic tumor microenvironment. Oncogene (2014) 33(14):1743–54. doi:10.1038/onc.2013.121
214. Varlotto J, Stevenson MA. Anemia, tumor hypoxemia, and the cancer patient. Int J Radiat Oncol Biol Phys (2005) 63(1):25–36. doi:10.1016/j.ijrobp.2005.04.049
215. Vaupel P, Mayer A. Hypoxia in cancer: significance and impact on clinical outcome. Cancer Metastasis Rev (2007) 26(2):225–39. doi:10.1007/s10555-007-9055-1
216. Rafat M, Ali R, Graves EE. Imaging radiation response in tumor and normal tissue. Am J Nucl Med Mol Imaging (2015) 5(4):317–32.
217. de Langen AJ, van den Boogaart VE, Marcus JT, Lubberink M. Use of H2(15)O-PET and DCE-MRI to measure tumor blood flow. Oncologist (2008) 13(6):631–44. doi:10.1634/theoncologist.2007-0235
218. Willats L, Calamante F. The 39 steps: evading error and deciphering the secrets for accurate dynamic susceptibility contrast MRI. NMR Biomed (2013) 26(8):913–31. doi:10.1002/nbm.2833
219. Loveless ME, Halliday J, Liess C, Xu L, Dortch RD, Whisenant J, et al. A quantitative comparison of the influence of individual versus population-derived vascular input functions on dynamic contrast enhanced-MRI in small animals. Magn Reson Med (2012) 67(1):226–36. doi:10.1002/mrm.22988
220. Barnes SL, Whisenant JG, Loveless ME, Yankeelov TE. Practical dynamic contrast enhanced MRI in small animal models of cancer: data acquisition, data analysis, and interpretation. Pharmaceutics (2012) 4(3):442–78. doi:10.3390/pharmaceutics4030442
221. Koh TS, Bisdas S, Koh DM, Thng CH. Fundamentals of tracer kinetics for dynamic contrast-enhanced MRI. J Magn Reson Imaging (2011) 34(6):1262–76. doi:10.1002/jmri.22795
222. Calamante F. Perfusion MRI using dynamic-susceptibility contrast MRI: quantification issues in patient studies. Top Magn Reson Imaging (2010) 21(2):75–85. doi:10.1097/RMR.0b013e31821e53f5
223. Barrett T, Brechbiel M, Bernardo M, Choyke PL. MRI of tumor angiogenesis. J Magn Reson Imaging (2007) 26(2):235–49. doi:10.1002/jmri.20991
224. Le Bihan D, Poupon C, Amadon A, Lethimonnier F. Artifacts and pitfalls in diffusion MRI. J Magn Reson Imaging (2006) 24(3):478–88. doi:10.1002/jmri.20683
225. Barrett T, Kobayashi H, Brechbiel M, Choyke PL. Macromolecular MRI contrast agents for imaging tumor angiogenesis. Eur J Radiol (2006) 60(3):353–66. doi:10.1016/j.ejrad.2006.06.025
226. Neeman M, Gilad AA, Dafni H, Cohen B. Molecular imaging of angiogenesis. J Magn Reson Imaging (2007) 25(1):1–12. doi:10.1002/jmri.20774
227. Kidwell CS, Hsia AW. Imaging of the brain and cerebral vasculature in patients with suspected stroke: advantages and disadvantages of CT and MRI. Curr Neurol Neurosci Rep (2006) 6(1):9–16. doi:10.1007/s11910-996-0003-1
228. van Elmpt W, Das M, Hullner M, Sharifi H, Zegers CM, Reymen B, et al. Characterization of tumor heterogeneity using dynamic contrast enhanced CT and FDG-PET in non-small cell lung cancer. Radiother Oncol (2013) 109(1):65–70. doi:10.1016/j.radonc.2013.08.032
229. Cyran CC, Paprottka PM, Eisenblatter M, Clevert DA, Rist C, Nikolaou K, et al. Visualization, imaging and new preclinical diagnostics in radiation oncology. Radiat Oncol (2014) 9:3. doi:10.1186/1748-717X-9-3
230. Chen M, Wang WP, Jia WR, Tang L, Wang Y, Zhan WW, et al. Three-dimensional contrast-enhanced sonography in the assessment of breast tumor angiogenesis: correlation with microvessel density and vascular endothelial growth factor expression. J Ultrasound Med (2014) 33(5):835–46. doi:10.7863/ultra.33.5.835
231. Koehl GE, Gaumann A, Geissler EK. Intravital microscopy of tumor angiogenesis and regression in the dorsal skin fold chamber: mechanistic insights and preclinical testing of therapeutic strategies. Clin Exp Metastasis (2009) 26(4):329–44. doi:10.1007/s10585-008-9234-7
232. Detre JA, Zhang W, Roberts DA, Silva AC, Williams DS, Grandis DJ, et al. Tissue specific perfusion imaging using arterial spin labeling. NMR Biomed (1994) 7(1–2):75–82. doi:10.1002/nbm.1940070112
233. Le Bihan D, Breton E, Lallemand D, Aubin ML, Vignaud J, Laval-Jeantet M. Separation of diffusion and perfusion in intravoxel incoherent motion MR imaging. Radiology (1988) 168(2):497–505. doi:10.1148/radiology.168.2.3393671
234. Garcia-Figueiras R, Padhani AR, Baleato-Gonzalez S. Therapy monitoring with functional and molecular MR imaging. Magn Reson Imaging Clin N Am (2016) 24(1):261–88. doi:10.1016/j.mric.2015.08.003
235. Ingrisch M, Sourbron S. Tracer-kinetic modeling of dynamic contrast-enhanced MRI and CT: a primer. J Pharmacokinet Pharmacodyn (2013) 40(3):281–300. doi:10.1007/s10928-013-9315-3
236. Pathak AP, Gimi B, Glunde K, Ackerstaff E, Artemov D, Bhujwalla ZM. Molecular and functional imaging of cancer: advances in MRI and MRS. Methods Enzymol (2004) 386:3–60. doi:10.1016/S0076-6879(04)86001-4
237. Yang M, Li L, Jiang P, Moossa AR, Penman S, Hoffman RM. Dual-color fluorescence imaging distinguishes tumor cells from induced host angiogenic vessels and stromal cells. Proc Natl Acad Sci U S A (2003) 100(24):14259–62. doi:10.1073/pnas.2436101100
238. Geraldes CF, Laurent S. Classification and basic properties of contrast agents for magnetic resonance imaging. Contrast Media Mol Imaging (2009) 4(1):1–23. doi:10.1002/cmmi.265
239. Leach MO, Brindle KM, Evelhoch JL, Griffiths JR, Horsman MR, Jackson A, et al. The assessment of antiangiogenic and antivascular therapies in early-stage clinical trials using magnetic resonance imaging: issues and recommendations. Br J Cancer (2005) 92(9):1599–610. doi:10.1038/sj.bjc.6602550
240. Bradley DP, Tessier JJ, Ashton SE, Waterton JC, Wilson Z, Worthington PL, et al. Correlation of MRI biomarkers with tumor necrosis in Hras5 tumor xenograft in athymic rats. Neoplasia (2007) 9(5):382–91. doi:10.1593/neo.07145
241. Hsu YH, Ferl GZ, Ng CM. GPU-accelerated nonparametric kinetic analysis of DCE-MRI data from glioblastoma patients treated with bevacizumab. Magn Reson Imaging (2013) 31(4):618–23. doi:10.1016/j.mri.2012.09.007
242. Brix G, Lucht R, Griebel J. Tracer kinetic analysis of signal time series from dynamic contrast-enhanced MR imaging. Biomed Tech (Berl) (2006) 51(5–6):325–30. doi:10.1515/BMT.2006.065
243. Sourbron SP, Buckley DL. Tracer kinetic modelling in MRI: estimating perfusion and capillary permeability. Phys Med Biol (2012) 57(2):R1–33. doi:10.1088/0031-9155/57/2/R1
244. Sourbron SP, Buckley DL. Classic models for dynamic contrast-enhanced MRI. NMR Biomed (2013) 26(8):1004–27. doi:10.1002/nbm.2940
245. O’Connor JP, Rose CJ, Waterton JC, Carano RA, Parker GJ, Jackson A. Imaging intratumor heterogeneity: role in therapy response, resistance, and clinical outcome. Clin Cancer Res (2015) 21(2):249–57. doi:10.1158/1078-0432.CCR-14-0990
246. Stoyanova R, Huang K, Sandler K, Cho H, Carlin S, Zanzonico PB, et al. Mapping tumor hypoxia in vivo using pattern recognition of dynamic contrast-enhanced MRI data. Transl Oncol (2012) 5(6):437–47. doi:10.1593/tlo.12319
247. Fritz V, Noel D, Bouquet C, Opolon P, Voide R, Apparailly F, et al. Antitumoral activity and osteogenic potential of mesenchymal stem cells expressing the urokinase-type plasminogen antagonist amino-terminal fragment in a murine model of osteolytic tumor. Stem Cells (2008) 26(11):2981–90. doi:10.1634/stemcells.2008-0139
248. Koyasu S, Tsuji Y, Harada H, Nakamoto Y, Nobashi T, Kimura H, et al. Evaluation of tumor-associated stroma and its relationship with tumor hypoxia using dynamic contrast-enhanced CT and 18F misonidazole PET in murine tumor models. Radiology (2015) 278(3):734–41. doi:10.1148/radiol.2015150416
249. Mancini M, Greco A, Salvatore G, Liuzzi R, Di Maro G, Vergara E, et al. Imaging of thyroid tumor angiogenesis with microbubbles targeted to vascular endothelial growth factor receptor type 2 in mice. BMC Med Imaging (2013) 13:31. doi:10.1186/1471-2342-13-31
250. Yousefi S, Zhi Z, Wang RK. Label-free optical imaging of lymphatic vessels within tissue beds. IEEE J Sel Top Quantum Electron (2014) 20(2):6800510. doi:10.1109/JSTQE.2013.2278073
251. Mumprecht V, Honer M, Vigl B, Proulx ST, Trachsel E, Kaspar M, et al. In vivo imaging of inflammation- and tumor-induced lymph node lymphangiogenesis by immuno-positron emission tomography. Cancer Res (2010) 70(21):8842–51. doi:10.1158/0008-5472.CAN-10-0896
252. Bhang SH, Won N, Lee TJ, Jin H, Nam J, Park J, et al. Hyaluronic acid-quantum dot conjugates for in vivo lymphatic vessel imaging. ACS Nano (2009) 3(6):1389–98. doi:10.1021/nn900138d
253. Podo F, Canevari S, Canese R, Pisanu ME, Ricci A, Iorio E. MR evaluation of response to targeted treatment in cancer cells. NMR Biomed (2011) 24(6):648–72. doi:10.1002/nbm.1658
254. Challapalli A, Aboagye EO. Positron emission tomography imaging of tumor cell metabolism and application to therapy response monitoring. Front Oncol (2016) 6:44. doi:10.3389/fonc.2016.00044
255. Cuccurullo V, Di Stasio GD, Evangelista L, Castoria G, Mansi L. Biochemical and pathophysiological premises to positron emission tomography with choline radiotracers. J Cell Physiol (2017) 232(2):270–5. doi:10.1002/jcp.25478
256. Haberkorn U, Markert A, Mier W, Askoxylakis V, Altmann A. Molecular imaging of tumor metabolism and apoptosis. Oncogene (2011) 30(40):4141–51. doi:10.1038/onc.2011.169
257. Zhu A, Marcus DM, Shu HK, Shim H. Application of metabolic PET imaging in radiation oncology. Radiat Res (2012) 177(4):436–48. doi:10.1667/RR2702.1
258. Ljungkvist AS, Bussink J, Kaanders JH, van der Kogel AJ. Dynamics of tumor hypoxia measured with bioreductive hypoxic cell markers. Radiat Res (2007) 167(2):127–45. doi:10.1667/RR0719.1
259. Robinson SP, Griffiths JR. Current issues in the utility of 19F nuclear magnetic resonance methodologies for the assessment of tumour hypoxia. Philos Trans R Soc Lond B Biol Sci (2004) 359(1446):987–96. doi:10.1098/rstb.2003.1376
260. Tatum JL, Kelloff GJ, Gillies RJ, Arbeit JM, Brown JM, Chao KS, et al. Hypoxia: importance in tumor biology, noninvasive measurement by imaging, and value of its measurement in the management of cancer therapy. Int J Radiat Biol (2006) 82(10):699–757. doi:10.1080/09553000601002324
261. Thorwarth D, Eschmann SM, Paulsen F, Alber M. A kinetic model for dynamic [18F]-Fmiso PET data to analyse tumour hypoxia. Phys Med Biol (2005) 50(10):2209–24. doi:10.1088/0031-9155/50/10/002
262. Zanzonico P, O’Donoghue J, Chapman JD, Schneider R, Cai S, Larson S, et al. Iodine-124-labeled iodo-azomycin-galactoside imaging of tumor hypoxia in mice with serial microPET scanning. Eur J Nucl Med Mol Imaging (2004) 31(1):117–28. doi:10.1007/s00259-003-1322-y
263. Rajendran JG, Krohn KA. F-18 fluoromisonidazole for imaging tumor hypoxia: imaging the microenvironment for personalized cancer therapy. Semin Nucl Med (2015) 45(2):151–62. doi:10.1053/j.semnuclmed.2014.10.006
264. Ackerstaff E, Suehiro M, Kruchevsky N, Carlin S, Rosenfeld EH, Burgman P, et al., editors. Trifluoromisonidazole detects hypoxia – an in vivo and in vitro multimodality study. ISMRM 19th Annual Meeting & Exhibition. Montreal, Canada (2011).
265. Serganova I, Humm J, Ling C, Blasberg R. Tumor hypoxia imaging. Clin Cancer Res (2006) 12(18):5260–4. doi:10.1158/1078-0432.CCR-06-0517
266. Penet MF, Krishnamachary B, Chen Z, Jin J, Bhujwalla ZM. Molecular imaging of the tumor microenvironment for precision medicine and theranostics. Adv Cancer Res (2014) 124:235–56. doi:10.1016/B978-0-12-411638-2.00007-0
267. Gulliksrud K, Vestvik IK, Galappathi K, Mathiesen B, Rofstad EK. Detection of different hypoxic cell subpopulations in human melanoma xenografts by pimonidazole immunohistochemistry. Radiat Res (2008) 170(5):638–50. doi:10.1667/RR1400.1
268. White DA, Zhang Z, Li L, Gerberich J, Stojadinovic S, Peschke P, et al. Developing oxygen-enhanced magnetic resonance imaging as a prognostic biomarker of radiation response. Cancer Lett (2016) 380(1):69–77. doi:10.1016/j.canlet.2016.06.003
269. Zhao D, Pacheco-Torres J, Hallac RR, White D, Peschke P, Cerdan S, et al. Dynamic oxygen challenge evaluated by NMR T1 and T2* – insights into tumor oxygenation. NMR Biomed (2015) 28(8):937–47. doi:10.1002/nbm.3325
270. Hallac RR, Zhou H, Pidikiti R, Song K, Stojadinovic S, Zhao D, et al. Correlations of noninvasive BOLD and TOLD MRI with pO2 and relevance to tumor radiation response. Magn Reson Med (2014) 71(5):1863–73. doi:10.1002/mrm.24846
271. Mason RP, Zhao D, Pacheco-Torres J, Cui W, Kodibagkar VD, Gulaka PK, et al. Multimodality imaging of hypoxia in preclinical settings. Q J Nucl Med Mol Imaging (2010) 54(3):259–80.
272. Lau J, Zhang Z, Jenni S, Kuo HT, Liu Z, Vullo D, et al. PET imaging of carbonic anhydrase IX expression of HT-29 tumor xenograft mice with (68)Ga-labeled benzenesulfonamides. Mol Pharm (2016) 13(3):1137–46. doi:10.1021/acs.molpharmaceut.5b00934
273. Lv PC, Roy J, Putt KS, Low PS. Evaluation of a carbonic anhydrase IX-targeted near-infrared dye for fluorescence-guided surgery of hypoxic tumors. Mol Pharm (2016) 13(5):1618–25. doi:10.1021/acs.molpharmaceut.6b00065
274. Lv PC, Putt KS, Low PS. Evaluation of nonpeptidic ligand conjugates for SPECT imaging of hypoxic and carbonic anhydrase IX-expressing cancers. Bioconjug Chem (2016) 27(7):1762–9. doi:10.1021/acs.bioconjchem.6b00271
275. Di Gialleonardo V, Wilson DM, Keshari KR. The potential of metabolic imaging. Semin Nucl Med (2016) 46(1):28–39. doi:10.1053/j.semnuclmed.2015.09.004
276. Glunde K, Bhujwalla ZM. Metabolic tumor imaging using magnetic resonance spectroscopy. Semin Oncol (2011) 38(1):26–41. doi:10.1053/j.seminoncol.2010.11.001
277. Valette J, Tiret B, Boumezbeur F. Experimental strategies for in vivo 13C NMR spectroscopy. Anal Biochem (2016). doi:10.1016/j.ab.2016.08.003
278. Dafni H, Ronen SM. Dynamic nuclear polarization in metabolic imaging of metastasis: common sense, hypersense and compressed sensing. Cancer Biomark (2010) 7(4):189–99. doi:10.3233/CBM-2010-0185
279. Chen AP, Tropp J, Hurd RE, Van Criekinge M, Carvajal LG, Xu D, et al. In vivo hyperpolarized 13C MR spectroscopic imaging with 1H decoupling. J Magn Reson (2009) 197(1):100–6. doi:10.1016/j.jmr.2008.12.004
280. Hu S, Lustig M, Balakrishnan A, Larson PE, Bok R, Kurhanewicz J, et al. 3D compressed sensing for highly accelerated hyperpolarized (13)C MRSI with in vivo applications to transgenic mouse models of cancer. Magn Reson Med (2010) 63(2):312–21. doi:10.1002/mrm.22233
281. He Q, Xu RZ, Shkarin P, Pizzorno G, Lee-French CH, Rothman DL, et al. Magnetic resonance spectroscopic imaging of tumor metabolic markers for cancer diagnosis, metabolic phenotyping, and characterization of tumor microenvironment. Dis Markers (2003) 19(2–3):69–94. doi:10.1155/2004/424395
282. Hetherington HP, Avison MJ, Shulman RG. 1H homonuclear editing of rat brain using semiselective pulses. Proc Natl Acad Sci U S A (1985) 82(10):3115–8. doi:10.1073/pnas.82.10.3115
283. Lee SC, Huang MQ, Nelson DS, Pickup S, Wehrli S, Adegbola O, et al. In vivo MRS markers of response to CHOP chemotherapy in the WSU-DLCL2 human diffuse large B-cell lymphoma xenograft. NMR Biomed (2008) 21(7):723–33. doi:10.1002/nbm.1250
284. Lee SC, Poptani H, Pickup S, Jenkins WT, Kim S, Koch CJ, et al. Early detection of radiation therapy response in non-Hodgkin’s lymphoma xenografts by in vivo 1H magnetic resonance spectroscopy and imaging. NMR Biomed (2010) 23(6):624–32. doi:10.1002/nbm.1505
285. Muruganandham M, Koutcher JA, Pizzorno G, He Q. In vivo tumor lactate relaxation measurements by selective multiple-quantum-coherence (Sel-MQC) transfer. Magn Reson Med (2004) 52(4):902–6. doi:10.1002/mrm.20206
286. Del Vecchio S, Zannetti A, Iommelli F, Lettieri A, Brunetti A, Salvatore M. Molecular imaging of tumor microenvironment: challenges and perspectives. Q J Nucl Med Mol Imaging (2010) 54(3):249–58.
287. Gillies RJ, Liu Z, Bhujwalla Z. 31P-MRS measurements of extracellular pH of tumors using 3-aminopropylphosphonate. Am J Physiol (1994) 267(1 Pt 1):C195–203.
288. Raghunand N. Tissue pH measurement by magnetic resonance spectroscopy and imaging. Methods Mol Med (2006) 124:347–64. doi:10.1385/1-59745-010-3:347
289. van Sluis R, Bhujwalla ZM, Raghunand N, Ballesteros P, Alvarez J, Cerdan S, et al. In vivo imaging of extracellular pH using 1H MRSI. Magn Reson Med (1999) 41(4):743–50. doi:10.1002/(SICI)1522-2594(199904)41:4<743::AID-MRM13>3.3.CO;2-Q
290. Provent P, Benito M, Hiba B, Farion R, Lopez-Larrubia P, Ballesteros P, et al. Serial in vivo spectroscopic nuclear magnetic resonance imaging of lactate and extracellular pH in rat gliomas shows redistribution of protons away from sites of glycolysis. Cancer Res (2007) 67(16):7638–45. doi:10.1158/0008-5472.CAN-06-3459
291. Perez-Mayoral E, Negri V, Soler-Padros J, Cerdan S, Ballesteros P. Chemistry of paramagnetic and diamagnetic contrast agents for magnetic resonance imaging and spectroscopy pH responsive contrast agents. Eur J Radiol (2008) 67(3):453–8. doi:10.1016/j.ejrad.2008.02.048
292. Gallagher FA, Kettunen MI, Brindle KM. Imaging pH with hyperpolarized 13C. NMR Biomed (2011) 24(8):1006–15. doi:10.1002/nbm.1742
293. Gallagher FA, Kettunen MI, Day SE, Hu DE, Ardenkjaer-Larsen JH, Zandt R, et al. Magnetic resonance imaging of pH in vivo using hyperpolarized 13C-labelled bicarbonate. Nature (2008) 453(7197):940–3. doi:10.1038/nature07017
294. Gallagher FA, Sladen H, Kettunen MI, Serrao EM, Rodrigues TB, Wright A, et al. Carbonic anhydrase activity monitored in vivo by hyperpolarized 13C-magnetic resonance spectroscopy demonstrates its importance for pH regulation in tumors. Cancer Res (2015) 75(19):4109–18. doi:10.1158/0008-5472.CAN-15-0857
295. Moon BF, Jones KM, Chen LQ, Liu P, Randtke EA, Howison CM, et al. A comparison of iopromide and iopamidol, two acidoCEST MRI contrast media that measure tumor extracellular pH. Contrast Media Mol Imaging (2015) 10(6):446–55. doi:10.1002/cmmi.1647
296. Chen LQ, Randtke EA, Jones KM, Moon BF, Howison CM, Pagel MD. Evaluations of tumor acidosis within in vivo tumor models using parametric maps generated with acido CEST MRI. Mol Imaging Biol (2015) 17(4):488–96. doi:10.1007/s11307-014-0816-2
297. Randtke EA, Granados JC, Howison CM, Pagel MD, Cardenas-Rodriguez J. Multislice CEST MRI improves the spatial assessment of tumor pH. Magn Reson Med (2016). doi:10.1002/mrm.26348
298. Vavere AL, Biddlecombe GB, Spees WM, Garbow JR, Wijesinghe D, Andreev OA, et al. A novel technology for the imaging of acidic prostate tumors by positron emission tomography. Cancer Res (2009) 69(10):4510–6. doi:10.1158/0008-5472.CAN-08-3781
299. Demoin DW, Wyatt LC, Edwards KJ, Abdel-Atti D, Sarparanta M, Pourat J, et al. PET imaging of extracellular pH in tumors with (64)Cu- and (18)F-labeled pHLIP peptides: a structure-activity optimization study. Bioconjug Chem (2016) 27(9):2014–23. doi:10.1021/acs.bioconjchem.6b00306
300. Viola-Villegas NT, Carlin SD, Ackerstaff E, Sevak KK, Divilov V, Serganova I, et al. Understanding the pharmacological properties of a metabolic PET tracer in prostate cancer. Proc Natl Acad Sci U S A (2014) 111(20):7254–9. doi:10.1073/pnas.1405240111
301. Macholl S, Morrison MS, Iveson P, Arbo BE, Andreev OA, Reshetnyak YK, et al. In vivo pH imaging with (99m)Tc-pHLIP. Mol Imaging Biol (2012) 14(6):725–34. doi:10.1007/s11307-012-0549-z
302. Martin GR, Jain RK. Noninvasive measurement of interstitial pH profiles in normal and neoplastic tissue using fluorescence ratio imaging microscopy. Cancer Res (1994) 54(21):5670–4.
303. Schreml S, Meier RJ, Wolfbeis OS, Landthaler M, Szeimies RM, Babilas P. 2D luminescence imaging of pH in vivo. Proc Natl Acad Sci U S A (2011) 108(6):2432–7. doi:10.1073/pnas.1006945108
304. Wang L, Fan Z, Zhang J, Changyi Y, Huang C, Gu Y, et al. Evaluating tumor metastatic potential by imaging intratumoral acidosis via pH-activatable near-infrared fluorescent probe. Int J Cancer (2015) 136(4):E107–16. doi:10.1002/ijc.29153
305. Shirmanova MV, Druzhkova IN, Lukina MM, Matlashov ME, Belousov VV, Snopova LB, et al. Intracellular pH imaging in cancer cells in vitro and tumors in vivo using the new genetically encoded sensor SypHer2. Biochim Biophys Acta (2015) 1850(9):1905–11. doi:10.1016/j.bbagen.2015.05.001
306. Chen Q, Liu X, Chen J, Zeng J, Cheng Z, Liu Z. A self-assembled albumin-based nanoprobe for in vivo ratiometric photoacoustic pH imaging. Adv Mater (2015) 27(43):6820–7. doi:10.1002/adma.201503194
307. Song J, Kim J, Hwang S, Jeon M, Jeong S, Kim C, et al. “Smart” gold nanoparticles for photoacoustic imaging: an imaging contrast agent responsive to the cancer microenvironment and signal amplification via pH-induced aggregation. Chem Commun (Camb) (2016) 52(53):8287–90. doi:10.1039/c6cc03100e
308. Khramtsov VV, Grigor’ev IA, Foster MA, Lurie DJ, Nicholson I. Biological applications of spin pH probes. Cell Mol Biol (Noisy-le-grand) (2000) 46(8):1361–74.
309. Samouilov A, Efimova OV, Bobko AA, Sun Z, Petryakov S, Eubank TD, et al. In vivo proton-electron double-resonance imaging of extracellular tumor pH using an advanced nitroxide probe. Anal Chem (2014) 86(2):1045–52. doi:10.1021/ac402230h
310. Chen Y, Ai K, Liu J, Sun G, Yin Q, Lu L. Multifunctional envelope-type mesoporous silica nanoparticles for pH-responsive drug delivery and magnetic resonance imaging. Biomaterials (2015) 60:111–20. doi:10.1016/j.biomaterials.2015.05.003
311. Zhang S, Qian X, Zhang L, Peng W, Chen Y. Composition-property relationships in multifunctional hollow mesoporous carbon nanosystems for pH-responsive magnetic resonance imaging and on-demand drug release. Nanoscale (2015) 7(17):7632–43. doi:10.1039/c5nr00451a
312. Min KH, Min HS, Lee HJ, Park DJ, Yhee JY, Kim K, et al. pH-controlled gas-generating mineralized nanoparticles: a theranostic agent for ultrasound imaging and therapy of cancers. ACS Nano (2015) 9(1):134–45. doi:10.1021/nn506210a
313. Lv R, Yang P, He F, Gai S, Yang G, Dai Y, et al. An imaging-guided platform for synergistic photodynamic/photothermal/chemo-therapy with pH/temperature-responsive drug release. Biomaterials (2015) 63:115–27. doi:10.1016/j.biomaterials.2015.05.016
314. Yu Q, Liu Y, Cao C, Le F, Qin X, Sun D, et al. The use of pH-sensitive functional selenium nanoparticles shows enhanced in vivo VEGF-siRNA silencing and fluorescence imaging. Nanoscale (2014) 6(15):9279–92. doi:10.1039/c4nr02423k
315. Bougnaud S, Golebiewska A, Oudin A, Keunen O, Harter PN, Mader L, et al. Molecular crosstalk between tumour and brain parenchyma instructs histopathological features in glioblastoma. Oncotarget (2016) 7(22):31955–71. doi:10.18632/oncotarget.7454
316. Ungefroren H, Sebens S, Seidl D, Lehnert H, Hass R. Interaction of tumor cells with the microenvironment. Cell Commun Signal (2011) 9:18. doi:10.1186/1478-811X-9-18
317. Langley RR, Fidler IJ. The seed and soil hypothesis revisited – the role of tumor-stroma interactions in metastasis to different organs. Int J Cancer (2011) 128(11):2527–35. doi:10.1002/ijc.26031
318. Witz IP. Tumor-microenvironment interactions: dangerous liaisons. Adv Cancer Res (2008) 100:203–29. doi:10.1016/S0065-230X(08)00007-9
319. Pietras K, Ostman A. Hallmarks of cancer: interactions with the tumor stroma. Exp Cell Res (2010) 316(8):1324–31. doi:10.1016/j.yexcr.2010.02.045
320. McMillin DW, Negri JM, Mitsiades CS. The role of tumour-stromal interactions in modifying drug response: challenges and opportunities. Nat Rev Drug Discov (2013) 12(3):217–28. doi:10.1038/nrd3870
321. Bhowmick NA, Moses HL. Tumor-stroma interactions. Curr Opin Genet Dev (2005) 15(1):97–101. doi:10.1016/j.gde.2004.12.003
322. Bruno S, Collino F, Iavello A, Camussi G. Effects of mesenchymal stromal cell-derived extracellular vesicles on tumor growth. Front Immunol (2014) 5:382. doi:10.3389/fimmu.2014.00382
323. Lee J, Condello S, Yakubov B, Emerson R, Caperell-Grant A, Hitomi K, et al. Tissue transglutaminase mediated tumor-stroma interaction promotes pancreatic cancer progression. Clin Cancer Res (2015) 21(19):4482–93. doi:10.1158/1078-0432.CCR-15-0226
324. Gangadhara S, Barrett-Lee P, Nicholson RI, Hiscox S. Pro-metastatic tumor-stroma interactions in breast cancer. Future Oncol (2012) 8(11):1427–42. doi:10.2217/fon.12.134
325. Luga V, Wrana JL. Tumor-stroma interaction: revealing fibroblast-secreted exosomes as potent regulators of Wnt-planar cell polarity signaling in cancer metastasis. Cancer Res (2013) 73(23):6843–7. doi:10.1158/0008-5472.CAN-13-1791
326. Zhao H, Yang L, Baddour J, Achreja A, Bernard V, Moss T, et al. Tumor microenvironment derived exosomes pleiotropically modulate cancer cell metabolism. Elife (2016) 5:e10250. doi:10.7554/eLife.10250
327. Holton SE, Bergamaschi A, Katzenellenbogen BS, Bhargava R. Integration of molecular profiling and chemical imaging to elucidate fibroblast-microenvironment impact on cancer cell phenotype and endocrine resistance in breast cancer. PLoS One (2014) 9(5):e96878. doi:10.1371/journal.pone.0096878
328. Ackerstaff E, Artemov D, Gillies RJ, Bhujwalla ZM. Hypoxia and the presence of human vascular endothelial cells affect prostate cancer cell invasion and metabolism. Neoplasia (2007) 9(12):1138–51. doi:10.1593/neo.07568
329. Gillies RJ, Galons JP, McGovern KA, Scherer PG, Lien YH, Job C, et al. Design and application of NMR-compatible bioreactor circuits for extended perfusion of high-density mammalian cell cultures. NMR Biomed (1993) 6(1):95–104. doi:10.1002/nbm.1940060115
330. Peng X, Recchia FA, Byrne BJ, Wittstein IS, Ziegelstein RC, Kass DA. In vitro system to study realistic pulsatile flow and stretch signaling in cultured vascular cells. Am J Physiol Cell Physiol (2000) 279(3):C797–805.
331. Casbas-Hernandez P, Fleming JM, Troester MA. Gene expression analysis of in vitro cocultures to study interactions between breast epithelium and stroma. J Biomed Biotechnol (2011) 2011:520987. doi:10.1155/2011/520987
332. Angelucci C, Maulucci G, Lama G, Proietti G, Colabianchi A, Papi M, et al. Epithelial-stromal interactions in human breast cancer: effects on adhesion, plasma membrane fluidity and migration speed and directness. PLoS One (2012) 7(12):e50804. doi:10.1371/journal.pone.0050804
333. Li H, Zhang J, Chen SW, Liu LL, Li L, Gao F, et al. Cancer-associated fibroblasts provide a suitable microenvironment for tumor development and progression in oral tongue squamous cancer. J Transl Med (2015) 13:198. doi:10.1186/s12967-015-0551-8
334. Icard P, Kafara P, Steyaert JM, Schwartz L, Lincet H. The metabolic cooperation between cells in solid cancer tumors. Biochim Biophys Acta (2014) 1846(1):216–25. doi:10.1016/j.bbcan.2014.06.002
335. Hagemann T, Robinson SC, Schulz M, Trumper L, Balkwill FR, Binder C. Enhanced invasiveness of breast cancer cell lines upon co-cultivation with macrophages is due to TNF-alpha dependent up-regulation of matrix metalloproteases. Carcinogenesis (2004) 25(8):1543–9. doi:10.1093/carcin/bgh146
336. Giatromanolaki A, Koukourakis MI, Koutsopoulos A, Mendrinos S, Sivridis E. The metabolic interactions between tumor cells and tumor-associated stroma (TAS) in prostatic cancer. Cancer Biol Ther (2012) 13(13):1284–9. doi:10.4161/cbt.21785
337. Morandi A, Chiarugi P. Metabolic implication of tumor:stroma crosstalk in breast cancer. J Mol Med (Berl) (2014) 92(2):117–26. doi:10.1007/s00109-014-1124-7
338. Choi J, Kim DH, Jung WH, Koo JS. Metabolic interaction between cancer cells and stromal cells according to breast cancer molecular subtype. Breast Cancer Res (2013) 15(5):R78. doi:10.1186/bcr3472
339. Guldner IH, Zhang S. A journey to uncharted territory: new technical frontiers in studying tumor-stromal cell interactions. Integr Biol (Camb) (2015) 7(2):153–61. doi:10.1039/c4ib00192c
340. Widder M, Lutzkendorf J, Caysa H, Unverzagt S, Wickenhauser C, Benndorf RA, et al. Multipotent mesenchymal stromal cells promote tumor growth in distinct colorectal cancer cells by a beta1-integrin-dependent mechanism. Int J Cancer (2015) 138(4):964–75. doi:10.1002/ijc.29844
341. Orimo A, Gupta PB, Sgroi DC, Arenzana-Seisdedos F, Delaunay T, Naeem R, et al. Stromal fibroblasts present in invasive human breast carcinomas promote tumor growth and angiogenesis through elevated SDF-1/CXCL12 secretion. Cell (2005) 121(3):335–48. doi:10.1016/j.cell.2005.02.034
342. Olumi AF, Grossfeld GD, Hayward SW, Carroll PR, Tlsty TD, Cunha GR. Carcinoma-associated fibroblasts direct tumor progression of initiated human prostatic epithelium. Cancer Res (1999) 59(19):5002–11. doi:10.1371/journal.pone.0068923
343. Subramaniam KS, Omar IS, Kwong SC, Mohamed Z, Woo YL, Mat Adenan NA, et al. Cancer-associated fibroblasts promote endometrial cancer growth via activation of interleukin-6/STAT-3/c-Myc pathway. Am J Cancer Res (2016) 6(2):200–13.
344. Domanska UM, Timmer-Bosscha H, Nagengast WB, Oude Munnink TH, Kruizinga RC, Ananias HJ, et al. CXCR4 inhibition with AMD3100 sensitizes prostate cancer to docetaxel chemotherapy. Neoplasia (2012) 14(8):709–18. doi:10.1593/neo.12324
345. McMillin DW, Delmore J, Weisberg E, Negri JM, Geer DC, Klippel S, et al. Tumor cell-specific bioluminescence platform to identify stroma-induced changes to anticancer drug activity. Nat Med (2010) 16(4):483–9. doi:10.1038/nm.2112
346. Sebens S, Schafer H. The tumor stroma as mediator of drug resistance – a potential target to improve cancer therapy? Curr Pharm Biotechnol (2012) 13(11):2259–72. doi:10.2174/138920112802501999
347. Maeda A, Kulbatski I, DaCosta RS. Emerging applications for optically enabled intravital microscopic imaging in radiobiology. Mol Imaging (2015) 14(9):452–74.
348. Hoffman RM. Imaging tumor angiogenesis with fluorescent proteins. APMIS (2004) 112(7–8):441–9. doi:10.1111/j.1600-0463.2004.apm11207-0806.x
349. Yang M, Jiang P, Hoffman RM. Whole-body subcellular multicolor imaging of tumor-host interaction and drug response in real time. Cancer Res (2007) 67(11):5195–200. doi:10.1158/0008-5472.CAN-06-4590
350. Wyckoff J, Wang W, Lin EY, Wang Y, Pixley F, Stanley ER, et al. A paracrine loop between tumor cells and macrophages is required for tumor cell migration in mammary tumors. Cancer Res (2004) 64(19):7022–9. doi:10.1158/0008-5472.CAN-04-1449
351. Dovas A, Patsialou A, Harney AS, Condeelis J, Cox D. Imaging interactions between macrophages and tumour cells that are involved in metastasis in vivo and in vitro. J Microsc (2013) 251(3):261–9. doi:10.1111/j.1365-2818.2012.03667.x
352. Perentes JY, McKee TD, Ley CD, Mathiew H, Dawson M, Padera TP, et al. In vivo imaging of extracellular matrix remodeling by tumor-associated fibroblasts. Nat Methods (2009) 6(2):143–5. doi:10.1038/nmeth.1295
353. Nakasone ES, Askautrud HA, Kees T, Park JH, Plaks V, Ewald AJ, et al. Imaging tumor-stroma interactions during chemotherapy reveals contributions of the microenvironment to resistance. Cancer Cell (2012) 21(4):488–503. doi:10.1016/j.ccr.2012.02.017
354. Nakasone ES, Askautrud HA, Egeblad M. Live imaging of drug responses in the tumor microenvironment in mouse models of breast cancer. J Vis Exp (2013) 73:e50088. doi:10.3791/50088
355. Ewald AJ, Werb Z, Egeblad M. Dynamic, long-term in vivo imaging of tumor-stroma interactions in mouse models of breast cancer using spinning-disk confocal microscopy. Cold Spring Harb Protoc (2011) 2011(2):db.to97. doi:10.1101/pdb.top97
356. Cherry SR. The 2006 Henry N. Wagner lecture: of mice and men (and positrons) – advances in PET imaging technology. J Nucl Med (2006) 47(11):1735–45.
357. Judenhofer MS, Catana C, Swann BK, Siegel SB, Jung WI, Nutt RE, et al. PET/MR images acquired with a compact MR-compatible PET detector in a 7-T magnet. Radiology (2007) 244(3):807–14. doi:10.1148/radiol.2443061756
358. Catana C, Wu Y, Judenhofer MS, Qi J, Pichler BJ, Cherry SR. Simultaneous acquisition of multislice PET and MR images: initial results with a MR-compatible PET scanner. J Nucl Med (2006) 47(12):1968–76.
359. O’Halloran PJ, Viel T, Murray DW, Wachsmuth L, Schwegmann K, Wagner S, et al. Mechanistic interrogation of combination bevacizumab/dual PI3K/mTOR inhibitor response in glioblastoma implementing novel MR and PET imaging biomarkers. Eur J Nucl Med Mol Imaging (2016) 43(9):1673–83. doi:10.1007/s00259-016-3343-3
Keywords: cancer, microenvironment, stroma, metabolic cooperation, tumor–stroma interaction, preclinical multimodal imaging
Citation: Ramamonjisoa N and Ackerstaff E (2017) Characterization of the Tumor Microenvironment and Tumor–Stroma Interaction by Non-invasive Preclinical Imaging. Front. Oncol. 7:3. doi: 10.3389/fonc.2017.00003
Received: 21 October 2016; Accepted: 05 January 2017;
Published: 31 January 2017
Edited by:
Franca Podo, Istituto Superiore di Sanità, ItalyReviewed by:
Piotr Kozlowski, University of British Columbia, CanadaCopyright: © 2017 Ramamonjisoa and Ackerstaff. This is an open-access article distributed under the terms of the Creative Commons Attribution License (CC BY). The use, distribution or reproduction in other forums is permitted, provided the original author(s) or licensor are credited and that the original publication in this journal is cited, in accordance with accepted academic practice. No use, distribution or reproduction is permitted which does not comply with these terms.
*Correspondence: Ellen Ackerstaff, YWNrZXJzdGVAbXNrY2Mub3Jn
Disclaimer: All claims expressed in this article are solely those of the authors and do not necessarily represent those of their affiliated organizations, or those of the publisher, the editors and the reviewers. Any product that may be evaluated in this article or claim that may be made by its manufacturer is not guaranteed or endorsed by the publisher.
Research integrity at Frontiers
Learn more about the work of our research integrity team to safeguard the quality of each article we publish.