- 1Laboratory of Cell Biology, Center for Cancer Research, National Cancer Institute, National Institutes of Health, Bethesda, MD, USA
- 2Nano System Institute, Seoul National University, Seoul, South Korea
Advances in cancer research have shown that a tumor can be likened to a foreign species that disrupts delicately balanced ecological interactions, compromising the survival of normal tissue ecosystems. In efforts to mitigate tumor expansion and metastasis, experimental approaches from ecology are becoming more frequently and successfully applied by researchers from diverse disciplines to reverse engineer and re-engineer biological systems in order to normalize the tumor ecosystem. We present a review on the use of 3D biomimetic platforms to recapitulate biotic and abiotic components of the tumor ecosystem, in efforts to delineate the underlying mechanisms that drive evolution of tumor heterogeneity, tumor dissemination, and acquisition of drug resistance.
Introduction
Patient survival following the diagnosis of certain types of cancers has significantly improved due to our current understanding of the molecular basis of tumor etiology (1–4). Tissue biopsies remain the first line of diagnosis for solid cancers (5). Histopathology provides physicians with local genetic and epigenetic information that guides the choice of an appropriate therapeutic regimen (5). Unfortunately, this initial intervention against a single molecular pathway or a specific mutant type may not be an effective way to treat the tumor as a whole or emerging phenotypes. Delivering targeted therapy that is consistently effective as the tumor evolves remains challenging (6, 7). One limitation may be due to the fact that a biopsy may not be representative of the larger tumor mass or is not predictive of a distant lesion in the event of metastatic disease. The other is that treatment in itself may select for tumor cells harboring less than desirable traits that represented a small fraction of cells in the original tumor (6, 7). Intratumoral heterogeneity is thought to allow for rapid adaptation to external stress, selecting for emergent phenotypes that can adapt to the foreign environments of distal organs and are drug-resistant (8). Regrettably, intratumoral heterogeneity persists after tumor cells have left the primary site and disseminated to establish metastatic lesions. Although many disseminated tumor cells (DTCs) circulate within the bloodstream, only a small subset of these will actually form a lesion. Ostensibly, many do not survive environmental pressures experienced during hematogenous or lymphatic spread (9). A subset of those that establish metastatic lesions may remain dormant for periods of time ranging from several months to decades (9) These quiescent DTCs may then awaken due to perturbations of the microenvironment (10–12) leading to tumor recurrence with inter- and intra-tumoral heterogeneity, largely resistant to drug intervention. Therefore, selecting for cells that either do not survive after dissemination or remain quiescent are possible areas for therapeutic intervention to prevent metastasis and increase treatment efficacy (9, 13, 14).
For some hematological cancers, such as acute myeloid leukemia, cancer stem cells (CSCs) or tumor-initiating cells are thought to drive the observed heterogeneities (15, 16). CSCs employ mechanisms such as self-renewal and differentiation into multiple tumor cell types to drive tumor growth, causing relapse, metastasis, and sometimes even recovery following therapeutic intervention (15, 17). Thus, CSCs increase the adaptive capability as they drive the evolution of distinct cancer cell populations to shape the tumor ecosystem. In such cases, targeting and removing the CSC population may sufficiently suppress the evolution of phenotypic and genotypic heterogeneities. Cairns and Nowell provided conceptual models that allow these observed tumor heterogeneities to be described by Darwinian principles (18, 19).
However, in most solid cancers, the evolution of heterogeneity is largely stochastic (20). The resultant genomic instability (21) and epigenetic changes are driven by heterotypic cell interactions within a dynamic extracellular milieu in response to external perturbations such as changes in oxygen tension, stiffness, or nutrient, and pH gradients (22). Diversity arises due to proliferation of sub-clones that survive selective pressures and environmental perturbations such as hypoxia, drug treatment, and reduced nutrients and evolve to increase phenotypic and functional heterogeneity within the tumor (22–24). Simply put, intratumoral heterogeneity does not merely develop from a random collection of mutated cells with uniform levels of proliferation (25). It also stems from interactions with the biotic (biochemical) and abiotic (physical) components of the environment. Drawing from lessons learned in the field of ecology, genetically reduced populations are more vulnerable to disease outbreaks, other environmental stresses (26), and the accumulation of deleterious mutations (27). So how do we design a therapy to reduce tumor heterogeneity?
The microenvironment is emerging as a critical factor in malignant progression, metastasis, and tumor etiology (20, 28, 29). The physical properties of the microenvironment, including the stiffness, dimension, and topography, work in concert with biochemical signals to have profound impacts on cell fate, tissue assembly, and malignancy (30, 31). A dynamic tumor microenvironment may not only contribute to systemic metastasis, but also significantly modify drug efficacy (31–33). These dynamic, bi-directional interactions between individual tumor cells and collectively with other cell types and with the extracellular matrix (ECM) milieu can be likened to a dynamic ecosystem (22, 34–36). In nature, an introduced foreign species or an aberrant member may disrupt the delicately balanced interactions that normally exist in an ecosystem between members of a species, predator and prey, animals and plants, or between life and the abiotic environment, compromising survival of other components, and perhaps even leading to the collapse of the entire system. Similarly, tumor cells detrimentally transform the balanced interactions within the surrounding normal tissue (e.g., between clonal subpopulations, immune cells and their targets, epithelial and stromal cells, or between cells and the extracellular microenvironment) to achieve and maintain tumor homeostasis (34, 35). Cancer can be likened to a disease state in which a new species evolves, eventually dominating the original organ. In many ways, interactions between tumor cells and their microenvironment mimic those observed during normal organogenesis. The concept of the tumor as an abnormal organ has been rigorously reviewed elsewhere (34, 35). Here, we build on this concept to understand the tumor dynamics of the transition from normal to malignant, from malignant to metastatic, and the meta-states that arise following treatment, in terms of the interactions between tumor cells and their microenvironment. One approach may be to model the evolutionary response of the tumor from an ecological perspective, so that a treatment would mitigate or undermine tumor heterogeneity. But first, we must understand the creation and evolution of the tumor microenvironment.
A recent review by Pienta and colleagues presents the argument for ecological therapy and consideration of the microenvironment as a possible approach to designing novel cancer therapeutics (37). An additional advantage of an ecological approach is the potential to exploit the existing mathematical and computational framework based on Darwinian dynamics that model the tumor heterogeneities in the context of microenvironmental regulation (20, 38). Increased efficacy of disease management may be achieved by combining treatments such as surgery, chemotherapy, or radiotherapy with approaches that are capable of reducing tumor diversity and “healing” the microenvironment (31, 39). Therefore, deciphering the underlying mechanisms that govern the establishment of the physico-chemical properties of the tumor-promoting niche is desirable. Continuing with our analogies drawn from the field of ecology, the concept of ecosystem engineering is the process whereby organisms modify (directly or indirectly) resources available to other species by causing physical state changes in biotic and abiotic components (40). Consequently, habitats are modified, synthesized, or maintained. Ecosystem engineers change environments by altering their physical structures (40). One example of ecosystem engineering, also referred to as niche construction (41), is dam creation by beavers (40). They physically remodel and destroy wooded areas, with profound effects on both soil drainage and water allocation (40). Another example is how earthworms chemically and physically modify soil as they burrow (40). Similarly, tumor cells work in concert with stromal cells to physically remodel and chemically define their environment, which then drives further tumor phenotypic evolution (42). So how do we recapitulate tumor diversification and the dynamics of physico-chemical microenvironment in vitro?
Three-dimensional (3D) culture systems in which extracellular matrices that biochemically and physically mimic the in vivo composition of organs have been extensively used to study normal epithelial organogenesis for the breast, prostate, salivary gland, and kidney (43–45). In addition, pharmaceutical studies involving endothelial cell-derived 3D spheroids have been extensively used for evaluating the pro- and anti-angiogenic potential of drugs targeting the tumor vasculature (46). In these biomimetic systems, cells adopt physiological morphologies and the appropriate cell signaling is achieved. 3D culture models can also be used to recapitulate the abiotic and biotic components of the tumor ecosystem. Namely, the abiotic components such as the spatio-temporal gradients of chemicals, oxygen tension, and mechanical cues can be robustly engineered using applications from microfluidics, electrospinning, and soft lithography (47, 48). The biotic components such as the ECM environment and heterotypic cell interactions can also be approximated using biomimetic platforms and co-culture systems. Although the conventional two-dimensional (2D) tissue culture system has contributed enormously to the progress of cancer biology, cancer cells encounter diverse 3D topographies and architectures in vivo. Thus, 3D culture models allow for deconstructing the complexity of cancer by recapitulating emergent, population-level characteristics of the tumor microenvironment.
Tumor Cells as Ecosystem Engineers – Defining the Tumor ECM Niche
A hallmark of malignant transformation is the loss of anatomical organization (49, 50). Tumor cells aberrantly proliferate, remodel, and rebuild a new microenvironment by releasing extracellular signaling molecules that promote tumor angiogenesis and ECM remodeling (34, 35, 42, 51). In a seminal paper, Dvorak postulated that tumors were simply “wounds that did not heal.” He reasoned that common cellular and molecular mechanisms are activated in wounds and cancerous tissue based on the observation that tumor stroma bore a strong similarity to the observed granulation tissue in skin wounds (52). However, the direct link between wound repair, chronic inflammation, and cancer was observed in later experiments where tumors were induced by the Rous sarcoma virus (a potent uncovers) only at the site of injections in newly hatched chickens but not in ovo (53). Wounding in an infected animal at the site of injury induced additional tumors away from the site of injection (53). Treatment with anti-inflammatory therapy prevented tumor formation, thus showing the effect of inflammation on tumorigenesis (53, 54). These observations highlight the importance of dynamic interplay between the tumor and the inflammatory microenvironment and have been reviewed extensively elsewhere (55–57). Here, we focus specifically on the ECM milieu in niche construction. The chemistry and physical properties of the ECM is dynamically tuned during de novo remodeling of the tumor microenvironment (42, 58). An overabundance of diverse ECM proteins and ECM remodeling enzymes is found in solid cancers (42, 51, 59–62). In addition to these chemical changes, physical properties of the tumors are altered. For example, tumors are often stiffer to the touch than the adjacent normal tissue (30, 63). In breast cancer, the fibrillar architecture of type I collagen surrounding the tumor is highly linearized as compared to normal tissue, which is thought to facilitate invasion into neighboring tissue (63, 64). These changes in ECM composition and architecture potentiate tumor-promoting changes in various signaling pathways (42). Specifically, perturbations in ECM synthesis, degradation, density, and rigidity promote cancer cell proliferation, migration, and invasion, and modulate inflammatory responses and lymphangiogenesis (35). The resulting abnormal microenvironment can exert selective pressure on cancer cell populations, increasing genomic instability and population diversity (42, 65).
Ecosystem Engineering during Metastatic Colonization – Defining the Metastatic ECM Niche
Disseminated tumor cells leave the original tumor to initiate the metastatic cascade (66). After successful navigation of the circulatory system, a subset of these DTCs then exits via capillaries at a distant site and infiltrates the tissue (66). These DTCs colonize their new environment by poorly understood mechanisms involving adherence, remodeling, and proliferation. The term “colonization” here defines the establishment of the tumor niche, net tumor cell proliferation, and angiogenesis in the formation of a metastatic lesion. In 1889, Paget hypothesized that the interaction between the tumor cells, the “seeds” and the host environment, the “soil,” determines metastatic outcome (67). This hypothesis predicted that the tissue-specific biological and biochemical conditions (defined by the resident cell populations, extracellular matrices, and vasculature) might selectively facilitate tumor metastasis, explaining the organ selectivity of certain metastatic cancers. It is now well appreciated that the continuous dynamic and reciprocal relationship between cells and their microenvironment in which the mechanical properties of tissue including the geometry, topography, and elasticity of the ECM can provide intrinsic signals to cells that have profound effects on cell physiology (30, 42, 68, 69). Hence, we include the caveat that the tumor cell “seeds” are motile and actively remodel the microenvironment “soil” in concert with stromal and immune cells to continue to “fertilize” the soil by secreting and assembling ECM components and other cytokines, altering both the physical and chemical properties of the tissue to successfully colonize organs.
In ecology, restoring the niche of a specific species has been a successful way to increase the population; thus, restoring the environment to that of normal tissue may be a good therapeutic strategy (37). We may exploit a specific signaling pathway, localize key proteins, or transplant cells that can reconstruct a niche to help the reconstruction of a normal microenvironment and suppress the activation of DTCs. Restoring the niche can normalize malignancy so that cancer cells will either enter quiescence or become phenotypically normal (31, 70–76). Specifically, teratocarcinoma cells revert to a normal phenotype when implanted into a normal mouse blastocyst (77). Additionally, modifying ECM inputs in a 3D hydrogel culture can normalize genotypically malignant cells (75, 78). Similar observations have been made in plant tumors, where crown-gall teratoma cells can generate normal shoots following grafting and successive healthy tobacco plants (79). Targeting the abnormal microenvironment or restoring or engineering the microenvironment could successfully supplement current cancer therapies by suppressing the development of malignant phenotypes or even reversing tumorigenesis (12, 76, 80).
Engineering the Biotic Microenvironment – Defining the ECM Niche
Three-dimensional (3D) biomimetic platforms allow for recapitulation of features of tissue architecture such as 3D cell–cell interactions, ECM composition and architecture, achieving in vivo- like mechanical properties and the diffusion profiles of signaling molecules (81–83). The simplest 3D culture model consists of single cancer cells or spheroids embedded in a natural matrix or cell-derived matrices of one ECM protein or hybrid of many proteins such as collagen type I, laminin-derived hydrogels, or alginate (44, 45, 84–86). Matrix porosity, fiber structure, and stiffness may be tuned by controlling polymerization conditions (85, 87, 88). Within such 3D models, cells rapidly proliferate and form spheroids or aggregates that recapitulate in vivo-observed tumor ECM formation, tumor cell–cell interactions, tumor-like molecular diffusion gradients, chemoresistance, and invasive metastasis characteristic of tumor progression (89–94). Using these naturally derived matrix mimetics, a dynamic range of stiffness can be realized usually in the range of ~100–1 MPa which allows for a closer approximation of in vivo mechanical cues (95, 96). However, due to the batch-to-batch variations of gels it is difficult to achieve consistency (32). Synthetic hydrogels derived from materials such as polyethylene glycol, self-assembling peptides, and poly (d, l-lactide-co-glycolide) offer the advantage of spatially defined environments with controllable physical parameters (97–99). Specific adhesion sites such as RGD-binding motifs can be incorporated into a hydrogel to model cell adhesion (97), and the controlled release of tumorigenic factors into hydrogels can shed light on specific aspects of tumor development (100). These engineered environments provide tractable platforms to dissect the role of external biochemical and mechanical stimuli on cell migration in 3D, mechanisms of cellular differentiation, gene expression, and cellular responses (83). Mixed materials featuring both natural and synthetic components offer the advantage of independently tuning variables such as mechanical stiffness, adhesion, peptide density, and matrix hydrophilicity (101).
Tissue is not homogeneous in vivo but has distinct topographies, as evidenced by differences in the architecture of fibrillar collagens and vasculature (48). In addition to stiffness, other physical properties such as dimension and topography have profound effects on cell fate and malignancy (30, 47, 48). Therefore, it is desirable to consider spatial heterogeneity in these artificial biological landscapes to incorporate contributions from both tissue stiffness and those due to topography. Laminin hydrogels form amorphous gels with no fibrils or spatial heterogeneities on the same scale as that of cells. To dynamically tune the protein concentration of these hydrogels, the proteins are commonly cross-linked. But matrix rigidity inevitably, and sometimes undesirably, increases with protein concentration (63). A key goal of developing biomimetic matrices currently underway is to develop a methodology that allows for independent control of material parameters such as protein distribution and alignment, and other physical properties (48).
Defining the Biotic Cellular Components within the Tumor Ecosystem: Tumor–Stromal Interactions
Tissue homeostasis and architectural integrity are facilitated by the dynamic interactions between normal epithelial cells and stromal cells. Cancer cells alter the normal tissue landscape in efforts to transform the tissue microenvironment into one that is tumor-permissive (34, 35, 42, 51). Within tissue, heterotypic cell interactions between tumor cells and stromal cells can either drive or mitigate tumor development and metastatic potential (102–106).
Immune cells such as Tie2-expressing monocytes (107), mast cells (108), myeloid cells (109, 110), B cells (111), and macrophages (56) form cooperative relationships with malignant cells to create a tumor-promoting niche. By contrast, other immune cells such as natural killer cells (112) and dendritic cells (113) act as tumor-suppressive agents. Furthermore, these interactions are contextual; immune cells can either act as tumor-promoting or tumor-suppressive agents, as occurs with neutrophils (114) and regulatory T cells (115, 116). Immunotherapies using vaccines, immune adjuvants, cytokines, immune-modulating antibodies, and effector cells that exploit the immune response to cancer have been implemented, often in combination with radiation and chemotherapy (113, 117–121).
Similarly, other cells found in the reactive tumor stroma such as cancer-associated fibroblasts (CAFs), endothelial cells and pericytes can adopt either tumor-promoting or tumor-suppressive roles (122). CAFs have been found to promote tumor progression in breast and pancreatic cancers (104, 122–124). They support tumor proliferation by altering their metabolism (125, 126), increasing ECM production, and producing and digesting metabolites (113, 122). They also secrete growth factors such as VEGF, EGF, and TGF-a and chemokines including IL-1, IL-6, and CCLs (122, 123, 127) to recruit endothelial cells, pericytes, and inflammatory cells in building the tumor niche. These interactions promote the development of the tumor by encouraging proliferation, angiogenesis, inflammation, and metastasis. Due to their cooperative roles in the tumor microenvironment, CAFs have been targeted by various cancer treatments (128).
However, the angiogenic environment remains one of the most potent druggable targets (129) and one of the most successful stromal targets in cancer treatment (39, 130, 131). Angiogenesis or the sprouting of neovessels is induced early during the evolution of invasive carcinomas as observed in animal models and in humans (131). This process involves recruitment of sprouting vessels from existing blood vessels where endothelial cells proliferate, migrate, and organize functional tubular structures. Not only is the generation of neovasculature important to sustain proliferation of macroscopic tumors, it may play a functional role in tumor etiology to facilitate the transition from premalignant neoplasia to full-blown malignancy (131). The nascent angiogenic environment remains an attractive target in benign lesions in efforts to prevent full-blown malignancy.
Recent studies have begun to implicate lesser-interrogated relationships between tumor cells and other cell types within the microenvironment such as adipose cells and neuronal cells (132). Cancer-associated adipocytes (CAAs) promote tumor development and progression (133) by promoting tumor cell migration and invasiveness (134, 135). The molecular mechanisms underlying adipocyte-cancer cell communication are possible targets for cancer diagnosis and treatment, and could help to reveal the relationship between obesity-related metabolic disorders and cancer (136). Neuronal (nerve) cells have also been implicated in promoting malignancy (137). Specifically, these cells secrete factors that facilitate increased tumor proliferation. In turn, tumor cells secrete neurotropic factors and exon guidance molecules that induce sprouting of sensory nerves within the bone to increase neurite formation (138, 139). Consequently, patients experience cancer-related bone pain which can be relieved by the inhibition of nerve growth factor (NGF) (139). Additional characterization of the neurotransmitters or neuropeptides that mediate the cooperation between cancer and neural cells could reveal potential targets for cancer treatment.
Building the Ecosystem – A Co-Culture Architecture in 3D
3D heterotypic platforms afford the flexibility of incorporating multiple cell types such as the co-culture of tumor cells and fibroblasts, macrophages adipocytes, and osteoblasts and endothelial cells (79, 140–143). These in vitro platforms simulate the in vivo network of interactions within the tumor ecosystem. From this point of view, when developing a co-culture system, one should consider (i) cell types and model complexity; (ii) the desired interactions between cell types; and (iii) population-level characteristics such as culture volume, cell density, population sizes, and the interactions between cell populations. These co-culture systems can be as simple as a sandwich model where one cell type is cultured on a confluent layer of a different cell type. This bilayer geometry can also be achieved using commercially available transwell culture systems and microfluidic platforms (144–148). A second commonly used geometrical arrangement involves the culture of multicellular tumor spheroids co-cultured with cells embedded in the surrounding surrogate matrix. These spheroids can be generated due to spontaneous aggregation, liquid overlay cultures, or using gyratory or spinner flasks or expansion from a single cell (149). These models have been used to study tumor progression and aid in the development of drug screening platforms (150). Spheroids generated from tumor or tissue biopsies may facilitate the development of individualized and patient-specific therapy (151). Additional complexity such as topography of interaction, degree of contact, and the number of cells that can be co-cultured can be achieved using a combination of microfluidics and micropatterning. Specifically, cell types that require different environments to maintain physiologically relevant signaling can be co-cultured in microfluidic channels to facilitate exchange of molecules without direct contact. Using this platform, their respective environments are separated by semi-permeable membranes or gels within microfluidic channels (152, 153) where their growth media are separated using laminar flow, or grown in semi-connected liquid compartments (154). To date, the majority of co-culture studies have involved two populations such as normal cells and malignant cells (155). However, many different types of cells are involved in the process of tumorigenesis, which intrinsically increases the complexity. The combination of microfluidics and 3D microfabrication of ECM scaffolds has been used to generate self-assembly of in vitro “organs on a chip” (156) such as the brain, liver, and gut (157–159). Interactions among more than two cell types have been studied in a defined synthetic ecology for bacteria (160), or in model systems of organs such as the endothelium (161). Thus, a future application to recapitulate these interactions might be achieved by incorporating tumor cells in these organotypic models.
Abiotic Components within the Tumor Ecosystem: Oxygen, pH, Stiffness, and Chemokine Gradients
The acellular microenvironment of a tumor is characterized by cells and biochemical components and by their complex interactions with the physical and chemical parameters such as stiffness, pH, oxygen tension, interstitial pressure, and fluid flux (42, 162–164). Both tumor and stromal cells create gradients of secreted cytokines and growth factors, which contribute to altered proliferation and directed cell migration to facilitate tumor progression, dissemination, and invasion (57, 165, 166).
As tumors proliferate, their energy and oxygen requirements often cannot be met by existing tissue vasculature. Hypoxia is also associated with drug resistance as low oxygen tensions affect the cell cycle and slow cycling cells are thought to be minimally affected by treatments targeting the cell cycle (167). It has also been implicated in upregulation of genes that regulate cell proliferation, ECM production, cell adhesion, and cell invasion through induction of the hypoxia-inducible factor (HIF) family of transcription factors. Neoangiogenesis in part alleviates growth-induced hypoxia but tumor vasculature lacks the normal hierarchical arrangement of artery–arteriole–capillary, resulting in intercellular gaps that leak fluids, blood, and fibrins, and inefficient oxygen delivery (168). Consequently, within the growing mass, there are regions of hypoxia where certain cancer cells are deprived of oxygen (169). In response, tumor cells may undergo a metabolic shift causing acidosis (170, 171). Hydrogen (H+) ions generated by tumor cells during both aerobic and anaerobic glycolysis, glutaminolysis, and ATP hydrolysis are transported to the extracellular milieu (172, 173) creating an acidic tumor microenvironment (174). These acidic environments are thought to be permissive for tumor invasion and successive metastasis by inducing cell death in the surrounding normal tissue and degradation of the ECM.
In addition to the chemical variations, a myriad of physical attributes of the tumor microenvironment also play important roles by imparting mechanical cues that promote the malignant transition of cancer cells and increased metastasis (30, 42, 175, 176). Cells encounter multiple mechanical cues at each step of the metastatic cascade. They traverse complex ECM arrangements and topographies during invasion into surrounding tissue and in the vascular system during intravasation and extravasation (30). These dynamic mechanical inputs are derived from changes in stiffness, fibril structural architecture, and shear stress. Shear flow directly affects extravasation by modulating adhesion at blood vessel interface (30). Stiffer areas of fibrosis with increased ECM deposition are found around some invasive ductal carcinomas and metastatic lesions residing at lymph nodes and bones (177). In these patients, a higher risk of developing bone and lymph node metastasis, and disease recurrence was observed (177). Tumor dormancy is modulated by the microenvironment. Cells remain quiescent in initially non-permissive microenvironments, but transition to a proliferative state based on changes in the ECM composition and stiffness (12, 178).
Building the Ecosystem-Manipulating Abiotic Conditions
3D culture models have been used to bring in vivo abiotic conditions to cultured tumor cells, with effects such as hypoxia and acidosis on tumorigenicity (179, 180). Using these platforms, angiogenesis (181–185), cell migration (166, 186, 187), invasion (188), metastasis (189), and epithelial–mesenchymal transition have also been examined (190). They have also provided a means to study phenotypic-based pharmaceutical efficacy to test the likelihood of acquisition of drug resistance (191–195). Specifically, cells grown under hypoxic conditions in 3D culture models show higher resistance to toxins than the same cells when cultured in 2D grown on plastic (196–199). Microfluidic chips fabricated with soft-lithography methods offer the possibility of simultaneously reproducing both physical and chemical abiotic components within tissue mimetics (166, 200–204). Defined geometries allow confined cell growth or cell–cell contact, with fluid flow controlled to generate physiological shear stresses or chemical gradients, with serial fluid exchange performed to deliver nutrients or chemicals on defined time-tables, or with integrated mechanical components able to exert periodic stresses on cells or the ECM (156, 191, 205–207). By combining these fabrication techniques, the organ-on-a-chip was developed, which recapitulates a broad range of in vivo physiological functions for organs such as the brain, liver, and gut (208–210). These devices also permit the analysis of tissue-specific responses in reaction to external physical stimuli such as stiffness or shear stress and biochemical perturbations such as drugs or toxins (211–213). A recent application in which dynamic hydraulic compression was applied to human bone marrow and adipocyte-derived stem-cells-on-chips resulted in increased bone differentiation as measured by osteogenic gene expression and production of bone-specific ECM components (214). These principles can be applied to cancer studies (215) in which in vitro 3D models of lymphoma, pancreatic, breast, prostate, and oral cancers have already produced clinically relevant results (192). These and the aformentioned model systems are summarized in Figure 1.
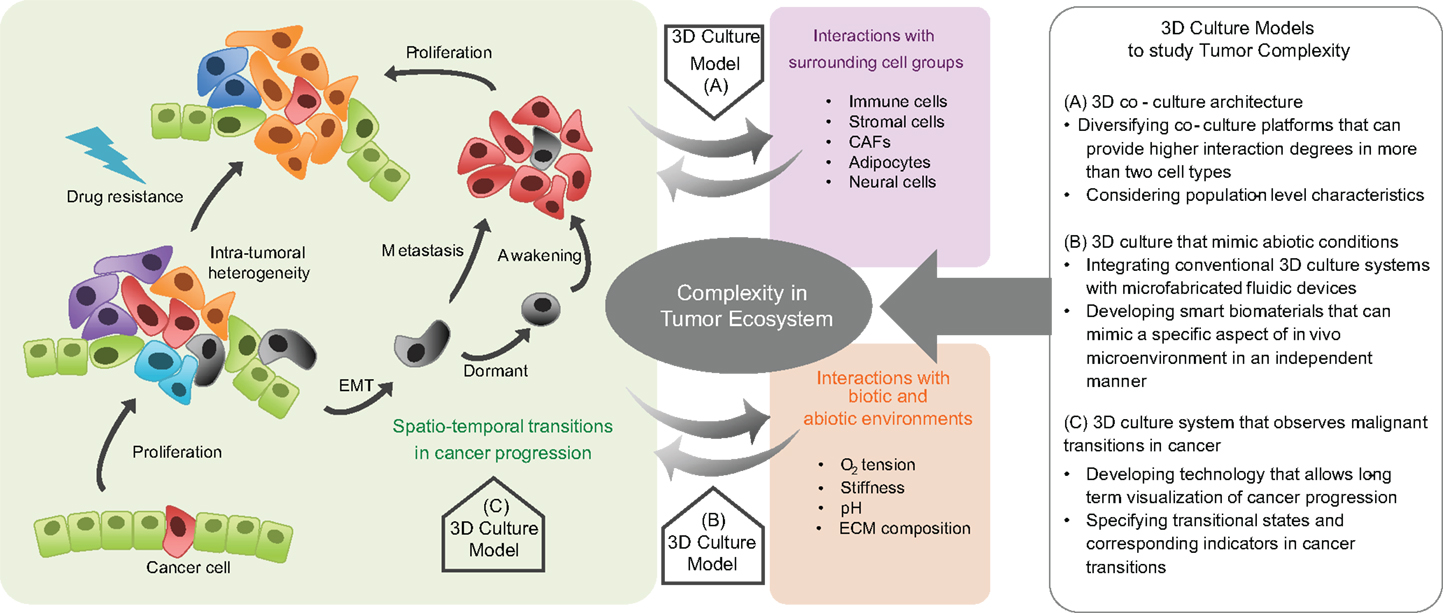
Figure 1. Complexity in tumor ecosystem and 3D culture models. Cancer is a complex disease in which the cancer cell population dynamically evolves and the diversity of heterotypic interactions between cancer cells, surrounding cells, and environmental factors is spatiotemporally regulated. Therefore, preclinical models that incorporate factors that play critical roles in the dynamic tumor progression, within a defined biomimetic landscape are needed. Three-dimensional culture models help to deconstruct the complexity of cancer. Model systems can be engineered to recapitulate tumor cell-surrounding cell interactions, the physicochemical characteristics in an abiotic tumor environment, and the malignant transitions in tumor progression. They can provide insight into the evolutionary and ecological aspects of tumor progression, with relevant therapeutic implications.
Malignant Transitions in the Tumor Ecosystem
The ecological perspective on cancer introduced so far has been based primarily on studies on many genetic and molecular factors that initiate, drive, and promote cancer. Even though many causative elements are involved in malignant progression, the emergent malignant properties such as drug resistance and metastatic potential are still poorly predicted and managed, because our knowledge about the population-level dynamics of cancer is limited (216–219). Many phenotypic transitions in cancer cell populations occur during tumor progression: transitions from a single cell to multiple cells; from slow to fast growth; from proliferation to migration; from oxidative phosphorylation to anaerobic glycolysis; from epithelial to mesenchymal phenotype; from drug-sensitive to drug-resistant; and from dormant to metastatic (220–223). Understanding these transitions in the context of population dynamics might open new avenues of cancer treatment, as preventing the transitions toward malignancy could make a tumor manageable and treatable by hampering its adaptive progression. Using these microfluidic platforms in which both the mechanical inputs and chemical gradients are controlled may yet simulate in vivo evolution of tumor heterogeneity and phenotypic plasticity due to environmental perturbations.
Visualizing Malignant Transitions in the Tumor Ecosystem
Imaging modalities such as magnetic resonance imaging (MRI), computed tomography (CT), positron emission tomography (PET), and microscopy offer the advantage of long-term monitoring in human patients with lesions can be imaged over a period of time (224). In particular, MRI and PET also detect changes in tumor metabolism and pH (174, 225). However, the use of such imaging methods to monitor human disease is faced with several drawbacks (226–229). Namely, disease can be detected only after several rounds of tumor cell proliferation, and single cell dynamics cannot be readily achieved (224). Ideally, diagnosis should occur at the earliest stage of malignant transition and/or single cell resolution if efforts are to be successful for therapeutic intervention. Murine models remain an attractive option to examine tumor etiology and progression as one can interrogate human cell lines using immune-compromised strains and similarly examine syngeneic cancer cell lines for each strain (230). But these preclinical models share the same imaging bottlenecks as observed in humans. The major limiting factor when imaging organs is that biological tissue is opaque and scatters light such that image quality deteriorates rapidly if one attempts to image deeper into thicker samples (231). Conventional confocal microscopy (one and two photon) cannot image deeper than a few hundred microns within organs that span millimeters to centimeters (232). Combining adaptive optics with two-photon fluorescent microscopy increases the achievable spatial resolution to penetration depths of ~1mm (233). Intravital microscopy using principles based on two-photon microscopy offers the flexibility of imaging single cell dynamics within thick tissue (234–236). However, longitudinal studies in murine models often require invasive techniques such as an imaging window and fluorescent reporters to visualize single and collective tumor cell dynamics (237). These windows often limit the area of interrogation. Hence, the sacrifice of many mice is needed to achieve acceptable statistics. In addition, these techniques require specific expertise, and are currently low throughput.
Combining imaging modalities and in vitro 3D culture models that recapitulate physiologically relevant aspects of tumor progression and metastatic disease as an alternative pre-clinical model allows us to image single cell dynamics (238). Using engineered platforms, single tumor cell intravasation and tumor cell proliferation/motility in different microenvironments have been visualized (239–243). These examples hint at the power of combining imaging modalities with 3D in vitro platforms to study heterotypic cell interactions, evolution of tumor heterogeneity, and acquisition of drug resistance. However, it would be advantageous if we could merge technologies that allow for visualization and quantitation of physical properties such as ECM stiffness and changes in topography of the tissue. In this section, we present some ideas for future consideration. One area involves monitoring the dynamic mechanical measurements of the ECM throughout the entire process of tumorigenesis. Several methods for determining physical properties of surrogate ECM gels and cells exist such as atomic force microscopy, which can be used to mechanically probe thin gels and superficially in excised tissues (~nm), and traction form microscopy, which can resolve forces within thick hydrogels where the physical properties are known (244–246). While these techniques are powerful, a technique that can probe the mechanical properties at depths >100 μm with sub micron resolution in thick samples eventually extending to an animal model without a priori knowledge of the physical properties of the tissue is desirable. Optical traps have been used to characterize gels and other materials (247), and recently have even shown the ability to characterize the viscoelastic properties of living cells (248). The combination of imaging the local structure and components of the ECM while simultaneously measuring its mechanical properties will reveal correlations between the two, and how they change with time as cells remodel and react to their environment. Combining light microscopy and an optical trap platform may be one method to simultaneously visualize and measure the changes in the physical properties of the local microenvironment in 3D in vitro platforms.
Estimating the Risk of Emergent Malignancy in Cancer
One way of modeling tumor growth is by a logistic growth curve in which growth is initially exponential but then stabilizes (249). Recently a review proposed consider an alternative approach from evolutionary dynamics, where an allee effect may be explored for therapeutics. Briefly, the Allee effect is defined as a decline in individual fitness at low population size or density. A population with a strong allee effect is one that is stable at intermediate numbers but may become extinct at numbers that are either too small or too large (249). Therefore, estimating the risk of such a transition in a cancer population may predict the likelihood of progression, and also provide opportunities to prevent population diversification, metastasis, and drug resistance. One possible application is to eradicate quiescent DTCs that are able to enter dormancy and evade targeted/conventional therapies (250). These cells are thought to be rare and few members of the original primary tumor cells (250). Discovering the dormancy mechanism of DTCs has been the subject of numerous studies, as it is considered an important mechanism of tumor recurrence that is responsible for a large proportion of tumor-related deaths (251). Understanding the microenvironmental regulation of producing a strong Allee effect on these rare cells might also help to advance current therapies by exploiting a rapid collapse of this tumor sub-population. In the field of ecology, a critical transition is an abrupt and unexpected change of a complex system, such as a sudden climate change with rapid extinction of a species (252). In homogeneous and highly connected networks, local losses due to external stresses can be normalized by subsidiary inputs from linked units in the network; however, when the amount of stress exceeds a certain critical level, the system rapidly collapses (253, 254). If we treat heterotypic cell interactions, as those observed for interspecies interactions, and tissue architecture likened to spatial organization of species, then tumors are similar to ecologically complex systems. It is possible that a critical transition where tumor cells become extinct or normalized may be induced by normalizing tissue architecture or eradicating supportive tumor–stromal interactions (249).
Unfortunately, there have been few empirical results demonstrating sudden systematic transitions in cancer cell populations. Several 3D models that recapitulate the process of epithelial–mesenchymal transition in cancer have been observed at the single spheroid level (255–257). However, theoretical studies on population level changes have supplemented these experimental observations (249, 258, 259).
In conclusion, studying ecological aspects of cancer provides us with the tools to understand cancer complexity, and the power to prevent further progression toward emergent malignancy. Direct visualization of tissue dynamics has refined our understanding of the basic principles of cell migration, lineage commitment, and the establishment of tissue architecture. Future studies in which 3D culture models are coupled with microfluidics and appropriate imaging modalities will begin to examine the singular inputs and interplay of biotic and abiotic components within tumor ecosystems. We may yet identify universal signals produced when a tumor approaches a malignant transition, such as acquisition of drug resistance, dormancy, or metastasis, to enable better management of cancer.
Transforming these ecological inputs into meaningful information for patient care will be challenging. Evolutionary and ecological theories may be the unifiers in our understanding of cancer. Advances in phylogenetic reconstruction and agent-based modeling will guide our understanding of somatic evolutionary pathways (5, 260, 261). In addition, mathematical models that incorporate tumor heterogeneity and the microenvironment allow for derivation of potential outcomes as tumor cells adapt to abiotic changes, such as hypoxia and acidosis, and to chemotherapy (20, 262–264). Models integrating observations and experimental data will continue to refine our understanding of the adaptive tumor landscape (263–266). Advanced 3D model systems will provide tools to interpret this complexity, which could lead to an alternative description of emergent cancer progression.
Conflict of Interest Statement
The authors declare that the research was conducted in the absence of any commercial or financial relationships that could be construed as a potential conflict of interest.
Acknowledgments
The authors apologize to authors whose work was not reflected in the references due to space constraints. This effort was supported by the Intramural Research Program of the National Institutes of Health, the National Cancer Institute. This research was also supported by a grant of the Korea Health Technology R&D Project through the Korea Health Industry Development Institute (KHIDI), funded by the Ministry of Health & Welfare, Republic of Korea (grant number: HI14C1161). We thank Jack R. Staunton and George Leiman for critically reading this manuscript.
References
1. Wagle N, Emery C, Berger MF, Davis MJ, Sawyer A, Pochanard P, et al. Dissecting therapeutic resistance to RAF inhibition in melanoma by tumor genomic profiling. J Clin Oncol (2011) 29(22):3085–96. doi:10.1200/JCO.2010.33.2312
2. Colozza M, de Azambuja E, Personeni N, Lebrun F, Piccart MJ, Cardoso F. Achievements in systemic therapies in the pregenomic era in metastatic breast cancer. Oncologist (2007) 12(3):253–70. doi:10.1634/theoncologist.12-3-253
3. Hennessy BT, Smith DL, Ram PT, Lu Y, Mills GB. Exploiting the PI3K/AKT pathway for cancer drug discovery. Nat Rev Drug Discov (2005) 4(12):988–1004. doi:10.1038/nrd1902
4. Bild AH, Yao G, Chang JT, Wang Q, Potti A, Chasse D, et al. Oncogenic pathway signatures in human cancers as a guide to targeted therapies. Nature (2006) 439(7074):353–7. doi:10.1038/nature04296
5. Gerlinger M, Rowan AJ, Horswell S, Larkin J, Endesfelder D, Gronroos E, et al. Intratumor heterogeneity and branched evolution revealed by multiregion sequencing. N Engl J Med (2012) 366(10):883–92. doi:10.1056/NEJMoa1113205
6. Ding L, Ley TJ, Larson DE, Miller CA, Koboldt DC, Welch JS, et al. Clonal evolution in relapsed acute myeloid leukaemia revealed by whole-genome sequencing. Nature (2012) 481(7382):506–10. doi:10.1038/nature10738
7. Mullighan CG, Phillips LA, Su X, Ma J, Miller CB, Shurtleff SA, et al. Genomic analysis of the clonal origins of relapsed acute lymphoblastic leukemia. Science (2008) 322(5906):1377–80. doi:10.1126/science.1164266
8. McGranahan N, Swanton C. Biological and therapeutic impact of intratumor heterogeneity in cancer evolution. Cancer Cell (2015) 27(1):15–26. doi:10.1016/j.ccell.2014.12.001
9. Chambers AF, Groom AC, MacDonald IC. Dissemination and growth of cancer cells in metastatic sites. Nat Rev Cancer (2002) 2(8):563–72. doi:10.1038/nrc865
10. Bragado P, Estrada Y, Parikh F, Krause S, Capobianco C, Farina HG, et al. TGF-beta 2 dictates disseminated tumour cell fate in target organs through TGF-beta-RIII and p38 alpha/beta signalling. Nat Cell Biol (2013) 15(11):1351–U1210. doi:10.1038/ncb2861
11. Malanchi I, Santamaria-Martínez A, Susanto E, Peng H, Lehr HA, Delaloye JF, et al. Interactions between cancer stem cells and their niche govern metastatic colonization. Nature (2012) 481(7379):85–95. doi:10.1038/nature10694
12. Barkan D, Green JE, Chambers AF. Extracellular matrix: a gatekeeper in the transition from dormancy to metastatic growth. Eur J Cancer (2010) 46(7):1181–8. doi:10.1016/j.ejca.2010.02.027
13. Schewe DM, Aguirre-Ghiso JA. ATF6 alpha-Rheb-mTOR signaling promotes survival of dormant tumor cells in vivo. Proc Natl Acad Sci U S A (2008) 105(30):10519–24. doi:10.1073/pnas.0800939105
14. Onder TT, Kara N, Cherry A, Sinha AU, Zhu N, Bernt KM, et al. Chromatin-modifying enzymes as modulators of reprogramming. Nature (2012) 483(7391):598–U119. doi:10.1038/nature10953
15. Reya T, Morrison SJ, Clarke MF, Weissman IL. Stem cells, cancer, and cancer stem cells. Nature (2001) 414(6859):105–11. doi:10.1038/35102167
16. Zhao C, Chen A, Jamieson CH, Fereshteh M, Abrahamsson A, Blum J, et al. Hedgehog signalling is essential for maintenance of cancer stem cells in myeloid leukaemia. Nature (2009) 458(7239):776–9. doi:10.1038/nature07737
17. Reya T, Clevers H. Wnt signalling in stem cells and cancer. Nature (2005) 434(7035):843–50. doi:10.1038/nature03319
19. Nowell PC. The clonal evolution of tumor cell populations. Science (1976) 194(4260):23–8. doi:10.1126/science.959840
20. Anderson AR, Weaver AM, Cummings PT, Quaranta V. Tumor morphology and phenotypic evolution driven by selective pressure from the microenvironment. Cell (2006) 127(5):905–15. doi:10.1016/j.cell.2006.09.042
21. Cahill DP, Kinzler KW, Vogelstein B, Lengauer C. Genetic instability and darwinian selection in tumours. Trends Cell Biol (1999) 9(12):M57–60. doi:10.1016/S0962-8924(99)01661-X
22. Liotta LA, Kohn EC. The microenvironment of the tumour-host interface. Nature (2001) 411(6835):375–9. doi:10.1038/35077241
23. Meacham CE, Morrison SJ. Tumour heterogeneity and cancer cell plasticity. Nature (2013) 501(7467):328–37. doi:10.1038/nature12624
24. Marusyk A, Polyak K. Tumor heterogeneity: causes and consequences. Biochim Biophys Acta (2010) 1805(1):105–17. doi:10.1016/j.bbcan.2009.11.002
25. Swanton C. Intratumor heterogeneity: evolution through space and time. Cancer Res (2012) 72(19):4875–82. doi:10.1158/0008-5472.CAN-12-2217
26. O’Brien SJ. A role for molecular genetics in biological conservation. Proc Natl Acad Sci U S A (1994) 91(13):5748–55. doi:10.1073/pnas.91.13.5748
27. Lynch M, Conery J, Burger R. Mutation accumulation and the extinction of small populations. Am Nat (1995) 146(4):489–518. doi:10.1086/285812
28. Bissell MJ, Radisky D. Putting tumours in context. Nat Rev Cancer (2001) 1(1):46–54. doi:10.1038/35094059
29. Villanueva J, Herlyn M. Melanoma and the tumor microenvironment. Curr Oncol Rep (2008) 10(5):439–46. doi:10.1007/s11912-008-0067-y
30. Kumar S, Weaver VM. Mechanics, malignancy, and metastasis: the force journey of a tumor cell. Cancer Metastasis Rev (2009) 28(1–2):113–27. doi:10.1007/s10555-008-9173-4
31. Swartz MA, Iida N, Roberts EW, Sangaletti S, Wong MH, Yull FE, et al. Tumor microenvironment complexity: emerging roles in cancer therapy. Cancer Res (2012) 72(10):2473–80. doi:10.1158/0008-5472.CAN-12-0122
32. Tanner K, Gottesman MM. Beyond 3D culture models of cancer. Sci Transl Med (2015) 7(283):283s9. doi:10.1126/scitranslmed.3009367
33. Netti PA, Berk DA, Swartz MA, Grodzinsky AJ, Jain RK. Role of extracellular matrix assembly in interstitial transport in solid tumors. Cancer Res (2000) 60(9):2497–503.
34. Radisky D, Hagios C, Bissell MJ. Tumors are unique organs defined by abnormal signaling and context. Semin Cancer Biol (2001) 11(2):87–95. doi:10.1006/scbi.2000.0360
35. Egeblad M, Nakasone ES, Werb Z. Tumors as organs: complex tissues that interface with the entire organism. Dev Cell (2010) 18(6):884–901. doi:10.1016/j.devcel.2010.05.012
37. Pienta KJ, McGregor N, Axelrod R, Axelrod DE. Ecological therapy for cancer: defining tumors using an ecosystem paradigm suggests new opportunities for novel cancer treatments. Transl Oncol (2008) 1(4):158–64. doi:10.1593/tlo.08178
38. Thomas F, Fisher D, Fort P, Marie JP, Daoust S, Roche B, et al. Applying ecological and evolutionary theory to cancer: a long and winding road. Evol Appl (2013) 6(1):1–10. doi:10.1111/eva.12021
39. Box C, Rogers SJ, Mendiola M, Eccles SA. Tumour-microenvironmental interactions: paths to progression and targets for treatment. Semin Cancer Biol (2010) 20(3):128–38. doi:10.1016/j.semcancer.2010.06.004
40. Jones CG, Lawton JH, Shachak M. Organisms as Ecosystem Engineers. Ecosystem Management. Springer (1996). p. 130–47.
41. Odling-Smee FJ, Laland KN, Feldman MW. Niche Construction: The Neglected Process in Evolution. Princeton University Press (2003).
42. Lu PF, Weaver VM, Werb Z. The extracellular matrix: a dynamic niche in cancer progression. J Cell Biol (2012) 196(4):395–406. doi:10.1083/jcb.201102147
43. O’Brien LE, Zegers MM, Mostov KE. Opinion: building epithelial architecture: insights from three-dimensional culture models. Nat Rev Mol Cell Biol (2002) 3(7):531–7. doi:10.1038/nrm859
44. Yamada KM, Cukierman E. Modeling tissue morphogenesis and cancer in 3D. Cell (2007) 130(4):601–10. doi:10.1016/j.cell.2007.08.006
45. Lee GY, Kenny PA, Lee EH, Bissell MJ. Three-dimensional culture models of normal and malignant breast epithelial cells. Nat Methods (2007) 4(4):359–65. doi:10.1038/nmeth1015
46. Kern J, Steurer M, Gastl G, Gunsilius E, Untergasser G. Vasohibin inhibits angiogenic sprouting in vitro and supports vascular maturation processes in vivo. BMC Cancer (2009) 9:284. doi:10.1186/1471-2407-9-284
47. Yang F, Murugan R, Wang S, Ramakrishna S. Electrospinning of nano/micro scale poly(L-lactic acid) aligned fibers and their potential in neural tissue engineering. Biomaterials (2005) 26(15):2603–10. doi:10.1016/j.biomaterials.2004.06.051
48. Khademhosseini A, Langer R, Borenstein J, Vacanti JP. Microscale technologies for tissue engineering and biology. Proc Natl Acad Sci U S A (2006) 103(8):2480–7. doi:10.1073/pnas.0507681102
49. Wellings SR, Jentoft VL. Organ cultures of normal, dysplastic, hyperplastic, and neoplastic human mammary tissues. J Natl Cancer Inst (1972) 49(2):329–38.
50. Tanner K. Regulation of the basement membrane by epithelia generated forces. Phys Biol (2012) 9(6):065003. doi:10.1088/1478-3975/9/6/065003
51. Kessenbrock K, Plaks V, Werb Z. Matrix metalloproteinases: regulators of the tumor microenvironment. Cell (2010) 141(1):52–67. doi:10.1016/j.cell.2010.03.015
52. Dvorak HF. Tumors: wounds that do not heal. Similarities between tumor stroma generation and wound healing. N Engl J Med (1986) 315(26):1650–9. doi:10.1056/NEJM198612253152606
53. Dolberg DS, Hollingsworth R, Hertle M, Bissell MJ. Wounding and its role in RSV-mediated tumor formation. Science (1985) 230(4726):676–8. doi:10.1126/science.2996144
54. Martins-Green M, Boudreau N, Bissell MJ. Inflammation is responsible for the development of wound-induced tumors in chickens infected with Rous sarcoma virus. Cancer Res (1994) 54(16):4334–41.
55. Coussens LM, Werb Z. Inflammation and cancer. Nature (2002) 420(6917):860–7. doi:10.1038/nature01322
56. Condeelis J, Pollard JW. Macrophages: obligate partners for tumor cell migration, invasion, and metastasis. Cell (2006) 124(2):263–6. doi:10.1016/j.cell.2006.01.007
57. Pickup M, Novitskiy S, Moses HL. The roles of TGFbeta in the tumour microenvironment. Nat Rev Cancer (2013) 13(11):788–99. doi:10.1038/nrc3603
58. Provenzano PP, Inman DR, Eliceiri KW, Keely PJ. Matrix density-induced mechanoregulation of breast cell phenotype, signaling and gene expression through a FAK-ERK linkage. Oncogene (2009) 28(49):4326–43. doi:10.1038/onc.2009.299
59. Nasser NJ. Heparanase involvement in physiology and disease. Cell Mol Life Sci (2008) 65(11):1706–15. doi:10.1007/s00018-008-7584-6
60. Ilan N, Elkin M, Vlodavsky I. Regulation, function and clinical significance of heparanase in cancer metastasis and angiogenesis. Int J Biochem Cell Biol (2006) 38(12):2018–39. doi:10.1016/j.biocel.2006.06.004
61. Frantz C, Stewart KM, Weaver VM. The extracellular matrix at a glance. J Cell Sci (2010) 123(24):4195–200. doi:10.1242/jcs.023820
62. Barker HE, Cox TR, Erler JT. The rationale for targeting the LOX family in cancer. Nat Rev Cancer (2012) 12(8):540–52. doi:10.1038/nrc3319
63. Levental KR, Yu H, Kass L, Lakins JN, Egeblad M, Erler JT, et al. Matrix crosslinking forces tumor progression by enhancing integrin signaling. Cell (2009) 139(5):891–906. doi:10.1016/j.cell.2009.10.027
64. Provenzano PP, Eliceiri KW, Campbell JM, Inman DR, White JG, Keely PJ. Collagen reorganization at the tumor-stromal interface facilitates local invasion. BMC Med (2006) 4(1):38. doi:10.1186/1741-7015-4-38
65. Radisky DC, Bissell MJ. Matrix metalloproteinase-induced genomic instability. Curr Opin Genet Dev (2006) 16(1):45–50. doi:10.1016/j.gde.2005.12.011
66. Pantel K, Brakenhoff RH. Dissecting the metastatic cascade. Nat Rev Cancer (2004) 4(6):448–56. doi:10.1038/nrc1370
67. Paget S. The distribution of secondary growths in cancer of the breast. 1889. Cancer Metastasis Rev (1989) 8(2):98–101.
68. Pickup MW, Mouw JK, Weaver VM. The extracellular matrix modulates the hallmarks of cancer. EMBO Rep (2014) 15(12):1243–53. doi:10.15252/embr.201439246
69. Tlsty TD, Coussens LM. Tumor stroma and regulation of cancer development. Annu Rev Pathol (2006) 1:119–50. doi:10.1146/annurev.pathol.1.110304.100224
70. DeCosse JJ, Gossens CL, Kuzma JF, Unsworth BR. Breast-cancer – induction of differentiation by embryonic tissue. Science (1973) 181(4104):1057–8. doi:10.1126/science.181.4104.1057
71. Ingber DE, Madri JA, Jamieson JD. Basement-membrane as a spatial organizer of polarized epithelia – exogenous basement-membrane reorients pancreatic epithelial tumor-cells invitro. Am J Pathol (1986) 122(1):129–39.
72. Chung LW, Zhau HE, Ro JY. Morphologic and biochemical alterations in rat prostatic tumors induced by fetal urogenital sinus mesenchyme. Prostate (1990) 17(2):165–74. doi:10.1002/pros.2990170210
73. Maffini MV, Calabro JM, Soto AM, Sonnenschein C. Stromal regulation of neoplastic development – age-dependent normalization of neoplastic mammary cells by mammary stroma. Am J Pathol (2005) 167(5):1405–10. doi:10.1016/S0002-9440(10)61227-8
74. Ackerman A, Klein O, McDermott DF, Wang W, Ibrahim N, Lawrence DP, et al. Outcomes of patients with metastatic melanoma treated with immunotherapy prior to or after BRAF inhibitors. Cancer (2014) 120(11):1695–701. doi:10.1002/cncr.28620
75. Kenny PA, Bissell MJ. Tumor reversion: correction of malignant behavior by microenvironmental cues. Int J Cancer (2003) 107(5):688–95. doi:10.1002/ijc.11491
76. Robey IF, Baggett BK, Kirkpatrick ND, Roe DJ, Dosescu J, Sloane BF, et al. Bicarbonate increases tumor pH and inhibits spontaneous metastases. Cancer Res (2009) 69(6):2260–8. doi:10.1158/0008-5472.CAN-07-5575
77. Mintz B, Illmensee K. Normal genetically mosaic mice produced from malignant teratocarcinoma cells. Proc Natl Acad Sci U S A (1975) 72(9):3585–9. doi:10.1073/pnas.72.9.3585
78. Weaver VM, Petersen OW, Wang F, Larabell CA, Briand P, Damsky C, et al. Reversion of the malignant phenotype of human breast cells in three-dimensional culture and in vivo by integrin blocking antibodies. J Cell Biol (1997) 137(1):231–45. doi:10.1083/jcb.137.1.231
79. Braun AC. A demonstration of the recovery of the crown-gall tumor cell with the use of complex tumors of single-cell origin. Proc Natl Acad Sci U S A (1959) 45(7):932–8. doi:10.1073/pnas.45.7.932
80. Ingber DE. Can cancer be reversed by engineering the tumor microenvironment? Semin Cancer Biol (2008) 18(5):356–64. doi:10.1016/j.semcancer.2008.03.016
81. Fisher MB, Mauck RL. Tissue engineering and regenerative medicine: recent innovations and the transition to translation. Tissue Eng Part B Rev (2013) 19(1):1–13. doi:10.1089/ten.TEB.2012.0723
82. Blehm BH, Jiang N, Kotobuki Y, Tanner K. Deconstructing the role of the ECM microenvironment on drug efficacy targeting MAPK signaling in a pre-clinical platform for cutaneous melanoma. Biomaterials (2015) 56:129–39. doi:10.1016/j.biomaterials.2015.03.041
83. Cukierman E, Pankov R, Yamada KM. Cell interactions with three-dimensional matrices. Curr Opin Cell Biol (2002) 14(5):633–9. doi:10.1016/S0955-0674(02)00364-2
84. Kleinman HK, Martin GR. Matrigel: basement membrane matrix with biological activity. Semin Cancer Biol (2005) 15(5):378–86. doi:10.1016/j.semcancer.2005.05.004
85. Ulrich TA, Jain A, Tanner K, MacKay JL, Kumar S. Probing cellular mechanobiology in three-dimensional culture with collagen-agarose matrices. Biomaterials (2010) 31(7):1875–84. doi:10.1016/j.biomaterials.2009.10.047
86. Even-Ram S, Yamada KM. Cell migration in 3D matrix. Curr Opin Cell Biol (2005) 17(5):524–32. doi:10.1016/j.ceb.2005.08.015
87. Benton G, Kleinman HK, George J, Arnaoutova I. Multiple uses of basement membrane-like matrix (BME/Matrigel) in vitro and in vivo with cancer cells. Int J Cancer (2011) 128(8):1751–7. doi:10.1002/ijc.25781
88. Beck JN, Singh A, Rothenberg AR, Elisseeff JH, Ewald AJ. The independent roles of mechanical, structural and adhesion characteristics of 3D hydrogels on the regulation of cancer invasion and dissemination. Biomaterials (2013) 34(37):9486–95. doi:10.1016/j.biomaterials.2013.08.077
89. Lovitt CJ, Shelper TB, Avery VM. Advanced cell culture techniques for cancer drug discovery. Biology (Basel) (2014) 3(2):345–67. doi:10.3390/biology3020345
90. Ashley N, Yeung TM, Bodmer WF. Stem cell differentiation and lumen formation in colorectal cancer cell lines and primary tumors. Cancer Res (2013) 73(18):5798–809. doi:10.1158/0008-5472.CAN-13-0454
91. Vega-Naredo I, Loureiro R, Mesquita KA, Barbosa IA, Tavares LC, Branco AF, et al. Mitochondrial metabolism directs stemness and differentiation in P19 embryonal carcinoma stem cells. Cell Death Differ (2014) 21(10):1560–74. doi:10.1038/cdd.2014.66
92. Chen L, Xiao Z, Meng Y, Zhao Y, Han J, Su G, et al. The enhancement of cancer stem cell properties of MCF-7 cells in 3D collagen scaffolds for modeling of cancer and anti-cancer drugs. Biomaterials (2012) 33(5):1437–44. doi:10.1016/j.biomaterials.2011.10.056
93. Vermeulen L, Todaro M, de Sousa Mello F, Sprick MR, Kemper K, Perez Alea M, et al. Single-cell cloning of colon cancer stem cells reveals a multi-lineage differentiation capacity. Proc Natl Acad Sci U S A (2008) 105(36):13427–32. doi:10.1073/pnas.0805706105
94. Anastasov N, Höfig I, Radulović V, Ströbel S, Salomon M, Lichtenberg J, et al. A 3D-microtissue-based phenotypic screening of radiation resistant tumor cells with synchronized chemotherapeutic treatment. BMC Cancer (2015) 15:466. doi:10.1186/s12885-015-1481-9
95. Keung AJ, Kumar S, Schaffer DV. Presentation counts: microenvironmental regulation of stem cells by biophysical and material cues. Annu Rev Cell Dev Biol (2010) 26:533–56. doi:10.1146/annurev-cellbio-100109-104042
96. Levental I, Georges PC, Janmey PA. Soft biological materials and their impact on cell function. Soft Matter (2007) 3(3):299–306. doi:10.1039/B610522J
97. Schwartz MP, Fairbanks BD, Rogers RE, Rangarajan R, Zaman MH, Anseth KS. A synthetic strategy for mimicking the extracellular matrix provides new insight about tumor cell migration. Integr Biol (Camb) (2010) 2(1):32–40. doi:10.1039/b912438a
98. Lutolf MP, Lauer-Fields JL, Schmoekel HG, Metters AT, Weber FE, Fields GB, et al. Synthetic matrix metalloproteinase-sensitive hydrogels for the conduction of tissue regeneration: engineering cell-invasion characteristics. Proc Natl Acad Sci U S A (2003) 100(9):5413–8. doi:10.1073/pnas.0737381100
99. Raeber GP, Lutolf MP, Hubbell JA. Molecularly engineered PEG hydrogels: a novel model system for proteolytically mediated cell migration. Biophys J (2005) 89(2):1374–88. doi:10.1529/biophysj.104.050682
100. Na K, Park KH, Kim SW, Bae YH. Self-assembled hydrogel nanoparticles from curdlan derivatives: characterization, anti-cancer drug release and interaction with a hepatoma cell line (HepG2). J Control Release (2000) 69(2):225–36. doi:10.1016/S0168-3659(00)00256-X
101. Roy R, Boskey A, Bonassar LJ. Processing of type I collagen gels using nonenzymatic glycation. J Biomed Mater Res A (2010) 93a(3):843–51. doi:10.1002/jbm.a.32231
102. Junttila MR, de Sauvage FJ. Influence of tumour micro-environment heterogeneity on therapeutic response. Nature (2013) 501(7467):346–54. doi:10.1038/nature12626
103. Gajewski TF, Schreiber H, Fu YX. Innate and adaptive immune cells in the tumor microenvironment. Nat Immunol (2013) 14(10):1014–22. doi:10.1038/ni.2703
104. Mao Y, Keller ET, Garfield DH, Shen K, Wang J. Stromal cells in tumor microenvironment and breast cancer. Cancer Metastasis Rev (2013) 32(1–2):303–15. doi:10.1007/s10555-012-9415-3
105. Quail DF, Joyce JA. Microenvironmental regulation of tumor progression and metastasis. Nat Med (2013) 19(11):1423–37. doi:10.1038/nm.3394
106. Grivennikov SI, Greten FR, Karin M. Immunity, inflammation, and cancer. Cell (2010) 140(6):883–99. doi:10.1016/j.cell.2010.01.025
107. De Palma M, Naldini L. Tie2-expressing monocytes (TEMs): novel targets and vehicles of anticancer therapy? Biochim Biophys Acta (2009) 1796(1):5–10. doi:10.1016/j.bbcan.2009.04.001
108. Khazaie K, Blatner NR, Khan MW, Gounari F, Gounaris E, Dennis K, et al. The significant role of mast cells in cancer. Cancer Metastasis Rev (2011) 30(1):45–60. doi:10.1007/s10555-011-9286-z
109. Murdoch C, Muthana M, Coffelt SB, Lewis CE. The role of myeloid cells in the promotion of tumour angiogenesis. Nat Rev Cancer (2008) 8(8):618–31. doi:10.1038/nrc2444
110. Gabrilovich DI, Ostrand-Rosenberg S, Bronte V. Coordinated regulation of myeloid cells by tumours. Nat Rev Immunol (2012) 12(4):253–68. doi:10.1038/nri3175
111. de Visser KE, Korets LV, Coussens LM. De novo carcinogenesis promoted by chronic inflammation is B lymphocyte dependent. Cancer Cell (2005) 7(5):411–23. doi:10.1016/j.ccr.2005.04.014
112. Vivier E, Tomasello E, Baratin M, Walzer T, Ugolini S. Functions of natural killer cells. Nat Immunol (2008) 9(5):503–10. doi:10.1038/ni1582
113. Palucka K, Banchereau J. Cancer immunotherapy via dendritic cells. Nat Rev Cancer (2012) 12(4):265–77. doi:10.1038/nrc3258
114. Mantovani A, Cassatella MA, Costantini C, Jaillon S. Neutrophils in the activation and regulation of innate and adaptive immunity. Nat Rev Immunol (2011) 11(8):519–31. doi:10.1038/nri3024
115. Lindau D, Gielen P, Kroesen M, Wesseling P, Adema GJ. The immunosuppressive tumour network: myeloid-derived suppressor cells, regulatory T cells and natural killer T cells. Immunology (2013) 138(2):105–15. doi:10.1111/imm.12036
116. von Boehmer H, Daniel C. Therapeutic opportunities for manipulating T-Reg cells in autoimmunity and cancer. Nat Rev Drug Discov (2013) 12(1):51–63. doi:10.1038/nrd3683
117. Qian X, Wang X, Jin H. Cell transfer therapy for cancer: past, present, and future. J Immunol Res (2014) 2014:525913. doi:10.1155/2014/525913
118. Lotze MT, Matory YL, Rayner AA, Ettinghausen SE, Vetto JT, Seipp CA, et al. Clinical effects and toxicity of interleukin-2 in patients with cancer. Cancer (1986) 58(12):2764–72.
119. Miller JS, Soignier Y, Panoskaltsis-Mortari A, McNearney SA, Yun GH, Fautsch SK, et al. Successful adoptive transfer and in vivo expansion of human haploidentical NK cells in patients with cancer. Blood (2005) 105(8):3051–7. doi:10.1182/blood-2004-07-2974
120. Scott AM, Wolchok JD, Old LJ. Antibody therapy of cancer. Nat Rev Cancer (2012) 12(4):278–87. doi:10.1038/nrc3236
121. Restifo NP, Dudley ME, Rosenberg SA. Adoptive immunotherapy for cancer: harnessing the T cell response. Nat Rev Immunol (2012) 12(4):269–81. doi:10.1038/nri3191
122. Kalluri R, Zeisberg M. Fibroblasts in cancer. Nat Rev Cancer (2006) 6(5):392–401. doi:10.1038/nrc1877
123. Ostman A, Augsten M. Cancer-associated fibroblasts and tumor growth – bystanders turning into key players. Curr Opin Genet Dev (2009) 19(1):67–73. doi:10.1016/j.gde.2009.01.003
124. Ohlund D, Elyada E, Tuveson D. Fibroblast heterogeneity in the cancer wound. J Exp Med (2014) 211(8):1503–23. doi:10.1084/jem.20140692
125. Koukourakis MI, Giatromanolaki A, Harris AL, Sivridis E. Comparison of metabolic pathways between cancer cells and stromal cells in colorectal carcinomas: a metabolic survival role for tumor-associated stroma. Cancer Res (2006) 66(2):632–7. doi:10.1158/0008-5472.CAN-05-3260
126. Basanta D, Scott JG, Fishman MN, Ayala G, Hayward SW, Anderson AR. Investigating prostate cancer tumour-stroma interactions: clinical and biological insights from an evolutionary game. Br J Cancer (2012) 106(1):174–81. doi:10.1038/bjc.2011.517
127. Franco OE, Shaw AK, Strand DW, Hayward SW. Cancer associated fibroblasts in cancer pathogenesis. Semin Cell Dev Biol (2010) 21(1):33–9. doi:10.1016/j.semcdb.2009.10.010
128. Capparelli C, Chiavarina B, Whitaker-Menezes D, Pestell TG, Pestell RG, Hulit J, et al. CDK inhibitors (p16/p19/p21) induce senescence and autophagy in cancer-associated fibroblasts, “fueling” tumor growth via paracrine interactions, without an increase in neo-angiogenesis. Cell Cycle (2012) 11(19):3599–610. doi:10.4161/cc.21884
129. Bergers G, Benjamin LE. Tumorigenesis and the angiogenic switch. Nat Rev Cancer (2003) 3(6):401–10. doi:10.1038/nrc1093
130. Zerbini G, Lorenzi M, Palini A. Tumor angiogenesis. N Engl J Med (2008) 359(7):763–763. doi:10.1056/NEJMc081278
131. Hanahan D, Folkman J. Patterns and emerging mechanisms of the angiogenic switch during tumorigenesis. Cell (1996) 86(3):353–64. doi:10.1016/S0092-8674(00)80108-7
132. Tan JX, Buache E, Chenard MP, Dali-Youcef N, Rio MC. Adipocyte is a non-trivial, dynamic partner of breast cancer cells. Int J Dev Biol (2011) 55(7–9):851–9. doi:10.1387/ijdb.113365jt
133. Dirat B, Bochet L, Dabek M, Daviaud D, Dauvillier S, Majed B, et al. Cancer-associated adipocytes exhibit an activated phenotype and contribute to breast cancer invasion. Cancer Res (2011) 71(7):2455–65. doi:10.1158/0008-5472.CAN-10-3323
134. Menendez JA, Lupu R. Fatty acid synthase and the lipogenic phenotype in cancer pathogenesis. Nat Rev Cancer (2007) 7(10):763–77. doi:10.1038/nrc2222
135. Nomura DK, Long JZ, Niessen S, Hoover HS, Ng SW, Cravatt BF. Monoacylglycerol lipase regulates a fatty acid network that promotes cancer pathogenesis. Cell (2010) 140(1):49–61. doi:10.1016/j.cell.2009.11.027
136. Ben Sahra I, Le Marchand-Brustel Y, Tanti JF, Bost F. Metformin in cancer therapy: a new perspective for an old antidiabetic drug? Mol Cancer Ther (2010) 9(5):1092–9. doi:10.1158/1535-7163.MCT-09-1186
137. Mancino M, Ametller E, Gascon P, Almendro V. The neuronal influence on tumor progression. Biochim Biophys Acta (2011) 1816(2):105–18. doi:10.1016/j.bbcan.2011.04.005
138. Liebig C, Ayala G, Wilks JA, Berger DH, Albo D. Perineural invasion in cancer: a review of the literature. Cancer (2009) 115(15):3379–91.
139. Jimenez-Andrade JM, Bloom AP, Stake JI, Mantyh WG, Taylor RN, Freeman KT, et al. Pathological sprouting of adult nociceptors in chronic prostate cancer-induced bone pain. J Neurosci (2010) 30(44):14649–56. doi:10.1523/JNEUROSCI.3300-10.2010
140. Hoffmann TK, Schirlau K, Sonkoly E, Brandau S, Lang S, Pivarcsi A, et al. A novel mechanism for anti-EGFR antibody action involves chemokine-mediated leukocyte infiltration. Int J Cancer (2009) 124(11):2589–96. doi:10.1002/ijc.24269
141. Ghajar CM, Peinado H, Mori H, Matei IR, Evason KJ, Brazier H, et al. The perivascular niche regulates breast tumour dormancy. Nat Cell Biol (2013) 15(7):807–17. doi:10.1038/ncb2767
142. Krause S, Maffini MV, Soto AM, Sonnenschein C. A novel 3D in vitro culture model to study stromal-epithelial interactions in the mammary gland. Tissue Eng Part C Methods (2008) 14(3):261–71. doi:10.1089/ten.tec.2008.0030
143. Yang CC, Burg KJ. Designing a tunable 3D heterocellular breast cancer tissue test system. J Tissue Eng Regen Med (2015) 9(3):310–4. doi:10.1002/term.1660
144. Derda R, Laromaine A, Mammoto A, Tang SK, Mammoto T, Ingber DE, et al. Paper-supported 3D cell culture for tissue-based bioassays. Proc Natl Acad Sci U S A (2009) 106(44):18457–62. doi:10.1073/pnas.0910666106
145. Derda R, Tang SK, Laromaine A, Mosadegh B, Hong E, Mwangi M, et al. Multizone paper platform for 3D cell cultures. PLoS One (2011) 6(5):e18940. doi:10.1371/journal.pone.0018940
146. Sakaguchi K, Shimizu T, Horaguchi S, Sekine H, Yamato M, Umezu M, et al. In vitro engineering of vascularized tissue surrogates. Sci Rep (2013) 3:1316. doi:10.1038/srep01316
147. Kang YB, Rawat S, Cirillo J, Bouchard M, Noh HM. Layered long-term co-culture of hepatocytes and endothelial cells on a transwell membrane: toward engineering the liver sinusoid. Biofabrication (2013) 5(4):045008. doi:10.1088/1758-5082/5/4/045008
148. Carrion B, Huang CP, Ghajar CM, Kachgal S, Kniazeva E, Jeon NL, et al. Recreating the perivascular niche ex vivo using a microfluidic approach. Biotechnol Bioeng (2010) 107(6):1020–8. doi:10.1002/bit.22891
149. Kim JB, Stein R, O’Hare MJ. Three-dimensional in vitro tissue culture models of breast cancer – a review. Breast Cancer Res Treat (2004) 85(3):281–91. doi:10.1023/B:BREA.0000025418.88785.2b
150. Hirschhaeuser F, Menne H, Dittfeld C, West J, Mueller-Klieser W, Kunz-Schughart LA. Multicellular tumor spheroids: an underestimated tool is catching up again. J Biotechnol (2010) 148(1):3–15. doi:10.1016/j.jbiotec.2010.01.012
151. Kross KW, Heimdal JH, Olsnes C, Olofsson J, Aarstad HJ. Co-culture of head and neck squamous cell carcinoma spheroids with autologous monocytes predicts prognosis. Scand J Immunol (2008) 67(4):392–9. doi:10.1111/j.1365-3083.2008.02072.x
152. Slater SC, Beachley V, Hayes T, Zhang D, Welsh GI, Saleem MA, et al. An in vitro model of the glomerular capillary wall using electrospun collagen nanofibres in a bioartificial composite basement membrane. PLoS One (2011) 6(6):e20802. doi:10.1371/journal.pone.0020802
153. Moraes C, Mehta G, Lesher-Perez SC, Takayama S. Organs-on-a-chip: a focus on compartmentalized microdevices. Ann Biomed Eng (2012) 40(6):1211–27. doi:10.1007/s10439-011-0455-6
154. Byun CK, Hwang H, Choi WS, Yaguchi T, Park J, Kim D, et al. Productive chemical interaction between a bacterial microcolony couple is enhanced by periodic relocation. J Am Chem Soc (2013) 135(6):2242–7. doi:10.1021/ja3094923
155. Nikkhah M, Strobl JS, Schmelz EM, Roberts PC, Zhou H, Agah M. MCF10A and MDA-MB-231 human breast basal epithelial cell co-culture in silicon micro-arrays. Biomaterials (2011) 32(30):7625–32. doi:10.1016/j.biomaterials.2011.06.041
156. Huh D, Matthews BD, Mammoto A, Montoya-Zavala M, Hsin HY, Ingber DE. Reconstituting organ-level lung functions on a chip. Science (2010) 328(5986):1662–8. doi:10.1126/science.1188302
157. Ebrahimkhani MR, Neiman JA, Raredon MS, Hughes DJ, Griffith LG. Bioreactor technologies to support liver function in vitro. Adv Drug Deliv Rev (2014) 6(9–70):132–57. doi:10.1016/j.addr.2014.02.011
158. Lancaster MA, Renner M, Martin CA, Wenzel D, Bicknell LS, Hurles ME, et al. Cerebral organoids model human brain development and microcephaly. Nature (2013) 501(7467):373–9. doi:10.1038/nature12517
159. Sato T, Clevers H. Growing self-organizing mini-guts from a single intestinal stem cell: mechanism and applications. Science (2013) 340(6137):1190–4. doi:10.1126/science.1234852
160. Kim HJ, Boedicker JQ, Choi JW, Ismagilov RF. Defined spatial structure stabilizes a synthetic multispecies bacterial community. Proc Natl Acad Sci U S A (2008) 105(47):18188–93. doi:10.1073/pnas.0807935105
161. van der Meer AD, Orlova VV, ten Dijke P, van den Berg A, Mummery CL. Three-dimensional co-cultures of human endothelial cells and embryonic stem cell-derived pericytes inside a microfluidic device. Lab Chip (2013) 13(18):3562–8. doi:10.1039/c3lc50435b
162. Sounni NE, Noel A. Targeting the tumor microenvironment for cancer therapy. Clin Chem (2013) 59(1):85–93. doi:10.1373/clinchem.2012.185363
163. Mierke CT. The fundamental role of mechanical properties in the progression of cancer disease and inflammation. Rep Prog Phys (2014) 77(7):076602. doi:10.1088/0034-4885/77/7/076602
164. Wojtkowiak JW, Verduzco D, Schramm KJ, Gillies RJ. Drug resistance and cellular adaptation to tumor acidic pH microenvironment. Mol Pharm (2011) 8(6):2032–8. doi:10.1021/mp200292c
165. Roussos ET, Condeelis JS, Patsialou A. Chemotaxis in cancer. Nat Rev Cancer (2011) 11(8):573–87. doi:10.1038/nrc3078
166. Chung S, Sudo R, Mack PJ, Wan CR, Vickerman V, Kamm RD. Cell migration into scaffolds under co-culture conditions in a microfluidic platform. Lab Chip (2009) 9(2):269–75. doi:10.1039/b807585a
167. Brown JM, Wilson WR. Exploiting tumour hypoxia in cancer treatment. Nat Rev Cancer (2004) 4(6):437–47. doi:10.1038/nrc1367
168. Dudley AC. Tumor endothelial cells. Cold Spring Harb Perspect Med (2012) 2(3):a006536. doi:10.1101/cshperspect.a006536
169. Harris AL. Hypoxia – a key regulatory factor in tumour growth. Nat Rev Cancer (2002) 2(1):38–47. doi:10.1038/nrc704
170. Khacho M, Tarabay M, Patten D, Khacho P, MacLaurin JG, Guadagno J, et al. Acidosis overrides oxygen deprivation to maintain mitochondrial function and cell survival. Nat Commun (2014) 5:3550. doi:10.1038/ncomms4550
171. Chiche J, Brahimi-Horn MC, Pouyssegur J. Tumour hypoxia induces a metabolic shift causing acidosis: a common feature in cancer. J Cell Mol Med (2010) 14(4):771–94. doi:10.1111/j.1582-4934.2009.00994.x
172. Helmlinger G, Sckell A, Dellian M, Forbes NS, Jain RK. Acid production in glycolysis-impaired tumors provides new insights into tumor metabolism. Clin Cancer Res (2002) 8(4):1284–91.
173. Vaupel P, Fortmeyer HP, Runkel S, Kallinowski F. Blood flow, oxygen consumption, and tissue oxygenation of human breast cancer xenografts in nude rats. Cancer Res (1987) 47(13):3496–503.
174. Kallinowski F, Schlenger KH, Runkel S, Kloes M, Stohrer M, Okunieff P, et al. Blood flow, metabolism, cellular microenvironment, and growth rate of human tumor xenografts. Cancer Res (1989) 49(14):3759–64.
175. Swaminathan V, Mythreye K, O’Brien ET, Berchuck A, Blobe GC, Superfine R. Mechanical stiffness grades metastatic potential in patient tumor cells and in cancer cell lines. Cancer Res (2011) 71(15):5075–80. doi:10.1158/0008-5472.CAN-11-0247
176. Mouw JK, Yui Y, Damiano L, Bainer RO, Lakins JN, Acerbi I, et al. Tissue mechanics modulate microRNA-dependent PTEN expression to regulate malignant progression. Nat Med (2014) 20(4):360–7. doi:10.1038/nm.3497
177. Hasebe T, Sasaki S, Imoto S, Mukai K, Yokose T, Ochiai A. Prognostic significance of fibrotic focus in invasive ductal carcinoma of the breast: a prospective observational study. Mod Pathol (2002) 15(5):502–16. doi:10.1038/modpathol.3880555
178. Barkan D, El Touny LH, Michalowski AM, Smith JA, Chu I, Davis AS, et al. Metastatic growth from dormant cells induced by a col-I-enriched fibrotic environment. Cancer Res (2010) 70(14):5706–16. doi:10.1158/0008-5472.CAN-09-2356
179. Wilson WR, Hay MP. Targeting hypoxia in cancer therapy. Nat Rev Cancer (2011) 11(6):393–410. doi:10.1038/nrc3064
180. Nyga A, Cheema U, Loizidou M. 3D tumour models: novel in vitro approaches to cancer studies. J Cell Commun Signal (2011) 5(3):239–48. doi:10.1007/s12079-011-0132-4
181. Shin Y, Jeon JS, Han S, Jung GS, Shin S, Lee SH, et al. In vitro 3D collective sprouting angiogenesis under orchestrated ANG-1 and VEGF gradients. Lab Chip (2011) 11(13):2175–81. doi:10.1039/c1lc20039a
182. Chung S, Sudo R, Zervantonakis IK, Rimchala T, Kamm RD. Surface-treatment-induced three-dimensional capillary morphogenesis in a microfluidic platform. Adv Mater (2009) 21(47):4863–7. doi:10.1002/adma.200901727
183. Sudo R, Chung S, Zervantonakis IK, Vickerman V, Toshimitsu Y, Griffith LG, et al. Transport-mediated angiogenesis in 3D epithelial coculture. FASEB J (2009) 23(7):2155–64. doi:10.1096/fj.08-122820
184. Jeon JS, Zervantonakis IK, Chung S, Kamm RD, Charest JL. In vitro model of tumor cell extravasation. PLoS One (2013) 8(2):e56910. doi:10.1371/journal.pone.0056910
185. Jeong GS, Han S, Shin Y, Kwon GH, Kamm RD, Lee SH, et al. Sprouting angiogenesis under a chemical gradient regulated by interactions with an endothelial monolayer in a microfluidic platform. Anal Chem (2011) 83(22):8454–9. doi:10.1021/ac202170e
186. Chung S, Sudo R, Vickerman V, Zervantonakis IK, Kamm RD. Microfluidic platforms for studies of angiogenesis, cell migration, and cell-cell interactions. Sixth international bio-fluid mechanics symposium and workshop March 28-30, 2008 Pasadena, California. Ann Biomed Eng (2010) 38(3):1164–77. doi:10.1007/s10439-010-9899-3
187. Jeong GS, Kwon GH, Kang AR, Jung BY, Park Y, Chung S, et al. Microfluidic assay of endothelial cell migration in 3D interpenetrating polymer semi-network HA-Collagen hydrogel. Biomed Microdevices (2011) 13(4):717–23. doi:10.1007/s10544-011-9541-7
188. Shin Y, Kim H, Han S, Won J, Jeong HE, Lee ES, et al. Extracellular matrix heterogeneity regulates three-dimensional morphologies of breast adenocarcinoma cell invasion. Adv Healthc Mater (2013) 2(6):790–4. doi:10.1002/adhm.201200320
189. Bersini S, Jeon JS, Dubini G, Arrigoni C, Chung S, Charest JL, et al. A microfluidic 3D in vitro model for specificity of breast cancer metastasis to bone. Biomaterials (2014) 35(8):2454–61. doi:10.1016/j.biomaterials.2013.11.050
190. Rizvi I, Gurkan UA, Tasoglu S, Alagic N, Celli JP, Mensah LB, et al. Flow induces epithelial-mesenchymal transition, cellular heterogeneity and biomarker modulation in 3D ovarian cancer nodules. Proc Natl Acad Sci U S A (2013) 110(22):E1974–83. doi:10.1073/pnas.1216989110
191. Bhatia SN, Ingber DE. Microfluidic organs-on-chips. Nat Biotechnol (2014) 32(8):760–72. doi:10.1038/nbt.2989
192. Ingram M, Techy GB, Ward BR, Imam SA, Atkinson R, Ho H, et al. Tissue engineered tumor models. Biotech Histochem (2010) 85(4):213–29. doi:10.3109/10520295.2010.483655
193. Li XJ, Valadez AV, Zuo P, Nie Z. Microfluidic 3D cell culture: potential application for tissue-based bioassays. Bioanalysis (2012) 4(12):1509–25. doi:10.4155/bio.12.133
194. Xu Z, Gao Y, Hao Y, Li E, Wang Y, Zhang J, et al. Application of a microfluidic chip-based 3D co-culture to test drug sensitivity for individualized treatment of lung cancer. Biomaterials (2013) 34(16):4109–17. doi:10.1016/j.biomaterials.2013.02.045
195. Hwang H, Park J, Shin C, Do Y, Cho YK. Three dimensional multicellular co-cultures and anti-cancer drug assays in rapid prototyped multilevel microfluidic devices. Biomed Microdevices (2013) 15(4):627–34. doi:10.1007/s10544-012-9733-9
196. Fischbach C, Kong HJ, Hsiong SX, Evangelista MB, Yuen W, Mooney DJ. Cancer cell angiogenic capability is regulated by 3D culture and integrin engagement. Proc Natl Acad Sci U S A (2009) 106(2):399–404. doi:10.1073/pnas.0808932106
197. Marushima H, Shibata S, Asakura T, Matsuura T, Maehashi H, Ishii Y, et al. Three-dimensional culture promotes reconstitution of the tumor-specific hypoxic microenvironment under TGFbeta stimulation. Int J Oncol (2011) 39(5):1327–36. doi:10.3892/ijo.2011.1142
198. DelNero P, Lane M, Verbridge SS, Kwee B, Kermani P, Hempstead B, et al. 3D culture broadly regulates tumor cell hypoxia response and angiogenesis via pro-inflammatory pathways. Biomaterials (2015) 55:110–8. doi:10.1016/j.biomaterials.2015.03.035
199. Kim JW, Ho WJ, Wu BM. The role of the 3D environment in hypoxia-induced drug and apoptosis resistance. Anticancer Res (2011) 31(10):3237–45.
200. Infanger DW, Lynch ME, Fischbach C. Engineered culture models for studies of tumor-microenvironment interactions. Annu Rev Biomed Eng (2013) 15:29–53. doi:10.1146/annurev-bioeng-071811-150028
201. Ma H, Xu H, Qin J. Biomimetic tumor microenvironment on a microfluidic platform. Biomicrofluidics (2013) 7(1):11501. doi:10.1063/1.4774070
202. Young EW. Cells, tissues, and organs on chips: challenges and opportunities for the cancer tumor microenvironment. Integr Biol (Camb) (2013) 5(9):1096–109. doi:10.1039/c3ib40076j
203. Wagner I, Materne EM, Brincker S, Süssbier U, Frädrich C, Busek M, et al. A dynamic multi-organ-chip for long-term cultivation and substance testing proven by 3D human liver and skin tissue co-culture. Lab Chip (2013) 13(18):3538–47. doi:10.1039/c3lc50234a
204. Haessler U, Kalinin Y, Swartz MA, Wu MW. An agarose-based microfluidic platform with a gradient buffer for 3D chemotaxis studies. Biomed Microdevices (2009) 11(4):827–35. doi:10.1007/s10544-009-9299-3
205. Lee PJ, Hung PJ, Lee LP. An artificial liver sinusoid with a microfluidic endothelial-like barrier for primary hepatocyte culture. Biotechnol Bioeng (2007) 97(5):1340–6. doi:10.1002/bit.21360
206. Esch MB, Sung JH, Yang J, Yu C, Yu J, March JC, et al. On chip porous polymer membranes for integration of gastrointestinal tract epithelium with microfluidic ‘body-on-a-chip’ devices. Biomed Microdevices (2012) 14(5):895–906. doi:10.1007/s10544-012-9669-0
207. Kim HJ, Huh D, Hamilton G, Ingber DE. Human gut-on-a-chip inhabited by microbial flora that experiences intestinal peristalsis-like motions and flow. Lab Chip (2012) 12(12):2165–74. doi:10.1039/c2lc40074j
208. Cimetta E, Cannizzaro C, James R, Biechele T, Moon RT, Elvassore N, et al. Microfluidic device generating stable concentration gradients for long term cell culture: application to Wnt3a regulation of beta-catenin signaling. Lab Chip (2010) 10(23):3277–83. doi:10.1039/c0lc00033g
209. Shin Y, Yang K, Han S, Park HJ, Seok Heo Y, Cho SW, et al. Reconstituting vascular microenvironment of neural stem cell niche in three-dimensional extracellular matrix. Adv Healthc Mater (2014) 3(9):1457–64. doi:10.1002/adhm.201300569
210. Han S, Yang K, Shin Y, Lee JS, Kamm RD, Chung S, et al. Three-dimensional extracellular matrix-mediated neural stem cell differentiation in a microfluidic device. Lab Chip (2012) 12(13):2305–8. doi:10.1039/c2lc21285d
211. DelNero P, Song YH, Fischbach C. Microengineered tumor models: insights & opportunities from a physical sciences-oncology perspective. Biomed Microdevices (2013) 15(4):583–93. doi:10.1007/s10544-013-9763-y
212. Ghaemmaghami AM, Hancock MJ, Harrington H, Kaji H, Khademhosseini A. Biomimetic tissues on a chip for drug discovery. Drug Discov Today (2012) 17(3–4):173–81. doi:10.1016/j.drudis.2011.10.029
213. Huh D, Hamilton GA, Ingber DE. From 3D cell culture to organs-on-chips. Trends Cell Biol (2011) 21(12):745–54. doi:10.1016/j.tcb.2011.09.005
214. Park SH, Sim WY, Min BH, Yang SS, Khademhosseini A, Kaplan DL. Chip-based comparison of the osteogenesis of human bone marrow- and adipose tissue-derived mesenchymal stem cells under mechanical stimulation. PLoS One (2012) 7(9):e46689. doi:10.1371/journal.pone.0046689
215. Horch RE, Boos AM, Quan Y, Bleiziffer O, Detsch R, Boccaccini AR, et al. Cancer research by means of tissue engineering – is there a rationale? J Cell Mol Med (2013) 17(10):1197–206. doi:10.1111/jcmm.12130
216. Garraway LA, Janne PA. Circumventing cancer drug resistance in the era of personalized medicine. Cancer Discov (2012) 2(3):214–26. doi:10.1158/2159-8290.CD-12-0012
217. Gordon RR, Nelson PS. Cellular senescence and cancer chemotherapy resistance. Drug Resist Updat (2012) 15(1–2):123–31. doi:10.1016/j.drup.2012.01.002
218. Chaffer CL, Weinberg RA. A perspective on cancer cell metastasis. Science (2011) 331(6024):1559–64. doi:10.1126/science.1203543
219. Valastyan S, Weinberg RA. Tumor metastasis: molecular insights and evolving paradigms. Cell (2011) 147(2):275–92. doi:10.1016/j.cell.2011.09.024
220. Physical Sciences – Oncology Centers Network, Agus DB, Alexander JF, Arap W, Ashili S, Aslan JE, et al. A physical sciences network characterization of non-tumorigenic and metastatic cells. Sci Rep (2013) 3:1449. doi:10.1038/srep01449
221. Gupta PB, Fillmore CM, Jiang G, Shapira SD, Tao K, Kuperwasser C, et al. Stochastic state transitions give rise to phenotypic equilibrium in populations of cancer cells (vol 146, pg 633, 2011). Cell (2011) 147(5):1197–1197. doi:10.1016/j.cell.2011.07.026
222. Findlay VJ, Wang C, Watson DK, Camp ER. Epithelial-to-mesenchymal transition and the cancer stem cell phenotype: insights from cancer biology with therapeutic implications for colorectal cancer. Cancer Gene Ther (2014) 21(5):181–7. doi:10.1038/cgt.2014.15
223. Friedl P, Locker J, Sahai E, Segall JE. Classifying collective cancer cell invasion. Nat Cell Biol (2012) 14(8):777–83. doi:10.1038/ncb2548
224. Balaban RS, Hampshire VA. Challenges in small animal noninvasive imaging. ILAR J (2001) 42(3):248–62. doi:10.1093/ilar.42.3.248
225. Zhang X, Lin Y, Gillies RJ. Tumor pH and its measurement. J Nucl Med (2010) 51(8):1167–70. doi:10.2967/jnumed.109.068981
226. Harisinghani MG, Barentsz J, Hahn PF, Deserno WM, Tabatabaei S, van de Kaa CH, et al. Noninvasive detection of clinically occult lymph-node metastases in prostate cancer. N Engl J Med (2003) 348(25):2491–9. doi:10.1056/NEJMoa022749
227. Bipat S, van Leeuwen MS, Comans EF, Pijl ME, Bossuyt PM, Zwinderman AH, et al. Colorectal liver metastases: CT, MR imaging, and PET for diagnosis – meta-analysis. Radiology (2005) 237(1):123–31. doi:10.1148/radiol.2371042060
228. Judenhofer MS, Wehrl HF, Newport DF, Catana C, Siegel SB, Becker M, et al. Simultaneous PET-MRI: a new approach for functional and morphological imaging. Nat Med (2008) 14(4):459–65. doi:10.1038/nm1700
229. Serres S, Soto MS, Hamilton A, McAteer MA, Carbonell WS, Robson MD, et al. Molecular MRI enables early and sensitive detection of brain metastases. Proc Natl Acad Sci U S A (2012) 109(17):6674–9. doi:10.1073/pnas.1117412109
230. Frese KK, Tuveson DA. Maximizing mouse cancer models. Nat Rev Cancer (2007) 7(9):645–58. doi:10.1038/nrc2192
231. Germain RN, Miller MJ, Dustin ML, Nussenzweig MC. Dynamic imaging of the immune system: progress, pitfalls and promise. Nat Rev Immunol (2006) 6(7):497–507. doi:10.1038/nri1884
232. Cahalan MD, Parker I, Wei SH, Miller MJ. Two-photon tissue imaging: seeing the immune system in a fresh light. Nat Rev Immunol (2002) 2(11):872–80. doi:10.1038/nri935
233. Rueckel M, Mack-Bucher JA, Denk W. Adaptive wavefront correction in two-photon microscopy using coherence-gated wavefront sensing. Proc Natl Acad Sci U S A (2006) 103(46):17137–42. doi:10.1073/pnas.0604791103
234. Condeelis J, Segall JE. Intravital imaging of cell movement in tumours. Nat Rev Cancer (2003) 3(12):921–30. doi:10.1038/nrc1231
235. Helmchen F, Denk W. Deep tissue two-photon microscopy. Nat Methods (2005) 2(12):932–40. doi:10.1038/nmeth818
236. Wyckoff JB, Wang Y, Lin EY, Li JF, Goswami S, Stanley ER, et al. Direct visualization of macrophage-assisted tumor cell intravasation in mammary tumors. Cancer Res (2007) 67(6):2649–56. doi:10.1158/0008-5472.CAN-06-1823
237. Alexander S, Weigelin B, Winkler F, Friedl P. Preclinical intravital microscopy of the tumour-stroma interface: invasion, metastasis, and therapy response. Curr Opin Cell Biol (2013) 25(5):659–71. doi:10.1016/j.ceb.2013.07.001
238. Tanner K, Mori H, Mroue R, Bruni-Cardoso A, Bissell MJ. Coherent angular motion in the establishment of multicellular architecture of glandular tissues. Proc Natl Acad Sci U S A (2012) 109(6):1973–8. doi:10.1073/pnas.1119578109
239. Wong AD, Searson PC. Live-cell imaging of invasion and intravasation in an artificial microvessel platform. Cancer Res (2014) 74(17):4937–45. doi:10.1158/0008-5472.CAN-14-1042
240. Chen MB, Whisler JA, Jeon JS, Kamm RD. Mechanisms of tumor cell extravasation in an in vitro microvascular network platform. Integr Biol (Camb) (2013) 5(10):1262–71. doi:10.1039/c3ib40149a
241. Zervantonakis IK, Hughes-Alford SK, Charest JL, Condeelis JS, Gertler FB, Kamm RD. Three-dimensional microfluidic model for tumor cell intravasation and endothelial barrier function. Proc Natl Acad Sci U S A (2012) 109(34):13515–20. doi:10.1073/pnas.1210182109
242. Sakaue-Sawano A, Kurokawa H, Morimura T, Hanyu A, Hama H, Osawa H, et al. Visualizing spatiotemporal dynamics of multicellular cell-cycle progression. Cell (2008) 132(3):487–98. doi:10.1016/j.cell.2007.12.033
243. Hoffman RM. In vivo cell biology of cancer cells visualized with fluorescent proteins. Curr Top Dev Biol (2005) 70:121–44. doi:10.1016/S0070-2153(05)70006-5
244. Yu H, Mouw JK, Weaver VM. Forcing form and function: biomechanical regulation of tumor evolution. Trends Cell Biol (2011) 21(1):47–56. doi:10.1016/j.tcb.2010.08.015
245. Plodinec M, Loparic M, Monnier CA, Obermann EC, Zanetti-Dallenbach R, Oertle P, et al. The nanomechanical signature of breast cancer. Nat Nanotechnol (2012) 7(11):757–65. doi:10.1038/nnano.2012.167
246. Legant WR, Choi CK, Miller JS, Shao L, Gao L, Betzig E, et al. Multidimensional traction force microscopy reveals out-of-plane rotational moments about focal adhesions. Proc Natl Acad Sci U S A (2013) 110(3):881–6. doi:10.1073/pnas.1207997110
247. Gardel ML, Kasza KE, Brangwynne CP, Liu J, Weitz DA. Chapter 19: Mechanical response of cytoskeletal networks. Methods Cell Biol (2008) 89: 487–519. doi:10.1016/S0091-679X(08)00619-5
248. Blehm BH, Schroer TA, Trybus KM, Chemla YR, Selvin PR. In vivo optical trapping indicates kinesin’s stall force is reduced by dynein during intracellular transport. Proc Natl Acad Sci U S A (2013) 110(9):3381–6. doi:10.1073/pnas.1219961110
249. Korolev KS, Xavier JB, Gore J. Turning ecology and evolution against cancer. Nat Rev Cancer (2014) 14(5):371–80. doi:10.1073/pnas.1219961110
250. Sosa MS, Bragado P, Aguirre-Ghiso JA. Mechanisms of disseminated cancer cell dormancy: an awakening field. Nat Rev Cancer (2014) 14(9):611–22. doi:10.1038/nrc3793
251. Husemann Y, Geigl JB, Schubert F, Musiani P, Meyer M, Burghart E, et al. Systemic spread is an early step in breast cancer. Cancer Cell (2008) 13(1):58–68. doi:10.1016/j.ccr.2007.12.003
252. Scheffer M, Carpenter SR, Lenton TM, Bascompte J, Brock W, Dakos V, et al. Anticipating critical transitions. Science (2012) 338(6105):344–8. doi:10.1126/science.1225244
253. van Nes EH, Scheffer M. Implications of spatial heterogeneity for catastrophic regime shifts in ecosystems. Ecology (2005) 86(7):1797–807. doi:10.1890/04-0550
254. Dunne JA, Williams RJ, Martinez ND. Network structure and biodiversity loss in food webs: robustness increases with connectance. Ecol Lett (2002) 5(4):558–67. doi:10.1371/journal.pbio.1001579
255. Katz E, Dubois-Marshall S, Sims AH, Gautier P, Caldwell H, Meehan RR, et al. An in vitro model that recapitulates the epithelial to mesenchymal transition (EMT) in human breast cancer. PLoS One (2011) 6(2):e17083. doi:10.1371/journal.pone.0017083
256. Li Q, Chen C, Kapadia A, Zhou Q, Harper MK, Schaack J, et al. 3D models of epithelial-mesenchymal transition in breast cancer metastasis: high-throughput screening assay development, validation, and pilot screen. J Biomol Screen (2011) 16(2):141–54. doi:10.1177/1087057110392995
257. Moreno-Bueno G, Peinado H, Molina P, Olmeda D, Cubillo E, Santos V, et al. The morphological and molecular features of the epithelial-to-mesenchymal transition. Nat Protoc (2009) 4(11):1591–613. doi:10.1038/nprot.2009.152
258. Ben-Jacob E, Coffey DS, Levine H. Bacterial survival strategies suggest rethinking cancer cooperativity. Trends Microbiol (2012) 20(9):403–10. doi:10.1016/j.tim.2012.06.001
259. Liu X, Johnson S, Liu S, Kanojia D, Yue W, Singh UP, et al. Nonlinear growth kinetics of breast cancer stem cells: implications for cancer stem cell targeted therapy. Sci Rep (2013) 3:2473. doi:10.1038/srep02473
260. Sprouffske K, Pepper JW, Maley CC. Accurate reconstruction of the temporal order of mutations in neoplastic progression. Cancer Prev Res (Phila) (2011) 4(7):1135–44. doi:10.1158/1940-6207.CAPR-10-0374
261. Attolini CS, Cheng YK, Beroukhim R, Getz G, Abdel-Wahab O, Levine RL, et al. A mathematical framework to determine the temporal sequence of somatic genetic events in cancer. Proc Natl Acad Sci U S A (2010) 107(41):17604–9. doi:10.1073/pnas.1009117107
262. Michor F, Hughes TP, Iwasa Y, Branford S, Shah NP, Sawyers CL, et al. Dynamics of chronic myeloid leukaemia. Nature (2005) 435(7046):1267–70. doi:10.1038/nature03669
263. Bozic I, Reiter JG, Allen B, Antal T, Chatterjee K, Shah P, et al. Evolutionary dynamics of cancer in response to targeted combination therapy. Elife (2013) 2:e00747. doi:10.7554/eLife.00747
264. Cunningham JJ, Gatenby RA, Brown JS. Evolutionary dynamics in cancer therapy. Mol Pharm (2011) 8(6):2094–100. doi:10.1021/mp2002279
265. Frieboes HB, Zheng X, Sun CH, Tromberg B, Gatenby R, Cristini V. An integrated computational/experimental model of tumor invasion. Cancer Res (2006) 66(3):1597–604. doi:10.1158/0008-5472.CAN-05-3166
Keywords: tumor microenvironment, 3D culture models, ecology, biomaterials and nanotechnology, hydrogels
Citation: Kim J and Tanner K (2015) Recapitulating the tumor ecosystem along the metastatic cascade using 3D culture models. Front. Oncol. 5:170. doi: 10.3389/fonc.2015.00170
Received: 09 March 2015; Accepted: 08 July 2015;
Published: 29 July 2015
Edited by:
Victoria Virador, Virador and Associates, USAReviewed by:
Silvia Pastorekova, Slovak Academy of Sciences, SlovakiaKeith R. Laderoute, SRI International, USA
Michelle Annette Digman, University of California Irvine, USA
Copyright: © 2015 Kim and Tanner. This is an open-access article distributed under the terms of the Creative Commons Attribution License (CC BY). The use, distribution or reproduction in other forums is permitted, provided the original author(s) or licensor are credited and that the original publication in this journal is cited, in accordance with accepted academic practice. No use, distribution or reproduction is permitted which does not comply with these terms.
*Correspondence: Kandice Tanner, Laboratory of Cell Biology, Center for Cancer Research, National Cancer Institute (NIH), 37 Convent Drive, Bethesda, MD 20892, USA,a2FuZGljZS50YW5uZXJAbmloLmdvdg==