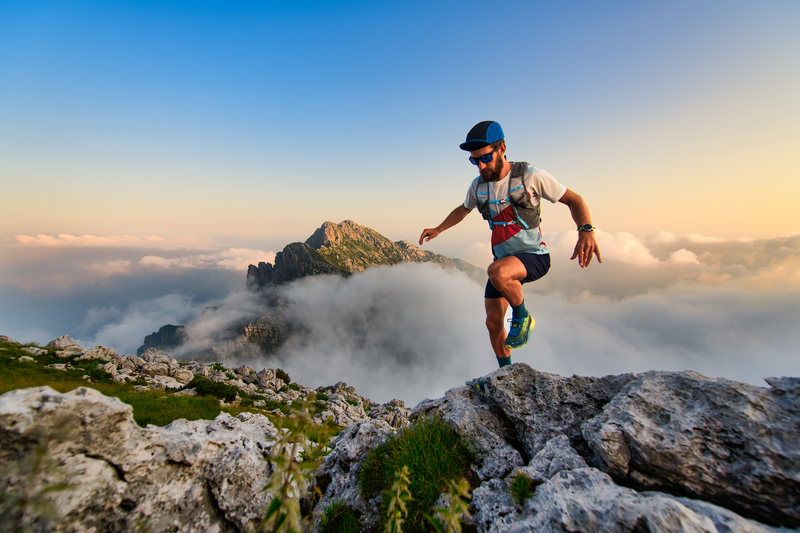
94% of researchers rate our articles as excellent or good
Learn more about the work of our research integrity team to safeguard the quality of each article we publish.
Find out more
REVIEW article
Front. Nucl. Eng. , 23 February 2024
Sec. Nuclear Reactor Design
Volume 3 - 2024 | https://doi.org/10.3389/fnuen.2024.1355630
This article is part of the Research Topic Artificial Intelligence in Advanced Nuclear Reactor Design View all 6 articles
Nuclear power plants produce a massive amount of clean energy and necessitate safe operation through intelligence technologies. Recently, the rapid advancements in communication infrastructures including artificial intelligence, big data computing, and Internet of Things devices moving the nuclear industries towards digitalization and intelligence to improve safety. The integration of these technologies into the nuclear sector offers effective tactics in addressing several challenges in the control and safe operation of nuclear power plants. This can be achieved through the insights generated from massive amounts of data. This paper comprehensively reviews the literature on artificial intelligence technologies and big data, seeking to provide a holistic perspective on their relations and how they can be integrated with nuclear power plants. The utilization of computing platforms boosts the deployment of artificial intelligence and big data analytics effectively in nuclear power plants. Further, this review also points out the future opportunities as well as challenges for applying artificial intelligence and big data computing in the nuclear industry.
The world economy is rapidly growing, and low-carbon policies are being promoted globally. The goal of these policies is to reduce the consumption of high-carbon assets as well as the emissions of greenhouse gases as much as possible to ensure environmental safety through the use of clean energy (Nian et al., 2022). In recent years, the need for non-fossil energy is increasing worldwide to fulfill various services in diverse sectors including heat production (Cleveland and McDonald, 2008; Upadhyaya and Kerlin, 2019; International Energy Agency, 2022; OECD and Nuclear Energy Agency, 2022), hydrogen production (Kalyakin et al., 2016; Balanin and Fomichenko, 2023; Okunlola et al., 2023; Tanbay and Durmayaz, 2023), water desalination (Khan et al., 2018; Rosen and Farsi, 2022), and space applications (Li et al., 2020; Chen et al., 2022; Peakman and Lindley, 2023; Zhang, 2023). Unlike fossil fuels, clean energy sources minimize the emission of greenhouse gases. Consequently, increasing the use of non-fossil sources of energy decreases the overall greenhouse gas emissions. The energy obtained from geothermal, wind, hydro, and solar are examples of non-fossil energy. Nevertheless, these sources of energy are unstable due to several reasons such as intermittency, volatility, and environmental effects (Li et al., 2015; Yao et al., 2016). In such situations, nuclear energy can be deployed as a decisive contributor and powerful alternative to offer a steady source of electricity for multiplying human labor to maximize productivity (Huang et al., 2023).
Nuclear energy is a kind of clean energy source that has received immense popularity and advancement for global electrification. It is a stable base-load and zero-carbon energy source, that can be leveraged as a powerful and stable supply of electricity (Basu and Miroshnik, 2019). It produces around 10% of the world’s electricity according to the IAEA estimation in 2019 (Birol, 2019). The distribution of this energy production varies significantly by country. It produces clean energy that plays a significant role in minimizing carbon emissions in order to reduce globalization (Hong et al., 2014). Fossil fuel energy massively pollutes the environment and contributes to the emission of greenhouse gases (Song et al., 2022). Besides, nuclear energy is cost-effective when compared with fossil fuel energy, and therefore, it has a pivotal role in the transition away from fossil fuel energy sources. More, civilian nuclear technologies are essential to maintain national security and energy diplomacy. It fosters harmonious relations among countries and opens up new opportunities in the nuclear business (Greg Hands, 2022). However, it is important to acknowledge that nuclear energy also concerns several issues including severe accidents and management of radioactive waste.
NPPs are large power industries that consist of numerous subsystems. These components involve time-dependent variables and face malfunctions. Thus, the operation and management of NPPs are complex issues. Instrumentation plays a significant part in the safe and efficient operation of nuclear reactors. It encompasses the use of various instruments to measure and monitor various parameters within the reactor. The common and essential instrumentation systems in a nuclear reactor include the measurements of power, temperature, pressure, flow rate, and radiation (Singh and Singh, 2021). Further, the instrumentation of a control system is deployed to handle the reactor power for maintaining stable and safe reactor conditions (Xi et al., 2020). Overall, instrumentation in nuclear reactors undergoes demanding design, calibration, and testing processes to ensure accuracy, reliability, and compliance with safety regulations. Nuclear regulatory organizations set specific requirements for instrumentation systems to maintain safe and secure reactor operations.
Different types of NPPs designs are in operation throughout the world for several applications such as heat generation, space application, and water desalination (Murakami, 2021). The PWR NPP is the most common reactor design which has several benefits over other types of reactors. It is simple to operate and uses water for cooling and neutron moderation. Further, the PWR core consists of fewer fissile materials, making the reactor safer and easier to manage. The NPPs are an integration of different components such as core, steam generator, pipings, plenums, and allied subsystems (Kerlin and Upadhyaya, 2019a). These systems should perform their functions to generate electricity. Overall, NPPs are nonlinear systems that integrate multiple fields including material science, nuclear physics, fluid dynamics, heat transfer, and radiation. The NPPs indeed generate a vast amount of data during operation. The data are important for optimization to increase the safety and efficiency of the reactors. The remaining sections of this paper are organized as follows: Section 2 offers an overview of the big data sources, while Section 3 investigates the application of AI techniques in NPPs. Section 4 explores the collaborative application of big data and AI technologies in NPPs. Section 5 addresses the challenges and opportunities presented by big data and AI technologies in the nuclear research sector. Finally, Section 6 recaps the conclusion of the study.
This section identifies the sources of big data for NPPs. Big data are extensive volumes of datasets that can not be managed, processed, and analyzed using traditional processing mechanisms easily (Dagan and Wilkins, 2023). The NPPs produce huge amounts of heterogeneous operational data. It involves diverse datasets that describe the characteristics of the NPPs and arisen the opportunity for understanding the system better and producing innovative applications according to the dataset. Big data technologies enable the collection, storage, and integration of this data from diverse sources to analyze easily for improved decision-making. The NPPs data is collected from various sources such as mathematical modeling, software, experiments, and plant sensors. A brief description of each of these data sources is provided in the following subsections.
The NPPs big data could be gathered from mathematical model. The NPPs model is developed using the first principle approach based on fundamental physical laws and assumptions (Vajpayee et al., 2020). It is a valuable and easily accessible data source during the lack of real observations of the NPPs. Big data incorporates diverse data formats and types, including structured, semi-structured, and unstructured data. The dataset is then stored in the database and enough amount of data should be extracted for the intended applications. The PWR model is utilized to generate the necessary amount of data for the prediction of transients under reactivity and inlet coolant temperature perturbations (Ejigu and Liu, 2023). The NPPs system dynamics model is established for studying the transients and designing a risk assessment platform (El-Sefy et al., 2019). Further, the data produced during simulation is employed for ANN training to estimate the NPP behavior and demonstrate the potential of AI in risk mitigation strategies (El-Sefy et al., 2021).
The mathematical model development for the NPPs also assists the plant operator in understanding transients and achieving the necessary safe operation. The solution of the model is obtained by system simulation through MATLAB and Python. The simulation offers several advantages including gaining fundamental concepts of the NPPs dynamics during transients, analyzing the transient of the NPP under normal maneuvering and accident situations, and plant operator training (Kerlin and Upadhyaya, 2019b). However, due to its nonlinearity characteristic, an accurate mathematical model development of the NPPs is a challenging task. The mathematical model of the reactors should be reasonably accurate and simple to accomplish the objectives.
The big data for NPPs is also collected from different software platforms. These sources can offer information for estimation, prediction, safety analysis, and maintenance in the NPPs. However, the availability and accessibility of NPPs data from software sources may vary depending on several factors including security restrictions, regulations, and user permissions. The NPPs data could be collected from RELAP5 thermal-hydraulics codes. It is applied to model the coupled behavior of the primary and secondary systems under various operational conditions. This modeling tool is also used to study the transients of the NPPs (Li R. et al., 2022). It is also employed to model natural circulation flow in the PWR fuel (Ni et al., 2021), estimation of the countercurrent flow in the downcomer (Li et al., 2023a), analysis of loss of flow accidents (Corzo et al., 2023), and study rod ejection accidents (El-Sahlamy et al., 2022). Further, the NPPs data is generated from the STAR-CCM+ CFD simulation tool for several applications (Marfaing et al., 2018; Benavides et al., 2020; Zhang et al., 2021; Yang et al., 2023). Besides, the big data of the NPPs could be collected from education and training simulators. These simulators offer an easy and effective means of examining the physics and engineering designs of multiple kinds of NPPs. Furthermore, the simulators are useful for both technical and non-technical individuals as introductory instructional tools. The IAEA provides several kinds of NPP simulators (Cabellos et al., 2018; Developing a Systematic Education and Training Approach, 2018).
The big data of the NPPs could be collected by conducting experiments. It is carried out in the laboratory to collect comprehensive and robust datasets with the help of apparatus. Experimental data sources can vary widely depending on research goals, and available resources. Experiments in nuclear engineering are performed for different applications (Geslot et al., 2023; Guillen et al., 2023; Zhang et al., 2023).
Sensor data is produced when an instrument recognizes and reacts to some form of physical input. These are the real sources of data (Schokker et al., 2022). The NPPs consist of numerous sensors and a tremendous volume of data could be collected by sensors from the plant site which is recorded over time and continuously. This data provides valuable insights for several applications in the estimation and control of reactor variables. The sensor is employed to regulate core outlet temperature in NPPs (Hyer et al., 2023) and measure the position of the control rod in a nuclear reactor (Hu et al., 2020).
Data mining includes data collection, preparation, and analysis. The purpose of data mining is to extract new information and relationships from the existing raw data. The data collected from the NPPs need preprocessing to ensure accurate, and efficient application. Data preprocessing techniques prepare the raw dataset for suitable model building and AI algorithm training to provide the desired output. Numerous kinds of statistical approaches are employed for preprocessing the NPPs big data. The common data preprocessing techniques include smoothing, cleaning, normalization, and removing (Morrisset et al., 2022). Figure 1 illustrates the procedures undertaken from NPPs big data collection until the required services are obtained in a flowchart. Further, Table 1 presents some big data mining methods.
FIGURE 1. NPPs big data services in a flowchart (Barja-Martinez et al., 2021; Boring et al., 2022).
The key roles of big data are big data engineering and analytics. Figure 2 presents a summary of these functions. Big data engineering incorporates essential steps for data collection, management, and regularization. The key components of big data engineering are acquisition, processing, storage, databases, and pipeline. Big data analytics are used to categorize, characterize, consolidate, predict, infer, and classify data to provide meaningful information. Prediction, classification, clustering, inference, and optimization can be summarized as the core operations, while statistics, data mining, expert knowledge, machine learning, and deep learning are frequently applied techniques (Li F. et al., 2023).
Big data can impact NPPs (Lorenz and Schmidt, 1986). The relationship between NPPs and big data lies in the potential application of big data computing techniques to enhance the performance, safety, and efficiency of NPPs. In order to address the intended application, traditional big data analysis tools face limitations. These drawbacks could be overcome through AI algorithms, that can process and handle the NPPs big data with fast computations. Scholars are creating and employing AI algorithms by considering the potential of big data collected from NPPs.
Recently, the concept of AI has gained popularity in a variety of disciplines for several applications such as prediction, maintenance, control design, fault detection, and safety analysis. AI is a wide and interdisciplinary research field that programs machines to think and learn to solve several real-world engineering problems and improve decision-making abilities. It simulates human intelligence by using computer systems capable of performing a variety of activities. However, the application of AI in the NPPs is in the early stages. Hence, it needs extensive research for ensuring the safety and reliability of the NPPs. With the progress in the development of advanced sensors and digitalization together with communication technologies, NPPs are accelerating the transition towards intelligence through the use of AI algorithms to enhance safety, efficiency, and performance (Huang et al., 2023).
The relationship between AI and NPPs is multifaceted. AI can enhance efficiency in power generation and consumption, and raise ethical and governance considerations. It is important to attach the potential of AI while carefully navigating its impact on power dynamics in society. AI algorithms process the PWR NPPs data to detect anomalies in order to take early preventive actions. It also analyzes a large amount of PWR NPPs data to predict the transients and forecast the future response to improve overall reliability (Ejigu and Liu, 2023). The integration of NPPs and AI necessitates careful considerations in safety regulations and cybersecurity. AI algorithms are also employed to detect operator errors and schedule maintenance in the NPPs (Gursel et al., 2023). It requires Internet of Things (IoT) devices to share information. Figure 3 depicts the integration of NPPs big data, AI, and IoT platforms. As illustrated in the figure, the IoT devices are incorporated to share data and information between the NPPs data and AI techniques to perform different services such as control and estimation.
AI has the potential to make the PWR NPPs autonomous by minimizing energy waste and reducing coat. It improves the operation of the power system and promotes clean and renewable sources of energy (Song et al., 2022). Besides, AI is applied in the promotion of clean energy sources through forecasting, stability, reliability, management, optimization distribution, and consumption (Barja-Martinez et al., 2021). Numerous AI algorithms such as GA, PSO, BAS, ML, ANN, and DL are employed in different reactor designs for optimization and prediction.
GA is an evolutionary mechanism that works based on natural selection. The basic idea of the GA starts with the population for the potential solution of a complex problem that evolves iteratively over generations. The GA is applied for the optimization of load and reloading of fuel assemblies in the nuclear reactor core (Sobolev et al., 2017). It is also employed for designing and simulating safe and effective fuel-loading patterns in nuclear reactors (Zhao et al., 1998). Further, the GA is utilized for designing efficient radiation shielding in SMR (Bagheri and Khalafi, 2023), development of an optimized thermodynamic model in a VVER-1200 reactor (Khan et al., 2022), optimal energy management in the HTGR (Sun J. et al., 2022), optimization of fuel loading pattern in the experimental fast reactor (Lima-Reinaldo and François, 2023), in-core fuel management in the PWR (Rodrigues et al., 2022) and optimal design of a core in VVER-1000 nuclear reactor (Kianpour et al., 2020). Yet, the GA shows limitations in both too small and large-scale population sizes to converge into optimal solutions (Cavallaro et al., 2024). Figure 4 demonstrates the overall steps of the GA in a flowchart.
FIGURE 4. The workflow of the GA (Mousakazemi, 2020).
PSO is a metaheuristic algorithm inspired by a group of animals. The concept of swarm intelligence is based on the social collaboration of individuals to learn with their own experience in a group. It is applied to optimize fuel reloading problems in PWR (Meneses et al., 2009), optimization of fuel core loading pattern in a VVER nuclear reactor (Babazadeh et al., 2009), optimization of secondary circuit system in marine NPP (Zhao et al., 2023), optimize the design parameters of radiation shielding system material (Lei et al., 2023), optimization of control drum operation for a microreactor under normal and transient conditions (Price et al., 2022), and designing of space nuclear reactor fuel (Rafiei and Ansarifar, 2022). Besides, the PSO mechanism is employed in NPPs for fault diagnosis (Wang H. et al., 2021), designing maintenance and safety systems (Wang J. et al., 2021), and control system development (Coban, 2011; Safarzadeh and Noori-kalkhoran, 2021; Ejigu and Liu, 2022a; Ayele Ejigu and Liu, 2022; Muthuraj et al., 2023). However, in a high-dimensional search space, the PSO tactic converges slowly toward the optimal solution and produces poor results (Bucz et al., 2018). In order to overcome this limitation, the PSO is combined with the GA (Rahnama and Ansarifar, 2021) and GD (Ejigu and Liu, 2022a) algorithms. Figure 5 displays the overall procedures of the PSO algorithm in a flowchart.
FIGURE 5. The flowchart of the PSO algorithm (Pant and Chatterjee, 2020).
The BAS is a metaheuristic algorithm that works with the foraging principle of the beetles using two antennae. The two antennae of the beetle explore the food odor randomly in the nearby area. The beetle takes a step towards the strong odor concentration using the two antennae. The searching performance of the beetles using two antennae could be used to formulate an optimization algorithm (Jiang and Li, 2017). It is employed for estimation (Xie et al., 2019; Zivkovic et al., 2021), fault detection (Huang et al., 2020), control system optimization (Fan et al., 2019), and cooperative and constrained control design (Ejigu and Liu, 2022b). Recently, the GA, PSO, and BAS algorithms have given more attention to training ANN algorithms for different applications (Li, 2020; Vasumathi and Moorthi, 2012; da Silva Veloso et al., 2020; Yadav and Anubhav, 2020; Jamali et al., 2019). However, the BAS algorithm faces several shortcomings as reported in Ref. (He et al., 2022). Figure 6 summarizes the working principle of the BAS optimization algorithm in a flowchart.
FIGURE 6. Flowchart of the BAS optimization algorithm (Zhu et al., 2022).
ML is a subfield of AI algorithm that builds a mathematical model based on the data for prediction and making decisions. ML is a powerful data-based modeling mechanism by processing a massive volume of data (Manley et al., 2022). In nuclear engineering, the ML algorithm is employed in NPP to model the surveillance test data (Lee et al., 2021), crack fault diagnosis (Zhong and Ban, 2022), probabilistic safety assessment for fire hazard model (Worrell et al., 2019), seismic fragile analysis (Wang Y. et al., 2023), and equivalence assessment between the simulation and operation data (Li X. et al., 2021). Yet, the ML shows limitations as reported in a review article in Ref (Xu et al., 2024). Figure 7 presents the workflow of ML that comprises different steps from loading the data to integration of the best-trained model into a production system.
ANNs are the most efficient nonlinear modeling and data processing units based on the functioning of a human brain. The ANN designing process involves defining the structure. The building blocks of the ANN are the layers (input, hidden, and output), neurons, and connection weights as shown in Figure 8. The input and output layers are connected by the hidden layer. Successive layers of the ANN are linked by weights. Each layer of the network consists of various amount of processing elements, called neurons. The dataset enters into the network through the input layer. The hidden neurons receive the weighted dataset and process it using the activation function. The output neurons then receive the processed dataset and send it to the users. More, connection weights are used to measure the data and transfer it into the next layer.
Designing the structure of ANNs and selecting an efficient training algorithm are challenging tasks. These issues are open problems for designers. More, the accuracy of training algorithms varies and is affected by the training data points (Zhou et al., 2022). Once the ANNs are trained with a necessary amount of representative quality data, they could be applied to estimate the response under new inputs.
ANNs give attention in nuclear engineering research fields to help plant operators in decision-making to take corrective actions during failure. These intelligence tools are recommended to detect faults in the resistance temperature detector sensors based on the fuel rod temperature profile through modeling (Messai et al., 2015). The ANNs are also suggested to estimate the PWR core state variables online in order to detect faults caused by measurement noise and sensor faults (Kumar and Devakumar, 2022). Further, these modeling mechanisms are used to design the core fuel assembly of the research reactor automatically (Kim et al., 2020). More, they are employed for optimization and burnup calculations of the reactor core (Afzali et al., 2022) as well as for the NPPs fault supervision (Khentout and Magrotti, 2023). The ANNs are suitable and effective mechanisms to diagnose transients of a nuclear reactor during operation and to improve safety (Santosh et al., 2007). Moreover, These potential technologies are employed to predict the state of the nuclear reactor, improve reactor assets as well as empower fast emergency response of nuclear power plants (El-Sefy et al., 2021).
Several types of ANN models are considered and applied for different purposes. The backpropagation neural network is one category of ANN. It is utilized to estimate the PWR core parameters for optimal fuel reloading patterns in order to overcome the restrictions of traditional fuel reloading problems in high-temperature gas-cooled reactors in a short time (Kim et al., 1993). The recurrent multilayer perception ANN model based on the backpropagation algorithm is implemented to model the core neutronics of the NPPs (Adali et al., 1997). Further, the RBFNN is a kind of ANN that has numerous advantages such as simple to design, strong tolerance to disturbance, good generalization, and efficient learning capabilities. Due to these characteristics, the RBFNN model is employed for different applications such as fault assessment, optimization (Sun M. et al., 2022), and adaptive control development (Feng et al., 2022). In nuclear engineering, the RBFNN is deployed to control the core power distribution and rebuild measurements of the core information of the reactor detector (Li W. et al., 2022). Overall, the ANNs seek effective training algorithms. Population-based tactics received more attention for ANN training recently. Figure 9 highlights the possible input and target variables of a reactor to train the ANN through population-based optimization algorithms in a block diagram.
FIGURE 9. Population-based optimization algorithm for ANN training (Kerlin and Upadhyaya, 2019b).
DL is a kind of ML and powerful modeling approach designed by using the DNN model. The DNN model is an intelligent algorithm that works based on the ANN to transform the data into amenable outputs for various applications. The structure of the DNN model comprises numerous hidden layers between input and output layers (Wang J-C. et al., 2023; Yassir et al., 2023), as depicted in Figure 10. As indicated in the block diagram, the workflow in the DNN model starts in the input layer and ends in the output layer. The size of neurons in the input and output layers relies on the input and target variables. However, the design of hidden layers and the corresponding neurons are challenging tasks and an open issue for engineers. These DNN components should be nominated carefully to remove computational challenges such as overfitting and underfitting. The hidden layers and hidden neurons of the DNN model should be simple enough to avoid complexity and reduce computational time. In general, the minimum size of the DNN model is necessary to incorporate good design.
The DNN algorithm is used to model complex systems by creating nonlinear relationships. The accuracy of the developed DNN model output relies on its structure and amount of training data. The advancements in computer systems initiate the use of the DNN model in different architectures in several research areas for various applications. In nuclear engineering, the DNN model is utilized for solving numerous problems such as fault diagnosis (Qian and Liu, 2022a), safety assessments (Bae et al., 2022), internal state prediction (Koo et al., 2021), and control system development (Ejigu and Liu, 2022a). Several DNN models including convolutional neural network (CNN), long short-term memory (LSTM), and multi-layer neural network (MLANN) are reported by (Arji et al., 2023; Sun et al., 2023). However, as presented in Ref. (He et al., 2023), the DNN model shows shortcomings such as overfitting and underfitting. Overall, Figure 11 demonstrates the framework of AI algorithms. The framework also presents the main applications of these AI techniques.
FIGURE 11. Framework of AI algorithms and their applications (Barja-Martinez et al., 2021; Bhat et al., 2023; Huang et al., 2023).
Recently, research on AI, big data, and IoT has been growing rapidly (Chen, 2020). Scholars should merge these interdisciplinary research fields instead of applying them independently from a variety of perspectives (Ahaidous et al., 2023). The implementation of AI in NPPs lies in the potential application of AI techniques to enhance safety, efficiency, and reliability. AI technologies are efficient data processing mechanisms that ensure intrinsically safe operation and successful accident investigation. Collaboration between nuclear experts, AI specialists, and regulatory bodies is crucial to connect the potential benefits while maintaining the highest standards of operational safety within the nuclear industry. Overall, AI algorithms are data-driven modeling techniques. Hence, it requires valuable and quality input-output data (Li V. O. K. et al., 2021; Anthopoulos and Kazantzi, 2022). The big data of NPPs need efficient analysis through statistical modeling and AI algorithms for several applications. Leveraging AI algorithms on the NPPs big data accelerates the existing system towards an environmental-friendly and cost-effective by improving performance. Further, it helps to create a novel business model in the nuclear sector to take advantage of huge data.
The interaction between NPPs, AI technologies, and big data lies in the potential integration of AI and big data analytics to enhance the safety, performance, and efficiency of the NPPs. The implementation of AI and big data analytics in NPPs requires validation, licensing, and commitment to safety standards and guidelines. The collaboration between domain experts, data scientists, and regulatory bodies is crucial to ensure the effectiveness, reliability, and safety of these integrated technologies within the nuclear industry. Besides, AI technologies and big data facilitate the integration of power systems with grids to enable efficient load management and improve stability (Barja-Martinez et al., 2021).
Besides, the incorporation of AI technologies and big data yields a DT. The DT is the virtual representation of a real physical asset. It is an emerging and global trend for various applications in the energy, construction, and manufacturing sectors (Rasheed et al., 2020; Ghenai et al., 2022; Sleiti et al., 2022; Mauro and Kana, 2023). This technology also receives increasing attention in the nuclear engineering field. The DT is constructed and calibrated autonomously for the NPPs core (Li et al., 2023d). It is also employed in nuclear reactors for parameter identification and state estimation (Gong et al., 2023), and anomaly detection (Cancemi et al., 2023).
The integration of big data with AI algorithms needs an IoT platform. Hence, AI, big data, and IoT overlap and should be considered when controlling NPPs. The conceptual overlap of AI, big data, and digital technology is described in Figure 12. As shown in the figure, the combination of AI with data mining provides processed data that enhance its training and performance. The AI is also combined with advanced digital technologies, such as IoT computing, to control and communicate with information systems and stakeholders. Furthermore, advanced digital technologies provide data storage and pipelines for the processed data to flow to the AI and the stakeholders; this fact makes it overlap with big data. Overall, the combination of AI, big data, and IoT technologies has the potential to transform the NPPs control for enhancing safe operations, efficiency, and security.
FIGURE 12. The connection between AI, big data, and digital technology in a Venn diagram (Li et al., 2023e).
Big data computing through AI using digital technologies is applied in different research fields such as in the health sector (Galetsi et al., 2022; Charalambous and Dodlek, 2023), smart energy management (Li et al., 2023e), addressing ecosystem services (Manley et al., 2022), and building smart education platforms (Zheng et al., 2023). Figure 13 depicts the application of big data computing through AI technologies in different research sectors. Further, Table 2 summarizes the application of big data computing through different AI methods NPPs.
FIGURE 13. Application of AI algorithms and big data computing (Shukla et al., 2019; Yüksel et al., 2023).
Overall, NPPs are complex power industries that face several challenges. The NPPs are exposed to model uncertainties, input disturbances, external aggression, and malfunctions. These factors contribute to instability and potential accidents that spread into the entire system. The Three Mile Island (USA), Chornobyl (Ukraine), and Fukushima (Japan) tragic accidents provide opportunities to conduct extensive research concerning into safety of the NPPs (Wheatley et al., 2017), and pre-accident assessment by estimating the current and future response of the nuclear reactor behavior.
The NPPs generate an enormous amount of diverse data (International Atomic Energy Agency, 2015). Thus, storing, managing, processing, and interpreting such immense datasets is a challenging and time-consuming task. Due to the size, complexity, and time-sensitive characteristics of the data, traditional processing tools are incapable of handling big data of the NPPs. As a result, this shortcoming aids the prospects to carry out research concerning intelligence data management mechanisms to extract meaningful insights and make data-driven decisions from big data.
In the nuclear sector, the interest in the use of data science and AI capabilities is increasing to solve several challenges. However, the big data and AI techniques in this domain are in the early stage and data-driven applications are not yet mature. This opens up new possibilities and opportunities for this attractive and emerging research direction. The primary triggering condition of this interest is the availability of real operational data from the NPPs and digitization (Rodionov, 2007). More, the real observations collected from the NPPs need security. Big data analytics in NPPs requires careful consideration of data security, privacy, and regulatory agreement. Robust data management techniques and commitment are essential to protect sensitive information and maintain the privacy and security of operational data within the nuclear industry (Lorenz and Schmidt, 1986; OECD and Nuclear Energy Agency, 2000).
Overall, the incorporation of AI and big data analytics in NPPs boost efficiency, safety, and performance. However, it also brings numerous challenges that need to be addressed carefully. The main challenges associated with AI and big data are presented below.
It is a difficult task and AI needs trusted data to capture real information efficiently (Momota and Morshed, 2022). Reliability of the data is an important aspect of science and engineering for making informed decisions, reaching valid findings, and producing credible outcomes. Establishing data reliability in AI is an ongoing and challenging process that necessitates regular monitoring, improvement, and adaptation.
AI and big data applications in NPPs necessitate access to massive amounts of sensitive and vital data. It is critical to protect this data against unwanted access, cyber-attacks, and hacking (Ayodeji et al., 2023). Nuclear data needs a strong cybersecurity framework to safeguard the privacy and security of information.
AI algorithms and big data analysis primarily rely on quality data for precise decision-making. The quality of data also directly impacts the performance generalization and decision-making capability of the AI models (Qi et al., 2022). Ensuring honest data sources and maintaining data quality over time is a significant challenge, especially considering the long operational lifetimes of NPPs.
NPPs are governed by strict rules and safety standards. The integration of AI technologies and big data analytics necessitates modifications to existing regulations and the development of new guidelines to assure compliance while maintaining safety and reliability.
AI models are complex and difficult to comprehend (Balasubramaniam et al., 2023). Transparency in AI decision-making processes is vital in safety-sensitive applications such as NPPs. Operators should understand the judgments of AI to trust and verify its behavior.
Introducing AI and big data into NPPs necessitates a transition in human responsibilities, from direct manual operation to supervisory and decision-support roles (Hiroshi et al., 2021). Effective collaboration between human operators and AI systems is important to ensure safe and optimal plant operation.
The adoption of AI technologies and big data analysis includes substantial costs such as infrastructure investment, personnel training, and ongoing maintenance (OECD and Nuclear Energy Agency, 2020). It might be difficult for NPPs operators to ensure the balance of the benefits with the expenses.
The nuclear industry needs trained and educated personnel who can use AI technologies and big data analytics (International Atomic Energy Agency, 1996). This can be accomplished by enrolling experts from other more mature industries and training specialists in big data approaches relevant to nuclear energy and AI. Combining these experts with other energy domain knowledge experts is recommended. The nuclear industry should also make investments in personnel training and reskilling to manage and operate the systems and assets of NPPs.
These are common issues encountered in the development of models using AI techniques, particularly in ML. Hence, understanding these concepts is vital for developing effective and reliable AI-based models (Rattan et al., 2022). Overfitting and underfitting issues could be overcome by data augmentation, regularization, adjustment of the model, and k-fold cross-validation methods (Mutasa et al., 2020).
Overall, these challenges could be overcome through cooperation among nuclear experts, data scientists, and AI developers. By efficiently managing these difficulties, AI and big data computing can significantly improve the safety, efficiency, and security of NPPs.
This study provides a comprehensive review of the application of AI and big data in the field of nuclear engineering specifically for NPPs. Its purpose is to equip researchers with knowledge and guidance on the advantages of applying AI and big data technologies to accelerate scientific and technological advancements through learning-based approaches. A key emphasis of this review is the importance of AI algorithms and big data computing providing fast estimations to support informed decision-making by users, while also ensuring the interpretability and reproducibility of the models. The goal is to develop and implement algorithms that can assist and augment human decision-makers in the loop, rather than replace them entirely. The study suggests leveraging modern research accelerators that facilitate virtual discussions and collaborations among researchers in various areas to foster innovation. These platforms enable active participation and exchange of ideas, leading to accelerated progress in nuclear research. Ultimately, the overarching objective is to achieve a safe and effective application of AI and big data computing methods in the dominion of nuclear science. By utilizing AI and big data computing approaches appropriately, researchers can enhance their ability to make reliable predictions and optimization for improving safety measures within the nuclear field.
DE: Conceptualization, Methodology, Writing–original draft, Writing–review and editing. YT: Conceptualization, Writing–review and editing. XL: Conceptualization, Funding acquisition, Methodology, Project administration, Resources, Supervision, Writing–review and editing.
The author(s) declare financial support was received for the research, authorship, and/or publication of this article. This work is financially supported by the National Key R&D Program of China (Grant No. 2020YFB1901900).
The authors declare that the research was conducted in the absence of any commercial or financial relationships that could be construed as a potential conflict of interest.
The author(s) declared that they were an editorial board member of Frontiers, at the time of submission. This had no impact on the peer review process and the final decision.
All claims expressed in this article are solely those of the authors and do not necessarily represent those of their affiliated organizations, or those of the publisher, the editors and the reviewers. Any product that may be evaluated in this article, or claim that may be made by its manufacturer, is not guaranteed or endorsed by the publisher.
AI, Artificial intelligence; ANN, Artificial neural network; BAS, Beetle antennae search; BN, Bayesian networks; CNN, Convolutional neural network; DL, Deep learning; DNN, Deep neural network; DRL, Deep reinforcement learning; DT, Digital twin; GA, Genetic algorithm; GAN, Generative adversarial networks; IAEA, International Atomic Energy Agency; IoT, Internet of things; LG, Logistic regression; LSTM, Long short-term memory; ML, Machine learning; MLNN, Multi-layer neural network; NPPs, Nuclear power plants; PSO, Particle swarm optimization; PWR, Pressurized water reactor; RBFNN, Radial basis function neural network; SVM, Support vector machine.
Adali, T., Bakal, B., Sönmez, M. K., Fakory, R., and Tsaoi, C. O. (1997). Modeling nuclear reactor core dynamics with recurrent neural networks. Neurocomputing 15, 363–381. doi:10.1016/S0925-2312(97)00018-0
Afzali, M., Allaf, M. A., Jahanfarnia, G., and Kheradmand, M. (2022). Optimization and burnup calculations of BNPP’s reactor core with the new generation fuels (TVS-2M) by artificial neural network. Prog. Nucl. Energy 150, 104290. doi:10.1016/j.pnucene.2022.104290
Ahaidous, K., Tabaa, M., and Hachimi, H. (2023). Towards IoT-Big Data architecture for future education. Procedia Comput. Sci. 220, 348–355. doi:10.1016/j.procs.2023.03.045
Anthopoulos, L., and Kazantzi, V. (2022). Urban energy efficiency assessment models from an AI and big data perspective: tools for policy makers. Sustain Cities Soc. 76, 103492. doi:10.1016/j.scs.2021.103492
Arji, G., Erfannia, L., Alirezaei, S., and Hemmat, M. (2023). A systematic literature review and analysis of deep learning algorithms in mental disorders. Inf. Med. Unlocked 40, 101284. doi:10.1016/j.imu.2023.101284
Ayele Ejigu, D., and Liu, X. (2022). Dynamic modeling and intelligent hybrid control of pressurized water reactor NPP power transient operation. Ann. Nucl. Energy 173, 109118. doi:10.1016/j.anucene.2022.109118
Ayodeji, A., Mohamed, M., Li, L., Di Buono, A., Pierce, I., and Ahmed, H. (2023). Cyber security in the nuclear industry: a closer look at digital control systems, networks and human factors. Prog. Nucl. Energy 161, 104738. doi:10.1016/j.pnucene.2023.104738
Babazadeh, D., Boroushaki, M., and Lucas, C. (2009). Optimization of fuel core loading pattern design in a VVER nuclear power reactors using Particle Swarm Optimization (PSO). Ann. Nucl. Energy 36, 923–930. doi:10.1016/j.anucene.2009.03.007
Bae, J., Park, J. W., and Lee, S. J. (2022). Limit surface/states searching algorithm with a deep neural network and Monte Carlo dropout for nuclear power plant safety assessment. Appl. Soft Comput. 124, 109007. doi:10.1016/j.asoc.2022.109007
Bagheri, S., and Khalafi, H. S. M. R. (2023). SMR, 3D source term simulation for exact shielding design based on genetic algorithm. Ann. Nucl. Energy 191, 109915. doi:10.1016/j.anucene.2023.109915
Balanin, A., and Fomichenko, P. (2023). Assessing potential of high temperature reactor facilities of Russian design for near-term deployment of hydrogen production. Int. J. Hydrog. Energy 52, 1347–1355. S0360319923053909. doi:10.1016/j.ijhydene.2023.10.242
Balasubramaniam, N., Kauppinen, M., Rannisto, A., Hiekkanen, K., and Kujala, S. (2023). Transparency and explainability of AI systems: from ethical guidelines to requirements. Inf. Softw. Technol. 159, 107197. doi:10.1016/j.infsof.2023.107197
Barja-Martinez, S., Aragüés-Peñalba, M., Munné-Collado, Í., Lloret-Gallego, P., Bullich-Massagué, E., and Villafafila-Robles, R. (2021). Artificial intelligence techniques for enabling Big Data services in distribution networks: a review. Renew. Sustain Energy Rev. 150, 111459. doi:10.1016/j.rser.2021.111459
Basu, D., and Miroshnik, V. W. (2019). The political economy of nuclear energy: prospects and retrospect. Cham: Springer International Publishing. doi:10.1007/978-3-030-27029-2
Benavides, J., Jiménez, G., López, S., Galbán, M., and Lloret, M. (2020). Modeling and results of the dry cask simulator (DCS) with STAR-CCM+. Nucl. Eng. Des. 369, 110820. doi:10.1016/j.nucengdes.2020.110820
Bernard, J. A. (1989). 6. Applications of artificial intelligence to reactor and plant control. Nucl. Eng. Des. 113, 219–227. doi:10.1016/0029-5493(89)90073-3
Bhat, D., Muench, S., and Roellig, M. (2023). Application of machine learning algorithms in prognostics and health monitoring of electronic systems: a review. E-Prime - Adv. Electr. Eng. Electron Energy 4, 100166. doi:10.1016/j.prime.2023.100166
Birol, F. (2019). Nuclear power in a clean cnergy system. Int. Energy Agency. doi:10.1787/fc5f4b7e-en
Boring, R., Mortenson, T., Ulrich, T., and Lew, R. (2022). Humans with/as big data in nuclear energy. doi:10.54941/ahfe1002221
Bucz, Š., and Kozáková, A. (2018). “Advanced methods of PID controller tuning for specified performance,” in PID control for industrial processes. Editor M. Shamsuzzoha (Bratislava, Slovak Republic: Faculty of Electrical Engineering and Information Technology, Slovak University of Technology). doi:10.5772/intechopen.76069
Cabellos, O., Ahnert, C., Cuervo, D., García-Herranz, N., Gallego, E., Mínguez, E., et al. (2018). Education and training of future nuclear engineers at DIN: from advanced computer codes to an interactive plant simulator.
Cancemi, S. A., Lo Frano, R., Santus, C., and Inoue, T. (2023). Unsupervised anomaly detection in pressurized water reactor digital twins using autoencoder neural networks. Nucl. Eng. Des. 413, 112502. doi:10.1016/j.nucengdes.2023.112502
Cavallaro, C., Cutello, V., Pavone, M., and Zito, F. (2024). Machine Learning and Genetic Algorithms: a case study on image reconstruction. Knowl-Based Syst. 284, 111194. doi:10.1016/j.knosys.2023.111194
Chander, S., Vijaya, P., Fernandes, R., Rodrigues, A. P., and Maheshwari, R. (2023). Dolphin-political optimized tversky index based feature selection in spark architecture for clustering big data. Adv. Eng. Softw. 176, 103331. doi:10.1016/j.advengsoft.2022.103331
Charalambous, A., and Dodlek, N. (2023). Big data, machine learning, and artificial intelligence to advance cancer care: opportunities and challenges. Semin. Oncol. Nurs. 39, 151429. doi:10.1016/j.soncn.2023.151429
Chen, C., Mei, H., He, M., and Li, T. (2022). Neutronics analysis of a 200 kWe space nuclear reactor with an integrated honeycomb core design. Nucl. Eng. Technol. 54, 4743–4750. doi:10.1016/j.net.2022.08.012
Chen, Y. (2020). IoT, cloud, big data and AI in interdisciplinary domains. Simul. Model Pract. Theory 102, 102070. doi:10.1016/j.simpat.2020.102070
Cleveland, J. C., and McDonald, A. (2008). Advanced applications of water cooled nuclear power plants. Vienna: International Atomic Energy Agency.
Coban, R. (2011). A fuzzy controller design for nuclear research reactors using the particle swarm optimization algorithm. Nucl. Eng. Des. 241, 1899–1908. doi:10.1016/j.nucengdes.2011.01.045
Corzo, S. F., Ugarte, R., Godino, D. M., and Ramajo, D. E. (2023). Loss of flow accident analysis in Atucha II nuclear power plant using RELAP5 model. Nucl. Eng. Des. 402, 112108. doi:10.1016/j.nucengdes.2022.112108
Dagan, D. T., and Wilkins, E. J. (2023). What is “big data” and how should we use it? The role of large datasets, secondary data, and associated analysis techniques in outdoor recreation research. J. Outdoor Recreat. Tour. 44, 100668. doi:10.1016/j.jort.2023.100668
da Silva Veloso, Y. M., de Almeida, M. M., de Alsina, O. L. S., Passos, M. L., Mujumdar, A. S., and Leite, M. S. (2020). Hybrid phenomenological/ANN-PSO modelling of a deformable material in spouted bed drying process. Powder Technol. 366, 185–196. doi:10.1016/j.powtec.2019.12.047
Developing a Systematic Education and Training Approach (2018). “Developing a Systematic Education and Training Approach Using Personal Computer Based Simulators for Nuclear Power Programmes: Proceedings of a Technical Meeting Held in Vienna,”in 15-19 May 2017, Lanham, Lanham. IAEA TECDOC No. 1836. Vienna, Austria: International Atomic Energy Agency Rowman and Littlefield Publishers. Incorporated [Distributor].
Ejigu, D. A., and Liu, X. (2022a). Gradient descent-particle swarm optimization based deep neural network predictive control of pressurized water reactor power. Prog. Nucl. Energy 145, 104108. doi:10.1016/j.pnucene.2021.104108
Ejigu, D. A., and Liu, X. (2022b). Pressurized water reactor core power control using BAS-RBF-PID approach during transient operation. Nucl. Sci. Eng. 197, 1239–1254. doi:10.1080/00295639.2022.2138688
Ejigu, D. A., and Liu, X. (2023). Predictive modeling of pressurized water reactor transients using nonlinear autoregressive with exogenous input neural network. Prog. Nucl. Energy 161, 104729. doi:10.1016/j.pnucene.2023.104729
El-Sahlamy, N. M., Hassan, M. I., Khedr, A. S., and D’Auria, F. (2022). Study of rod ejection accident at hot zero power condition in a PWR using RELAP5. Prog. Nucl. Energy 144, 104100. doi:10.1016/j.pnucene.2021.104100
El-Sefy, M., Ezzeldin, M., El-Dakhakhni, W., Wiebe, L., and Nagasaki, S. (2019). System dynamics simulation of the thermal dynamic processes in nuclear power plants. Nucl. Eng. Technol. 51, 1540–1553. doi:10.1016/j.net.2019.04.017
El-Sefy, M., Yosri, A., El-Dakhakhni, W., Nagasaki, S., and Wiebe, L. (2021). Artificial neural network for predicting nuclear power plant dynamic behaviors. Nucl. Eng. Technol. 53, 3275–3285. doi:10.1016/j.net.2021.05.003
Fan, Y., Shao, J., and Sun, G. (2019). Optimized PID controller based on beetle antennae search algorithm for electro-hydraulic position servo control system. Sensors 19, 2727. doi:10.3390/s19122727
Feng, H., Song, Q., Ma, S., Ma, W., Yin, C., Cao, D., et al. (2022). A new adaptive sliding mode controller based on the RBF neural network for an electro-hydraulic servo system. ISA Trans. 129, 472–484. doi:10.1016/j.isatra.2021.12.044
Galetsi, P., Katsaliaki, K., and Kumar, S. (2022). The medical and societal impact of big data analytics and artificial intelligence applications in combating pandemics: a review focused on Covid-19. Soc. Sci. Med. 301, 114973. doi:10.1016/j.socscimed.2022.114973
Geslot, B., Kooyman, T., Casoli, P., Leconte, P., Boffy, R., Truchet, G., et al. (2023). A pile noise experiment in the reference core of the nuclear research reactor LR-0. Ann. Nucl. Energy 189, 109833. doi:10.1016/j.anucene.2023.109833
Ghenai, C., Husein, L. A., Al Nahlawi, M., Hamid, A. K., and Bettayeb, M. (2022). Recent trends of digital twin technologies in the energy sector: a comprehensive review. Sustain Energy Technol. Assess. 54, 102837. doi:10.1016/j.seta.2022.102837
Gohel, H. A., Upadhyay, H., Lagos, L., Cooper, K., and Sanzetenea, A. (2020). Predictive maintenance architecture development for nuclear infrastructure using machine learning. Nucl. Eng. Technol. 52, 1436–1442. doi:10.1016/j.net.2019.12.029
Gong, H., Zhu, T., Chen, Z., Wan, Y., and Li, Q. (2023). Parameter identification and state estimation for nuclear reactor operation digital twin. Ann. Nucl. Energy 180, 109497. doi:10.1016/j.anucene.2022.109497
Gueta, T., and Carmel, Y. (2016). Quantifying the value of user-level data cleaning for big data: a case study using mammal distribution models. Ecol. Inf. 34, 139–145. doi:10.1016/j.ecoinf.2016.06.001
Guillen, D. P., Wharry, J. P., Housley, G. K., Hale, C. D., Brookman, J. V., and Gandy, D. W. (2023). Experiment design for the neutron irradiation of PM-HIP alloys for nuclear reactors. Nucl. Eng. Des. 402, 112114. doi:10.1016/j.nucengdes.2022.112114
Gursel, E., Reddy, B., Khojandi, A., Madadi, M., Coble, J. B., Agarwal, V., et al. (2023). Using artificial intelligence to detect human errors in nuclear power plants: a case in operation and maintenance. Nucl. Eng. Technol. 55, 603–622. doi:10.1016/j.net.2022.10.032
He, W., Li, Z., Liu, T., Liu, Z., Guo, X., Du, J., et al. (2023). Research progress and application of deep learning in remaining useful life, state of health and battery thermal management of lithium batteries. J. Energy Storage 70, 107868. doi:10.1016/j.est.2023.107868
He, W., Lin, C., Wu, T.-A., Tang, X., Chen, X., and Xu, J. (2022). An improved beetle antennae search algorithm with Lévy flight and its application in micro-laser assisted turning. Adv. Eng. Inf. 54, 101732. doi:10.1016/j.aei.2022.101732
Hiroshi, K., Shiro, T., and Takayuki, K. (2021). Automatic plant operation utilizing AI and big data analysis in waste-to-energy plants.
Hong, S., Bradshaw, C. J. A., and Brook, B. W. (2014). Nuclear power can reduce emissions and maintain a strong economy: rating Australia’s optimal future electricity-generation mix by technologies and policies. Appl. Energy 136, 712–725. doi:10.1016/j.apenergy.2014.09.062
Hu, G., Zhang, H., and Liu, Q. (2020). Review on sensors to measure control rod position for nuclear reactor. Ann. Nucl. Energy 144, 107485. doi:10.1016/j.anucene.2020.107485
Huang, Q., Peng, S., Deng, J., Zeng, H., Zhang, Z., Liu, Y., et al. (2023). A review of the application of artificial intelligence to nuclear reactors: where we are and what’s next. Heliyon 9, e13883. doi:10.1016/j.heliyon.2023.e13883
Huang, Q., Xie, L., Yin, G., Ran, M., Liu, X., and Zheng, J. (2020). Acoustic signal analysis for detecting defects inside an arc magnet using a combination of variational mode decomposition and beetle antennae search. ISA Trans. 102, 347–364. doi:10.1016/j.isatra.2020.02.036
Hyer, H. C., Giuliano, D. R., and Petrie, C. M. (2023). Toward local core outlet temperature monitoring in gas-cooled nuclear reactors using distributed fiber-optic temperature sensors. Appl. Therm. Eng. 230, 120847. doi:10.1016/j.applthermaleng.2023.120847
International Atomic Energy Agency (1996). Nuclear power plant personnel training and its evaluation: a guidebook. Vienna: International Atomic Energy Agency.
International Atomic Energy Agency (2015). Quality and reliability aspects in nuclear power reactor fuel engineering. Vienna: IAEA.
International Energy Agency (2022). Nuclear power and secure energy transitions: from today’s challenges to tomorrow’s clean energy systems. Paris, France: OECD. doi:10.1787/aca1d7ee-en
Jaiswal, V., Saurabh, P., Lilhore, U. K., Pathak, M., Simaiya, S., and Dalal, S. (2023). A breast cancer risk predication and classification model with ensemble learning and big data fusion. Decis. Anal. J. 8, 100298. doi:10.1016/j.dajour.2023.100298
Jamali, B., Rasekh, M., Jamadi, F., Gandomkar, R., and Makiabadi, F. (2019). Using PSO-GA algorithm for training artificial neural network to forecast solar space heating system parameters. Appl. Therm. Eng. 147, 647–660. doi:10.1016/j.applthermaleng.2018.10.070
Jiang, X., and Li, S. (2017). BAS: beetle antennae search algorithm for optimization problems. ArXiv171010724 Cs. Available at: http://arxiv.org/abs/1710 (Accessed January 21, 2021).
Kalyakin, S. G., Kozlov, F. A., Sorokin, A. P., Bogoslovskaya, G. P., Ivanov, A. P., Konovalov, M. A., et al. (2016). Investigations for the substantiation of high-temperature nuclear power generation technology using fast sodium-cooled reactor for hydrogen production and other innovative applications (Part 1). Nucl. Energy Technol. 2, 282–286. doi:10.1016/j.nucet.2016.11.016
Kerlin, T. W., and Upadhyaya, B. R. (2019a). “Nuclear reactor designs,” in Dynamics and control of nuclear reactors (Elsevier), 5–16. doi:10.1016/B978-0-12-815261-4.00002-0
Kerlin, T. W., and Upadhyaya, B. R. (2019b). “Reactor control,” in Dynamics and control of nuclear reactors (Elsevier), 89–104. doi:10.1016/B978-0-12-815261-4.00008-1
Khan, A. H., Hossain, S., Hasan, M., Islam, M. S., Rahman, M. M., and Kim, J.-H. (2022). Development of an optimized thermodynamic model for VVER-1200 reactor-based nuclear power plants using genetic algorithm. Alex Eng. J. 61, 9129–9148. doi:10.1016/j.aej.2022.02.052
Khan, S. U.-D., Khan, S. U.-D., Danish, S. N., Orfi, J., Rana, U. A., and Haider, S. (2018). “Nuclear energy powered seawater desalination,” in Renewable energy powered desalination handbook (Elsevier), 225–264. doi:10.1016/B978-0-12-815244-7.00006-4
Khentout, N., and Magrotti, G. (2023). Fault supervision of nuclear research reactor systems using artificial neural networks: a review with results. Ann. Nucl. Energy 185, 109684. doi:10.1016/j.anucene.2023.109684
Kianpour, R., Ansarifar, G. R., and Fathi, M. (2020). Optimal design of a VVER-1000 nuclear reactor core with dual cooled annular fuel based on the reactivity temperature coefficients using Thermal hydraulic and neutronic analysis by implementing the genetic algorithms. Ann. Nucl. Energy 148, 107682. doi:10.1016/j.anucene.2020.107682
Kim, H. G., Chang, S. H., and Lee, B. H. (1993). Pressurized water reactor core parameter prediction using an artificial neural network. Nucl. Sci. Eng. 113, 70–76. doi:10.13182/NSE93-A23994
Kim, S. H., Shin, S. G., Han, S., Kim, M. H., and Pyeon, C. H. (2020). Feasibility study on application of an artificial neural network for automatic design of a reactor core at the Kyoto University Critical Assembly. Prog. Nucl. Energy 119, 103183. doi:10.1016/j.pnucene.2019.103183
Koo, Y. D., Seon Jo, H., Na, M. G., Yoo, K. H., and Kim, C.-H. (2021). Prediction of the internal states of a nuclear power plant containment in LOCAs using rule-dropout deep fuzzy neural networks. Ann. Nucl. Energy 156, 108180. doi:10.1016/j.anucene.2021.108180
Kumar, S. R., and Devakumar, J. (2022). Performance evaluation of neural network topologies for online state estimation and fault detection in pressurized water reactor. Ann. Nucl. Energy 175, 109235. doi:10.1016/j.anucene.2022.109235
Lang, M., Zhang, Z., Chen, Z., Cheng, Z., Santosh, M., and Kusky, T. M. (2023). Classification and nomenclature of volcanic rocks using immobile elements: a novel approach based on big data analysis. Lithos 454-455, 107274–107455. doi:10.1016/j.lithos.2023.107274
Lee, G.-G., Kim, M.-C., and Lee, B.-S. (2021). Machine learning modeling of irradiation embrittlement in low alloy steel of nuclear power plants. Nucl. Eng. Technol. 53, 4022–4032. doi:10.1016/j.net.2021.06.014
Lei, J., Yang, C., Zhang, H., Liu, C., Yan, D., Xiao, G., et al. (2023). Radiation shielding optimization design research based on bare-bones particle swarm optimization algorithm. Nucl. Eng. Technol. 55, 2215–2221. doi:10.1016/j.net.2023.02.018
Li, K., Bian, H., Liu, C., Zhang, D., and Yang, Y. (2015). Comparison of geothermal with solar and wind power generation systems. Renew. Sustain Energy Rev. 42, 1464–1474. doi:10.1016/j.rser.2014.10.049
Li, R., Cheng, M., and Dai, Z. (2022). Improvement of the delayed neutron precursor transport model in RELAP5 for liquid-fueled molten salt reactor. Nucl. Eng. Des. 394, 111817. doi:10.1016/j.nucengdes.2022.111817
Li, S., He, H., Zhao, P., and Cheng, S. (2022). Data cleaning and restoring method for vehicle battery big data platform. Appl. Energy 320, 119292. doi:10.1016/j.apenergy.2022.119292
Li, W., Ding, P., Xia, W., Chen, S., Yu, F., Duan, C., et al. (2022). Artificial neural network reconstructs core power distribution. Nucl. Eng. Technol. 54, 617–626. doi:10.1016/j.net.2021.08.015
Li, X. (2020). An enhanced selective ensemble deep learning method for rolling bearing fault diagnosis with beetle antennae search algorithm. Mech. Syst. Signal Process, 20. doi:10.1016/j.ymssp.2020.106752
Li, X., Cheng, K., Huang, T., and Tan, S. (2021). Equivalence analysis of simulation data and operation data of nuclear power plant based on machine learning. Ann. Nucl. Energy 163, 108507. doi:10.1016/j.anucene.2021.108507
Li, V. O. K., Lam, J. C. K., and Cui, J. (2021). AI for social good: AI and big data approaches for environmental decision-making. Environ. Sci. Policy 125, 241–246. doi:10.1016/j.envsci.2021.09.001
Li, X., Sun, W., Ding, S., Huang, T., Ma, Z., Zhang, L., et al. (2023a). Analysis of RELAP5 prediction of countercurrent flow limitation in downcomer at upper plenum test facility. Prog. Nucl. Energy 158, 104608. doi:10.1016/j.pnucene.2023.104608
Li, F., Laili, Y., Chen, X., Lou, Y., Wang, C., Yang, H., et al. (2023c). Towards big data driven construction industry. J. Ind. Inf. Integr. 35, 100483. doi:10.1016/j.jii.2023.100483
Li, X., Yang, T., Zhu, Z., Zeng, Z., Zeng, G., Liang, J., et al. (2023b). A study on air pollution exposure of “first and last mile” urban commuters under space-behavior dual verification based on big data, land-use regression model and space syntax. J. Clean. Prod. 419, 138244. doi:10.1016/j.jclepro.2023.138244
Li, W., Cai, J., Lu, H., Wang, J., Cai, L., Tang, Z., et al. (2023d). Constructing a probability digital twin for reactor core with Bayesian network and reduced-order model. Ann. Nucl. Energy 193, 110016. doi:10.1016/j.anucene.2023.110016
Li, J., Herdem, M. S., Nathwani, J., and Wen, J. Z. (2023e). Methods and applications for artificial intelligence, big data, Internet of things, and blockchain in smart energy management. Energy ai. 11, 100208. doi:10.1016/j.egyai.2022.100208
Li, Z., Sun, J., Liu, M., Lang, M., and Shi, L. (2020). Design of a hundred-kilowatt level integrated gas-cooled space nuclear reactor for deep space application. Nucl. Eng. Des. 361, 110569. doi:10.1016/j.nucengdes.2020.110569
Lima-Reinaldo, Y., and François, J.-L. (2023). Fuel loading pattern optimization of ALLEGRO fast reactor using genetic algorithms. Ann. Nucl. Energy 180, 109451. doi:10.1016/j.anucene.2022.109451
Lorenz, A., and Schmidt, J. J. (1986). Nuclear data: serving basic needs of science and technology. IAEA Bull.
Ma, S., Huang, Y., Liu, Y., Liu, H., Chen, Y., Wang, J., et al. (2023). Big data-driven correlation analysis based on clustering for energy-intensive manufacturing industries. Appl. Energy 349, 121608. doi:10.1016/j.apenergy.2023.121608
Manley, K., Nyelele, C., and Egoh, B. N. (2022). A review of machine learning and big data applications in addressing ecosystem service research gaps. Ecosyst. Serv. 57, 101478. doi:10.1016/j.ecoser.2022.101478
Marfaing, O., Guingo, M., Laviéville, J., Mimouni, S., Baglietto, E., Lubchenko, N., et al. (2018). Comparison and uncertainty quantification of two-fluid models for bubbly flows with NEPTUNE_CFD and STAR-CCM+. Nucl. Eng. Des. 337, 1–16. doi:10.1016/j.nucengdes.2018.05.028
Mauro, F., and Kana, A. A. (2023). Digital twin for ship life-cycle: a critical systematic review. Ocean. Eng. 269, 113479. doi:10.1016/j.oceaneng.2022.113479
Meneses, AADM, Machado, M. D., and Schirru, R. (2009). Particle swarm optimization applied to the nuclear reload problem of a pressurized water reactor. Prog. Nucl. Energy 51, 319–326. doi:10.1016/j.pnucene.2008.07.002
Messai, A., Mellit, A., Abdellani, I., and Massi Pavan, A. (2015). On-line fault detection of a fuel rod temperature measurement sensor in a nuclear reactor core using ANNs. Prog. Nucl. Energy 79, 8–21. doi:10.1016/j.pnucene.2014.10.013
Miraclin Joyce Pamila, J. C., Senthamil Selvi, R., Santhi, P., and Nithya, T. M. (2022). Ensemble classifier based big data classification with hybrid optimal feature selection. Adv. Eng. Softw. 173, 103183. doi:10.1016/j.advengsoft.2022.103183
Momota, M. M. R., and Morshed, B. I. (2022). ML algorithms to estimate data reliability metric of ECG from inter-patient data for trustable AI-based cardiac monitors. Smart Health 26, 100350. doi:10.1016/j.smhl.2022.100350
Morrisset, D., Santamaria, S., Hadden, R., and Emberley, R. (2022). Implications of data smoothing on experimental mass loss rates. Fire Saf. J. 131, 103611. doi:10.1016/j.firesaf.2022.103611
Mousakazemi, S. M. H. (2020). Computational effort comparison of genetic algorithm and particle swarm optimization algorithms for the proportional–integral–derivative controller tuning of a pressurized water nuclear reactor. Ann. Nucl. Energy 136, 107019. doi:10.1016/j.anucene.2019.107019
Murakami, T. (2021). A historical review and analysis on the selection of nuclear reactor types and implications to development programs for advanced reactors; A Japanese study. Energy Rep. 7, 3428–3436. doi:10.1016/j.egyr.2021.05.049
Mutasa, S., Sun, S., and Ha, R. (2020). Understanding artificial intelligence based radiology studies: what is overfitting? Clin. Imaging 65, 96–99. doi:10.1016/j.clinimag.2020.04.025
Muthuraj, T., Arul, J., Surjagade, P. V., Vajpayee, V., Becerra, V., and Deng, J. (2023). An inherently fault tolerant control of sodium cooled fast reactors and its stability analysis using particle swarm optimization. Prog. Nucl. Energy 160, 104676. doi:10.1016/j.pnucene.2023.104676
Ni, S., Liu, M., and Gu, H. (2021). Modeling and validation of RELAP5 for natural circulation flow in a single PWR fuel assembly. Ann. Nucl. Energy 151, 107940. doi:10.1016/j.anucene.2020.107940
Nian, V., Mignacca, B., and Locatelli, G. (2022). Policies toward net-zero: benchmarking the economic competitiveness of nuclear against wind and solar energy. Appl. Energy 320, 119275. doi:10.1016/j.apenergy.2022.119275
OECD, Nuclear Energy Agency (2000). Monitoring and data management strategies for nuclear emergencies. Boulogne-Billancourt, France: OECD. doi:10.1787/9789264180956-en
OECD, Nuclear Energy Agency (2020). Unlocking reductions in the construction costs of nuclear: a practical guide for stakeholders. Boulogne-Billancourt, France: OECD. doi:10.1787/33ba86e1-en
OECD, Nuclear Energy Agency (2022). High-temperature gas-cooled reactors and industrial heat applications. Boulogne-Billancourt, France: OECD. doi:10.1787/7406b814-en
Okunlola, A., Davis, M., and Kumar, A. (2023). Assessing the cost competitiveness of electrolytic hydrogen production from small modular nuclear reactor-based power plants: a price-following perspective. Appl. Energy 346, 121290. doi:10.1016/j.apenergy.2023.121290
Pant, P., and Chatterjee, D. (2020). Prediction of clad characteristics using ANN and combined PSO-ANN algorithms in laser metal deposition process. Surf. Interfaces 21, 100699. doi:10.1016/j.surfin.2020.100699
Paulraj, D., Mohamed Junaid, K. A., Sethukarasi, T., Vigilson Prem, M., Neelakandan, S., Alhudhaif, A., et al. (2024). A novel efficient Rank-Revealing QR matrix and Schur decomposition method for big data mining and clustering (RRQR-SDM). Inf. Sci. 657, 119957. doi:10.1016/j.ins.2023.119957
Peakman, A., and Lindley, B. (2023). A review of nuclear electric fission space reactor technologies for achieving high-power output and operating with HALEU fuel. Prog. Nucl. Energy 163, 104815. doi:10.1016/j.pnucene.2023.104815
Price, D., Radaideh, M. I., and Kochunas, B. (2022). Multiobjective optimization of nuclear microreactor reactivity control system operation with swarm and evolutionary algorithms. Nucl. Eng. Des. 393, 111776. doi:10.1016/j.nucengdes.2022.111776
Qi, B., Xiao, X., Liang, J., Po, L. C., Zhang, L., and Tong, J. (2022). An open time-series simulated dataset covering various accidents for nuclear power plants. Sci. Data 9, 766. doi:10.1038/s41597-022-01879-1
Qian, G., and Liu, J. (2022a). A comparative study of deep learning-based fault diagnosis methods for rotating machines in nuclear power plants. Ann. Nucl. Energy 178, 109334. doi:10.1016/j.anucene.2022.109334
Qian, G., and Liu, J. (2022b). Development of deep reinforcement learning-based fault diagnosis method for rotating machinery in nuclear power plants. Prog. Nucl. Energy 152, 104401. doi:10.1016/j.pnucene.2022.104401
Rafiei, M., and Ansarifar, G. R. (2022). Space nuclear reactor fuel design based on dynamic analysis and GA & PSO optimization. Prog. Nucl. Energy 143, 104043. doi:10.1016/j.pnucene.2021.104043
Rahnama, Z., and Ansarifar, G. R. (2021). Predicting and optimizing the thermal-hydraulic, natural circulation, and neutronics parameters in the NuScale nuclear reactor using nanofluid as a coolant via machine learning methods through GA, PSO and HPSOGA algorithms. Ann. Nucl. Energy 161, 108375. doi:10.1016/j.anucene.2021.108375
Rasheed, A., San, O., and Kvamsdal, T. (2020). Digital twin: values, challenges and enablers from a modeling perspective. IEEE Access 8, 21980–22012. doi:10.1109/ACCESS.2020.2970143
Rattan, P., Penrice, D. D., and Simonetto, D. A. (2022). Artificial intelligence and machine learning: what you always wanted to know but were afraid to ask. Gastro Hep Adv. 1, 70–78. doi:10.1016/j.gastha.2021.11.001
Rodionov, A. (2007). Overview of NPPs component reliability data collection with regards to time-dependent reliability analysis applications.
Rodrigues, P. H. S., Maiorino, J. R., Asano, R., and Asano, P. T. L. (2022). New approach to find the equilibrium cycle in PWR reactors using genetic algorithms. Ann. Nucl. Energy 178, 109333. doi:10.1016/j.anucene.2022.109333
Rosen, M., and Farsi, A. (2022). “Seawater desalination systems using sustainable energy technologies,” in Sustainable energy technologies for seawater desalination (Elsevier), 277–360. doi:10.1016/B978-0-323-99872-7.00010-3
Safarzadeh, O., and Noori-kalkhoran, O. (2021). A fractional PID controller based on fractional point kinetic model and particle swarm optimization for power regulation of SMART reactor. Nucl. Eng. Des. 377, 111137. doi:10.1016/j.nucengdes.2021.111137
Sandhu, H. K., Bodda, S. S., and Gupta, A. (2023). A future with machine learning: review of condition assessment of structures and mechanical systems in nuclear facilities. Energies 16, 2628. doi:10.3390/en16062628
Santosh, T. V., Vinod, G., Saraf, R. K., Ghosh, A. K., and Kushwaha, H. S. (2007). Application of artificial neural networks to nuclear power plant transient diagnosis. Reliab Eng. Syst. Saf. 92, 1468–1472. doi:10.1016/j.ress.2006.10.009
Schokker, D., Poppe, M., Ten Napel, J., Athanasiadis, I. N., Kamphuis, C., and Veerkamp, R. F. (2022). Rapid turnover of sensor data to genetic evaluation for dairy cows in the cloud. J. Dairy Sci. 105, 9792–9798. doi:10.3168/jds.2022-22113
Shukla, A. K., Janmaijaya, M., Abraham, A., and Muhuri, P. K. (2019). Engineering applications of artificial intelligence: a bibliometric analysis of 30 years (1988–2018). Eng. Appl. Artif. Intell. 85, 517–532. doi:10.1016/j.engappai.2019.06.010
Singh, P., and Singh, L. K. (2021). Instrumentation and control systems design for nuclear power plant: an interview study with industry practitioners. Nucl. Eng. Technol. 53, 3694–3703. doi:10.1016/j.net.2021.05.025
Sleiti, A. K., Kapat, J. S., and Vesely, L. (2022). Digital twin in energy industry: proposed robust digital twin for power plant and other complex capital-intensive large engineering systems. Energy Rep. 8, 3704–3726. doi:10.1016/j.egyr.2022.02.305
Sobolev, A. V., Gazetdinov, A. S., and Samokhin, D. S. (2017). Genetic algorithms for nuclear reactor fuel load and reload optimization problems. Nucl. Energy Technol. 3, 231–235. doi:10.1016/j.nucet.2017.07.002
Song, H., Song, M., and Liu, X. (2022). Online autonomous calibration of digital twins using machine learning with application to nuclear power plants. Appl. Energy 326, 119995. doi:10.1016/j.apenergy.2022.119995
Sun, J., Zhang, R., Wang, M., Zhang, J., Qiu, S., Tian, W., et al. (2022). Multi-objective optimization of helical coil steam generator in high temperature gas reactors with genetic algorithm and response surface method. Energy 259, 124976. doi:10.1016/j.energy.2022.124976
Sun, M., Li, B., Yi, Z., Cao, K., Li, A., and Wang, Y. (2022). Optimization of surface free energy parameters for asphalt binder-aggregate system based on RBF neural network model. Constr. Build. Mater 357, 129382. doi:10.1016/j.conbuildmat.2022.129382
Sun, W., Song, C., Tang, C., Pan, C., Xue, P., Fan, J., et al. (2023). Performance of deep learning algorithms to distinguish high-grade glioma from low-grade glioma: a systematic review and meta-analysis. iScience 26, 106815. doi:10.1016/j.isci.2023.106815
Tanbay, T., and Durmayaz, A. (2023). Multiobjective optimization of a pressurized water reactor cogeneration plant for nuclear hydrogen production. Int. J. Hydrog. Energy 54, 49–65. S0360319923022292. doi:10.1016/j.ijhydene.2023.05.001
Toft, H. B., Müller, K., Hendrikx, J., Jaedicke, C., and Bühler, Y. (2023). Can big data and random forests improve avalanche runout estimation compared to simple linear regression? Cold Reg. Sci. Technol. 211, 103844. doi:10.1016/j.coldregions.2023.103844
Upadhyaya, B., and Kerlin, T. (2019). Dynamics and control of nuclear reactors. 1st ed. San Diego: Academic press is an imprint of Elsevier.
Vajpayee, V., Becerra, V., Bausch, N., Deng, J., Shimjith, S. R., and Arul, A. J. (2020). Dynamic modelling, simulation, and control design of a pressurized water-type nuclear power plant. Nucl. Eng. Des. 370, 110901. doi:10.1016/j.nucengdes.2020.110901
Vasumathi, B., and Moorthi, S. (2012). Implementation of hybrid ANN–PSO algorithm on FPGA for harmonic estimation. Eng. Appl. Artif. Intell. 25, 476–483. doi:10.1016/j.engappai.2011.12.005
Wang, H., Peng, M., Xu, R., Ayodeji, A., and Xia, H. (2020). Remaining useful life prediction based on improved temporal convolutional network for nuclear power plant valves. Front. Energy Res. 8, 584463. doi:10.3389/fenrg.2020.584463
Wang, J., Ge, D., Chen, S., Wang, Z., Guo, D., Xu, Z., et al. (2021). Maintenance strategy design for nuclear reactors safety systems using a constraint particle swarm evolutionary methodology. Ann. Nucl. Energy 150, 107878. doi:10.1016/j.anucene.2020.107878
Wang, H., Peng, M., Ayodeji, A., Xia, H., Wang, X., and Li, Z. (2021). Advanced fault diagnosis method for nuclear power plant based on convolutional gated recurrent network and enhanced particle swarm optimization. Ann. Nucl. Energy 151, 107934. doi:10.1016/j.anucene.2020.107934
Wang, J.-C., Shu, Y.-C., Lin, C.-Y., Wu, W.-T., Chen, L.-R., Lo, Y.-C., et al. (2023). Application of deep learning algorithms in automatic sonographic localization and segmentation of the median nerve: a systematic review and meta-analysis. Artif. Intell. Med. 137, 102496. doi:10.1016/j.artmed.2023.102496
Wang, K., Jia, J., Polat, K., Sun, X., Alhudhaif, A., and Alenezi, F. (2023). Smooth quantile regression and distributed inference for non-randomly stored big data. Expert Syst. Appl. 215, 119418. doi:10.1016/j.eswa.2022.119418
Wang, Y., Zheng, Z., Ji, D., Pan, X., and Tian, A. (2023). Machine learning-driven probabilistic seismic demand model with multiple intensity measures and applicability in seismic fragility analysis for nuclear power plants. Soil Dyn. Earthq. Eng. 171, 107966. doi:10.1016/j.soildyn.2023.107966
Wheatley, S., Sovacool, B., and Sornette, D. (2017). Of disasters and dragon kings: a statistical analysis of nuclear power incidents and accidents. Risk Anal. 37, 99–115. doi:10.1111/risa.12587
Worrell, C., Luangkesorn, L., Haight, J., and Congedo, T. (2019). Machine learning of fire hazard model simulations for use in probabilistic safety assessments at nuclear power plants. Reliab Eng. Syst. Saf. 183, 128–142. doi:10.1016/j.ress.2018.11.014
Xi, W., Liu, W., Bai, T., Ye, W., and Shi, J. (2020). An automation test strategy based on real platform for digital control system software in nuclear power plant. Energy Rep. 6, 580–587. doi:10.1016/j.egyr.2020.11.186
Xie, S., Chu, X., Zheng, M., and Liu, C. (2019). Ship predictive collision avoidance method based on an improved beetle antennae search algorithm. Ocean. Eng. 192, 106542. doi:10.1016/j.oceaneng.2019.106542
Xu, K., Liu, Z., Chen, Q., Zhang, Q., Ling, X., Cai, X., et al. (2024). Application of machine learning in wellbore stability prediction: a review. Geoenergy Sci. Eng. 232, 212409. doi:10.1016/j.geoen.2023.212409
Yadav, R. K., and Anubhav, (2020). PSO-GA based hybrid with Adam Optimization for ANN training with application in Medical Diagnosis. Cogn. Syst. Res. 64, 191–199. doi:10.1016/j.cogsys.2020.08.011
Yang, Q., Pan, Q., and Liu, X. (2023). Burnup-control drum coupling characteristics investigation of lead–bismuth eutectic-cooled solid reactor. Ann. Nucl. Energy 193, 110018. doi:10.1016/j.anucene.2023.110018
Yao, L., Yang, B., Cui, H., Zhuang, J., Ye, J., and Xue, J. (2016). Challenges and progresses of energy storage technology and its application in power systems. J. Mod. Power Syst. Clean. Energy 4, 519–528. doi:10.1007/s40565-016-0248-x
Yassir, A., Jai Andaloussi, S., Ouchetto, O., Mamza, K., and Serghini, M. (2023). Acoustic fish species identification using deep learning and machine learning algorithms: a systematic review. Fish. Res. 266, 106790. doi:10.1016/j.fishres.2023.106790
Yüksel, N., Börklü, H. R., Sezer, H. K., and Canyurt, O. E. (2023). Review of artificial intelligence applications in engineering design perspective. Eng. Appl. Artif. Intell. 118, 105697. doi:10.1016/j.engappai.2022.105697
Zhang, X., Yang, H., Ding, Y., Dong, B., Song, Y., Zhou, W., et al. (2023). PLIF experiment and verification of boron mixed diffusion model driven by turbulence in nuclear reactor. Ann. Nucl. Energy 184, 109682. doi:10.1016/j.anucene.2023.109682
Zhang, Y. (2023). Advanced nuclear power engine: a brief overview of gas core reactor for space exploration. Int. J. Adv. Nucl. React. Des. Technol. 5, 53–71. doi:10.1016/j.jandt.2023.05.001
Zhang, Y., Gao, P., Tian, C., He, X., Chen, C., and Wang, Z. (2021). Coupling one-dimensional user code and STAR-CCM+ for simulation of rolling conditions in a rod bundle channel. Ann. Nucl. Energy 151, 107888. doi:10.1016/j.anucene.2020.107888
Zhao, J., Knight, B., Nissan, E., and Soper, A. (1998). FuelGen: a genetic algorithm-based system for fuel loading pattern design in nuclear power reactors. Expert Syst. Appl. 14, 461–470. doi:10.1016/S0957-4174(98)00005-0
Zhao, J., Li, Y., Bai, J., Ma, L., Shi, C., Zhang, G., et al. (2023). Multi-objective optimization of marine nuclear power secondary circuit system based on improved multi-objective particle swarm optimization algorithm. Prog. Nucl. Energy 161, 104740. doi:10.1016/j.pnucene.2023.104740
Zheng, L., Wang, C., Chen, X., Song, Y., Meng, Z., and Zhang, R. (2023). Evolutionary machine learning builds smart education big data platform: data-driven higher education. Appl. Soft Comput. 136, 110114. doi:10.1016/j.asoc.2023.110114
Zhong, X., and Ban, H. (2022). Crack fault diagnosis of rotating machine in nuclear power plant based on ensemble learning. Ann. Nucl. Energy 168, 108909. doi:10.1016/j.anucene.2021.108909
Zhou, X., Wang, J., Wang, H., and Lin, J. (2022). Panel semiparametric quantile regression neural network for electricity consumption forecasting. Ecol. Inf. 67, 101489. doi:10.1016/j.ecoinf.2021.101489
Zhu, F., Wu, X., Zhou, M., Sabri, M. M. S., and Huang, J. (2022). Intelligent design of building materials: development of an AI-based method for cement-slag concrete design. Materials 15, 3833. doi:10.3390/ma15113833
Keywords: nuclear power plants, artificial intelligence, big data, data-driven model, safety, clean energy
Citation: Ejigu DA, Tuo Y and Liu X (2024) Application of artificial intelligence technologies and big data computing for nuclear power plants control: a review. Front. Nucl. Eng. 3:1355630. doi: 10.3389/fnuen.2024.1355630
Received: 14 December 2023; Accepted: 13 February 2024;
Published: 23 February 2024.
Edited by:
Jianjun Xiao, Karlsruhe Institute of Technology (KIT), GermanyReviewed by:
Yandong Hou, Northeast Electric Power University, ChinaCopyright © 2024 Ejigu, Tuo and Liu. This is an open-access article distributed under the terms of the Creative Commons Attribution License (CC BY). The use, distribution or reproduction in other forums is permitted, provided the original author(s) and the copyright owner(s) are credited and that the original publication in this journal is cited, in accordance with accepted academic practice. No use, distribution or reproduction is permitted which does not comply with these terms.
*Correspondence: Xiaojing Liu, eGlhb2ppbmdsaXVAc2p0dS5lZHUuY24=
Disclaimer: All claims expressed in this article are solely those of the authors and do not necessarily represent those of their affiliated organizations, or those of the publisher, the editors and the reviewers. Any product that may be evaluated in this article or claim that may be made by its manufacturer is not guaranteed or endorsed by the publisher.
Research integrity at Frontiers
Learn more about the work of our research integrity team to safeguard the quality of each article we publish.