Amyotrophic lateral sclerosis (ALS) is a neurodegenerative disease with degeneration of motor neurons and characterized by progressive muscle weakness and atrophy. After an average of 3–5 years, patients ultimately loose the ability to initiate and control all voluntary movements, except those of the eyes, becoming tetraplegic and anarthric. Some patients enter the locked-in and totally locked-in stage. Two of the main issues about this neurological disorder are related to the assessment of the cognitive status (
Iversen et al., 2008
;
Kotchoubey et al., 2003
;
Ringholz et al., 2005
) and the restoration of communication with the social environment, especially during the late stage (
Kübler and Birbaumer, 2008
). Recent studies focused on the effectiveness of the BCI technology as a communication tool for patients affected by ALS or other severe motor disabilities. A wide range of brain signals could be used for this purpose, such as slow cortical potentials (SCPs,
Kuebler et al., 1998
), sensorimotor rhythms (SMRs,
Wolpaw et al., 1991
), steady state visual evoked potentials (
Cheng et al., 2002
), near infrared spectroscopy (
Coyle et al., 2004
), and event-related potentials (ERPs,
Farwell and Donchin, 1988
). Some of these studies involved ALS patients, mainly in the late stage of the disease, in order to offer them a reliable instrument for communication (
Kübler and Birbaumer, 2008
).
Birbaumer et al. (1999)
trained two severely paralysed subjects to spell words using SCP-control, resulting in the first verbal message written by a locked-in patient with a BCI. Over the last decade other studies reported similar BCI communication results with severely paralysed subjects, using different brain signals (ERPs,
Sellers and Donchin, 2006
; SMRs,
Wolpaw and McFarland, 2004
; SMRs,
Kübler et al., 2005
; ERPs,
Nijboer et al., 2008
; SMRs,
Neuper et al., 2003
). Moreover, the BCI technology was also successfully used for movement restoration (
Buch et al., 2008
;
Hochberg et al., 2006
;
Müller-Putz and Pfurtscheller, 2008
;
Pfurtscheller et al., 2003
).
Summarizing, it has been shown that patients with severe physical impairment, and patients in the locked-in state (LIS), were able to successfully communicate using a BCI. Nonetheless, some authors reported that the BCI technology has been unable “to restore basic communication (yes/no) in patients who were in the complete locked-in state (CLIS, without measurable eye movements) at the beginning of the BCI training” (
Birbaumer, 2006b
;
Kübler and Birbaumer, 2008
). To prevent the failure of completely paralysed patients to achieve BCI communication, the same authors have suggested that patients should begin the BCI training before entering the LIS. Two reasons were proposed that could affect the learning of BCI-skills: decrease of cognitive abilities and/or “extinction of output-directed and goal-oriented thoughts” (
Birbaumer, 2006a
). About 50% of the ALS patients at the late stage of the disease may be affected by mild to severe cognitive impairment (
Ringholz et al., 2005
); moreover, without a feedback, it is difficult to prove correct comprehension and use of the BCI task. To date, all studies using BCI for maintenance of communication in ALS employed very few patients and used different types of BCI-systems and different brain signals. In a meta-analysis of all reviewed publications
Kübler and Birbaumer (2008)
concluded that there was no relationship between severity of the disease and the BCI communication skill, except for completely locked-in ALS patients unable to learn to use a BCI. Moreover, only seven completely locked-in patients were investigated and reported in this meta-analysis, again with heterogeneous BCI-methodology, making it difficult to reach definite conclusion. Kübler and Birbaumer’s opinion contrasts with the
Piccione et al. (2006)
study (of our group) reporting reduced BCI-control with disease progression. In addition, because of the small number of patients employed and only successful performance reported, it is still unclear how many ALS patients may learn to reliably control a BCI and whether those who learn the skill are able to maintain it.
Considering these crucial observations, the present study was aimed to address/investigate in a larger group whether ALS patients in the early and intermediate stage of the disease could successfully use a P300-based BCI, and how many fail during follow-up before entering the LIS; secondarily, to investigate the relationship between acquired BCI-skill and the clinical status, including the cognitive status and the degree of physical impairment. This report is the first of a longitudinal study, aimed to clarify patient’s performance early and in the late stage of the disease.
Design of the Study
Amyotrophic lateral sclerosis patients were evaluated at admission to the study and 1 year later. Participants underwent four types of measurements: (i) physical disability, (ii) neuropsychological status, (iii) neurophysiological assessment, and (iv) communication with the BCI. All measurement were carried out at S.Camillo Hospital in Venice, during the rehabilitation period of the patients. The research was approved by the Ethical Committee of the San Camillo Hospital. Written informed consent was obtained from patients and families according to the Declaration of Helsinki.
Participants
A group of 21 patients with ALS diagnosis based on the El Escorial criteria, admitted to the S.Camillo Hospital for rehabilitation treatment, participated in the study. Due to a long hospital admission interval (at least 1 year), and in two cases death, only five of these patients performed the follow-up. The physical disability level of patients was assessed using the revised ALS functional rating scale (ALSFRS-R,
Cedarbaum et al., 1999
) which includes autonomic, respiratory, and motor functions. LIS or CLIS patients were not admitted to the study. The ALSFRS-R score was re-evaluated in five patients who performed the follow-up. We also assessed the disease duration as the number of months since the appearance of the first symptoms in all patients (see Tables
1 and
2
).
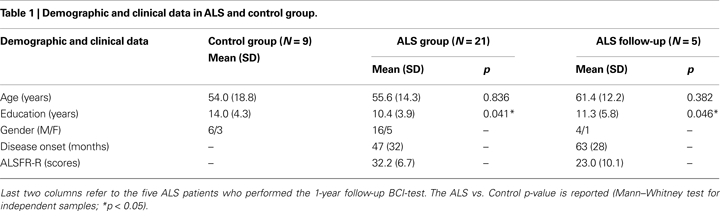
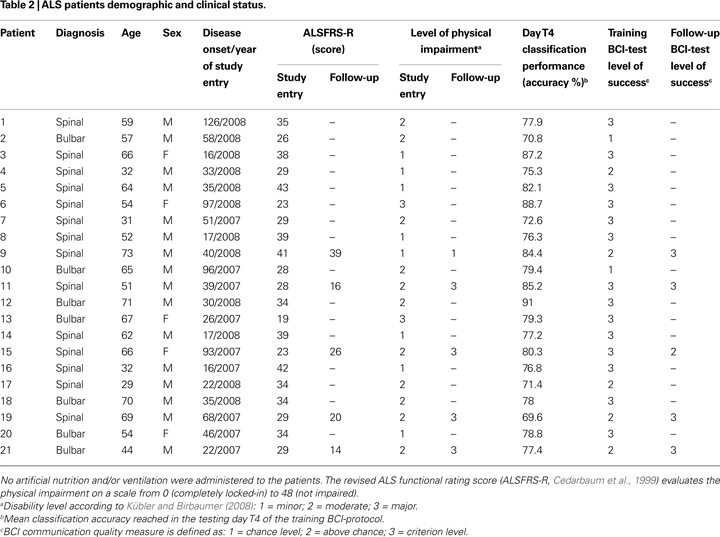
Following
Kübler and Birbaumer (2008)
, we divided our patients in three categories according to the definitions provided by the authors:
minor (normal speech, slightly impaired movement);
moderate (restricted limb movement and unaffected speech, or intact limb movement without speech);
major (almost tetraplegic with restricted speech). Table
2
reports clinical description of each patient. An age matched control group of nine healthy participants were also recruited to compare cognitive capabilities and the communication skill obtained with the BCI-system.
Neuropsychological Evaluation
Global cognitive impairment was assessed with the mini-mental state examination (MMSE,
Folstein et al., 1975
). A battery of psychometric tests (
Phukan et al., 2007
; see Table
3
) was administered to evaluate
non-verbal intelligence (Raven’s coloured matrices,
Raven, 1965
),
attention (attentive matrices,
Spinnler and Tognoni, 1987
),
executive functions [modified Wisconsin card sorting test (WCST),
Nelson, 1976
; phonemic verbal fluency,
Spinnler and Tognoni, 1987
; digit span backward,
Wechsler, 1998
; trail making test A and B,
Reitan, 1958
,
short-term memory (verbal digit span forward,
Wechsler, 1998
; Corsi blocks tapping test,
Spinnler and Tognoni, 1987
),
long-term memory (prose memory,
Spinnler and Tognoni, 1987
), and
language (semantic verbal fluency,
Spinnler and Tognoni, 1987
). All patients had almost preserved speech, reading and writing abilities to perform neuropsychological tests, except two patients with
major impairment who were unable to write. Table
3
reports the mean psychometric scores of the two groups; depending on patients’ abilities, some statistics were evaluated in a restricted number of patients. The five follow-up patients received the same assessment after 1 year.
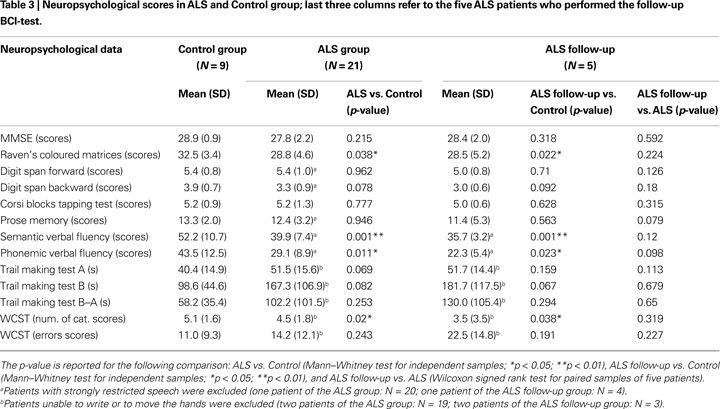
ERPs Data Acquisition
The following set-up was used for the neurophysiological assessment and for BCI experiments. Silver-chloride electrodes were placed according to the international 10–20 system at FZ, CZ, PZ, and OZ; the electrooculogram (EOG) was recorded from a pair of electrodes below and laterally to the left eye; all electrodes were referenced to the left earlobe. The five channels were amplified by SynAmps (NeuroSoft, Inc.), band-pass filtered between 0.15 and 30 Hz, and digitized (with a 16-bit resolution) at 200 Hz sampling rate. Because ERPs recordings need a sequence of stimuli a trigger signal was stored at the EEG channels; the inter-stimulus interval was paradigm-dependent. Every ERP epoch, synchronized with the trigger, began 500 ms before the stimulus onset, up to 1000 ms after the stimulus. Thus, after each epoch the system recorded a matrix of 300 samples for five channels, available for off-line and on-line data processing.
Neurophysiological Assessment
Event-related potentials recordings were performed using an active auditory odd-ball paradigm (
Piccione et al., 2006
;
Polich, 2007
). Non-target tones (1000 Hz, 70 dB,
p = 0.8) were alternated with target tones (2000 Hz, 70 dB,
p = 0.2) in a pseudo-random sequence. A sequence of 60 stimuli were presented with an average inter-stimulus interval of 2 s (duration up to 2 min); participants were asked to count the target stimuli. Four sequences were administered to each participant, leading to a total of 240 stimuli for each subject in each session. All epochs with an EEG amplitude larger than 100 μV (
Cohen and Polich, 1997
) were removed; thus, the remaining traces were filtered with a 4-order and zero-phase low-pass filter at 15 Hz, and grouped according to the stimulus type. ERP components were obtained by averaging at least 20 artefact-free target EEG epochs (see Figure
1
). The P300 component was identified as the most positive peak after 270 ms, while N200 component was distinguished as the most prominent negativity preceding the P300. Three examiners manually measured the P300 latencies and absolute peak-to-peak amplitudes (N200-P300) from average data of channel PZ. The examiners were blind to each other’s results. To compare the P300 parameters we used normative data of the International Federation of Clinical Neurophysiology (
Heinze et al., 1999
) and data of 50 healthy participants recruited from our laboratory. Table
4
shows the mean P300 latencies and amplitudes of the two groups, as well as the norm data.
Figure 1. Auditory odd-ball paradigm ERPs grand averages. (A) Control, (B) ALS. Mean ± SD number of target stimuli: 34 ± 9 (non-target stimuli: 168 ± 23). The auditory odd-ball paradigm was administered to each participant before the BCI training.
BCI-System
Participants were seated in a sound-attenuated chamber facing a computer screen. They were asked to control the movement of a cursor (blue circle) from a central starting point to one out of four target-points in peripheral positions of the screen, corresponding to four icons representing basic needs (i.e. “I’m hungry”, “I’m sleepy”, “I would like to eat an apple”, “I need a doctor”, “I would like to drink something”, “I need to wash myself”, etc.). The initial distance between the starting point and the target-icon encompassed four discrete steps. Upward, rightward, downward, and leftward arrows were randomly flashed in peripheral positions of the screen, near the position of the icons (Figure
2
A). Each arrow indicated one out of four possible directions concerning the movement of the cursor. The participants were asked to move the cursor, along the horizontal or vertical meridian towards the target-icon specified by the computer; they had to pay attention to one arrow indicating the required direction (i.e. flashed target arrow;
p = 0.25), but to ignore the arrows indicating the wrong directions (i.e. distracting arrows;
p = 0.75). Each trial consisted of flashing an arrow for 150 ms (Figure
2
B), followed by data processing necessary for P300 recognition, and by the generation of feedback concerning the movement of the cursor (Figure
2
C). The time interval between two flashed arrows (inter-trial interval) was fixed to 2.5 s (
Polich, 2007
) to achieve optimal on-line data processing. A session was defined as the complete sequence of trials necessary to reach the target-icon (range: 13–92 trials, from about 30 s up to 3 min and 50 s); when an icon was reached the interface program plays a sound file verbalizing the requested wish. Every time the P300 was detected during the trial, the cursor moved at the screen in the direction of the flashed arrow. Each participant performed a training BCI-protocol composed of eight acquisition sessions (AS), and 16 testing sessions (TS) spread over 2 weeks (see Table
5
). On a testing day one icon for each direction was chosen as a target; for each testing day the collection of the four target-icons was different.
Figure 2. Graphics during a single trial. (A) the cursor, the target-icons and the four arrows; (B) the flashing arrow; (C) the movement of the cursor after P300 recognition.
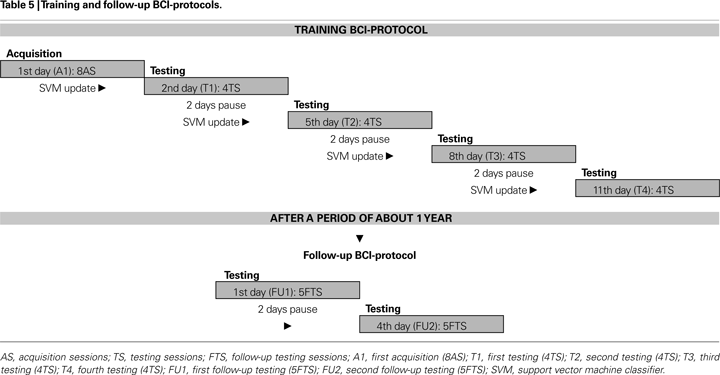
During this protocol (from A1 to T3, see Table
5
) all participants, except those with
major impairment, were asked to press a key every time a target arrow flashed. After a period of 12 months, five participants performed a follow-up BCI-protocol composed by ten testing sessions (FTS), except one patient who performed only eight FTS; these sessions were spread over 1 week as depicted in Table
5
. During day T4 and during follow-up tests the “press-key” instruction was removed. During the AS the cursor made one step towards the target-icon, every time the corresponding target arrow flashed. In contrast, during the TS the cursor moved on the screen only when a brain wave were recognized as a P300 using a support vector machine classifier (SVM, see “BCI Data Analysis” ). After the first day (A1) only TS were carried out, updating the classifier before each testing day; in this way the feedback to the participant was always real during the learning. ERPs recordings during the execution of the BCI task were analysed with the procedure described in “Neurophysiological Assessment”; for this purpose all target and non-target epochs of the training BCI-protocol (16 sessions, from T1 to T4) were analysed. Mean P300 latencies and amplitudes of the two groups are reported in Table
6
; Figure
3
shows the target and non-target grand averages.
Figure 3. Training BCI-test ERPs grand averages. (A) Control, (B) ALS. Mean ± SD number of target stimuli: 181 ± 54 (non-target stimuli: 541 ± 189).
BCI Data Analysis
A modified version of the classification procedure reported in a previous study (
Piccione et al., 2006
) was used for the BCI-system. Before each testing day (i.e. T1, T2, T3, and T4, see Table
5
), a classifier adapted for each participant separately was trained with a stepwise procedure: independent component analysis (ICA) decomposition (
Hyvarinen, 1999
;
Makeig et al., 1997
) and ICA component selection (
Beverina et al., 2004
), fixed features extraction (
Piccione et al., 2006
), and SVM classification (
Joachims, 1999
;
Piccione et al., 2008
;
Thulasidas et al., 2006
). To decompose ERPs signals into spatially fixed and temporally independent components we used the FastICA implementation (
Hyvarinen, 1999
). In the second step an automated selection of the component which reflects the ERP’s morphology was performed. This automated selection was based on a fuzzy method that determines the most salient P300 deflection (
Beverina et al., 2004
). The selection was based on fuzzy rules, while the P300 template parameters were derived from the ERPs recordings of the AS for each participant (Table
5
, A1 sessions). Then, these P300 template parameters were used to adjust the fuzzy system membership function parameters. Considering the selected component, for each ERP epoch we obtained a single-sweep normalized data vector (300 points) used for feature extraction. In the third step, 78 values (features) were extracted from the 300 points ICA-transformed data (
Piccione et al., 2006
). These features constituted a single vector pattern used as input to train and to test the classification system. All these operations were performed off-line before training the SVM classifier. For each updating of the SVM classifier we collected all available ERPs epochs in a dataset, except those of the last session. Before training the classifier with a 20-fold cross-validation procedure, the dataset was reduced excluding all epochs with an EEG activity larger than 100 μV (
Cohen and Polich, 1997
). All these epochs were ICA-transformed and synthesized by the features (leading to a
N × 78 patterns matrix, were
N depends on the number of trials of each single session). Each training set consisted of a randomly chosen pattern subset corresponding to 80% of the pattern dataset, while each validation testing set was composed of the remaining 20% of patterns. A further validation was performed using all data of the excluded session. To train and to test each SVM classifier we used the implementation provided by the software SVM
light ver. 5.00 (
Joachims, 1999
). All classifiers were trained with the radial basis function kernel option which uses two parameters:
gamma and
cost factor. The
gamma parameter was fixed, while the
cost factor was optimized before starting each 20-fold cross-validation procedure; this optimization was obtained minimizing an objective function that takes into account the target and non-target errors. We did not change the SVM classifier for the follow-up sessions, hence, the classifier trained for the testing day T4 was used in the follow-up tests too; with this procedure all FTS sessions were compared with the sessions of the fourth testing day (T4). During on-line recordings, the classification procedure (ICA, component selection, features, SVM) was applied to every single-sweep synchronized with the stimulus, while the output of the classifier was converted to a binary value (1: P300 detected; 0: P300 not detected) to control the discrete movements of the cursor. If the cursor reached the target-icon, the session was defined “successfully completed”; this implied that at least four epochs related to the target direction were correctly classified; otherwise it was defined “unsuccessful”. Moreover, the number of stimuli needed to reach the first time a target-icon (different for each participant) was defined as training number of stimuli (TNS); it estimates the minimal length, in terms of stimuli, needed to achieve an effective BCI-control.
BCI Performance
Brain–computer interface performance was described by the following five measures (all 16 TS were considered, see Table
7 and Figure
4
A): mean classification performance (i.e. target and non-target single-sweep classification accuracy%), transfer bit rate (bit/min, according to
Piccione et al., 2006
), percentage of “successfully completed” sessions (%), TNS, and the classification performance trend for the 16 TS of the training BCI-protocol (%/session), evaluated with a linear regression. Two further measures was used to monitor the effect of on-line artefacts on participants’ performance (epochs with an EEG activity larger than 100 μV were considered as an artefact,
Cohen and Polich, 1997
): BCI-system
weakness as the percentage of target epochs classified as
true positive (TP) and containing an artefact with respect to all target epochs; BCI-system
robustness as the percentage of non-target epochs classified as
true negative (TN) and containing an artefact, with respect to all non-target epochs with artefacts.
Weakness measure refers to the influence of on-line artefacts on correct movements of the cursor;
robustness measure refers to the capability of the algorithm to reduce/avoid wrong movements of the cursor caused by artefacts (
false positive reduction). Follow-up BCI-skill performance was evaluated with the same parameters described above, except for the TNS measure (see Table
8 and Figure
4
B).
Figure 4. (A) Target and non-target single-sweep classification accuracy (%) of the two groups, Control and ALS, during training BCI-test; (B) target and non-target single-sweep classification accuracy (%) of five ALS patients during training and follow-up BCI-test.
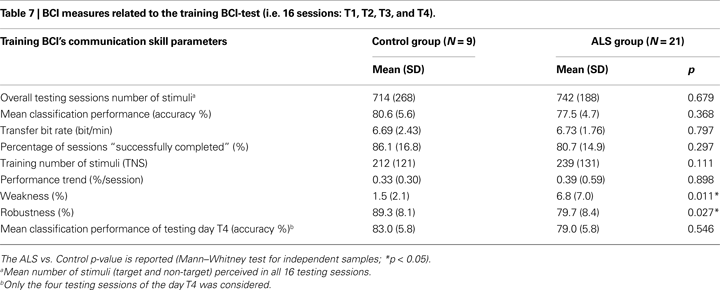
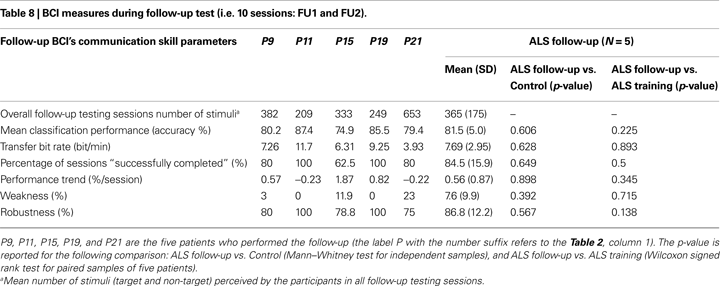
To evaluate the quality of the BCI-based communication and to carry out a comparison with the results reported by
Kübler and Birbaumer (2008)
, we defined three different level of success: first
chance level, second
above chance, and third
criterion level (
Kübler and Birbaumer, 2008
). Given a single session, two outcomes are possible: “successful” or “unsuccessful” (each of which with probability
p and
q = 1 −
p). Repeating sessions without a classifier updating the probability of success of each session is almost constant (each session was independent from each other session). These two conditions allowed us to use the binomial distribution law to decide whether a participant achieved a given level of success (
Bernoulli, 1713
;
Müller-Putz et al., 2008
). For this purpose we evaluated the chance probability to obtain the “successful” outcome in
N sessions. Hence, for each participant, we used the last four sessions of the training BCI-protocol (testing day T4) to evaluate the level of success counting the number of “successful” outcomes and calculating the chance probability (during T4 we did not update the SVM classifier). With the same hypothesis we evaluated the level of success of each patient during the follow-up. Given one selection (session), the probability to select a correct (target) icon by chance was
p = 1/
N, were
N is the number of choices (with
N = 4 icons,
p = 0.25), and the probability to select a wrong (non-target) icon by chance was
q = 1 −
p.
Given
n selections (i.e. four target-icons to be selected during day T4) the probability to select
k correct icons by chance is
pn(
S =
k) = [
n!/(
k!(
n −
k)!)]
pkqn−k, where
S constitutes the number of successes. With
pn(
S =
k) ≥ 0.05 we denoted the
chance level, meaning that no communication is possible with the BCI. For example, successfully completing two sessions in the testing day T4 only the
chance level is reached [given
n = 4,
pn(
S = 2) = 0.211, see Table
9
. The number
X of icons necessary to be
above the chance level of α < 0.05, is determined by
pn(
S =
X) < 0.05; the
above chance level, indicates that cursor control and the classification rates reached statistical significance, but this does not mean that a reliable BCI-based communication can be achieved. Finally, we defined with
Y the number of icons necessary to reach the
criterion level of α < 0.01, determined by
pn(
S =
Y) < 0.01 (see Table
9 and Figure
5
A); for this case a reliable communication is possible. To allow a comparison with
Kübler and Birbaumer’s (2008)
results level of success was ranked over the entire patient sample using two criteria: first the level of success and then the classification accuracy in the testing day T4. We first grouped all patients on the basis of their level of success. In a second stage, we ranked patients from least successful to the most successful (i.e. from 1 to 21) starting with the group which operated at
chance level; for each patient at the
chance level we assigned the rank on the basis of the classification accuracy (highest rank for highest accuracy). Then we continued the ranking considering the second group (
above chance); also in this case we assigned the consecutive ranks on the basis of the classification accuracy. Finally we completed the ranking considering the third group (
criterion level); the consecutive ranks were assigned again according to the classification accuracy. Summarizing, to the patients who reached the
chance level the assigned ranks were 1 and 2; to the patients who reached the
above chance level the assigned ranks started from 3 up to 7; to the patients who reached the
criterion level the assigned ranks started from 8 up to 21 (see Tables
2 and
9
). In this way, the ranking procedure assigns more importance to the successful task completion instead of the single trials successful classification. Then, ranks were grouped for each category of impairment and mean values were calculated, dividing the sum of ranks by the number of patients in each category (see Figure
5
B). All these calculations were carried out for the five patients who performed the follow-up BCI-test too.
Figure 5. (A) Chance probability of successful selection depending on the number of selections; with “group A” we refer to the four icons to be selected during testing day T4 (at least three successful selections to be above chance); with “group B” we refer to the eight icons to be selected during follow-up test by one ALS patient (at least five successful selections to be above chance); with “group C” we refer to the 10 icons to be selected during follow-up test by the remaining four ALS patients (at least six successful selections to be above chance). Above the red line (α = 0.05) level of success was considered at chance level; between red and black lines level of success was considered above chance; under the black line (α = 0.01) level of success was considered at criterion level. (B) ALS patients’ mean rank of level of success (training BCI-test), and degree of physical impairment; as showed the mean level of success is almost constant for each category of impairment.
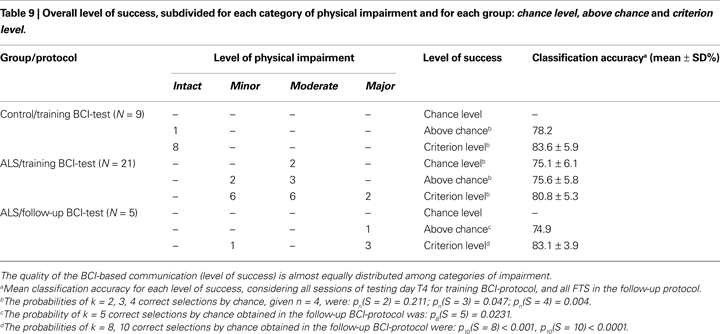
Statistical Evaluation
We report participants mean (SD) of the following variables: demographic and clinical (Table
1
; Table
2
for details); neuropsychological (Table
3
); neurophysiological (Tables
4 and
6
); training BCI-test communication skill (Table
7
); follow-up BCI-test communication skill (Table
8
); quality of the BCI-based communication (level of success, Table
9
). To evaluate the differences between ALS and Control group we used Mann–Whitney non-parametric test; Wilcoxon signed rank test was also used to compare training and follow-up BCI-test communication skill parameters (paired samples). Due to the small sample size of the follow-up measures (
N = 5), we reported individual’s BCI-performance parameters (Table
8
). To examine the possible relations among clinical, neuropsychological, neurophysiological, and BCI data, Spearman’s rank correlation was used (referred below with the symbol “
r”). In particular, the relationship between physical impairment and BCI performance was assessed in two ways: firstly we calculated the Spearman’s rank correlation between ALSFRS-R and BCI measures; in a second stage, we employed the Kruskal–Wallis rank test (for
n independent samples), using the ranks obtained with the ranking procedure reported above, although the number of patients with
major physical impairment was small (
N = 2). The result of the Kruskal–Wallis test may be compared with the results obtained by
Kübler and Birbaumer (2008)
.
Considering demographics data, only the education level differed significantly between Control and ALS groups (
U = 58.5,
p = 0.041). The subjects of the Control group had longer education because them were recruited from academia; most ALS patients have reached only the secondary school degree. All participants, healthy subjects and ALS patients, performed within normal ranges in all neuropsychological tests. In the first comparison (nine Control vs. 21 ALS, Table
3
) a mild cognitive deficit in ALS was evidenced by significant differences in tests mainly related to the attentive and executive functions: semantic verbal fluency (
U = 28.5,
p = 0.001), phonemic verbal fluency (
U = 42,
p = 0.011), Raven’s coloured matrices (
U = 53,
p = 0.038), WCST cat. (
U = 48.5,
p = 0.02). These results may suggest a sub-clinical cognitive impairment in ALS. The results of the follow-up neuropsychological evaluation (see Table
3
) indicate the same cognitive profile after 1 year, although the small sample size strongly limits this result (
N = 5, and in some test
N = 3, see Table
3
footnotes).
The neurophysiological data (see Table
4
) revealed odd-ball P300 waves parameters (amplitude and latency) within norm data collected in our laboratory. A significant difference for P300 amplitude during the training BCI task was found between ALS and Control group (
U = 25,
p = 0.004), ALS showing smaller amplitudes (see Table
6
).
Comparing BCI-performance measures of the two groups in the training BCI-protocol, we found the following significant differences:
weakness measure (
U = 32,
p = 0.011), and
robustness measure (
U = 38,
p = 0.027); since there were no significant differences in classification accuracy (
U = 63.5,
p = 0.368) and percentage of “successfully completed” sessions (
U = 61,
p = 0.297), the negative effects of the BCI-system
weakness and
robustness seem to be compensated. No other significant differences were found in the training BCI-protocol (see Table
7
), showing that ALS and Control groups achieved a comparable cursor control. Considering the five ALS patients who performed the follow-up, a comparison between BCI-skill of the training and follow-up protocols did not revealed any difference (see Table
8
). Similarly, no other significant differences were found in the ALS follow-up vs. Control comparison (see Table
8
). With the small sample size limitation (
N = 5), these data support the hypothesis that patients maintained their performance over time. These results were also corroborated by the “level of success” of each participant (see Tables
2 and
9
), even if the overall performance of one patient decreased in the follow-up (see Table
2
, patient 15). Moreover, the statistical analysis revealed a significant correlation between ALS patients’ age and some BCI parameters: mean classification performance (
r = 0.62,
p = 0.006), transfer bit rate (
r = 0.49,
p = 0.037), and mean classification performance of the testing day T4 (
r = 0.47,
p = 0.032). With increasing age better performance was achieved.
No other significant correlations were found between BCI-skills and clinical data of the ALS group as reported by
Nijboer et al. (2008)
. The following comparisons refer to the training BCI-test (
N = 21): mean classification accuracy and ALSFRS-R (
r = 0.07,
p = 0.772); mean classification accuracy and disease duration (
r = 0.02,
p = 0.947); percentage of “successful sessions” and ALSFRS-R (
r = 0.17,
p = 0.491); percentage of “successful sessions” and disease duration (
r = −0.27,
p = 0.286). Using the ALSFRS-R scores and the BCI measures of the follow-up we obtained the following correlation coefficients (
N = 5): mean classification accuracy and ALSFRS-R (
r = −0.2,
p = 0.747); percentage of “successful sessions” and ALSFRS-R (
r = −0.37,
p = 0.541).
Even if the number of patients with
major physical impairment was small (
N = 2), we compared the correlation between level of success (training BCI-test) and physical disability with the results reported by
Kübler and Birbaumer (2008)
. Ranks of level of success were grouped as described above ( “BCI Performance”, Figure
5
B). Ranks did not differ between the categories (χ
2 = 2.93, df = 2,
p = 0.231, Kruskal–Wallis rank test for independent samples) confirming the results of the Spearman’s rank correlation reported above, and the results of these authors. Finally, we found that the participants who reached the
criterion level of success had a mean classification accuracy (testing day T4) of about 80% (see Table
9
), which differed slightly from 70% generally reported (
Choularton and Dale, 2004
;
Kübler and Birbaumer, 2008
;
Kübler et al., 2004
;
Nijboer et al., 2008
;
Perelmouter and Birbaumer, 2000
); while the participants who reached the
above chance level had a mean classification accuracy of about 75%. These findings suggest that on-line operations, with immediate feedback, the type of paradigm/interface, and the classification system, could be relevant factors to determine a
criterion level accuracy threshold.
In this study we investigated whether a larger group of ALS patients, in the early and middle stage of the disease, successfully use a P300-based BCI and maintain this skill during follow-up before entering the LIS; in addition, we tested if a relationship exists between acquired BCI-skill and the clinical status of the patients. The study involved also a control group of healthy subjects.
Neuropsychological results (21 ALS vs. 9 Control) indicated a mild cognitive impairment in ALS patients, mainly evident in the attentive and executive functions, comparable to results reported by
Ringholz et al. (2005)
. It cannot be excluded, however, that these mild cognitive deficits are a consequence of subtle motor or sensory impairments (
Birbaumer, 2006a
). The follow-up neuropsychological data comparison suggests the absence of a further cognitive decline, although the small sample size limits this result.
Neurophysiological data revealed a smaller P300 wave’s amplitude of the ALS group during the training BCI task; however no significant difference was found in the P300 wave’s amplitude of the classical odd-ball task during neurophysiological assessment. Examining our 4-class (4 selections) BCI task and the two-class odd-ball task, at least three differences should be noted: the total number of stimuli which was 4 for the BCI task instead of two used in the odd-ball task; the time required to complete the task which lasted up to 4 min for the BCI task instead of 2 min required for the odd-ball task; between two consecutive flashed arrows the BCI paradigm provides a visual feedback, while the odd-ball paradigm does not provide a feedback between auditory stimuli. The BCI task feedback captures subjects’ attention and engages processing resources. These differences between the two tasks imply a higher complexity of the BCI task compared to the odd-ball task making it more difficult. Hence, considering the BCI paradigm, it seems that the combination of more attentional resources with the sustained processing resources demanding task, produced a smaller P300 in ALS patients (
Miniussi et al., 1999
;
Nobre, 2001
;
Polich, 2007
); then, it is plausible that the complex BCI task affected the available attentional resources of these patients.
Addressing the first question of this study, we analysed the BCI-skill performance of the Control and ALS groups, which were reported in Tables
7 and
8
: the average of “successfully completed” sessions was about 80%; the training time was very short for both groups, participants performing the first successful session within a few days; the communication speed was low compared to others studies (
Hoffmann et al., 2008
;
Nijboer et al., 2008
), but there is also a slight positive trend of classification performance over time, indicating a
learning process (
Millán, 2003
). The main differences of the two groups are related to the
weakness and
robustness of the BCI-system; the
weakness depends on target events (
p = 0.25), while the
robustness depends on non-target stimuli (
p = 0.75); for the ALS group, it may be argued that with lower
robustness, the higher would be the number of wrong cursor movements (
false positive); also the increase of BCI-system
weakness leads to an higher number of correct cursor movements; therefore patients found an optimal strategy to compensate for high error rate. No significant differences were found in classification accuracy and percentage of “successfully completed” sessions of the training BCI-test; this findings seem to strengthen the hypothesis of the
robustness/
weakness compensation, and shows that the two groups, Control and ALS, achieved a comparable cursor control. A further comparison between the BCI-skill of the training and follow-up protocols did not revealed any difference, although the sample size is small. With this limitation, this result confirms the assumption that these patients maintained their communication abilities even after a long period, and even if the physical disability progresses (see Table
2
). Furthermore we observed that all participants reached at least the
above chance level control of the cursor movements during training BCI-protocol, except two patients with a
moderate physical impairment; in particular, 14 patients reached the
criterion level of the cursor control selecting a target-icon. During follow-up BCI-test four patients reached the
criterion level, while one patient reached the
above chance level.
The positive correlation between patients’ age and some BCI-skill parameters may indicate that age and other individual factors could have positively influenced acquisition of the BCI-skill. The older a patient is, the higher would be his attribution of the BCI communication tool’s utility, especially with the awareness of the late stage disease’s implications; consequently the higher would be his motivation to achieve control over a BCI. A similar result was found by
Kübler and Birbaumer (2008)
.
Considering the second question of the study, we did not found any significant relationship between the BCI-skill of the patients and their clinical status, including the cognitive capabilities. In particular, a comparison between training BCI performance and ALSFRS-R score did not revealed any significant relationship, supporting the results reported by
Nijboer et al. (2008)
. Comparing the same data of the follow-up evaluation the result was confirmed, even if the small sample size (
N = 5) limits this conclusion. An analysis of the disability level and the BCI level of success (only training BCI-protocol) supports the conclusion reported above, according to the results reported by
Kübler and Birbaumer (2008)
. These results encourage further studies with ALS patients at the early and late stage of the disease. Observing the follow-up data of each patient (Tables
2 and
8
), we may hypothesize that the disease progression did not influence the BCI performance and level of success in four patients, while one patient showed decreased performance (from
criterion level down to
above chance); this may be due to the difficult compensation of high errors rate because of eye movement artefacts (see Table
8
). If severely impaired patients who were already trained 1 year before perform any better than similarly impaired patients who were not trained before, remains an open question; probably, more follow-up tests may be helpful to further investigate this question; however, other variables should be taken into account such as the cognitive capabilities and the type of the physical impairment.
Finally, we found the classification accuracy threshold of 80% necessary for the
criterion level. If we look at the number of possible selections in our BCI, the paradigm could be considered a 4-class paradigm. Classifying a single session outcome as “successful” or “unsuccessful” we simplified the problem and changed it into a 2-class problem. Hence, in these conditions (2-class with different probability), one can expect that higher accuracies are required in order to achieve satisfactory BCI-control. Another problem concerns the accuracy threshold of
chance and
above chance levels, which are identical (75%). This may be due to the small number of patients (
N = 2) who did not outperform the
chance level. In particular one of these two patients performed well during training BCI-protocol except for the day T4, during which he successfully completed only two sessions, and then reaching only the
chance level [
pn(
S = 2) = 0.211, Table
9
. His oscillating performance partially influenced the results represented in Table
9
.
Enabling these patients to control a computer cursor may allow them, in the late stage of disease, to communicate by moving the cursor to a specific word or icon on a computer screen. This interface could be adapted to allow patients to perform other actions besides communicating, such as internet navigation, controlling a house-like applications (turn on/off lights, open/close doors, etc.) or to move a robot.
The present study underlined that follow-up and the clinical status do not affect ALS patients communication skill in the early and middle stage of the disease. To meet patient’s needs in the late stage, and to maintain basic yes/no communication, further follow-up sessions of the remaining patients will be reported.
Conflict of Interest Statement
The authors declare that the research was conducted in the absence of any commercial or financial relationships that could be construed as a potential conflict of interest.
We are very grateful to our patients. We thank our colleagues Flavio Giorgi, Gianni Sorarù, Arianna Palmieri, Maria Rosaria Stabile, and Laura Cocco for their contribution in this work.
Bernoulli, J. (1713). Ars Conjectandi. Basilea, Impensis Thurnisiorum Fratrum.
Beverina, F., Giorgi, F., Giove, S., Piccione, F., and Silvoni, S. (2004). P300 off-line detection: a fuzzy-based support system. In Soft Computing Applications, A. Bonarini, ed. (New York, NY, Springer), pp. 155–164.
Birbaumer, N. (2006a). Brain–computer-interface research: coming of age.
Clin. Neurophysiol. 117, 479–483.
Birbaumer, N. (2006b). Breaking the silence: brain–computer interfaces (BCI) for communication and motor control.
Psychophysiology 43, 517–532.
Birbaumer, N., Ghanayim, N., Hinterberger, T., Iversen, I., Kotchoubey, B., Kübler, A., Perelmouter, J., Taub, E., and Flor, H. (1999). A spelling device for the paralysed.
Nature 398, 297–298.
Buch, E., Weber, C., Cohen, L. G., Braun, C., Dimyan, M. A., Ard, T., Mellinger, J., Caria, A., Soekadar, S., Fourkas, A., and Birbaumer, N. (2008). Think to move: a neuromagnetic brain–computer interface (BCI) system for chronic stroke.
Stroke 39, 910–917.
Cedarbaum, J. M., Stambler, N., Malta, E., Fuller, C., Hilt, D., Thurmond, B., and Nakanishi, A. (1999). The ALSFRS-R: a revised ALS functional rating scale that incorporates assessments of respiratory function. BDNF ALS Study Group (Phase III).
J. Neurol. Sci. 169, 13–21.
Cheng, M., Gao, X., Gao, S., and Xu, D. (2002). Design and implementation of a brain–computer interface with high transfer rates.
IEEE Trans. Biomed. Eng. 49, 1181–1186.
Choularton, S., and Dale, R. (2004). User responses to speech recognition errors: consistency of behaviour across domains. In 10th Australian International Conference on Speech Science and Technology (Macquarie University, Sydney, NSW, Australian Speech Science and Technology Association Inc.), pp. 457–462.
Cohen, J., and Polich, J. (1997). On the number of trials needed for P300.
Int. J. Psychophysiol. 25, 249–255.
Coyle, S., Ward, T., Markham, C., and McDarby, G. (2004). On the suitability of near-infrared (NIR) systems for next-generation brain–computer interfaces.
Physiol. Meas. 25, 815–822.
Farwell, L. A., and Donchin, E. (1988). Talking off the top of your head: toward a mental prosthesis utilizing event-related brain potentials.
Electroencephalogr. Clin. Neurophysiol. 70, 510–523.
Folstein, M. F., Folstein, S. E., and McHugh, P. R. (1975). “Mini-mental state”. A practical method for grading the cognitive state of patients for the clinician.
J. Psychiatr. Res. 12, 189–198.
Heinze, H. J., Münte, T. F., Kutas, M., Butler, S. R., Näätänen, R., Nuwer, M. R., and Goodin, D. S. (1999). “Cognitive event-related potentials”, Recommendation for the practice of clinical neurophysiology: guidelines of the International Federation of Clinical Neurophysiology.
Electroencephalogr. Clin. Neurophysiol. 52, 91–95.
Hochberg, L. R., Serruya, M. D., Friehs, G. M., Mukand, J. A., Saleh, M., Caplan, A. H., Branner, A., Chen, D., Penn, R. D., and Donoghue, J. P. (2006). Neuronal ensemble control of prosthetic devices by a human with tetraplegia.
Nature 442, 164–171.
Hoffmann, U., Vesin, J. M., Ebrahimi, T., and Diserens, K. (2008). An efficient P300-based brain–computer interface for disabled subjects.
J. Neurosci. Methods 167, 115–125.
Hyvarinen, A. (1999). Fast and robust fixed-point algorithms for independent component analysis.
IEEE Trans Neural Netw. 10, 626–634.
Iversen, I. H., Ghanayim, N., Kübler, A., Neumann, N., Birbaumer, N., and Kaiser, J. (2008). A brain–computer interface tool to assess cognitive functions in completely paralyzed patients with amyotrophic lateral sclerosis.
Clin. Neurophysiol. 119, 2214–2223.
Joachims, T. (1999). Making large-scale SVM learning practical. In Advances in Kernel Methods – Support Vector Learning, B. Schölkopf, C. Burges and A. Smola, eds (Cambridge, MA, MIT Press), pp. 169–184.
Kotchoubey, B., Lang, S., Winter, S., and Birbaumer, N. (2003). Cognitive processing in completely paralyzed patients with amyotrophic lateral sclerosis.
Eur J Neurol. 10, 551–558.
Kübler, A., and Birbaumer, N. (2008). Brain–computer interfaces and communication in paralysis: Extinction of goal directed thinking in completely paralysed patients?
Clin. Neurophysiol. 119, 2658–2666.
Kübler, A., Neumann, N., Wilhelm, B., Hinterberger, T., and Birbaumer, N. (2004). Predictability of brain–computer communication.
J. Psychophysiol. 18, 121–129.
Kübler, A., Nijboer, F., Mellinger, J., Vaughan, T. M., Pawelzik, H., Schalk, G., McFarland, D. J., Birbaumer, N., and Wolpaw, J. R. (2005). Patients with ALS can use sensorimotor rhythms to operate a brain–computer interface.
Neurology 64, 1775–1777.
Kuebler, A., Kotchoubey, B., Salzmann, H. P., Ghanayim, N., Perelmouter, J., Hömberg, V., and Birbaumer, N. (1998). Self-regulation of slow cortical potentials in completely paralyzed human patients.
Neurosci. Lett. 252, 171–174.
Makeig, S., Jung, T. P., Bell, A. J., Ghahremani, D., and Sejnowski, T. J. (1997). Blind separation of auditory event-related brain responses into independent components.
Proc. Natl. Acad. Sci. U. S. A. 94, 10979–10984.
Millán, del R. J. (2003). Adaptive brain interfaces.
Commun. ACM 46, 74–80.
Miniussi, C., Wilding, E. L., Coull, J. T., and Nobre, A. C. (1999). Orienting attention in time. Modulation of brain potentials.
Brain 122 (Pt 8), 1507–1518.
Müller-Putz, G., and Pfurtscheller, G. (2008). Control of an electrical prosthesis with an SSVEP-based BCI.
IEEE Trans. Biomed. Eng. 55, 361–364.
Müller-Putz, G., Scherer, R., Brunner, C., Leeb, R., and Pfurtscheller, G. (2008). Better than random: a closer look on BCI results.
Int. J. Bioelectromagn. 10, 52–55.
Nelson, H. E. (1976). Modified Card Sorting Test. Firenze, O.S.
Neuper, C., Müller, G. R., Kübler, A., Birbaumer, N., Pfurtscheller, G. (2003). Clinical application of an EEG-based brain–computer interface: a case study in a patient with severe motor impairment.
Clin. Neurophysiol. 114, 399–409.
Nijboer, F., Sellers, E. W., Mellinger, J., Jordan, M. A., Matuz, T., Furdea, A., Halder, S., Mochty, U., Krusienski, D. J., Vaughan, T. M., Wolpaw, J. R., Birbaumer, N., and Kübler, A. (2008). A P300-based brain–computer interface for people with amyotrophic lateral sclerosis.
Clin. Neurophysiol. 119, 1909–1916.
Nobre, A. C. (2001). Orienting attention to instants in time.
Neuropsychologia 39, 1317–1328.
Perelmouter, J., and Birbaumer, N. (2000). A binary spelling interface with random errors.
IEEE Trans. Rehabil. Eng. 8, 227–232.
Pfurtscheller, G., Müller, G. R., Pfurtscheller, J., Gerner, H. J., and Rupp, R. (2003). ‘Thought’-control of functional electrical stimulation to restore hand grasp in a patient with tetraplegia.
Neurosci. Lett. 351, 33–36.
Phukan, J., Pender, N. P., and Hardiman, O. (2007). Cognitive impairment in amyotrophic lateral sclerosis.
Lancet Neurol. 6, 994–1003.
Piccione, F., Giorgi, F., Tonin, P., Priftis, K., Giove, S., Silvoni, S., Palmas, G., and Beverina, F. (2006). P300-based brain–computer interface: reliability and performance in healthy and paralysed participants.
Clin. Neurophysiol. 117, 531–537.
Piccione, F., Volpato, C., Marchetti, M., Priftis, K., Merico, A., Cavinato, M., Sorarù, G., Palmieri, A., Tonin, L., and Silvoni, S. (2008). Amyotrophic lateral sclerosis patients are able to direct a computer screen cursor using a P300-based BCI. In Proceedings of 4th International Brain-Computer Interface Workshop and Training Course, Graz, pp. 327–332.
Polich, J. (2007). Updating P300: an integrative theory of P3a and P3b.
Clin. Neurophysiol. 118, 2128–2148.
Raven, J. C. (1965). RCPM: Guide to Using the Colored Progressive Matrices. New York, NY, Psychological Corp.
Reitan, R. M. (1958). Validity of the trail making test as an indicator of organic brain damage.
Percept Mot Skills 8, 271–276.
Ringholz, G. M., Appel, S. H., Bradshaw, M., Cooke, N. A., Mosnik, D. M., and Schulz, P. E. (2005). Prevalence and patterns of cognitive impairment in sporadic ALS.
Neurology 65, 586–590.
Sellers, E. W., and Donchin, E. (2006). A P300-based brain–computer interface: initial tests by ALS patients.
Clin. Neurophysiol. 117, 538–548.
Spinnler, H., and Tognoni, G. (1987). Standardizzazione taratura italiana dei test neuropsicologici.
Italian J. Neurol. Sci. 8, 1–120.
Thulasidas, M., Guan, C., and Wu, J. (2006). Robust classification of EEG signal for brain–computer interface.
IEEE Trans. Neural. Syst. Rehabil. Eng. 14, 24–29.
Wechsler, D. (1998). Wechsler Adult Intelligence Scale Revised (WAIS-R) (Italian version edited by C. Laicardi). Firenze, A. Orsini.
Wolpaw, J. R., and McFarland, D. J. (2004). Control of a two-dimensional movement signal by a noninvasive brain–computer interface in humans.
Proc. Natl. Acad. Sci. U. S. A. 101, 17849–17854.
Wolpaw, J. R., McFarland, D. J., Neat, G. W., and Forneris, C. A. (1991). An EEG-based brain–computer interface for cursor control.
Electroencephalogr. Clin. Neurophysiol. 78, 252–259.