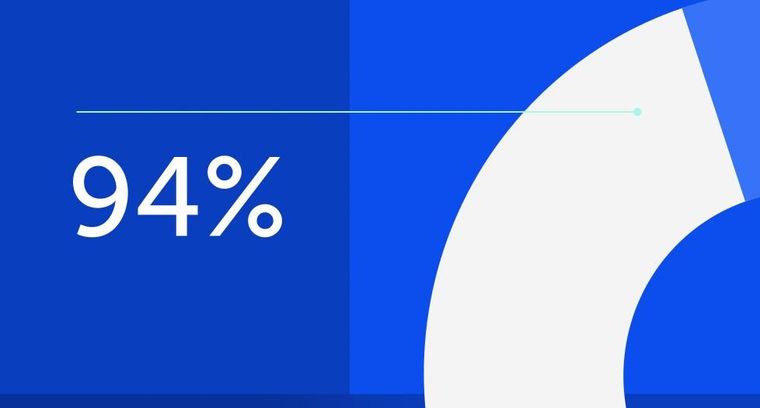
94% of researchers rate our articles as excellent or good
Learn more about the work of our research integrity team to safeguard the quality of each article we publish.
Find out more
ORIGINAL RESEARCH article
Front. Neurosci.
Sec. Translational Neuroscience
Volume 19 - 2025 | doi: 10.3389/fnins.2025.1581373
This article is part of the Research TopicRecent Advances in Translational Neurovascular and Cerebroprotection ResearchView all articles
The final, formatted version of the article will be published soon.
Select one of your emails
You have multiple emails registered with Frontiers:
Notify me on publication
Please enter your email address:
If you already have an account, please login
You don't have a Frontiers account ? You can register here
This study aimed to extract the radiomic features of intracranial aneurysm (IA) and parent artery (PA) walls from high-resolution vessel wall imaging (HR-VWI) images and construct and validate machine learning (ML) predictive models by comparing them with the radiomics score (Rad-score). Methods: In this study, 356 IAs from 306 patients were retrospectively analyzed at Yuzhong Center and randomly divided into training and test cohorts in an 8:2 ratio. Additionally, 66 IAs from 58 patients were used at Jiangnan Center to validate the predictive model. Radiomic features of the IA and PA walls were extracted from the contrast-enhanced HR-VWI images. Univariate and least absolute shrinkage and selection operator (LASSO) regression analyses were performed on the training cohort features to identify optimal rupture-associated features. The Rad-score model was constructed by calculating the total score derived from the weighted sum of optimal radiomic features, and three ML models were built using the XGBoost, LightGBM, and CART algorithms, and evaluated using both the test and external validation cohorts. Results: Eight optimal IA wall features and four PA wall features were identified. The Rad-score model demonstrated an area under the curve (AUC) of 0.858, 0.800, and 0.770 for the training, test, and external validation cohorts, respectively. Among the three ML models, the XGBoost model performed best across all cohorts, with AUC values of 0.983, 0.891, and 0.864, respectively. Compared to the Rad-score model, the XGBoost model exhibited superior AUC values (p < 0.05), better calibration curve Brier scores, and greater net clinical benefit. Conclusion: The radiomic features extracted from HR-VWI images demonstrated robust predictive utility for IA rupture risk in both the Rad-score and ML models. The XGBoost-based ML model outperformed the Rad-score model in efficacy and performance, and proved to be a noninvasive, efficient, and accurate tool for identifying high-risk IA patients.
Keywords: Radiomics, Intracranial Aneurysm, High-resolution vessel wall imaging, machine learning, Rupture
Received: 22 Feb 2025; Accepted: 07 Apr 2025.
Copyright: © 2025 Yuan, Jiang, Wang, Yan, Jiang, Guo and Chen. This is an open-access article distributed under the terms of the Creative Commons Attribution License (CC BY). The use, distribution or reproduction in other forums is permitted, provided the original author(s) or licensor are credited and that the original publication in this journal is cited, in accordance with accepted academic practice. No use, distribution or reproduction is permitted which does not comply with these terms.
* Correspondence:
Dajing Guo, Department of Radiology, the Second Affiliated Hospital of Chongqing Medical University., No.74 Linjiang Rd, Yuzhong District, 400010, Chongqing, China
Ting Chen, Department of Radiology, the Second Affiliated Hospital of Chongqing Medical University., No.74 Linjiang Rd, Yuzhong District, 400010, Chongqing, China
Disclaimer: All claims expressed in this article are solely those of the authors and do not necessarily represent those of their affiliated organizations, or those of the publisher, the editors and the reviewers. Any product that may be evaluated in this article or claim that may be made by its manufacturer is not guaranteed or endorsed by the publisher.
Supplementary Material
Research integrity at Frontiers
Learn more about the work of our research integrity team to safeguard the quality of each article we publish.